Fingerprint matching using ridges
Lecture 8 - Fingerprint Analysis

Hasan Fleyeh
4
Level 1 (The Global Level)
Ridges often run smoothly in parallel but exhibit one or more regions where they assume distinctive shapes characterized by
θ yo xo
yo
xo
30/11/2010 Hasan Fleyeh 12
Ambiguity with Minutiae
In practice, an ambiguity exists between termination and bifurcation minutiae. Depending D di on the th finger fi pressure against the surface where h the h fingerprint fi i is i left, l f terminations may appear as bifurcation and vice versa.
Loop (shape like ∩ ) Delta (shape like Δ) Whorl (shape like O)
30/11/2010
Hasan Fleyeh
5
Level 1
Singular regions (white boxes) and core points (small circles) in fingerprint images
High curvature, Frequent ridge Terminations T i ti
These shapes are called as singularities or singular regions. They Th are classified l ifi d into i t three th types t
optimal fingerprint method

optimal fingerprint methodThe Optimal Fingerprint Method (OFM) is a technique used in the field of image processing and computer vision for fingerprint recognition. The goal of OFM is to extract and represent fingerprint images in a way that allows for efficient and accurate recognition and matching.The basic idea behind OFM is to divide the fingerprint image into small regions or blocks, and then提取每个区域的特征。
These features can include things like the orientation and frequency of the指纹纹路, as well as the presence or absence of certain features such as ridges and valleys.Once the features have been extracted from each region, they are combined into a vector or matrix that represents the entire fingerprint image. This vector or matrix is then used as input to a recognition algorithm, which compares it to other fingerprints in a database to determine whether there is a match.One of the advantages of OFM is that it is relatively simple and efficient, and can be implemented using standard image processing techniques. Additionally, it is able to handle a wide variety of fingerprint images, including those that are distorted or have noise.However, OFM also has some limitations. For example, it may not be able to accurately represent fingerprints that have very complex or irregular patterns. Additionally, it may be vulnerable to attacks such as spoofing or presentation attacks.Overall, the Optimal Fingerprint Method is a useful technique for fingerprint recognition, but it is important to consider its limitations and potential vulnerabilities when using it in practical applications.。
尸体与活体指纹微观细节特征分析的科学基础
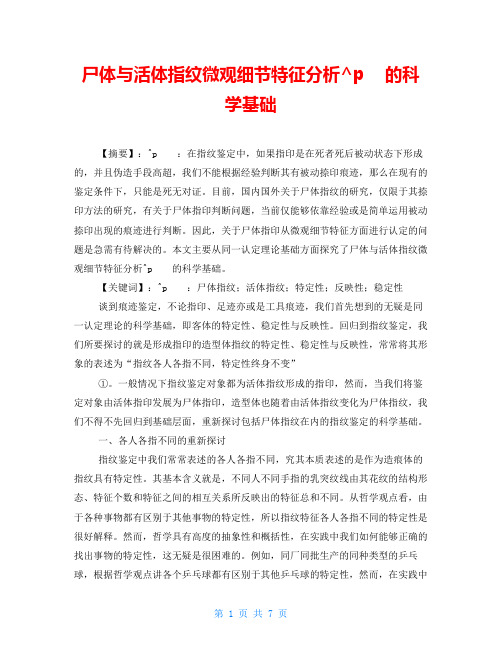
尸体与活体指纹微观细节特征分析^p 的科学基础【摘要】:^p :在指纹鉴定中,如果指印是在死者死后被动状态下形成的,并且伪造手段高超,我们不能根据经验判断其有被动捺印痕迹,那么在现有的鉴定条件下,只能是死无对证。
目前,国内国外关于尸体指纹的研究,仅限于其捺印方法的研究,有关于尸体指印判断问题,当前仅能够依靠经验或是简单运用被动捺印出现的痕迹进行判断。
因此,关于尸体指印从微观细节特征方面进行认定的问题是急需有待解决的。
本文主要从同一认定理论基础方面探究了尸体与活体指纹微观细节特征分析^p 的科学基础。
【关键词】:^p :尸体指纹;活体指纹;特定性;反映性;稳定性谈到痕迹鉴定,不论指印、足迹亦或是工具痕迹,我们首先想到的无疑是同一认定理论的科学基础,即客体的特定性、稳定性与反映性。
回归到指纹鉴定,我们所要探讨的就是形成指印的造型体指纹的特定性、稳定性与反映性,常常将其形象的表述为“指纹各人各指不同,特定性终身不变”①。
一般情况下指纹鉴定对象都为活体指纹形成的指印,然而,当我们将鉴定对象由活体指印发展为尸体指印,造型体也随着由活体指纹变化为尸体指纹,我们不得不先回归到基础层面,重新探讨包括尸体指纹在内的指纹鉴定的科学基础。
一、各人各指不同的重新探讨指纹鉴定中我们常常表述的各人各指不同,究其本质表述的是作为造痕体的指纹具有特定性。
其基本含义就是,不同人不同手指的乳突纹线由其花纹的结构形态、特征个数和特征之间的相互关系所反映出的特征总和不同。
从哲学观点看,由于各种事物都有区别于其他事物的特定性,所以指纹特征各人各指不同的特定性是很好解释。
然而,哲学具有高度的抽象性和概括性,在实践中我们如何能够正确的找出事物的特定性,这无疑是很困难的。
例如,同厂同批生产的同种类型的乒乓球,根据哲学观点讲各个乒乓球都有区别于其他乒乓球的特定性,然而,在实践中我们如何找出各个乒乓球的特定性这是困难所在。
二十世纪初,学者们虽然已经从统计学、遗传生物学等诸多方面,论证了从形态方面指纹的确有各人各指不同的特定性,但实践中,我将如何保证正确认识指纹的特定性的问题仍需不断探索。
《有趣的指纹》英语作文

