Estimation and Rejection of Unknown Sinusoidal Disturbances Using a
一种改进的自适应匹配滤波方法
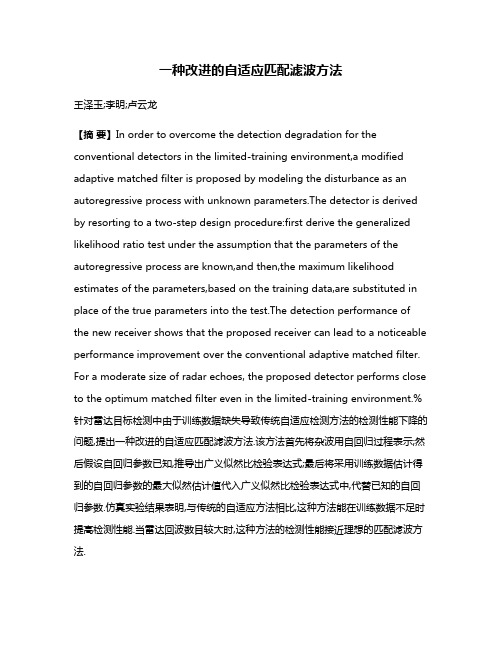
一种改进的自适应匹配滤波方法王泽玉;李明;卢云龙【摘要】In order to overcome the detection degradation for the conventional detectors in the limited-training environment,a modified adaptive matched filter is proposed by modeling the disturbance as an autoregressive process with unknown parameters.The detector is derived by resorting to a two-step design procedure:first derive the generalized likelihood ratio test under the assumption that the parameters of the autoregressive process are known,and then,the maximum likelihood estimates of the parameters,based on the training data,are substituted in place of the true parameters into the test.The detection performance of the new receiver shows that the proposed receiver can lead to a noticeable performance improvement over the conventional adaptive matched filter. For a moderate size of radar echoes, the proposed detector performs close to the optimum matched filter even in the limited-training environment.%针对雷达目标检测中由于训练数据缺失导致传统自适应检测方法的检测性能下降的问题,提出一种改进的自适应匹配滤波方法.该方法首先将杂波用自回归过程表示;然后假设自回归参数已知,推导出广义似然比检验表达式;最后将采用训练数据估计得到的自回归参数的最大似然估计值代入广义似然比检验表达式中,代替已知的自回归参数.仿真实验结果表明,与传统的自适应方法相比,这种方法能在训练数据不足时提高检测性能.当雷达回波数目较大时,这种方法的检测性能接近理想的匹配滤波方法.【期刊名称】《西安电子科技大学学报(自然科学版)》【年(卷),期】2018(045)001【总页数】6页(P12-16,82)【关键词】雷达检测;自适应匹配滤波;自回归建模【作者】王泽玉;李明;卢云龙【作者单位】西安电子科技大学雷达信号处理国家重点实验室,陕西西安710071;西安电子科技大学雷达信号处理国家重点实验室,陕西西安710071;西安电子科技大学雷达信号处理国家重点实验室,陕西西安710071【正文语种】中文【中图分类】TN953在协方差矩阵未知的杂波环境下对目标进行检测是雷达最基本的任务.通常,假设存在一组不含目标的训练数据来估计未知的杂波协方差矩阵.在高斯杂波环境下,文献[1]提出了基于广义似然比检验(Generalized Likelihood Ratio Test, GLRT)的检测方法,该方法需要求得所有未知参数的最大似然估计.为了减少计算量,文献[2]提出了自适应匹配滤波(Adaptive Matched Filter, AMF)方法,首先假设杂波的协方差矩阵已知,推导出广义似然比检验表达式,然后将采用训练数据估计得到的协方差矩阵的最大似然估计值代入检验表达式中来代替已知的协方差矩阵.随后,其他的检测方法[3-6](如Rao检测,Wald检测等)被相继提出.然而,这些检测方法至少需要两倍系统自由度的训练数据来估计杂波的协方差矩阵[7-9].在实际检测环境中,这个条件难以满足.利用杂波的性质能有效地解决训练数据缺失情况下检测性能下降的问题.众所周知,杂波可以用阶数较低的自回归过程来表示.文献[10]利用自回归频谱估计提出一种自适应滤波方法来抑制杂波.在色高斯噪声环境下,假设目标信号已知,文献[11]将噪声用自回归过程来表示并采用广义似然比检验准则来进行检测.考虑到文献[11]中的目标模型较为简单,文献[12]针对未知幅度的信号,提出一种自回归广义似然比检测方法.渐进性能分析表明,该检测方法具有渐进恒虚警特性.文献[13]在完全均匀场景和不同距离单元的杂波协方差矩阵结构不同的非均匀场景中,针对自回归过程的阶数已知和未知两种情形设计了4种基于广义似然比检验的检测器.近几年,基于多通道自回归过程的检测器也得到了广泛的研究[14-16].针对训练数据缺失情况下传统检测方法检测性能下降的问题,笔者提出基于自回归的自适应匹配滤波方法.该方法首先将杂波用自回归过程来表示,然后假设自回归参数已知,利用一步广义似然比检验准则设计检测器,最后利用训练数据对自回归参数进行估计,并将得到的自回归参数的最大似然估计值代入一步广义似然比检验表达式中,得到最终的基于自回归的自适应匹配滤波器.假设回波包含N个相干脉冲,目标检测问题可以用以下的二元假设检验来表示:其中,z0∈CN×1,表示待检测距离单元的数据;zt∈CN×1,t=1,…,K,表示不含目标的一组训练数据;nt∈ CN×1,t=0,…,K,是均值为零、协方差矩阵为R的独立复高斯向量;p= [1,exp(j Ω),…,exp(j(N-1)Ω)]T,是导向矢量;Ω是目标多普勒;α表示未知的目标幅度.假设杂波信号nt可以用阶数为M的自回归过程来表示:其中,a(m)=[a(1),…,a(M)]T,是复自回归参数向量;wt(l)表示均值为零、方差为σ2的复白高斯噪声,σ2是未知常量.为了解决上述问题,采用自适应匹配滤波(即两步广义似然比准则)进行检测.首先假设自回归参数a和σ2已知,基于广义似然比准则推导检验表达式;然后采用训练数据对a和σ2进行估计,将得到的最大似然估计值代入检验表达式中得到最终的结果.当N≫M时,z0在H0和H1条件下的概率密度函数可以分别表示为[17]其中,(·)H表示共轭转置.ut=[zt(M+1),…,zt(N)]T,t=0,…,K,是 N-M 维的复列向量;q= [p(M+1),…,p(N)]T,是 N-M 维的复列向量是 (N-M)× M维的矩阵是 (N-M)× M维的矩阵.首先假设a和σ2已知,推导广义似然比检验表达式:其中,η表示检测门限.由式(4)和式(5)可以看出,参数α的最大似然估计可以通过对表达式J(α)= [u0+ Y0a- α(q+ P a)]H [u0+ Y0a- α(q+ P a)]求α的最小值得到.将J(α)展开,可以得到其中,Re[·]表示取实部.显然,当包含绝对值的第1项等于0时,表达式J(α)取得最小值.因此,α的最大似然估计值为将α的最大似然估计值即式(7)代入到表达式J(α)中,得到其中,H=I-(q+P a)(q+P a)H/[(q+P a)H(q+P a)],是一个幂等矩阵.将式(3)~(4)和式(8)代入式(5)中,得到基于广义似然比的检验表达式其中,=H u0,=H Y0.利用训练数据对自回归参数a和σ2进行估计,并将得到的自回归参数的最大似然估计值代入一步广义似然比检验表达式(9)中代替已知的a和σ2.训练数据zt的联合概率密度函数可以表示为对联合概率密度函数取对数,得到对式(11)关于σ2求导并令导数等于零,即可得到σ2的最大似然估计值将式(12)代入到式(11)中,可得从式(13)可以看出,参数a的最大似然估计可以通过对表达式求关于a的最小值得到.将表达式Q(a)展开,可得其中,由于SY Y是非负定的,且式(14)中的第2项和第3项与a无关,可以得到[18]参数a的最大似然估计为将a和σ2的最大似然估计值(即式(12)和式(16))代入式(9)中并化简,得到基于自回归的自适应匹配滤波器:其中,ηAR-AMF表示检测门限.对所提出的基于自回归的自适应匹配滤波方法的检测性能进行分析.仿真参数设置为: Ω=1,a= [-0.25+ 0.25j,0.3]T,σ2=2,Pfa= 10-2.信干噪比定义为RSINR= pHR-1p,R表示杂波的协方差矩阵,可以通过a和σ2确定.检测概率和门限分别通过 100/ Pfa和 1 000/ Pfa次独立的蒙特卡罗实验确定.图1和图2分别为K=2和K=20情况下,N取不同值时检测概率随着信干噪比变化的曲线.为了进行对比,理想的匹配滤波器(Matched Filter, MF)[2]的检测概率曲线也在图中画出.虽然理想的匹配滤波器在实际中无法实现,然而其提供了对比的基准.以下的实验结果均采用理想匹配滤波器检测概率的理论值.从图1和图2可以看出,随着脉冲数N的增加,基于自回归的自适应匹配滤波方法的检测性能逐渐提高.由图1可知,当 K=2,N=8,Pd=0.9 时,笔者提出的方法相对于理想的匹配滤波器的性能损失约为 5 dB;当脉冲数N增加到40时,性能损失减小到 1 dB;当脉冲数N增加到100时,性能损失小于 1 dB.因此,当脉冲数较大时,即使在训练数据严重缺失的情况下,笔者提出的方法仍然能获得与理想的匹配滤波器相近的检测性能.从图2可以看出,当 K=20,N>40 时,笔者提出的方法相对于理想匹配滤波器的性能损失可以忽略.因此,当脉冲数不是很小时,笔者提出的方法是训练数据缺失情况下检测目标的一种有效方法.图3表示N=30,K=5,30,60,150的情况下,检测概率随信干噪比变化的曲线.为了进行对比,传统的广义似然比检测器[1]和自适应匹配滤波器[2]的检测性能也在图中画出.从图中可以看出,随着信干噪比的增加,笔者提出的方法(AR-AMF)、传统的广义似然比检测器(GLRT)和自适应匹配滤波器(AMF)的检测性能均逐渐提高.这是由于训练数据的增加使得估计得到的参数值更加精确.在图3(a)中,传统的广义似然比检测器和自适应匹配滤波并没有画出,这是由于 K<N 使得传统方法中的采样协方差矩阵奇异.从图3(b)可以看出,当训练数据较小时,传统的广义似然比检测器和自适应匹配滤波方法的检测性能损失严重,笔者提出的方法相比于传统的方法有明显的性能增益.这是由于笔者提出的方法利用了杂波的性质,使得检测性能有所提高.随着训练数据的增加,传统方法相对于笔者提出的方法的性能损失逐渐减少,而笔者提出的方法仍优于传统的方法.由图3(c)和图3(d)可知,当 K=60,检测概率 Pd=0.9 时,笔者提出的方法相对于传统方法的增益差约为 3 dB;当训练数据 K=5N,Pd=0.9 时,笔者提出的方法与传统自适应匹配滤波器的性能增益差小于 1 dB.从以上仿真可知,在训练数据缺失的情况下,笔者提出的基于自回归的自适应匹配滤波方法通过利用杂波的性质使检测性能有所改善.仿真结果验证了笔者提出方法的有效性.针对训练数据缺失情况下传统自适应检测方法检测性能下降的问题,笔者提出一种基于自回归的自适应匹配滤波方法.该方法首先利用杂波的性质,将杂波用一个自回归过程表示;然后假设自回归参数已知,推导广义似然比检验表达式;最后利用训练数据估计未知的自回归参数,并将得到的自回归参数的最大似然估计值代入广义似然比检验表达式中,得到基于自回归的自适应匹配滤波检测器.仿真实验表明,在只有少量训练数据存在的情况下,笔者提出的方法优于传统的自适应检测方法.同时,当脉冲数较大时,笔者提出方法的检测性能接近理想的匹配滤波器.下一步的工作拟解决脉冲数较小情况下的自适应检测问题.[1] KELLY E J. An Adaptive Detection Algorithm[J]. IEEE Transactions on Aerospace and Electronic Systems, 1986, 22(1):115-127.[2] ROBEY F C, FUHRMANN D R, KELLY E J, et al. A CFAR Adaptive Matched Filter Detector[J]. IEEE Transactions on Aerospace and Electronic Systems, 1992, 28(1): 208-216.[3] de MAIO A. Rao Test for Adaptive Detection in Gaussian Interference with Unknown Covariance Matrix[J]. IEEE Transactions on Signal Processing, 2007, 55(7): 3577-3584.[4] de MAIO A. A New Derivation of the Adaptive Matched Filter[J]. IEEE Signal Processing Letters, 2004, 11(10): 792-793.[5] KRAUT S, SCHARF L L. CFAR Adaptive Subspace Detector is a Scale-invariant GLRT[J]. IEEE Transactions on Signal Processing, 1999, 47(9):2538-2541.[6] PULSONE N B, RADER C M. Adaptive Beamformer OrthogonalRejection Test[J]. IEEE Transactions on Signal Processing, 2001, 49(3): 521-529.[7] REED I S, MALLETT J D, BRENNAN L E. Rapid Convergence Rate in Adaptive Arrays[J]. IEEE Transactions on Aerospace and Electronic Systems, 1974, 10(6): 853-863.[8] 周宇, 张林让, 刘楠. 贝叶斯雷达自适应检测算法研究[J]. 西安电子科技大学学报, 2012, 39(1): 36-42.ZHOU Yu, ZHANG Linrang, LIU Nan. Research on Bayesian Radar Adaptive Detection[J]. Journal of Xidian University, 2012, 39(1): 36-42.[9] 高永婵, 廖桂生, 朱圣棋. 复合高斯噪声中知识辅助的贝叶斯Rao检测方法[J]. 西安电子科技大学学报, 2013, 40(6): 46-51.GAO Yongchan, LIAO Guisheng, ZHU Shengqi. Knowledge-aided Bayesian Rao Detection Approach in Compound Gaussian Noise[J]. Journal of Xidian University, 2013, 40(6): 46-51.[10] BOWYER D E, RAJASEKARAN P K, GEBHART W W. Adaptive Clutter Filtering Using Autoregressive Spectral Estimation[J]. IEEE Transactions on Aerospace and Electronic Systems, 1979, 15(4): 538-546.[11] KAY S M. Asymptotically Optimal Detection in Unknown Colored Noise via Autoregressive Modeling[J]. IEEE Transactions on Acoustics, Speech, and Signal Processing, 1983, 31(4): 927-940.[12] SHEIKHI A, NAYEBI M M, AREF M R. Adaptive Detection Algorithm for Radar Signals in Autoregressive Interference[J]. IEE Proceedings: Radar, Sonar and Navigation, 1998, 145(5): 309-314.[13] ALFANO G, de MAIO A, FARINA A. Model-based Adaptive Detectionof Range-spread Targets[J]. IEE Proceedings: Radar, Sonar and Navigation, 2004, 151(1): 2-10.[14] WANG P, WANG Z, LI H B, et al. Knowledge-aided Parametric Adaptive Matched Filter with Automatic Combining for Covariance Estimation[J]. IEEE Transactions on Signal Processing, 2014, 62(18): 4713-4722.[15] BOUKABAA T, ZOUBIRB A M, BERKANIA D. Parametric Detection in Non-stationary Correlated Clutter Using a Sequential Approach[J]. Digital Signal Processing: a Review Journal, 2015, 36(C): 69-81.[16] GAO Y C, LIAO G S, ZHU S Q, et al. Generalised Persymmetric Parametric Adaptive Coherence Estimator for Multichannel Adaptive Signal Detection[J]. IET Radar, Sonar and Navigation, 2015, 9(5): 550-558. [17] HAYKIN S S, STEINHARDT A. Adaptive Radar Detection and Estimation[M]. New York: Wiley-Interscience, 1992.[18] LI J, HALDER B, STOICA P, et al. Computationally Efficient Angle Estimation for Signals with Known Waveforms[J]. IEEE Transactions on Signal Processing, 1995, 43(9): 2154-2163.。
心理学专业英语词汇
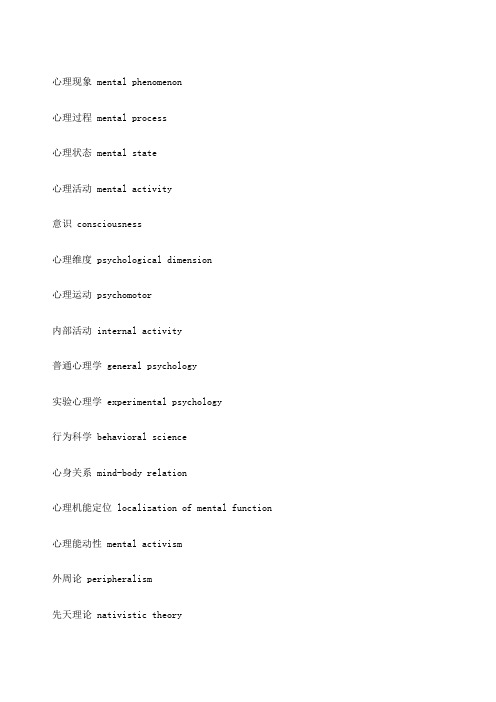
心理现象 mental phenomenon心理过程 mental process心理状态 mental state心理活动 mental activity意识 consciousness心理维度 psychological dimension心理运动 psychomotor内部活动 internal activity普通心理学 general psychology实验心理学 experimental psychology行为科学 behavioral science心身关系 mind-body relation心理机能定位 localization of mental function 心理能动性 mental activism外周论 peripheralism先天理论 nativistic theory强调遗传素质决定人心理的产生与发展。
遗传 heredity目的论 teleology认为生物和人类的活动受一定目的的引导。
活动 activity活动理论 activity theory认知心理学 cognitive psychology认知 cognition相对于情感、意志等心理过程的所有认识过程的总称。
包括知觉、注意、表象、学习记忆、问题解决、思维和言语等心理过程。
