HYSTERETIC NOISE IN DC SQUIDS
噪音对海洋影响英语作文

噪音对海洋影响英语作文Title: The Impact of Noise Pollution on the Ocean。
The ocean, a vast expanse of water covering over 70% of our planet, is not as silent as one might think. In recent years, the issue of noise pollution in the ocean has gained increasing attention. This essay explores the various sources of oceanic noise pollution and its profound impact on marine life and ecosystems.To begin with, let us delve into the primary sources of noise pollution in the ocean. One major source is anthropogenic activities, including shipping, industrial operations, and naval exercises. The incessant hum of ship engines, the deafening blasts of underwater construction, and the sonar pings of military vessels all contribute to the cacophony beneath the waves. Additionally, offshore drilling and seismic surveys for oil and gas exploration emit intense bursts of noise, disrupting the marine environment.Furthermore, marine life itself can generatesignificant noise, particularly from marine mammals such as whales and dolphins. These creatures rely on sound for communication, navigation, and hunting. However, their vocalizations can be drowned out by human-generated noise, leading to communication breakdowns and behavioral changes.Now, let us explore the profound impact of noise pollution on marine life and ecosystems. The most evident effect is on marine mammals, whose sensitive hearing is vital for survival. Prolonged exposure to high-intensity noise can result in auditory damage, disorientation, and even strandings. Whales, in particular, have been known to alter their migration routes and feeding behaviors to avoid noisy areas.Moreover, noise pollution can disrupt the natural balance of marine ecosystems. For instance, certain fish species rely on sound for mating calls and territorial defense. Excessive noise can interfere with these crucial behaviors, leading to decreased reproductive success andpopulation decline. Additionally, noise can mask the sounds of prey and predators, affecting the dynamics of marinefood webs.Furthermore, noise pollution poses a significant threat to sensitive marine habitats, such as coral reefs and seagrass meadows. These ecosystems provide essential breeding grounds and nurseries for countless marine species. However, the relentless din of human activities can stress corals, impairing their growth and resilience. Similarly, noise can disturb the delicate equilibrium of seagrass ecosystems, affecting the abundance and diversity of associated marine life.In conclusion, noise pollution is a pervasive and detrimental threat to the health of the ocean and its inhabitants. From the rumble of ships to the roar ofoffshore drilling, human-generated noise permeates every corner of the marine environment. Its impacts are far-reaching, affecting marine mammals, fish, and delicate ecosystems alike. To mitigate the effects of noise pollution, concerted efforts are needed, including stricterregulations, technological innovations, and public awareness campaigns. Only by reducing our noise footprint can we ensure the tranquility and well-being of the ocean for generations to come.。
红外影像奇偶元条纹噪声自适应去除算法
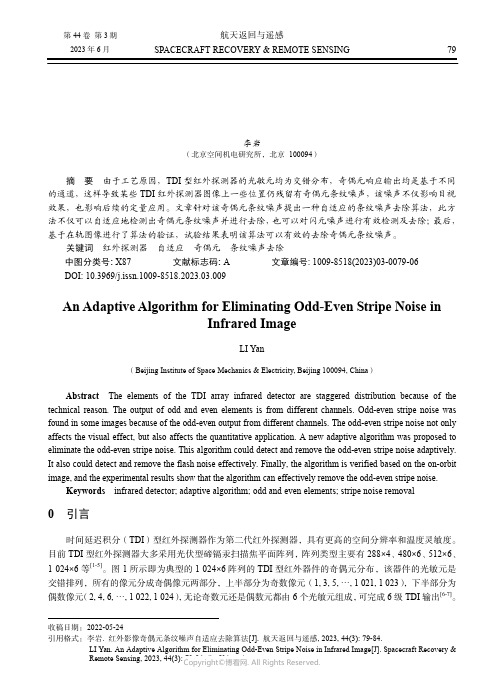
第44卷第3期航天返回与遥感2023年6月SPACECRAFT RECOVERY & REMOTE SENSING79红外影像奇偶元条纹噪声自适应去除算法李岩(北京空间机电研究所,北京100094)摘要由于工艺原因,TDI型红外探测器的光敏元均为交错分布,奇偶元响应输出均是基于不同的通道,这样导致某些TDI红外探测器图像上一些位置仍残留有奇偶元条纹噪声,该噪声不仅影响目视效果,也影响后续的定量应用。
文章针对该奇偶元条纹噪声提出一种自适应的条纹噪声去除算法,此方法不仅可以自适应地检测出奇偶元条纹噪声并进行去除,也可以对闪元噪声进行有效检测及去除;最后,基于在轨图像进行了算法的验证,试验结果表明该算法可以有效的去除奇偶元条纹噪声。
关键词红外探测器自适应奇偶元条纹噪声去除中图分类号: X87文献标志码: A 文章编号: 1009-8518(2023)03-0079-06DOI: 10.3969/j.issn.1009-8518.2023.03.009An Adaptive Algorithm for Eliminating Odd-Even Stripe Noise inInfrared ImageLI Yan(Beijing Institute of Space Mechanics & Electricity, Beijing 100094, China)Abstract The elements of the TDI array infrared detector are staggered distribution because of the technical reason. The output of odd and even elements is from different channels. Odd-even stripe noise was found in some images because of the odd-even output from different channels. The odd-even stripe noise not only affects the visual effect, but also affects the quantitative application. A new adaptive algorithm was proposed to eliminate the odd-even stripe noise. This algorithm could detect and remove the odd-even stripe noise adaptively. It also could detect and remove the flash noise effectively. Finally, the algorithm is verified based on the on-orbit image, and the experimental results show that the algorithm can effectively remove the odd-even stripe noise.Keywords infrared detector; adaptive algorithm; odd and even elements; stripe noise removal0 引言时间延迟积分(TDI)型红外探测器作为第二代红外探测器,具有更高的空间分辨率和温度灵敏度。
Common Phase Error due to Phase Noise in OFDM - Estimation and Suppression

COMMON PHASE ERROR DUE TO PHASE NOISE IN OFDM-ESTIMATION AND SUPPRESSIONDenis Petrovic,Wolfgang Rave and Gerhard FettweisV odafone Chair for Mobile Communications,Dresden University of Technology,Helmholtzstrasse18,Dresden,Germany{petrovic,rave,fettweis}@ifn.et.tu-dresden.deAbstract-Orthogonal frequency division multiplexing (OFDM)has already become a very attractive modulation scheme for many applications.Unfortunately OFDM is very sensitive to synchronization errors,one of them being phase noise,which is of great importance in modern WLAN systems which target high data rates and tend to use higher frequency bands because of the spectrum availability.In this paper we propose a linear Kalmanfilter as a means for tracking phase noise and its suppression.The algorithm is pilot based.The performance of the proposed method is investigated and compared with the performance of other known algorithms.Keywords-OFDM,Synchronization,Phase noise,WLANI.I NTRODUCTIONOFDM has been applied in a variety of digital commu-nications applications.It has been deployed in both wired systems(xDSL)and wireless LANs(IEEE802.11a).This is mainly due to the robustness to frequency selective fading. The basic principle of OFDM is to split a high data rate data stream into a number of lower rate streams which are transmitted simultaneously over a number of orthogonal subcarriers.However this most valuable feature,namely orthogonality between the carriers,is threatened by the presence of phase noise in oscillators.This is especially the case,if bandwidth efficient higher order modulations need to be employed or if the spacing between the carriers is to be reduced.To compensate for phase noise several methods have been proposed.These can be divided into time domain[1][2]and frequency domain approaches[3][4][5].In this paper we propose an algorithm for tracking the average phase noise offset also known as the common phase error(CPE)[6]in the frequency domain using a linear Kalmanfilter.Note that CPE estimation should be considered as afirst step within more sophisticated algorithms for phase noise suppression[5] which attempt to suppress also the intercarrier interference (ICI)due to phase noise.CPE compensation only,can however suffice for some system design scenarios to suppress phase noise to a satisfactory level.For these two reasons we consider CPE estimation as an important step for phase noise suppression.II.S YSTEM M ODELAn OFDM transmission system in the presence of phase noise is shown in Fig. 1.Since all phase noise sources can be mapped to the receiver side[7]we assume,without loss of generality that phase noise is present only at the front end of the receiver.Assuming perfect frequency and timing synchronization the received OFDM signal samples, sampled at frequency f s,in the presence of phase noise can be expressed as r(n)=(x(n) h(n))e jφ(n)+ξ(n).Each OFDM symbol is assumed to consist of a cyclic prefix of length N CP samples and N samples corresponding to the useful signal.The variables x(n),h(n)andφ(n)denote the samples of the transmitted signal,the channel impulse response and the phase noise process at the output of the mixer,respectively.The symbol stands for convolution. The termξ(n)represents AWGN noise with varianceσ2n. The phase noise processφ(t)is modelled as a Wiener process[8],the details of which are given below,with a certain3dB bandwidth∆f3dB.,0,1,2...m lX l=,0,1,2...m lR l=Fig.1Block diagram of an OFDM transmission chain.At the receiver after removing the N CP samples cor-responding to the cyclic prefix and taking the discrete Fourier transform(DFT)on the remaining N samples,the demodulated carrier amplitude R m,lkat subcarrier l k(l k= 0,1,...N−1)of the m th OFDM symbol is given as[4]:R m,lk=X m,lkH m,lkI m(0)+ζm,lk+ηm,lk(1)where X m,lk,H m,lkandηm,lkrepresent the transmitted symbol on subcarrier l k,the channel transfer function andlinearly transformed AWGN with unchanged variance σ2n at subcarrier l k ,respectively.The term ζm,l k represents intercarrier interference (ICI)due to phase noise and was shown to be a gaussian distributed,zero mean,randomvariable with variance σ2ICI =πN ∆f 3dB s[7].The term I m (0)also stems from phase noise.It does not depend on the subcarrier index and modifies all subcarriers of one OFDM symbol in the same manner.As its modulus is in addition very close to one [9],it can be seen as a symbol rotation in the complex plane.Thus it is referred to in the literature as the common phase error (CPE)[6].The constellation rotation due to CPE causes unaccept-able system performance [7].Acceptable performance can be achieved if one estimates I m (0)or its argument and compensates the effect of the CPE by derotating the received subcarrier symbols in the frequency domain (see Eq.(1)),which significantly reduces the error rate as compared to the case where no compensation is used.The problem of esti-mating the CPE was addressed by several authors [3][4][10].In [3]the authors concentrated on estimating the argument of I m (0)using a simple averaging over pilots.In [10]the argument of I m (0)was estimated using an extended Kalman filter,while in [4]the coefficient I m (0)itself was estimated using the LS algorithm.Here we introduce an alternative way for minimum mean square estimation (MMSE)[11]of I m (0)using a linear scalar Kalman filter.The algorithm is as [4]pilot based.III.P HASE N OISE M ODELFor our purposes we need to consider a discretized phase noise model φ(n )=φ(nT s )where n ∈N 0and T s =1/f s is the sampling period at the front end of the receiver.We adopt a Brownian motion model of the phase noise [8].The samples of the phase noise process are given as φ(n )=2πf c √cB (n )where f c is the carrier frequency,c =∆f 3dB /πf 2c [8]and B (n )represents the discretizied Brownian motion process,Using properties of the Brownian motion [12]the fol-lowing holds:B (0)=0and B (n +1)=B (n )+dB n ,n ∈N 0where each increment dB n is an independent random variable and dB n ∼√T s N (0,1).Noting that φ(n )=2πf c √cB (n )we can write the discrete time phase noise process equation asφ(n +1)=φ(n )+w (n )(2)where w (n )∼N (0,4π2f 2c cT s )is a gaussian randomvariable with zero mean and variance σ2w =4π2f 2c cT s .IV.CPE E STIMATION U SING A K ALMAN F ILTER Since all received subcarriers within one OFDM symbolare affected by the same factor,namely I m (0),the problem at hand can be seen as an example of estimating a constant from several noisy measurements given by Eq.