场桥调度32
混堆模式下多箱区场桥联合调度
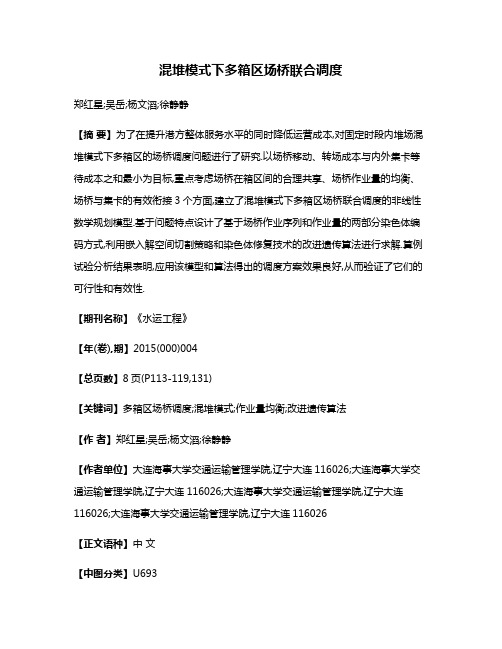
混堆模式下多箱区场桥联合调度郑红星;吴岳;杨文滔;徐静静【摘要】为了在提升港方整体服务水平的同时降低运营成本,对固定时段内堆场混堆模式下多箱区的场桥调度问题进行了研究.以场桥移动、转场成本与内外集卡等待成本之和最小为目标,重点考虑场桥在箱区间的合理共享、场桥作业量的均衡、场桥与集卡的有效衔接3个方面,建立了混堆模式下多箱区场桥联合调度的非线性数学规划模型.基于问题特点设计了基于场桥作业序列和作业量的两部分染色体编码方式,利用嵌入解空间切割策略和染色体修复技术的改进遗传算法进行求解.算例试验分析结果表明,应用该模型和算法得出的调度方案效果良好,从而验证了它们的可行性和有效性.【期刊名称】《水运工程》【年(卷),期】2015(000)004【总页数】8页(P113-119,131)【关键词】多箱区场桥调度;混堆模式;作业量均衡;改进遗传算法【作者】郑红星;吴岳;杨文滔;徐静静【作者单位】大连海事大学交通运输管理学院,辽宁大连116026;大连海事大学交通运输管理学院,辽宁大连116026;大连海事大学交通运输管理学院,辽宁大连116026;大连海事大学交通运输管理学院,辽宁大连116026【正文语种】中文【中图分类】U693集装箱堆场是集装箱变装的缓冲区,一方面通过内集卡的衔接影响船舶作业时间,另一方面通过外集卡提送箱关联货主满意度,因此提高堆场的作业效率是港方提升服务水平、增强竞争力的重要保障。
影响堆场作业效率的因素主要有3个:堆场布局、堆存策略和场桥调度。
其中,堆场布局属于港方长期规划内容,短期变动很小;优化的堆存策略和合理的场桥调度,是当前堆场研究的焦点。
为了提高堆场利用率,混堆模式的堆存策略被多数港口采纳,针对混堆模式进行的研究中,李建忠[1]利用两阶段模型研究混堆箱区箱位分配问题,首先平衡各箱区进出口箱量,然后以集卡运输距离最短为目标将每艘船的进出口箱分配到箱区内具体贝位;郑红星[2]引入新增压箱数的概念进行了混堆模式下进场箱的箱位指派建模。
混堆装船箱区多场桥调度

混堆装船箱区内多场桥调度研究郑红星董译文于凯刘进平(大连海事大学交通运输管理学院,大连116026,辽宁省)摘要:研究了一个混堆装船箱区的多场桥调度问题,重点考虑了内外集卡的优先级别、截止时间和倒箱量,构建了一个以港方费用最小的整数规划模型,提出了一个带有滚动时域的启发式算法用于求解该模型。
基于求解一系列的小规模算例实验,对比分析了该算法与用CPLEX求解的效果,还比较了它与相关文献中的改进遗传算法求解一系列小、大规模算例的效果;实验结果表明它能有效地求解混堆装船箱区的多场桥调度问题。
关键词:混堆;启发式算法;多场桥调度;滚动时域Hongxing Zheng*, Kai Yua, Yiwen Donga, Jinping Liuaa Tranportation Management College, Dalian Maritime University , Dalian 116026, Liaoning, PR chinaAbstract: This study addresses the yard crane (YC) scheduling problem at a mixed storage yard during vessel loading. It considers the container truck’s priority and deadline along with the container relocation volume. We develop an integer programming model to minimize the total cost. A heuristic algorithm is then developed to solve this problem, in which a rolling time domain is incorporated to improve the solution quality. The solution of the proposed heuristic algorithm is compared with the optimal solutions obtained by solving the model using the CPLEX software for small-sized problems. We also compare the solution of the proposed algorithm with optimal solutions obtained by solving the model using the improved genetic algorithm, which is provided in literature. Experiments show that the proposed algorithm in this paper can efficiently solve the multiple YC scheduling problem for mixed storage.Keywords:mixed storage, heuristic algorithm,multiple yard crane scheduling, rolling time domain1引言堆场作为集装箱码头中集港和疏港的关键环节,堆场中核心作业设备的效率关系到整个码头的运营。
集装箱堆场布局与场桥调度优化研究的开题报告
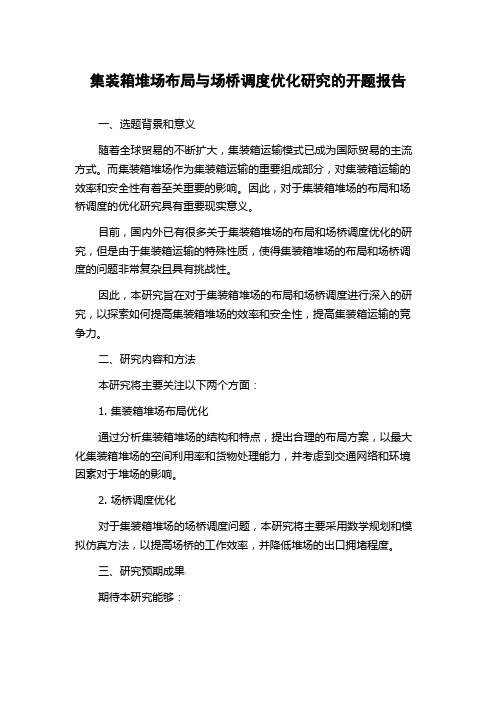
集装箱堆场布局与场桥调度优化研究的开题报告一、选题背景和意义随着全球贸易的不断扩大,集装箱运输模式已成为国际贸易的主流方式。
而集装箱堆场作为集装箱运输的重要组成部分,对集装箱运输的效率和安全性有着至关重要的影响。
因此,对于集装箱堆场的布局和场桥调度的优化研究具有重要现实意义。
目前,国内外已有很多关于集装箱堆场的布局和场桥调度优化的研究,但是由于集装箱运输的特殊性质,使得集装箱堆场的布局和场桥调度的问题非常复杂且具有挑战性。
因此,本研究旨在对于集装箱堆场的布局和场桥调度进行深入的研究,以探索如何提高集装箱堆场的效率和安全性,提高集装箱运输的竞争力。
二、研究内容和方法本研究将主要关注以下两个方面:1. 集装箱堆场布局优化通过分析集装箱堆场的结构和特点,提出合理的布局方案,以最大化集装箱堆场的空间利用率和货物处理能力,并考虑到交通网络和环境因素对于堆场的影响。
2. 场桥调度优化对于集装箱堆场的场桥调度问题,本研究将主要采用数学规划和模拟仿真方法,以提高场桥的工作效率,并降低堆场的出口拥堵程度。
三、研究预期成果期待本研究能够:1. 提出一套适用于集装箱堆场布局的优化方案,以最大化集装箱堆场的空间利用率和货物处理能力,同时考虑到交通网络和环境因素的影响。
2. 提出一套适用于场桥调度的优化方案,以提高场桥的工作效率,并降低堆场的出口拥堵程度。
3. 对于集装箱堆场的布局和场桥调度进行模拟和仿真,以验证所提出方案的有效性和可行性。
四、论文结构本研究的论文结构如下:第一章:绪论第二章:集装箱堆场布局优化第三章:场桥调度优化第四章:模拟与仿真第五章:结论与展望五、预期时间安排本研究预计历时12个月,具体时间安排如下:第一至第二个月:文献综述和理论分析第三至第六个月:集装箱堆场布局的优化算法研究及实现第七至第九个月:场桥调度的优化算法研究及实现第十至第十一个月:模拟与仿真第十二个月:论文撰写及评审注:以上时间安排仅供参考,具体实施可能会有所调整。
场桥调度31
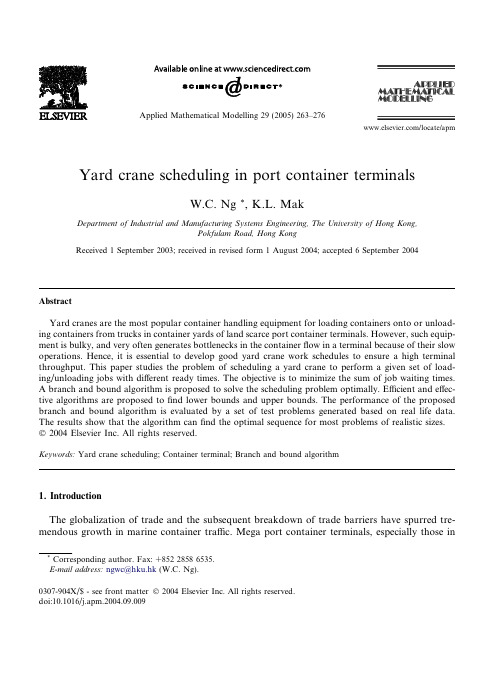
Yard crane scheduling in port container terminalsW.C.Ng *,K.L.MakDepartment of Industrial and Manufacturing Systems Engineering,The University of Hong Kong,Pokfulam Road,Hong Kong Received 1September 2003;received in revised form 1August 2004;accepted 6September 2004AbstractYard cranes are the most popular container handling equipment for loading containers onto or unload-ing containers from trucks in container yards of land scarce port container terminals.However,such equip-ment is bulky,and very often generates bottlenecks in the container flow in a terminal because of their slow operations.Hence,it is essential to develop good yard crane work schedules to ensure a high terminal throughput.This paper studies the problem of scheduling a yard crane to perform a given set of load-ing/unloading jobs with different ready times.The objective is to minimize the sum of job waiting times.A branch and bound algorithm is proposed to solve the scheduling problem optimally.Efficient and effec-tive algorithms are proposed to find lower bounds and upper bounds.The performance of the proposed branch and bound algorithm is evaluated by a set of test problems generated based on real life data.The results show that the algorithm can find the optimal sequence for most problems of realistic sizes.Ó2004Elsevier Inc.All rights reserved.Keywords:Yard crane scheduling;Container terminal;Branch and bound algorithm1.IntroductionThe globalization of trade and the subsequent breakdown of trade barriers have spurred tre-mendous growth in marine container traffic.Mega port container terminals,especially those in0307-904X/$-see front matter Ó2004Elsevier Inc.All rights reserved.doi:10.1016/j.apm.2004.09.009*Corresponding author.Fax:+852********.E-mail address:ngwc@hku.hk (W.C.Ng)./locate/apmApplied Mathematical Modelling 29(2005)263–276Asia,have been working at,or close to,capacity.In addition,the rising competition among ports has put considerable pressure on them to improve their customer service.Among all the common service performance measures,the terminal turnaround time,i.e.,the average period of time that a vessel stays in a terminal,is the key one.In most terminals,a large portion of the terminal turn-around time is spent on discharging and loading containers for a vessel.In a port container terminal,a number of vessels are often berthed alongside,and each vessel is served by multiple quay cranes which are supported by a large number of yard cranes in the yard. Fig.1depicts the typicalflow of containers in a container terminal.When a vessel arrives at the terminal,containers are normally discharged from the vessel,mounted onto trucks by quay cranes,and then unloaded by yard cranes at various locations in the yard for storage.In the load-ing operation,export containers loaded onto trucks by yard cranes at the yard are off-loaded at the quay and loaded onto a vessel by quay cranes.In both discharging and loading operations,the dispatching and scheduling of equipment are key planning problems faced by terminal planners.A few days before a vessel arrival,terminal planners determine the sizes and locations of stor-age areas for the containers to be loaded onto the vessel,and then determine the containers to be