认知过程 cognitive process认知结构 cognitive structure元认知 metacognition认知失调 cognitive dissonance认知地图 cognitive map认知技能 cognitive skill认知方式 cognitive style信息 information信息论 information theory信息加工 information processing信息加工心理学 information processing psychology 信息加工理论 information processing theory信息加工模型 information processing model中央处理器模型 central processor model信息储存 information storage信息提取 information retrieval人工智能 artificial intelligence, AI计算机类比 computer analogy计算机模拟 computer simulation计算机模型 computer model唯心主义心理学 idealistic psychology意动心理学 act psychology唯意志论 voluntarism唯灵论 spiritualism强调超自然精神作用。
心理学专业英语词汇汇总
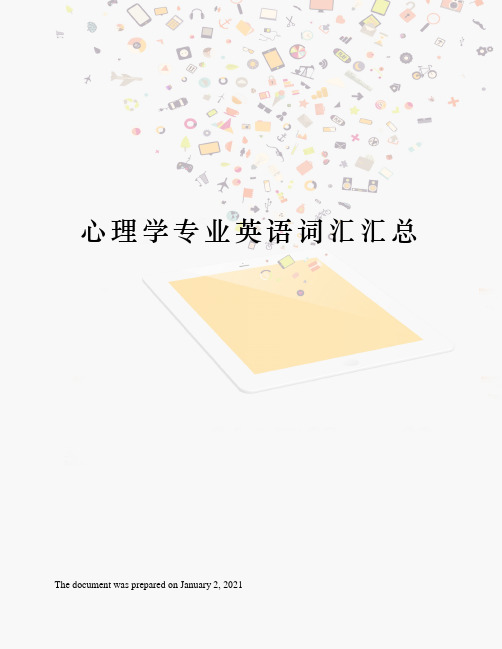
心理学专业英语词汇汇总The document was prepared on January 2, 2021心理现象 mental phenomenon心理过程 mental process心理状态 mental state心理活动 mental activity意识 consciousness心理维度 psychological dimension心理运动 psychomotor内部活动 internal activity普通心理学 general psychology实验心理学 experimental psychology行为科学 behavioral science心身关系 mind-body relation心理机能定位 localization of mental function 心理能动性 mental activism外周论 peripheralism先天理论 nativistic theory强调遗传素质决定人心理的产生与发展.遗传 heredity目的论 teleology认为生物和人类的活动受一定目的的引导.活动 activity活动理论 activity theory认知心理学 cognitive psychology认知 cognition相对于情感、意志等心理过程的所有认识过程的总称.包括知觉、注意、表象、学习记忆、问题解决、思维和言语等心理过程.认知过程 cognitive process认知结构 cognitive structure元认知 metacognition认知失调 cognitive dissonance认知地图 cognitive map认知技能 cognitive skill认知方式 cognitive style信息 information信息论 information theory信息加工 information processing信息加工心理学 information processing psychology 信息加工理论 information processing theory信息加工模型 information processing model中央处理器模型 central processor model信息储存 information storage信息提取 information retrieval人工智能 artificial intelligence, AI计算机类比 computer analogy计算机模拟 computer simulation计算机模型 computer model唯心主义心理学 idealistic psychology意动心理学 act psychology唯意志论 voluntarism唯灵论 spiritualism强调超自然精神作用.心灵学 parapsychology心灵决定论 psychic determinism心灵致动 psychokinesis, PK心理技术学 psychotechnics内省 introspection内省法 introspective method直觉主义 intuitionalism条件反射 conditioned reflex, CR非条件反射 unconditioned reflex经典性条件作用 classical conditioning工具性条件作用 instrumental conditioning 操作性条件作用 operant conditioning操作主义 operationalism操作性定义 operational definition斯金纳箱 Skinner box迷箱 puzzle box强化 reinforcement二级强化 secondary reinforcement强化理论 reinforcement theory反馈 feedback生物反馈 biofeedback次级控制 secondary control皮肤电传导 skin conductance皮肤电反应 galvanic skin response测谎器 lie detector心理物理学 psychophysics心理物理学方法 psychophysical method 评定量表法 rating scale method阈限 threshold, limen刺激阈限 stimulus threshold绝对感受性 absolute sensitivity绝对阈限 absolute threshold差别感受性 differential sensitivity差别阈限 differential threshold最小可觉差 just noticeable difference, 极限法 limit method 下限 lower limit差别阈限法 differential limen method上差别阈 upper difference threshold下差别阈 lower difference threshold调整法 method of adjustment不确定间距 interval of uncertainty恒定刺激法 method of constant stimulus次数法 frequency method等距法 method of equal interval最小变化法 method of minimal change递增系列 ascending series递减系列 descending series预期误差 anticipation error等级法 ranking method数值评估法 method of magnitude estimation分段法 fractionation method误差 error平均误差法 method of average error恒定误差 constant error偶然误差 accidental error主观相等点 point of subjective equality, PSE 正误法 method of right and wrong cases二分法 method of dichotomic classification 双重分离 double dissociation消除法 method of elimination阶梯法 staircase method对偶比较法 method of paired comparison单一刺激法 method of single stimulus 费希纳定律 Fechner's law韦伯分数 Weber fraction韦伯比例 Weber ratio韦伯定律 Weber's law韦伯-费希纳定律 Weber-Fechner law幂函数定律 power function law史蒂文斯定律 Stevens' law对数定律 logarithmic law刺激变量 stimulus variable标准刺激 standard stimulus近端刺激 proximal stimulus等距变量 equal interval variable顺序变量 ordinal variable反应变量 response variable被试变量 subject variable反应 response反应偏向 response bias自然实验 natural experiment开窗实验 experiment of open window 双盲实验 double blind experiment准实验 quasi-experiment实验设计 experimental design实验组 experimental group, EG控制组 control group, CG又称“对照组”.控制 control控制变量 controlled variable独立组设计 independent group design 组内设计 within-group design组间设计 between-group design匹配组设计 matched-group design拉丁方设计 Latin square design随机组设计 random group design混合设计 mixed design反应时 reaction time, RT简单反应时 simple reaction time选择反应时 choice reaction time选择时间 selection time辨别反应时 discriminative reaction time 迫选法 forced-choice method口头报告 verbal report自我观察 self-observation观察法 observational method模拟法 simulation method印象法 impression method传记法 biographical method访谈法 interview method问卷法 questionnaire表情法 method of expression有无法 yes-no method提示法 anticipation method抽象分析法 method of abstract analysis信号检测理论 signal detection theory信号检测 signal detection信号噪声分配 signal-to-noise distribution似然比 likelihood ratio内部噪声 internal noise正确否定 correct rejection击中 hit肯定判断 affirmative judgement虚报 false alarm接受者操作特征曲线 receiver operating characteristic curve, ROC curve 等感受性曲线 isosensitivity curve感觉 sensation感觉道 sense modality跨通道匹配 cross-modality matching感觉运动 sensorimotor感觉适应 sensory adaptation感觉剥夺 sensory deprivation感觉阈限 sensory limen感觉属性 attribute of sensation感觉器官相互作用 interaction of sense organs 感官特殊能量说 law of specific sense energy 特殊神经能量 specific nerve energy特异说 theory of idiosyncrasy视觉 vision瞳孔 pupil人工瞳孔 artificial pupil视网膜 retina物体大小 object size网象大小 retinal size视网膜对称点 corresponding retinal points两个视网膜重叠起来彼此重合的点.注视点 fixation point视角 visual angle视野 visual field视野计 perimeter中央视觉 central vision中央窝视觉 foveal vision用眼睛中央窝的视网膜区注视物体所产生的视觉.周边视觉 peripheral vision盲点 blind spot中心盲 central scotoma可见光 visible light强度 intensity照度 illuminance视网膜照度 retinal illuminance明度 brightness明度对比 brightness contrast亮度 luminance视见函数 luminosity function又称“光亮度函数”.亮度对比 luminance contrast亮暗比 light-dark ratio视觉阈限 visual threshold能见度 visibility能见度曲线 visibility curve等能光谱 equal energy spectrum光谱光效率曲线 spectral luminous efficiency curve 明视觉 photopic vision暗视觉 scotopic vision视觉适应 visual adaptation明适应 bright adaptation暗适应 dark adaptation暗适应曲线 dark adaptation curve 马赫带 Mach band对比 contrast同时对比 simultaneous contrast 浦肯野现象 Purkinje phenomenon 颜色视觉 color vision颜色 color彩色 chromatic color非彩色 achromatic color光谱色 spectral color基色 fundamental color补色 complementary color原色 primary color三原色 three primary colors三色视觉 trichromatism表面色 surface color立体色 bulky color又称“膨胀色”.容量色 volume color饱和度 saturation颜色饱和度 color saturation色度 chromaticity色度图 chromaticity diagram色区 color zone色温 color temperature色误差 chromatic error显色指数 color-rendering index 单色仪 monochromator产生单一颜色光的仪器.色环 color circle色轮 color wheel色表系 color appearance system颜色方程 color equation颜色立体 color solid芒塞尔颜色立体 Munsell color solid 颜色四方形 color square颜色三角 color triangle颜色爱好 color preference颜色宽容度 color tolerance颜色适应 chromatic adaptation颜色匹配 color matching同色异谱匹配 metameric matching颜色对比 color contrast颜色混合 color mixture双眼混色 binocular color mixture加色混合 additive color mixture减色混合 subtractive color mixture颜色混合律 law of color mixture补色律 law of complementary color中间色律 law of intermediary color代替律 law of substitution色诱导 color induction诱导色 induced color二色视觉 dichromatic vision斯特鲁普效应 Stroop effect亥姆霍茨视觉说 Helmholtz's theory of vision 黑林视觉说 Hering's theory of vision视觉双重说 duplicity theory of vision又称“双视觉理论”.阶段说 stage theory发生说 genetic theory色弱 color weakness红色弱 red weakness色盲 color blindness红色盲 red blindness绿色盲 deuteranopia又称“乙型色盲”.红绿色盲 red-green blindness, protanopia 又称“甲型色盲”.全色盲 monochromatism又称“单色视觉”.蓝色盲 tritanopia又称“丙型色盲”.蓝黄色盲 blue-yellow blindness视敏度 visual acuity分辨 discrimination朗多环视标 Landolt ring曾称“兰道环视标”.闪烁临界频率 critical flicker frequency, CFF 闪烁光度法 flicker photometry闪光融合器 flicker-fusion apparatus闪光盲 flash blindness后象 after-image负后象 negative after-image正后象 positive after-image视觉后象 visual after-image听觉后象 auditory after-image运动后象 movement after-image动觉后效 kinesthetic after-effect麦科洛效应 McCollough effect听觉 hearing, audition单耳听觉 monaural hearing双耳听觉 binaural hearing心理声学 psychoacoustics内耳 inner ear中耳 middle ear耳蜗 cochlea耳膜 eardrum半规管 semicircular canal频率 frequency振幅 amplitude of vibration主波长 dominant wavelength基线时间 baseline time反映 reflection反射系数 reflection coefficient 基音 fundamental tone纯音 pure tone复合音 compound tone倍音 overtone。
随机预测控制经典参考文献2

