PrimerSeq_technical_manual
《分子生物学》课件——一代测序技术
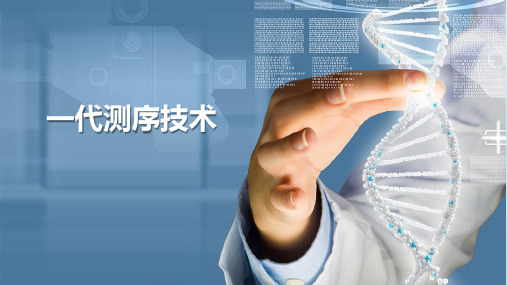
发展历程
第二代测序使用最为广泛。以 Roche公司的454技术, Illumina公司的Solexa, HiSeq技术为代表
一代测序技术
01 基本介绍
02 一代测序技术的方法
01 基本介绍
基本介绍——第一代测序的发明人
Fred Sanger及其同事
在1997年发明了利用DNA聚合酶和 双脱氧链终止测定DNA核苷酸序列的方法。
第一代DNA测序技术的核心技术是什么?
感谢观看
实验步骤
取待测DNA片段
将标记完的样品,分成四份
1.G反应,"硫酸二甲酯"
2.G+A反应 "甲酸"
3.T+C反应 "肼"
4.C反应 在NaCl存在时,只有C与肼反应,得到一 系列C末端的片段
一代测序技术的方法
形成大小不一的DNA链 电泳分离DNA链 根据同位素标记自显影后读出序列
Maxam-Gilbert化学降解测序法的弊端
基本介绍——第一代测序的发明人
第一代测序技术
概念:传统的链终止法,化学降解法,以及 在它们的基础上的各种DNA测序技术。
02 一代测序技术的方法
一代测序技术的方法
Maxam-Gilbert 化学降解测序法
01 对DNA片段5’或3’末端进行放射性标记。 02 采用特异性化学试剂修饰和裂解特定的碱基位点。
一代测序技术的方法
Sanger双脱氧链终止测序法原理
原理:是以待测单链DNA为模板,以dNTP为底物,在寡 核苷酸引物的引导下依据碱基互补配对原则。 在此对引物进行了事先标记,可以实现4个测序 反应在同一反应管中进行。可以利用自显影技术。
复制转录翻译
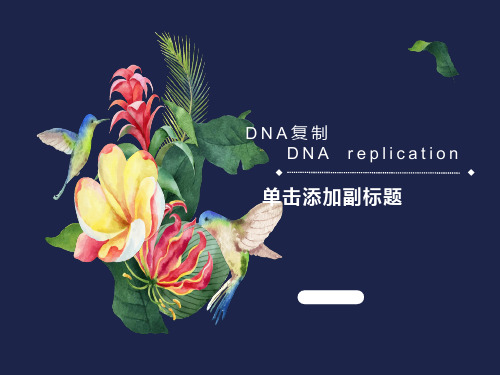
3´
结构式
半不连续复制 先导链 随后链 冈崎片断 引物 primer
复制叉 replication fork
DNA复制过程
DNA双螺旋的解除:DNA的解旋过程由DNA解旋酶催化解开后,单链DNA 结合蛋白(SSB )马上结合在分开的单链上,从而保持其伸展状态。随着解链的进行,在DNA 复制叉前就会形成一种张力而导致超螺旋的产生。现在发现这种张力主要是通过DNA拓扑异构酶的作用消除的。
起始:在一种特殊的RNA聚合酶-DNA引物酶的催化下,先合成一段长5—60个核苷酸的RNA引物,提供3'端自由-OH。然后,在DNA聚合酶Ⅲ的作用下进行DNA的合成。
延伸:只有一条DNA链的合成是连续的,而另一条链的合成是不连续的。所以从整个DNA分子水平来看,DNA两条新链的合成方向是相反的,但是都是从5’端向3’方向延伸。现在一般把一直从5’向3’方向延伸的链称作前导链,它是连续合成的。而另一条先沿5’ —3’方向合成一些片段,然后再由连接酶将其连起来成为长链,称为后随链,其合成是不连续的。这种不连续合成是由冈崎等人首先发现的,所以现在将后随链上合成的DNA不连续单链小片段称为冈崎片段。
b、校对作用:氨酰- tRNA合成酶的水解 部位可以水解错误活化的氨基酸。
原核生物多肽链的合成过程
1、肽链合成的起始
原核生物多肽链的合成分为三个阶段:肽链合成的起始、肽链的延伸、肽链合成的终止和释放。
肽链合成的终止及释放
肽链的延长
30S亚基• mRNA IF3- IF1复合物
30S• mRNA • GTP- fMet –tRNA- IF2- IF1复合物
复制子 replicon
单击此处添加小标题
一个复制起始点控制下复制的一段DNA
RNA-seq基础知识

RNA-seq基础知识1.RNA-Seq名词解释2.测序名词解释3.高通量测序常用名词解释4.转录组测序问题集锦RNA-Seq名词解释1.index 测序的标签,用于测定混合样本,通过每个样本添加的不同标签进行数据区分,鉴别测序样品。
2.碱基质量值(Quality Score或Q-score)是碱基识别(Base Calling)出错的概率的整数映射。
碱基质量值越高表明碱基识别越可靠,碱基测错的可能性越小。
3.Q30 碱基质量值为Q30代表碱基的精确度在99.9%。
4.FPKM(Fragments Per Kilobase of transcript per Millionfragments mapped)每1百万个map上的reads中map到外显子的每1K个碱基上的fragment个数。
计算公式为公式中,cDNAFragments 表示比对到某一转录本上的片段数目,即双端Reads数目;Mapped Reads(Millions)表示Mapped Reads总数,以10为单位;Transcript Length(kb):转录本长度,以kb个碱基为单位。
5.FC(Fold Change)即差异表达倍数。
6.FDR(False Discovery Rate)即错误发现率,定义为在多重假设检验过程中,错误拒绝(拒绝真的原(零)假设)的个数占所有被拒绝的原假设个数的比例的期望值。
通过控制FDR来决定P值的阈值。
7.P值(P-value)即概率,反映某一事件发生的可能性大小。
统计学根据显著性检验方法所得到的P 值,一般以P<0.05为显著,P<0.01为非常显著,其含义是样本间的差异由抽样误差所致的概率小于0.05或0.01。
8.可变剪接(Alternative splicing)有些基因的一个mRNA前体通过不同的剪接方式(选择不同的剪接位点)产生不同的mRNA剪接异构体,这一过程称为可变剪接(或选择性剪接,alternative splicing)。
常用分子生物学和细胞生物学实验技术介绍

常用分子生物学和细胞生物学实验技术介绍(2011-04-2311:01:29)转载▼标签:分子生物学细胞生物学常用实用技术基本实验室技术生物学实验教育常用的分子生物学基本技术核酸分子杂交技术由于核酸分子杂交的高度特异性及检测方法的灵敏性,它已成为分子生物学中最常用的基本技术,将单链记的探针进行那时交反应,用放射性自显影或酶反应显色,检测特定大小分子的含量。
可进行克隆基因的酶切图谱分析、基因组基因的定性及定量分析、基因突变分析及限制性长度多态性分析(RELP)等。
Northern印迹杂交:由Southerm印杂交法演变而来,其被测样品是RNA。
经甲醛或聚乙二醛变性及电泳分离后,转移到固相支持物上,进行杂交反应,以鉴定基中特定mRNA分子的量与大小。
该法是研究基因表达常用的方法,可推臬出癌基因的表达程度。
差异杂交(differentialhybridization)是将基因组文库的重组噬菌体DNA转移至硝酸纤维素膜上,用两种混合的不同cDNA探针(如:转移性和非转移性癌组织的mRNA逆转录后的cDNA)分别与滤膜上的DNA杂交,分析两张滤膜上对应位置杂交信息以分离差异表达的基因。