stacked in each storage area in each day.A few hours before a vessel arrives,they determine the sequence of discharging and loading containers for the vessel and the quay cranes to be assigned to the vessel.Once handling operations start on the vessel,handling jobs of the quay cranes will generate transportation jobs for the trucks and handling jobs for the yard cranes.The transpor-tation jobs are dispatched to highly mobile trucks on a real-time basis through voice communi-cation or a wireless computer network.On the other hand,the movement of each of the less mobile yard cranes running on rubber tires is normally restricted to a predetermined zone within a container yard for a few hours before the crane moves to another zone,to ensure yard traffic safety and to avoid long zone-to-zone travelling time.Within a zone,a yard crane can move freely to perform all handling jobs generated by different vessels.The physical handling rate of a yard crane is generally about half of that of a quay crane,and very often,the containerflow in a ter-minal is bottlenecked because of the slow yard crane operations.Hence,a good yard crane sched-ule can increase a terminalÕs throughput by increasing the containerflow to and from vessels as a result of reducing the truck waiting time.Fig.2shows a typical partial container yard layout of a container terminal:the yard is divided into multiple blocks called yard blocks;each yard block consists of a contiguous stretch of slots (40–60slots);and each slot has several rows(6–8rows).Each ground slot,denoted as a rectanglein the diagram,can store5–7containers.In most container terminals,zones are normallyformedFig.1.Typicalflow of containers in terminal operations.264W.C.Ng,K.L.Mak/Applied Mathematical Modelling29(2005)263–276W.C.Ng,K.L.Mak/Applied Mathematical Modelling29(2005)263–276265by grouping adjacent yard blocks together so as to simplify the control of yard crane movements and to reduce the amount of time in which the yard cranes occupy truck travelling lanes.In the layout depicted in Fig.2,there are two zones in the yard,zones1and2,formed by grouping blocks1and2,and blocks3and4together,respectively.The location of each job to be handled by a yard crane,as specified in the sequence of discharging and loading vessels is marked as a shaded rectangle in the diagram.In mega port container terminals like those in Singapore and Hong Kong,it is important to re-duce the waiting time of trucks by coordinating yard crane and truck movements.A terminal oper-ations control system would normally determine the expected arrival times of the trucks for transporting containers from or to each yard block during the coming hour.Currently,human judg-ment is used to determine a schedule for each yard crane to perform handling jobs for the arriving trucks.The scheduling objective is to reduce the amount of the trucksÕwaiting time for the cranes. Issues unique to port container terminal operations are receiving more attention due to increas-ing importance of marine transportation systems.The most important objective for a port con-tainer terminal is to increase its throughput or,in particular,to decrease the turnaround times of ships(see[9]).The turnaround time of a ship depends on the effectiveness of allocating and scheduling key resources,such as berths,yards,quay cranes,yard cranes and trucks. Problems of scheduling resources,like vehicles and material handling equipment,arise fre-quently in logistics systems and these problems have been extensively studied under different set-tings(see[2]).Unfortunately,the results reported in most research literature are not directly applicable to a port container terminal due to its unique characteristics.Research that specifically focuses on scheduling of quay cranes has been conducted by various researchers.Peterkofsky and Daganzo[10]and Daganzo[4]studied the static quay crane sched-uling problem with the objective of minimizing the aggregate vessel delay cost and they proposed various heuristics and exact algorithms to solve the quay crane scheduling problem.Daganzo[5] studied the impact of quay crane scheduling strategies on terminal throughput and ship delay. Several researchers have focused on the operational level issues particular to container loading or discharging operations of vessels,such as dispatching trucks and determining storage locationsfor containers.Bish et al.[1]studied the problem of assigning each discharging container to a yard location and assigning dispatching vehicles to the containers so as to minimize the time to dis-charge all the containers from a ship.Kim et al.[7]developed a dynamic programming method to determine the storage location of export containers to minimize the number of relocation movements expected for the loading operation.Recently,deployment and scheduling of container handling equipment in a container yard have been investigated by a number of researchers.Zhang et al.[11]studied the yard crane deployment problem with the objective offinding the times and routes of yard crane movements among yard blocks to minimize the total delayed workload in a container yard.A mixed integer programming model was proposed to determine the number of yard cranes to be deployed in each yard block in each planning period.A Lagrangian relaxation-based algorithm was used to obtain the optimal solution.Cheung et al.[3]extended the ZhangÕs model by relaxing the restriction that crane move-ment must be completed within a period.Kim and Kim[6]studied the problem of routing a single yard crane to support the loading operation of a vessel.A mixed integer program was proposed to determine the number of container pickups at each location and the sequence of locations to be visited by the yard crane,and an efficient optimal algorithm was developed.However,the assump-tion of having a dedicated group of yard cranes just to support vesselsÕloading operations is not a realistic one for port container terminals with a large number of berths.In this paper,the problem of scheduling a yard crane to perform handling jobs with different ready times within its movement zone to minimize the sum of jobwaiting times is analyzed in de-tail.A mixed integer programming model is proposed in Section2.In Sections3–5,the properties of the problem are studied thoroughly and a branch and bound algorithm with efficient and effec-tive bounding procedures is proposed on the basis of such properties.A numerical example is solved to illustrate the algorithm.Section6presents the results of computational experiments and the