《有趣的指纹》英语作文英文回答:Fingerprints are intricate patterns that adorn the fingertips of every human being. These unique impressions serve as an intrinsic identification tool, providing a reliable means of distinguishing individuals from one another. The formation of fingerprints occurs during the early stages of fetal development, influenced by genetic factors and the interaction of the fetus with the amniotic fluid.The development of fingerprint patterns is a complex process that involves the formation of ridges and valleys on the fingertips. These ridges, also known as friction ridges, serve a functional purpose by enhancing grip and providing tactile sensitivity. The arrangement of these ridges, along with the patterns formed by the intervening valleys, creates the unique fingerprint patterns that are characteristic of each person.The study of fingerprints, known as dactylography, has a long history, dating back to the late 19th century. The first systematic classification of fingerprints was developed by Sir Francis Galton, an English scientist, in the 1880s. Galton's work laid the foundation for the use of fingerprints in criminal investigations and personal identification.Over the years, the science of fingerprint analysis has advanced significantly. Today, automated fingerprint identification systems (AFIS) are widely used by law enforcement agencies to rapidly search and match fingerprints against large databases. AFIS systems rely on sophisticated algorithms to extract and compare characteristic features from fingerprints, enablingefficient and accurate identification of individuals.Fingerprints have also proven to be valuable in a variety of other applications. In the medical field, fingerprint patterns can be used to identify genetic disorders and certain medical conditions. In forensicscience, fingerprints serve as crucial evidence in criminal investigations, linking individuals to crime scenes. Additionally, fingerprints are utilized in biometric security systems for access control and personal authentication.中文回答:指纹是装饰在每个人指尖上的复杂图案。
FingerPrint
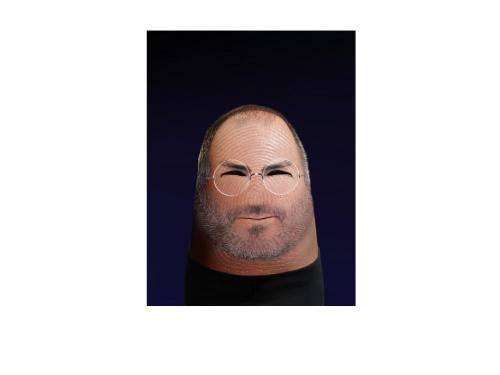
Type of fingerprint
Fingerprint Feature Extraction and Numeric Meta-base Creation
1. Fingerprint Image Pre-processing The main steps involved in the pre-processing include: (a) enhancement (b) binarization (c) segmentation, (d) thinning. 2. Fingerprint Feature Extraction and Numeric Meta-base Creation
FingerPrint
History of using fingerprint as identification
1. Antiquity and the medieval period
Fingerprints have been found on ancient Babylonian clay tablets, seals, and pottery. They have also been found on the walls of Egyptian tombs and on Minoan, Greek, and Chinese pottery, as well as on bricks and tiles from ancient Babylon and Rome. Some of these fingerprints were deposited unintentionally by the potters and masons as a natural consequence of their work, and others were made in the process of adding decoration. However, on some pottery, fingerprints have been impressed so deeply into the clay that they were possibly intended to serve as an identifying mark by the maker.
%B5%DA%B6%FE%BD%DA_%D6%B8%CE%C6%CA%B6%B1%F0
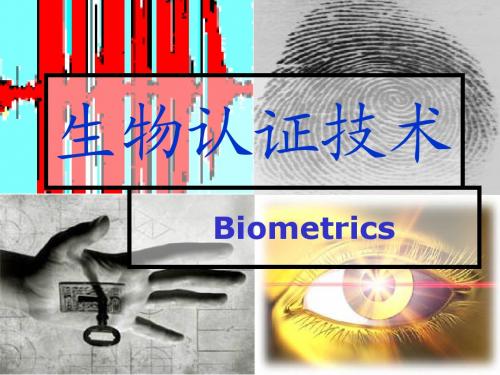
指纹解剖图
表皮由角质层和生发层组成,角质层在最外层,是完全角质化的细胞。 生发层在角质层下面,不断的向表层增生,补充角质层细胞的脱落。 真皮部分包括乳头层和网状层。乳头层有丰富的血管和神经末梢,并 且呈“波浪”状分布,因此在表皮层也表现为“波浪”状。 这里的“波浪”实际上就是指指纹的“纹形” 。
指纹的分类
Three levels of a fingerprint’s friction ridge
the flow of the friction ridges (Level 1 Detail) the presence or absence of features along the individual friction ridge paths and their sequence (Level 2 Detail the intricate detail of a single ridge (Level 3 Detail).