(1)for which purpose a Kalman filter is well suited [11].For a Kalmanfilter to be used we need to define the state space model of the system.Define first the set L ={l 1,l 2,l 3,...l P }as a subset of the subcarrier set {0,1,...N −1}.Using Eq.(1)one can writeR m,l k =A m,l k I m,l k (0)+εm,l k(3)where A m,l k =X m,l k H m,l k and I m,l k (0)=I m (0)for all k =1,2...,P .Additional indexing of the CPE terms is done here only for convenience of notation.On the other hand one can writeI m,l k +1(0)=I m,l k (0).(4)Equations (3)and (4)are the measurement and processequation of the system state space model,where A m,l k represents the measurement matrix,while the process matrix is equal to 1and I m,l k (0)corresponds to the state of the system.The measuring noise is given by εm,l k which combines the ICI and AWGN terms in Eq.(1),the varianceof which for all l k equals σ2ε=(σ2ICI +σ2n ).The process noise equals zero.Note that the defined state space model is valid only for one OFDM symbol.For the state space model to be fully defined,knowledge of the A m,l k =X m,l k H m,l k is needed.Here we assume to have ideal knowledge of the channel.On the other hand we define the subset L to correspond to the pilot subcarrier locations within one OFDM symbol so that X m,q ,q ∈L are also known.We assume that at the beginning of each burst perfect timing and frequency synchronization is achieved,so that the phase error at the beginning of the burst equals zero.After the burst reception and demodulation,the demodulated symbols are one by one passed to the Kalman filter.For a Kalman filter initialization one needs for eachOFDM symbol an a priori value for ˆI m,l 1(0)and an a priori error variance K −m,1.At the beginning of the burst,when m =1,it is reasonable to adopt ˆI −1,l 1(0)=1.Within each OFDM symbol,say m th,the filter uses P received pilot subcarriers to recursively update the a priori value ˆI −1m,l 1(0).After all P pilot subcarriers are taken into account ˆI m,l P (0)is obtained,which is adopted as an estimate ofthe CPE within one OFDM symbol,denoted as ˆIm (0).The Kalman filter also provides an error variance of the estimateof I m,l P (0)as K m,P .ˆI m,l P(0)and K m,P are then used as a priori measures for the next OFDM symbol.The detailed structure of the algorithm is as follows.Step 1:InitializationˆI −m,l 1(0)=E {I −m,l 1(0)}=ˆI m −1(0)K −m,1=E {|I m (0)−ˆIm −1(0)|2}∼=E {|φm −ˆφm −1|2}=σ2CP E +K m −1,Pwhere σ2CP E =4π2N 2+13N +N CP ∆f 3dBf s(see [10]),K 0,P =0and φm =arg {I m (0)}.Repeat Step2and Step3for k=1,2,...,P Step2:a-posteriori estimation(update)G m,k=K−m,kH H m,lkH m,lkK−m,kH Hm,l k+(σ2ICI+σ2n)ˆIm,l k (0)=ˆI−m,l k(0)+G m,k[R m,lk−H m,l kˆI−m,l k(0)]K m,k=(1−G m,k H m,lk )K−m,kStep3:State and error variance propagationK−m,k+1=K m,k(5)ˆI−m,l k+1(0)=ˆI m,lk(0)Note that no matrix inversions are required,since the state space model is purely scalar.V.CPE C ORRECTIONThe easiest approach for CPE correction is to derotate all subcarriers l k of the received m th symbol R m,lkby φm=−arg{ˆI m(0)}.Unambiguity of the arg{·}function plays here no role since any unambiguity which is a multiple of2πrotates the constellation to its equivalent position in terms of its argument.The presented Kalmanfilter estimation algorithm is read-ily applicable for the decision feedback(DF)type of algo-rithm presented in[4].The idea there was to use the data symbols demodulated after thefirst CPE correction in a DFE manner to improve the quality of the estimate since that is increasing the number of observations of the quantity we want to estimate.In our case that would mean that after thefirst CPE correction the set L={l1,l2,l3,...l P}of the subcarriers used for CPE estimation,which previously corresponded to pilot subcarriers,is now extended to a larger set corresponding to all or some of the demodulated symbols. In this paper we have extended the set to all demodulated symbols.The Kalmanfilter estimation is then applied in an unchanged form for a larger set L.VI.N UMERICAL R ESULTSThe performance of the proposed algorithm is investigated and compared with the proposal of[4]which is shown to outperform other known approaches.The system model is according to the IEEE802.11a standard,where64-QAM modulation is used.We investigate the performance in AWGN channels and frequency selective channels using as an example the ETSI HiperLAN A-Channel(ETSI A). Transmission of10OFDM symbols per burst is assumed.A.Properties of an EstimatorThe quality of an estimation is investigated in terms of the mean square error(MSE)of the estimator for a range of phase noise bandwidths∆f3dB∈[10÷800]Hz.Table1 can be used to relate the phase noise bandwidth with other quantities.Figures2and3compare the MSE of the LS estimator from[4]and our approach for two channel types and both standard correction and using decision feedback. Note that SNRs are chosen such that the BER of a coded system after the Viterbi algorithm in case of phase noise free transmission is around1·10−4.Kalmanfilter shows better performance in all cases and seems to be more effective for small phase noise bandwidths. As expected when DF is used the MSE of an estimator is smaller because we are taking more measurements into account.Fig.2MSE of an estimator for AWGN channel.Fig.3MSE of an estimator for ETSI A channel.Table 1Useful relationsQuantitySymbolRelationTypical values for IEEE802.11aOscillator constant c [1radHz]8.2·10−19÷4.7·10−18Oscillator 3dB bandwidth ∆f 3dB [Hz]∆f 3dB =πf 2cc 70÷400Relative 3dB bandwidth ∆f 3dB ∆f car∆f 3dBfsN 2·10−4÷13·10−4Phase noise energy E PN [rad]E PN =4π∆f 3dB∆fcar0.0028÷0.016Subcarrier spacing∆f car∆f car =f s N312500HzB.Symbol Error Rate DegradationSymbol error rate (SER)degradation due to phase noise is investigated also for a range of phase noise bandwidths ∆f 3dB ∈[10÷800]Hz and compared for different correc-tion algorithms.Ideal CPE correction corresponds to the case when genie CPE values are available.In all cases simpleconstellation derotation with φ=−arg {ˆIm (0)}is used.Fig.4SER degradation for AWGN channel.In Figs.4and 5SER degradation for AWGN and ETSI A channels is plotted,respectively.It is interesting to note that as opposed to the ETSI A channel case in AWGN channel there is a gap between the ideal CPE and both correction approaches.This can be explained if we go back to Eq.(1)where we have seen that phase noise affects the constellation as additive noise.Estimation error of phase noise affects the constellation also in an additive manner.On the other hand the SER curve without phase noise in the AWGN case is much steeper than the corresponding one for the ETSI A channel.A small SNR degradation due to estimation errors will cause therefore large SER variations.This explains why the performance differs much less in the ETSI A channel case.Generally from this discussion a conclusion can be drawn that systems with large order of diversity are more sensitive to CPE estimation errors.Note that this ismeantFig.5SER degradation for ETSI A channel.not in terms of frequency diversity but the SER vs.SNR having closely exponential dependence.It can be seen that our approach shows slightly better performance than [4]especially for small phase noise bandwidths.What is also interesting to note is,that DF is not necessary in the case of ETSI A types of channels (small slope of SER vs.SNR)while in case of AWGN (large slope)it brings performance improvement.VII.C ONCLUSIONSWe investigated the application of a linear Kalman filter as a means for tracking phase noise and its suppression.The proposed algorithm is of low complexity and its performance was studied in terms of the mean square error (MSE)of an estimator and SER degradation.The performance of an algorithm is compared with other algorithms showing equivalent and in some cases better performance.R EFERENCES[1]R.A.Casas,S.Biracree,and A.Youtz,“Time DomainPhase Noise Correction for OFDM Signals,”IEEE Trans.on Broadcasting ,vol.48,no.3,2002.[2]M.S.El-Tanany,Y.Wu,and L.Hazy,“Analytical Mod-eling and Simulation of Phase Noise Interference in OFDM-based Digital Television Terrestial Broadcast-ing Systems,”IEEE Trans.on Broadcasting,vol.47, no.3,2001.[3]P.Robertson and S.Kaiser,“Analysis of the effects ofphase noise in OFDM systems,”in Proc.ICC,1995.[4]S.Wu and Y.Bar-Ness,“A Phase Noise SuppressionAlgorithm for OFDM-Based WLANs,”IEEE Commu-nications Letters,vol.44,May1998.[5]D.Petrovic,W.Rave,and G.Fettweis,“Phase NoiseSuppression in OFDM including Intercarrier Interfer-ence,”in Proc.Intl.OFDM Workshop(InOWo)03, pp.219–224,2003.[6]A.Armada,“Understanding the Effects of PhaseNoise in Orthogonal Frequency Division Multiplexing (OFDM),”IEEE Trans.on Broadcasting,vol.47,no.2, 2001.[7]E.Costa and S.Pupolin,“M-QAM-OFDM SystemPerformance in the Presence of a Nonlinear Amplifier and Phase Noise,”IEEE mun.,vol.50, no.3,2002.[8]A.Demir,A.Mehrotra,and J.Roychowdhury,“PhaseNoise in Oscillators:A Unifying Theory and Numerical Methods for Characterisation,”IEEE Trans.Circuits Syst.I,vol.47,May2000.[9]S.Wu and Y.Bar-ness,“Performance Analysis of theEffect of Phase Noise in OFDM Systems,”in IEEE 7th ISSSTA,2002.[10]D.Petrovic,W.Rave,and G.Fettweis,“Phase NoiseSuppression in OFDM using a Kalman Filter,”in Proc.WPMC,2003.[11]S.M.Kay,Fundamentals of Statistical Signal Process-ing vol.1.Prentice-Hall,1998.[12]D.J.Higham,“An Algorithmic Introduction to Numer-ical Simulation of Stochastic Differential Equations,”SIAM Review,vol.43,no.3,pp.525–546,2001.。
噪声相关英文文献
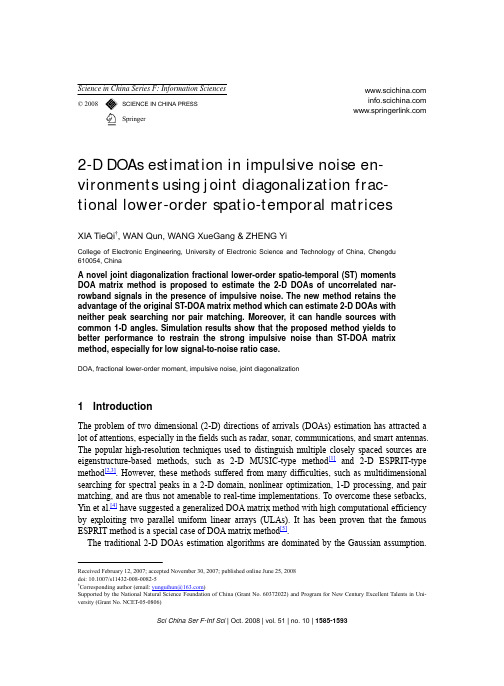