1971[5] Kushner,H.J. Introduction to stochastic control. Holt, Rinehartand Winston, 1971.1983[24] T. Morozan, “Stabilization of some stochastic discrete-time control-systems,”Stochast. Anal. Applicat. , vol. 1, no. 1, pp. 89–116, 1983.1986[12] Robert FS.1986.Stochastic optimal control:theory and application[M].Wiley.[] Kumar,P.R.,&Varaiya,P. Stochastic systems : estimation, identification, and adaptive control. Prentice Hall.19861987[16] D.W. Clarke, C. Mothadi, and P.S. Tuffs. Generalized predictive control. Auto-matica , 23:137–160, 1987.1997[10] J. Birge and F. Louveaux, Introduction to Stochastic Programming[M]. Springer, New York, 1997.[9] McLachlan GJ, Thriyambakam Krishnan. The EM algorithm and extensions[M]. Wiley.1997.[6]Meadows ES.Dynamic Programming and Model Predictive Control[C].Proceedings of the American Control Conference,1635-1639.1997.[2] Lee J,Yu Z. Worst-case formulation of model predictive control for systems with bounded parameters[J].Automatica 33,763–781. 1997.1998[6] Schwarm A,Nikolaou M.1998.Chance constrained model predictive control[J].AIChE Journal,45,1743–1752.[21] J.H. Lee and B.L. Cooley. Optimal feedback control strategies for state-space systems with stochastic parameters. IEEE Trans. Autom. Control, 43(10):1469–1475, 1998.[14] P.O.M. Scokaert and D.Q. Mayne. Min-max feedback model predictive control for constrained linear systems. IEEE Trans. Autom. Control , 43:1136–1142, 1998.[25] Koroleva N. Robust stability of uncertain stochastic differential delay equations [J]. Systems & Control Letters,1998,35(9):325-336.[] Kamen, E. W., & Su, J. K. Introduction to optimal estimation. London, UK:Springer.1999. 1999[4] Bemporad A, Morari M. Robust model predictive control: A survey[J]. Robustness in Identification and Control, 1999, 245: 207-226.[5] Blanchini F. Set invariance in control[J]. Automaica, 1999,35(11): 1747-1767.2000[3] Mayne D Q, Rawlings J B, Rao C V. Constrained model predictive control: Stability and optimality[J]. Automatica, 2000,36(6): 789-814.2001[10] 杜晓宁,席裕庚,李少远.约束预测控制的一种快速算法[J].上海交通大学学报,2001,35(11):1624-1627.Du X N, Xi Y G, Li S Y. Fast algorithm of constrained model predictive control[J]. Journal of Shanghai Jiaotong University, 2001, 35(11): 1624-1627.[11] Chisci L, Rossiter J A, Zappa G. Systems with persistent disturbance: Predictive control with restricted constraints[J]. Automatica, 2001, 37(7): 1019-1028.[23] Gershon R,Shaked U. H ∞control and filtering of discrete-time stochastic systems with multiplicative noise[J].Automatica,2001,37(3):409-417.[] I. Batina, A. A. Stoorvogel, and S. Weiland. Stochastic disturbance rejection in model predictive control by randomized algorithms. In proceedings of American Control Conference, pages 732–737, 2001.[1] I. Batina, A. A. Stoorvogel, and S. Weiland. Feed-back model predictive control by randomized algorithms. In proceedings of European Control Conference, pages 3185–3189, 2001.[2] I. Batina, A. A. Stoorvogel, and S. Weiland. Model predictive control by randomized algorithms for systems with constrained inputs and stochastic disturbances. IEEE Transactions on Automatic Control, December 2001.[11] D. H. van Hessem, C. W. Scherer, and O. H. Bosgra, “LMI-based closed-loop economic optimization of stochastic process operation under state and input constraints,”in Proc. 40th IEEE Conf. Decision Control, Orlando, FL, 2001, pp. 4228–4233.2002[17] P. Li, M. Wendt, and G. Wozny. A probabilistically constrained model predictive controller. Automatica, 38(7):1171–1176, 2002.[3] Kouvaritakis B,Cannon M,Tsachouridis V. Recent developments in stochastic MPC and sustainable development[J].Annual Reviews in Control,2002,volume 28,23–35. 2002.[4] I. Batina, A. A. Stoorvogel, and S. Weiland. Optimal control of linear, stochastic systems with state and input constraints. In proceedings of European Control Conference, pages 1564–1569, 2002.[7] vanHessem,D.H.,&Bosgra,O.H. A conic reformulation of model predictive control including boundedand stochastic disturbances understate and input constraints. In Proc.41st IEEE conf.Decision control (pp.4643 4648), 2002.2003[9] Liu B, Xi Y G. An aggregation based robust model predictive controller[J]. Acta Automatic Sinica, 2003, 29(6): 801-808.[19] A.J. Felt. Stochastic linear model predictive control using nested decomposition.In Proc. American Control Conf., pages 3602–3607, 2003.[15] A. Bemporad, F. Borrelli, and M. Morari. Min-max control of constrained uncertain discrete-time linear systems. IEEE Trans. Automatic Control , 48(9):1600–1606, 2003.[] vanHessem,D.H.,&Bosgra,O.H. A full solution to the constrained stochastic closed-loop MPC problem via state and innovations feedback and its receding horizon implementation, In Proceedings of the 42nd IEEE conference on decision and control,vol.1,(pp.929–934).2003.2004[12] Foss S, Konstantopoulos T. An overview of some stochastic stability methods[J]. Journal of Operations Research of Japan, 2004, 47(4): 275-303.[18] I. Batina. Model predictive control for stochastic systems by randomized algorithms . PhD thesis, Technische Universiteit Eindhoven, 2004.[24] Zhang WeiHai. On stabilizability and exact observability of stochastic systems with their applications [J], Automatic,2004,40(7):87-94.[7] Munoz D de la Pena, Alamo T, Bemporad A, Camacho EF. 2004. A Dynamic Programming Approach for Determining the explicit solution of linear MPC controllers[C]. Proceedings of 43rd IEEE Conference on Decision and Control, 2479-2484.[1] Batina,I. Model predictive control for stochastic systems by randomized algorithms. Ph.D.thesis, Technische Universiteit Eindhoven,2004.[4] Kouvaritakis B., Cannon M., Tsachouridis V. Recent developments in stochastic MPC and sustainable development. Annual Reviewsin Control, 28(1),23 35. 2004.[15] J. Dupaˇcova, G. Consigli, and S. W. Wallace, “Scenarios for multistage stochastic programs,”Ann. Operat. Res., vol. 100, pp. 25–53, 2004.[24] Zhang WeiHai.On stabilizability and exact observability of stochastic systems with their applications[J],Automatic,40(7):87-94, 2004.2005[13] 方洋旺.随机系统最优控制[M].北京:清华大学出版社,2005.Fang Y W. Optimal control for stochastic system[M]. Beijing: Tsinghua University Press, 2005.[4]Munoz D de la Pena,Bemporad A,Alamo T. Stochastic Programming Applied to Model Predictive Control[C].Proceeding of the 44th IEEE CDC,1361-1366.2005.[] Yan, J., & Bitmead, R. R. Incorporating state estimation into model predictive control and its application to network traffic control.Automatica, 595–604. 2005.2006[31] Couchman P, Kouvaritakis B, Cannon M. MPC on state space models with stochastic input map [C]. Decision and Control, 2006 45th IEEE Conference on. IEEE, 2006: 3216-3221.[20] B. Kouvaritakis, M. Cannon, and P. Couchman. MPC as a tool for sustain-able development integrated policy assessment. IEEE Trans. Autom. Control ,51(1):145–149, 2006.[47] Couchman PD,Cannon M,Kouvaritakis B. Stochastic MPC with inequality stability constraints [J]. Automatica, 2006, 42(6):2169-2174.Couchman, P., Cannon, M., & Kouvaritakis, B. (2006). Stochastic model predictive control with nonlinear models. Automatica , submitted for Publication.[3] Couchman P, Kouvaritakis B, Cannon, M. MPC on state space models with stochastic input map. InProc. 45th IEEE Conf. Decision and Control (pp.3216 3221).20062007[28] Cannon M, Couchman P, Kouvaritakis B. MPC for stochastic systems [J]. Assessment and Future Directions of Nonlinear Model Predictive Control, 2007: 255-268.[5] Cannon M,Couchman P,Kouvaritakis B. MPC for stochastic systems.Assessment and Future Directions of Nonlinear Model Predictive Control[J].Springer LNCIS,vol 358,255-268.2007.[11]Maciejowski JM,Visintini AL,Lygeros J. NMPC for Complex Stochastic Systems Using a Markov Chain Monte Carlo Approach [J]. Springer-Verlag, Assessment and Future Directions, LNCIS 358,pp.269-281. 2007.[3] Bertsimas, D., & Brown, D. B. (2007). Constrained stochastic LQC: a tractable approach. IEEE Transactions on Automatic Control, 52(10),1826–1841.[5]Primbs, J.A. A soft constraint approach to stochastic receding horizon control . InProc . 46th IEEE Conf.Decision Control. 2007.2008[26] Cannon M, Kouvaritakis B, Couchman P. [C]. Proceedings of 17th IFAC World Congress. 2008: 15321-15326.[9] Wang C, Ong C J, Sim M. Constrained linear system with disturbance: convergence under disturbance feedback.Au-tomatica, 2008, 44(10): 2583--2587[16] G. C. Goodwin, J.stergaard, D. E. Quevedo, and A. Feuer, “A vector quantization approach to scenario generation for stochastic NMPC,”in Int. Workshop Assess. Future Direct. Nonlinear Model Predict. Con-trol , Pavia, Italy, 2008.2009[11] Mark Cannon, Basil Kouvaritakis, Xingjian Wu, Model predictive control for systems with stochastic multiplicative uncertainty and probabilistic constraints, Automatica, V olume 45, Issue 1, January 2009, Pages 167-172[13] Cannon M, Kouvaritakis B, Ng D. Probabilistic tubes in linear stochastic model predictive control [J]. Systems & Control Letters, 2009, 58(10): 747-753.[4] Cannon M, Kouvaritakis B, Wu X J. Probabilistic constrained MPC for multiplicative and additive stochastic uncertainty.IEEE Transactions on Automatic Control,54(7),1626–1632. 2009[4] Cannon M. Ng, D,Kouvaritakis, B. Successive linearization NMPC for a class of stochastic nonlinear systems. InLNCIS: V ol. 384. Nonlinear model predictive control :towards new challenging applications (pp.249 262).2009.[6] Primbs J, Sung C H. Stochastic receding horizon control of constrained linear systems with state and control multiplicative noise[J]. IEEE Transactions on Automatic Control, 2009, 54(2):221-230.[8] Hokayem P, Chatterjee D, Lygeros J. On stochastic model predictive control with bounded control inputs[C]//Proceedings of the Combined 48th IEEE Conference on Decision & Control and 28th Chinese Control Conference. Piscataway, NJ, USA: IEEE, 2009: 6359-6364.[8]Wang C, Ong C J, Sim M. Convergence properties of con-strained linear system under MPC control law using a±nedisturbance feedback.Automatica, 2009, 45(7): 1715-1720.[] Wang, Y., & Boyd, S. (2009). Performance bounds for linear stochastic control. SystemsandControlLetters, 58(3),178–182.[] Magni.L.,Pala.D,Scattolini,R.Stochastic model predictive control of constrained linear systems with additive uncertainty. In European control conference (pp.2235–2240).Budapest, Hungary, 2009.[] Eugenio.E,Agarwal.M,Chatterjee,D.Lygeros,J. Convexity and convex approximations of discete-time stochastic control problems with constraints. Automatica, 47(9), 2082–2087.2011.