Discovering the hidden structure of complex dynamic systems
导游词英文
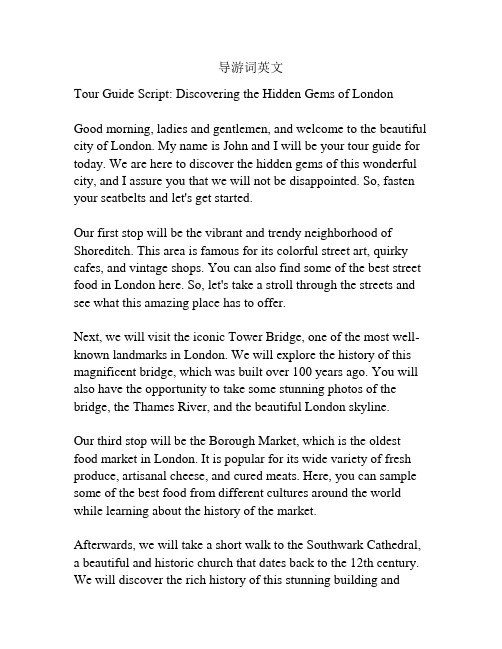
导游词英文Tour Guide Script: Discovering the Hidden Gems of LondonGood morning, ladies and gentlemen, and welcome to the beautiful city of London. My name is John and I will be your tour guide for today. We are here to discover the hidden gems of this wonderful city, and I assure you that we will not be disappointed. So, fasten your seatbelts and let's get started.Our first stop will be the vibrant and trendy neighborhood of Shoreditch. This area is famous for its colorful street art, quirky cafes, and vintage shops. You can also find some of the best street food in London here. So, let's take a stroll through the streets and see what this amazing place has to offer.Next, we will visit the iconic Tower Bridge, one of the most well-known landmarks in London. We will explore the history of this magnificent bridge, which was built over 100 years ago. You will also have the opportunity to take some stunning photos of the bridge, the Thames River, and the beautiful London skyline.Our third stop will be the Borough Market, which is the oldest food market in London. It is popular for its wide variety of fresh produce, artisanal cheese, and cured meats. Here, you can sample some of the best food from different cultures around the world while learning about the history of the market.Afterwards, we will take a short walk to the Southwark Cathedral, a beautiful and historic church that dates back to the 12th century. We will discover the rich history of this stunning building andlearn about its role in shaping the culture and history of London.Finally, we will end our tour at the Covent Garden, a bustling and vibrant area in the heart of London. This area is famous for its street performers, luxury shops, and stylish restaurants. You can also find the Royal Opera House here, which is a must-visit attraction for any culture lover.In conclusion, I hope you enjoyed this tour, and more importantly, I hope you discovered the hidden gems of London. This city has so much to offer, and I encourage you to continue exploring and experiencing all of its wonders. Thank you for joining me today, and have a fantastic rest of your time in London.As we wrap up our tour, I would like to share some additional hidden gems that you may want to explore during your time in London. These are lesser known but equally fascinating places that offer a unique glimpse into the city's rich history and culture.First on the list is the Leake Street Tunnel, also known as the Banksy Tunnel. This is a hidden gem that is tucked away in the Waterloo area. This underground tunnel is famous for its street art, and many of the pieces are created by well-known artists like Banksy. You can stroll through the tunnel and admire the colorful and thought-provoking art that covers the walls.Another hidden gem that you may want to visit is the Garden Museum. This museum is located in Lambeth, and it is dedicated to the art, history, and design of gardens. It is housed in the beautiful St. Mary-at-Lambeth church, which has a long and fascinating history. The museum offers a range of exhibitions,talks, and events, and it is a peaceful and inspiring place to visit.If you are interested in literary history, you may want to visit Dr. Johnson's House. This hidden gem is located in the heart of the city, and it is the former home of Samuel Johnson, the famous 18th-century writer who compiled the first comprehensive English dictionary. The house is now a museum that offers a fascinating insight into Johnson's life and work.For a more relaxing and natural experience, you can explore the Hampstead Heath. This is a large park that covers over 790 acres and offers stunning views of the city. It is a popular spot for hiking, picnicking, and enjoying the natural beauty of London. You can also visit Kenwood House, which is a stately home located on the edge of Hampstead Heath that houses a collection of artworks.Finally, you may want to visit the Grant Museum of Zoology, which is located in Bloomsbury. This hidden gem is home to over 80,000 specimens, including skeletons, taxidermy, and preserved animals. It offers a fascinating insight into the world of zoology, and it is a great place to visit with kids or as a science lover.In conclusion, London is a city that is full of hidden gems waiting to be discovered. Whether you are interested in history, art, culture, or nature, there is something for everyone. I encourage you to continue exploring and discovering all that this wonderful city has to offer. Thank you for joining me on this tour, and I hope you have a fantastic time during your stay in London.。
介绍保定景点的英语作文
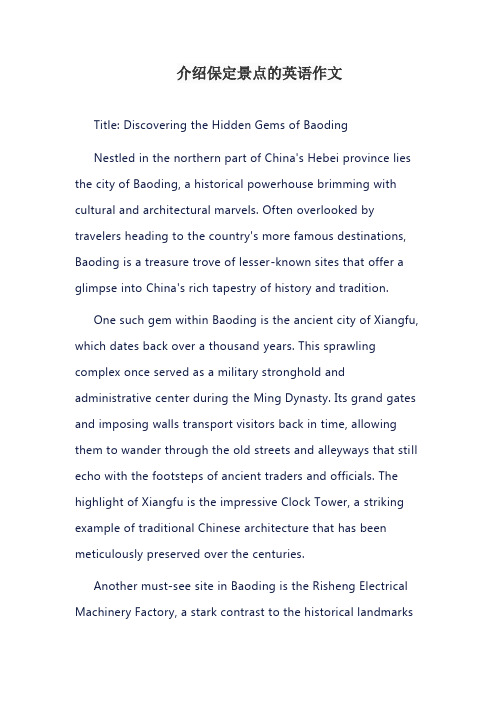
介绍保定景点的英语作文Title: Discovering the Hidden Gems of BaodingNestled in the northern part of China's Hebei province lies the city of Baoding, a historical powerhouse brimming with cultural and architectural marvels. Often overlooked by travelers heading to the country's more famous destinations, Baoding is a treasure trove of lesser-known sites that offer a glimpse into China's rich tapestry of history and tradition.One such gem within Baoding is the ancient city of Xiangfu, which dates back over a thousand years. This sprawling complex once served as a military stronghold and administrative center during the Ming Dynasty. Its grand gates and imposing walls transport visitors back in time, allowing them to wander through the old streets and alleyways that still echo with the footsteps of ancient traders and officials. The highlight of Xiangfu is the impressive Clock Tower, a striking example of traditional Chinese architecture that has been meticulously preserved over the centuries.Another must-see site in Baoding is the Risheng Electrical Machinery Factory, a stark contrast to the historical landmarksbut equally significant in its own right. This factory, established during the Qing Dynasty, played a pioneering role in modernizing China's industrial landscape. Today, it stands as a monument to the country's industrial evolution, offering tours that reveal the fascinating process of how electrical machinery was crafted in the early days of industrialization.For those seeking serenity amidst urban bustle, Baoding's numerous temples provide a haven of tranquility. The Fushan Temple, nestled at the foot of Fushan Mountain, is a Buddhist sanctuary that has watched over the city for hundreds of years. Its elegant pavilions, peaceful courtyards, and exquisite sculptures are a testament to the devotion and artistry of ancient Chinese craftsmen.The beauty of nature can also be experienced at the Baiyangdian Lake, a vast wetland area that serves as a natural oasis near the city. Home to abundant wildlife and aquatic plants, this ecological wonder offers a chance to escape the city's hustle and immerse oneself in the tranquility of water birds gliding across the lake's surface.Baoding's culinary scene is another reason to visit, as the city is renowned for its unique flavors and traditional dishes.The local specialty, Baoding meatballs, are a delicious reminder of the city's rich heritage, combining succulent meats with spices and cooking techniques passed down through generations.In conclusion, Baoding may not be as well-known as some of China's other cities, but it certainly holds its own against them with its blend of historical sites, natural beauty, and culinary delights. A trip to this unassuming city promises an adventure through time, where the past and present coexist in harmony, and the stories of ancient China come to life. For those seeking an off-the-beaten-path experience in China, Baoding is a destination that will undoubtedly charm and captivate.。