last section concludes the paper.2.Mathematical modelIn this section,the problem of scheduling a yard crane to handle all the jobs with different ready times within its movement zone is formulated as an integer program.There are n jobs in the zone to be handled by the yard crane in the current planning period.Let r i,i=1,2,...,n,be the ready time(truck arrival time)of job i,h i,i=1,2,...,n,be the time required by the yard crane to han-dle job i,d ij,i=1,2,...,n and j=1,2,...,n,be the time required for the yard crane to travel from the location of job i to the location of job j,and d0j be the time required for the yard crane to travel from the initial location of the yard crane to the location of job j.The n jobs are indexed in such a way that r i6r i+1.The following decision variables are needed to formulate the yard crane scheduling problem mathematically:t i the time at which the yard crane completes handling of job i;X ij¼1if job i is handled before job j; 0otherwise:&266W.C.Ng,K.L.Mak/Applied Mathematical Modelling29(2005)263–276Denote the set of t i by T and the set of X ij by X .For the truck dispatched to job i ,its waiting time in the yard is given by t i Àh i Àr i .With given r i ,the problem of finding the optimal schedule that minimizes the sum of jobwaiting times can b e stated as Problem YCS:Minimize X n i ¼1ðt i Àh i Àr i Þsubject tot i P r i þh i ;i ¼1;2;;...;n ;ð1Þt j Àt i P d ij þh j Àð1ÀX ij ÞM ;i ;j ¼1;2;...;n ;and i ¼j ;ð2ÞX ij þX ji ¼1;i ;j ¼1;2;...;n ;and i ¼j ;ð3ÞX ij 2f 0;1g ;i ;j ¼1;2;...;n ;and i ¼j :ð4ÞThe objective of Problem YCS is to minimize the sum of job waiting times.Constraints (1)give the relationship between a job Õs completion time,ready time and handling time.Constraints (2)give the relationship between the completion time of a job and that of its successors.Constraints (3)ensure the correctness of the precedence relationship specified by X .Constraints (4)are simple binary constraints.As the set of t i ,i =1,2,...,n ,that minimizes P ni ¼1t i ,the total completion time,would also minimize P ni ¼1ðt i Àh i Àr i Þ,Problem YCS can be simplified as minimizing P ni ¼1t i subject to constraints (1)–(4).Denote the jobhandled in the i th position of an n -jobsequence b y [i ]and the completion time of the jobb y t [i ].For the case of r i =0for i =1,2,...,n ,it can be shown thatt ½i ¼X i j ¼1ðh ½j þd ½j À1 ½j Þ;and that the total completion time is equal toX n i ¼1X i j ¼1ðh ½j þd ½j À1 ½j Þ¼Xn i ¼1ðn Ài þ1Þðh ½i þd ½i À1 ½i Þ:It is noted that for this case,Problem YCS is a problem of non-preemptive scheduling with dif-ferent jobready times on a single machine to minimize total completion time and the schedulingproblem is an NP-complete problem (see [8]).A branch and bound algorithm is proposed in this paper to solve Problem YCS optimally.In the following section,the procedure for computing lower bounds is discussed in detail.3.Lower boundIt can be shown from the definitions of t [i ],h [i ],r [i ]and d [i -1][i ]that for i =1,2,...,n ,t ½i ¼h ½i þmax f t ½i À1 þd ½i À1 ½i ;r ½i g ;ð5Þwhere t [0]is defined to be 0.It can be shown from the above equation thatW.C.Ng,K.L.Mak /Applied Mathematical Modelling 29(2005)263–276267t½i P maxX ij¼1ðh½j þd½jÀ1 ½j Þ;r½i þh½i():ð6ÞThe above expression can be used tofind a lower bound for t[i].In stating the lower bound,some extra notations are needed.Let a i=min{h j+d ij j j=1,2,...,n},b i=min{h i+d ji j j=1, 2,...,n},c i=r i+h i,and a[i],b[i]and c[i]be the i th smallest elements of the sets{a1,a2,...,a n}, {b1,b2,...,b n}and{c1,c2,...,c n},respectively.It can be deduced from the definitions of a[i],b[i] and c[i]and from expression(6)that there exists a lower bound for t[i], t½i ,satisfying the following expression:t ½i ¼max a0þX iÀ1j¼1a½j ;X ij¼1b½j ;c½i();ð7Þwhere a0is defined to be min{h j+d0j j j=1,2,...,n}.It can be shown from the above expression that the total completion time of the n jobs satisfies the conditionX n i¼1t i PX ni¼1t½i:ð8ÞIt is obvious that expression(8)gives a lower bound for P ni¼1t i.In the following,the analysis forfinding better lower bounds is outlined.Let S k={I1,I2,...,I k}denote a partial sequence of length k,k=1,2,...,n,where I i, i=1,2,...,k,denotes the i th jobhandled in the partial sequence.S k is said to be feasible if I i, i=1,2,...,k,are all distinct.Furthermore,let S k(j)be a feasible partial sequence of length k with job j as the last jobin the partial sequence(i.e.,I k=j)and g(S k(j))be the makespan of S k(j).For a given S k(j),consider the feasible partial sequence formed by appending job i to S k(j).It can be deduced from constraints(1)and(2)and the definition of t½kþ1 that the following lemma is true.Lemma1.g({S k(j),i})=max{r i+h i,g(S k(j))+d ji+h i}where g({S k(j),i})is the makespan of the feasible partial sequence formed by appending job i to S k(j).It can easily be shown from the definition of t½kþ1 and Lemma1that the following lemma is also true.Lemma2.The minimum makespan of any feasible partial sequences of length k+1with job i as the last job is given bymax t½kþ1 ;r iþh i;minall feasible S kðjÞgðS kðjÞÞþd jiþh i ÂÃ&':Let h k(j)be the set containing m shortest feasible partial sequences of length k with job j as the last job.Hence,the makespan of a feasible partial sequence of length k with job j as the last job, not belonging to h k(j),is greater than or equal to the makespan of S k(j),S k(j)2h k(j).Thus,it is obvious that the following lemma is true.Lemma3.If no feasible partial sequence can be formed by appending job i to S k(j)for allS k(j)2h k(j),max Sk ðjÞ2h kðjÞf gðS kðjÞÞþd jiþh ig is a lower bound for the minimum makespan of268W.C.Ng,K.L.Mak/Applied Mathematical Modelling29(2005)263–276any feasible partial sequences of length k+1with job i as the last job and job j as the second last job.Let k(i)be the set of job index j,j=1,2,...,n,such that appending job i to each S k(j)2h k(j) results in infeasible partial sequences.By considering feasible partial sequences as well as infeasi-ble partial sequences of length k+1formed by appending job i to S k(j),it can be shown using Lemmas2and3that Lemma4is true.Lemma4maxt½kþ1r iþh iminminj2kðiÞminS kðjÞ2h kðjÞgðS kðjÞÞþd jiþh i!minj2kðiÞmaxS kðjÞ2h kðjÞgðS kðjÞÞþd jiþh i!8>>><>>>:8>>>>>>>>><>>>>>>>>>:is a lower bound for the minimum makespan of any feasible partial sequences of length k+1with job i as the last job.Denote the lower bound for the makespan of any feasible partial sequence of length k with job j as the last jobin the sequence(i.e.,I k=j)b y g kðjÞ:It is clear from the definition