指纹的局部特征-特征点类型
可分为6类,最典型的是终结点和分叉点。
终结点(Ending) 一条纹路在此终结。
分叉点(Bifurcation) 一条纹路在此分开成为两条或更多的纹路。
分歧点(Ridge Divergence) 两条平行的纹路在此分开。
指纹的局部特征-特征点类型
孤立点(Dot or Island) 一条特别短的纹路,以至于成为一点。
Latent Prints
Once the finger touches a surface, body perspiration(汗 液) and/or oils present on the finger ridges are transferred to that surface, leaving an impression. Prints deposited in this manner are invisible to the eye and are commonly referred to as latent or invisible fingerprints.
fingerprintidentification(指纹识别)
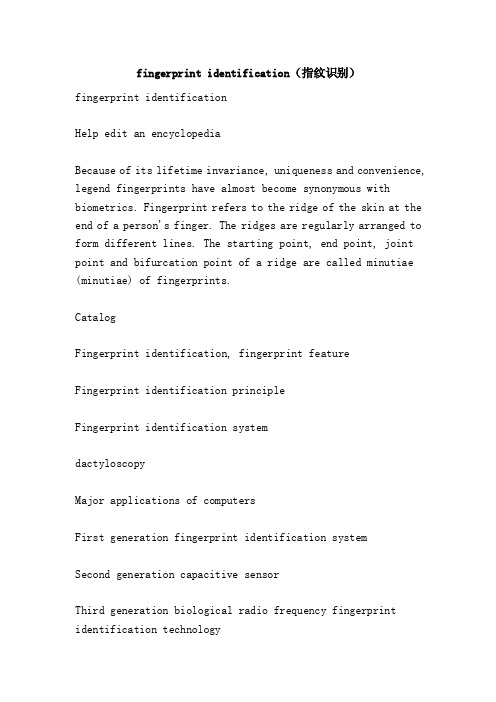
fingerprint identification(指纹识别)fingerprint identificationHelp edit an encyclopediaBecause of its lifetime invariance, uniqueness and convenience, legend fingerprints have almost become synonymous with biometrics. Fingerprint refers to the ridge of the skin at the end of a person's finger. The ridges are regularly arranged to form different lines. The starting point, end point, joint point and bifurcation point of a ridge are called minutiae (minutiae) of fingerprints.CatalogFingerprint identification, fingerprint featureFingerprint identification principleFingerprint identification systemdactyloscopyMajor applications of computersFirst generation fingerprint identification systemSecond generation capacitive sensorThird generation biological radio frequency fingerprint identification technologyAlgorithm overview, fingerprint identification, fingerprint featuresFingerprint identification principleFingerprint identification systemdactyloscopyMajor applications of computersFirst generation fingerprint identification systemSecond generation capacitive sensorThird generation biological radio frequency fingerprint identification technologyAlgorithm overviewEdit this section of fingerprint identification profilesFingerprint characteristicsEnglish Name: (fingerprinting)Fingerprint identification principleFingerprint identification refers to the identification of minutiae by comparing the minutiae of different fingerprints.Because each person's fingerprints are different, that is, between the ten fingers of the same person, there is also a significant difference between fingerprints, so fingerprints can be used for identification. In fact, in ancient China, early use of fingerprint (fingerprint) to sign. In 1684, Grew, the botanical engineer, published his first scientific paper on fingerprints. Fingerprint identification principleIn 1809, Bewick used his fingerprints as a trademark. In 1823, Purkije dissected the fingerprints into nine categories. In 1880, Faulds in nature called for fingerprints to be used to identify criminals. In 1891, Galton proposed the famous Galton classification system. After that, the British, American, German and other police departments have adopted fingerprint identification as the main method of identification. Along with the development of computer and information technology, FBI and Paris police station began to research and develop automatic fingerprint identification system (AFIS) for criminal case detection in 60s. At present, the automatic identification system has been widely used in police stations all over the world. In 90s, automatic fingerprint identification system for personal identification was developed and applied. Because each stamp of the range is not exactly the same, focus on the different will lead to different degree of deformation, and there are a lot of fuzzy fingerprint, feature extraction and how to realize the correct matching, fingerprint recognition technology is the key. Fingerprint identification technology involves many fields, such as image processing, pattern recognition, machine learning, computer vision, mathematical morphology and wavelet analysis.Edit this section of fingerprint identification systemPreprocessing system of fingerprint identificationFingerprint identification system is a typical pattern recognition system, including fingerprint image acquisition, processing, feature extraction and peer to peer module. Fingerprint image acquisition: through a special fingerprint acquisition instrument, you can collect living fingerprint images. At present, fingerprint acquisition instruments mainly include living optics, capacitive and pressure-sensitive devices. For the technical indicators such as resolution and acquisition area, the public security industry has formed international and domestic standards, but other standards are still lacking. According to the fingerprint area can be divided into rolling press fingerprint and plane fingerprints, public security industry commonly used rolling press fingerprint. In addition, fingerprint images can be obtained by scanners, digital cameras, and so on. Fingerprint image compression: large capacity fingerprint database must be compressed and stored to reduce storage space. The main methods include JPEG, WSQ, EZW and so on. Fingerprint image processing includes fingerprint area detection, image quality judgment, direction and frequency estimation, image enhancement, two value reduction and thinning of fingerprint images.Edit this section of fingerprint classificationFingerprint identification securityThe pattern is the basic classification of fingerprints, whichis divided into the basic pattern of the center pattern and the triangle. Of or relating to the shape of a central line. In our country, the ten fingerprint analysis divides the fingerprint into three major types and nine forms.In general, the automatic fingerprint identification system divides the fingerprint into arcuate (arc, account, shape), skip (left, right, left), bucket and miscellaneous patterns. Extraction form and detail features of fingerprint: fingerprint features including Center (upper and lower) and delta (left and right), the details of fingerprint feature points including the starting point and end point, combining ridge point and bifurcation point. Fingerprint matching: it can be coarsely matched according to the shape of the fingerprint, and then use the fingerprint shape and minutiae to make the exact matching, and give the similarity score of the