Finding structure in time
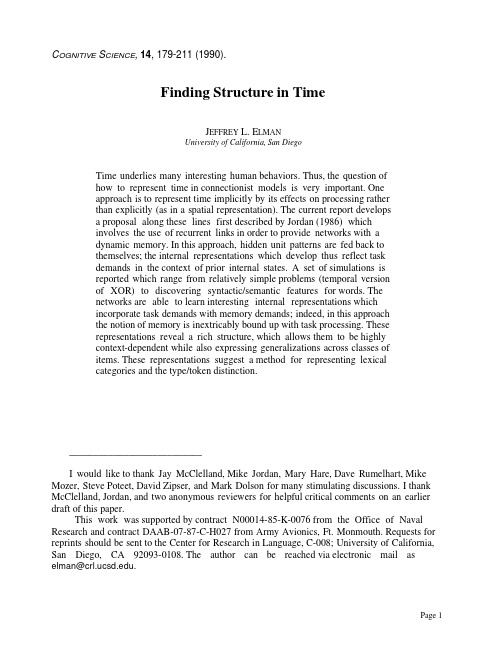
C OGNITIVE S CIENCE,14, 179-211 (1990).Finding Structure in TimeJ EFFREY L. E LMANUniversity of California, San DiegoTime underlies many interesting human behaviors. Thus, the question ofhow to represent time in connectionist models is very important. Oneapproach is to represent time implicitly by its effects on processing ratherthan explicitly (as in a spatial representation). The current report developsa proposal along these lines first described by Jordan (1986) whichinvolves the use of recurrent links in order to provide networks with adynamic memory. In this approach, hidden unit patterns are fed back tothemselves; the internal representations which develop thus reflect taskdemands in the context of prior internal states. A set of simulations isreported which range from relatively simple problems (temporal versionof XOR) to discovering syntactic/semantic features for words. Thenetworks are able to learn interesting internal representations whichincorporate task demands with memory demands; indeed, in this approachthe notion of memory is inextricably bound up with task processing. Theserepresentations reveal a rich structure, which allows them to be highlycontext-dependent while also expressing generalizations across classes ofitems. These representations suggest a method for representing lexicalcategories and the type/token distinction.___________________________I would like to thank Jay McClelland, Mike Jordan, Mary Hare, Dave Rumelhart, Mike Mozer, Steve Poteet, David Zipser, and Mark Dolson for many stimulating discussions. I thank McClelland, Jordan, and two anonymous reviewers for helpful critical comments on an earlier draft of this paper.This work was supported by contract N00014-85-K-0076 from the Office of Naval Research and contract DAAB-07-87-C-H027 from Army Avionics, Ft. Monmouth. Requests for reprints should be sent to the Center for Research in Language, C-008; University of California, San Diego, CA 92093-0108. The author can be reached via electronic mail as elman@.IntroductionTime is clearly important in cognition. It is inextricably bound up with many behaviors (such as language) which express themselves as temporal sequences. Indeed, it is difficult to know how one might deal with such basic problems as goal-directed behavior, planning, or causation without some way of representing time.The question of how to represent time might seem to arise as a special problem unique to parallel processing models, if only because the parallel nature of computation appears to be at odds with the serial nature of temporal events. However, even within traditional (serial) frameworks, the representation of serial order and the interaction of a serial input or output with higher levels of representation presents challenges. For example, in models of motor activity an important issue is whether the action plan is a literal specification of the output sequence, or whether the plan represents serial order in a more abstract manner (e.g., Lashley, 1951; MacNeilage, 1970; Fowler, 1977, 1980; Kelso, Saltzman, & Tuller, 1986; Saltzman & Kelso, 1987; Jordan & Rosenbaum, 1988). Linguistic theoreticians have perhaps tended to be less concerned with the representation and processing of the temporal aspects to utterances (assuming, for instance, that all the information in an utterance is somehow made available simultaneously in a syntactic tree); but the research in natural language parsing suggests that the problem is not trivially solved (e.g., Frazier & Fodor; 1978; Marcus, 1980). Thus, what is one of the most elementary facts about much of human activity -that it has temporal extent -is sometimes ignored and is often problematic.In parallel distributed processing models, the processing of sequential inputs has been accomplished in several ways. The most common solution is to attempt to “parallels time” by giving it a spatial representation. However, there are problems with this approach, and it is ultimately not a good solution. A better approach would be to represent time implicitly rather than explicitly. That is, we represent time by the effect it has on processing and not as an additional dimension of the input.This paper describes the results of pursuing this approach, with particular emphasis on problems that are relevant to natural language processing. The approach taken is rather simple, but the results are sometimes complex and unexpected. Indeed, it seems that the solution to the problem of time may interact with other problems for connectionist architectures, including the problem of symbolic representation and how connectionist representations encode structure. The current approach supports the notion outlined by Van Gelder (1989; see also Smolensky, 1987, 1988; Elman, 1989), that connectionist representations may have a functional compositionality without being syntactically compositional.The first section briefly describes some of the problems that arise when time is represented externally as a spatial dimension. The second section describes the approach used in this work. The major portion of this report presents the results of applying this new architecture to a diverse set of problems. These problems range in complexity from a temporal version of the Exclusive-OR function to the discovery of syntactic/semantic categories in natural language data.The Problem with TimeOne obvious way of dealing with patterns that have a temporal extent is to represent time explicitly by associating the serial order of the pattern with the dimensionality of the patternvector. The first temporal event is represented by the first element in the pattern vector, the second temporal event is represented by the second position in the pattern vector; and so on. The entire pattern vector is processed in parallel by the model. This approach has been used in a variety of models (e.g., Cottrell, Munro, & Zipser, 1987; Elman & Zipser, 1988; Hanson & Kegl, 1987).There are several drawbacks to this approach, which basically uses a spatial metaphor for time. First, it requires that there be some interface with the world which buffers the input so that it can be presented all at once. It is not clear that biological systems make use of such shift registers. There are also logical problems; how should a system know when a buffer’s contents should be examined?Second, the shift-register imposes a rigid limit on the duration of patterns (since the input layer must provide for the longest possible pattern), and further suggests that all input vectors be the same length. These problems are particularly troublesome in domains such as language, where one would like comparable representations for patterns that are of variable length. This is as true of the basic units of speech (phonetic segments) as it is of sentences.Finally, and most seriously, such an approach does not easily distinguish relative temporal position from absolute temporal position. For example, consider the following two vectors.