2008 Received February 12, 2007; accepted November 30, 2007; published online June 25, 2008doi: 10.1007/s11432-008-0082-5†Corresponding author (email: yunguihun@ )Supported by the National Natural Science Foundation of China (Grant No. 60372022) and Program for New Century Excellent Talents in Uni-versity (Grant No. NCET-05-0806)Sci China Ser F-Inf Sci | Oct. 2008 | vol. 51 | no. 10 | 1585-1593 eigenstructure-based methods, such as 2-D MUSIC-type method [1] and 2-D ESPRIT-type ESPRIT method is a special case of DOA matrix method [5].Unfortunately, there are also many phenomena in signal processing which are decidedly non-Gaussian [6―13], such as atmospheric noise, urban radio channels, man-made signals, and so on. Recently, it has been shown that impulsive noise can be modeled as a complex symmetric α-stable(S αS) process. Since S αS does not possess finite variance when 2α<, minimum dis-persion criterion is a good choice to evaluate the S αS process. Lv et al.[6,7] have proposed several methods using cross-covariation matrix to estimate 2-D DOAs of the signals in the presence of impulsive noise.In some applications, useful information can be obtained by introducing time domain process-ing [8,9,14―16]. He et al.[8,9] presented a DOA estimation method in impulsive noise environments using fractional lower-order spatio-temporal (FLOST) matrix. Jin [15,16] proposed a spatio-tempo- ral DOA matrix (ST-DOA) algorithm which takes advantages of the a priori in time domain. How- ever, not only 2-D ESPRIT method [2,6], but also DOA matrix method [4,5,7] and ST-DOA matrix method [15,16] cannot estimate signals with common 1-D angles or in some curved plane, which degrades the estimation performance of those algorithms.In this paper, we proposed a novel joint diagonalization FLOST matrix method (JD-FLOM- ST-DOA). The method can obtain the 2-D DOAs of the array based on joint diagonalization di-rectly with neither peak searching nor pair matching. Moreover, compared with ST-DOA matrix method, the significances of the novel algorithms are as follows: 1) it can work in impulsive noise environments; 2) it can estimate signals with common 1-D angles in any plane. Simulation results show the effectiveness of the proposed method.2 Complex S αS random variables [11]A complex random variable is S αS if and are joint S αS, and then their characteristic function is written as1j X X X =+2⎤1X 2X (1)122*11221122,12(){exp[j ()]}{exp[j()]}exp ||d (,),X X S E X E X X x x x x αϕωωωωωωΓ=+⎡=−+⎢⎥⎣⎦∫R where 12,j ωωω=+ is the real part operator, and is a symmetric measure on theunit sphere , called the spectral measure of the random variable The characteristic expo-nent is restricted to the values []i R 12,X X Γ2S .X 02,α<≤ and it determines the shape of the distribution. The smaller the characteristic exponent α, the heavier the tails of the density.A complex random variable 1j 2X X X =+ is isotropic if and only if hasa uniform spectral measure. In this case, the characteristic function of X can be written as12(,)X X (2)*(){exp[j ()]}exp(||),E X αϕωωγω==−R where (0)γγ> is the dispersion of the distribution. The dispersion plays a role analogous to the role that the variance plays for the second-order processes. Namely, it determines the spread of the probability density function around the origin. A method for generating complex isotropic S αS random variables is given in ref. [11]. Several in-phase components of the time series with different characteristic exponents are given in Figure 1, which shows the impulsiveness of the S αS distribution.1586 XIA TieQi et al. Sci China Ser F-Inf Sci | Oct. 2008 | vol. 51 | no. 10 | 1585-1593Figure 1 Time series of S αS random variables.A complex isotropic S αS random variable X has finite fractional lower-order moments (FLOMs) 2,p α<≤ i.e., {||},p E X <∞ 2.p α∀<≤ Obviously, S αS signals are of infinite variance because their second-order moments are infinite. The FLOM between ξ and η is defined as in ref. [10]:12*[,]{}{||},12,p p f E E p ξηξηξηηα−−==<<≤ (3) where, 1*||,p p ηηη−= and the superscript * denotes complex conjugate.3 Array configuration and signal model3.1 Assumptions and data modelConsider a uniform linear array consisting of M -element as shown in Figure 2. The spacing be-tween the first M −1 sensors is d x while the spacing between the first and the M th sensor is d y . Assume that there are D narrowband independent signals () (1,2,,)k s t k D =… with common carrier impinging on the array from 2-D directions (,).k k θβ As made in refs. [8,9], we assume the signal vector s (t ) satisfying2T H 1{s()[(|s()|)s ()]}diag[(),,()],p D E t t t τρτρτ−+= … (4) where superscript H denotes complex conjugate transpose, denotes Hadamard product (ele-ment-by-element product), and diag[]⋅ is the diagonal matrix formed with the elements of its vector valued argument. Eq. (4) means that the signals () (1,2,,)k s t k D =… are mutuallyFLOST uncorrelated. denotes the auto-FLOST moment of2*()[()|()|()]p k k k k E s t s t s t ρττ−=+().k s t The baseband signals of the t th snapshot of the array output measured by the array can be expressed asXIA TieQi et al. Sci China Ser F-Inf Sci | Oct. 2008 | vol. 51 | no. 10 | 1585-1593 158712π()()exp j (1)cos()(), 1,,1,Di k x k i k x t s t i d n t i M θλ=⎡⎤=−+=⎢⎥⎣⎦∑…− (5) 12π()()exp j cos()().D M k y k M k x t s t d n βλ=⎡⎤=⎢⎥⎣⎦∑t +(6)Figure 2 Array configuration for independent 2-D DOAs estimation.Write eqs. (5) and (6) into matrix form, and we have()()(),t t =+x As n t T D(7)T T 111[(),,()], ()[(),,()], [(),,()],M M x t x t t n t n t s t s t ===......x n s T 11[,,,,], [,,,,],k D k k ik Mk a a a ==............A a a a a (8)2πexp j (1)cos(), 1,,1,ik x k a i d i θλ⎡⎤M =−=⎢⎥⎣⎦…− (9) 2πexp j cos(),Mk y k a d βλ⎡⎤=⎢⎥⎣⎦(10) where is additive uniform complex isotropic S αS noise with dispersion γ, independent of the signals, i.e.,()(1,2,...,)i n t i M = 2T H {()[(|()|)()]}(),p M E t t t τ−+ n n n I γδτ= (11) where ()δτ is Kronecker function, and M I is an M M × dimensional identity matrix.3.2 FLOM-ST-DOA matrix method Under the above assumptions, we have the following array outputs of FLOST moments:2**1()[(),()][()|()|()][()],(1,2,,,0,,,,,,i M k k p x x i M f i M M D),s s Mk ik s s s s k R x t x t E x t x t x t R a a i M NT T T NT ττττττ−==+=+==≠=−−∑ (12)where 2*()[(),()][()|()|()].k k p s s k k f k k kR s t s t E s t s t s t τττ−=+=+ 2****1()[(),()][()|()|()][()], (0,1,2,,,1,2,,,1).i l k k p x x i l f i l l Dlks s Mk ik k Mk R x t x t E x t x t x t a R a a i M l L L M a τττττ−==+=+=≠==∑……=− (13)1588 XIA TieQi et al. Sci China Ser F-Inf Sci | Oct. 2008 | vol. 51 | no. 10 | 1585-1593Let (),X τR ()l Y R τ and (),S τR respectively, be1T ()[(),,(),,()],M i M M M X x x x x x x R R R ττττ=……R (14)1T ()[(),,(),,()],l l i l M l Y x x x x x x R R R ττττ=……R (15)11**1()[(),,(),,()].k k D D S s s M s s Mk s s MD R a R a R a ττττ=⋅⋅⋅……R *T (16)Write eqs. (12) and (13) into matrix form,()(),X S ττ=R AR (17)()(),l Y l S ττ=R A R Φ (18) where is a D ×D dimensional matrix,l Φ 11***1***1j2π/[cos (1)cos ]j2π/[cos (1)cos ]j2π/[cos (1)cos ]j2π/[cos (1)cos ]1diag ,,,,diag{e ,,e ,, e ,,e }diag[,,,,x y x p y p x q y q x D y D l lk lD l M Mk MD d d l d d l d d l d d l l gl a a a a a a λβθλβθλβθλβθφφ−−−−−−−−⎡⎤=⎢⎥⎣⎦==………………Φ,,], 1.ql Dl g q D φφ≠...≤≤ (19)By collecting the “pseudo snapshots” at 2N lags ,,,,,,s s s s NT T T NT τ=−−…… the “pseudo snapshots” data matrices are formed as follows:[(),,(),(),,()],X s X s X s X s NT T T NT =−−……X R R R R[(),,(),(),,()],l l l l l Y s Y s Y s Y s NT T T NT =−−……Y R R R R[(),,(),(),,()].S s S s S s S s NT T T NT =−−……S R R R R Then, eqs. (17) and (18) can be rewritten into,=X AS (20). (21) l l =Y A S ΦDefine FLOM-ST-DOA matrix as(22) †[],l TS l =⋅R Y X where denotes pseudoinverse, i.e.,†[]X†H H [][].1−=X X XX (23)Theorem 1. FLOM-ST-DOA matrix algorithm: if A and S is nonsingular and has un-equal elements, then the FLOM-ST-DOA matrix has its D non-zero eigenvalues equal to the D diagonal elements of and the corresponding eigenvectors equal to the D column vec-tors of matrix A , i.e.,l Φl TS R l Φ.l TS l =R A A Φ (24)The proof is similar to that given in ref. [5]. By eigendecomposition, we have A and . Then, the l Φk θ’s are obtained using the first M −1 elements of according to eq. (9), while (1)k D ≤≤k a k β’s are given by the M th element of according to eq. (10). The above theorem means that an estimate can be obtained if and only if there exists a matrix , which has (1)k D ≤≤k a l Φ XIA TieQi et al. Sci China Ser F-Inf Sci | Oct. 2008 | vol. 51 | no. 10 | 1585-1593 1589unequal entries. However, it is easy to construct examples where each l Φ(1)l L ≤≤ has a de-generate eigenvalue spectrum, and then the FLOM-ST-DOA matrix algorithm will fail. In the next section, we will propose an improved robust JD-FLOM-ST-DOA matrix algorithm to over-come this problem.4 JD-FLOM-ST-DOA matrix mehtod4.1 WhiteningThe first step of our JD-FLOM-ST-DOA matrix algorithm procedure consists of whitening the signal part of the pseudo-observation. This is achieved by applying a whitening matrix W to them, i.e., an ()X s nT R M D × matrix verifying the following:H H H H H [()()]X s X s X E nT nT .==WR R W WQ W WAA W I =,, (25)where and we assume thatH [()()]X X s X s E nT nT =Q R R H[()()](1/2)S S E N ττ=R R H ,0()()N S s S s n N n nT nT =−≠=∑R R Iand ()S τR has unit variance so that the dynamic range of ()S τR is accounted for by the mag-nitude of the corresponding column of A , which does not affect the estimation of the 2-D DOAs. Eq. (25) shows that if W is a whitening matrix, then is a WA D D × dimensional unitary ma-trix. It follows that for any whitening matrix W , there exists a unitary matrix U such that . As a consequence, matrix A can be factored as=WA U (26) †.=A W U Note that this whitening procedure reduces the determination of the M D × dimensional mixture matrix A to that of a unitary D D × dimensional matrix U . The whitened process still obeys a linear model:(27) ()(),X X n =z WR n n H H ..}, (28) ()().l l Y Y n =z WR Define the following cross-correlation matrix between and()l Y n z (),X n z (29)H H H H H [()()][()()][()()]l l l Y X Y X Y X l S S l E n n E n n E ττ====G z z WR R W WA R R A W U U ΦΦ4.2 Determining the unitary factor UThe second step of our JD-FLOM-STDOA matrix algorithm procedure is to determine a unitary factor U , which is obtained by performing a joint diagonalization of the combined set of The essential uniqueness of joint diagonalization is guaranteed by Theorem 2.1{,,,,}l L Y X Y X Y X= ……G G G G Theorem 2. Essential uniqueness of joint diagonalization: let be a set of L matrices where, for matrix is in the form withU a uni- tary matrix. Any joint diagonalizer of is essentially equal (the definition is given in ref. 1{,,,,l L Y X Y X Y X= ……G G G G 1l L ≤≤l Y X G H l U U Φ G [14]) 1590 XIA TieQi et al. Sci China Ser F-Inf Sci | Oct. 2008 | vol. 51 | no. 10 | 1585-1593to U if and only if1, , 1, pl ql p q D l l L .