of g kðjÞthat for j=1,2,...,n,S kðjÞP g kðjÞand hence,it can be shown from Lemma4that the following lemma is true.Lemma5gkþ1ðiÞ¼max t½kþ1 ;r iþh i;minj¼igkðjÞþd jiþh iÂÃ&':The above lemmas give useful insights for developing an algorithm tofind a lower bound for any feasible partial sequence of length k,k=1,2,...,n.It follows from Lemma5thatg 1ðiÞ¼max t½1 ;r iþh i;d0iþh iÈÉis a lower bound for the makespan of any feasible partial se-quences of length1with job i as the last jobin the sequences.For k=2,3,...,n,the following procedure is proposed forfinding g kðiÞ,i=1,2,...,n,a lower bound for the makespan of any partial sequences of length k with job i as the last job.Procedure LBStep0.Set the lower bound for the makespan of the partial feasible sequence of length1with jobi as the last jobin the sequence, g1ðiÞ,to max t½1 ;r iþh i;d0iþh iÈÉfor i=1,2,...,n.Initialize the set of the feasible partial sequence of length1with job i as the last jobin the sequence,h1(i)={i}for i=1,2,...,n.Initialize the length of partial sequences to be considered by setting x=2.Step1.Set jobindex i=1.Step2.Set j=1,R=Q=null set,and min=1.W.C.Ng,K.L.Mak/Applied Mathematical Modelling29(2005)263–276269Step 3.If i 5j thenif h x À1(j )=null set then if max t ½x ;r i þh i ; g x À1ðj Þþd ji þh i ÈÉ<min then set min ¼max t ½x ;r i þÈh i ; g x À1ðj Þþd ji þh i g ;else,for each partial sequence S x À1(j )2h x À1(j ),if the partial sequence of length x formed by appending i to S x À1(j ),{S x À1(j ),i },is feasible,i.e.i and all the job indexes in S x À1(j )are distinct,thenQ =Q [the feasible partial sequence of length x formed;set the makespan of the feasible partial sequence formed =max t ½x ;r i þh i ;g ðS x À1ðj ÞÞþd ji þh iÂÃÈÉelse,R =R [the infeasible partial sequence of length x formed;else,goto Step 5.Step 4.If Q is empty thenif the maximum makespan of the partial sequences in R <min then set min =the max-imum makespan of the partial sequences in R .Step 5.Set j =j +1.If j 6n goto Step 3.Step 6.If Q is non-empty,i.e.there exist some feasible partial sequences of length x with job i asthe last job,thenh x (i )=the set of the first m elements in Q ranked in ascending order of makespan withtheir makespans <min,set gx ði Þ=the minimum makespan of the feasible partial sequences in h x (i );else,set g x ði Þ¼max f r i þh i ; t ½x ;min g .Step 7.Set i =i +1to consider the next job;if i 6n goto Step 2.Step 8.Set x =x +1.if x 6k then goto Step 1;else,terminate.In the above procedure,Step 0determines a lower bound for the makespan of the feasible se-quence of length 1with job i as the last jobusing the result of Lemma 5.For each i ,i =1,2,...,n ,and x ,x =2,3,...,k ,by considering all possible j as the (x À1)th job,Steps 2–7finds h x (i ),the set containing feasible partial sequences of length x with job i as the last joband with makespan shorter than the shortest makespan of infeasible partial sequences,if they exist,and set the lower bound equal to the shortest makespan of all the feasible partial sequences in h x (i ).Otherwise,thelower bound is set to the value of min found in Steps 3and 4.Then,Step 6determines gx ði Þbased on the results of Lemmas 4and 5.It is noted that the n -jobsequence determined b y Procedure LB possesses the interesting prop-erty given in the following lemma.Lemma 6.Let i k be the i that minimizes g k ði Þ,k =1,2,...,n,and S Ãn ¼f I Ã1;I Ã2;...;I Ãn g bethe sequence determined in Procedure LB that minimizes g n ði n Þ.If S Ãn is feasible (i.e.I Ãk are all distinct)and I Ãk ¼i k for k =1,2,...,n then S Ãn is the optimal sequence for Problem YCS.270W.C.Ng,K.L.Mak /Applied Mathematical Modelling 29(2005)263–276Proof.If I Ãk are all distinct,X ij ,implied by I Ãk found,and gk ði k Þ,determined in Steps 3and 6of Procedure LB,are feasible to constraints (1)–(4).Let f ^T;^X g be the optimal solution of Problem YCS and ^Y ki be a parameter assuming value of 1if job i is the k th jobhandled in the optimal sequence.It follows from the definition of i k , g k ði Þ,^t i and ^Y ki that P ni ¼1^Yki ^t i P g k ði k Þfor all k .Thus,if I Ãk ¼i k for k =1,2,...,n ,f X ij ; gk ði k Þg is the optimal solution of Problem YCS.If S Ãn determined in the lower bounding procedure does not possess the property stated in the above lemma, g k ði Þcan still be used to find a lower bound for Pn i ¼1t i .It is obvious from the def-inition of gk ði Þthat if ^Y ki ¼1then ^t i P g k ði Þ.Hence,X n i ¼1X n k ¼1^Y ki ^t i P Xn i ¼1X n k ¼1^Y ki g k ði Þ:ð9ÞHowever,P n i ¼1P nk ¼1^Y ki g k ði Þcannot be used directly as a lower bound for Pn i ¼1t i as one wouldnot know ^Y ki without determining the optimal solution of Problem YCS.In the following,a meth-od is proposed to determine a lower bound for P n i ¼1P nk ¼1^Y ki gk ði Þ.Let Z ki be a binary variable assuming value of 1if job i is the k th jobhandled in a sequence.Consider the following optimization problem:MinimizeXn i ¼1X n k ¼1Z ki g k ði Þsubject toX n k ¼1Z ki ¼1;i ¼1;2;...;n ;ð10ÞX n k ¼1Z ki ¼1;k ¼1;2;...;n ;ð11ÞZ ki 20;1;i ;k ¼1;2;...;n :ð12ÞThe above optimization problem is a simple assignment problem which can easily be solved.Let ^Zki ,i ,k =1,2,...,n ,be the optimal solution of the above problem.It is obvious form the def-inition of ^Y ki that ^Y ki ,i ,k =1,2,...,n ,satisfies constraints (10)–(12)and hence is a feasible solu-tion of the above assignment problem.Hence,P n i ¼1P nk ¼1w i ^Y ki g k ði ÞP P n i ¼1P n k ¼1w i ^Z ki g k ði Þand thus,it is obvious from expression (9)that P n i ¼1P n k ¼1w i ^Y ki ^t i P P n i ¼1P n k ¼1w i ^Z ki g k ði Þ,which clearly indicates that P n i ¼1P nk ¼1w i ^Zki g k ði Þis a lower bound for the optimal objective value of Problem YCS.A numerical example is used in the following to illustrate each step of the procedure for finding a lower bound for the optimal solution of Problem YCS.Consider the problem of scheduling a yard crane to perform 5handling jobs with different job ready times:h i =4for i =1,2,...,5,r 1=2,r 2=5,r 3=7,r 4=13,and r 5=15.The travelling time between locations of jobs i and j is:d 01=2,d 02=5,d 03=2,d 04=1,d 05=2,d 12=3,d 13=0,d 14=1,d 15=4,d 23=3,d 24=4,d 25=7,d 34=1,d 35=4,d 45=3and d ij =d ji .With the above problem parameters,it can be determined from expression (7)that t ½1 ¼6, t ½2 ¼9,t ½3 ¼13, t ½4 ¼19and t ½5 ¼26.The lower bound given by expression (8)is 73.Table 1summarizes the working of Procedure LB for the case of m =1.W.C.Ng,K.L.Mak /Applied Mathematical Modelling 29(2005)263–276271Since S Ãn is infeasible,the optimality condition in Lemma 6is not met and thus,one