Self-injective algebras and the second Hochschild cohomology group
全国代数会议日程(定稿)
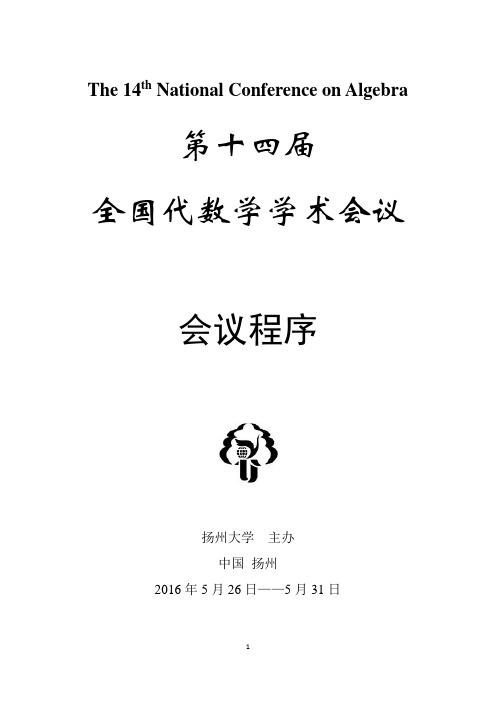
The 14th National Conference on Algebra
第十四届
全国代数学学术会议
会议程序
扬州大学主办
中国扬州
2016年5月26日——5月31日
注意:各报告场地以本手册为准。
一、会议日程
二、会议日程简表
三、会议日程详单
5月26日(星期四)扬州会议中心报到
5月27日(星期五)开幕式与大会报告地点:杏园楼百畅厅
5月28日(星期六)分组报告(第一组) 地点:群贤楼会议室二
5月28日(星期六)分组报告(第二组) 地点:群贤楼会议室三
5月28日(星期六)分组报告(第三组) 地点:群贤楼贵宾室一
5月28日(星期六)分组报告(第四组) 地点:群贤楼贵宾室二
5月28日(星期六)分组报告(第五组) 地点:群贤楼多功能厅
5月28日(星期六)分组报告(第六组) 地点:群贤楼贵宾室四
5月28日(星期六)分组报告(第七组) 地点:群贤楼会议室六
5月28日(星期六)分组报告(第八组) 地点:群贤楼会议室七
5月29日(星期日上午)分组报告(第二组) 地点:群贤楼会议室三
5月29日(星期日上午)分组报告(第四组) 地点:群贤楼贵宾室二
5月29日(星期日上午)分组报告(第六组) 地点:群贤楼贵宾室四
5月29日(星期日下午)邀请报告(第二组) 地点:群贤楼贵宾室二
5月29日(星期日下午)邀请报告(第三组) 地点:群贤楼贵宾室四
5月30日(星期一)大会报告与闭幕式地点:春晖楼春台庆禧厅。
Duality for modules over finite rings and applications to coding theory
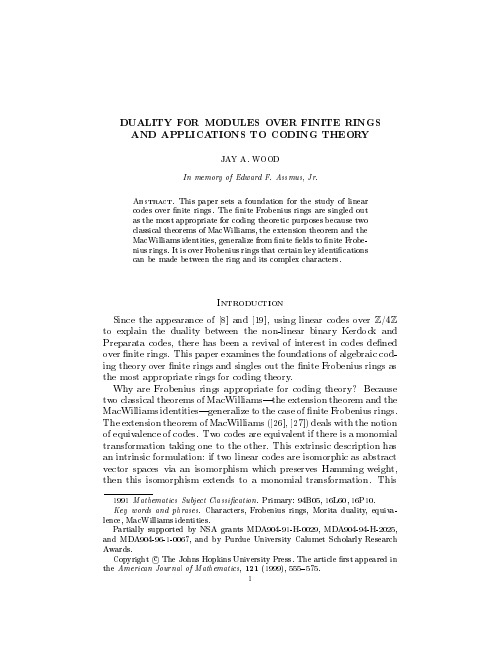
1991 Mathematics Subject Classi cation. Primary: 94B05, 16L60, 16P10. Key words and phrases. Characters, Frobenius rings, Morita duality, equivalence, MacWilliams identities. Partially supported by NSA grants MDA904-91-H-0029, MDA904-94-H-2025, and MDA904-96-1-0067, and by Purdue University Calumet Scholarly Research Awards. Copyright c The Johns Hopkins University Press. The article rst appeared in the American Journal of Mathematics, 121 1999, 555 575. 1
Computer-Vision计算机视觉英文ppt
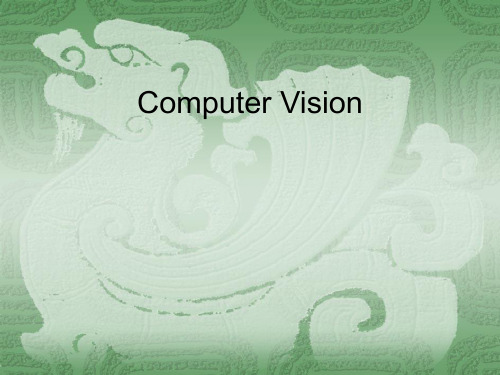
Its mainstream research is divided into three stages:
Stage 1: Research on the visual basic method ,which take the model world as the main object;
Stage 2: Research on visual model ,which is based on the computational theory;
the other is to rebuild the three dimensional object according to the two-dimensional projection images .
History of computer vision
1950s: in this period , statistical pattern recognition is most applied in computer vision , it mainly focuse on the analysis and identification of two-dimensional image,such as: optical character recognition, the surface of the workpiece, the analysis and interpretation of the aerial image.
students approach to learning(学习品质)
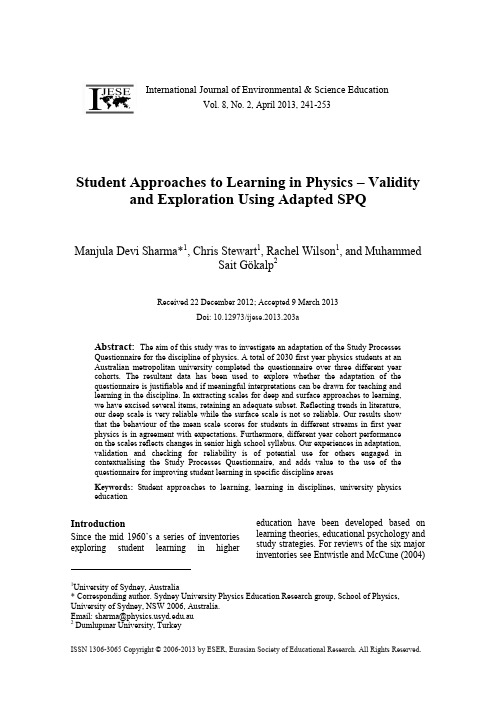
ISSN 1306-3065 Copyright © 2006-2013 by ESER, Eurasian Society of Educational Research. All Rights Reserved.Student Approaches to Learning in Physics – Validityand Exploration Using Adapted SPQManjula Devi Sharma*1, Chris Stewart 1, Rachel Wilson 1, and MuhammedSait Gökalp 2Received 22 December 2012; Accepted 9 March 2013Doi: 10.12973/ijese.2013.203aAbstract: The aim of this study was to investigate an adaptation of the Study ProcessesQuestionnaire for the discipline of physics. A total of 2030 first year physics students at an Australian metropolitan university completed the questionnaire over three different year cohorts. The resultant data has been used to explore whether the adaptation of the questionnaire is justifiable and if meaningful interpretations can be drawn for teaching and learning in the discipline. In extracting scales for deep and surface approaches to learning, we have excised several items, retaining an adequate subset. Reflecting trends in literature, our deep scale is very reliable while the surface scale is not so reliable. Our results show that the behaviour of the mean scale scores for students in different streams in first year physics is in agreement with expectations. Furthermore, different year cohort performance on the scales reflects changes in senior high school syllabus. Our experiences in adaptation, validation and checking for reliability is of potential use for others engaged in contextualising the Study Processes Questionnaire, and adds value to the use of the questionnaire for improving student learning in specific discipline areasKeywords: Student approaches to learning, learning in disciplines, university physics education1University of Sydney, Australia* Corresponding author. Sydney University Physics Education Research group, School of Physics, University of Sydney, NSW 2006, Australia. Email: sharma@.au 2Dumlupınar University, TurkeyIntroductionSince the mid 1960’s a series of inventories exploring student learning in highereducation have been developed based on learning theories, educational psychology and study strategies. For reviews of the six major inventories see Entwistle and McCune (2004)International Journal of Environmental & Science EducationVol. 8, No. 2, April 2013, 241-253242C o p y r i g h t © 2006-2013 b y E S E Rand Biggs (1993a). As can be seen from the reviews, these inventories have two common components. One of these components is related to study strategies and the other one is about cognitive processes. Moreover, these inventories usually have similar conceptual structures and include re-arrangement of the items (Christensen et al., 1991; Wilson et al., 1996).In the current study, as one of these inventories, The Study Processes Questionnaire (SPQ) has been selected to be adapted for use in physics. The SPQ is integrated with the presage-process-product model (3P model) of teaching and learning (Biggs, 1987). Several studies have successfully used the SPQ across different culture s and years to compare students’ approaches in different disciplines (Gow et al., 1994; Kember & Gow, 1990; Skogsberg & Clump, 2003; Quinnell et al., 2005; Zeegers, 2001). Moreover, several other researchers used modified version of the SPQ at their studies (Crawford et al. 1998a,b; Fox, McManus & Winder, 2001; Tooth, Tonge, & McManus, 1989; Volet, Renshaw, & Tietzel, 1994). For example, Volet et al (2001) used a shortened SPQ included 21 items to assess cross cultural differences. Fox et al (2001) modified the SPQ and tested its structure with confirmatory factor analysis. In their study the modified version of the SPQ had 18 items, and this shortened version had same factor structure as the original SPQ. In another study, Crawford et al. (1998a, b) adapted the SPQ for the discipline of mathematics. That adapted questionnaire was named as Approaches to Learning Mathematics Questionnaire.Three different approaches of the students to learning are represented in the SPQ: surface, deep, and achieving approaches. Idea of approaches to learning was presented by Marton and Säljö (1976) and further discussed by several other researchers (eg. Biggs, 1987; Entwistle & Waterston, 1988). Basically, surface approach indicates that the students’ motivation to learn is only for external consequences such as getting the appreciation of the teacher. More specifically, it is enough to fulfill course requirements for the students with surface approach. On theother hand, a deep approach to learning indicates that the motivation is intrinsic. This approach involves higher quality of learning outcomes (Marton & Säljö, 1976; Biggs, 1987). Students with deep approach to learning try to connect what they learn with daily life and they examine the content of the instruction more carefully. On the other hand, achieving approach is about excelling in a course by doing necessary things to have a good mark. However, current study is not focused on this approach. Only the first two approaches were included in the adapted SPQ.Inventories like the SPQ are used in higher education because of several reasons. Such inventories can help educators to evaluate teaching environments (Biggs, 1993b; Biggs, Kember, & Leung, 2001). Moreover, with the use of these inventories, university students often relate their intentions and study strategies for a learning context in a coherent manner. On the other hand, the SPQ is not a discipline specific inventory. It can be used across different disciplines. However, in a research study, if the research questions are related with the common features of learning and teaching within 3P model framework, the SPQ can be used satisfactorily for all disciplines. But, a discipline specific version of the SPQ is required if resolution of details specific to a discipline area is necessary for the research questions. Moreover, in order to reduce systematic error and bias that can be resulted from students in different discipline areas; a discipline specific version may be required. As a community of educators, we are aware that thinking, knowing, and learning processes can differ across discipline areas. A direct consequence of this acknowledgement is the need to understand and model learning in specific discipline areas, such as by adapting the SPQ. However, for the theoretical framework to be valid the conceptual integrity of the inventory must be maintained.This paper reports on how the SPQ has been adapted for physics. The teaching context is first year physics at a research focused Australian university where students are grouped according to differing senior243Student approaches to learning in physicsC o p y r i g h t © 2006-2013 b y E S E Rhigh school experiences into Advanced, Regular, and Fundamentals streams.We report on the selection of items for the deep and surface scales and reliability and validity analyses. A comparison of the Advanced, Regular and Fundamentals streams is carried out to ensure that interpretations associated with the deep and surface scales are meaningful. This is a stage of a large-scale project. The project aims to understand and improve student learning based on the deep and surface approaches to learning inherent in the 3P model (Marton & Säljö, 1976; Biggs, 1987).The studyAs mentioned before, The SPQ has been designed for higher education; however, this questionnaire is not discipline specific. Therefore, in this study, we adapted the SPQ to physics for the following reasons: (1) The first year students have confusions about university studies when they come to university (White et al., 1995). This can lead to misinterpretation of the items. However, specific items related to physics can reduce these misinterpretations. For example students enrolled in a general science degree would view questions related to employment differently to those in professional degrees, and the students we have surveyed are from a range of degree programs. (2) In order to compare the students from the different discipline areas, we need discipline specific inventories. (3) We believe that there are contentious items in the original SPQ and aspects that are specific to physics. For example the use of “truth” in the following item was strongly challenged by a group of physicists validating the questionnaire. While I realize that truth is forever changing as knowledge is increasing, I feel compelled to discover what appears to me to be the truth at this time (Biggs, 1987, p. 132).The item was changed to the following, more in line with the post-positivist paradigm and agreeable to physicists.While I realize that ideas are always changing as knowledge is increasing, I feel a need to discover for myselfwhat is understood about the physical world at this time.One could argue that this is an issue of clarifying the item rather than being specific to physics. However, to our