适用于基因组不太复杂的真核生物(如酵母)表达基因的比较,假阳性率较低。
但对基因组非常复杂的盐酸核生物(如人),则因工作量太大,表达的序列所占百分比较低(仅5%左右),价值不大。
cDNA微点隈杂交(cDNAmicroarrayhybridization)是指将20-50nt 的混合是利用两种来源一致而功能不同的组织细胞提取mRNA(或逆转录成的cDNA),在一定的条件下以过量的驱动mRNA或cDNA与测试的单链cDNA或mRNA进行液相杂交,通过羟基磷灰石柱层析筛选除去两者间同源的杂交体。
经多轮杂交策选、除去两者之间相同的基因成分,保留特异表达的目的基因或工基因片段。
以后者筛选cDNA文库,可获得特异表达的目的基因cDNA全长序列。
DNA测序技术参考手册-简

DNA测序技术参考手册上海生工生物工程技术服务有限公司—加拿大Sangon独资企业DNA测序样品的要求:本公司提供如下通用测序引物:M13+,M13-,T7, SP6, T7 ter,BGH rev, pGL3+, pGL3-,pGEX5’,pGEX3’。
其他引物请自带或提供序列由我们合成。
常用载体特征PCR类型测序模板注意事项1、总反应体积建议为50uL或100uL,扩增结束后取3ul用1%左右的琼脂糖电泳检测,应为单一的条带。
3ul样品总量不低于50ng(非常小的PCR产物可以酌情减小),PCR产物产量过低说明PCR扩增结果不是很理想,应改进条件重新扩增。
2、对于有杂带的PCR产物,需经琼脂糖电泳将目的片段切下来并回收。
有杂带的和扩增弥散的PCR产物如果不经过处理,测序不可能得到好的结果。
许多公司都有现成的从琼脂糖中回收DNA的试剂盒可供选用。
另外,该情况建议将PCR产物作克隆后进行测序。
3、经上述检测合格的PCR产物,需经过纯化去除未反应的引物,dNTP等影响测序反应的组分。
有多种纯化方法可供选择,Promega,Qiagen和生工等公司都有相应的产品可供选择。
纯化后的PCR产物经电泳检测估计总量应不低于200...(非常小的PCR产物可以酌情减小)。
...ng../Kb4、与质粒模板相比,PCR产物彼此间差异很大,因此,每个PCR模板应尽可能提供相应的PCR退火温度.....,以供测序时参考。
...产物的长度....和PCR5、PCR模板一般不应短于200bp,过短的PCR产物应经克隆后进行测序。
6、由于在PCR扩增过程中将产生一定比例的错误,因此测序结果一般不会比质粒模板好。
对于个别位置所测的序列可能与文献有差异,这是不可避免的,为了尽量减少此类情况发生,建议使用高保真Taq酶,以尽量减少PCR过程中的错误。
7、纯化好的PCR产物应溶于双蒸水..中,1xTE缓冲液将比较严...中或0.1x....TE重地影响测序反应。
单细胞RNA测序的样本制备指南

单细胞RNA测序的样本制备指南单细胞RNA测序(single-cell RNA sequencing, scRNA-seq)是一种高通量的基因表达分析技术,能够在单个细胞水平上测量基因转录水平的差异。
样本制备是scRNA-seq研究中至关重要的一步,合理的样本制备可以保证数据的质量和准确性。
以下是一份关于单细胞RNA测序样本制备的指南。
1.手套和无菌操作:在样品制备过程中,戴手套以避免污染。
所有操作需在无菌条件下进行,以防止细菌和其他污染物的干扰。
2. 细胞预处理:对粘附细胞进行酶消化,使用离心管先加入少量的酶溶液(如TrypLE Express),耐心地将细胞冲洗和颠倒使酶液均匀覆盖在细胞上,保持20-30分钟酶解,用无菌培养基停止消化。
3.单细胞分离:根据所研究的细胞类型和目的,可以选择不同的分离方法。
常用的方法包括流式细胞仪、微操作、动态孔阵列和微滴分离等。
选择合适的分离方法可以确保单个细胞的纯度和完整性。
4.细胞计数:使用细胞计数器或血球计数器等方法对细胞进行计数,以确保细胞的输入量在测序范围内。
5.细胞洗涤:用积累的细胞过滤网在细胞培养基中将细胞洗涤一次,去除残余的细胞碎片和无菌工具的残留物。
6. lysis缓冲液的添加:对细胞进行裂解是将细胞内的RNA和蛋白质释放出来的重要步骤。
在洁净的工作台上,用lysis缓冲液轻轻洗涤细胞沉淀,使细胞均匀悬浮,并在冷冻离心机中孵育10分钟。
7.RNA纯化:使用RNA纯化试剂盒提取总RNA。
按照试剂盒说明书的建议进行操作,确保提取的RNA质量和纯度。
使用RNA质量分析仪评估RNA的完整性和浓度。
8. 反转录:将提取的RNA转录为互补DNA(cDNA)。
使用反转录试剂盒和RNA模板-primer混合物,根据厂家的推荐步骤进行反转录反应。
反转录过程中需注意对RNA模板进行去除,以避免反转录产生的假阳性结果。
9.前处理:对生成的cDNA进行PCR扩增,将PCR产物纯化和浓缩,以提高下一步骤的测序效率。
ric-seq技术原理
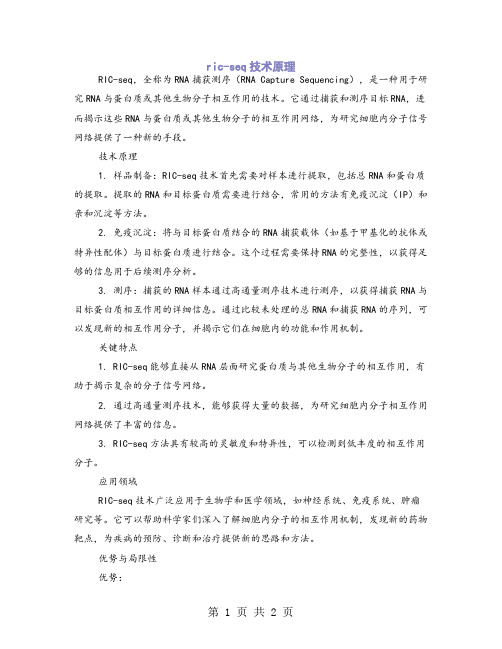
ric-seq技术原理RIC-seq,全称为RNA捕获测序(RNA Capture Sequencing),是一种用于研究RNA与蛋白质或其他生物分子相互作用的技术。
它通过捕获和测序目标RNA,进而揭示这些RNA与蛋白质或其他生物分子的相互作用网络,为研究细胞内分子信号网络提供了一种新的手段。
技术原理1. 样品制备:RIC-seq技术首先需要对样本进行提取,包括总RNA和蛋白质的提取。
提取的RNA和目标蛋白质需要进行结合,常用的方法有免疫沉淀(IP)和亲和沉淀等方法。
2. 免疫沉淀:将与目标蛋白质结合的RNA捕获载体(如基于甲基化的抗体或特异性配体)与目标蛋白质进行结合。
这个过程需要保持RNA的完整性,以获得足够的信息用于后续测序分析。
3. 测序:捕获的RNA样本通过高通量测序技术进行测序,以获得捕获RNA与目标蛋白质相互作用的详细信息。
通过比较未处理的总RNA和捕获RNA的序列,可以发现新的相互作用分子,并揭示它们在细胞内的功能和作用机制。
关键特点1. RIC-seq能够直接从RNA层面研究蛋白质与其他生物分子的相互作用,有助于揭示复杂的分子信号网络。
2. 通过高通量测序技术,能够获得大量的数据,为研究细胞内分子相互作用网络提供了丰富的信息。
3. RIC-seq方法具有较高的灵敏度和特异性,可以检测到低丰度的相互作用分子。
应用领域RIC-seq技术广泛应用于生物学和医学领域,如神经系统、免疫系统、肿瘤研究等。
它可以帮助科学家们深入了解细胞内分子的相互作用机制,发现新的药物靶点,为疾病的预防、诊断和治疗提供新的思路和方法。
优势与局限性优势:1. 直接从RNA层面研究蛋白质与其他生物分子的相互作用,能够揭示复杂的分子信号网络。
2. 高通量测序技术能够获取大量的数据,为研究细胞内分子相互作用网络提供了丰富的信息。
3. 方法具有较高的灵敏度和特异性,可以检测到低丰度的相互作用分子。