has to solve the assignment problem.It is found that the lower bound given by the optimal objective function value of the assignment problem,P n i ¼1P n k ¼1^Z ki g k ði Þ,is 82.4.Upper boundTo make a branch and bound algorithm more efficient,an efficient and effective heuristic is needed.The heuristic proposed in this section,which is a simple modification of Procedure LB,computes the amount of contribution of each job to the total completion time and then finds the partial sequence with the smallest sum of these contributions.It can be shown from expression (5)thatt ½i ¼X i j ¼1f ½j ;Table 1Summary of computations of Procedure LB for the example problem k i g k ði Þh k (i )116{1}29{2}311{3}417{4}519{5}2115{3,1}213{1,2}311{1,3}417{1,4}519{1,5}3115Null set 218{1,3,2}319Null set 417{1,3,4}519{1,3,5}4122Null set 222Null set 319Null set 420Null set 523Null set 5126Null set 226Null set 326Null set 426Null set 527Nullset272W.C.Ng,K.L.Mak /Applied Mathematical Modelling 29(2005)263–276where f [j ]=h [j ]+max{d [j -1][j ],r [j ]Àt [j -1]}.Hence,the total completion time is given byX n i ¼1t ½i ¼X n i ¼1ðn Ài þ1Þf ½i :Thus,in view of the above equation,(n Ài +1)f [i ]can be used to measure the amount of contri-bution of the job in position i to the total completion time.Detailed steps of the upper bound heu-ristic are as follows:Heuristic UBStep 0.Initialize the set of unscheduled jobs ={1,2,...,n }and i =1.Step 1.Find job i *,the jobin the set of unscheduled jobs with the smallest (n Ài +1)f [i ],schedule job i *as the i th joband delete the jobfrom the set of unscheduled job s.Repeat this step for i =2,3,...,n .The yard crane scheduling problem in Section 3is again used to illustrate the steps of Heuristics UB.The sequence determined by Heuristic UB is 1–3–4–5–2with a total completion time of 93.5.Branch and bound algorithmIn this section,a branch and bound algorithm is developed,which uses the lower bound and the upper bound derived in the previous sections.The branching rule used is to select the job with the smallest lower bound in the set of jobs to be sequenced.In order to obtain a feasible solution quickly and to control the computer storage requirement,the search strategy used is depth first.It is worthwhile to note that with some simple modifications,the analysis results presented in Section 3can easily be extended to find a lower bound for the optimal objective value of Problem YCS with a given feasible partial sequence.For a given p q (q =1,2,...,n ),the sequence of the first q jobs,Procedure LB can easily be modified to find a lower bound for the sum of completion times from the (q +1)th jobto the n th jobb y replacing g 1ði Þ¼max t ½1 ;r i þh i ;d 0i þh i ÈÉby gq þ1ði Þ¼max t ½q þ1 ;r i þh i ;d ½q i þh i ÈÉand k =2b y k =q +2in Step 0.Likewise,with some min-or modifications on Lemma 6,the lemma can be used to test,for a given p q ,whether the optimal solution of the corresponding assignment problem gives the optimal sequence for the remaining (n Àq )jobs.If the optimal sequence is found,the node is fathomed.The example problem in Section 3is again used to illustrate the algorithm.The initial upper bound determined in Section 4is ing the lower bounding procedure in Section 3,the lower bound for the set of pending nodes in the tree can easily be found.Fig.3shows the solution tree of the problem.In the above figure,the number above a node is the lower bound found by the lower bounding method described in Section 3taking into account the scheduling decisions made up to that node,and an underlined number above a node indicates the case that the lower bound found is the same as the smallest total completion time attainable for the scheduling decisions made up to that node,i.e.the lower bounding method finds the optimal schedule along the branch leading to the node.The branch and bound algorithm stops when it finds that the lower bound of the branch 1–3–4。
中转港口集装箱堆场合理布局的模拟研究

【 关键词 】 码头; 堆场布局 ; 装卸效率; 箱区;l s T F xi e mC
集装箱堆 场是码 头的重要 资源 , 合理 的堆 场布局 软 件 中离 散事 件 系统 理论 建立 集 装箱 港 口系 统 中设 可 以提高堆场 机械 的提 箱效率 , 减少集 卡在堆 场的等 备 运动学模 型 ; U Z O等 研究集装 箱码头 的仿 MA RII 待时 间 , 而提高桥 吊装卸效 率 , 从 减少船舶在 码头的装 真型 , 给出组件架构并且用 Jv 给予实现 , aa 对提 出的模 卸时 间。 因此 , 如何对 码头有 限的堆场 面积进行有 效 型进行 校准和验 证 ;E E I P T R NG 研究码 头堆场 的箱 区 的分配 和管理 , 如何更 高效地 使用堆场现有资 源 , 进而 长度 和在 区域 内场桥数量 如何 影响桥 吊的长期平 均效 提高码头堆 场利用率和装卸效 率成为码头管 理和学术 率 , 虑到港 口实 际作业 的所 有机械 , 并考 运用系统 和离 界广泛关 注 的问题 。 文通过模 拟和仿 真的方式研 究 散方法 事件仿真 , 出最 优解 。 本 得 在既定 堆场面积 下 , 不同的箱 区长度 和堆 场龙 门吊布 置对桥 吊装卸效 率 的影 响。
I 工_ 哩 第 3 第1期 2 1 1 ‘ 苎 r , 3卷 1 0 年1月 1
中转港口集装Байду номын сангаас堆场合理布局的 模 拟 研 究
王 刁\ 变
( 海海事大 学科 学研 究院 , 上 上海 203 ) 015
【 摘 要 】 合理的堆场布局可以提 高码头机械的操作效率 , 为减少船舶等待 时间, 提升码头 综合竞 争力 , 通过建 立不 同箱 区长度 下的最 小化 堆场龙 门 吊数量模 型 , 出不 同箱 区长度 下的龙 得
《集卡调度下的集装箱码头场桥调度及堆场分配问题》范文

《集卡调度下的集装箱码头场桥调度及堆场分配问题》篇一一、引言随着全球贸易的不断发展,集装箱码头作为货物运输的关键节点,其作业效率与整体物流的顺畅性息息相关。
在集装箱码头作业中,场桥作为核心设备,负责集装箱的装卸与转运,而集卡则承担着集装箱在码头内部的运输任务。
本文将重点探讨集卡调度下的集装箱码头场桥调度及堆场分配问题,旨在提高码头作业效率,优化资源配置。
二、场桥调度问题及重要性场桥调度是集装箱码头作业中的关键环节,它涉及到集装箱的装卸、转运以及与集卡、堆场的协同作业。
合理的场桥调度能够确保集装箱的快速、准确转运,减少作业等待时间,提高码头的吞吐能力。
然而,由于码头作业环境的复杂性,如船舶靠泊时间的不确定性、集装箱数量的动态变化等,使得场桥调度成为一个具有挑战性的问题。
三、集卡调度与场桥调度的关系集卡作为码头内部的运输工具,其调度与场桥调度紧密相关。
集卡负责将集装箱从堆场运至场桥下进行装卸作业,因此集卡的调度效率直接影响到场桥的作业效率。
在集卡调度中,需要考虑的因素包括集卡的数量、行驶路径、与场桥的协同等。
通过合理的集卡调度,可以减少集卡的空驶和等待时间,提高码头的整体作业效率。
四、堆场分配问题及其影响堆场是集装箱码头的重要组成部分,用于存放待装卸的集装箱。
堆场分配是指根据集装箱的类型、目的地等信息,合理安排堆存位置。
合理的堆场分配能够提高码头的作业效率和资源利用率,减少场桥的作业距离和时间。
然而,由于码头作业的动态性和不确定性,堆场分配问题也具有一定的复杂性。
五、集卡调度下的场桥调度及堆场分配策略针对集卡调度下的场桥调度及堆场分配问题,本文提出以下策略:1. 优化集卡调度:通过智能调度系统,实时掌握集卡的数量、位置和状态信息,合理安排集卡的行驶路径和任务。
同时,采用先进的通讯技术,实现集卡与场桥、堆场之间的信息共享和协同作业。
2. 智能场桥调度:利用现代信息技术和人工智能算法,对场桥的作业任务进行智能分配和优化。
集装箱码头装船堆区场桥调度优化

集装箱码头装船堆区场桥调度优化
随着国际间贸易量的增多,以及船舶大型化趋势的加剧,集装箱码头正面临着更为严峻的竞争环境。
科学有效的安排场桥调度,可大幅度提升船舶的装卸效率,进而缩短船舶的在港时间,为港方带来经济效益的同时,提高货主及船方的满意度。
相比于客户的存取箱过程,船舶的装卸过程对场桥作业的要求更高,该部分的作业效率直接影响着整个码头的作业效能,一直以来都是学术界的热点话题。
因此,专门针对集装箱码头装船堆区场桥调度问题的研究具有很重要的现实意义和理论价值。
首先,本文对国内外研究现状进行详细论述,分析已有研究的不足,以集装箱码头装船堆区场桥调度问题作为本文的研究方向。
其次,考虑场桥在作业过程中需保持安全距离和不可跨越等现实约束,兼顾场桥在箱区间转场问题以及倒箱因素的影响,构建了以最小化带有惩罚因子的内集卡提箱时间偏差值为目标的非线性规划模型。
之后,基于所研究问题的特点,设计了改进模拟退化算法用于问题的求解。
最后,结合实地调查数据进行算例分析,给出了多台场桥在多个箱区内的具体调度方案;设计敏感性分析实验,分析了算例规模以及压箱量对本文算法的影响。
实验表明,本文方案可有效解决集装箱码头装船堆区场桥调度问题,验证了本文算法及模型的有效性。
基于混合遗传算法的混堆箱区内场桥调度研究
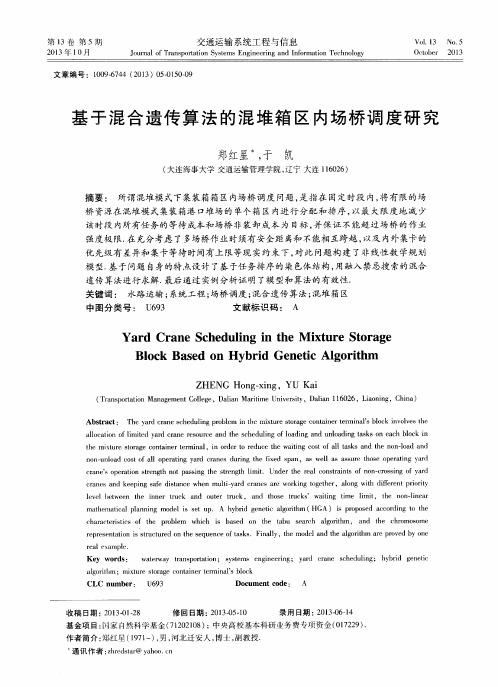
2 0 1 3 年 1 0 月
交通运输 系统 工程 与信息
J o u r n a l o f T r a n s p o r t a t i o n S y s t e ms E n g i n e e r i n g a n d I n f o r ma t i o n T e c h n o l o g y
强度极 限. 在 充分考 虑 了多场桥 作业 时须有 安 全距 离和 不 能相 互跨 越 , 以及 内外集卡 的 优 先级 有差 异和 集卡等待 时间有上 限等现 实约 束 下 , 对 此 问题 构 建 了非 线性数 学规 划 模型. 基 于问题 自身 的特 点设 计 了基 于任 务排序 的 染 色体 结构 , 用融入 禁忌搜 索的 混合 遗 传 算法进 行 求解. 最后 通过 实例 分析证 明 了模 型和 算 法的有效 性.