knowledge the clarity of this item has not been debated in literature.Just after we commenced this study in 2001, we became aware that Biggs et al (2001) had produced a revised Study Processes Questionnaire (R-SPQ- 2F). However, it was too late for our study and we did not switch midway. There are four main differences between the SPQ and the R-SPQ-2F; first, the removal of all items on employment after graduation; second, increased emphasis on examination; third, removal of words that imply specificity; and fourth exclusion of the contentious achieving factor identified by Christensen et al., 1991. We focus on the deep and surface approaches and not on the strategy and motive sub-scales as these are not pertinent to our larger study. The SPQ deep and surface scales, in particular, have been shown to be robust (see for example Burnett & Dart, 2000).The participant of the current study was from a university in New South Wales, Australia. Students are provided three basic physics units in the School during their first semester of university: Fundamentals, Regular or Advanced. Students are divided into these three groups of physics units based on their senior high school physics backgrounds. The students from the Fundamentals unit have done no physics in senior high school or have done poorly. On the other hand, in the Regular unit, there were the students had scored high grades in senior high school physics. The last unit, the Advanced unit, is suitable for those who have done extremely well overall in physics during all their years in senior high school.The three physics units that students can register in are for the degree programs in Engineering, Medical Science and Arts. Students who intend to major in physics as well as postgraduate physics students are selected from those enrolled in all three basic physics course in their first semester at university. The largest proportion of students of physics major is from the Advanced244C o p y r i g h t © 2006-2013 b y E S E Rstream, followed by those in the Regular stream, and finally the Fundamentals stream. The data was collected from these streams from 2001 to 2004. From 2001 to 2004, the high school physics syllabi and assessment system was changed in the state of New South Wales in Australia. The details of the changes can be seen in Binnie (2004). Due to these changes, the 2004 cohort of students in this study were instructed using a different curriculum.Within the above context, we have adapted the SPQ to generate a Study Processes Questionnaire for Physics (SPQP). The research questions addressed in this paper are as follows.(a) How do the factor solutions for the SPQP compare with those of the SPQ? (b) Is the SPQP reliable and valid?(c) Are the scales robust enough to reflect detail in senior high school syllabus change? The answers to the research questions will determine if the SPQP is a reliable and valid measure of student approaches to learning physics in our context.MethodRevising the items for the SPQPWe have adapted the SPQ by simply inserting the word “physics” in some items and making substantial changes to others. The adaptations are based on our experiences of student responses to open-ended questions and discipline knowledge, and have been extensively discussed amongst a group of physics educators. The adaptations are of the types listed below. (See appendix A for all items and the types of adaptations.) Type 0: No changeType 1: A simple insertion of terms such as “physics”, “studying physics”.I find that at times studying gives me afeeling of deep personal satisfaction. I find that at times studying physics gives me a feeling of deep personal satisfaction.Type 2: A substantial change in wording that can change the meaning, without intending to.I usually become increasingly absorbed in my work the more I do. When studying physics, I become increasingly absorbed in my work the more I do.Type 3: An intentional change in meaning.My studies have changed my views about such things as politics, my religion, and my philosophy of life. My studies in physics have challenged my views about the way the world works.The number of items corresponding to each Type of change is displayed in Table 1, as are the number of items selected from each Type for inclusion in the SPQP. Type 1 items were more useful in generating the items used in the SPQP.Administering the SPQPThe SPQP was administered at the beginning of the first semester to students in the Advanced, Regular and Fundamentals streams in 2001, 2002 and 2004, respectively. On the questionnaire, the students were requested to indicate their level of agreement with each item on a Likert scale with the options of Strongly Disagree, Disagree, Neutral, Agree, and Strongly Agree . Response rates of 2001, 2002, and 2004 cohorts was 95%, 65%, and 85%, respectively. Except 2002 cohort, the response rates were satisfactory. The main reasons of the lower response rate of 2002 cohort were the changes in class organization and questionnaire administration. Over these245Student approaches to learning in physicsC o p y r i g h t © 2006-2013 b y E S E Rthree years, a total of 2030 first year physics student were responded the SPQP: 63 percent of students in the Fundamentals stream was female, and about 30 percent of them was females in the Regular and Advanced streams. Nevertheless, the three streams are similar in other respects. The sample size of 2030 is large enough to access the natural variance within the diverse population. However, due to missing answers some of the cases were excluded from the analysis. These exclusions were only about 3% of the whole sample. Therefore, we can say that this missing data did not affect the overall results. Data Analysis MethodsFollowing analyses were carried out to answer research questions.(a) Both exploratory and confirmatory factor analyses were performed to validate the two-factor solution: the deep and surface scales. (b) Cronbach’s alpha coefficients were calculated to determine the reliability for the deep and surface scales for the complete data set and for each stream.(c) ANOVA and boxplots were used to determine if the SPQP is able to differentiate between the three streams and changes in syllabus.ResultsFactor analysisIn order to gain construct related evidence for the validity of the SPQP, exploratory and confirmatory factor analysis were conducted. Exploratory factor analysis (EFA) was carried out by using principal components as factor extraction method with quartimax, an orthogonal rotation. The complete data set was included in this analysis. Before proceeding to interpret the results, each item was checked for normality and sphericity. In order to check multicollinearity, the correlation matrix was examined. In terms of multicollinearity, we expect the items to be intercorrelated; however, these correlations should not be so high (0.90 or higher), whichcauses to multicollinearity and singularity. The intercorrelation was checked by Bartlett’s test of sphericity. This test showed that the correlation matrix is not an identity matrix. Moreover, multicollinearity was checked with the determinant of the correlation matrix. The determinant was more than 0. This showed that there is no multicollinearity (Field, 2000). Extraction of factors was based on two criteria: Scree test and Kaiser criterion (eigen values). Based on eigen values and the Scree test, two factors, which accounts for 48% of the variance, were extracted. The items with factor loadings of less than .4 were excluded from the further analyses (Field, 2000). Appendix A shows the two-factor solution for all items including loadings. Those that were retained for the SPQP are starred – 10 items form the deep scale and 6 items the surface scale.According to the results of the EFA, we note that the deep scale is in better agreement with Biggs ’s deep scale than the surface scale - there are more “usable” items on the deep scale than on the surface scale.After having results of the EFA, confirmatory factor analysis (CFA) was performed. This second step of the factor analysis helped us to ensure the factor structure of the SPQP (see Figure 1). Maximum likelihood (ML) was used as the method of estimation at the CFA. The results of the study showed that relative chi-square, which is the “chi square/degree of freedom” ratio is 3.1. Moreover, RMSEA and CFI were found to be 0.07 and 0.69 respectively. According to Browne and Cudeck (1992), RMSEA values less than 0.05 indicate close fit and models with values greater than 0.10 should not be employed. Here, RMSEA indicates moderate fit of the model whereas relative chi-square indicates good fit. However, the CFI should be over 0.90 to have good fit. Nonetheless, we can say that the first two indices support this two-factor model of the SPQP and indicate moderate fit.246C o p y r i g h t © 2006-2013 b y E S E RFigure 1. Validated two-factor structure of the SPQP.Reliability of the SPQPCronbach alpha coefficients of each scale were calculated for each stream and whole data. The results are shown in Table 2. It is apparent that the surface scale has the lowest Cronbach alpha coefficients at each stream. Similar findings were also reported at other studies (Biggs, 1987; Biggs et al, 2001; Wilson and Fowler, 2005). The foundational efficacy of these scales, given such low reliability, is questionable. However, in ourstudy higher levels of internal consistency were apparent (lowest α =.61).Comparing reliabilities across streams, students who have less experience with physics report surface approaches more reliably than students with more experience. On the other hand, students who have more experience with physics report deep approaches more reliably than those who have less experience. Considering reliabilities within streams, the Fundamentals students report deep approaches as reliably as surface247Student approaches to learning in physicsC o p y r i g h t © 2006-2013 b y E S E Rapproaches with values greater than 0.80, while the Advanced students report very different reliabilities for the two scales. The trends are not surprising since Advanced students would tend to be more confident in content and study strategies.The above trends also raise the question: Are the persistently low reliabilities noted for the su rface scale due to student ‘behaviours’ or poor items on the inventory? An adequate reliability measure for the surface scale for the Fundamentals stream α > .80, one that is similar in magnitude to that of the deep scale, implies that there is internal consistency amongst the items for each scale for this group of students. We note that the Fundamentals students have experienced junior high school physics, and are doing concurrent science and mathematics subjects at university. University students tend to have high internal coherence among learning components, intentions and study strategies and are able to adapt their ideas of knowledge