two fingerprints. According to the different applications, the similarity score of the fingerprint is sorted or given whether the result of the same fingerprint is same.Edit the main application of this paragraphFingerprint recognition keyboardApplications in computersIn today's computer applications, including many very confidential file protection, most of them use the "user ID+ password" approach to user authentication and access control. However, if the password is forgotten or stolen by others, the security problems of the computer system and files arethreatened. With the development of science and technology, fingerprint identification technology has gradually begun to enter the computer world. At present, many companies and research institutions in the field of fingerprint recognition technology achieved great progress breakthrough, many application products introduced the combination of fingerprint identification and traditional IT technology perfect, these products have been recognized by more and more users. Fingerprint identification technology is applied in the business field of the relatively high security requirements, and a well-known brand in the business field of mobile office building Fujitsu, Samsung and IBM and other international has a fingerprint recognition system technology and application of mature, the following brief introduction on the application of fingerprint identification system in the notebook computer.First generation fingerprint identification systemAs we all know, two years ago, some brands of notebooks used fingerprint identification technology for user login identification, the first generation of optical fingerprint readerHowever, the fingerprint system introduced at the time belonged to the optical identification system, which should belong to the first generation of fingerprint identification technology. Optical fingerprint recognition system because light can not penetrate the surface of the skin (dead skin layer), so can only scan the surface of finger skin, or scan the dead skin layer, but not deep dermis. In this case, the cleanliness of the finger surface directly affects the recognition effect. If the user'sfinger is stuck with more dust, there may be an identification error. And, if people follow a finger, a fingerprint fingerprint recognition system, it may also be, for users, it is not very safe and stable.Second generation capacitive sensorLater, the second generation of capacitive sensors, capacitive sensor technology is the use of alternating command and arrangement and sensor panels, alternating plate of the second generation capacitive sensorsThe forms are two capacitive plates, and the fingerprint valleys and ridges become dielectric between plates. The dielectric constant sensor to detect changes between the generated fingerprint image. But because the sensor surface is the use of silicon material is easy to damage lead to loss of life, and it is through fingerprint valleys and ridges to form a concave convex fingerprint image so the dirty fingers wet fingers, fingers difficult low recognition rate.Third generation biological radio frequency fingerprint identification technologyTo today, the third generation of RF biological fingerprint recognition technology (radio frequency fingerprint core technology principle leather (line collector)), RF sensor technology is through the sensor itself emits trace RF signal to penetrate the epidermal layer, finger control layer texture measurement, to obtain the best fingerprint image. Therefore, on the dry finger, the Han third generation biological RFsensorsDry fingers, fingers and other difficulties through the fingers can be as high as 99@%, fingerprint anti-counterfeiting ability, fingerprint recognition principle of sensor only to human dermal skin reaction, fundamentally eliminate the artificial fingerprint, wide temperature range: especially suitable for cold or hot special area.Radio frequency technology is the most reliable and powerful solution because RF sensors produce high quality images. In addition, the high quality image also allows the reduction of the sensor, no need to sacrifice reliability certification, thereby reducing the cost and makes the application of RF sensor thought to any field of mobile and unfettered in size.Edit this section algorithm overviewAs people usually put in fingerprint into dustpan, bucket type, in the research of automatic fingerprint recognition, fingerprint is divided into five types: arch, left and right ring ring, pointed arch, vortex type (i.e. "bucket"). The main purpose of fingerprint classification is to facilitate the management of large capacity fingerprint libraries, and to reduce the search space and speed up the fingerprint matching process. Fingerprint classification is based on the overall flow direction of fingerprint ridges or valleys, and the core point of fingerprints. Many researchers have tried to solve the problem of fingerprint classification, but the classification algorithm still has a higher recognition rate. How to improve the accuracy of fingerprint classification is a key problem inautomatic fingerprint identification research.。
描写指纹的英语作文
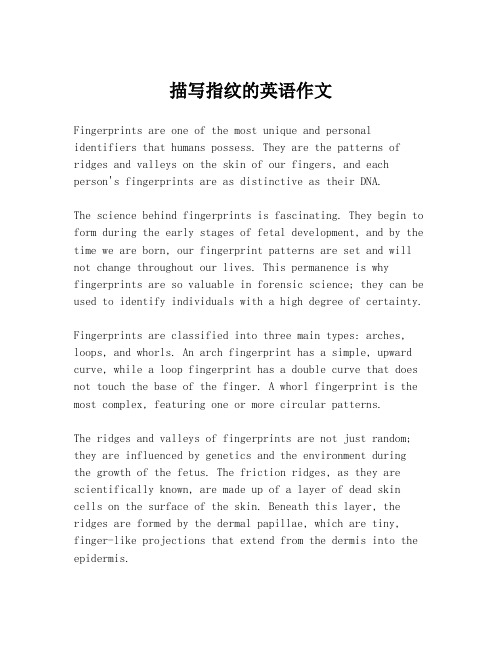