关 键词 : 水路运 输 ; 系统 工程 ; 场桥 调度 ; 混合 遗传 算法 ; 混堆 箱 区
中 图分 类号 : U 6 9 3 文 献标识 码S c h e d u l i n g i n t h e Mi x t ur e S t o r a g e Bl o c k Ba s e d o n Hy b r i d Ge n e t i c Al g o r i t h m
V【 ) 1 .1 3
No . 5
Oc t o b e r 2 01 3
文章编号 :1 0 0 9  ̄7 4 4( 2 0 1 3 )0 5 - 0 1 5 0 — 0 9
基 于 混合 遗 传算 法 的 混堆 箱 区 内场 桥 调 度研 究
郑红星 , 于 凯
( 大连海事大学 交通运输管理学院 , 辽宁 大连 1 1 6 0 2 6 )
- 1、下载文档前请自行甄别文档内容的完整性,平台不提供额外的编辑、内容补充、找答案等附加服务。
- 2、"仅部分预览"的文档,不可在线预览部分如存在完整性等问题,可反馈申请退款(可完整预览的文档不适用该条件!)。
- 3、如文档侵犯您的权益,请联系客服反馈,我们会尽快为您处理(人工客服工作时间:9:00-18:30)。
Dynamic crane deployment in container storage yardsChuqian Zhang a ,Yat-wah Wan a ,Jiyin Liua,*,Richard J.Linn b aDepartment of Industrial Engineering and Engineering Management,The Hong Kong University of Science and Technology,Clear Water Bay,Kowloon,Hong Kong b Department of Industrial and Systems Engineering,Florida International University,Miami,FL,USAReceived 7February 2000;received in revised form 2April 2001;accepted 9April 2001AbstractStorage yards at container terminals serve as temporary buffers for inbound and outbound containers.Rubber tyred gantry cranes (RTGCs)are the most frequently used equipment in yards for container han-dling.The efficiency of yard operations heavily depends on the productivity of these RTGCs.As the workload distribution in the yard changes over time,dynamic deployment of RTGCs among storage blocks (container stacking areas)is an important issue of terminal operations management.This paper addresses the crane deployment problem.Given the forecasted workload of each block in each period of a day,the objective is to find the times and routes of crane movements among blocks so that the total delayed workload in the yard is minimized.The problem is formulated as a mixed integer programming (MIP)model and solved by Lagrangean relaxation.To improve the performance of this approach,we augment the Lagrangean relaxation model by adding additional constraints and modify the solution procedure putational experiments show that the modified Lagrangean relaxation method generates excellent solutions in short time.Ó2002Elsevier Science Ltd.All rights reserved.Keywords:Container terminal;Crane deployment;Mixed integer programming;Lagrangean relaxation1.IntroductionA container terminal plays an important role in global manufacturing and international business by serving as a multi-modal interface,usually between the sea and land transports.Its three basic functions are as follows:(a)delivering containers to (carriers for)consignees and receiving containers from shippers,(b)loading containers onto and discharging containers from vessels,and (c)storing containers temporarily to account for the differences in arrival times ofthe Transportation Research Part B 36(2002)537–/locate/trb*Corresponding author.Tel.:+852-2358-7100;fax:+852-2358-0062.E-mail address:jyliu@ust.hk (J.Liu).0191-2615/02/$-see front matter Ó2002Elsevier Science Ltd.All rights reserved.PII:S0191-2615(01)00017-0sea and land carriers.A container terminal works under multiple operational objectives.Two main ones are to minimize the ship turnaround time and to maximize the terminal throughput.They can be achieved by efficient loading and discharging of vessels.Therefore,quay crane (QC)rate is usually taken as the key performance measure for the operational efficiency of a terminal.Let Q be the total number of QCs used in period [0,t ];S i and C i be the start time and the completion time of the i th QC,respectively;W be the total number of containers loaded and discharged in [0,t ].Then the QC rate in [0,t ]is W =P Q i ¼1ðC i ÀS i Þ.The operations of QCs,and hence the QC rate,are the results of several interrelated container flows in a container terminal.A schematic diagram of these flows is shown in Fig.1in which we assume a typical sea-land inter-modal interface.Without loss of generality,we take trucks as the means of land transport.Other means such as trains have similar processes.An inbound container is brought in by a vessel and is to be loaded on a truck,while an outbound container is brought in by a truck and is to be loaded onto a vessel.Both inbound and outbound flows take three handling stages in a terminal,namely the gatehouse ,the storage yard ,and the quayside .On the land side,external trucks (XTs)bring in outbound containers before the loading process of the relevant vessel,and they pick up inbound containers after the discharging process of the vessel.All XTs first go through the gatehouse for documentation and,if applicable,con-tainer inspection.After dropping offthe outbound containers and/or picking up the inbound containers in the yard,they leave the terminal through the gatehouse.On the quayside,vessels bring inbound containers to the terminal and leave with outbound containers on board according to vessel schedules.Each arriving vessel first docks at an assigned berth.Then it is discharged and loaded by QCs.The transportation of discharged inbound containers from the quayside to the storage yard and the transportation of outbound containers from the storage yard to the quayside for loading are carried out by internal trucks (ITs).At the storage blocks in the yard,yard cranes carry out all the container handling work which includes grounding (lifting containers from the trucks,ITs and XTs,and storing them at storage locations)and picking-up (retrieving containers from their storage locations and putting them on the trucks).Because yard cranes serve both ITs and XTs,they play a very important role in the storage yard and are usually the bottleneck in the container handling process.Without an effective operation planning of yard cranes,ITs may have to wait in the yard,and consequently QCs will be idle in waiting for ITs.Such waiting times reduce the QC rate.Therefore,to achieve high productivity,the use of yard cranes should be well planned.There are several kinds of common yard cranes.A rail-mounted gantry crane runs on rails with a single storage block between the rails.A rubber tyred gantry crane (RTGC)runs onrubber-tyre538 C.Zhang et al./Transportation Research Part B36(2002)537–555wheels and can move among storage blocks.See Fig.2in Section 2for an RTGC and a typical storage block.Other cranes such as straddle carriers which carry containers between their legs,mobile harbor cranes which are inherited from pre-containerized era,and heavy-duty forklift trucks which are more used for empty containers are used for different spatial-economical reasons.RTGCs are the most popular type of yard cranes at the container terminals in Hong Kong as well as in many other ports in the world.In this paper we focus on the deployment of RTGCs among blocks to minimize the total work delayed so as to raise the container handling efficiency.The rest of the paper is organized as follows:Section 2describes the dynamic crane deployment problem in detail.A literature review on previous work in the area of container terminal operation management is provided in Section 3.Section 4presents a mixed integer programming (MIP)model of the problem.In Section 5,the Lagrangean relaxation method is used to solve the model.Improvement measures are introduced to the standard procedure to reduce the large duality gap.Section 6reports the results of computational experiments on a large set of representative problem instances.Conclusions are drawn in Section 7.2.Problem descriptionThe container storage yard is used to temporarily store the inbound and outbound containers until they are picked up by trucks or loaded onto vessels.A large-scale yard may be divided into a number of large areas called zones .In each zone,containers are stacked side by side and one on top of the other to form rectangular shaped heaps called blocks .A typical block,as shown in Fig.2,may have 6lanes of containers placed side by side,5containers in height for each lane and usually more than 20containers in length,depending on the geographical shape of the storage yard.Containers’retrieval and stacking within each block are performed by RTGCs.Depending on the vessel schedule and the policy for container allocation in the yard,the workloads of blocks vary among blocks and with time.It is important that the workload can be finished within the planned time periods so that vessels can leave at their scheduled departure times.Since RTGCs are very expensive equipment,terminals usually cannot allocate and keepa C.Zhang et al./Transportation Research Part B36(2002)537–555539fixed number of RTGCs in each block.To fully utilize the RTGCs and overcome the workload imbalance among blocks,an RTGC needs to move to a block with heavy workload after finishing the light workload in its current block.The allocation and the movement of RTGCs among blocks are collectively called crane deployment .As shown in Fig.3,if two blocks are adjacent and align longitudinally,e.g.blocks B 1and B 2,an RTGC can move easily from one block to the other along a straight line without any turning.In other cases,e.g.,to move between blocks B 1and B 3,or between blocks B 1and B 4,an RTGC has to make a 90°turn (of its wheels)twice to move from one