and study methods to their expectations of studying in a particular context. The internal coherence is demonstrated in the reliability scores. So why are the reliabilities for the surface scale as low as 0.61 for the Advanced stream? Is it because the nature of the surface approach is different for the Advanced and Fundamentals streams, requiring possibly different items? Or is it because the Advanced students adapt their surface approaches in diverse ways, hence report this scale less reliably? Answers to such questions will indeed add to our understanding of student learning.ANOVA and BoxplotsTo determine if the SPQP is able to differentiate between the three streams, item and scale means were compared using one-way ANOVA.When comparing the means of the three streams for each item on the SPQP, the assumption of homogeneity of variances underpinning ANOVA was tested using Mulachy’s test of Sphericity. Items A5, A13 and A25 were excluded from ANOVA because they violated the assumption of sphericity. This does not affect their use on the SPQP scales. The results of ANOVA showed that there is a significant differenceamong the SPQP scores of the students from Fundamentals, Regular, and Advanced streams for both surface and deep scales (p < .05).There is a debate among the researchers to use ANOVA with the ordinal data mainly because of the normality concern. As stated in Glass, Peckham, and Sanders (1972), violation of normality is not fatal to the ANOVA. Moreover, performing ANOVA with the ordinal data, likert type items in this case, is a controversial issue among the researchers. Here, we have a big sample and this surely increases the power of the test. Therefore, failure to meet normality assumption will not affect the results of the ANOVA. Moreover, we performed Kruskal Wallis test as non-parametric alternative of the ANOVA. The results of that test supported the results of the ANOVA given above.Moreover, in order to investigate if SPQP is robust enough to be able to differentiate changes in syllabus even when sum of the items is used instead of factor scores, boxplots were checked (see Figure 2). The boxplots show a sequence for each scale with the first panel representing the factor scores, the second panel the simple sums of the items scores for the SPQP and the third panel the simple sums of all 16 item scores that should have loaded on each scale. We note two important features. First, the three panels representing the deep scale are sufficiently similar implying that if an adaptation such as that in this study is made, the sums of the 10 SPQP item scores, and indeed all 16 items scores provide a reasonable measure of deep approaches to learning. However, this is not so for the surface scale, while the sum of the 6 SPQP item scores provides a reasonable measure of surface approaches to learning, the sum of all 16 items does not do so. This raises concerns regarding the surface scale and is a reflection of the low reliabilities for the scale.DiscussionAs we are particularly interested in issues to do with learning physics, the rationale and manner in which items were modified and the SPQ adapted are discussed in detail. The advantages of adapting an established, well248C o p y r i g h t © 2006-2013 b y E S E RFigure 2. A comparison by stream and year of the deep and surface scales. The boxplots show a vertical sequence for each scale with panel (a) representing the factor scores for the SPQP deep scale and panel (d) those for the SPQP surface scale. Panel (b) represents the simple sum of item scores for the SPQP deep scale and panel (e) those for the SPQP surface scale. Panel (c) represents the simple sum of all 14 item scores that were intended to load on the deep scale and panel (f) those for the surface scale.implemented inventory with a sound theoretical framework, both for itsdevelopment and for its practical use in a teaching environment, are evident in the249Student approaches to learning in physicsC o p y r i g h t © 2006-2013 b y E S E Rmeaningful interpretations of our results summarized below.1. The SPQ items were modified for our context based on our experiences and any changes were extensively discussed amongst a group of physics educators. Ten items were retained for the deep scale and six for the surface. The rejection of items that had anomalous factor loadings could be conceptually justified. This two-factor solution of the SPQP confirmed with the EFA and CFA and supported the factor structure of the original SPQ (Biggs, 1987).2. The trends in reliabilities according to streams are as expected, with students with less experience in physics reporting less reliably on the deep scale and more reliably on the surface scale and vice versa. The issue of low reliabilities of the surface scale for the Advanced stream raises the question of whether Advanced students have more diverse forms of exhibiting surface approaches to learning. Moreover, the issue with the surface scale coincides with the previous studies (Biggs, 1987; Biggs et al, 2001; Wilson and Fowler, 2005).3. Comparisons of deep factor scores, simple sums of the 10 SPQP items and all 16 items suggest that the deep scale is reliable and particularly robust, see Figure 2. The surface factor scores compare well with the simple sums of the 6 SPQP items, but not with all 16 items, suggesting that reliability and validity checks are particularly important for the surface scale. The implication is twofold: first the SPQ is robust when contextualised as shown by reliability scores; and second, the contextualisation did not alter the overall coherency of the inventory as shown by the meaningful interpretations across streams and years. This, together with the conceptual meanings associated with the items, provides confidence that the SPQP is consistent with the theoretical framework of the SPQ.4. Changes in senior high school physics syllabus have impacted on approaches to study in the cohorts sampled in this study. The SPQP can illustrate differences between streams and years. From our study we are confident that the SPQP is a reliable andvalid measure of approaches to learning physics in our context.5. The adaptation of the SPQ into physics adds value to our project findings as it allows us to illustrate physics’ specific detail between the streams. We are confident that features that could have systematically biased the findings have been minimized. Lastly the ways of thinking, learning and knowing in physics are embedded in the larger context of intentions and study methods in higher education.ConclusionWe have adapted the Study Processes Questionnaire into physics and confirmed that a two-factor solution provides two subsets of selected items representing deep and surface approaches to learning. The resulting inventory is called the Study Processes Questionnaire for Physics, or SPQP. Further reliability and validation checks demonstrate that the two-scale SPQP is a useable inventory for our context. Reliabilities for the Advanced, Regular and Fundamentals streams are adequate and the behaviour of the mean scale scores for the three streams is not contradictory to expected student behaviours.The process of adapting the SPQ has provided useful insights into the way physicists interpret the items, and how deep and surface approaches can be conceptualised in physics. The sound theoretical framework and research underpinning the SPQ has added value to the use of questionnaires for understanding student learning in our project. Such contextualised inventories have the potential to provide context-specific understandings of teaching and learning issues and for improving student learning.AcknowledgementsThe authors acknowledge Science Faculty Education Research (SciFER) grants, University of Sydney, and support from staff and students. The authors are grateful to Professor David Boud for his constructive feedback on this paper.。
《神经网络与深度学习综述DeepLearning15May2014
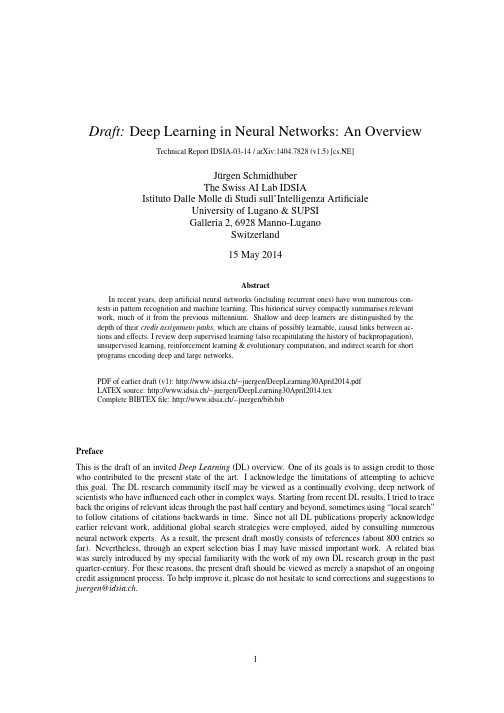
Draft:Deep Learning in Neural Networks:An OverviewTechnical Report IDSIA-03-14/arXiv:1404.7828(v1.5)[cs.NE]J¨u rgen SchmidhuberThe Swiss AI Lab IDSIAIstituto Dalle Molle di Studi sull’Intelligenza ArtificialeUniversity of Lugano&SUPSIGalleria2,6928Manno-LuganoSwitzerland15May2014AbstractIn recent years,deep artificial neural networks(including recurrent ones)have won numerous con-tests in pattern recognition and machine learning.This historical survey compactly summarises relevantwork,much of it from the previous millennium.Shallow and deep learners are distinguished by thedepth of their credit assignment paths,which are chains of possibly learnable,causal links between ac-tions and effects.I review deep supervised learning(also recapitulating the history of backpropagation),unsupervised learning,reinforcement learning&evolutionary computation,and indirect search for shortprograms encoding deep and large networks.PDF of earlier draft(v1):http://www.idsia.ch/∼juergen/DeepLearning30April2014.pdfLATEX source:http://www.idsia.ch/∼juergen/DeepLearning30April2014.texComplete BIBTEXfile:http://www.idsia.ch/∼juergen/bib.bibPrefaceThis is the draft of an invited Deep Learning(DL)overview.One of its goals is to assign credit to those who contributed to the present state of the art.I acknowledge the limitations of attempting to achieve this goal.The DL research community itself may be viewed as a continually evolving,deep network of scientists who have influenced each other in complex ways.Starting from recent DL results,I tried to trace back the origins of relevant ideas through the past half century and beyond,sometimes using“local search”to follow citations of citations backwards in time.Since not all DL publications properly acknowledge earlier relevant work,additional global search strategies were employed,aided by consulting numerous neural network experts.As a result,the present draft mostly consists of references(about800entries so far).Nevertheless,through an expert selection bias I may have missed important work.A related bias was surely introduced by my special familiarity with the work of my own DL research group in the past quarter-century.For these reasons,the present draft should be viewed as merely a snapshot of an ongoing credit assignment process.To help improve it,please do not hesitate to send corrections and suggestions to juergen@idsia.ch.Contents1Introduction to Deep Learning(DL)in Neural Networks(NNs)3 2Event-Oriented Notation for Activation Spreading in FNNs/RNNs3 3Depth of Credit Assignment Paths(CAPs)and of Problems4 4Recurring Themes of Deep Learning54.1Dynamic Programming(DP)for DL (5)4.2Unsupervised Learning(UL)Facilitating Supervised Learning(SL)and RL (6)4.3Occam’s Razor:Compression and Minimum Description Length(MDL) (6)4.4Learning Hierarchical Representations Through Deep SL,UL,RL (6)4.5Fast Graphics Processing Units(GPUs)for DL in NNs (6)5Supervised NNs,Some Helped by Unsupervised NNs75.11940s