[ 0 1 1 1 0 0 0 0 0 ][ 0 0 0 1 1 1 0 0 0 ]These two vectors appear to be instances of the same basic pattern, but displaced in space (or time, if we give these a temporal interpretation). However, as the geometric interpretation of these vectors makes clear, the two patterns are in fact quite dissimilar and spatially distant.1 PDP models can of course be trained to treat these two patterns as similar. But the similarity is a consequence of an external teacher and not of the similarity structure of the patterns themselves, and the desired similarity does not generalize to novel patterns. This shortcoming is serious if one is interested in patterns in which the relative temporal structure is preserved in the face of absolute temporal displacements.What one would like is a representation of time which is richer and which does not have these problems. In what follows here, a simple architecture is described which has a number of desirable temporal properties and has yielded interesting results.Networks with MemoryThe spatial representation of time described above treats time as an explicit part of the input. There is another, very different possibility, which is to allow time to be represented by the effect it has on processing. This means giving the processing system dynamic properties which are responsive to temporal sequences. In short, the network must be given memory.1.The reader may more easily be convinced of this by comparing the locations of the vectors [100], [010], and [001] in 3-space. Although these patterns might be considered ’temporally displaced’ versions of the same basic pat-tern, the vectors are very different.activate the output units. The hidden units also feed back to activate the context units. This constitutes the forward activation. Depending on the task, there may or may not be a learning phase in this time cycle. If so, the output is compared with a teacher input and backpropagation of error (Rumelhart, Hinton, & Williams, 1986) is used to incrementally adjust connection strengths. Recurrent connections are fixed at 1.0 and are not subject to adjustment.3 At the next time step t+1 the above sequence is repeated. This time the context units contain values which are exactly the hidden unit values at time t. These context units thus provide the network with memory.Internal Representation of Time. In feedforward networks employing hidden units and a learning algorithm, the hidden units develop internal representations for the input patterns which recode those patterns in a way which enables the network to produce the correct output for a given input. In the present architecture, the context units remember the previous internal state. Thus, the hidden units have the task of mapping both an external input and also the previous internal state to some desired output. Because the patterns on the hidden units are what are saved as context, the hidden units must accomplish this mapping and at the same time develop representations which are useful encodings of the temporal properties of the sequential input. Thus, the internal representations that develop are sensitive to temporal context; the effect of time is implicit in these internal states. Note, however, that these representations of temporal context need not be literal. They represent a memory which is highly task and stimulus-dependent.Consider now the results of applying this architecture to a number of problems which involve processing of inputs which are naturally presented in sequence.Exclusive-ORThe Exclusive-OR (XOR) function has been of interest because it cannot be learned by a simple two-layer network. Instead, it requires at least three-layers. The XOR is usually presented as a problem involving 2-bit input vectors (00, 11, 01, 10) yielding 1-bit output vectors (0, 0, 1, 1; respectively).This problem can be translated into a temporal domain in several ways. One version involves constructing a sequence of 1-bit inputs by presenting the 2-bit inputs one bit at a time (i.e., in two time steps), followed by the 1-bit output; then continuing with another input/output pair chosen at random. A sample input might be:1 0 1 0 0 0 0 1 1 1 1 0 1 0 1. . .Here, the first and second bits are XOR-ed to produce the third; the fourth and fifth are XOR-ed to give the sixth; and so on. The inputs are concatenated and presented as an unbroken sequence.In the current version of the XOR problem, the input consisted of a sequence of 3000 bits constructed in this manner. This input stream was presented to the network shown in Figure 2 (with 1 input unit, 2 hidden units, 1 output unit, and 2 context units), one bit at a time. The task of the network was, at each point in time, to predict the next bit in the sequence. That is, given 3. A little more detail is in order about the connections between the context units and hidden units. In the network used here there were one-for-one connections between each hidden unit and each context unit. This implies there are an equal number of context and hidden units. The upward connections between the context units and the hidden units were fully distributed, such that each context unit activates all the hidden units.averaging of error which was done for Figure 3. If one looks at the output activations it is apparent from the nature of the errors that the network predicts successive inputs to be the XOR of the previous two. This is guaranteed to be successful every third bit, and will sometimes—-fortuitously—also result in correct predictions at other times.It is interesting that the solution to the temporal version of XOR is somewhat different than the static version of the same problem. In a network with 2 hidden units, one unit is highly activated when the input sequence is a series of identical elements (all 1s or 0s), whereas the other unit is highly activated when the input elements alternate. Another way of viewing this is that the network developed units which were sensitive to high and low-frequency inputs. This is a different solution than is found with feedforward networks and simultaneously presented inputs. This suggests that problems may change their nature when cast in a temporal form. It is not clear that the solution will be easier or more difficult in this form; but it is an important lesson to realize that the solution may be different.In this simulation the prediction task has been used in a way which is somewhat analogous to autoassociation. Autoassociation is a useful technique for discovering the