局限性:1. RIC-seq技术需要特定的实验设备和条件,操作过程相对复杂,需要专业技术人员进行操作。
如何使用Primer_Premier_5.0
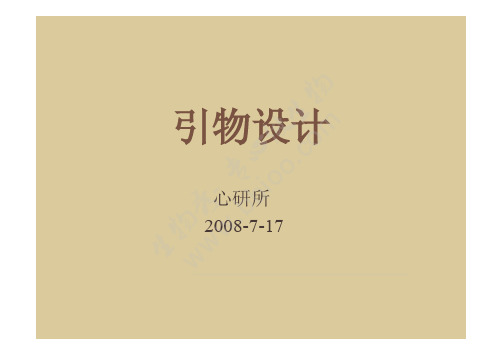
生物秀-专心做生物w w w .b b i o o .c o m生物秀-专心做生物w w w .b b i o o .c o m生物秀-专心做生w w w .b b i o o .c o m生物秀-专心做生物w w .b b i o o .c o m物秀-专心做生物.b b i o o .c o m生物秀-专心做生物w w w .b b i o o .c o m生物秀-专心做生w w w .b b i o o .c o m生物秀-专心做生w w w .b b i o o .c o m生物秀-专心做生w w w .b b i o o .c o m生物秀-专心做生w w w .b b i o o .c o m生物秀-专心做生物w w w .b b i o o .c o m生物秀-专心做生物w w w .b b i o o .c o m生物秀-专心做生物w w w .b b i o o .c o m生物秀-专心做生w w w .b b i o o .c o m生物秀-专心做生w w w .b b i o o .c o m生物秀-专心做生w w w .b b i o o .c o m生物秀-专心做生物w w w .b b i o o .c o m生物秀-专心做生物w w w .b b i o o .c o m生物秀-专心做生物w w w .b b i o o .c o m生物秀-专心做生w w w .b b i o o .c o m生物秀-专心做w w w .b b i o o .c o m生物秀-专心w w w .b b i o o .c生物秀-专心做w w w .b b i o o .c o m生物秀-专心做w w w .b b i o o .c o m①代表这些序列与引物匹配的得分值小于40分②代表这些序列与引物匹配的得分值位于40~50分③代表这些序列与引物匹配的得分值位于50-80分,④代表这些序列与引物匹配的得分值位于80-120分生物秀-专心做生物w w .b b i o o .c o m秀-专心做生物b b i o o .c o m。
- 1、下载文档前请自行甄别文档内容的完整性,平台不提供额外的编辑、内容补充、找答案等附加服务。
- 2、"仅部分预览"的文档,不可在线预览部分如存在完整性等问题,可反馈申请退款(可完整预览的文档不适用该条件!)。
- 3、如文档侵犯您的权益,请联系客服反馈,我们会尽快为您处理(人工客服工作时间:9:00-18:30)。
ContentOverview (2)Input (2)Exon Coordinates (2)Gene Annotation (3)Valid Gene IDs (3)Invalid Gene IDs (3)Sorting (3)Genomic Sequence (3)Aligned Reads (3)Splice Graph (4)Alternative Splicing Modules (ASM) (4)Overview (4)Biconnected Components (BCC) (4)Biconnected Components Algorithm (5)Equivalence of BCC and DiffSplice ASM (5)1. Single entry (6)2. Single exit (6)3. Alternative Paths (6)4. Minimal (6)Generating Isoforms (7)Overview (7)Only GTF Splice Junctions (7)GTF & Novel Splice Junctions (7)Estimating Isoform Counts & Exon Inclusion (7)Overview (7)Method (8)Choosing Flanking Exons (8)Overview (8)1. No-Estimation Method (8)2. Estimation Method (8)Obtaining Designed Primers (8)References (8)OverviewPrimerSeq designs RT-PCR primers that evaluate alternative splicing events by incorporating RNA-Seq data. PrimerSeq is particularly advantageous for designing a large number of primers for validating alternative splicing events found in RNA-Seq data. PrimerSeq incorporates RNA-Seq data in the design process to weight exons by their read counts. Essentially, the RNA-Seq data allows primers to be placed using actually expressed transcripts. This could be for your particular cell line or experimental condition, rather than using annotations that incorporate transcripts that are not expressed for your data. Alternatively, you can design primers that are always on constitutive exons. PrimerSeq does not limit the use of gene annotations and can be used for a wide array of species. For detailed examples, please visit the website at/.Fig. 1 PrimerSeq provides a Graphical User Interface (GUI) to allow convenient access by a wide range of users. PrimerSeq is available on the Windows operating system.InputUsers must specify target