and Earlier (7)5.2Around1960:More Neurobiological Inspiration for DL (7)5.31965:Deep Networks Based on the Group Method of Data Handling(GMDH) (8)5.41979:Convolution+Weight Replication+Winner-Take-All(WTA) (8)5.51960-1981and Beyond:Development of Backpropagation(BP)for NNs (8)5.5.1BP for Weight-Sharing Feedforward NNs(FNNs)and Recurrent NNs(RNNs)..95.6Late1980s-2000:Numerous Improvements of NNs (9)5.6.1Ideas for Dealing with Long Time Lags and Deep CAPs (10)5.6.2Better BP Through Advanced Gradient Descent (10)5.6.3Discovering Low-Complexity,Problem-Solving NNs (11)5.6.4Potential Benefits of UL for SL (11)5.71987:UL Through Autoencoder(AE)Hierarchies (12)5.81989:BP for Convolutional NNs(CNNs) (13)5.91991:Fundamental Deep Learning Problem of Gradient Descent (13)5.101991:UL-Based History Compression Through a Deep Hierarchy of RNNs (14)5.111992:Max-Pooling(MP):Towards MPCNNs (14)5.121994:Contest-Winning Not So Deep NNs (15)5.131995:Supervised Recurrent Very Deep Learner(LSTM RNN) (15)5.142003:More Contest-Winning/Record-Setting,Often Not So Deep NNs (16)5.152006/7:Deep Belief Networks(DBNs)&AE Stacks Fine-Tuned by BP (17)5.162006/7:Improved CNNs/GPU-CNNs/BP-Trained MPCNNs (17)5.172009:First Official Competitions Won by RNNs,and with MPCNNs (18)5.182010:Plain Backprop(+Distortions)on GPU Yields Excellent Results (18)5.192011:MPCNNs on GPU Achieve Superhuman Vision Performance (18)5.202011:Hessian-Free Optimization for RNNs (19)5.212012:First Contests Won on ImageNet&Object Detection&Segmentation (19)5.222013-:More Contests and Benchmark Records (20)5.22.1Currently Successful Supervised Techniques:LSTM RNNs/GPU-MPCNNs (21)5.23Recent Tricks for Improving SL Deep NNs(Compare Sec.5.6.2,5.6.3) (21)5.24Consequences for Neuroscience (22)5.25DL with Spiking Neurons? (22)6DL in FNNs and RNNs for Reinforcement Learning(RL)236.1RL Through NN World Models Yields RNNs With Deep CAPs (23)6.2Deep FNNs for Traditional RL and Markov Decision Processes(MDPs) (24)6.3Deep RL RNNs for Partially Observable MDPs(POMDPs) (24)6.4RL Facilitated by Deep UL in FNNs and RNNs (25)6.5Deep Hierarchical RL(HRL)and Subgoal Learning with FNNs and RNNs (25)6.6Deep RL by Direct NN Search/Policy Gradients/Evolution (25)6.7Deep RL by Indirect Policy Search/Compressed NN Search (26)6.8Universal RL (27)7Conclusion271Introduction to Deep Learning(DL)in Neural Networks(NNs) Which modifiable components of a learning system are responsible for its success or failure?What changes to them improve performance?This has been called the fundamental credit assignment problem(Minsky, 1963).There are general credit assignment methods for universal problem solvers that are time-optimal in various theoretical senses(Sec.6.8).The present survey,however,will focus on the narrower,but now commercially important,subfield of Deep Learning(DL)in Artificial Neural Networks(NNs).We are interested in accurate credit assignment across possibly many,often nonlinear,computational stages of NNs.Shallow NN-like models have been around for many decades if not centuries(Sec.5.1).Models with several successive nonlinear layers of neurons date back at least to the1960s(Sec.5.3)and1970s(Sec.5.5). An efficient gradient descent method for teacher-based Supervised Learning(SL)in discrete,differentiable networks of arbitrary depth called backpropagation(BP)was developed in the1960s and1970s,and ap-plied to NNs in1981(Sec.5.5).BP-based training of deep NNs with many layers,however,had been found to be difficult in practice by the late1980s(Sec.5.6),and had become an explicit research subject by the early1990s(Sec.5.9).DL became practically feasible to some extent through the help of Unsupervised Learning(UL)(e.g.,Sec.5.10,5.15).The1990s and2000s also saw many improvements of purely super-vised DL(Sec.5).In the new millennium,deep NNs havefinally attracted wide-spread attention,mainly by outperforming alternative machine learning methods such as kernel machines(Vapnik,1995;Sch¨o lkopf et al.,1998)in numerous important applications.In fact,supervised deep NNs have won numerous of-ficial international pattern recognition competitions(e.g.,Sec.5.17,5.19,5.21,5.22),achieving thefirst superhuman visual pattern recognition results in limited domains(Sec.5.19).Deep NNs also have become relevant for the more generalfield of Reinforcement Learning(RL)where there is no supervising teacher (Sec.6).Both feedforward(acyclic)NNs(FNNs)and recurrent(cyclic)NNs(RNNs)have won contests(Sec.5.12,5.14,5.17,5.19,5.21,5.22).In a sense,RNNs are the deepest of all NNs(Sec.3)—they are general computers more powerful than FNNs,and can in principle create and process memories of ar-bitrary sequences of input patterns(e.g.,Siegelmann and Sontag,1991;Schmidhuber,1990a).Unlike traditional methods for automatic sequential program synthesis(e.g.,Waldinger and Lee,1969;Balzer, 1985;Soloway,1986;Deville and Lau,1994),RNNs can learn programs that mix sequential and parallel information processing in a natural and efficient way,exploiting the massive parallelism viewed as crucial for sustaining the rapid decline of computation cost observed over the past75years.The rest of this paper is structured as follows.Sec.2introduces a compact,event-oriented notation that is simple yet general enough to accommodate both FNNs and RNNs.Sec.3introduces the concept of Credit Assignment Paths(CAPs)to measure whether learning in a given NN application is of the deep or shallow type.Sec.4lists recurring themes of DL in SL,UL,and RL.Sec.5focuses on SL and UL,and on how UL can facilitate SL,although pure SL has become dominant in recent competitions(Sec.5.17-5.22). Sec.5is arranged in a historical timeline format with subsections on important inspirations and technical contributions.Sec.6on deep RL discusses traditional Dynamic Programming(DP)-based RL combined with gradient-based search techniques for SL or UL in deep NNs,as well as general methods for direct and indirect search in the weight space of deep FNNs and RNNs,including successful policy gradient and evolutionary methods.2Event-Oriented Notation for Activation Spreading in FNNs/RNNs Throughout this paper,let i,j,k,t,p,q,r denote positive integer variables assuming ranges implicit in the given contexts.Let n,m,T denote positive integer constants.An NN’s topology may change over time(e.g.,Fahlman,1991;Ring,1991;Weng et al.,1992;Fritzke, 1994).At any given moment,it can be described as afinite subset of units(or nodes or neurons)N= {u1,u2,...,}and afinite set H⊆N×N of directed edges or connections between nodes.FNNs are acyclic graphs,RNNs cyclic.Thefirst(input)layer is the set of input units,a subset of N.In FNNs,the k-th layer(k>1)is the set of all nodes u∈N such that there is an edge path of length k−1(but no longer path)between some input unit and u.There may be shortcut connections between distant layers.The NN’s behavior or program is determined by a set of real-valued,possibly modifiable,parameters or weights w i(i=1,...,n).We now focus on a singlefinite episode or epoch of information processing and activation spreading,without learning through weight changes.The following slightly unconventional notation is designed to compactly describe what is happening during the runtime of the system.During an episode,there is a partially causal sequence x t(t=1,...,T)of real values that I call events.Each x t is either an input set by the environment,or the activation of a unit that may directly depend on other x k(k<t)through a current NN topology-dependent set in t of indices k representing incoming causal connections or links.Let the function v encode topology information and map such event index pairs(k,t)to weight indices.For example,in the non-input case we may have x t=f t(net t)with real-valued net t= k∈in t x k w v(k,t)(additive case)or net t= k∈in t x k w v(k,t)(multiplicative case), where f t is a typically nonlinear real-valued activation function such as tanh.In many recent competition-winning NNs(Sec.5.19,5.21,5.22)there also are events of the type x t=max k∈int (x k);some networktypes may also use complex polynomial activation functions(Sec.5.3).x t may directly affect certain x k(k>t)through outgoing connections or links represented through a current set out t of indices k with t∈in k.Some non-input events are called output events.Note that many of the x t may refer to different,time-varying activations of the same unit in sequence-processing RNNs(e.g.,Williams,1989,“unfolding in time”),or also in FNNs sequentially exposed to time-varying input patterns of a large training set encoded as input events.During an episode,the same weight may get reused over and over again in topology-dependent ways,e.g.,in RNNs,or in convolutional NNs(Sec.5.4,5.8).I call this weight sharing across space and/or time.Weight sharing may greatly reduce the NN’s descriptive complexity,which is the number of bits of information required to describe the NN (Sec.4.3).In Supervised Learning(SL),certain NN output events x t may be associated with teacher-given,real-valued labels or targets d t yielding errors e t,e.g.,e t=1/2(x t−d t)2.A typical goal of supervised NN training is tofind weights that yield episodes with small total error E,the sum of all such e t.The hope is that the NN will generalize well in later episodes,causing only small errors on previously unseen sequences of input events.Many alternative error functions for SL and UL are possible.SL assumes that input events are independent of earlier output events(which may affect the environ-ment through actions causing subsequent perceptions).This assumption does not hold in the broaderfields of Sequential Decision Making and Reinforcement Learning(RL)(Kaelbling et al.,1996;Sutton and Barto, 1998;Hutter,2005)(Sec.6).In RL,some of the input events may encode real-valued reward signals given by the environment,and a typical goal is tofind weights that yield episodes with a high sum of reward signals,through sequences of appropriate output actions.Sec.5.5will use the notation above to compactly describe a central algorithm of DL,namely,back-propagation(BP)for supervised weight-sharing FNNs and RNNs.