[8] Chatterjee, D., Hokayem, P., & Lygeros, J. Stochastic receding horizon control with bounded control inputs: a vector space approach. IEEE Transactions on AutomaticControl,2009.[2] Bernardini D,Bemporad A. Scenario-based model predictive control of stochastic constrained linear systems. In IEEE conference on decision and control (pp.6333–6338).Shanghai,China.2009[4] Oldewurter,F.,Jones,C.N.,Morari,M..A tractable approximation of chance constrained stochastic MPC based on affine disturbance feedback . InProc.48th IEEE Conf. Decision Control.Cancun .2009.2010[27] Kouvaritakis B, Cannon M, RakovićS V, et al. Explicit use of probabilistic distributions in linear predictive control [J]. Automatica, 2010, 46(10): 1719-1724.[10]Wang C, Ong C J, Sim M. Model predictive control using segregated disturbance feedback. IEEE Transactions on Automatic Control, 2010, 55(4): 831--840[48] Minyong Shin.Primbs constrained stochastic MPC under multiplicative noise for financial applications[C].49th IEEE Conference on Decision and Control,Atlanta,2010,6101-6106.[] Ramponi, F., Chatterjee, D., Milias-Argeitis, A., Hokayem, P., & Lygeros, J. Attaining mean square boundedness of a marginally stable stochastic linear system with a bounded control input.IEEE Transactions on Automatic Control,55(10),2414–2418. 2010.[] Hokayem,P.,Chatterjee,D.,Ramponi,F.,Chaloulos,G.,Lygeros,J. Stable stochastic receding horizon control of linear systems with bounded control inputs. InInt. Symposium on mathematical theory of networks and systems (MTNS) (pp.31–36, Budapest).Hungary, July 2010.[] Hokayem, P., Cinquemani, E., Chatterjee. D,Lygeros, J. Stochastic MPC with imperfect state information and bounded controls .In Proceedings of the UKACC int. Conference on control. Coventry, UK, Sept.2010.[20] A. Bemporad, T. Gabbriellini, L. Puglia, and L. Bellucci, “Scenario-based stochastic model predictive control for dynamic option hedging,”in Proc. 49th IEEE Conf. Decision Control , Atlanta, GA, 2010, pp. 6089–6094[19] D. Bernardini, M. C. F. Donkers, A. Bemporad, and W. P. M. H.Heemels, “A model predictive control approach for stochastic networked control systems,”inProc. 2nd IFAC Workshop Estimation and Control Netw. Syst. , Annecy, France, 2010, pp. 7–12.[18] M. Bichi, G. Ripaccioli, S. Di Cairano, D. Bernardini, A. Bemporad, and I. Kolmanovsky, “Stochastic model predictive control with driver behavior learning for improved powertrain control,”in Proc. 49th IEEE Conf. Decision Control, Atlanta, GA, 2010, pp. 607–6082.[17] G. Ripaccioli, D. Bernardini, S. Di Cairano, A. Bemporad, and I. V. Kolmanovsky, “A stochastic model predictive control approach for series hybrid electric vehicle power management,”in Proc. Amer. Contr.Conf., Baltimore, MD, 2010, pp. 5844–5849.2011[7] Chatterjee D, Hokayem P, Lygeros J. Stochastic receding horizon control with boundedcontrol inputs –A vector space approach[J]. IEEE Transactions on Automatic Control, 2011,56(11): 2704-2710.[5]Cannon M, Kouvaritakis B, Rakovic S V, Cheng Q F. Stochastic tubes in model predictive control with probabilistic constraints. IEEE Transactions on Automatic Control,56(1),194-200, 2011[7]Wen J W, Liu F. Receding horizon control for constrained Markovian jump linear systems with bounded disturbance.ournal of Dynamic Systems, Measurement, and Control,2011[2]Su Y, Tan K K, Lee T H. Comments on \ Model predictive control for systems with stochastic multiplicative uncertainty and probabilistic constraints. Automatica, 2011[9] Eugenio.E,Agarwal.M,Chatterjee,D.Lygeros,J. Convexity and convex approximations of discete-time stochastic control problems with constraints. Automatica, 47(9), 2082–2087.2011. 2012[11] Hokayem P, Cinquemani E, Chatterjee D, Ramponi F, Lygeros J. Stochastic receding horizon control with output feedback and bounded controls. Automatica, 2012, 48(1):77--88[6] Bernardini D, Bemporad A. Stabilizing model predic tive control of stochastic constrained linear systems. IEEE Transactions on Automatic Control, 2012[50] Mark Cannon, Cheng QiFeng.Stochastic tube MPC with state estimation[J].Automatica, 2012,48 (7):536-5412013[5]Oldewurtel.F,Sturzenegger.D,Esfahani.P.M,Andersson.G,Morari.M,Lygeros.J. A daptively Constrained Stochastic Model Predictive Control for Closed-Loop Constraint Satisfaction . American Control Conference (ACC), pages 4674 - 4681,2013冯德兴.1995.凸分析基础[M].北京:科学出版社.方洋旺.2005.随机系统最优控制[M].北京:清华大学出版社.郭尚来.1999.随机控制[M].北京:清华大学出版社.钱积新,赵均,徐祖华.2007.预测控制[M].北京:化学工业出版社.王金德.1990.随机规划[M].南京:南京大学出版社.席裕庚.1993.预测控制[M].北京:国防工业出版社.。
Robust Control and Estimation

Robust Control and Estimation Robust control and estimation are crucial aspects of engineering and applied mathematics, particularly in fields like aerospace, automotive, and robotics. They deal with the design of systems that can perform reliably under various uncertainties and disturbances. The essence of robust control lies in ensuring stability and performance despite uncertainties in system parameters, external disturbances, or modeling errors. One perspective on robust control is its significance in real-world applications. In aerospace, for example, where systems operate in harsh and unpredictable environments, robust control techniques are indispensable for ensuring the safety and stability of aircraft and spacecraft. Uncertainties such as aerodynamic variations, engine faults, and atmospheric disturbances pose significant challenges that robust control methodologies address effectively. By designing controllers that can accommodate these uncertainties, engineers can enhance the reliability and performance of aerospace systems. Another perspective involves the theoretical foundations of robust control theory. It draws upon principles from control theory, optimization, and mathematical analysis to develop techniques for handling uncertainties in system dynamics. Robust control methods often rely on mathematical frameworks such as H-infinity control, mu-synthesis, and worst-case analysis to quantify and mitigate uncertainties. These methods provide a rigorous foundation for designing controllers that can guarantee stability and performance across a range of operating conditions. Moreover, robust control techniques are essential for addressing practical challenges in modern engineering systems. For instance, in autonomous vehicles, robust control plays a crucial role in ensuring safe and reliable operation in diverse driving conditions. Uncertainties such as road friction, sensor noise, and dynamic obstacles necessitate robust controlstrategies to maintain stability and avoid accidents. By incorporating robust control algorithms, autonomous vehicles can navigate complex environments with greater confidence and resilience. Additionally, the field of robust estimation complements robust control by addressing uncertainties in system state estimation. Estimation techniques such as Kalman filtering, robust filtering, and particle filtering are essential for accurately inferring the state of a dynamic systemfrom noisy sensor measurements. Robust estimation methods are particularly valuable in scenarios where sensor measurements are corrupted by outliers or biases, enabling more robust and reliable state estimation. From a practical standpoint, the implementation of robust control and estimation algorithms often involves trade-offs between performance and complexity. While robust control techniques offer enhanced stability and disturbance rejection, they may also lead to more complex controller designs that are challenging to implement and tune. Engineers must carefully balance these trade-offs to ensure that the benefits of robust control outweigh the associated costs in terms of computational resources and implementation complexity. Furthermore, the future of robust control and estimation lies in addressing emerging challenges in complex and interconnected systems. With the advent of cyber-physical systems, autonomous technologies, and intelligent infrastructure, the need for robust and adaptive control methodologies is more pronounced than ever. Robust control techniques must evolve to handle increasingly complex and uncertain environments, where traditional modeling approaches may fall short. This requires interdisciplinary research efforts that combine insights from control theory, machine learning, and computer science to develop innovative solutions for next-generation systems. In conclusion, robust control and estimation play critical roles in ensuring the reliability, safety, and performance of engineering systems in the face of uncertainties and disturbances. From aerospace to autonomous vehicles, robust control techniques provide a framework for designing controllers that can effectively handle uncertainties and variations in system dynamics. Moreover, robust estimation methods enable accurate state estimation in the presence of noise and outliers, further enhancing the robustness of control systems. Looking ahead, the continued advancement of robust control and estimation will be essential for addressing the challenges of increasingly complex and interconnected systems in the modern world.。