φφ∀≠∃≠≤≤≤≤ (30)The essential uniqueness condition (30) is of course much weaker than the requirement thatthere should exist a matrix in which is uniquely unitarily diagonalizable. In particular, it is easy to construct examples, where each matrix in has a degenerate eigenvalue spectrum but such that the joint diagonalizer of is nonetheless essentially unique. The proof of Theorem 2 is similar to the proof given in ref. G GG [14].Theorem 3. Sufficiency condition: if then (,)[0,π)[0,π),k k αβ∈×, 1,l l L ∃≤≤1∀≤. ,p q D ≠≤pl ql φφ≠Proof. If for the DOAs of the g th source (,)[0,π)[0,π),k k αβ∈×(,)g g αβ and q th source (,),q q αβ ,g q ≠ there exist two cases:1) if ,p q ββ≠ then 11;p q φφ≠2) if ,p q ββ=,p q αα≠ then ,pl ql φφ≠2l L ≤≤.Theorem 3 means that there exists at least one matrix satisfies (1)l l L ≤≤Φ.gl ql φφ≠Then, matrix A can be obtained by eq. (26) and the 2-D DOAs can be estimated according to eqs.(9) and (10).4.3 Implementation of the JD-FLOM-ST-DOA matrix methodBased on the previous sections, we can introduce a 2-D DOAs estimation method based on FLOST processing. The JD-FLOM-ST-DOA matrix method is defined by the following imple-mentation:1) Estimate the FLOM-ST matrix of the array outputs according to eqs. (13) and (14).2) Form the new pseudo-observation vectors X and .l Y 3) Estimate the sample covariance from the X Q 2M N × pseudo-observation X . Denote 1,,,D λλ… the D largest eigenvalues and 1,,D …h h the corresponding eigenvectors of As .X Q 0,τ≠ the whitening matrix W is formed by1/21/2H 11[,,D D λλ−−=…W h h ].4) Form the cross-correlation matrix according to eq. (29).l Y X G (1)l L ≤≤5) A unitary matrix U is then obtained as joint diagonalizer of the set{|1,,}.l Y X l L =…G 6) The matrix A is estimated as then the 2-D DOAs can be estimated according to eqs. (9) and (10).†,=A W U 5 Simulation results and performance analysisExample 1. Assume the three narrowband signals impinge from directions (40°, 50°), (55°, 80°), and (70°, 65°). We assume that α is already known, in practice, it can be estimated by some algorithms [13]. Simulation results are also compared with those of the ST-DOA matrix method [15]. α=1.4, p =1.1, M =6, d x =d y =λ/2. T = 500, 2N = 500([250,1]n ∈−−∪[1,250]). The performance of the estimators is obtained from 300 Monte-Carlo simulations, by calculating the RMSEs of the XIA TieQi et al. Sci China Ser F-Inf Sci | Oct. 2008 | vol. 51 | no. 10 | 1585-1593 1591DOA estimates. The RMSE is defined as RMSE(,)k k θβ= and GSNR is defined as 21GSNR 10log[(|()|)/].Tt s t T γ==∑ Figure 3 shows the RMSEs in degrees of the estimates of the three signals versus GSNR. We can see that the robustness is increased with our JD-FLOM-ST-DOA matrix method at low GSNRs.Example 2. In this example, the DOA parameters of the three signals are the same as Exam-ple 1. We fix GSNR=10 dB, p =1.05. The number of snapshots is T = 400, 2N = 400 The performance of the estimators is obtained from 300 Monte-Carlo simulations, by calculating the RMSEs of the DOA estimates. The RMSEs of the estimates of the three signals versus α are shown in Figure 3. We can see that the robustness is increased with our JD-FLOM-ST-DOA matrix method in strong impulsive noise environments.([200,1][1,200]).n ∈−−∪Example 3. Assume the three signals that impinge from the directions (59°, 59°), (70°, 80°), and (80°, 80°). Note that in this case for each l Φ(1,2,3)l = has a degenerate eigenvalue spec-trum. α=1.5, p =1.1, M =4, T = 450, 2N = 450([225,1][1,225]),n ∈−−∪ GSNR=15 dB. To obtain a measure of statistical repeatability, we make 100 Monte-Carlo simulations. Figure 4 shows that the FLOM-ST-DOA matrix algorithm can only estimate one of the three signals because the other two signals have a degenerate eigenvalue spectrum, but JD-FLOM-ST-DOA matrix method can estimate three signals successfully for its integration of the information in the three FLOM-ST-DOA matrices.Figure 3 RMSEs for the three signals versus GSNR. Figure 4 RMSEs for the three signals versus α. 6 ConclusionA novel 2-D DOAs estimation method based on joint diagonalization FLOST matrices is pro-posed, which makes full use of the data in time domain, as well as in spatial domain, to define generalized FLOST matrices. Theoretical analysis and simulation results show that the method is robust against S αS noise and it remedies the lack of the traditional subspace-based techniques employing second-order or higher-order moments cannot be applied in impulsive noise environ-ments. The method retains the advantage of the original ST-DOA matrix method which can esti- mate 2-D DOAs with neither peak searching nor pair matching. Moreover, it can estimate sources 1592 XIA TieQi et al. Sci China Ser F-Inf Sci | Oct. 2008 | vol. 51 | no. 10 | 1585-1593Figure 5Scatter plot of the three signals. (a) ST-DOA matrix method, l=1; (b) ST-DOA matrix method, l=2; (c) ST-DOA matrix method, l=3; (d) JD-FLOM-ST-DOA matrix method.with common 1-D angles in any plane, which outperforms the original ST-DOA matrix method significantly.1 Chan A Y J, Litva J. MUSIC and maximum likelihood techniques on two-dimensional DOA estimation with uniform cir-cular array. IEE P-Radar Son Nav, 1995, 3(142): 105―114 [DOI]2 Kedia V S, Chandna B. A new algorithm for 2-D DOA estimation. Signal Process, 1997, 60(3): 325―332 [DOI]3 Wang J Y, Chen T Q. Joint frequency, 2D AOA and polarization estimation using fourth-order cumulants. Sci China SerE-Tech Sci, 2000, 43(3): 297―3034 Yin Q Y, Zou L H, Newcomb R. A high resolution approach to 2-D signal parameter estimation-DOA matrix method. JChina I Commun (in Chinese), 1991, 4(12): 1―65 Yin Q Y. High Resolution Direction of arrival estimation. Doctor Dissertation. Xi’an: Xi’an Jiao Tong University (in Chi-nese), 19896 Lv Z J, Xiao X C. A novel method for estimating 2-D DOA in the presence of impulsive noise. J Electron Inf Tech (inChinese), 2004(3): 350―3567 Lv Z J,Xiao X C. A covariation-based algorithm for estimating 2-D DOA in the presence of impulsive noise. Acta Acust(in Chinese), 2004(2): 149―1548 Lv Z J, Xiao X C. A subspace-based direction finding algorithm using temporal and spatial processing of time lag frac-tional order correlation function. Signal Process (in Chinese), 2003, 2(19): 51―549 He J, Liu Z. DOA estimation in impulsive noise environments using fractional lower order spatial-temporal matrix. ActaAeronaut Astronaut Sin (in Chinese), 2006, 1(27): 104―10810 Liu T H, Mendel J M. A subspace-based direction finding algorithm using fractional lower order statistics. IEEE Trans Sig-nal Process, 2001, 8(49): 1606―161311 Tsakalides P, Nikias C L. The robust covariation-based MUSIC (ROC-MUSIC) algorithm for bearing estimation in impul-sive noise environments. IEEE Trans Signal Process, 1996, 7(44): 1623―1633 [DOI]12 Szajnowski W J, Wynne J B. Simulation of dependent samples of symmetric alpha-stable clutter. IEEE Trans Signal Proc-ess lett, 2001, 5(8): 151―152 [DOI]13 Tsihrintzis G A, Nikias C L. Fast estimation of the parameters of alpha-stable impulsive interference. IEEE Trans SignalProcess, 1996, 6(44): 1492―1503 [DOI]14 Belouchrani A, Abed-Meraim K, Cardoso J F, et al. A blind source separation technique using second-order statistics. IEEETrans Signal Process, 1997, 2(45): 434―444 [DOI]15 Jin L, Yin Q Y. Space-time DOA matrix method. Acta Electron Sin (in Chinese), 2000, 7(28): 8―1216 Jin L, Yin Q Y. Analysis and generalization of space-time DOA matrix method. Acta Electron Sin (in Chinese), 2001, 3(39):XIA TieQi et al. Sci China Ser F-Inf Sci | Oct. 2008 | vol. 51 | no. 10 | 1585-15931593300―3031594XIA TieQi et al. Sci China Ser F-Inf Sci | Oct. 2008 | vol. 51 | no. 10 | 1585-1593。
SQUID(超导量子干涉仪)
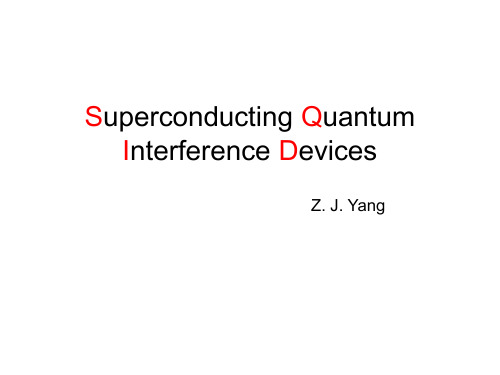
x y
Supercon.2 Ψ2
2qB0 d
z
y )(d t 2a)
2qB0 d y) I c sin qB0 dLy sin(2 1 )
Isolator
dx
Ly
2 Ly 2
dy jc sin(2 1
qB0 dLy
sin(2 1 ) 1 I达到极值,
超导中的磁通量子化
超导体内js=0, Cooper对波函数相位满足
1 2m 2eA [ js 2eA] ns e
取一个回路,作环路积分
.dl
2e
A.dl
2e
B
黄昆《固体物理学》
超导中没有磁场,这就是通过环孔 的磁通量,左端为一周的相位增加, 为使波函数回到同一点不会发生实 质变化于是 B 2n
ns 2 ei2代入,经处理得
j 2q
ns1 4qk ns1 ns 2 sin(2 1 ) jc sin t
在外加电压为零时,有一超导电流,其数 值由位相差决定。 V
交流约瑟夫森效应
Supercon.1 Supercon.2
Ψ1
Ψ2
若在结的两侧加上电压,则方程变为 1 i qV 1 k 2 t
c
b
A.dl J .dl
b
c
2 d c 2 0
代入并整理后得
d
c
A.dl
2 a d 0
a
d
A.dl J .dl
d
a
2 1 2n
i 2 I C cos(
0
热噪声在引力波探测中的影响

Overview
Motivation of accurate thermal noise models for Advanced Virgo: Required as input for important design decisions Required as input for sensitivity optimization Current status of the Advanced Virgo GWINC model: Documentation, availability, history… Coating Brownian Current implementation Beam sizes and asymmetric ROCs Test mass shape? Substrate Brownian Thermo-optic Examples of recent investigations Thermal noise issues in case of non-degenerate recycling cavities. Sensitivity improvement from application of LG modes My personal wish list for the future
Stefan Hild ET/ADV WP2, Rome, February 2009 Slide 6
Optimization Parameter 1: Signal-Recycling (de)tuning
Advanced Virgo, Power = 125W, SR-transmittance = 4%
Stefan Hild
ET/ADV WP2, Rome, February 2009
电化学噪声的分析与应用__电化学噪声的分析原理