描写指纹的英语作文Fingerprints are one of the most unique and personal identifiers that humans possess. They are the patterns of ridges and valleys on the skin of our fingers, and each person's fingerprints are as distinctive as their DNA.The science behind fingerprints is fascinating. They begin to form during the early stages of fetal development, and by the time we are born, our fingerprint patterns are set and will not change throughout our lives. This permanence is why fingerprints are so valuable in forensic science; they can be used to identify individuals with a high degree of certainty.Fingerprints are classified into three main types: arches, loops, and whorls. An arch fingerprint has a simple, upward curve, while a loop fingerprint has a double curve that does not touch the base of the finger. A whorl fingerprint is the most complex, featuring one or more circular patterns.The ridges and valleys of fingerprints are not just random; they are influenced by genetics and the environment during the growth of the fetus. The friction ridges, as they are scientifically known, are made up of a layer of dead skin cells on the surface of the skin. Beneath this layer, the ridges are formed by the dermal papillae, which are tiny, finger-like projections that extend from the dermis into the epidermis.The use of fingerprints in identification has a long history, dating back to ancient China where they were used incontracts and legal documents. However, it was not until the late 19th century that Sir Francis Galton developed a systematic method for classifying and recording fingerprints, which laid the foundation for modern fingerprinting techniques.Today, fingerprints are used for a variety of purposes beyond criminal investigations. They are used in biometric security systems, to unlock smartphones, and even to authenticate payments. The technology has advanced to the point where fingerprints can be scanned and analyzed digitally, makingthe process faster and more accurate.In conclusion, fingerprints are a remarkable aspect of human biology. They are not only a testament to the uniqueness of each individual but also a testament to the advancements in technology and science that allow us to harness this uniqueness for practical purposes. As we continue to innovate, the role of fingerprints in our lives is likely to expand, making them an even more integral part of our personal and professional security.。
- 1、下载文档前请自行甄别文档内容的完整性,平台不提供额外的编辑、内容补充、找答案等附加服务。
- 2、"仅部分预览"的文档,不可在线预览部分如存在完整性等问题,可反馈申请退款(可完整预览的文档不适用该条件!)。
- 3、如文档侵犯您的权益,请联系客服反馈,我们会尽快为您处理(人工客服工作时间:9:00-18:30)。
Pattern Recognition 39(2006)2131–2140/locate/patcogFingerprint matching using ridgesJianjiang Feng ∗,Zhengyu Ouyang,Anni CaiSchool of Telecommunication Engineering,Beijing University of Posts and Telecommunications,Box 113,Beijing 100876,PR ChinaReceived 15February 2006;accepted 11May 2006AbstractTraditionally,fingerprint matching is minutia-based,which establishes the minutiae correspondences between two fingerprints.In this paper,a novel fingerprint matching algorithm is presented,which establishes both the ridge correspondences and the minutia correspondences between two fingerprints.First N initial substructure (including a minutia and adjacent ridges)pairs are found by a novel alignment method.Based on each of these substructure pairs,ridge matching is performed by incrementally matching ridges and minutiae,and then a matching score is computed.The maximum one of the N scores is used as the final matching score of two fingerprints.Preliminary results on FVC2002databases show that ridge matching approach performs comparably with the minutia-based one.᭧2006Pattern Recognition Society.Published by Elsevier Ltd.All rights reserved.Keywords:Fingerprint;Minutiae;Ridge matching;Curve matching;Alignment;Dynamic programming1.IntroductionFingerprints have been used in identification of individ-uals for many years because of the famous fact that each finger has a unique pattern.Many fingerprint identification and verification methods have been proposed,such as im-age correlation [1],graph matching [2],structural matching [3,4],and matching with transform features [5],and so on.Among them the most widely used one is methods based on point pattern matching [6,7].However,fingerprint recognition is still a challenging problem due to the following difficulties:(1)Low quality fingerprint images are often captured dueto dry or wet skin,dirty or injured fingers,and non-uniform pressures.Feature detector operated on such images will miss genuine minutiae and introduce spu-rious minutiae.∗Corresponding author.Tel.:+861062283118x15;fax:+861062285133.E-mail addresses:fjianjiang@ (J.Feng),brian122@ (Z.Ouyang),annicai@ (A.Cai).0031-3203/$30.00᭧2006Pattern Recognition Society.Published by Elsevier Ltd.All rights reserved.doi:10.1016/j.patcog.2006.05.001(2)The solid-state sensors are increasingly used,whichcapture only a portion of a finger.This causes the de-ficiency of minutiae information.In such a case,it is difficult to make a reliable decision whether two finger-prints are from the same finger.(3)The imaging process introduces elastic deformation inridge pattern and minutiae locations of the fingerprint image.While large bounding box can be used during matching to tolerate it,the side-effect is that the false accept rate will increase.In recent years,new representations of fingerprint image and new matching algorithms have been proposed to re-solve the problems above.FingerCode representation and matching scheme introduced by Jain et al.[5],which cap-tures the global and local features of fingerprints,is robust to low quality images and has an advantage of fixed-length feature vectors.Ross et al.[8]present a hybrid matcher that combines minutiae and texture features.Tico and Kuosma-nen [9]introduce an orientation-based minutia descriptor to identify corresponding minutiae and compute the matching score.Kovács-Vajna [10]uses a triangular matching method to deal with the nonlinear deformation,which is based on the fact that local distortion is less than global distortion.2132J.Feng et al./Pattern Recognition39(2006)2131–2140Fig.1.