intrinsic structure possessed by a set of patterns. This occurs because the network must transform the patterns into more compact representations; it generally does so by exploiting redundancies in the patterns. Finding these redundancies can be of interest for what they tell us about the similarity structure of the data set (cf. Cottrell, Munro, & Zipser, 1987; Elman & Zipser, 1988).In this simulation the goal was to find the temporal structure of the XOR sequence. Simple autoassociation would not work, since the task of simply reproducing the input at all points in time is trivially solvable and does not require sensitivity to sequential patterns. The prediction task is useful because its solution requires that the network be sensitive to temporal structure.Structure in Letter SequencesOne question which might be asked is whether the memory capacity of the network architecture employed here is sufficient to detect more complex sequential patterns than the XOR. The XOR pattern is simple in several respects. It involves single-bit inputs, requires a memory which extends only one bit back in time, and there are only four different input patterns. More challenging inputs would require multi-bit inputs of greater temporal extent, and a larger inventory of possible sequences. Variability in the duration of a pattern might also complicate the problem.An input sequence was devised which was intended to provide just these sorts of complications. The sequence was composed of six different 6-bit binary vectors. Although the vectors were not derived from real speech, one might think of them as representing speech sounds, with the six dimensions of the vector corresponding to articulatory features. Table 1 shows the vector for each of the six letters.The sequence was formed in two steps. First, the 3 consonants (b, d, g) were combined in random order to obtain a 1000-letter sequence. Then each consonant was replaced using the rulesb ¥ bad ¥ diig ¥ guuconsists of a 6-bit rather than a 1-bit vector. One might have reasonably thought that the more extended sequential dependencies of these patterns would exceed the temporal processing capacity of the network. But almost the opposite is true. The fact that there are subregularities (at the level of individual bit patterns) enables the network to make partial predictions even in cases where the complete prediction is not possible. All of this is dependent on the fact that the input is structured, of course. The lesson seems to be that more extended sequential dependencies may not necessarily be more difficult to learn. If the dependencies are structured, that structure may make learning easier and not harder.Discovering the notion “word”It is taken for granted that learning a language involves (among many other things) learning the sounds of that language, as well as the morphemes and words. Many theories of acquisition depend crucially on such primitive types as word, or morpheme, or more abstract categories as noun, verb, or phrase (e.g., Berwick & Weinberg, 1984; Pinker, 1984). It is rarely asked how it is that a language learner knows to begin with that these entities exist. These notions are often assumed to be innate.Yet in fact, there is considerable debate among linguists and psycholinguists about what are the representations used in language. Although it is commonplace to speak of basic units such as “phoneme,” “morpheme,” and “word”, these constructs have no clear and uncontroversial definition. Moreover, the commitment to such distinct levels of representation leaves a troubling residue of entities that appear to lie between the levels. For instance, in many languages, there are sound/meaning correspondences which lie between the phoneme and the morpheme (i.e., sound symbolism). Even the concept “word” is not as straightforward as one might think (cf. Greenberg, 1963; Lehman, 1962). Within English, for instance, there is no consistently definable distinction between words (e.g., “apple”), compounds (“apple pie”) and phrases (“Library of Congress” or “man in the street”). Furthermore, languages differ dramatically in what they treat as words. In polysynthetic languages (e.g., Eskimo) what would be called words more nearly resemble what the English speaker would call phrases or entire sentences.Thus, the most fundamental concepts of linguistic analysis have a fluidity which at the very least suggests an important role for learning; and the exact form of the those concepts remains an open and important question.In PDP networks, representational form and representational content often can be learned simultaneously. Moreover, the representations which result have many of the flexible and graded characteristics which were noted above. Thus, one can ask whether the notion “word” (or something which maps on to this concept) could emerge as a consequence of learning the sequential structure of letter sequences which form words and sentences (but in which word boundaries are not marked).Let us thus imagine another version of the previous task, in which the letter sequences form real words, and the words form sentences. The input will consist of the individual letters (we will imagine these as analogous to speech sounds, while recognizing that the orthographic input is vastly simpler than acoustic input would be). The letters will be presented in sequence, one at a time, with no breaks between the letters in a word, and no breaks between the words of different sentences.Such a sequence was created using a sentence generating program and a lexicon of 15 words.4 The program generated 200 sentences of varying length, from 4 to 9 words. Thesentences were concatenated, forming a stream of 1,270 words. Next, the words were broken into their letter parts, yielding 4,963 letters. Finally, each letter in each word was converted into a 5-bit random vector.The result was a stream of 4,963 separate 5-bit vectors, one for each letter. These vectors were the input and were presented one at a time. The task at each point in time was to predict the next letter. A fragment of the input and desired output is shown in Table 2.Table 6:Input Output0110m0000a0000a0111n0111n1100y1100y1100y1100y0010e0010e0000a0000a1001r1001r1001s1001s0000a0000a0011g0011g0111o0111o0000a0000a0001b0001b0111o0111o1100y1100y0000a0000a0111n0111n0010d0010d0011g0011g0100i0100i1001r1001r0110l0110l1100y1100y4.The program used was a simplified version of the program described in greater detail in the next simulation.as a cue as to the boundaries of linguistic units which must be learned, and it demonstrates the ability of simple recurrent networks to extract this information.Discovering lexical classes from word orderConsider now another problem which arises in the context of word sequences. The order of words in sentences reflects a number of constraints. In languages such as English (so-called “fixed word-order” languages), the order is tightly constrained. In many other languages (the “free word-order” languages), there is greater optionality as to word order (but even here the order is not free in the sense