收稿日期:2000 10 21;修订日期:2000 11 28基金项目:国家重点基础研究规划基金资助项目(G1*******),国家自然科学基金(50071054)作者简介:张鉴清,男,1948年生,教授,博士生导师,研究方向为电化学电化学噪声的分析与应用.电化学噪声的分析原理张鉴清1,2 张昭1 王建明1 曹楚南1,2(1 浙江大学化学系杭州310027)(2 金属腐蚀与防护国家重点实验室沈阳110016)摘要:概述了电化学噪声的产生机理、分类方法及电化学噪声技术相对于其它研究手段的优良特性.介绍了电化学噪声的测量方法及测量过程中的注意事项,重点讨论了电化学噪声的数据处理技术(时域分析、频域分析和电化学发射光谱等),比较了它们各自的优缺点.并根据目前电化学噪声技术分析中所存在的问题,提出了今后的研究及应用方向.关键词:电化学噪声 噪声分类 产生机理 数据处理中图分类号:O646;T G174.3 文献标识码:A 文章编号:1005 4537(2001)05 0310 111前言电化学噪声(Electrochemical noise,简称EN)是指电化学动力系统演化过程中,其电学状态参量(如:电极电位、外测电流密度等)的随机非平衡波动现象[1,2].B.A.T a 等1967年首先注意到了这个现象[3],之后,电化学噪声技术作为一门新兴的实验手段在腐蚀与防护科学领域得到了长期的发展[4~11].电化学噪声的起因很多,常见的有腐蚀电极局部阴阳极反应活性的变化、环境温度的改变、腐蚀电极表面钝化膜的破坏与修复、扩散层厚度的改变、表面膜层的剥离及电极表面气泡的产生等[1,12~20].迄今为止,已有很多技术用于表征电极的界面状态,但是它们都存在着各自不同的缺陷.例如:基于真空技术的低能电子衍射法(LEED)和俄歇电子能谱法(AES)等以及基于电磁波原理的椭圆偏光法(Ellipsometry)和扩展X-射线吸收精细结构技术(EXAFS)等诸多光学方法都不能对电极腐蚀现象进行原位观察[15,21~25];基于对研究电极施加外界扰动信号的极化曲线法等传统电化学研究方法则可能因为外加信号的介入而影响腐蚀电极的腐蚀过程,同样无法对被测体系进行原位监测[26,27].而电化学噪声技术相对于诸多传统的腐蚀监测技术(如:重量法、容量法、极化曲线法和电化学阻抗谱等)具有明显的优良特性.首先,它是一种原位无损的监测技术,在测量过程中无须对被测电极施加可能改变腐蚀电极腐蚀过程的外界扰动[28~31];其次,它无须预先建立被测体系的电极过程模型[26];第三,它无须满足阻纳的三个基本条件;最后,检测设备简单,且可以实现远距离监测[27,32].2电化学噪声的分类根据所检测到的电学信号视电流或电压信号的不同,可将电化学噪声分为电流噪声或电第21卷第5期2001年10月 中国腐蚀与防护学报Journal of C hinese Society for Corrosion and Protection Vol 21N o 5Oct 2001压噪声[33~36].根据噪声的来源不同又可将其分为热噪声、散粒效应噪声和闪烁噪声[1,29,37,38]:(1)热噪声是由自由电子的随机热运动引起的,是最常见的一类噪声.电子的随机热运动带来一个大小和方向都不确定的随机电流,它们流过导体则产生随机的电压波动.但在没有外加电场存在的情况下,这些随机波动信号的净结果为零.1928年贝尔实验室的J.B.Johnson 首先对热噪声进行了详细地实验研究(所以热噪声又称为约翰逊噪声),之后,H.Nyquist 根据热力学原理在理论上对其进行了大量探讨.实验与理论结果表明,电阻中热噪声电压的均方值E [V 2N ]正比于其本身的阻值大小(R )及体系的绝对温度(T ):E [V 2N ]=4K B TR !∀(1)式中,V 是噪声电位值,!∀是频带宽,K B 是Boltzmann 常数[K B = 1.38!(-23)J/K].上式在直到1013H z 频率范围内都有效,超过此频率范围后量子力学效应开始起作用.此时,功率谱将按量子理论预测的规律而衰减.热噪声的谱功率密度一般很小,例如,1M #的电阻在室温298K 时所产生的热噪声的谱功率密度的最大值仅为0.0169∃V 2/Hz.因此,在一般情况下,在电化学噪声的测量过程中,热噪声的影响可以忽略不计.热噪声值决定了待测体系的待测噪声的下限值,因此当后者小于监测电路的热噪声时,就必须采用前置信号放大器对被测体系的被测信号进行放大处理.(2)散粒效应噪声是Schottky 于1918年研究此类噪声时,用子弹射入靶子时所产生的噪声命名的.因此,它又称为散弹噪声或颗粒噪声.在电化学研究中,当电流流过被测体系时,如果被测体系的局部平衡仍没有被破坏,此时被测体系的散粒效应噪声可以忽略不计.然而,在实际工作中,特别当被测体系为腐蚀体系时,由于腐蚀电极存在着局部阴阳极反应,整个腐蚀电极的Gibbs 自由能!G 为:!G =-(E a +E c )zF =-E 外测zF (2)式中,E c 和E a 为局部阴阳极的电极电位,E 外测为被测电极的外测电极电位,z 为局部阴阳极反应所交换的电子数,F 为Faraday 常数.所以,即使外测E 外测或流过被测体系的电流很小甚至为零,腐蚀电极的散粒效应噪声也决不能忽略不计.散粒噪声类似于温控二极管中由阴极发射而达到阳极的电子在阳极所产生的噪声.Schottky 从理论上证明了该噪声符合下列公式:E [I 2N ]=2eI 0!∀(3)式中,e 为电子电荷,等于1.59!(-19)C,I 0为平均电流.在电化学研究中,e 应该用q 代替,而q 是远大于电子电荷的电量.例如,在单晶Ag 的电结晶过程中,q 相当于在基体表面上生长一单层Ag 所需的电荷;在电极腐蚀过程中,q 相当于一个孔蚀的产生或单位钝化膜的破坏所消耗的电量.公式(3)在频率小于100MH z 的范围内成立.热噪声和散粒噪声均为高斯型白噪声,它们主要影响频域谱中SPD 曲线的水平部分.(3)闪烁噪声又称为1/f %噪声,%一般为1、2、4,也有取6或更大值的情况.与散粒噪声一样,它同样与流过被测体系的电流有关、与腐蚀电极的局部阴阳极反应有关;所不同的是引起散粒噪声的局部阴阳极反应所产生的能量耗散掉了,且E 外测表现为零或稳定值[26],而对应于闪烁噪声的E 外测则表现为具有各种瞬态过程的变量[39,40].局部腐蚀(如孔蚀)能显著地改变腐蚀电极上局部微区的阳极反应电阻值,从而导致E a 的剧烈变化.因此,当电极发生局部腐蚀时,如果在开路电位下测定腐蚀电极的电化学噪声,则电极电位会发生负移,之后伴随着电3115期张鉴清等:电化学噪声的分析与应用 .电化学噪声的分析原理极局部腐蚀部位的修复而正移;如果在恒压情况下测定,则在电流-时间曲线上有一个正的脉冲尖峰.关于电化学体系中闪烁噪声的产生机理有很多假说,如∀时间常数假说#和∀渗透理论假说#等,但迄今能为大多数人接受的只有∀钝化膜破坏/修复#假说.该假说认为:钝化膜本身就是一种半导体,其中必然存在着位错、缺陷、晶体不均匀及其它一些与表面状态有关的不规则因素,从而导致通过这层膜的阳极腐蚀电流的随机非平衡波动,于是导致电化学体系中产生了类似半导体中1/f%噪声[3].闪烁噪声主要影响频域谱中SPD曲线的高频(线性)倾斜部分.3电化学噪声的测定电化学噪声的测定可以在恒电位极化或在电极开路电位的情况下进行[28,41].当在开路电位下测定EN时,检测系统一般采用双电极体系,它又可以分为两种方式:同种电极系统和异种电极系统:(1)传统测试方法一般采用异种电极系统,即一个研究电极和一个参比电极.参比电极一般为饱和甘汞电极(SCE)或Pt电极,也有采用其它形式的参比电极的(如Ag-AgCl参比电极等)[42-47].电化学噪声用参比电极的选择原则为:除了符合作为参比电极的一般要求以外,还要满足电阻小(以减少外界干扰)、电位稳定和噪声低等要求[1,44].(2)同种电极测试系统是近年才发展起来的,它的研究电极与参比电极均为被研究的材料[48,49].研究表明:电极面积影响噪声电阻,采用具有不同研究面积的同种电极系统测定体系的电化学噪声时有利于获取电极过程的机理[27].当在恒电位极化的情况下测定EN时,一般采用三电极测试系统.在双电极测试系统的基础上外加一个辅助电极给研究电极提供恒压极化.测试系统应置于屏蔽相中,以减少外界干扰[3].应采用无信号漂移的低噪声前置放大器,特别是其本身的闪烁噪声应该很小,否则将极大程度地限制仪器在低频部分的分辨能力[1].4电化学噪声的分析4 1频域分析电化学噪声技术发展的初期主要采用频谱变换的方法处理噪声数据,即将电流或电位随时间变化的规律(时域谱)通过某种技术转变为功率密度谱(SPD)曲线(频域谱),然后根据SPD曲线的水平部分的高度(白噪声水平)、曲线转折点的频率(转折频率)、曲线倾斜部分的斜率和曲线没入基底水平的频率(截止频率)等SPD曲线的特征参数来表征噪声的特性,探寻电极过程的规律.常见的时频转换技术有快速傅立叶变换(Fast Fourier Transform,FFT)、最大熵值法(M ax imum Entropy M ethod,MEM)、小波变换(Wavelets T ransform,WT).特别是其中的小波变换,它是傅立叶变换的重要发展,既保留了傅氏变换的优点又能克服其不足.因此,它代表了电化学噪声数据时频转换技术的发展方向.在进行噪声的时频转换之前应剔除噪声的直流部分,否则SPD曲线的各个特征将变得模糊不清,影响分析结果的可靠性.(1)傅立叶变换(FFT)[15,50,51]傅立叶变换是时频变换最常用的方法.假设信号为s(t),则由该信号经Fourier变换后得到频谱s(&)=1∃s(t)e-j&t d t,及其相应的能量密度频谱(频率密度)P(&)=|s(&)|2.根312中国腐蚀与防护学报第21卷据信号瞬变过程的不同特征,s (t)有不同的表达形式,从而得到具有不同噪声指数%的1/f a 噪声.(2)最大熵值法(MEM)[52~55]M EM 频谱分析法是J.P.Burg 于1967年提出来的.之后,coss 等又从数学的角度对它进行了详细地讨论,他们认为MEM 频谱分析法相对于其它频谱分析法(如FFT)具有很多优点:(a)对于某一特定的时间序列而言,M EM 在时间(空间)域上具有较高的分辨率;(b)M EM 特别适用于分析有限时间序列的特征,无须假定该时间序列是周期性的或假定有限时间序列之外的所有数据均为零.根据MEM 的原理,某一有限时间序列的功率P E 为:P E =p !t E * E *式中, =col (1 r 1 r 2%%r n -1);!t 为采样周期;E =col (1 e j ( e j 2(%%e j ((N-1);p 和r i 由式R *=P 迭代得到,式中P 为列矩阵P =col (p 0 0 0 0 0),R 为过程的N !N 自相关矩阵.通过FFT 和M EM 转换得到的SPD 曲线的特征参数(白噪声水平W 、高频线性部分的斜率k 、截止频率f c ),在一定程度上能较好地反映腐蚀电极的腐蚀情况,但并不能在整个腐蚀过程中很好地描述腐蚀过程的规律[56].为此,浙江大学张鉴清课题组综合SPD 曲线的各个特征参数,推导出了两个准数S E 和S G .其中,S E 的大小正比于采样时间内噪声的最大幅值和分布的非对称程度,而S G 的作用仍在进一步地研究中[57].(3)小波分析(FWT )[58~63]1984年,法国地球物理学家J.Morlet 在分析地震波的局部性质时,发现存在着传统的Fourier 变换难以达到的要求,因此他引入小波概念于信号分析中对信号进行分解[63].随后,理论物理学家A.Grossman 对Morlet 的这种信号按一个确定函数的伸缩,平移系|a |-1/2)x -b a:a,b &R ,a ∋0展开的可行性进行了研究,这为小波分析的形成开了先河[64];1984年A.Grossm an 和J.M orlet 又共同引入了积分小波变换IWT (Integral Wavelet T ransform)[65].IWT 具有所谓变焦距性质,它对于只在瞬间出现的高频信号具有很窄的时间窗口;而在低频段,具有很宽的时间窗口.严格地说,小波(母函数))(t)是指满足一定条件的且具有零均值的窗函数:∃+(-()^(&)2|&|-1d &<+( ()^(&)指)(t)的傅立叶变换)(4)由此,小波母函数通过平移和伸缩而得到的连续小波函数族)a,b(t)为:)a,b(t )=|a |-1/2)x-b a a,b &R ,a ∋0(5)于是,对于某一信号f (t),以小波)(t)作为窗函数的小波变换定义为:W )f (b,a)=1a ∃+(-(f (t )) x -b a d t )∗)a,b +f , a,b &R ,a ∋0(6)式(6)亦称为f (t )的连续小波变换.其中a 和b 分别称为伸缩平移因子, )表示)的复共轭.由式(6)可知,小波变换已将函数f (t )窗口化,中心在t 0=b,宽度为2a !)−,于是得到f (t )的时 频(t -&)局部化;其在(t -&)平面上的时频窗口为:[(7)3135期张鉴清等:电化学噪声的分析与应用 .电化学噪声的分析原理式中,!^)为!)的傅立叶变换,它也为一个窗函数;而!)可表示为:!)=∃+(-((t -t 0)2)(t )2d t 1/2∃+(-()(t )2d t(8) 通过小波变换后,可以得到电化学噪声的时频相平面图.它以时间为横轴,归一化为1.纵轴为尺度变量的倒数的对数值(代表频率).尺度较小时,时频相平面图左右两端的阴影部分为边缘效应,此处结果不正确;当尺度较大时,只含几个频率成分,随着放大倍数的增加,噪声信号中所包含的频率成分也增多,并显现出复杂的分岔结构,最后出现无限多个周期,进入混沌状态.从大尺度周期状态到小尺度混沌状态只要几次分岔即可达到.另外,在上述时频相平面图中还存在着一种∀自相似#的分形结构,由此可以推测出,在金属的腐蚀过程中,其状态参量的演化具有一种∀混沌吸引子#的结构[66,67],相关问题的研究仍待进一步地深入.通过对电化学数据的频域分析可以得到一些电极过程信息,如腐蚀类型、腐蚀倾向等.但是,很难得到腐蚀速率的确切大小,并且许多有用信息在变换过程中消失了[52,55].同时,由于目前仪器的限制(采样点数少、采样频率低),进一步阻碍了频域分析技术的应用.谱噪声电阻(Spectral Noise Impedance,R 0sn )是利用频域分析技术处理电化学噪声数据时引入的一个新的统计概念,它是F.M ansfeld 和H.Xiao 于1993年研究铁的电化学噪声的特征时首先提出来的[31,48,56,68].F.M ansfeld 和H.Xiao 认为:分别测定相同电极体系的电位和电流噪声后,将其分别进行时频转换,得到相应于每一个频率下的谱噪声响应R sn (Spectral NoiseResponse):R sn(f)=V fft(f)I fft(f)1/2.而谱噪声电阻R 0sn 被定义为R sn 在频率趋于零时的极限值R 0sn =lim f .0R sn(f) (9)一般认为R 0sn 的大小正比于电极反应电阻R p [31,56].在EN 的频域分析中,还可以将频域分析技术与分形理论结合起来进行研究,从而从更深层次上去探寻电化学噪声的本质[69].4 2时域分析由于仪器的缺陷(采样点数少、采样频率低等)和时频转换技术本身的不足(如:转换过程中某些有用信息的丢失、难于得到确切的电极反应速率等),一方面迫使电化学工作者不断探索新的数据处理手段,以便利用电化学噪声频域分析的优势来研究电极过程机理;另一方面又将人们的注意力部分转移到时域谱的分析上,从最原始的数据中归纳出电极过程的一级信息.在电化学噪声时域分析中,标准偏差(Standard Deviation)S 、噪声电阻R n 和孔蚀指标PI 等是最常用的几个基本概念,它们也是评价腐蚀类型与腐蚀速率大小的依据:(1)标准偏差又分为电流和电位的标准偏差两种,它们分别与电极过程中电流或电位的瞬时(离散)值和平均值所构成的偏差成正比[25]:S =!n i=1x i -!ni=1x i /n 2/(n -1)(10)式中,x i 为实测电流或电位的瞬态值,n 为采样点数.对于腐蚀研究来说,一般认为随着腐蚀速率的增加,电流噪声的标准偏差S I 随之增加,而电位噪声的标准偏差S V 随之减少.(2)孔蚀指标PI 被定义为电流噪声的标准偏差S I 与电流的均方根(Root Mean Square)I RMS 的比值[25,56]:314 中国腐蚀与防护学报第21卷PI =S I /I RMS (11)一般认为,PI 取值接近1.0时,表明孔蚀的产生;当PI 值处于0.1~ 1.0之间时,预示着局部腐蚀的发生;PI 值接近于零则意味着电极表面出现均匀腐蚀或保持钝化状态.另外,也有不少作者对PI 的作用提出了质疑.(3)噪声电阻R n [31,56,70]的概念是Eden 于1986年提出来的.之后,F.M ansfeld,H.Xiao 和G.Gusmano 等学者从实验室论证了它们之间的一致性;J.F.Chen 和W.F.Bogaerts 等学者则根据Butter-Volmer 方程从理论上证明了噪声电阻与线性极化电阻R P 的一致性,其证明的前提条件为:(a)阴阳极反应均为活化控制,(b)研究电极电位远离阴阳极反应的平衡电位,(c)阴阳极反应处于稳态.噪声电阻被定义为电位噪声与电流噪声的标准偏差比值,即R n =S V /S I(12)R n 与R sn 之间存在着内在的联系[50,68].Gordon P.Bierw agen 从物理学原理出发,导出了另一个噪声电阻的概念,但有的学者对公式推导的严谨性提出了质疑[71].(4)Hurst 指数(H)[69,72,73]是E.H.Hurst 于1956年采用标度变换技术(R/S)研究分维Brow nian 运动(fBm )的时间序列时提出来的.之后,E.H.H urst 与L.T.Fan 和B.B.Mandel brot 等学者先后独立提出时间序列的极差R (t,s)与标准偏差S (t,s)之间存在着下列关系:R (t,s )/S (t,s )=S H 0<H <1(13)式中下标t 为选定的取样时间,s 为时间序列的随机步长(某种微观长度),H 为Hurst 指数.H 与闪烁噪声1/f %的噪声指数%之间存在着%=2H +1的函数关系;同时,H 的大小反映了时间序列变化的趋势.一般而言,当H >1/2时,时间序列的变化具有持久性,而当H <1/2时,时间序列的变化具有反持久性,当H =1/2时,时间序列的变化表现为白噪声且增量是平稳的(在频域分析中,H 也可以由频域谱求出).另外,根据分形理论可知,时间序列的局部分维D fl 与Hurst 指数H 之间存在着下列关系,即:D fl =2-H (0<H <1).D fl 的越大,特别是系统的局部分维D fl 与系统的拓扑维数D t 之差(D fl -D t )越大,则系统的非规则性越强,说明电极过程进行得越剧烈.(5)非对称度Sk 和突出度Ku [30],Sk 是信号分布对称性的一种量度,它的定义如下:S k =1(N -1)S 3!N i=1(I i -I mean )3(14)Sk 指明了信号变化的方向及信号瞬变过程所跨越的时间长度.如果信号时间序列包含了一些变化快且变化幅值大的尖峰信号,则Sk 的方向正好与信号尖峰的方向相反;如果信号峰的持续时间长,则信号的平均值朝着尖峰信号的大小方向移动,因此Sk 值减小;Sk =0,则表明信号时间序列在信号平均值周围对称分布.K u 值给出了信号在平均值周围分布范围的宽窄、指明了信号峰的数目多少及瞬变信号变化的剧烈程度.K u >0表明信号时间序列是多峰分布的,K u =0或K u <0则表明信号在平均值周围很窄的范围内分布,当时间序列服从Gaussian 分布时,K u =3,如果K u >3,则信号的分布峰比Gaussian 分布峰尖窄,反之亦然.K u 可用下式表达:K u =1(N -1)S 4!N i=1(I i -I mean )4(15)在电化学噪声的时域分析中,除了上述方法外,应用得较多的还有统计直方图(Histogram Representation),它分为两种.第一种统计直方图是以事件发生的强度为横坐标,以事件发生3155期张鉴清等:电化学噪声的分析与应用 .电化学噪声的分析原理的次数为纵坐标所构成的直观分布图[70].实验表明,当腐蚀电极处于钝态时,统计直方图上只有一个正态(Gaussian)分布;而当电极发生孔蚀时,该图上出现双峰分布.另一种是以事件发生的次数或事件发生过程的进行速度为纵坐标,以随机时间步长为横坐标所构成[29,74].该图能在某一个给定的频率(如取样频率)将噪声的统计特性定量化.4 3电化学发射光谱法(EES)电化学发射光谱(EES)[26]是在传统的电化学噪声测试技术基础上发展起来的一种新方法.该方法采用三电极体系(参比电极、工作电极和微阴极),其中微阴极应该足够小,以致于工作电极的腐蚀情况不会因为该工作电极与微阴极组成回路的原因而产生变化.根据Butter-Volmer方程可导出:A C,k+1=!I k+1!V k+1=I k+1-I kV k+1-V k=2 303I corr,kb a+I c o rr,k-I kb c(16)式(16)中的I k和V k分别为k时刻的噪声电流和电压;I corr,k为k时刻工作电极的腐蚀电流;A C,k+1是k+1时刻腐蚀电极的导纳;b c和b a分别为工作电极阴阳极反应的Tafel斜率.如果I corr,k∀I k,则式(16)可以进一步简化.由式(16)求出的A C,k+1不仅可以用来计算均匀腐蚀的腐蚀速率,而且可用于区分均匀腐蚀与局部腐蚀.如果工作电极发生均匀腐蚀,则A C,k+1>0;如果工作电极发生局部腐蚀,则A C,k+1<0.K.H abib于2000年在EES技术的基础上提出了改进的电化学发射光谱方法(Modified Electrochemical Em ission Spectroscopy,MEES),实际上只是改用光学方法测定腐蚀电流,而其它方面与EES完全一致[74].即在MEES方法中,工作电极的腐蚀电流I corr,k的测定不是采用传统的零电阻安培计,而是采用光学腐蚀仪:I corr,k=F|Z|duMT(17)式(17)中I corr,k为k时刻的腐蚀电流,F为Faraday常数,|Z|为电子转移数,M为组成工作电极材料的原子的原子量,T是测定工作电极时阳极电流流过的时间,d是工作电极材料的密度,u为电极材料的光学参数.5电化学噪声技术的发展展望从1967年提出电化学噪声的概念以来,电化学噪声技术得到了迅速地发展.然而,迄今为止,它的产生机理仍不完全清楚、它的处理方法仍存在欠缺.因此,寻求更先进的数据解析方法已成为当前电化学噪声技术的一个关键问题.另外,结合当今微观世界的最新研究成果来分析电化学噪声的产生机理,以及结合非线性数学理论(如:分形理论)来描述电化学噪声的特征都可能代表了电化学噪声将来的研究方向.而电化学噪声技术在生物化学领域的应用则代表了它的发展方向.致谢:本文作者衷心感谢中科院金属所腐蚀与防护国家重点实验室林海潮研究员,史志明、李瑛和严川伟副研究员的无私帮助!参考文献:[1]Bertocci U,Huet F.Noise analysis applied to electrochemical sys tems[J].Corrosion,1995,51(2):131~144[2]Budevski E,Obretenov W,Bostanov W,S tai kov G.Noise analysis in metal deposition-expectati ons and limits[J].Electrochimical Acta,1989,34(8):1023~1029316中国腐蚀与防护学报第21卷[3]Lin H C.Cao C N.A study of electrochemical noise during pitting corrosion of iron in neutral solutions[J].Journal Chinese Society of Corrosion and Protection,1986,6(2):141(林海潮,曹楚南.孔蚀过程的电化学噪声研究[J].