Estimation of Deterministic and Stochastic IMU

Inertial navigation systems need acceleration and angular rate measurements in the x, y and z- directions to calculate attitude, position and velocity. Therefore, inertial measurement units contain three accelerometer and three gyroscope. For this reason, IMU error model is determined with equation ( 1) and (2) [3,4].
Estimation of Deterministic and Stochastic IMU Error Parameters
Derya UNSAL Department of Guidance and Control Design Roketsan Missiles Industries Inc. Ankara, Turkey dunsal@.tr Abstract- Inertial Measurement Units,
Kerim DEMIRBAS Department of Electrical and Electronics Engineering Middle East Technical University Ankara, Turkey demirbas@.tr signals and this is the major drawback of GPS. However, INS uses IMU outputs to construct position velocity and attitude by processing the navigation equations. Therefore IMUs are the major part of inertial navigation systems. An IMU is a device, which is used to measure linear acceleration and angular rate. Inertial measurement units contain two types of sensor, accelerometer and gyroscope. An accelerometer measures linear acceleration about its sensitivity axis and integrated acceleration measurements are used to calculate velocity and position. Besides a gyroscope measures angular rate about its sensitivity axis and gyroscope outputs are used to maintain orientation in space. The cost of an IMU increases when the sensor performance requirements increase. The major reasons for the cost increase can be explained in two ways. The first reason is the highly skilled production line requirement and the second reason is the decrease in the percentage of utilizable sensor in the batch. Therefore, in order to improve the performance of inertial sensors, the calibration algorithms and the error compensation models were researched and developed. Thereby both low-cost and high-performance IMUs could be produced. The main objective of this article is to develop methods in order to estimate deterministic and stochastic error parameters of MEMS based inertial measurement units. Additionally, improving the performance of IMUs is aimed by using these estimated parameters. Therefore an error calibration algorithm is implemented and estimated parameters are used in this algorithm. II. I MU ERROR MODEL
文献——精选推荐
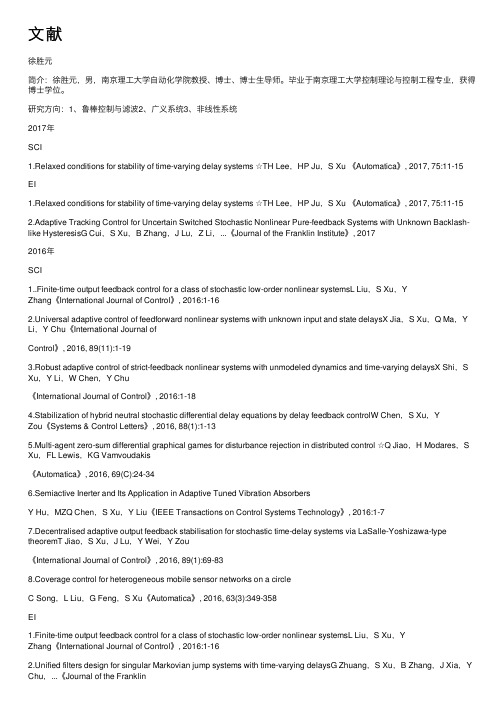
⽂献徐胜元简介:徐胜元,男,南京理⼯⼤学⾃动化学院教授、博⼠、博⼠⽣导师。
毕业于南京理⼯⼤学控制理论与控制⼯程专业,获得博⼠学位。
研究⽅向:1、鲁棒控制与滤波2、⼴义系统3、⾮线性系统2017年SCI1.Relaxed conditions for stability of time-varying delay systems ☆TH Lee,HP Ju,S Xu 《Automatica》, 2017, 75:11-15EI1.Relaxed conditions for stability of time-varying delay systems ☆TH Lee,HP Ju,S Xu 《Automatica》, 2017, 75:11-152.Adaptive Tracking Control for Uncertain Switched Stochastic Nonlinear Pure-feedback Systems with Unknown Backlash-like HysteresisG Cui,S Xu,B Zhang,J Lu,Z Li,...《Journal of the Franklin Institute》, 20172016年SCI1..Finite-time output feedback control for a class of stochastic low-order nonlinear systemsL Liu,S Xu,YZhang《International Journal of Control》, 2016:1-162.Universal adaptive control of feedforward nonlinear systems with unknown input and state delaysX Jia,S Xu,Q Ma,Y Li,Y Chu《International Journal ofControl》, 2016, 89(11):1-193.Robust adaptive control of strict-feedback nonlinear systems with unmodeled dynamics and time-varying delaysX Shi,S Xu,Y Li,W Chen,Y Chu《International Journal of Control》, 2016:1-184.Stabilization of hybrid neutral stochastic differential delay equations by delay feedback controlW Chen,S Xu,YZou《Systems & Control Letters》, 2016, 88(1):1-135.Multi-agent zero-sum differential graphical games for disturbance rejection in distributed control ☆Q Jiao,H Modares,S Xu,FL Lewis,KG Vamvoudakis《Automatica》, 2016, 69(C):24-346.Semiactive Inerter and Its Application in Adaptive Tuned Vibration AbsorbersY Hu,MZQ Chen,S Xu,Y Liu《IEEE Transactions on Control Systems Technology》, 2016:1-77.Decentralised adaptive output feedback stabilisation for stochastic time-delay systems via LaSalle-Yoshizawa-type theoremT Jiao,S Xu,J Lu,Y Wei,Y Zou《International Journal of Control》, 2016, 89(1):69-838.Coverage control for heterogeneous mobile sensor networks on a circleC Song,L Liu,G Feng,S Xu《Automatica》, 2016, 63(3):349-358EI1.Finite-time output feedback control for a class of stochastic low-order nonlinear systemsL Liu,S Xu,YZhang《International Journal of Control》, 2016:1-162.Unified filters design for singular Markovian jump systems with time-varying delaysG Zhuang,S Xu,B Zhang,J Xia,Y Chu,...《Journal of the FranklinInstitute》, 2016, 353(15):3739-37683.Improvement on stability conditions for continuous-time T–S fuzzy systemsJ Chen,S Xu,Y Li,Z Qi,Y Chu《Journal of the Franklin Institute》, 2016, 353(10):2218-22364.Universal adaptive control of feedforward nonlinear systems with unknown input and state delaysX Jia,S Xu,Q Ma,Y Li,Y Chu《International Journal ofControl》, 2016, 89(11):1-195.H∞ Control with Transients for Singular Systems Z Feng,J Lam,S Xu,S Zhou 《Asian Journal of Control》, 2016,18(3):817-8272015年SCI1.Pinning control for cluster synchronisation of complex dynamical networks withsemi-Markovian jump topologyTH Lee,Q Ma,S Xu,HP Ju《International Journal of Control》, 2015, 88(6):1223-12352..Anti-disturbance control for nonlinear systems subject to input saturation via disturbance observer ☆Y Wei,WX Zheng,S Xu《Systems & ControlLetters》, 2015, 85:61-693.Exact tracking control of nonlinear systems with time delays and dead-zone inputZ Zhang,S Xu,B Zhang《Automatica》, 2015, 52(52):272-276EI1.Further studies on stability and stabilization conditions for discrete-time T–S systems with the order relation information of membership functionsJ Chen,S Xu,Y Li,Y Chu,Y Zou《Journal of the Franklin Institute》, 2015, 352(12):5796-5809 .2 .Stability analysis of random systems with Markovian switching and its application T Jiao,J Lu,Y Li,Y Chu,SXu《Journal of the Franklin Institute》, 2015, 353(1):200-220 3.Exact tracking control of nonlinear systems with time delays and dead-zone inputZ Zhang,S Xu,B Zhang《Automatica》, 2015, 52(52):272-2764.Event-triggered average consensus for multi-agent systems with nonlinear dynamics and switching topologyD Xie,S Xu,Y Chu,Y Zou《Journal of the Franklin Institute》, 2015, 352(3):1080-1098葛树志简介:葛树志,男,汉族,1963年9⽉20⽇⽣于⼭东省安丘县景芝的葛家彭旺村。
国际商务谈判(英文)教案讲义Chapter8LawofTrust信任法则
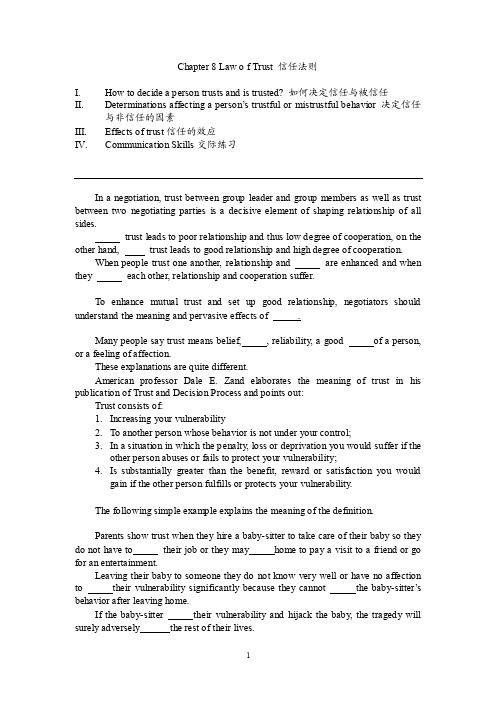
Chapter 8 Law o f Trust 信任法则I.How to decide a person trusts and is trusted? 如何决定信任与被信任II.Determinations affecting a person’s trustful or mistrustful behavior 决定信任与非信任的因素III.Effects of trust信任的效应munication Skills交际练习In a negotiation, trust between group leader and group members as well as trust between two negotiating parties is a decisive element of shaping relationship of all sides.trust leads to poor relationship and thus low degree of cooperation, on the other hand, trust leads to good relationship and high degree of cooperation.When people trust one another, relationship and are enhanced and when they each other, relationship and cooperation suffer.To enhance mutual trust and set up good relationship, negotiators should understand the meaning and pervasive effects of .Many people say trust means belief, , reliability, a good of a person, or a feeling of affection.These explanations are quite different.American professor Dale E. Zand elaborates the meaning of trust in his publication of Trust and Decision Process and points out:Trust consists of:1.Increasing your vulnerability2.To another person whose behavior is not under your control;3.In a situation in which the penalty, loss or deprivation you would suffer if theother person abuses or fails to protect your vulnerability;4.Is substantially greater than the benefit, reward or satisfaction you wouldgain if the other person fulfills or protects your vulnerability.The following simple example explains the meaning of the definition.Parents show trust when they hire a baby-sitter to take care of their baby so they do not have to their job or they may home to pay a visit to a friend or go for an entertainment.Leaving their baby to someone they do not know very well or have no affection to their vulnerability significantly because they cannot the baby-sitter’s behavior after leaving home.If the baby-sitter their vulnerability and hijack the baby, the tragedy will surely adversely the rest of their lives.But if the baby-sitter their vulnerability and take good care of the baby, then the parents can keep their mind on their work or enjoy their meeting with their friend or a party.There are three fundamental elements: information, influence and control.A person shows trust when he reveals he need not disclose.He increases his by telling others his goals, purpose, plans, alternatives or his problems.Others may make use of the information to impede or undermine his efforts.For example,A designer struck on a brilliant idea which he told a colleague