(FNNs may be viewed as RNNs with certainfixed zero weights.)Sec.6will address the more general RL case.3Depth of Credit Assignment Paths(CAPs)and of ProblemsTo measure whether credit assignment in a given NN application is of the deep or shallow type,I introduce the concept of Credit Assignment Paths or CAPs,which are chains of possibly causal links between events.Let usfirst focus on SL.Consider two events x p and x q(1≤p<q≤T).Depending on the appli-cation,they may have a Potential Direct Causal Connection(PDCC)expressed by the Boolean predicate pdcc(p,q),which is true if and only if p∈in q.Then the2-element list(p,q)is defined to be a CAP from p to q(a minimal one).A learning algorithm may be allowed to change w v(p,q)to improve performance in future episodes.More general,possibly indirect,Potential Causal Connections(PCC)are expressed by the recursively defined Boolean predicate pcc(p,q),which in the SL case is true only if pdcc(p,q),or if pcc(p,k)for some k and pdcc(k,q).In the latter case,appending q to any CAP from p to k yields a CAP from p to q(this is a recursive definition,too).The set of such CAPs may be large but isfinite.Note that the same weight may affect many different PDCCs between successive events listed by a given CAP,e.g.,in the case of RNNs, or weight-sharing FNNs.Suppose a CAP has the form(...,k,t,...,q),where k and t(possibly t=q)are thefirst successive elements with modifiable w v(k,t).Then the length of the suffix list(t,...,q)is called the CAP’s depth (which is0if there are no modifiable links at all).This depth limits how far backwards credit assignment can move down the causal chain tofind a modifiable weight.1Suppose an episode and its event sequence x1,...,x T satisfy a computable criterion used to decide whether a given problem has been solved(e.g.,total error E below some threshold).Then the set of used weights is called a solution to the problem,and the depth of the deepest CAP within the sequence is called the solution’s depth.There may be other solutions(yielding different event sequences)with different depths.Given somefixed NN topology,the smallest depth of any solution is called the problem’s depth.Sometimes we also speak of the depth of an architecture:SL FNNs withfixed topology imply a problem-independent maximal problem depth bounded by the number of non-input layers.Certain SL RNNs withfixed weights for all connections except those to output units(Jaeger,2001;Maass et al.,2002; Jaeger,2004;Schrauwen et al.,2007)have a maximal problem depth of1,because only thefinal links in the corresponding CAPs are modifiable.In general,however,RNNs may learn to solve problems of potentially unlimited depth.Note that the definitions above are solely based on the depths of causal chains,and agnostic of the temporal distance between events.For example,shallow FNNs perceiving large“time windows”of in-put events may correctly classify long input sequences through appropriate output events,and thus solve shallow problems involving long time lags between relevant events.At which problem depth does Shallow Learning end,and Deep Learning begin?Discussions with DL experts have not yet yielded a conclusive response to this question.Instead of committing myself to a precise answer,let me just define for the purposes of this overview:problems of depth>10require Very Deep Learning.The difficulty of a problem may have little to do with its depth.Some NNs can quickly learn to solve certain deep problems,e.g.,through random weight guessing(Sec.5.9)or other types of direct search (Sec.6.6)or indirect search(Sec.6.7)in weight space,or through training an NNfirst on shallow problems whose solutions may then generalize to deep problems,or through collapsing sequences of(non)linear operations into a single(non)linear operation—but see an analysis of non-trivial aspects of deep linear networks(Baldi and Hornik,1994,Section B).In general,however,finding an NN that precisely models a given training set is an NP-complete problem(Judd,1990;Blum and Rivest,1992),also in the case of deep NNs(S´ıma,1994;de Souto et al.,1999;Windisch,2005);compare a survey of negative results(S´ıma, 2002,Section1).Above we have focused on SL.In the more general case of RL in unknown environments,pcc(p,q) is also true if x p is an output event and x q any later input event—any action may affect the environment and thus any later perception.(In the real world,the environment may even influence non-input events computed on a physical hardware entangled with the entire universe,but this is ignored here.)It is possible to model and replace such unmodifiable environmental PCCs through a part of the NN that has already learned to predict(through some of its units)input events(including reward signals)from former input events and actions(Sec.6.1).Its weights are frozen,but can help to assign credit to other,still modifiable weights used to compute actions(Sec.6.1).This approach may lead to very deep CAPs though.Some DL research is about automatically rephrasing problems such that their depth is reduced(Sec.4). In particular,sometimes UL is used to make SL problems less deep,e.g.,Sec.5.10.Often Dynamic Programming(Sec.4.1)is used to facilitate certain traditional RL problems,e.g.,Sec.6.2.Sec.5focuses on CAPs for SL,Sec.6on the more complex case of RL.4Recurring Themes of Deep Learning4.1Dynamic Programming(DP)for DLOne recurring theme of DL is Dynamic Programming(DP)(Bellman,1957),which can help to facili-tate credit assignment under certain assumptions.For example,in SL NNs,backpropagation itself can 1An alternative would be to count only modifiable links when measuring depth.In many typical NN applications this would not make a difference,but in some it would,e.g.,Sec.6.1.be viewed as a DP-derived method(Sec.5.5).In traditional RL based on strong Markovian assumptions, DP-derived methods can help to greatly reduce problem depth(Sec.6.2).DP algorithms are also essen-tial for systems that combine concepts of NNs and graphical models,such as Hidden Markov Models (HMMs)(Stratonovich,1960;Baum and Petrie,1966)and Expectation Maximization(EM)(Dempster et al.,1977),e.g.,(Bottou,1991;Bengio,1991;Bourlard and Morgan,1994;Baldi and Chauvin,1996; Jordan and Sejnowski,2001;Bishop,2006;Poon and Domingos,2011;Dahl et al.,2012;Hinton et al., 2012a).4.2Unsupervised Learning(UL)Facilitating Supervised Learning(SL)and RL Another recurring theme is how UL can facilitate both SL(Sec.5)and RL(Sec.6).UL(Sec.5.6.4) is normally used to encode raw incoming data such as video or speech streams in a form that is more convenient for subsequent goal-directed learning.In particular,codes that describe the original data in a less redundant or more compact way can be fed into SL(Sec.5.10,5.15)or RL machines(Sec.6.4),whose search spaces may thus become smaller(and whose CAPs shallower)than those necessary for dealing with the raw data.UL is closely connected to the topics of regularization and compression(Sec.4.3,5.6.3). 4.3Occam’s Razor:Compression and Minimum Description Length(MDL) Occam’s razor favors simple solutions over complex ones.Given some programming language,the prin-ciple of Minimum Description Length(MDL)can be used to measure the complexity of a solution candi-date by the length of the shortest program that computes it(e.g.,Solomonoff,1964;Kolmogorov,1965b; Chaitin,1966;Wallace and Boulton,1968;Levin,1973a;Rissanen,1986;Blumer et al.,1987;Li and Vit´a nyi,1997;Gr¨u nwald et al.,2005).Some methods explicitly take into account program runtime(Al-lender,1992;Watanabe,1992;Schmidhuber,2002,1995);many consider only programs with constant runtime,written in non-universal programming languages(e.g.,Rissanen,1986;Hinton and van Camp, 1993).In the NN case,the MDL principle suggests that low NN weight complexity corresponds to high NN probability in the Bayesian view(e.g.,MacKay,1992;Buntine and Weigend,1991;De Freitas,2003), and to high generalization performance(e.g.,Baum and Haussler,1989),without overfitting the training data.Many methods have been proposed for regularizing NNs,that is,searching for solution-computing, low-complexity SL NNs(Sec.5.6.3)and RL NNs(Sec.6.7).This is closely related to certain UL methods (Sec.4.2,5.6.4).4.4Learning Hierarchical Representations Through Deep SL,UL,RLMany methods of Good Old-Fashioned Artificial Intelligence(GOFAI)(Nilsson,1980)as well as more recent approaches to AI(Russell et al.,1995)and Machine Learning(Mitchell,1997)learn hierarchies of more and more abstract data representations.For example,certain methods of syntactic pattern recog-nition(Fu,1977)such as grammar induction discover hierarchies of formal rules to model observations. The partially(un)supervised Automated Mathematician/EURISKO(Lenat,1983;Lenat and Brown,1984) continually learns concepts by combining previously learnt concepts.Such hierarchical representation learning(Ring,1994;Bengio et al.,2013;Deng and Yu,2014)is also a recurring theme of DL NNs for SL (Sec.5),UL-aided SL(Sec.5.7,5.10,5.15),and hierarchical RL(Sec.6.5).Often,abstract hierarchical representations are natural by-products of data compression(Sec.4.3),e.g.,Sec.5.10.4.5Fast Graphics Processing Units(GPUs)for DL in NNsWhile the previous millennium saw several attempts at creating fast NN-specific hardware(e.g.,Jackel et al.,1990;Faggin,1992;Ramacher et al.,1993;Widrow et al.,1994;Heemskerk,1995;Korkin et al., 1997;Urlbe,1999),and at exploiting standard hardware(e.g.,Anguita et al.,1994;Muller et al.,1995; Anguita and Gomes,1996),the new millennium brought a DL breakthrough in form of cheap,multi-processor graphics cards or GPUs.GPUs are widely used for video games,a huge and competitive market that has driven down hardware prices.GPUs excel at fast matrix and vector multiplications required not only for convincing virtual realities but also for NN training,where they can speed up learning by a factorof50and more.Some of the GPU-based FNN implementations(Sec.5.16-5.19)have greatly contributed to recent successes in contests for pattern recognition(Sec.5.19-5.22),image segmentation(Sec.5.21), and object detection(Sec.5.21-5.22).5Supervised NNs,Some Helped by Unsupervised NNsThe main focus of current practical applications is on Supervised Learning(SL),which has dominated re-cent pattern recognition contests(Sec.5.17-5.22).Several methods,however,use additional Unsupervised Learning(UL)to facilitate SL(Sec.5.7,5.10,5.15).It does make sense to treat SL and UL in the same section:often gradient-based methods,such as BP(Sec.5.5.1),are used to optimize objective functions of both UL and SL,and the boundary between SL and UL may blur,for example,when it comes to time series prediction and sequence classification,e.g.,Sec.5.10,5.12.A historical timeline format will help to arrange subsections on important inspirations and techni-cal contributions(although such a subsection may span a time interval of many years).Sec.5.1briefly mentions early,shallow NN models since the1940s,Sec.5.2additional early neurobiological inspiration relevant for modern Deep Learning(DL).Sec.5.3is about GMDH networks(since1965),perhaps thefirst (feedforward)DL systems.Sec.5.4is about the relatively deep Neocognitron NN(1979)which is similar to certain modern deep FNN architectures,as it combines convolutional NNs(CNNs),weight pattern repli-cation,and winner-take-all(WTA)mechanisms.Sec.5.5uses the notation of Sec.2to compactly describe a central algorithm of DL,namely,backpropagation(BP)for supervised weight-sharing FNNs and RNNs. It also summarizes the history of BP1960-1981and beyond.Sec.5.6describes problems encountered in the late1980s with BP for deep NNs,and mentions several ideas from the previous millennium to overcome them.Sec.5.7discusses afirst hierarchical stack of coupled UL-based Autoencoders(AEs)—this