exon(s), gene annotation (GTF), genome sequence (FASTA), and RNA-Seq mapped reads (BAM or SAM, optional). The following sections detail technical aspects of the input. For information regarding loading the input into PrimerSeq, please see the getting started web page at /getting_started.html.Exon CoordinatesUsers specify the target exon(s) as a list of (+|-)chr:start-end in 0-based format (e.g. -chr17:73969705-73969866).Gene AnnotationPrimerSeq uses GTF files to define gene annotations. The GTF file defines the exons (nodes) and the minimum number of splice junctions (edges) in the splice graph (see Splice Graph). More junctions may be found based on the RNA-Seq data if the user allows this option. PrimerSeq ignores lines in the GTF that are not “exon” features. Users can download GTFs known to work from the PrimerSeq SourceForge website, download GTFs from UCSC or Ensembl, or use GTF output from transcript assemblers. However, unsorted GTFs or invalid gene IDs in GTF files can cause issues if not corrected.Valid Gene IDsIf the GTF has valid gene IDs, then the splice graph can be constructed using transcripts in the annotation that all have the same gene ID. Thus it is easy to know which exons (nodes) and splice junctions (edges) are included in the splice graph (see Splice Graph).Invalid Gene IDsSplice junctions and exons are included in the pertinent splice graph if they are weakly connected to the target exon. This could possibly include annotated transcripts that are not from the same gene. GTFs with invalid gene IDs are often obtained from the UCSC Table Browser. SortingThe GTF file must be sorted by, in order, contig name (chromosome), gene name, transcript name, start position, and end position. PrimerSeq provides an option to sort the GTF although the memory requirements may be too high for some desktops. Selected pre-sorted GTFs can be obtained from the download pages at /downloads.html. Genomic SequencePrimerSeq uses the FASTA file format as input for genomic sequences. It is more convenient if the FASTA file contains the entire genome of the species of interest, although no restriction is applied. The chromosome names in the FASTA should match the chromosome names of the target exons. Pygr is used to extract sequences in the middle of the FASTA(https:///p/pygr/). After pygr indexes the FASTA once, sequence lookups occur rapidly without heavy memory overhead.Aligned ReadsOne or multiple SAM or BAM files can be specified as input to PrimerSeq. If the file does not end with “.sorted.bam” then the file is converted to a sorted BAM file with a “.sorted.bam” extension. The SAM-JDK is used to perform this conversion (/). The SAM-JDK is also used to extract mapped reads in BAM files by indexing the BAM, which only occurs once.Splice GraphBuilding the splice graph consists of constructing a weighted directed acyclic graph (DAG) where nodes are exons and edges connect the exons. Exons (nodes) are defined by a unique pair of start and end positions. We used NetworkX, a python graph library, to implement the splice graph (Hagberg, et al., 2008).