block to another.Since RTGCs are big in size and slow in motion,their movements occupy a large amount of road space for a long time,obstructing the traffic on the roads between blocks and delaying other terminal operations.Moreover,any block-to-block RTGC movement takes time,and will results in the loss in the productivity of cranes.Therefore,determining the optimal RTGC deployment among blocks is very critical to the efficiency of container handling operations in the storage yard.The container yard operates three shifts round the clock every day of the year.Each shift is further divided into two 4-h planning periods,leading to a total of six planning periods per day:00:00–4:00,4:00–8:00,8:00–12:00,12:00–16:00,16:00–20:00,and 20:00–24:00.Every evening,the fore-casted workloads in the block for the following day are assembled.The operating plan of RTGCs,including any possible deployment,is determined for each of the six planning periods.Such op-erating and deployment plans are made by crane operation supervisors based on their experience.Basically,given the forecasted workload in each block in each planning period,the supervisors make the following two decisions so as to minimize the total workload overflow between periods:(a)the times to deploy (move)each RTGC,and(b)the routes of RTGC movements (i.e.,the origin and the destination blocks of each RTGC)for each period of the planning horizon.While the supervisors have a good sense of the operations,the size of the deployment problem practically precludes any person from making sound decisions continuously.In our research,we formulate the problem as a mathematical program and provide suggestions for supervisors and terminal management to follow.3.Related workThe QC rate of a container terminal depends on the effective and efficient usage,including allocation and scheduling,of the expensive resources such as berths,QCs,storage space andyard540 C.Zhang et al./Transportation Research Part B36(2002)537–555C.Zhang et al./Transportation Research Part B36(2002)537–555541 cranes.Each of these resources plays an indispensable role in the interlocking processes in a container terminal.See Zhang(2000)for a comprehensive review of literature on various models and aspects of allocating and scheduling of these resources.Here,we only provide a brief review of existing studies related to terminal resources.Berths are essential resources of a terminal.Therefore,under heavy traffic of vessels,it is critical to allocate berths to vessels to avoid undue vessel delay.The berth allocation problem was studied by using non-linear integer programming model(Imai et al.,1997),modified time-space network (Chen and Hsieh,1999)and simulation(Lai and Shih,1992)to minimize the vessel berthing time and maximize berth utilization.QCs are the handling equipment at berths for discharging and loading berthed vessels.Allo-cation and scheduling of QCs directly affect the utilization of berths.Daganzo(1989)used an integer programming model and a simple scheduling strategy to solve the static QC scheduling problem with the objective of minimizing the aggregate vessel delay cost.Peterkofsky and Dag-anzo(1990)considered the problem as an‘‘open shop’’scheduling problem with parallel and identical machines(QCs).An integer programming model was built to minimize ship delay costs (weighted tardiness)and a branch and bound method is used to obtain an optimal solution.Based on the above results,Daganzo(1990)further studied the effect of QC scheduling strategies on terminal throughput and ship delay in the long run,assuming that the workload distribution(over time)for ships at berths is the same as the workload distribution for the ship’s population as a whole.Tabernacle(1995)applied learning concept to QC operations in order to improve the QC performance with time.The yard operation is the most complex part at a terminal because both inbound and out-bound containerflows are handled there simultaneously.Resource planning in the yard deter-mines the terminal efficiency to a great extent.There are two clear research topics related to yard operation:the assignment of storage locations to containers(i.e.,the grounding policy)and the allocation and scheduling of yard equipment.Grounding policy is a very critical issue that ul-timately determines the amount of workload in each storage block.Taleb-Ibrahimi et al.(1993) and De Castilho and Daganzo(1993)compared the amount of required storage space and the resulting workload under different grounding policies for export and import containers respec-tively.Kim and Kim(1994,1996),Kim and Park(1998)and Kim and Kim(1998)developed various mathematical programming and cost models tofind the grounding policy under various criteria.There has not been much research done on crane allocation and i and Lam (1994)used simulation to compare throughputs,equipment utilizations,and waiting times for several allocation strategies of yard equipment.Kim and Kim(1999)minimized the sum of the set up time and the travel time of cranes in a container storage block by modeling the process as a mixed integer program.There is also research that considers several operational decisions simultaneously.Kozan and Preston(1999)determined the optimal grounding policy and the corresponding crane schedule using an integer program to minimize the maximal working time among all yard cranes assigned to a vessel.Ballis and Abacoumkin(1996)built a simulation model that incorporates traffic congestion and delay due to mismatch of equipment.They used the model to evaluate yard layout,equipment amount,truck arrivals,and operations rules of a terminal.Kozan(1997a) studied the throughput of a train container terminal.He developed heuristics to assign trains to542 C.Zhang et al./Transportation Research Part B36(2002)537–555tracks and to assign cranes,forklifts,and highstacks to containers.An analytically based simu-lation was used to evaluate these heuristics for different performance measures.In another paper Kozan(1997b)modeled the operations as a batch-arrival multi-server queue and discussed the major factors affecting the transfer efficiency of container terminals.It is clear from the literature review that there have not been systematic studies on the RTGC deployment problem.Given the popularity of RTGCs in container terminals and their important role in the yard operation,effective RTGC deployment is very important for high terminal effi-ciency.In the following,we will develop an integer programming model to deploy RTGCs so that the total work delayed in the yard is minimized.Near-optimal solutions are then obtained through Lagrangean relaxation heuristic.4.Model development4.1.Modeling assumptionsWe make the following assumptions in our RTGC deployment model.All these assumptions are from observing the real-life practice.•The capacity of an RTGC is measured in crane minutes.All cranes have the same capacity of 240min in each4-h planning period.Similarly,the workload is also measured in crane minutes: Wefirst get the nominal number of container moves,which is the total number of containers to be discharged from and loaded onto vessels,and to be grounded from and picked up by XTs during each planning period.Since containers are stacked on each other and may be stored in a pre-defined pattern,each nominal container retrieval or storage may take more than one real crane move.So the workload,the total number of container moves,is converted into the work-load in crane minutes by multiplying the average number of real moves per nominal move with the average crane time needed per move.•Because of the limitation of block sizes and the potential danger of crane collision,each block holds at most two RTGCs at any time.With at most only two RTGCs per block,we do not allow more than one RTGC moving from one block to another in a period.•In practice,to minimize the loss of crane hours and the traffic blockage,inter-zone RTGC movements are carried out only once per day at midnight.Consequently,in our study of real-time crane deployment in day time,we do not need to consider any inter-zone moves but focus only on intra-zone moves within each24-h planning horizon.•Each RTGC is moved at most once in each4-h planning period.This is the trade offadopted in practice to avoid frequent RTGC moves that block traffic and lose crane hours on one hand and infrequent RTGC moves that waste surplus capacity on the other hand.•Any crane move starts andfinishes within the same planning period.