中国腐蚀与防护学报,1986,6(2):141~148)[4]Benzaid.A,Gabrielli C,Huet F,et al.Investigation of the electrochem i cal noise generated duri ng the stress corrosion crack i ng ofa 42CD4s teel electrode[J].M ateri als Science Forum,1992,111/112:167~176[5]Blanc G,Gabrielli C,Ksouri M ,W iart R.Experimental study of the relationships betw een the electrochem i cal noise an d thestructure of the electrodeposits of metals[J].Electrochim Acta,1978,23:337~340[6]Lengyel B,M eszaros L,M eszaros G,et al.Electrochemical methods to determine the corrosion rate of a metal protected by apaint film[J].Progress in Organic Coati ng,1999,36:11~14[7]Gabrielli C,Ksouri M ,Wiart R.Electrocrystallization noise:a phenomenological model[J].J.Electroanal.Chem.,1978,86:233~239[8]Alexander G Volkov.Green plants:electroch emical interfaces[J].J.Electroanalytical Ch emistry,2000,483:150~156[9]Oli veira Brett A M ,M acedo T R A,R ai mundo D,et al.Electrochem ical oxidation of m i toxantrone at a glassy carbon electrode[J].Analytical Chimica Acta,1999,385:401~408[10]M w esigw a J,Collins D J ,Volkov A G.Electroch emical signaling in green plants:effects of 2,4-dinitrophenol on variation andaction potenti als in soybean [J].Bioelectrochemistry,2000,51:201~205[11]M artinet S ,Durand R,Ozil P,Blanchard P.Application of electrochemical noise analysis to the study of batteries:state-of-charge determination and overcharge detecti on[J].J Pow er Sources,1999,83(1/2):93~99[12]Danielson M.M odeling of certain electrode parameters on the electrochemical noise response[J].Corrosi on,1997,53(10):770~777[13]Okada T.A theoretical analysi s of the electrochem i cal noise during the inducti on period of pitting corrosion in passive metals:Part 1.Th e current noise associated with the adsorption/desorption processes of halide ions on the passive film surface[J].J.Electroanal Chem.,1991,297:349~359[14]Okada T.A theoretical analysi s of the electrochem i cal noise during the inducti on period of pitting corrosion in passive metals:Part 2.The current noise associated w ith the halide nucleus formation in the passive fil m [J].J.Electroanal.Chem.,1991,297:361~375[15]Gabri elli C,Keddam M.Review of applicati ons of i m pedance an d noise analysis to un i form and localized corrosion[J].Corrosi on,1992,48(10):794~811[16]Cottis R A,Loto C A.Electrochemical noise gen erati on during SCC of a high-strength carbon steel[J].Corrosion,1990,46(1):12~19[17]Loto C A,Cottis R A.Electrochemical noise generation duri ng stres s corrosion cracking of alpha-brass[J].Corrosion,1987,43(8):499~504[18]Loto C A,Cottis R A.Electrochemical noi se generation during stress corrosion cracking of the h i gh -strength aluminumAA7075-T6alloy[J].Corrosion,1989,45(2):136~141[19]Tyagai V A.Faradaic noi se of complex electrochemical reactions[J].Electrochimica Acta,1971,16:1647~1654[20]Liu C,M acDonald D D,M edina E,et al.Probing corrosion activity in high subcritical and supercritical water through electrochemical noise analysis[J].Corrosion,1994,50(9):687~694[21]Guo J X,Li n T F,Qian X R.Study M ethod of M odern Physics and Its Application i n Corrosi on S cience[M ].Beijing:ChemicalIndustrial Pres s Corporation,1990(顾浚祥等.现代物理研究方法及其在腐蚀科学中的应用[M ].北京:化学工业出版社,1990,3)[22]Buchheit R G,M oran J P,Stoner G E.Localized corrosion behavior of alloy 2090 th e role of microstructural heterogeneity[J].Corrosion,1990,46(8):610~617[23]Salaita G N,Tate P H.Application of surface and surface i magi ng techniques in the study of erosion -corrosion in stainlesss teel[J].Corrosi on,1996,52(7):493~495[24]Chmiel J,Cieszczyk-Chmiel A,M atysik J.The use of i nterferometry in corrosi on i n vestigations[J].Corrosion ,1990,46(6):509~5122[25]Cheng Y F,Luo J L.Passivi ty an d pi tting of carbon steel in chromate solution[J].Electrochimica Acta,1999,44:4795~48043175期张鉴清等:电化学噪声的分析与应用 .电化学噪声的分析原理。
IEEE standard for Terminology and Test methods for ADC Std 1241-2000

IEEE Std1241-2000 IEEE Standard for Terminology and Test Methods for Analog-to-Digital ConvertersSponsorWaveform Measurement and Analysis Technical Committeeof theof theIEEE Instrumentation and Measurement SocietyApproved7December2000IEEE-SA Standards BoardAbstract:IEEE Std1241-2000identifies analog-to-digital converter(ADC)error sources and provides test methods with which to perform the required error measurements.The information in this standard is useful both to manufacturers and to users of ADCs in that it provides a basis for evaluating and comparing existing devices,as well as providing a template for writing specifications for the procurement of new ones.In some applications,the information provided by the tests described in this standard can be used to correct ADC errors, e.g.,correction for gain and offset errors.This standard also presents terminology and definitions to aid the user in defining and testing ADCs.Keywords:ADC,A/D converter,analog-to-digital converter,digitizer,terminology,test methodsThe Institute of Electrical and Electronics Engineers,Inc.3Park Avenue,New York,NY10016-5997,USACopyrightß2001by the Institute of Electrical and Electronics Engineers,Inc.All rights reserved. Published 13 June 2001. Printed in the United States of America.Print:ISBN0-7381-2724-8SH94902PDF:ISBN0-7381-2725-6SS94902No part of this publication may be reproduced in any form,in an electronic retrieval system or otherwise,without the prior written permission of the publisher.IEEE Standards documents are developed within the IEEE Societies and the Standards Coordinating Committees of the IEEE Standards Association(IEEE-SA)Standards Board.The IEEE develops its standards through a consensus development process,approved by the American National Standards Institute,which brings together volunteers representing varied viewpoints and interests to achieve thefinal product.Volunteers are not necessarily members of the Institute and serve without compensation.While the IEEE administers the process and establishes rules to promote fairness in the consensus development process,the IEEE does not independently evaluate,test,or verify the accuracy of any of the information contained in its standards.Use of an IEEE Standard is wholly voluntary.The IEEE disclaims liability for any personal injury,property or other damage,of any nature whatsoever,whether special,indirect,consequential,or compensatory,directly or indirectly resulting from the publication,use of,or reliance upon this,or any other IEEE Standard document.The IEEE does not warrant or represent the accuracy or content of the material contained herein,and expressly disclaims any express or implied warranty,including any implied warranty of merchantability orfitness for a specific purpose,or that the use of the material contained herein is free from patent infringement.IEEE Standards documents are supplied‘‘AS IS.’’The existence of an IEEE Standard does not imply that there are no other ways to produce,test,measure,purchase, market,or provide other goods and services related to the scope of the IEEE Standard.Furthermore,the viewpoint expressed at the time a standard is approved and issued is subject to change brought about through developments in the state of the art and comments received from users of the standard.Every IEEE Standard is subjected to review at least everyfive years for revision or reaffirmation.When a document is more thanfive years old and has not been reaffirmed,it is reasonable to conclude that its contents,although still of some value,do not wholly reflect the present state of the art. Users are cautioned to check to determine that they have the latest edition of any IEEE Standard.In publishing and making this document available,the IEEE is not suggesting or rendering professional or other services for,or on behalf of,any person or entity.Nor is the IEEE undertaking to perform any duty owed by any other person or entity to another.Any person utilizing this,and any other IEEE Standards document,should rely upon the advice of a competent professional in determining the exercise of reasonable care in any given circumstances.Interpretations:Occasionally questions may arise regarding the meaning of portions of standards as they relate to specific applications.When the need for interpretations is brought to the attention of IEEE,the Institute will initiate action to prepare appropriate responses.Since IEEE Standards represent a consensus of concerned interests,it is important to ensure that any interpretation has also received the concurrence of a balance of interests.For this reason, IEEE and the members of its societies and Standards Coordinating Committees are not able to provide an instant response to interpretation requests except in those cases where the matter has previously received formal consideration. Comments for revision of IEEE Standards are welcome from any interested party,regardless of membership affiliation with IEEE.Suggestions for changes in documents should be in the form of a proposed change of text,together with appropriate supporting ments on standards and requests for interpretations should be addressed to:Secretary,IEEE-SA Standards Board445Hoes LaneP.O.Box1331Piscataway,NJ08855-1331USANote:Attention is called to the possibility that implementation of this standard may require use of subjectmatter covered by patent rights.By publication of this standard,no position is taken with respect to theexistence or validity of any patent rights in connection therewith.The IEEE shall not be responsible foridentifying patents for which a license may be required by an IEEE standard or for conducting inquiriesinto the legal validity or scope of those patents that are brought to its attention.IEEE is the sole entity that may authorize the use of certification marks,trademarks,or other designations to indicate compliance with the materials set forth herein.Authorization to photocopy portions of any individual standard for internal or personal use is granted by the Institute of Electrical and Electronics Engineers,Inc.,provided that the appropriate fee is paid to Copyright Clearance Center. To arrange for payment of licensing fee,please contact Copyright Clearance Center,Customer Service,222Rosewood Drive,Danvers,MA01923USA;(978)750-8400.Permission to photocopy portions of any individual standard for educational classroom use can also be obtained through the Copyright Clearance Center.Introduction(This introduction is not a part of IEEE Std1241-2000,IEEE Standard for Terminology and Test Methods for Analog-to-Digital Converters.)This standard defines the terms,definitions,and test methods used to specify,characterize,and test analog-to-digital converters(ADCs).It is intended for the following:—Individuals and organizations who specify ADCs to be purchased—Individuals and organizations who purchase ADCs to be applied in their products —Individuals and organizations whose responsibility is to characterize and write reports on ADCs available for use in specific applications—Suppliers interested in providing high-quality and high-performance ADCs to acquirersThis standard is designed to help organizations and individuals—Incorporate quality considerations during the definition,evaluation,selection,and acceptance of supplier ADCs for operational use in their equipment—Determine how supplier ADCs should be evaluated,tested,and accepted for delivery to end users This standard is intended to satisfy the following objectives:—Promote consistency within organizations in acquiring third-party ADCs from component suppliers—Provide useful practices on including quality considerations during acquisition planning —Provide useful practices on evaluating and qualifying supplier capabilities to meet user requirements—Provide useful practices on evaluating and qualifying supplier ADCs—Assist individuals and organizations judging the quality and suitability of supplier ADCs for referral to end usersSeveral standards have previously been written that address the testing of analog-to-digital converters either directly or indirectly.These include—IEEE Std1057-1994a,which describes the testing of waveform recorders.This standard has been used as a guide for many of the techniques described in this standard.