working in the same office. The colleague used the idea to advance his own interests and was promoted soon.So a person who does not others will conceal or distort relevant information.He will facts, his purpose and his feelings.A person show trust when he shows others to his decisions since he increases his by asking for others’ advice which may deliberately him.For example,As a China’s famous story of Fighting in Chibi in Tale of Three States depicts Caocao (premier of East Han Dynasty) asked for Pangtong’s (a counselor secretly working for Dongwu, Caocao’s enemy) advice for defeating Dongwu. Pangtong offered him a seemingly clever advice, which turned out to be a part of fatal plot inducing him and his troops into a trap.Therefore, a person who shows will resist others’influence, deny and reject their suggestions and advice.A person shows when he delegates and permits others to act on their own on his behalf.By this way he increases his because he has to rely on others to make a judgment and to implement his plan and others may serious errors, ____ implementation and his plan.So if a person does not trust, he will try to impose over others and ____ his dependence on others.For example,The principal of a middle school had peep holes installed in all the doors of classrooms in order for the administrative to have a tighter over students.However the device produced skeptical atmosphere among staff members as wellbecause anyone teaching or staying in the classroom feels he is being watched over and he is not .In negotiation or in people’s daily life, elements affecting a person’s trustful or mistrustful behavior come form main sources: which is inalterable and grown-up experiences, which are changeable.Childhood educationStudies on childhood education on trust began in the 1950s, with the publication of Erik Erikon’s Childhood and Society. Since then many developmental psychologists have viewed trust and; mistrust as the cornerstones of human development.A child’s understanding of trust is from his own and the environment he is up.When a child’s desires and reliability are fulfilled in most cases, he tends to conclude that people are , otherwise, he may draw the from his unfulfillment that people are trustful.A child will tend to trust others if he is brought up in a simple, warm and _____ environment, and he is told the stories of help and trust.However in other context, a child’s parents and other people intentionally inform him of examples of mistrust to drive the that “Y ou can’t trust people”.Understandably, such child inclines not to others when he grows up.Professional or special trainingA person’s professional or special training can incline him a different orientation to .For instance, accountants and financial workers are oriented to be of financial statements full of identical numbers and how much they may differ from the real data.People working in human resources department appear to more the accuracy of a person’s description of his educational and working background, and it is an important reason that the application documents should be, at the request, _____by official certificates.Past credit recordA person’s willingness to trust another person on his knowledge of the other’s past record.We will not rust someone who to perform his duty or is to complete a task or fails to keep his .The reason for the failure may differ from time to time, however, if there isrepeated record of the person’s failure, is the natural results.Surely, a person can do better to improve his record and thus win others’trust.Competence of others to perform a taskA person’s willingness to trust another person depends on his estimation of the other’s ability to complete a task .At this point, there should be a distinction between capability and affection.For instance, you will not trust a teenager to send a large sum of money to a far away place although you him very much.When is confused with capability in one’s trust decision, more often than ____his plan will be undermined.Intentions of othersOur willingness to trust another person is determined by our interpretation of the other’s intentions. i.e. We will not trust those we believe who have intention, and who will exploit our resources and take of our trust to advance his own interests.We will not trust such kind of person even though he is and he has a good _____record.People’s interpretation of others’intentions and motives can be different from time to time depending on their understanding of others.Reward systemIn win-lose reward system, when competition is rewarded, i.e., our gain is the other’s loss and our loss is the other’s gain, trust the other is clearly not in our self-interest.Companies selling the same products are rivals in business and understandably they will conceal or information, withhold facts and their ideas.Things will be different in joint reward system in which is encouraged because in such reward system things won’t be done without joint efforts, so it is all natural that the two sides will each other, information, each other’s advice and reach common conclusion.Of course, in today’s world there are neither competitors nor cooperators. Where will things be directed depends on our efforts. It is hoped that trustful relationship among people should be on account of positive effects of trust.Trust is a decisive element in people’s relationship. We need trust between peers, superiors and , between producers and , teachers and .Studies show that trust intellectual development and originality, and leadsto emotional stability and self-control.Trust acceptance and openness of expression.Trust cooperation and mutual understanding, and it is fundamental for establishing sound relationship among negotiating team as well as between negotiating .People working in a team high in trust signal of trust to each other and _____trust form each other, which will increase level of trust among the members. Negotiations based on high level of trust can increase of double win results.Conversely, mistrust rejection and defensiveness, collaboration and relationship of team members and negotiating parties.The striking contrast of trust and mistrust between negotiating parties tells us that it is worthwhile we take great pains to find out ways to mutual trust.Some tentative suggestions are made here for consideration:1.Encourage mutual trust by establishing trust-rewarded system in_____education, in training and in .2.Buildup people’s confidence in trust bit by bit through giving ,influence, self-control and concessions, and seek reciprocation from theother.3.Discuss frankly with the other party what is generating innegotiation.4.Be sincere and honest to your negotiating team members andyour .1. What comment would you make in the following situations?1)Y ou’ve just heard news of a train crash.2)One of your colleagues is always chatting to everybody.3)One of your colleagues regularly works a twelve-hour day.4)Y ou’ve just had a very good meal.5)One of your colleagues keeps himself to himself.6)Y ou’ve just seen an exhibition you expected to be good; in fact it was not.7) A person you’ve just met says he is a film director.8)One of your colleagues looks very smart today.2. Answer the following questions1)How many means are needed for the sellers to inform the buyers of the quality? What are they?2)When selling some mechanical and electrical products, what means are generally used to express their quality?3)When negotiating on the packing, what aspect should the buyers pay attention to besides the right type of packing?4)When goods are sold on a CIF basis, who is under obligation to present a marine insurance policy or an insurance certificate at the time of negotiation, the seller or the buyer?5)Which method is safer and better for the seller, D/P or D/A?6)Why is commodity inspection indispensable in international trade?7)What’s the first step in a successful sales negotiation?8)Is it necessary for the seller to make an equal concession when the buyer grants him or her a concession?9)At the beginning of the negotiation, should the sellers open high or modest?10)When you meet with negotiators who harshly bargain with you, will you cancel the negotiation or continue?11) If a negotiator s ays: “I don’t have the authority to grant you that concession”, does it really mean that he or she hasn’t such authority or is it only negotiating tactics?5. Translate the following situational dialogue into English(交货Delivery)贺先生,我很高兴我们就价格和付款方式问题达成了协议。
- 1、下载文档前请自行甄别文档内容的完整性,平台不提供额外的编辑、内容补充、找答案等附加服务。
- 2、"仅部分预览"的文档,不可在线预览部分如存在完整性等问题,可反馈申请退款(可完整预览的文档不适用该条件!)。
- 3、如文档侵犯您的权益,请联系客服反馈,我们会尽快为您处理(人工客服工作时间:9:00-18:30)。