(a)Intensity image,(b)ridge image,(c)synthesized image. Bazen[11]estimates nonlinear distortion between two minu-tiae sets using thin-plate splines,and removes the distortion prior to the matching stage.All these methods perform rea-sonably well in certain circumstances;some methods do well for low qualityfingerprints,some do well when nonlinear deformation exists,and others do well when the overlapped region of twofingerprint images is small.In this paper,however,a novel approach based on ridges is proposed with the aim to try to solve all these problems. Ridge image,also called thinned image or skeleton image,is an intermediate image in many feature extraction algorithms. Since minutiae are generally thought of as enough to identify a person,ridge image is just used to extract minutiae from it. After that,the ridge image is discarded.However,we think ridge images have much more usages.In our opinion,ridge image has the following features:(1)Ridge image is an effective representation of thefin-gerprint image.From a ridge image,we can synthesize an image similar to the enhanced version of the orig-inalfingerprint image.On the contrary,it is definitely impossible to do so from a minutia set.An example of a ridge image and its synthesized image is given in (Fig.1).The synthesized image is generated using the following steps.To compute the distance transform ofa ridge image(b),to replace the intensity value greaterthan a threshold T(e.g.5)with T,and to scale the inten-sity range[0T]to[0255],then we can obtain a syn-thesized image(c)that looks like the enhanced version of the originalfingerprint image(a).(2)Ridge image is also a compact representation of thefingerprint image.Ridge images can be efficiently ap-proximated by polygonal lines,so the size of a template file is small.We have conducted an experiment and found that forfingerprints in FVC2002DB1(388*374, 500dpi),the average size of templatefiles is1.3KBytes, which meets the storage requirement of“light”system in FVC2004competition[12].(3)Similar minutiae patterns do not mean similar ridge pat-terns.Actually from experiments,we observed that the ridge patterns of most differentfingerprints which have similar minutiae patterns are significantly different. (4)Unlike minutiae whose distribution on afingerprintseems to be random,ridges cover the whole regionof afingerprint.As a result,with the reduction of the effective region of twofingerprints,the performance of the ridge-based system will not degrade dramatically.(5)The topology information in ridge patterns is reliable(especially in the direction normal to ridges)and invari-ant to nonlinear distortion.Ridges have been used for different purposes by some researchers.Ridges associated with corresponding minutiae are used to align two minutiae patterns by Jain[7].Fin-gerprint classification algorithm in[13]is based on fea-tures extracted from ridge images.Ridges associated with corresponding minutiae are used to estimate the nonlinear distortion between twofingerprints[14].Although ridges have been used in a number of aspects related tofingerprint recognition technology,to our knowledge,there have been no work published that reports matching two ridge images directly.The algorithm proposed in this paper is novel on that it establishes both the ridge correspondences and the minutia correspondences between twofingerprints.The algorithm consists of three stages:preprocessing,alignment,and matching.In the preprocessing stage,ridges are extracted from the thinned image and sampled equidistantly,and re-lations between ridges and minutiae are established.In the alignment stage,a set of N initial substructure pairs is found using a novel approach.In the matching stage,for each of the N initial substructure pairs,ridge matching is performed to produce a matching score.Finally,the maximum of the N scores is used as thefinal matching score of the two fingerprints.The idea underlying our alignment algorithm focuses on how to choose a reliable local feature pair as the base of matching.This is accomplishedfirst by defining a substruc-ture that contains as much local information(one minutia and several ridges)as possible,and secondly byfinding the substructure pair which have the most consistent substruc-ture pairs around.In our matching algorithm,during the process of ridge matching,minutiae are also paired,and the matching score is computed according to both the matched minutiae and the matched ridges.Many existing algorithms use a single global transfor-mation to align twofingerprints[7,9].Different from these algorithms,we apply different local transformations in different regions.The transformation is estimated using matched substructures,and applied to nearby ridges. Experiments have been conducted on FVC2002databases [15]and the preliminary results have demonstrated the validity of the proposed approach.The rest of the paper is organized as follows.The next three sections are,respectively,devoted to the three stages of the algorithm,namely,preprocessing,alignment and match-ing.In Section5,the experimental results and evaluation are presented.Finally in Section6,summary and plans for future work are given.J.Feng et al./Pattern Recognition 39(2006)2131–214021332.Preprocessing 2.1.Feature extractionOur matching algorithm is based on two types of features:minutiae and ridges.For a given grayscale fingerprint image,we obtain the ridge image (thinned image)and the minutiae set using an algorithm developed in our laboratory,which follows the common preprocessing steps in traditional minu-tiae matching algorithms.First the directional field is com-puted and the fingerprint is segmented into the foreground region and the background region.Then directional filtering is performed to improve the image quality,and the enhanced image is binarilized and thinned to produce the ridge image.At last minutiae are detected on the ridge image.2.2.Ridge representationDue to noise in fingerprint images and imperfectness of the feature extraction methods,there are complex structures,such as loops and bridges,in the obtained ridge image.In order to make the ridge structures simple and consequently the matching algorithm easier,an operation,called clear-up,is performed:(i)closed ridges are disconnected at an arbitrary point;(ii)ridges associated with bifurcations are split into three ridges;(iii)short ridges are removed.An example is given in Fig.2to illustrate the effect of this operation.Each ridge in the cleared ridge image is sampled at a constant interval to obtain a short list of sampled points.The ridges in one fingerprint are then represented as SP ={{(x i,j ,y i,j ,s i,j )}nr i j =1}N ri =1,where x i,j and y i,j denotes the coordinates of the j th point of the i th ridge,N r the num-ber of ridges in the ridge image,nr i the number of points of the i th ridge,s i,j the matching status of the point,s i,j being 0represents the point has been matched;otherwise s i,j is set to the segment number of the sub-ridge that the point belongs to.The segment number uniquely identifies the sub-ridge in the ridge considered.For example,dur-ing the matching,the statuses of the points of a ridge are [2222000000011111].This means that,at that time,the middle portion of the ridge is matched and the sub-ridges 1and 2areunmatched.Fig.2.