—IEEE Std746-1984[B16]b,which addresses the testing of analog-to-digital and digital-to-analog converters used for PCM television video signal processing.—JESD99-1[B21],which deals with the terms and definitions used to describe analog-to-digital and digital-to-analog converters.This standard does not include test methods.IEEE Std1241-2000for analog-to-digital converters is intended to focus specifically on terms and definitions as well as test methods for ADCs for a wide range of applications.a Information on references can be found in Clause2.b The numbers in brackets correspond to those in the bibliography in Annex C.As of October2000,the working group had the following membership:Steve Tilden,ChairPhilip Green,Secretary&Text EditorW.Thomas Meyer,Figures EditorPasquale Arpaia Giovanni Chiorboli Tom Linnenbrink*B.N.Suresh Babu Pasquale Daponte Solomon MaxAllan Belcher David Hansen Carlo MorandiDavid Bergman Fred Irons Bill PetersonEric Blom Dan Kien Pierre-Yves RoyDan Knierim*Chairman,TC-10CommitteeContributions were also made in prior years by:Jerry Blair John Deyst Norris NahmanWilliam Boyer Richard Kromer Otis M.SolomonSteve Broadstone Yves Langard T.Michael SoudersThe following members of the balloting committee voted on this standard:Pasquale Arpaia Pasquale Daponte W.Thomas MeyerSuresh Babu Philip Green Carlo MorandiEric Blom Fred Irons William E.PetersonSteven Broadstone Dan Knierim Pierre-Yves RoyGiovanni Chiorboli T.E.Linnenbrink Steven J.TildenSolomon MaxWhen the IEEE-SA Standards Board approved this standard on21September2000,it had the following membership:Donald N.Heirman,ChairJames T.Carlo,Vice-ChairJudith Gorman,SecretarySatish K.Aggarwal James H.Gurney James W.MooreMark D.Bowman Richard J.Holleman Robert F.MunznerGary R.Engmann Lowell G.Johnson Ronald C.PetersenHarold E.Epstein Robert J.Kennelly Gerald H.Petersonndis Floyd Joseph L.Koepfinger*John B.PoseyJay Forster*Peter H.Lips Gary S.RobinsonHoward M.Frazier L.Bruce McClung Akio TojoRuben D.Garzon Daleep C.Mohla Donald W.Zipse*Member EmeritusAlso included are the following nonvoting IEEE-SA Standards Board liaisons:Alan Cookson,NIST RepresentativeDonald R.Volzka,TAB RepresentativeDon MessinaIEEE Standards Project EditorContents1.Overview (1)1.1Scope (1)1.2Analog-to-digital converter background (2)1.3Guidance to the user (3)1.4Manufacturer-supplied information (5)2.References (7)3.Definitions and symbols (7)3.1Definitions (7)3.2Symbols and acronyms (14)4.Test methods (18)4.1General (18)4.2Analog input (41)4.3Static gain and offset (43)4.4Linearity (44)4.5Noise(total) (51)4.6Step response parameters (63)4.7Frequency response parameters (66)4.8Differential gain and phase (71)4.9Aperture effects (76)4.10Digital logic signals (78)4.11Pipeline delay (78)4.12Out-of-range recovery (78)4.13Word error rate (79)4.14Differential input specifications (81)4.15Comments on reference signals (82)4.16Power supply parameters (83)Annex A(informative)Comment on errors associated with word-error-rate measurement (84)Annex B(informative)Testing an ADC linearized with pseudorandom dither (86)Annex C(informative)Bibliography (90)IEEE Standard for Terminology and Test Methods for Analog-to-Digital Converters1.OverviewThis standard is divided into four clauses plus annexes.Clause1is a basic orientation.For further investigation,users of this standard can consult Clause2,which contains references to other IEEE standards on waveform measurement and relevant International Standardization Organization(ISO) documents.The definitions of technical terms and symbols used in this standard are presented in Clause3.Clause4presents a wide range of tests that measure the performance of an analog-to-digital converter.Annexes,containing the bibliography and informative comments on the tests presented in Clause4,augment the standard.1.1ScopeThe material presented in this standard is intended to provide common terminology and test methods for the testing and evaluation of analog-to-digital converters(ADCs).This standard considers only those ADCs whose output values have discrete values at discrete times,i.e., they are quantized and sampled.In general,this quantization is assumed to be nominally uniform(the input–output transfer curve is approximately a straight line)as discussed further in 1.3,and the sampling is assumed to be at a nominally uniform rate.Some but not all of the test methods in this standard can be used for ADCs that are designed for non-uniform quantization.This standard identifies ADC error sources and provides test methods with which to perform the required error measurements.The information in this standard is useful both to manufacturers and to users of ADCs in that it provides a basis for evaluating and comparing existing devices,as well as providing a template for writing specifications for the procurement of new ones.In some applications, the information provided by the tests described in this standard can be used to correct ADC errors, e.g.,correction for gain and offset errors.The reader should note that this standard has many similarities to IEEE Std1057-1994.Many of the tests and terms are nearly the same,since ADCs are a necessary part of digitizing waveform recorders.IEEEStd1241-2000IEEE STANDARD FOR TERMINOLOGY AND TEST METHODS 1.2Analog-to-digital converter backgroundThis standard considers only those ADCs whose output values have discrete values at discrete times, i.e.,they are quantized and sampled.Although different methods exist for representing a continuous analog signal as a discrete sequence of binary words,an underlying model implicit in many of the tests in this standard assumes that the relationship between the input signal and the output values approximates the staircase transfer curve depicted in Figure1a.Applying this model to a voltage-input ADC,the full-scale input range(FS)at the ADC is divided into uniform intervals,known as code bins, with nominal width Q.The number of code transition levels in the discrete transfer function is equal to 2NÀ1,where N is the number of digitized bits of the ADC.Note that there are ADCs that are designed such that N is not an integer,i.e.,the number of code transition levels is not an integral power of two. Inputs below thefirst transition or above the last transition are represented by the most negative and positive output codes,respectively.Note,however,that two conventions exist for relating V min and V max to the nominal transition points between code levels,mid-tread and mid-riser.The dotted lines at V min,V max,and(V minþV max)/2indicate what is often called the mid-tread convention,where thefirst transition is Q/2above V min and the last transition is3Q/2,below V max. This convention gets its name from the fact that the midpoint of the range,(V minþV max)/2,occurs in the middle of a code,i.e.,on the tread of the staircase transfer function.The second convention,called the mid-riser convention,is indicated in thefigure by dashed lines at V min,V max,and(V minþV max)/2. In this convention,V min isÀQ from thefirst transition,V max isþQ from the last transition,and the midpoint,(V minþV max)/2,occurs on a staircase riser.The difference between the two conventions is a displacement along the voltage axis by an amount Q/2.For all tests in this standard,this displacement has no effect on the results and either convention may be used.The one place where it does matter is when a device provides or expects user-provided reference signals.In this case the manufacturer must provide the necessary information relating the reference levels to the code transitions.In both conventions the number of code transitions is 2NÀ1and the full-scale range,FSR,is from V min to V max.Even in an ideal ADC,the quantization process produces errors.These errors contribute to the difference between the actual transfer curve and the ideal straight-line transfer curve,which is plotted as a function of the input signal in Figure1b.To use this standard,the user must understand how the transfer function maps its input values to output codewords,and how these output codewords are converted to the code bin numbering convention used in this standard.As shown in Figure1a,the lowest code bin is numbered0, the next is1,and so on up to the highest code bin,numbered(2NÀ1).In addition to unsigned binary(Figure1a),ADCs may use2’s complement,sign-magnitude,Gray,Binary-Coded-Decimal (BCD),or other output coding schemes.In these cases,a simple mapping of the ADC’s consecutive output codes to the unsigned binary codes can be used in applying various tests in this standard.Note that in the case of an ADC whose number of distinct output codes is not an integral power of2(e.g.,a BCD-coded ADC),the number of digitized bits N is still defined,but will not be an integer.Real ADCs have other errors in addition to the nominal quantization error shown in Figure1b.All errors can be divided into the categories of static and dynamic,depending on the rate of change of the input signal at the time of digitization.A slowly varying input can be considered a static signal if its effects are equivalent to those of a constant signal.Static errors,which include the quantization error, usually result from non-ideal spacing of the code transition levels.Dynamic errors occur because of additional sources of error induced by the time variation of the analog signal being sampled.Sources include harmonic distortion from the analog input stages,signal-dependent variations in the time of samples,dynamic effects in internal amplifier and comparator stages,and frequency-dependent variation in the spacing of the quantization levels.1.3Guidance to the user1.3.1InterfacingADCs present unique interfacing challenges,and without careful attention users can experience substandard results.As with all