concept resurfaced in the new millennium(Sec.5.15).Sec.5.8is about applying BP to CNNs,which is important for today’s DL applications.Sec.5.9explains BP’s Fundamental DL Problem(of vanishing/exploding gradients)discovered in1991.Sec.5.10explains how a deep RNN stack of1991(the History Compressor) pre-trained by UL helped to solve previously unlearnable DL benchmarks requiring Credit Assignment Paths(CAPs,Sec.3)of depth1000and more.Sec.5.11discusses a particular WTA method called Max-Pooling(MP)important in today’s DL FNNs.Sec.5.12mentions afirst important contest won by SL NNs in1994.Sec.5.13describes a purely supervised DL RNN(Long Short-Term Memory,LSTM)for problems of depth1000and more.Sec.5.14mentions an early contest of2003won by an ensemble of shallow NNs, as well as good pattern recognition results with CNNs and LSTM RNNs(2003).Sec.5.15is mostly about Deep Belief Networks(DBNs,2006)and related stacks of Autoencoders(AEs,Sec.5.7)pre-trained by UL to facilitate BP-based SL.Sec.5.16mentions thefirst BP-trained MPCNNs(2007)and GPU-CNNs(2006). Sec.5.17-5.22focus on official competitions with secret test sets won by(mostly purely supervised)DL NNs since2009,in sequence recognition,image classification,image segmentation,and object detection. Many RNN results depended on LSTM(Sec.5.13);many FNN results depended on GPU-based FNN code developed since2004(Sec.5.16,5.17,5.18,5.19),in particular,GPU-MPCNNs(Sec.5.19).5.11940s and EarlierNN research started in the1940s(e.g.,McCulloch and Pitts,1943;Hebb,1949);compare also later work on learning NNs(Rosenblatt,1958,1962;Widrow and Hoff,1962;Grossberg,1969;Kohonen,1972; von der Malsburg,1973;Narendra and Thathatchar,1974;Willshaw and von der Malsburg,1976;Palm, 1980;Hopfield,1982).In a sense NNs have been around even longer,since early supervised NNs were essentially variants of linear regression methods going back at least to the early1800s(e.g.,Legendre, 1805;Gauss,1809,1821).Early NNs had a maximal CAP depth of1(Sec.3).5.2Around1960:More Neurobiological Inspiration for DLSimple cells and complex cells were found in the cat’s visual cortex(e.g.,Hubel and Wiesel,1962;Wiesel and Hubel,1959).These cellsfire in response to certain properties of visual sensory inputs,such as theorientation of plex cells exhibit more spatial invariance than simple cells.This inspired later deep NN architectures(Sec.5.4)used in certain modern award-winning Deep Learners(Sec.5.19-5.22).5.31965:Deep Networks Based on the Group Method of Data Handling(GMDH) Networks trained by the Group Method of Data Handling(GMDH)(Ivakhnenko and Lapa,1965; Ivakhnenko et al.,1967;Ivakhnenko,1968,1971)were perhaps thefirst DL systems of the Feedforward Multilayer Perceptron type.The units of GMDH nets may have polynomial activation functions imple-menting Kolmogorov-Gabor polynomials(more general than traditional NN activation functions).Given a training set,layers are incrementally grown and trained by regression analysis,then pruned with the help of a separate validation set(using today’s terminology),where Decision Regularisation is used to weed out superfluous units.The numbers of layers and units per layer can be learned in problem-dependent fashion. This is a good example of hierarchical representation learning(Sec.4.4).There have been numerous ap-plications of GMDH-style networks,e.g.(Ikeda et al.,1976;Farlow,1984;Madala and Ivakhnenko,1994; Ivakhnenko,1995;Kondo,1998;Kord´ık et al.,2003;Witczak et al.,2006;Kondo and Ueno,2008).5.41979:Convolution+Weight Replication+Winner-Take-All(WTA)Apart from deep GMDH networks(Sec.5.3),the Neocognitron(Fukushima,1979,1980,2013a)was per-haps thefirst artificial NN that deserved the attribute deep,and thefirst to incorporate the neurophysiolog-ical insights of Sec.5.2.It introduced convolutional NNs(today often called CNNs or convnets),where the(typically rectangular)receptivefield of a convolutional unit with given weight vector is shifted step by step across a2-dimensional array of input values,such as the pixels of an image.The resulting2D array of subsequent activation events of this unit can then provide inputs to higher-level units,and so on.Due to massive weight replication(Sec.2),relatively few parameters may be necessary to describe the behavior of such a convolutional layer.Competition layers have WTA subsets whose maximally active units are the only ones to adopt non-zero activation values.They essentially“down-sample”the competition layer’s input.This helps to create units whose responses are insensitive to small image shifts(compare Sec.5.2).The Neocognitron is very similar to the architecture of modern,contest-winning,purely super-vised,feedforward,gradient-based Deep Learners with alternating convolutional and competition lay-ers(e.g.,Sec.5.19-5.22).Fukushima,however,did not set the weights by supervised backpropagation (Sec.5.5,5.8),but by local un supervised learning rules(e.g.,Fukushima,2013b),or by pre-wiring.In that sense he did not care for the DL problem(Sec.5.9),although his architecture was comparatively deep indeed.He also used Spatial Averaging(Fukushima,1980,2011)instead of Max-Pooling(MP,Sec.5.11), currently a particularly convenient and popular WTA mechanism.Today’s CNN-based DL machines profita lot from later CNN work(e.g.,LeCun et al.,1989;Ranzato et al.,2007)(Sec.5.8,5.16,5.19).5.51960-1981and Beyond:Development of Backpropagation(BP)for NNsThe minimisation of errors through gradient descent(Hadamard,1908)in the parameter space of com-plex,nonlinear,differentiable,multi-stage,NN-related systems has been discussed at least since the early 1960s(e.g.,Kelley,1960;Bryson,1961;Bryson and Denham,1961;Pontryagin et al.,1961;Dreyfus,1962; Wilkinson,1965;Amari,1967;Bryson and Ho,1969;Director and Rohrer,1969;Griewank,2012),ini-tially within the framework of Euler-LaGrange equations in the Calculus of Variations(e.g.,Euler,1744). Steepest descent in such systems can be performed(Bryson,1961;Kelley,1960;Bryson and Ho,1969)by iterating the ancient chain rule(Leibniz,1676;L’Hˆo pital,1696)in Dynamic Programming(DP)style(Bell-man,1957).A simplified derivation of the method uses the chain rule only(Dreyfus,1962).The methods of the1960s were already efficient in the DP sense.However,they backpropagated derivative information through standard Jacobian matrix calculations from one“layer”to the previous one, explicitly addressing neither direct links across several layers nor potential additional efficiency gains due to network sparsity(but perhaps such enhancements seemed obvious to the authors).。
LinearAlgebraStrang4thSolutionManual
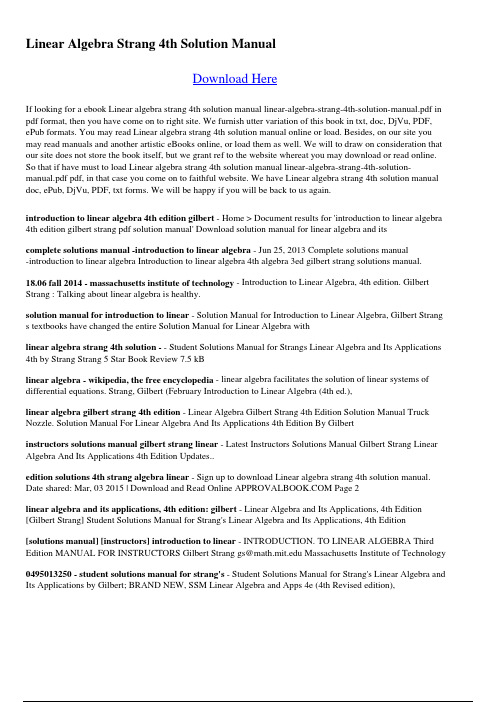
Linear Algebra Strang 4th Solution ManualDownload HereIf looking for a ebook Linear algebra strang 4th solution manual linear-algebra-strang-4th-solution-manual.pdf in pdf format, then you have come on to right site. We furnish utter variation of this book in txt, doc, DjVu, PDF, ePub formats. You may read Linear algebra strang 4th solution manual online or load. Besides, on our site you may read manuals and another artistic eBooks online, or load them as well. We will to draw on consideration that our site does not store the book itself, but we grant ref to the website whereat you may download or read online. So that if have must to load Linear algebra strang 4th solution manual linear-algebra-strang-4th-solution-manual.pdf pdf, in that case you come on to faithful website. We have Linear algebra strang 4th solution manual doc, ePub, DjVu, PDF, txt forms. We will be happy if you will be back to us again.introduction to linear algebra 4th edition gilbert - Home > Document results for 'introduction to linear algebra 4th edition gilbert strang pdf solution manual' Download solution manual for linear algebra and itscomplete solutions manual -introduction to linear algebra - Jun 25, 2013 Complete solutions manual-introduction to linear algebra Introduction to linear algebra 4th algebra 3ed gilbert strang solutions manual.18.06 fall 2014 - massachusetts institute of technology - Introduction to Linear Algebra, 4th edition. Gilbert Strang : Talking about linear algebra is healthy.solution manual for introduction to linear - Solution Manual for Introduction to Linear Algebra, Gilbert Strang s textbooks have changed the entire Solution Manual for Linear Algebra withlinear algebra strang 4th solution - - Student Solutions Manual for Strangs Linear Algebra and Its Applications 4th by Strang Strang 5 Star Book Review 7.5 kBlinear algebra - wikipedia, the free encyclopedia - linear algebra facilitates the solution of linear systems of differential equations. Strang, Gilbert (February Introduction to Linear Algebra (4th ed.),linear algebra gilbert strang 4th edition - Linear Algebra Gilbert Strang 4th Edition Solution Manual Truck Nozzle. Solution Manual For Linear Algebra And Its Applications 4th Edition By Gilbertinstructors solutions manual gilbert strang linear - Latest Instructors Solutions Manual Gilbert Strang Linear Algebra And Its Applications 4th Edition Updates..edition solutions 4th strang algebra linear - Sign up to download Linear algebra strang 4th solution manual. Date shared: Mar, 03 2015 | Download and Read Online Page 2linear algebra and its applications, 4th edition: gilbert - Linear Algebra and Its Applications, 4th Edition [Gilbert Strang] Student Solutions Manual for Strang's Linear Algebra and Its Applications, 4th Edition [solutions manual] [instructors] introduction to linear - INTRODUCTION. TO LINEAR ALGEBRA Third***************************************************.eduMassachusettsInstituteofTechnology 0495013250 - student solutions manual for strang's - Student Solutions Manual for Strang's Linear Algebra and Its Applications by Gilbert; BRAND NEW, SSM Linear Algebra and Apps 4e (4th Revised edition),introduction to linear algebra 4th solution | - Tricia Joy. Register; Terms Sponsored High Speed Downloads introduction to linear algebra 4th edition solution manual Introduction To Linear Algebra Gilbertgilbert strang introduction to linear algebra 4th - Gilbert Strang Introduction To Linear Algebra 4th Edition Solutions Manual Pdf downloads Linear Algebra Strang 4th Solution Manual. Linear Algebra Gilbert Strangstudent solutions manual for strang's linear - : Student Solutions Manual for Strang's Linear Algebra and Its Applications, 4thstudent solutions manual for linear algebra and its - Student Solutions Manual for Linear Algebra and Its Applications Linear Algebra and Its Applications, 4th Editionintroduction to linear algebra, 4th edition - mit mathematics - Introduction to Linear Algebra, 4th I hope this website will become a valuable resource for everyone learning and doing linear algebra. 