(a)Intensity image (500dpi),(b)ridge image before clear-up,(c)ridge image after clear-up and sampling (the sampling interval is 6pixels,and the sampled points areconnected).Fig. 3.b and c are the left and the right neighboring points of a i ,respectively.2.3.Relations between ridgesAdjacent relations between ridges are important con-straints for ridge matching.We use the relations between points of adjacent ridges to represent the relations between ridges since each ridge has been represented by a set of sampled points.For a point,a i ,on a ridge,draw a line segment centered at a i ,normal to the local ridge direction,with a length of w 1pixels.On each side of the ridge,the nearest point to a i on the first ridge intersected by the line segment is regarded as a neighboring point of a i .A neighboring point of a i (if have)is classified as a left or right neighboring point by checking its position when moving along (a i −1→a i →a i +1).An example is given in Fig.3.To record the neighborhood information of a point,the point representation [x,y,s ]is expanded to [x,y,s,lr,lp,rr,rp ],where lr and lp denote the ridge number and the point number of the left neighboring point respectively,rr and rp denote those of the right neighboring point.If a point has no neighboring point on either side,the corresponding element is set to −1.3.AlignmentSince the pose of different impressions of the same fin-ger can be different,the correspondences between features (minutiae,or sampled points of ridges)of two fingerprints are ambiguous.In such cases,the search for the best corre-spondence in the space of all possible correspondences will be time-consuming.The objective of the alignment process is to recover the transformation between two fingerprints by some means and to align them as well as possible.After that,the ambiguity of correspondence is reduced greatly and the search for the best correspondence will be less complex.Alignment is generally done by finding the most similar substructure pairs [7,9]or by finding the substructure pairs most supported by other substructure pairs [17,18].Such substructures can be minutiae subsets [17,18]or ridges as-sociated with minutiae [7].In this paper,we propose a novel approach to aligning two fingerprints.The substructure we used consists of one minutia and several associated ridges.The similarity of sub-structures and the support from other substructure pairs are2134J.Feng et al./Pattern Recognition 39(2006)2131–21401 12 43 54 35 21 2 2 33 14 65 7 or (b)(c)Fig.4.(a)Substructure of a termination (solid circle represents the minutia,hollow circle represents the projection point,and the labels of ridges are given),(b)substructure of a bifurcation,(c)two possible correspondences between ridges when a termination is matched to a bifurcation.combined to measure the goodness of substructure pairs.Since using only the best one for alignment may be too risky,top N substructure pairs can be used,respectively.3.1.SubstructureIn our approach,a substructure is constructed with the following way.First,each minutia is classified into termi-nation or bifurcation by counting the number of ridges in a 3×3window centered at this minutia.If the number is 1,the minutia is a termination;if the number is 3,it is a bifurcation.For a termination,in addition to the ridge that the termination belongs to,two more adjacent ridges are in-cluded (see Fig.4(a)).For a bifurcation,in addition to the three ridges that the bifurcation belongs to,two more adja-cent ridges are also included (see Fig.4(b)).Each adjacent ridge is split into two sub-ridges by a neighboring point of the minutia,which is called the projection point of the minu-tia,and each sub-ridge is assigned a label.The ridges of a substructure are labeled according to the order:first,the ridge that the minutia belongs to,and then the adjacent sub-ridges of the minutia.The three ridges that the bifurcation belongs to are labeled by checking the rela-tive directions between the ridges.The adjacent sub-ridges of the minutia are labeled by checking the relative positions and the relative directions of the sub-ridges with respect to the minutia.Figs.4(a)and (b)show examples for labeling of substructures of termination and bifurcation respectively.The necessary condition for a match of two substructures is that their minutiae are of the same type and their corre-sponding ridges have the same labels,or their minutiae are of different types,but their ridge labels have one of the two possible correspondences shown in Fig.4(c).There are several advantages for choosing such substruc-tures:(i)it contains all the topological information (minu-tia and ridges)in a local area;(ii)it allows different types of minutiae to be matched.This is desirable since the type of minutiae (termination and bifurcation)cannot be reliably detected during preprocessing;(iii)this helps to estimate de-formation more accurately compared with based only on one ridge,when the ridge density of the two fingerprints differs.The substructures contained in a fingerprint imageare denoted as AR ={{(r i,j ,sp i,j ,ep i,j )}7j =1}N mi =1,where (r i,j ,sp i,j ,ep i,j )represent the ridge number,the point number of the starting point,and the point number of theendpoint of the j th labeled ridges in the i th substructure,and N m the number of substructures (same as the num-ber of minutiae)in the fingerprint image.The element with no corresponding ridge is set to −1.For easy check of topological relations among substructures,associated minutiae of