mixed-signal devices,ADCs perform as expected only when the analog and digital domains are brought together in a well-controlled fashion.The user should fully understand the manufacturer’s recommendations with regard to proper signal buffering and loading,input signal connections,transmission line matching,circuit layout patterns,power supply decoupling,and operating conditions.Edge characteristics for start-convert pulse(s)and clock(s)must be carefully chosen to ensure that input signal purity is maintained with sufficient margin up to the analog input pin(s).Most manufacturers now provide excellent ADC evaluation boards,which demonstrate IN P U T IN P U T(a)Figure 1—Staircase ADC transfer function,having full-scale range FSR and 2N À1levels,corresponding to N -bit quantizationIEEE FOR ANALOG-TO-DIGITAL CONVERTERS Std 1241-2000IEEEStd1241-2000IEEE STANDARD FOR TERMINOLOGY AND TEST METHODS recommended layout techniques,signal conditioning,and interfacing for their ADCs.If the characteristics of a new ADC are not well understood,then these boards should be analyzed or used before starting a new layout.1.3.2Test conditionsADC test specifications can be split into two groups:test conditions and test results.Typical examples of the former are:temperature,power supply voltages,clock frequency,and reference voltages. Examples of the latter are:power dissipation,effective number of bits,spurious free dynamic range (SFDR),and integral non-linearity(INL).The test methods defined in this standard describe the measurement of test results for given test conditions.ADC specification sheets will often give allowed ranges for some test condition(e.g.,power supply ranges).This implies that the ADC will function properly and that the test results will fall within their specified ranges for all test conditions within their specified ranges.Since the test condition ranges are generally specified in continuous intervals,they describe an infinite number of possible test conditions,which obviously cannot be exhaustively tested.It is up to the manufacturer or tester of an ADC to determine from design knowledge and/or testing the effect of the test conditions on the test result,and from there to determine the appropriate set of test conditions needed to accurately characterize the range of test results.For example,knowledge of the design may be sufficient to know that the highest power dissipation(test result)will occur at the highest power supply voltage(test condition),so the power dissipation test need be run only at the high end of the supply voltage range to check that the dissipation is within the maximum of its specified range.It is very important that relevant test conditions be stated when presenting test results.1.3.3Test equipmentOne must ensure that the performance of the test equipment used for these tests significantly exceeds the desired performance of the ADC under ers will likely need to include additional signal conditioning in the form offilters and pulse shapers.Accessories such as terminators, attenuators,delay lines,and other such devices are usually needed to match signal levels and to provide signal isolation to avoid corrupting the input stimuli.Quality testing requires following established procedures,most notably those specified in ISO9001: 2000[B18].In particular,traceability of instrumental calibration to a known standard is important. Commonly used test setups are described in4.1.1.1.3.4Test selectionWhen choosing which parameters to measure,one should follow the outline and hints in this clause to develop a procedure that logically and efficiently performs all needed tests on each unique setup. The standard has been designed to facilitate the development of these test procedures.In this standard the discrete Fourier transform(DFT)is used extensively for the extraction of frequency domain parameters because it provides numerous evaluation parameters from a single data record.DFT testing is the most prevalent technique used in the ADC manufacturing community,although the sine-fit test, also described in the standard,provides meaningful data.Nearly every user requires that the ADC should meet or exceed a minimum signal-to-noise-and-distortion ratio(SINAD)limit for the application and that the nonlinearity of the ADC be well understood.Certainly,the extent to whichthis standard is applied will depend upon the application;hence,the procedure should be tailored for each unique characterization plan.1.4Manufacturer-supplied information1.4.1General informationManufacturers shall supply the following general information:a)Model numberb)Physical characteristics:dimensions,packaging,pinoutsc)Power requirementsd)Environmental conditions:Safe operating,non-operating,and specified performance tempera-ture range;altitude limitations;humidity limits,operating and storage;vibration tolerance;and compliance with applicable electromagnetic interference specificationse)Any special or peculiar characteristicsf)Compliance with other specificationsg)Calibration interval,if required by ISO10012-2:1997[B19]h)Control signal characteristicsi)Output signal characteristicsj)Pipeline delay(if any)k)Exceptions to the above parameters where applicable1.4.2Minimum specificationsThe manufacturer shall provide the following specifications(see Clause3for definitions):a)Number of digitized bitsb)Range of allowable sample ratesc)Analog bandwidthd)Input signal full-scale range with nominal reference signal levelse)Input impedancef)Reference signal levels to be appliedg)Supply voltagesh)Supply currents(max,typ)i)Power dissipation(max,typ)1.4.3Additional specificationsa)Gain errorb)Offset errorc)Differential nonlinearityd)Harmonic distortion and spurious responsee)Integral nonlinearityf)Maximum static errorg)Signal-to-noise ratioh)Effective bitsi)Random noisej)Frequency responsek)Settling timel)Transition duration of step response(rise time)m)Slew rate limitn)Overshoot and precursorso)Aperture uncertainty(short-term time-base instability)p)Crosstalkq)Monotonicityr)Hysteresiss)Out-of-range recoveryt)Word error rateu)Common-mode rejection ratiov)Maximum common-mode signal levelw)Differential input impedancex)Intermodulation distortiony)Noise power ratioz)Differential gain and phase1.4.4Critical ADC parametersTable1is presented as a guide for many of the most common ADC applications.The wide range of ADC applications makes a comprehensive listing impossible.This table is intended to be a helpful starting point for users to apply this standard to their particular applications.Table1—Critical ADC parametersTypical applications Critical ADC parameters Performance issuesAudio SINAD,THD Power consumption.Crosstalk and gain matching.Automatic control MonotonicityShort-term settling,long-term stability Transfer function. Crosstalk and gain matching. Temperature stability.Digital oscilloscope/waveform recorder SINAD,ENOBBandwidthOut-of-range recoveryWord error rateSINAD for wide bandwidthamplitude resolution.Low thermal noise for repeatability.Bit error rate.Geophysical THD,SINAD,long-term stability Millihertz response.Image processing DNL,INL,SINAD,ENOBOut-of-range recoveryFull-scale step response DNL for sharp-edge detection. High-resolution at switching rate. Recovery for blooming.Radar and sonar SINAD,IMD,ENOBSFDROut-of-range recovery SINAD and IMD for clutter cancellation and Doppler processing.Spectrum analysis SINAD,ENOBSFDR SINAD and SFDR for high linear dynamic range measurements.Spread spectrum communication SINAD,IMD,ENOBSFDR,NPRNoise-to-distortion ratioIMD for quantization of smallsignals in a strong interferenceenvironment.SFDR for spatialfiltering.NPR for interchannel crosstalk.Telecommunication personal communications SINAD,NPR,SFDR,IMDBit error rateWord error rateWide input bandwidth channel bank.Interchannel crosstalk.Compression.Power consumption.Std1241-2000IEEE STANDARD FOR TERMINOLOGY AND TEST METHODS2.ReferencesThis standard shall be used in conjunction with the following publications.When the following specifications are superseded by an approved revision,the revision shall apply.IEC 60469-2(1987-12),Pulse measurement and analysis,general considerations.1IEEE Std 1057-1994,IEEE Standard for Digitizing Waveform Recorders.23.Definitions and symbolsFor the purposes of this standard,the following terms and definitions apply.The Authoritative Dictionary of IEEE Standards Terms [B15]should be referenced for terms not defined in this clause.3.1Definitions3.1.1AC-coupled analog-to-digital converter:An analog-to-digital converter utilizing a network which passes only the varying ac portion,not the static dc portion,of the analog input signal to the quantizer.3.1.2alternation band:The range of input levels which causes the converter output to alternate between two adjacent codes.A property of some analog-to-digital converters,it is the complement of the hysteresis property.3.1.3analog-to-digital converter (ADC):A device that converts a continuous time signal into a discrete-time discrete-amplitude signal.3.1.4aperture delay:The delay from a threshold crossing of the analog-to-digital converter clock which causes a sample of the analog input to be taken to the center of the aperture for that sample.COMINT ¼communications intelligence DNL ¼differential nonlinearity ENOB ¼effective number of bits ELINT ¼electronic intelligence NPR ¼noise power ratio INL ¼integral nonlinearity DG ¼differential gain errorSIGINT ¼signal intelligenceSINAD ¼signal-to-noise and distortion ratio THD ¼total harmonic distortion IMD ¼intermodulation distortion SFDR ¼spurious free dynamic range DP ¼differential phase errorTable 1—Critical ADC parameters (continued)Typical applicationsCritical ADC parametersPerformance issuesVideoDNL,SINAD,SFDR,DG,DP Differential gain and phase errors.Frequency response.Wideband digital receivers SIGINT,ELINT,COMINTSFDR,IMD SINADLinear dynamic range fordetection of low-level signals in a strong interference environment.Sampling frequency.1IEC publications are available from IEC Sales Department,Case Postale 131,3rue de Varemb,CH 1211,Gen ve 20,Switzerland/Suisse (http://www.iec.ch).IEC publications are also available in the United States from the Sales Department,American National Standards Institute,25W.43rd Street,Fourth Floor,New York,NY 10036,USA ().2IEEE publications are available from the Institute of Electrical and Electronics Engineers,445Hoes Lane,P.O.Box 1331,Piscataway,NJ 08855-1331,USA (/).。