∙Exons, , are obtained from GTF annotation∙Exons are naturally topologically sorted by chromosome position∙Junctions, , are found from the GTF or both GTF and RNA-Seq data∙Junction counts are encoded in edge weightsThe user decides whether junctions are found either from the GTF or by both the GTF and RNA-Seq data. Users can override the default value for the minimum number of junction reads to define a novel splice junction and the minimum number of reads to assign a splice junction that is known from the annotation. This feature is useful for very low read counts. PrimerSeq does not use dummy source nodes or sink nodes like DiffSplice (Hu, et al., 2012) since the goal of PrimerSeq is to find real exons that can be used for primer design.Alternative Splicing Modules (ASM)OverviewTo find regions where alternative splicing occurs, PrimerSeq uses the biconnected components (BCC) algorithm (Sacomoto, et al., 2012). The splice graph is treated as an undirected graph for the purpose of finding the biconnected components. Only the ASM subgraph that includes the user’s target exon is used for further analysis. Previous use of the idea of Alternative Splicing Modules can be seen in the software DiffSplice. The BCC algorithm is implemented in many graph libraries and thus provides an easy to use tool for finding ASMs.Biconnected Components (BCC)An undirected graph is biconnected if the number of connected components does not increase even after removing a vertex and any edges incident to that vertex. The biconnected components of a graph are the maximal subset of vertices such that the biconnected property holds within each component. Note by technical conditions, every vertex in a graph is a part of at least one biconnected component and cut vertices are defined as being vertices that are a part of more than one biconnected component. Cut vertices if removed from the graph will cause the graph to no longer be connected. More precisely, there will be an increase in the number of distinct connected components. In terms of the splice graph, cut vertices are effectively “constitutive” exons because they cannot be skipped. Despite all vertices being a part of a biconnected component, useful information can be obtained since not all vertices are a part of trivial biconnected components. Trivial biconnected components consist of components that are less than three exons. Since meaningful AS events only occur with three exons, these trivialbiconnected components are filtered from the biconnected components list. This leaves only complex biconnected components with multiple paths through the biconnected component. By definition of biconnected components, all vertices (exons) that are not