•Unfinished work in a block at the end of a planning period will be carried over to the next pe-riod.As a result,the workload of a block in a planning period is the sum of the workload of the current period and the workload carried over from the previous planning period.The workload carried over from the previous period will befinished during the early part in the current pe-riod.We refer the unfinished workload in a period as‘‘delayed work’’.4.2.Crane deployment modelThe deployment decision process can be formulated as a MIP model to find the optimal movements of cranes and the timing of the movements in the yard (or a zone in a large-scale yard)during each planning period of a day.The following notations are used:X ii 0the number of cranes in block i at the beginning of the planning horizon,Cthe capacity of one crane within a planning period;C ¼240min,Nthe total number of blocks under consideration,Tthe total number of planning periods in a planning horizon;T ¼6periods,B itthe workload in block i within planning period t ,t ij the traveling time of a crane from block i to block j .The decision variables are defined as follows (Fig.4):X ijtthe number of cranes moving from block i to block j during planning period t (note that when i ¼j ,these cranes stay in the same block during period t ),Z ijtthe workload fulfilled in block i by cranes that move from block i to block j during planning period t (refer to Fig.4),Y ijtthe workload fulfilled in block j by cranes that move from block i to block j during planning period t (refer to Fig.4),W it the workload left in block i at the end of planning period t .The model is presented below,followed by a brief explanation.ðCD P ÞMin X T t ¼1X N i ¼1W it :Subject to:XN j ¼1X ijt ¼X N j ¼1X ji ðt À1Þfor i ¼1;2;...;N ;t ¼1;2;...;T ;ð1ÞXN j ¼1X ijt þX N j ¼1j ¼i X jit 62for i ¼1;2;...;N ;t ¼1;2;...;T ;ð2ÞC.Zhang et al./Transportation Research Part B36(2002)537–555543W i ðt À1ÞþB it ÀXN j ¼1Z ijtþX N j ¼1Y jit !ÀW it ¼0for i ¼1;2;...;N ;t ¼1;2;...;T ;ð3ÞZ ijt þY ijt 6ðC –t ij ÞX ijt for i ¼1;2;...;N ;j ¼1;2;...;N ;t ¼1;2;...;T ;ð4ÞW i 0¼0for i ¼1;2;...;N ;ð5ÞX ij 0¼0for i ¼1;2;...;N ;j ¼1;2;...;N ;i ¼j ;ð6ÞW it P 0;Z ijt P 0;Y ijt P 0for i ¼1;2;...;N ;j ¼1;2;...;N ;t ¼1;2;...;T ;ð7ÞX iit 2f 0;1;2g ;X ijt 2f 0;1gfor i ¼1;2;...;N ;j ¼1;2;...;N ;i ¼j ;t ¼1;2;...;T :ð8ÞAs stated in the assumptions,the workload within one planning period can be carried over to the next one if it cannot be finished.However,all jobs in a block have their own due times and ideally should always be completed on time.Although these due times are flexible to some extent,the work should not be delayed for long.Therefore,it is not appropriate to use only the workload left at the end of the last period,P N i ¼1W iT ,as the objective function of the model.Instead,a sum of workload left at the end of each planning period or the total amount of delayed work,P T t ¼1P N i ¼1W it ,is used as the objective function.Constraints (1)maintain the crane flow,or movement conservation in each block when cranes are deployed from one period to the next.Constraints (2)ensure that only two cranes can serve a block in a planning period.These constraints have the following implication:•If there are already two cranes in a block at the beginning of a planning period,no crane can be moved into the block even after one or both of the cranes are moved out during the period.•If there is only one crane in a block at the beginning of a planning period,at most one crane can be moved in from other blocks even after the original crane is moved out during the period.•If there is no crane in a block at the beginning of a planning period,then at most two cranes from other blocks can be moved in during the period.These modeling implications match the practical operations.In a terminal,cranes should finish the work in blocks where they start and then be moved out to help others.Therefore,if one or both cranes can be moved out to help other blocks’work,it is not necessary for other cranes to move in to help that block.Constraints (3)maintain the balance between the workload that should be finished (W i ðt À1ÞþB it )and the workload that can be finished ðP N j ¼1Z ijt þP N j ¼1Y jit Þin each block by using slack variables W it .Constraints (4)ensure that the total workload fulfilled by a crane in each planning period cannot exceed its total net crane capacity.Constraints (5)initialize workload at the be-ginning of a planning horizon;any delayed workload from the previous planning horizon is counted as the workload in the first planning period.Constraints (6),together with the parameters X ii 0,define the initial locations of the cranes.Constraints (7)and (8)are non-negative and integer constraints.Constraints (8)also restrict that at most one RTGC can be moved from one block to another in a period.Recall that X iit is the number of cranes staying in block i during the whole period t and therefore it my take a value of 2.544 C.Zhang et al./Transportation Research Part B36(2002)537–555For each crane,if the total workload that it fulfills in block i and j plus its transportation time between the two blocks is less than its capacity in a period,the crane will be in an idle state for some time within the period either in block i ,block j or both.Based on the definition of Z ijt and Y ijt as shown in Fig.4,the crane can be moved from block i to j at any time in the range ðZ ijt ;C ÀY ijt Àt ij Þ.grangean heuristicAs indicated in Section 4.2,the model is a mixed-integer programming model.A common way to solve such a problem is to relax some coupling constraints and decompose the problem into several sub-problems that are easy to solve.See Section 5.1for the application of the method to our problem.Lagrangean relaxation has been successfully applied to problems in different settings,for ex-amples,Holmberg and Hellstrand (1998),Luh and Hoitomt (1993),Nilsson and Sjelvgren (1996),Rana and Vickson (1991)and Kim and Park (1998).Although Lagrangean relaxation is a heu-ristic method and cannot guarantee optimal solutions in general,it has the advantage of identi-fying the bounds of the optimal solution as part of the solution.The procedure suggests a solution and gives a measure of how well that solution approximates the optimal solution (Pirkul and Schilling,1998).Readers interested in the detail of Lagrangean relaxation are invited to read Fisher (1981)and Geoffrion (1974).grangean relaxation of the problemThe Lagrangean relaxation method is a heuristic method that first dualizes one or more con-straints to produce a Lagrangean problem,then solves the Lagrangean dual with subgradient optimization and at last finds a primal feasible solution by eliminating the infeasibilities in the dual solution.The objective value J of any primal feasible solution is an upper bound of the optimal objective value J Ãwhile the corresponding optimal dual objective value L Ãis a lower bound of J Ã.The difference between J Ãand L Ãis known as the duality gap.An upper bound of the duality gap is provided by J ÀL Ã,and ðJ ÀL ÃÞ=L Ãis thus often used a measure of sub-optimality of the feasible solution with respect to the optimal one (Luh and Hoitomt,1993).An approach like this can be quite efficient for a problem with a special structure.Below we describe a pro-cedure to solve problem CD_P based on the approach.5.1.1.Formulation of the dual problemThe capacity constraints (4)which combine the continuous and integer variables in model CD_P are relaxed by using the non-negative Lagrangean multipliers p ijt to form the relaxed problem:ðCD D0ÞMin LSubject to ð1Þ–ð3Þand ð5Þ–ð8Þ;C.Zhang et al./Transportation Research Part B36(2002)537–555545Edited by Foxit Reader Copyright(C) by Foxit Corporation,2005-2010For Evaluation Only.whereL¼X Tt¼1X Ni¼1W itþX Ni¼1X Nj¼1X Tt¼1p ijt½ðZ ijtþY ijtÞÀðCÀt ijÞX ijt¼X Tt¼1X Ni¼1W itþX Ni¼1X Nj¼1X Tt¼1p ijtðZ ijtþY ijtÞÀX Ni¼1X Nj¼1X Tt¼1p ijtðCÀt ijÞX ijt:This leads to two decomposed sub-problems:ðCD D1ÞMin L1¼X Tt¼1X Ni¼1W itþX Ni¼1X Nj¼1X Tt¼1p ijtðZ ijtþY ijtÞSubject to constraintsð3Þ;ð5Þandð7Þ:ðCD D2ÞMin L2¼ÀX Ni¼1X Nj¼1X Tt¼1p ijtðCÀt ijÞX ijtSubject to constraintsð1Þ;ð2Þ;ð6Þandð8Þ:Problem CD_D1involves only continuous variables and can be solved easily.Problem CD_D2 involves integer variables but it has a structure similar to the networkflow problem and can also be solved easily.Thus,for a given set of Lagrangean multipliers p ijt,problems CD_D1and CD_D2can be solved accordingly.The Lagrangean dual problem is:ðCD DÞMaxp P0L with L¼MinðL1þL2Þ¼Min L1þMin L2¼LÃ1þLÃ2Subject toð1Þ–ð3Þandð5Þ–ð8Þ:5.1.2.Solving the dual problemSince the sub-problem involves discrete variables,the objective function in CD_D is a piecewise linear concave function and may not be differentiable at certain points in the p space(Fisher, 1981).A subgradient method is therefore used to solve the dual problem(Held et al.,1974; Polyak,1969).In the algorithm,the multiplier p is updated iteratively according top nþ1 ijt ¼p nijtþh n D nijt;where n is the iteration number index,D nijt is the subgradient of L in CD_D with respect top;D nijt ¼ðZ nijtþY nijtÞÀðCÀt ijÞX nijt,h n is the step size determined by the rule given in Polyak(1969).h n¼kJÃÀL nPi¼1Pj¼1Pt¼1ðD nijtÞ;ð9Þwhere JÃis the best primal feasible solution found up to iteration n,L n is the optimal dual ob-jective value at the n th iteration,k is a parameter used to adjust the step size and usually k¼2 initially.When the dual solution does not increase for certain number of iterations,k is reduced by half.As proved by Polyak(1969),this method ensures the convergence of the Lagrangean dual solution at a geometric rate.546 C.Zhang et al./Transportation Research Part B36(2002)537–555。