1.1 Vectors and Linear linear algebra and its applications 4th edition textbook - Access Linear Algebra and Its Applications 4th Edition solutions now. Linear Algebra and Its Applications | 4th Edition. Solutions Manual; Scholarships;introduction to linear algebra 4th edition - Access Introduction to Linear Algebra 4th Edition solutions now. Our solutions are written by Chegg experts so you can be assured of the Solutions Manual;linear algebra strang solutions manual 4th - Tricia's Compilation for 'linear algebra strang solutions manual 4th instructor' Follow. solutions manual to Linear Algebra, 4th Filetype: Submitter:gilbert strang linear algebra 4th edition - Gilbert Strang Linear Algebra 4th Edition Solutions Truck Nozzle. GILBERT STRANG LINEAR ALGEBRA 4TH EDITION SOLUTIONS. DOWNLOAD: GILBERT STRANG LINEAR ALGEBRA 4TH EDITIONfree! solution manual of linear algebra by gilbert - Download solution manual of linear algebra by gilbert strang 4th edition ebooks and manuals at PdfDigest: For: Solution manual of linear algebra by gilber introduction to linear algebra 4th edition by - Introduction to Linear Algebra 4th Edition by Gilbert Strang fully written solutions / or book Introduction to Linear Algebra 4th Edition bylinear algebra and its applications, 4th edition - Renowned professor and author Gilbert Strang demonstrates that linear algebra is a Linear Algebra and Its Applications, Student Solutions Manualinstructor's solutions manual for strang's linear algebra and - schema:name " Instructor's solutions manual for Strang's Linear algebra and its applications, fourth edition "@en; schema:productID " 85780336" ;student solutions manual for strang 's linear algebra and its - Student Solutions Manual for Strang's Linear Algebra and Its Applications, 4th 4 edition Published October 1, 2005 byneed a solutions manual-- linear algebra and its - Oct 07, 2009 Need a solutions manual--Linear Algebra and Its Applications, 4th Ed, by Gilbert Strang?solutions manual instructors introduction to - Instructor S Solutions Manual For Strang S Linear Algebra And Its Applications rapidshare links Strang Introduction Linear Algebra 4th Edition Solution ManualRelated PDFs:1991 yamaha yz125 service manual, briggs and stratton 18 hp ic manuals, math foundations 11 study guide, solution guide management accounting 6e, e6b flight manual, 06 ktm 250 xcw manual, haas vf2 service manual, awwa manual m 51, kia rio car manual, isuzu fvr 1000 manual, hayward abg 100 manual, iq 2020 control box manual, junior maths 3 by a dasgupta manual, 61h booster relay manual, joseph topich chemistry solutionsmanual 6th edition, science a closer look pacing guide, wisconsin civil service exam study guide maintenance, honda civic 1995 1996 1997 98 1999 workshop manual download, principles of macroeconomics 5th edition study guide, owners manual for mini chopper motorcycle, harley fxwg manual, ford transit mini bus manual, 97 cavalier haynes repair manual, peugeot 505 workshop manual, golf manual derkeiler com, repair manual kawasaki ninja 250r, nrx 1800 service manual, bait of satan leaders guide, buick lacrosse manual, does northstar study guide work, salvation army pricing guide, d3306 caterpillar operation manual, ford expedition factory service manual, manual for suzuki rm85, navigation manual 2015 crv, vw golf v5 manual, 1999 kawasaki nomad manual, mercedes c 180 workshop manual, honda crv 2015 factory manual, colt 1903 pocket hammerless manual。
关于QUASIFROBENIUS环的一些研究
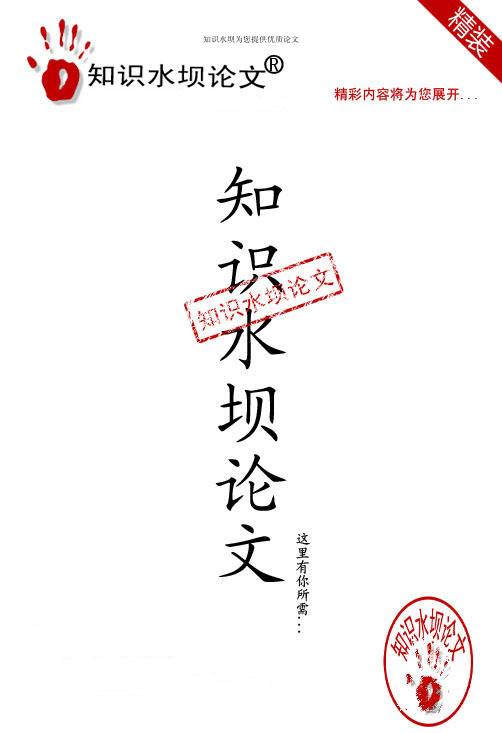
知识水坝为您提供优质论文证明若R为左完全右自内射环,则R满足有限生成右理想的左零化子的升链条件·此结果表明Faith猜想在左完全环条件情形是十分自然的.最后,我们证明若R为左完全右自内射环,则每个内射右R-模E都是其某个投射子模的内射包.这个结果很好地逼近了Faith猜想.关键词:Quasi—Frobenius环极大内射性Faith猜想ⅡSomeStudiesofQuasi--FrobeniusRingsSpecialtyFundamentalMathemticsCandidateZHAOGuoSupervisorWANGMingylQuasi-Frobeniusringsareoneofthemostimportantclassesofrings.Historically,quasi_Frobeniusringswereintroducedasgeneralizationofgroupalgebrasofafinitegroupoverafield.In1940,Baerinitiallyintroducedtheconceptofinjectivemodules,whichgreatlyenrichedthestudyofringtheory.Itturnsoutthatinjectivityplayanimportantroleincharacterizingquasi-Frobeniusrings.InviewofBaer’SCriterion,wehavethefollowingseriesofpropergeneralizationofself-injectivityofringsFP—injectiviOpjP—injectiviOp≥GP—injectivi纱jmin-injectiv妙Andthereisavastliteratureonthecharacterizationofquasi—Frobertiusringsintermsofweakenedinjectivity.Forinstance,thegistoftheFaith-MenalconjectureistOcharacterizequasi-FrobeniusbymeansofFP-injectivity.Motivatedandinspiredbytherecentworkinthisfield,weproperlygeneralizedtheconceptionofinjectivitytothatofmaximalinjectivityandusethelatertostudythefamousopenproblemofV.S.RamamurthiconcerningVonNeumannregularringsaswellasthefamousFaithconjectureconcemingquaSi-Frobeniusrings.Inchapter2,weinitiallyintroducetheconceptionofmaximalinjectivityandconstructanexampletoshowthatmaximalinjectivityisapropergeneralizationofinjectivity.Then,weshowthatforamaximallyinjectivering,itsrightsingularidealiscontainedinitsJacobsonradical.Consequently,weextendtheconceptionofIllinjectivedimensionstothatofmaximallyinjectivedimensions,whichturnsouttobepreciselythesupremumofprojectivedimensionsofsimpleR-modules.MotivatedbythefamousLambek’SCriterion。
Artificial Intelligence in Education
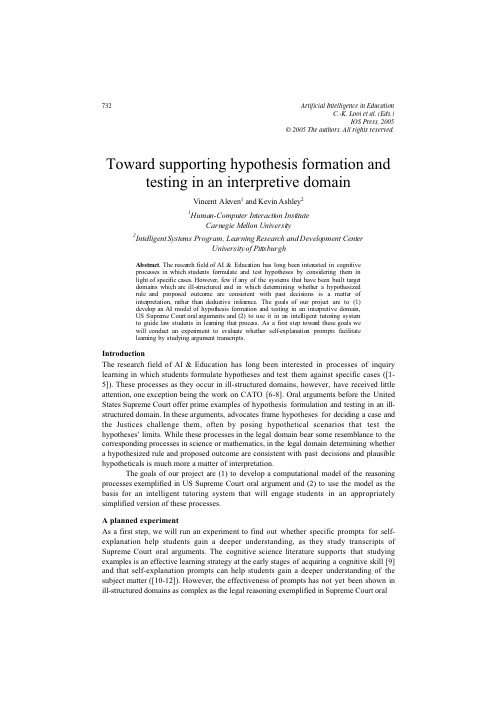
Toward supporting hypothesis formation andtesting in an interpretive domainVincent Aleven 1and Kevin Ashley 21Human-Computer Interaction InstituteCarnegie Mellon University2Intelligent Systems Program,Learning Research and Development CenterUniversity of PittsburghAbstr act .The resea rch field of AI &Educa tion has long been inter ested in cognitiveproce sses in which stude nts formulate and test hypotheses by considering them inlight of specific cases.However,few if any of the syste ms that have been built targe tdomains which are ill-structured and in which deter mining whether a hypothesizedrule and proposed outcome are consistent with past decisions is a matte r ofinter pretation,rathe r than deduc tive infer ence.The goals of our proje ct are to (1)develop an AI model of hypothesis forma tion and testing in an inter pretive domain,US Supre me Court oral arguments and (2)to use it in an intelligent tutor ing syste mto guide law stude nts in learning that proce ss.As a first step towar d these goals wewill conduct an exper iment to evaluate whether self-explanation prompts facilitatelearning by studying argument transcripts.IntroductionThe research field of AI &Education has long been interested in processes of inquiry learning in which students formulate hypotheses and test them against specific cases ([1-5]).These processes as they occur in ill-structured domains,however,have received little attention, one exception being the work on CATO [6-8].Oral arguments before the United States Supreme Court offer prime examples of hypothesis formulation and testing in an ill-structured domain. In these arguments, advocates frame hypotheses for deciding a case and the Justices challenge them,often by posing hypothetical scenarios that test the hypotheses’limits.While these processes in the legal domain bear some resemblance to the corresponding processes in science or mathematics, in the legal domain determining whether a hypothesized rule and proposed outcome are consistent with past decisions and plausible hypotheticals is much more a matter of interpretation.The goals of our project are (1)to develop a computational model of the reasoning processes exemplified in US Supreme Court oral argument and (2)to use the model as the basis for an intelligent tutoring system that will engage students in an appropriately simplified version of these processes.A planned experimentAs a first step,we will run an experiment to find out whether specific prompts for self-explanation help students gain a deeper understanding,as they study transcripts of Supreme Court oral arguments.The cognitive science literature supports that studying examples is an effective learning strategy at the early stages of acquiring a cognitive skill [9]and that self-explanation prompts can help students gain a deeper understanding of the subject matter ([10-12]).However,the effectiveness of prompts has not yet been shown in ill-structured domains as complex as the legal reasoning exemplified in Supreme Court oralArtificial Intelligence in EducationC.-K. Looi et al. (Eds.)IOS Press, 2005© 2005 The authors. All rights reserved.732Table1:Excerpt of transcript of oral argument made before the US Supreme Court in California v.Carney,105S.Ct.2066(1985),with self-explanation prompts addedArgument Transcript Self-Explanation PromptsQUESTION:Well,what if the vehicle is inone of these mobile home parks and hookedup to water and electricity but still has itswheels on?MR.HANOIAN:[*9]If it still has itswheels and it still has its engine,it iscapable of movement and it is capable ofmovement very quickly.1.Do you think H's response is effective?QUESTION:Even though the people areliving in it as a home and are paying rent forthe trailer space,and so forth?2.Why are the Justices adding these features to the hypothetical?QUESTION: Well, there are places where people can plug into water,and electricity, and do.There are many places,for example, in the state I came from where people go and spend the winter in a mobile home.And you think there would be no expectation of privacy in such circumstances?3.Why does it matter whether there would be expectations ofprivacy?4.If it was clear that there is,or should be,a high expectation ofprivacy in the current fact situation,would that favor H'sposition?5.Nothing is said from which we can infer how this particularhypothetical should be decided.Does that matter?That is,what good is it to use hypotheticals whose outcome is unknown?Wouldn't it be better to cite past cases,whose outcome we do know?MR.HANOIAN:Well,I am not suggesting that there is no expectation of privacy in those circumstances,Your Honor.6.By conceding that there are expectations of privacy in thehypothetical scenarios sketched by the judges,does H notreduce his chances of winning the case at hand?7.Does H concede that the mobile home park hypothetical shouldhave the opposite result as the case at hand?8.How would H distinguish the current case from the mobilehome park hypothetical?arguments.In light of the evidence that prompts do not benefit all students equally([10, 11]), it is important to ask how effective prompts are in such challenging domains.Table1shows excerpts from oral arguments made in the case of California v. Carney,105S.Ct.2066(1985),with self-explanation prompts inserted.This case involved the legality under the4th Amendment of the US Constitution of a warrantless search of a motor home located in a downtown San Diego parking lot.Police suspected defendant Carney of trading marijuana for sex acts.After they questioned a boy leaving Carney’s motor home,agents entered the motor home without a warrant or Carney’s consent, observed marijuana,and arrested Carney.The case pitted two conflicting principles:the State’s right to deal effectively with the exigent possibility that evidence of a crime will disappear versus the citizen’s constitutionally protected expectation of autonomy