the first or last exon of a biconnected component must be skippable. First and last exons of a biconnected component, as defined here, have no predecessor nodes and no successor nodes, respectively, in the biconnected component. Note, including the first and last node in the ASM differs from the convention of ASMs in DiffSplice. For further details about BCCs, please see Equivalence of BCC and DiffSplice ASM.Biconnected Components AlgorithmThe biconnected components algorithm uses Depth-first Search (DFS) with two stored variables to find the biconnected components in an undirected graph.1.DFS depth of2.Lowest depth of all neighboring nodes of all descendant ofThe DFS based approach results in linear time complexity, ||||. We use a python BCC algorithm implementation from the NetworkX graph library (Hagberg, et al., 2008). Equivalence of BCC and DiffSplice ASMDiffSplice utilizes the property of dominance in a DAG to define where several exons could alternatively be included, i.e., ASM. Their methodology relies on a source node which has an edge to all first exons in a transcript and a sink node where every last exon in a transcript has an edge to the sink node. Thus every exon (node) has a path from a source node and to a sink node. DiffSplice describes formally an ASM as an induced subgraph H of a graph G with the following properties.1.Single entry2.Single exit3.Alternative paths4.MinimalUsing the BCC algorithm on the same splice graph with source and sink nodes, we would identify exactly the same ASMs as the dominance-based approach used by DiffSplice. In an effort to show equivalence we will show that the BCC algorithm satisfies all of the above criteria specified by DiffSplice. We will first introduce some terms used by DiffSplice.Single entry– all nodes come from a single node, the single entry node (possibly source node) Single exit– all edges go to a single node, the single exit node (possibly sink node)Post-dominate– If all paths from a vertex to a single exit node always includes a vertex then is said to post-dominate .Pre-dominate– If all paths from a single entry node to a vertex always includes a vertex then is said to pre-dominate .Immediate pre/post-dominator– a vertex that pre/post-dominates a vertex but does not pre/post-dominate any other pre/post-dominators of .1. Single entryFirst, suppose the BCC algorithm identified a component, an induced subgraph H, with two entry nodes. Since there is a source node, there must be a single node that immediately pre-dominates the two proposed entry nodes, possibly the source node in the original DAG. Thus at minimum there are two distinct paths which lead to the entry nodes from the immediate pre-dominator. This contradicts the maximal subset of vertices property of BCCs since at minimum the immediate pre-dominator node should be included in the BCC since its inclusion allows two alternative paths into