of random). Syntactic structure, selectional restrictions, subcategorization, and discourse considerations are among the many factors which join together to fix the order in which words occur. Thus, the sequential order of words in sentences is neither simple nor is it determined by a single cause. In addition, it has been argued that generalizations about word order cannot be accounted for solely in terms of linear order (Chomsky, 1957; Chomsky, 1965). Rather, there is an abstract structure which underlies the surface strings and it is this structure which provides a more insightful basis for understanding the constraints on word order.While it is undoubtedly true that the surface order of words does not provide the most insightful basis for generalizations about word order, it is also true that from the point of view of the listener, the surface order is the only thing visible (or audible). Whatever the abstract underlying structure be, it is cued by the surface forms and therefore that structure is implicit in them.In the previous simulation, we saw that a network was able to learn the temporal structure of letter sequences. The order of letters in that simulation, however, can be given with a small set of relatively simple rules.5 The rules for determining word order in English, on the other hand, will be complex and numerous. Traditional accounts of word order generally invoke symbolic processing systems to express abstract structural relationships. One might therefore easily believe that there is a qualitative difference in the nature of the computation required for the last simulation, and that required to predict the word order of English sentences. Knowledge of word order might require symbolic representations which are beyond the capacity of (apparently) non-symbolic PDP systems. Furthermore, while it is true, as pointed out above, that the surface strings may be cues to abstract structure, considerable innate knowledge may be required in order to reconstruct the abstract structure from the surface strings. It is therefore an interesting question to ask whether a network can learn any aspects of that underlying abstract structure.Simple sentences. As a first step, a somewhat modest experiment was undertaken. A sentence generator program was used to construct a set of short (two and three-word) utterances. 13 classes of nouns and verbs were chosen; these are listed in Table 3. Examples of each category are given; it will be noticed that instances of some categories (e.g, VERB-DESTROY) may be included in others (e.g., VERB-TRAN). There were 29 different lexical items.5.In the worst case, each word constitutes a rule. More hopefully, networks will learn that recurring orthographic regularities provide additional and more general constraints (cf. Sejnowski & Rosenberg, 1987).Table 3: Categories of lexical items used in sentence simulationCategory ExamplesNOUN-HUM man, womanNOUN-ANIM cat, mouseNOUN-INANIM book, rockNOUN-AGRESS dragon, monsterNOUN-FRAG glass, plateNOUN-FOOD cookie, sandwichVERB-INTRAN think, sleepVERB-TRAN see, chaseVERB-AGPA move, breakVERB-PERCEPT smell, seeVERB-DESTROY break, smashVERB-EA eatThe generator program used these categories and the 15 sentence templates given in Table 4Table 4: Templates for sentence generatorWORD 1WORDS WORD 3NOUN-HUM VERB-EA T NOUN-FOODNOUN-HUM VERB-PERCEPT NOUN-INANIMNOUN-HUM VERB-DESTROY NOUN-FRAGNOUN-HUM VERB-INTRANNOUN-HUM VERB-TRAN NOUN-HUMNOUN-HUM VERB-AG PAT NOUN-INANIMNOUN-HUM VERB-AG PATNOUN-ANIM VERB-EA T NOUN-FOODNOUN-ANIM VERB-TRAN NOUN-ANIMNOUN-ANIM VERB-AG PAT NOUN-INANIMNOUN-ANIM VERB-AG PATNOUN-INANIM VERB-AG PATNOUN-AGRESS VERB-DESTORY NOUN-FRAGNOUN-AGRESS VERB-EA T NOUN-HUMNOUN-AGRESS VERB-EA T NOUN-ANIMNOUN-AGRESS VERB-EA T NOUN-FOODto create 10,000 random two- and three-word sentence frames. Each sentence frame was thenfilled in by randomly selecting one of the possible words appropriate to each category. Each word was replaced by a randomly assigned 31-bit vector in which each word was represented by a different bit. Whenever the word was present, that bit was flipped on. Two extra bits were reserved for later simulations. This encoding scheme guaranteed that each vector was orthogonal to every other vector and reflected nothing about the form class or meaning of the words. Finally, the 27,354 word vectors in the 10,000 sentences were concatenated, so that an input stream of 27,354 31-bit vectors was created. Each word vector was distinct, but there were no breaks between successive sentences. A fragment of the input stream is shown in column 1 of Table 5, with the English gloss for each vector in parentheses. The desired output is given in column 2.Table 5: Fragment of training sequences for sentence simulationINPUT OUTPUT 0000000000000000000000000000010(woman)0000000000000000000000000010000(smash) 0000000000000000000000000010000(smash)0000000000000000000001000000000(plate) 0000000000000000000001000000000(plate)0000010000000000000000000000000(cat) 0000010000000000000000000000000(cat)0000000000000000000100000000000(move) 0000000000000000000100000000000(move)0000000000000000100000000000000(man) 0000000000000000100000000000000(man)0001000000000000000000000000000(break) 0001000000000000000000000000000(break)0000100000000000000000000000000(car) 0000100000000000000000000000000(car)0100000000000000000000000000000(boy) 0100000000000000000000000000000(boy)0000000000000000000100000000000(move) 0000000000000000000100000000000(move)0000000000001000000000000000000(girl) 0000000000001000000000000000000(girl)0000000000100000000000000000000(eat) 0000000000100000000000000000000(eat)0010000000000000000000000000000(bread) 0010000000000000000000000000000(bread)0000000010000000000000000000000(dog) 0000000010000000000000000000000(dog)0000000000000000000100000000000(move) 0000000000000000000100000000000(move)0000000000000000001000000000000(mouse) 0000000000000000001000000000000(mouse)0000000000000000001000000000000(mouse) 0000000000000000001000000000000(mouse)0000000000000000000100000000000(move) 0000000000000000000100000000000(move)1000000000000000000000000000000(book) 1000000000000000000000000000000(book)0000000000000001000000000000000(lionFor this simulation a network was used which was similar to that in the first simulation, except that the input layer and output layers contained 31 nodes each, and the hidden and context layers contained 150 nodes each.The task given to the network was to learn to predict the order of successive words. The training strategy was as follows. The sequence of 27,354 31-bit vectors formed an input sequence. Each word in the sequence was input, one at a time, in order. The task on each input cycle was to predict the 31-bit vector corresponding to the next word in the sequence. At the end of the 27,354 word sequence, the process began again without a break starting with the first。
寻找隐藏的地方的英语作文
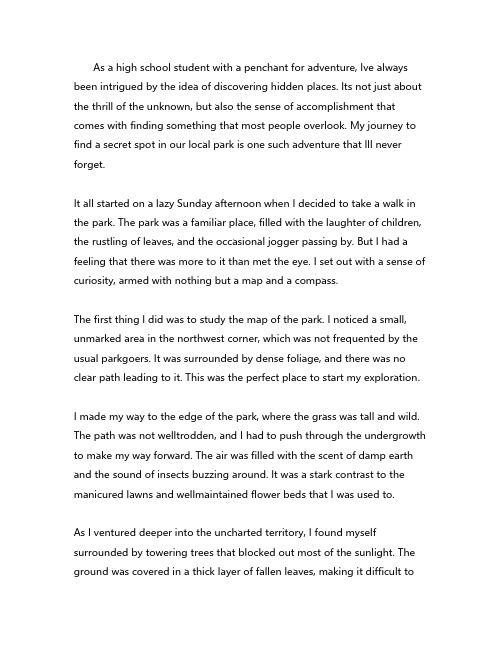