Proceedings of the 2007 American Control ConferenceMarriott Marquis Hotel at Times SquareThC01.2 New York City, USA, July 11-13, 2007Estimation and Rejection of Unknown Sinusoidal Disturbances Using aGeneralized Adaptive Forced Balancing MethodElnaz Vahedforough, Bahram Shafai, and Stuart BealeAbstract— This paper presents a method for estimation and rejection of sinusoidal disturbances with unknown amplitudes and frequencies arising in feedback control systems. A method known as adaptive forced balancing has been used successfully to reject sinusoidal disturbances with known frequency and unknown amplitude in magnetic bearing control systems. The occurrence of such a disturbance is a result of a common prob- lem to all mechanical systems with rotating shaft known as mass unbalance. Because mass unbalance consists of a sinusoidal sensor disturbance within the bandwidth, conventional methods suffer from instability or fail to preserve desired bandwidth. AFB overcomes these problems and offers unique features which preserve stability and performance robustness. In this paper, we extend AFB with a frequency tracking capability. This is achieved by using two different adaptive observer structures. We show that the modified version of AFB is capab le of estimating both amplitude and frequency of the disturbance signal and demonstrate its rejection in the feedback loop.I. INTRODUCTIONThe problem of disturbance rejection has always been a critical issue in control systems. Among various forms of disturbances, sinusoidal signals occur frequently in rotational machinery. In such applications it is desirable to estimate unknown amplitude, frequency and phase of disturbance signals. The literature reports a vast variety of methods for this purpose. Hsu and Regalia improved the performanceof notch filters and introduced an adaptive IIR version ofit in [1], [2]. Later on Marino and Tomei introduced the adaptive observers to estimate the frequency of sinusoidal disturbances in [3]. Guo and Bodson considered frequency estimation and tracking of multiple sinusoidal components using magnitude/phase locked loop in [4]. Among other solutions, Kalman Filtering [5], power spectrum method and harmonic decomposition methods, were also reported by researchers in [6], [7], and [8]. In spite of the fact thatmany researchers partially solved the problem, combined estimation of unknown frequency and amplitude of sinu- soidal disturbance signal in different scenarios; particularly in feedback control systems, still remains a challenging task.A particularly important application that requires estimation and compensation of disturbances is in active magnetic bearing control systems. Analysis and design of such systems have been studied by many researchers in the past. For an overview of results in this direction, refer to [9]-[14] and the references therein.In this paper we consider the problem of rejecting sinu- soidal disturbance signal in active magnetic bearing control systems. Such a signal occurs as a result of vibration caused by mass unbalance: the condition of a rotor in which its principle axis of inertia is not coincident with its axis of geometry. In [15], this disturbance is modeled as a sinusoidal signal with known rotational frequency and unknown ampli- tude. A novel approach called Adaptive Forced Balancing (AFB) was used to estimate the amplitude of the disturbance signal and made its rejection possible. However, a more de- sirable solution for the disturbance accommodation problem is when both amplitude and frequency are not known. In most applications including magnetic bearing control systems, it is essential to estimate the frequency in addition to amplitude. This is due to the fact that, if rotational sensors are not available, it is required to estimate the frequency as well. The modified AFB proposed in this paper incorporates a frequency tracking algorithm, which enables to estimate both amplitude and frequency of the disturbance signal. AFB offers additional advantages, which makes it worthwhile to use in the control loops. One of the main characteristics of this method as compared to the previous filter based methods is that it operates external to the basic feedback loop. The proposed structure of AFB has negligible effect on frequency response of the designed closed loop system performance.In contrast with model based methods, it doesn’t needthe knowledge of plant dynamics and therefore is robustto modeling errors and plant parameter variations. In this paper we discuss the Adaptive Forced Balancing structurein connection to magnetic bearing control systems. Then,we generalize the method by including a frequency tracker, which consists of a Proportional Integral Adaptive Observer, to the basic AFB structure. We demonstrate that estimation and rejection of unknown sinusoidal disturbances is possible by using the generalized AFB method. The simulation results show the fast convergence rate of frequency and amplitude estimations as well as disturbance cancelation at the output.II. ADAPTIVE FORCED BALANCING INCONTROL LOOPAdaptive Forced Balancing is designed to estimate the amplitude and possibly the frequency of the disturbance signal in order to be able to reject the effect of the disturbance in feedback control loop. This method is neither a model based nor resides in the inner control loop. It is an outer control loop which provides a synchronous, rotor position reference signal for the inner control loop. The design of AFB with the proposed set up does not degrade the stability margin of the closed loop control system. It also offers additional robustness in comparison to previous methods. Fig. 1 demonstrates the block diagram of the Adaptive Forced Balancing system in a control loop. The input ofthe AFB system is the control signal represented by u(t),1-4244-0989-6/07/$25.00 ©2007 IEEE. 3529ThC01.2and its output is r Ω(t) which represents the synchronous reference signal. We assume that the disturbance d Ω(t) has the following time domain representation:d Ω(t) = αd sin(ωx t) + βd cos(ωx t),(1)where α(t), β(t) are the Fourier Coefficients of the sinu - soidal disturbance. It is required to cancel out the effect of d Ω(t) by designing AFB system. To accomplish this taskFig. 2.The structure of Adaptive Forced Balancing systemwe need to provide a synchronous signal with the following structure:Butterworth and Chebychev filters. In fact the filter is capable of passing the DC components of demodulated signal. The r Ω(t) = αd (t) sin(ωx t) + βd (t) cos(ωx t).(2) cut off frequency is less than or equal to ωx /3 , while the If we represent the sensitivity function of the closed loop system as:P (s)C(s)T (s) = , (3)1 + P (s)C(s) then the system output can be written as:sampling frequency of filter should be greater than or equal to 100ωx .B. Fourier Co efficient ComputerThe Fourier Coefficient Calculator is the main part of our adaptive forced balancing system. The coefficients of sine Y (s) = T (s)r(s) + T (s) (r Ω(s) − d Ω(s)) ,(4) and cosine are calculated on line such that it cancels out the effect of disturbance. The adaptive laws which govern α(k), and the effect of the disturbance at the output is eliminatedβ(k) are generated by:as r Ω(t) → d Ω(t). This can only be realized if:α(k + 1) = α(k) + (1 + q(k)) p +1(k)n(k),(6) lim r Ω(t) → d Ω(t).t→∞(5) β(k + 1) = β(k) + (1 − q(k)) p −1(k)n(k),(7)III. THE STRUCTURE OF AFBAs it is shown in Fig. 2, AFB system is composed of three fundamental parts:1) Synchronous RMS Calculator 2) Fourier Coefficient Computer 3) Modulator(Signal Generator)A. Synchronous RMS CalculatorIn this part we calculate the ”energy” of the synchronous component of control signal. This energy serves as an error input to an algorithm which calculates the amplitude and phase of a synchronous correction signal. To obtain the norm signal of n u (t), the control signal u(t) is demodulated and Where α(k), β(k) are the discrete forms of the corre-sponding time varying Fourier Coefficients and are calculated in the sampling period of T s . The input to the system is represented as n(k) which is updated every T s seconds. The variables p(k) and q(k) get values equal to {+1, −1} according to the algorithm, shown in Fig. 4.In this process an ”enable” variable q(k) ∈ {−1, +1} holds one Fourier Coefficient constant while the other is getting adapted, and ”polarity” variables p +1(k), p −1(k) ∈ {−1, +1} insert the corresponding signs in (6), (7), respec- tively, so that the norm of error ǫ(k) decreases with time. The error ǫ(k) represents the amount of aviation from desired values of αd , βd , which is shown by:filtered with low pass Bessel filter. Then a complex mag ni- ǫ(k) = (α(k) − αd ) + j(β(k) − βd ).(8)tude calculation is performed as illustrated in Fig. 3. Here we used Bessel filter to overcome the overshoot problem ofIf the sampling frequency ωs is chosen to be small enough such that ωs ≪ ωx ; then [α(k) − α(k − 1)]/T s , and [β(k) − β(k −1)]/T s become sufficiently small. Hence we can make the following assumption:u(t) ≈ µ (ωx ) [(αr (t) − αd ) sin(ωx t + Θωx )+(9)Fig. 1.Adaptive Forced Balancing system in a control loopFig. 