the proposed BCC and removing individually the immediate pre-dominator or the two proposed entry nodes as a vertex leaves the BCC as still connected. Therefore with source and sink nodes defined, a BCC cannot have more than one entry node.2. Single exitSame logic as “1. Single entry” except order reversed for sink node.3. Alternative PathsThe alternative paths criterion requires a simple filtering step of BCCs due to the formal definition of BCC as a subgraph that does not increase the number of connected components after removal of any single vertex. Graphs with less than three connected nodes cannot be separated into two non-connected graphs by removal of a node because the maximal number of connected components in any graph is || (i.e. there are no edges in the graph). Therefore BCCs with a number of nodes less than 3 are removed. BCCs with at least three nodes must have alternative paths since the subgraph remains connected even after removing any vertex, implying there is another path from the single entry node to the single exit node.4. MinimalThe ‘minimal’ condition in DiffSplice states that any node in the ASM (excluding the entry and exit node) cannot post-dominate the entry node or pre-dominate the exit node. Suppose for a BCC that a node other than the entry or exit node could either pre-dominate the exit node or post-dominate the entry node. Removal of that node, a cut vertex, would separate the BCC into a graph before the node and after the node as a result of the definition of pre-dominance or post-dominance. More precisely, this dominance criterion indicates that all paths from entry node to exit node must use a certain node which indicates that its removal would separate the entry node and exit node into two distinct connected graphs. This clearly violates the property of BCC and thus a BCC always follow the ‘minimal’ condition.Generating IsoformsOverviewGeneration of isoforms depends on whether the user allows the finding of novel splice junctions from read support in the RNA-Seq data. If the user does not, the isoforms for only the ASM containing the target exon are exactly the isoforms defined in the GTF annotation. If the user allows novel splice junctions, novel generated isoforms for the ASM are added to the list of isoforms that are known from the annotation. It is an important note that the isoforms are only obtained in the region of the ASM and not for the full length of the gene.Only GTF Splice JunctionsBy default, isoforms are obtained from the GTF annotation.GTF & Novel Splice JunctionsIn addition to isoforms in the GTF annotation, novel isoforms are generated based on the novel junctions. All possible paths with in the ASM are considered and only isoforms (a path in the splice graph) that contain at least one novel junction are added to the list of known isoforms from the GTF.Estimating Isoform Counts & Exon InclusionOverviewA common