As a high school student with a penchant for adventure, Ive always been intrigued by the idea of discovering hidden places. Its not just about the thrill of the unknown, but also the sense of accomplishment that comes with finding something that most people overlook. My journey to find a secret spot in our local park is one such adventure that Ill never forget.It all started on a lazy Sunday afternoon when I decided to take a walk in the park. The park was a familiar place, filled with the laughter of children, the rustling of leaves, and the occasional jogger passing by. But I had a feeling that there was more to it than met the eye. I set out with a sense of curiosity, armed with nothing but a map and a compass.The first thing I did was to study the map of the park. I noticed a small, unmarked area in the northwest corner, which was not frequented by the usual parkgoers. It was surrounded by dense foliage, and there was no clear path leading to it. This was the perfect place to start my exploration.I made my way to the edge of the park, where the grass was tall and wild. The path was not welltrodden, and I had to push through the undergrowth to make my way forward. The air was filled with the scent of damp earth and the sound of insects buzzing around. It was a stark contrast to the manicured lawns and wellmaintained flower beds that I was used to.As I ventured deeper into the uncharted territory, I found myself surrounded by towering trees that blocked out most of the sunlight. The ground was covered in a thick layer of fallen leaves, making it difficult towalk without making a sound. I could hear the distant calls of birds and the occasional rustle of small animals in the underbrush.After about an hour of walking, I stumbled upon a small clearing. It was a hidden gem, a place untouched by human hands. The sunlight filtered through the canopy, casting a soft glow on the ground below. There was a small pond in the center, surrounded by wildflowers that danced in the breeze. It was a tranquil oasis, a stark contrast to the bustling city life just beyond the parks borders.I spent the rest of the afternoon exploring the clearing, marveling at the beauty of nature. I found a spot under a large tree and sat down, taking in the sights and sounds around me. The tranquility was a welcome respite from the constant noise and chaos of everyday life.As the sun began to set, I made my way back to the main path, feeling a sense of accomplishment. I had discovered a hidden place, a secret spot that was mine alone. It was a reminder that there is always more to explore, more to discover, even in the most familiar of places.This experience taught me the importance of stepping out of my comfort zone and embracing the unknown. It showed me that adventure is not always about traveling to faroff lands or embarking on grand expeditions. Sometimes, its about taking the road less traveled, exploring the hidden corners of our own backyard.In conclusion, the journey to find a hidden place in the park was anunforgettable adventure that taught me valuable lessons about curiosity, exploration, and the beauty of nature. It was a reminder that sometimes, the most rewarding experiences are the ones that we stumble upon unexpectedly. And as I walked back home, I couldnt help but feel a sense of excitement for the next adventure that awaited me.。
假如我是海豚英语作文七年级
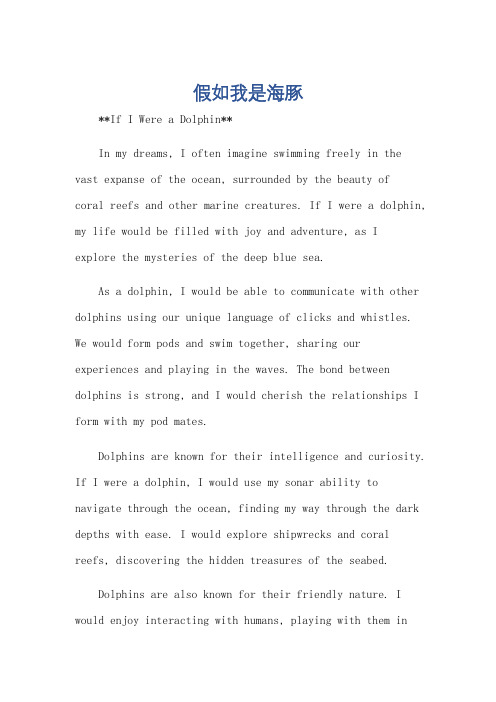
假如我是海豚**If I Were a Dolphin**In my dreams, I often imagine swimming freely in the vast expanse of the ocean, surrounded by the beauty ofcoral reefs and other marine creatures. If I were a dolphin, my life would be filled with joy and adventure, as Iexplore the mysteries of the deep blue sea.As a dolphin, I would be able to communicate with other dolphins using our unique language of clicks and whistles. We would form pods and swim together, sharing our experiences and playing in the waves. The bond between dolphins is strong, and I would cherish the relationships I form with my pod mates.Dolphins are known for their intelligence and curiosity. If I were a dolphin, I would use my sonar ability to navigate through the ocean, finding my way through the dark depths with ease. I would explore shipwrecks and coral reefs, discovering the hidden treasures of the seabed.Dolphins are also known for their friendly nature. I would enjoy interacting with humans, playing with them inthe water and even helping them in times of need. There are many stories of dolphins saving people from drowning, and I would be proud to be part of such a compassionate species. However, being a dolphin also means facing challenges. The ocean can be a dangerous place, and dolphins are often hunted and killed for their meat and fins. If I were a dolphin, I would strive to protect my species and the ocean we call home. I would倡导和平与保护海洋生态的重要性,希望humans would understand the importance of preserving our ocean and its creatures.If I were a dolphin, I would also strive to raise awareness about the need to protect the ocean frompollution and plastic waste. Dolphins often become entangled in fishing nets and other debris, which can cause serious injuries. By raising awareness, we can help reduce the amount of plastic waste in the ocean and protect the habitats of dolphins and other marine life.In conclusion, if I were a dolphin, my life would be filled with adventure, joy, and the responsibility of protecting our beautiful ocean. I would cherish the relationships I form with other dolphins and theopportunity to interact with humans. I would strive to protect our species and the ocean we call home, hoping that humans would join me in this effort to preserve the beauty and health of our planet.**假如我是海豚**假如我是海豚,那我的生活将会充满无尽的自由和欢乐。
学认识身边的植物英语作文
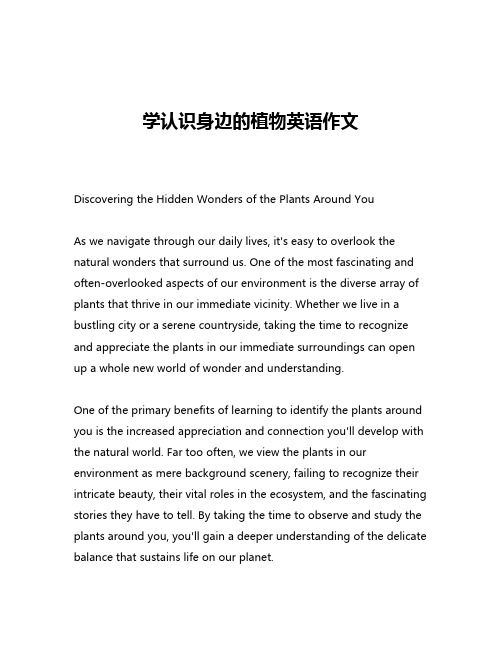
学认识身边的植物英语作文Discovering the Hidden Wonders of the Plants Around YouAs we navigate through our daily lives, it's easy to overlook the natural wonders that surround us. One of the most fascinating and often-overlooked aspects of our environment is the diverse array of plants that thrive in our immediate vicinity. Whether we live in a bustling city or a serene countryside, taking the time to recognize and appreciate the plants in our immediate surroundings can open up a whole new world of wonder and understanding.One of the primary benefits of learning to identify the plants around you is the increased appreciation and connection you'll develop with the natural world. Far too often, we view the plants in our environment as mere background scenery, failing to recognize their intricate beauty, their vital roles in the ecosystem, and the fascinating stories they have to tell. By taking the time to observe and study the plants around you, you'll gain a deeper understanding of the delicate balance that sustains life on our planet.Moreover, recognizing the plants in your immediate surroundings can also have practical benefits. Many plants have medicinal or culinary uses, and being able to identify them can open up a world of natural remedies and delicious culinary experiences. Additionally, understanding the specific plants that thrive in your local environment can help you make informed decisions about landscaping, gardening, and even urban planning, ensuring thatyou're