3.The RMS Calculator structure3530ωx 2 ωx 2 1/2ThC01.2IV. THE GENERALIZED STRUCTURE OF AFBWITH FREQUENCY TRACKER In previous section AFB is used to compensate the sinu- soidal disturbances while the fundamental frequency ωx was known. However if hardware (e.g., a tachometer, resolver, or another type of rotational sensor) is not available to generate these waveforms, a frequency tracking algorithm, operating in synergy with AFB, must be included to provide estimates of ωx . Therefore a Frequency Tracker system is needed to estimate the frequency while the amplitude is estimated by AFB system. Fig. 5 demonstrates the generalized structure of AFB which consists of AFB system along with frequency tracker. The estimated frequency signal enters the sine and cosine generators and then is injected to AFB to estimate the amplitude enabling the disturbance rejection in the next stage.A. Frequency TrackerConsidering the disturbance signal y = A sin(ωt + φ), with its state space representation model as:Fig. 4.The Fourier Coef ficient Calculator algorithmx ˙ 1 x ˙ 2=0 1 −α 0 x 1 x 2(14)d(t) = y(t) = H T x = x 1(βr (t) − βd ) cos(ωx t + Θωx )],where y(t) is the disturbance signal entering the system, α = ω2, with initial conditions x 1(0) = A sin(φ), and where µ(ω) = |(S(j ω)C(j ω)|, Θ(ω) =x 2(0) = A ω cos(φ). since the system described by (14)arg(S(j ω)C(j ω)) and S(j ω) is the sensitivity function given by S(j ω) = 1/1 + P (s)C(s). Therefore n(k) can be expressed as:has the observable canonical form, we can use the adaptive observer method to estimate the frequency of the signal. In the following sections we will first discuss the adaptive n(k) = n u (kT s ),= v{[h(kT s ) ∗ (u(kT s ) sin(2πkω)] + (10)observer procedure and then extend it to Proportional Integral Adaptive Observer.1) adaptive observer method: Adaptive Observer method first was introduced by Robert Carroll and then was mod-n(k) =v2[h(kT s ) ∗ (u(kT s ) cos(2πk )] } , ωsµ(ωx ) (α(k) − αd )2 + (β(k) − βd )2,(11)i fied by Narndra and Kudva for parameter estimation [16], [17]. Such an adaptive observer has been applied in many scenarios. In this paper we use it for frequency estimation in active magnetic bearing control system. Considering theand it can be simpli fied as:n(k) =1 2µ(ωx )|ǫ(k)|.(12)Therefore the adaptation of p(k) and q(k) enables us to control the coef ficients error such that:|ǫ(k + 1)| < |ǫ(k)|.C. ModulatorAs it is shown in Fig. 2, Modulator receives the output signal from FCC and modulates it to the following form:r Ω(t) = αr (t) sin(ωx t) + βr (t) cos(ωx t),(13)This provides the generation of the synchronous signal needed for disturbance cancelation.3531Fig. 5.The AFB Compensator with Frequency Trackerρ(s) = 2ψ(s) = 2 yˆ(t) = h z ThC01.2z˙1 = k1(y − yˆ) + z2 + n1 (y − yˆ) (20)z˙2 = k2(y − yˆ) + n2 (y − yˆ) −αˆyyˆ(t) = h T zwhere n1 and n2 are positive scalars. Using the secondorder filters:Fig. 6. The Frequency Tracker−ss + d1s + d2y(s), (21)system described by (14), the conventional adaptive observer−c1s − c2s + d1s + d2y(s), (22)structure assumes the following form: the above state space form can be summarized in followingz˙ = Kz + [k − aˆ(t)]x1 + v + wT where K is a stable matrix described by: (15)form:yˆ˙ (t) = k c y + k p(y − yˆ) + αˆ(t)ρ(t) + ψ(t)αˆ˙ = −Γρ(y − yˆ)(23)K = −k1−k21,where c1, c2, d1, d2 are positive scalars. The PI AdaptiveObserver offers a fast the convergence rate in comparisonto adaptive observers. The simulations in the next sectionsand h T = 1 0 and v, w are the auxiliary signals. The demonstrate its performance in frequency estimation.objective is to :lim aˆ(t) → a,t→∞B. Amplitude TrackerAs it was described before,α(t), β(t) are the Fourierwhere a represents 0 −αT . Using auxiliary filters: Coefficients which provide the amplitude for sine and cosinerespectively. Therefore the magnitude of the amplitude isv˙ = −λv − y, (16) calculated from (24) as it is shown in (25).w˙ = −λw −λ2y, (17) Aˆ(t) = α(t) sin(ωx t) + β(t) cos(ωx t) (24) the state space form of (15) can be transformed to the follow- |Aˆ(t)| = α(t)2 + β(t)2 (25)ing single input single output form, which was formalized in [3]. V. SIMULATION RESULTS FOR AFB WITH UNKNOWN FREQUENCYyˆ˙ = λ(y − yˆ) + λy + vαˆ + w (18) We simulated the control loop for the case when all pa-rameters of sinusoidal disturbance are unknown. ConsideringThe overall schematic diagram of frequency tracker system is shown in Fig. 6. In this system the disturbance signal passes through the low pass filters described by (16), (17). The generated auxiliary signal is used in identification block to estimate the frequency at the next level which is given by: the disturbance asd(t) = α sin(ωx t + φ) + β cos(ωx t + φ),where,α = 20, β = 10, ω = 25, andφ represents an arbitrary phase shift. As shown in Fig. 5 the disturbanceαˆ˙ = −Γv(y − yˆ) (19) is acting on frequency tracker and AFB system. Frequency estimation simulations are carried out using the adaptive observer method and modified PI Adaptive Observer which2) PI adaptive observer method: Using the concept of adaptive observers discussed above we generalized the es- timation procedure by using Proportional Integral Adaptive Observer. The PI Adaptive Observer consists of the regular proportional term in addition with an Integral term which improves the convergence of estimated signal in both single and multi parameter cases. The following state space form represents the PI formulation for the purpose of frequency estimation.are illustrated in Fig. 7 and Fig. 8, respectively. As figuresdemonstrate, the PI adaptive observer improves the fast con- vergence of the signal. The parameters used in simulationsfor PI adaptive observer are k p = 5, k c = 10,Γ = 500,where the poles of the auxiliary filters are adjusted to 2.The estimated frequency is applied to AFB system where the amplitudesα, β are estimated and the resulting signalis used as reference signal to reject the disturbance at theoutput. Fig. 9 illustrates that the fourier coefficientsα(t), 3532ThC01.235The Estimated Frequency Using Adaptive Observer302520151050 051015202530Time(second)Fig. 7.3025The estimated frequency using Adaptive ObserverThe Estimated Frequency Using PI Adaptive Observer2520 Fig. 9. Estimated Fourier Coef ficientsThe Estimated Amplitude20 15151010550 051015Time(second)2025300 0510 15 Time(second)2025Fig. 8.The estimated frequency using PI Adaptive ObserverFig. 10.Estimated Amplitudeβ(t) converge to the amplitudes of each sinusoidal term. The effective amplitude is then calculated as shown in Fig. 10. The results of estimated reference signal and its rejectedd Ω(t) =nαd(2i+1) sin(ωx(2i+1))+βd(2i+1) cos(ωx(2i+1)),effect at the output are demonstrated in Fig. 11 and Fig. 12, respectively.VI. CONCLUSIONSIn this paper we designed an Adaptive Forced Balancing (AFB) system to estimate the amplitude and frequency of sinusoidal disturbances. We considered the application scenario of magnetic bearing control systems whereby a si- nusoidal signal characterizes the disturbance caused by mass unbalance. First we designed the AFB under the assumption of known rotational frequency and unknown amplitude. A frequency tracker system is introduced with the original AFB in a generalized structure to estimate both the amplitude and frequency of the sinusoidal disturbance signal. Simulation results show that the generalized AFB method proposed in this paper makes it possible to completely reject unknown sinusoidal disturbance in a closed loop control system with negligible effect on the stability and performance robustness. This system is designed to estimate the fundamental fre- quency. However in some applications when the fundamental frequency is considerably small, it is needed to extend it to higher harmonics to achieve better estimation results. In this case, we de fine:i=1which demonstrates the disturbance signal composed of (2n + 1) harmonics, where α, β are the estimated am- plitude set, obtained by Fourier Coef ficient Calculators of AFB. Therefore, the estimated values for higher harmonic frequencies can be constructed by multiple modules of the frequency tracker con figuration shown in Fig. 5. It should be pointed out that the above analysis also enables us to reject disturbances of pulse shape through Fourier series expansion. This will be elaborated in the future publication.R EFERENCES[1] L. Hsu, R. Omega, and G. Damm, ”A globally convergent frequencyestimator ”, IEEE Trans. Automat. Contr., vol. 44, pp. 698-713, 1999. [2] P. A. Regalia, ”An improved Lattice-based IIR notch filter ”, IEEETrans. Signal Processing , vol. 39, pp. 2124-2128, 1991.[3] R. Marino and P. Tomei, ”Global adaptive compensation of noiseswith unknown frequency ”, IEEE Trans. Decision and Control , vol. 5, pp. 4926-4927, 2000.[4] X. Guo and M. Bodson, ”Frequency estimation and tracking ofmultiple sinusoidal components ”, IEEE Conf. Decision and Control , vol. 5, pp. 5360-5365, 2003.[5] S. Bittanti and S. M. Savaresi, ”Frequency tracking via extendedKalman filter: parameter design ”, IEEE Trans. American Control Conference , vol. 4, pp. 2225-2229, 2002.[6] T. Lobos, T. Kozina, and H. J. Koglin, ”Power system harmonics esti-mation using linear least squares method and SVD ”, IEE Proceedings in Generation Tarnsmission Distributon , vol. 148, pp. 567-572, 2001.3533感谢您试用AnyBizSoft PDF to Word。