occurrence is that a splice junction occurs in more than one isoform. To avoid inaccurate estimates of isoforms, an EM algorithm was used to estimate the MLE for the probabilities, , of a multinomial distribution. Specifically the probabilities, , are the probability that a read came from a certain isoform (i.e. not normalized by length). The MLE estimate for probability of each isoform defines the expected sufficient statistic (i.e. expected read counts) by the equation where is the total number of reads and is an index for each isoform. Assuming expected inclusion counts for exons are binomially distributed, the MLE for exon inclusion, ̂, is defined by the following equation:̂∑ | |∑Where denotes indexes corresponding to inclusion forms and denotes indexes for all isoforms. | | denotes the number of junctions in isoform . It is an important note that read counts are normalized by junction number after the EM algorithm as indicated by the above equation.MethodPrimerSeq uses a previous multinomial EM algorithm to approximate inclusion levels (Xing, et al., 2006). Primerseq does not use EST fragments like Xing et al. but rather we applied the fundamental algorithm to work with RNA-Seq data and the corresponding Splice Graph. Choosing Flanking ExonsOverviewThere are two methods for systematically selecting flanking exons to place primers on. The first method does not require estimation of exon inclusion level because the user defined that the flanking exons must be 100% included. The second method uses the ̂ estimates of exons to determine which flanking exons are above a user-defined threshold.1. No-Estimation MethodThe cut vertices of the biconnected component are chosen as the flanking exons.2. Estimation MethodThe two closest flanking exons with ̂ greater than the user-defined threshold are chosen. Obtaining Designed PrimersOnce the flanking exons are chosen, the sequences for the flanking exons and the target exon are obtained from the FASTA. The sequence and associated Primer3 parameters are used as input to Primer3 which then designs the primers. Information about the result is saved as a text file, displayed in the GUI, and viewable as a web page. For detailed user instructions please see the PrimerSeq website at .ReferencesHagberg, A.A., Schult, D.A. and Swart, P.J. (2008) Exploring Network Structure, Dynamics, and Function using NetworkX. Proceedings of the 7th Python in Science Conference. Pasadena, CA USA, pp. 11 - 15.Hu, Y., et al. (2012) DiffSplice: the genome-wide detection of differential splicing events with RNA-seq. Nucleic Acids Res.Sacomoto, G.A., et al. (2012) KISSPLICE: de-novo calling alternative splicing events from RNA-seq data. BMC Bioinformatics, 13 Suppl 6, S5.Xing, Y., et al. (2006) An expectation-maximization algorithm for probabilistic reconstructions of full-length isoforms from splice graphs. Nucleic Acids Res, 34, 3150-3160.。