supporting the natural systems that sustain us.To begin your journey of plant discovery, start by taking a closer look at the plants in your own backyard or neighborhood. Observe the shapes and colors of the leaves, the patterns of the flowers, and the unique characteristics that set each plant apart. Use field guides, online resources, or even consult with local botanists or naturalists to help you identify the species you encounter.As you delve deeper into the world of plant recognition, you'll likely be surprised by the sheer diversity of species that exist in even the most seemingly ordinary environments. From the towering oak trees that provide shade and shelter to the delicate wildflowers that dot the roadsides, each plant has its own story to tell and its own role to play in the intricate web of life.One of the most fascinating aspects of plant recognition is the opportunity to learn about the historical and cultural significance ofthe plants around you. Many plants have been used for centuries in traditional medicine, spiritual practices, or even as sources of food and fiber. By understanding the rich history and cultural traditions associated with the plants in your area, you'll gain a deeper appreciation for the interconnectedness of human and natural systems.Moreover, recognizing the plants around you can also be a powerful tool for conservation and environmental stewardship. By understanding the specific plants that thrive in your local ecosystem, you can take steps to protect and preserve these valuable resources, ensuring that they continue to provide vital ecosystem services and enrich the lives of future generations.As you continue to explore the world of plant recognition, you'll likely find that your understanding of the natural world around you deepens and expands in unexpected ways. You may discover new and unexpected uses for common plants, uncover the hidden stories and legends associated with particular species, or even stumble upon rare or endangered plants that require our protection.Ultimately, the act of recognizing the plants around you is not just an academic exercise, but a deeply rewarding and transformative experience that can enrich your life in countless ways. By cultivating a deeper connection with the natural world, you'll not only gain agreater appreciation for the complexity and beauty of our shared environment, but you'll also become a more informed and engaged steward of the precious resources that sustain us all.So, the next time you find yourself surrounded by the plants in your immediate vicinity, take a moment to pause, observe, and marvel at the hidden wonders that are waiting to be discovered. Who knows what fascinating stories and insights you might uncover, and how those discoveries might shape your understanding of the world around you.。
朋友圈配风景照句子英文
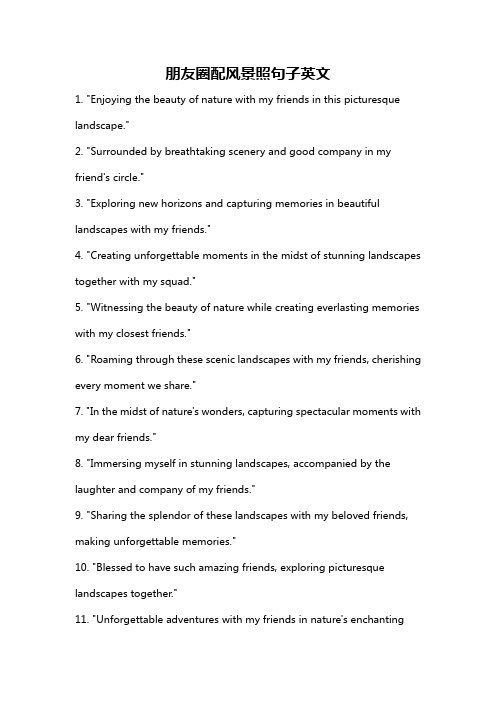
朋友圈配风景照句子英文1. "Enjoying the beauty of nature with my friends in this picturesque landscape."2. "Surrounded by breathtaking scenery and good company in my friend's circle."3. "Exploring new horizons and capturing memories in beautiful landscapes with my friends."4. "Creating unforgettable moments in the midst of stunning landscapes together with my squad."5. "Witnessing the beauty of nature while creating everlasting memories with my closest friends."6. "Roaming through these scenic landscapes with my friends, cherishing every moment we share."7. "In the midst of nature's wonders, capturing spectacular moments with my dear friends."8. "Immersing myself in stunning landscapes, accompanied by the laughter and company of my friends."9. "Sharing the splendor of these landscapes with my beloved friends, making unforgettable memories."10. "Blessed to have such amazing friends, exploring picturesque landscapes together."11. "Unforgettable adventures with my friends in nature's enchantingembrace."12. "Exploring the world's most breathtaking landscapes with my incredible friends."13. "Enjoying the serenity of this stunning landscape with my forever friends."14. "Capturing unforgettable memories amidst picturesque landscapes with my dear friends."15. "Escaping the ordinary and discovering the extraordinary alongside my closest friends."16. "Embracing the beauty of nature with my true friends in this mesmerizing landscape."17. "Making memories that will last a lifetime in these captivating landscapes with my friends."18. "Admiring the beauty of nature's artwork while surrounded by my loved ones."19. "Chasing adventures and capturing breathtaking landscapes with my adventurous friends."20. "Finding solace in the beauty of nature and the warmth of my friends' company."21. "Embracing the beauty of this stunning landscape while creating lifelong memories with my friends."22. "Exploring the path less traveled with my friends, amidst strikinglandscapes."23. "Sharing laughter, adventure, and the beauty of nature with my amazing friends in these picturesque landscapes."24. "Appreciating the wonders of nature and the joy of friendship in these breathtaking landscapes."25. "Every step in these scenic landscapes is sweeter with my best friends by my side."26. "In the company of my friends, discovering the hidden gems of nature's grandeur."27. "Journeying through nature's beauty with my friends, capturing moments that take our breath away."28. "Witnessing the magic of nature's palette with my best friends by my side."29. "Surrounded by incredible friends in this enchanting landscape, creating beautiful memories."30. "Grateful for friends who not only share the love for travel but also the love for nature's wonders."31. "Creating unforgettable memories with my friends while exploring mesmerizing landscapes around the world."32. "In the embrace of nature's splendor, cherishing the bond of friendship with my companions."33. "Finding inner peace while being in the presence of bothbreathtaking landscapes and amazing friends."34. "Capturing the essence of each place we visit, alongside my friends in the most stunning landscapes."35. "Roaming through nature's wonders with my friends, making every moment an extraordinary experience."36. "Finding joy and bliss in this beautiful landscape, accompanied by my closest friends."37. "Embracing nature's stunning beauty with my friends, making every journey a remarkable one."38. "In awe of nature's majesty, sharing striking landscapes with the best company of friends."39. "Laughing, enjoying, and exploring the world's beauty with my incredible friends."40. "Grateful for these cherished friendships that make every adventure in scenic landscapes so much more meaningful."。
搜索森林探险之女作文英文
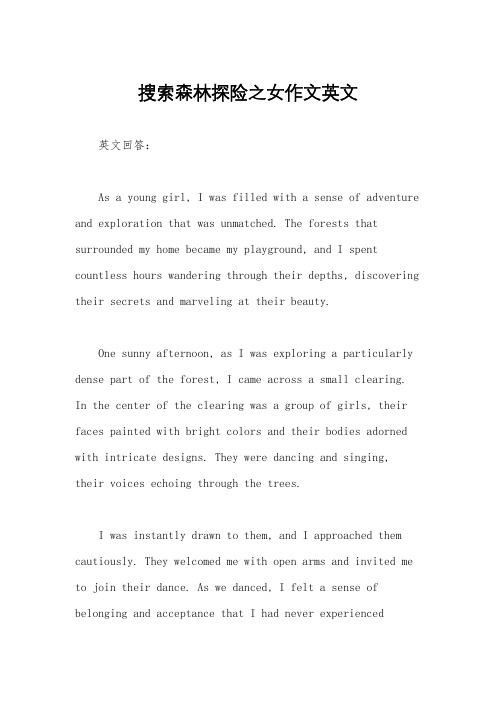