and privacy in his home.In the oral argument,the State’s attorney,Mr.Hanoian,proposed a bright line test: if the vehicle/home is capable of self-locomotion, then no warrant is required to search it.As shown in Table1,he then has to respond to the Justice’s challenge hypothetical:what result would his test produce when applied to a summer motor home with wheels that is hooked up to utilities?Mr.Hanoian responds that such a vehicle still might be moved in a hurry,but concedes the owners would have some expectation of privacy. Some of the self-explanation prompts focus on the effectiveness of that response. Others focus on the Justices’strategies and possible reasons for posing hypotheticals. DiscussionIn order to evaluate the effect of the self-explanation prompts,the study will compare the learning results of students studying argument transcripts with and without self-explanation V.Aleven and K.Ashley/Toward Supporting Hypothesis Formation and Testing733prompts.A pilot study involving two law students,a first-year student and a second-year student,provided some evidence that the prompts are useful.The students went through the Carney transcript twice,the first time without self-explanation prompts,the second time with.Each time,they were asked to answer a number of questions about the argument exchange they had just studied.We saw a difference in the quality of the answers between the first-year and the second-year student,indicating that the material is challenging.Further,we saw that the answers of the first-year student improved,after studying the transcript with the self-explanation prompts.Of course,such evidence is preliminary,due to the “small N”.Also,the improvement in the answers could be attributed simply to the fact that the student went through the transcript twice.This confound will be avoided in the actual experiment by having a control group.We are currently working on developing a suitable task by which we can measure any improvement in students’argument-making capabilities, a preliminary challenge for any research in an ill-structured domain.We expect the study to yield information about how students understand and make arguments. This information will help us start to build an argument model and develop an intelligent tutoring system.The study will also contribute to cognitive science by testing whether specific self-explanation prompts can help students to learn to engage in a process of hypothesis formation and testing in an ill-structured domain.AcknowledgementsThis research is sponsored by NSF Award IIS-0412830.The contents of the paper are solely the responsibility of the authors and do not necessarily represent the official views of the NSF.References[1].Bunt,A.,C.Conati,and K.Muldner,Scaffolding Self -Explanation to Impr ove Learning in Exploratory Learning Environments,in Proce edings of the 7th International Conference on Inte lligent TutoringSyste ms,ITS 2004,J.Lester,R.M.Vicar io,and F.Paraguaçu,Editors.2004,Springer:Berlin.[2].Collins,A.and A.L.Stevens,Goals and Strategies of I nquiry Tea chers,in Advances in InstructionalPsychology,R.G laser,Editor.1982,Lawrence Erlbaum:H illsdale,NJ.p.65-119.[3].Murra y,T.,L.Winship,and N.Stillings,Evaluation of the SimForest Inquiry Learning Environment:Inquiry Cycles and Collaborative Te aching Pra ctices,in AERA.2004:San Diego,CA.[4].Shute ,V.J.and R.Glaser ,A Large-Scale Evaluation of an Intelligent Discovery World:Smithtow n.Interactive Learning Environments,1990.1:p.51-77.[5].Woolf ,B.P.,et al.,Trac king Stude nt Propositions in a n Inquiry System,in Proce edings of the 11thInternational Conference on Artific ial Intelligence in Education,AIED 2003,U.H oppe,F.Verde jo,and J.Ka y,Editors.2003,IO S Press:A msterdam.p.21-28.[6].Aleve n,V.,Using Background Knowle dge in Case-Based Le gal Reasoning:A Computationl Model andan Intelligent Learning Environment.Artificial Inte lligence,2003.150:p.183-238.[7].Aleve n,V.and K.D.Ashle y,Teaching Case-Based Argumentation Thr ough a Model and Exa mples:Empir ical Evaluation of a n Intelligent Learning Environment,in Proce edings of the 8th InternationalConfe rence on Artificial Intelligence and Education,AI -ED '97,B.du Boula y and R.Mizoguchi,Editors.1997,IOS Press:Amsterda m.p.87-94.[8].Ashle y,K.D.,R.Desai,a nd J.Levine,Teaching Case-Ba sed Argume ntation Concepts Using DialecticArguments vs.D idactic Explanations,in Proce edings of the Sixth International Conference on Intelligent Tutoring System s,ITS 2002,S.A.Cerri,G.Gouar dères,and F.Paraguaçu,Editors.2002,Spinger:Berlin.p.585-595.[9].Atkinson,R.K.,et al.,Learning from e xamples:I nstructional principles from the worked examplesresea rch.Revie w of Educational Research,2000.70(2):p.181-214.[10].Chi,M.T.H.,et al.,Elic iting Self -Explanations Improves Understanding.Cognitive Science,1994.18:p.439-477.[11].Renkl,A.,et a l.,Learning from Worked-Out Examples:the Effects of Example Variability and ElicitedSelf-Explanations.Conte mporary Educational Psychology ,1998.23:p.90-108.[12].Schworm,S.and A.Renkl,Learning by solved example problems:I nstructional explana tions reduce self-expla nation activity,in Proce eding of the 24th Annual Confe rence of the Cognitive Science Society,W.D.Gray and C.D.Schunn,Editors.2002,La wrence Erlbaum:Mahw ah,NJ.p.816-821.V .Aleven and K.Ashley /Toward Supporting Hypothesis Formation and Testing734。
- 1、下载文档前请自行甄别文档内容的完整性,平台不提供额外的编辑、内容补充、找答案等附加服务。
- 2、"仅部分预览"的文档,不可在线预览部分如存在完整性等问题,可反馈申请退款(可完整预览的文档不适用该条件!)。
- 3、如文档侵犯您的权益,请联系客服反馈,我们会尽快为您处理(人工客服工作时间:9:00-18:30)。
Acknowledgements This paper is a part of my PhD. thesis at the University of Leicester. I thank Taif University in Saudi Arabia for funding my PhD. research, and Dr. Nicole Snashall for her helpful supervision and valuable suggestions. The author also thanks the referee for their helpful comments. Introduction In this paper we study the second Hochschild cohomology group HH2 (Λ) of all finite dimensional self-injective algebras Λ of finite representation type over an algebraically closed field K . In general, finite dimensional self-injective algebras of finite representation type over an algebraically closed field K were shown by Riedtmann in [9] to fall into one of the types A, D or E , depending on the tree class of the stable Auslander-Reiten quiver of the algebra. Riedtmann classified the stable equivalence representatives of these algebras of type A in [10]; Asashiba then showed that the stable equivalence classes are exactly the derived equivalence classes for types A, D in [2, Theorem 2.2]. In [1],the derived equivalence class representatives are given explicitly by quiver and relations. Happel showed in [8] that Hochschild cohomology is invariant under derived equivalence. So if A and B are derived equivalent then HH2 (A) ∼ = HH2 (B ). Hence 2 to study HH (Λ) for all finite dimensional self-injective algebras of finite representation type over an algebraically closed field K , it is enough to study HH2 (Λ) for the representatives of the derived equivalence classes. The algebras of type A fall into two types: Nakayama algebras and M¨ obius algebras, and the Hochschild cohomology of these algebras has already been studied. In [3], Erdmann and Holm give the dimension of the second Hochschild cohomology group of a Nakayama algebra. In [6], Green and Snashall find the second Hochschild cohomology group for the M¨ obius algebras. The main work of this paper is thus in determining HH2 (Λ) for the finite dimensional self-injective algebras of finite representation type of types D and E . In
SELF-INJECTIVE ALGEBRAS AND THE SECOND HOCHSCHILD COHOMOLOGY GROUP
arXiv:0709.0986v2 [math.RT] 22 Aug 2008
பைடு நூலகம்
DEENA AL-KADI Abstract. In this paper we study the second Hochschild cohomology group HH2 (Λ) of a finite dimensional algebra Λ. In particular, we determine HH2 (Λ) where Λ is a finite dimensional self-injective algebra of finite representation type over an algebraically closed field K and show that this group is zero for most such Λ; we give a basis for HH2 (Λ) in the few cases where it is not zero.
Date : August 22, 2008.
1
2
AL-KADI
Section 1 we give a summary of [1] which gives the explicit derived equivalence representatives we consider. Section 2 gives a short description of the projective resolution of [6] which we use to find HH2 (Λ). In Section 3, we give a general theorem, Theorem 3.6, which we use to show that HH2 (Λ) = 0 for most of our algebras. This is motivated by work in [6]. The strategy of the theorem is to show that every element in Hom(Q2 , Λ) is a coboundary so that HH2 (Λ) = 0, where Q2 is the second projective in a minimal projective resolution of Λ as a Λ,Λbimodule. For all other cases which are not covered by Theorem 3.6, we determine HH2 (Λ) by direct calculation, and find a basis for HH2 (Λ) in the instances where HH2 (Λ) = 0. The standard algebras are considered in Sections 4 and 5 and the non-standard algebras in Section 6. Finally Theorem 6.5 summarises our results and describes HH2 (Λ) for all finite dimensional self-injective algebras Λ of finite representation type over an algebraically closed field. As a consequence, we show that dim HH2 (Λ) = dim HH2 (Λ′ ) for a non-standard algebra Λ and its standard form Λ′ , where Λ and Λ′ are of type (D3m , 1/3, 1). This gives an alternative proof that Λ and Λ′ are not derived equivalent. 1. The derived equivalence representatives We give here Asashiba’s full classification from [1] and [2] of the derived equivalence class representatives of the finite dimensional self-injective algebras of finite representation type over an algebraically closed field. These derived equivalence class representatives are listed according to their type. From [9], the stable Auslander Reiten quiver of a self-injective algebra Λ of finite representation type has the form Z∆/ g , where ∆ is a Dynkin graph, g = ζτ −r such that r is a natural number, ζ is an automorphism of the quiver Z∆ with a fixed vertex, and τ is the Auslander-Reiten translate. Then typ(Λ) := (∆, f, t), where t is the order of ζ and f := r/m∆ such that m∆ = n, 2n − 3, 11, 17 or 29 as ∆ = An , Dn , E6 , E7 or E8 , respectively. We take the following results from [2]. Proposition 1.1. [2, Theorem 2.2] Given Λ a self-injective algebra of finite representation type then the type typ(Λ) is an element of one of the following sets: {(An , s/n, 1)|n, s ∈ N}; {(A2p+1 , s, 2)|p, s ∈ N}; {(Dn , s, 1)|n, s ∈ N, n ≥ 4}; {(Dn , s, 2)|n, s ∈ N, n ≥ 4}; {(D4 , s, 3)|s ∈ N}; {(D3m , s/3, 1)|m, s ∈ N, m ≥ 2, 3 ∤ s}; {(En , s, 1)|n = 6, 7, 8, s ∈ N}; and {(E6 , s, 2)|s ∈ N}. Theorem 1.2. [2, Theorem 2.2] Let Λ and Π be self-injective algebras of finite representation type. (i) If Λ is standard and Π is non-standard then Λ and Π are not derived equivalent. (ii) If Λ and Π are either both standard or both non-standard then the following are equivalent: 1) Λ and Π are derived equivalent; 2) Λ and Π are stably equivalent; 3) typ(Λ) = typ(Π).