搜索森林探险之女作文英文英文回答:As a young girl, I was filled with a sense of adventure and exploration that was unmatched. The forests that surrounded my home became my playground, and I spent countless hours wandering through their depths, discovering their secrets and marveling at their beauty.One sunny afternoon, as I was exploring a particularly dense part of the forest, I came across a small clearing. In the center of the clearing was a group of girls, their faces painted with bright colors and their bodies adorned with intricate designs. They were dancing and singing,their voices echoing through the trees.I was instantly drawn to them, and I approached them cautiously. They welcomed me with open arms and invited me to join their dance. As we danced, I felt a sense of belonging and acceptance that I had never experiencedbefore.The girls introduced me to their leader, a wise and beautiful woman named Maya. Maya told me that they were part of a secret society of forest explorers, and that they were on a mission to protect the forest from harm.Maya explained that the forest was a living, breathing entity, and that it was home to countless creatures, both seen and unseen. She told me that the forest provided us with food, water, and shelter, and that it was our duty to protect it.I was inspired by Maya's words, and I vowed to do everything I could to help her and her followers protect the forest. Together, we embarked on countless adventures, exploring the hidden corners of the forest and learning its secrets. We built treehouses, tracked animals, and discovered ancient ruins.As the years passed, I grew into a strong and capable young woman, and I became one of the most trusted membersof Maya's society. I used my skills as an explorer to guide lost travelers, to rescue injured animals, and to defend the forest from those who sought to harm it.One day, I was leading a group of explorers through the forest when we came across a group of hunters who were illegally killing animals. I knew that I had to do something to stop them, so I charged into their camp and confronted them.The hunters were surprised by my boldness, and they hesitated for a moment. But then they regained their composure and attacked me. I fought back with all my strength, and eventually, I was able to defeat them.The other explorers were grateful for my help, and they praised me for my courage. But I knew that I was just doing what was right. The forest was my home, and I would do whatever it took to protect it.My adventures in the forest taught me many valuable lessons about life and the importance of protecting theenvironment. I learned that courage is not the absence of fear, but the willingness to act in spite of it. I learned that strength comes from unity, and that we can accomplish anything if we work together.And most importantly, I learned that the forest is a magical place, full of wonder and beauty. It is a place where anything is possible, and where dreams can come true.中文回答:作为一个年轻女孩,我充满了无与伦比的冒险和探索精神。
- 1、下载文档前请自行甄别文档内容的完整性,平台不提供额外的编辑、内容补充、找答案等附加服务。
- 2、"仅部分预览"的文档,不可在线预览部分如存在完整性等问题,可反馈申请退款(可完整预览的文档不适用该条件!)。
- 3、如文档侵犯您的权益,请联系客服反馈,我们会尽快为您处理(人工客服工作时间:9:00-18:30)。
to the complexity of the E-step. The E-step uses inference to complete the missing data; the inference process propagates messages which are explicit distributions over the set of state variables, so that their representation is exponential in the size of this set. In particular, if a join tree algorithm Jensen et al. 1990; Shenoy and Shafer 1990] is used for inference, the tree essentially reduces to one huge clique for each pair of consecutive time slices. This exponential cost is prohibitive in all but the simplest DBNs. This problem also appears in the simpler case of learning DBN parameters given the structure. For that setting, Boyen and Koller 1998a] propose an approximate E-step algorithm. This algorithm propagates approximate messages represented as factorized products over independent clusters. This representation allows the propagation of messages from one time slice to the other using a join tree with much smaller cliques than in the exact method. They show that the error in sufcient statistics resulting from approximation is small, and that the in uence on the progress of the learning algorithm is negligible. Even for small networks, order of magnitude speedups can easily be obtained. In this paper, we extend this technique to the problem of structure learning. In parametric EM, as used in Boyen and Koller 1998a], each family in the DBN is guaranteed to be contained in some clique in the clique tree for the two consecutive time slices. Thus, su cient statistics could easily be accumulated during the execution of the inference algorithm. In SEM, on the other hand, the results of the same inference process must be used for scoring a variety of di erent candidate structures. Each of these requires su cient statistics for a di erent set of events. While inference algorithms can in principle be used to compute the probability of any event, this procedure is fairly expensive, especially for a large number of arbitrary events. In Section 4, we propose a new approximation that circumvents this bottleneck. Roughly speaking, this approximation estimates the posterior probability of the event for which we want statistics by a product of small factors. As we show, this estimate is quite good, and can be computed e ciently as a by-product of the inference algorithm. Our nal contribution addresses a fundamental problem in learning models for dynamic systems. In order to learn models that are statistically robust and computationally tractable, we must often introduce hidden variables into our structure. These variables serve many roles: enabling the Markov property, capturing hidden in uences of the observables, etc. It is possible, in theory, to discover hidden variables simply by
1 Introduction
Many real world phenomena are naturally modeled as dynamic systems: the stock market, measurements of a patient's vital signs in an intensive care unit, vehicles on a freeway, etc. Knowledge of a system's dynamics is essential for many tasks, including prediction, monitoring, and the detection of anomalies.
Daphne Koller
Abstract
Dynamic Bayesian networks provide a compact and natural representation for complex dynamic systems. However, in many cases, there is no expert available from whom a model can be elicited. Learning provides an alternative approach for constructing models of dynamic systems. In this paper, we address some of the crucial computational aspects of learning the structure of dynamic systems, particularly those where some relevant variables are partially observed or even entirely unknown. Our approach is based on the Structural Expectation Maximization (SEM) algorithm. The main computational cost of the SEM algorithm is the gathering of expected sufcient statistics. We propose a novel approximation scheme that allows these su cient statistics to be computed e ciently. We also investigate the fundamental problem of discovering the existence of hidden variables without exhaustive and expensive search. Our approach is based on the observation that, in dynamic systems, ignoring a hidden variable typically results in a violation of the Markov property. Thus, our algorithm searches for such violations in the data, and introduces hidden variables to explain them. We provide empirical results showing that the algorithm is able to learn the dynamics of complex systems in a computationally tractable way.