ridges in a fingerprint image are recorded.Here associated minutiae of a ridge mean minutiae on the ridge or outside the ridge but in the substructures that contain portions of the ridge.The associated minutiae aredenoted as AM ={{(m i,j ,p i,j ,label i,j )}n ij =1}N r i =1,where (m i,j ,p i,j ,label i,j )represent the minutia number,the point number of the minutia (or the projection point when the minutia lies outside the ridge),and the label of the sub-ridge in the substructure for the j th associated minutia of the i th ridge.3.2.Similarity between substructuresThe objective of this step is not to compute a similarity degree between two substructures,but to give a binary de-cision if these two substructures are possibly matched.For each pair of corresponding ridges,r 1and r 2,which both contain more than four sampled points,the similarity degree of them is computed as follows.Let p 1,1and p 2,1be the starting points of r 1and r 2,p 1,2and p 2,2be the endpoints of r 1and r 2.Assume the shorter one of two ridges is r 1,p 1,2is the i th point counting from p 1,1,and q is the i th point counting from p 2,1.Then the similarity degree between the two ridges issr =1−|d 1−d 2|/max (d 1,d 2),(1)where d 1denotes the Euclid distance between p 1,1and p 1,2,and d 2the Euclid distance between p 2,1and q .Assume that the similarity degrees of n pairs of ridges are computed for a pair of substructures.These two substructures are regarded as possibly matched,if the following two conditions are both satisfied:sr i > 1,∀i ∈[1,2,...,n ],(1/n)n i =1sr i > 2,(2)where 1and 2are two thresholds,and 1< 2.3.3.Support from other substructure pairsTo compute the support of a substructure pair,which is possibly matched,from other substructure pairs,we perform a fast match for minutiae associated with each pair of cor-responding ridges in these two substructures,and take the total number of matched minutiae as the support degree.For each pair of corresponding ridges in the substructure pair m 1,1and m 2,1,we search the two lists of associated minutiae for matched minutiae (see Fig.5).Take a pair of ridges as example (the wider ridges in Fig.5).Two associ-ated minutiae,m 1,2and m 2,2,are said to be matched if the lengths (in terms of the number of sampled points)of theJ.Feng et al./Pattern Recognition 39(2006)2131–21402135mFig.5.Match associated minutiae of a pair of corresponding ridges (wider ridges).two sub-ridges between two pairs of reference points (minu-tiae or projection points)differ less than 3,and the two sub-ridges are correspondent ridges in the substructure pair m 1,2and m 2,2.In Fig.5,in addition to m 1,2and m 2,2that are matched,m 1,3and m 2,3are also matched.The substructure pairs with the top N degrees of support will be selected as the initial substructure pairs for matching.3.4.Estimation of transformation between two substructuresWhile several researchers [11,14]have used thin plate spline to model the global deformation between finger-prints,we found affine transformation can approximate the local deformation between fingerprints well.In addition,estimation and application of affine transformation need less computation.Therefore,we choose affine transformation as permitted transformation and employ local transformations of matched substructure pairs to model nonlinear deforma-tions in fingerprint images.The transformation parameters are estimated based on corresponding point sets of the substructure pair using the least square error technique.4.MatchingGiven an initial substructure pair obtained in the align-ment stage,we will gradually match other ridges and minutiae.A list,MRList ,is used to store matched ridges,and another one,MMList ,is used to store matched minutiae.The algorithm repeatedly generates candidate ridge pairs (CRP)and matches them,until no more ridges can be matched.A CRP consists of the ridge numbers,segment numbers,and candidate point pairs (CPP)of the two ridges (or sub-ridges).A priority queue,CRPQueue ,is used to store CRPs.The CRP with more CPPs will have higher priority value.The matching algorithm is described as follows.(1)Generate CRPs for the initial substructure pair.Estimatethe affine transformation between the two substructures.Push CRPs,the estimated transformation and priority values into CRPQueue .(2)If CRPQueue is empty,go to step 9;otherwise,pop upthe first CRP,crp ,in CRPQueue .(3)Check the validity of crp .If invalid,then generate newCRPs and push the new CRPs into CRPQueue ,and then go to step 2.(4)Match crp using dynamic programming matching algo-rithm.If there is no matched portion between these two ridges,go to step 2.(5)Store the matched portion and set the statuses of pointsto 0.The remaining portions are considered as new sub-ridges and the statuses of points are set to the new segment number.(6)Generate CRPs from the newly matched ridges,andpush CRPs,the current transformation and priority val-ues into CRPQueue .(7)Search the newly matched ridges for matched minutiae.If matched minutiae are found,for each minutia (sub-structure)pair,generate CRPs,compute the transfor-mation and priority values based on the newly matched substructures,and push them into CRPQueue .(8)Go to step 2.(9)Compute the matching score.The above procedure is performed for each of N initial substructure pairs and the one with the highest matching score is chosen as the final result.4.1.Generating CRPsTo reduce the ambiguity of correspondence,CRPs are always selected from ridges adjacent to already matched ridges or matched minutiae.4.1.1.CRPs from matched minutiaeThere are two cases in which we will generate CRPs from matched minutiae.The first case is when the match-ing process starts with an initial minutia pair.The second case is when new minutiae are matched during the matching process.For a given pair of matched minutiae (substructures),the CRPs generated are the corresponding ridges in the substructure pair and the transformation is computed as described in Section 3.The CRPs generated from matched minutiae always have higher priority values than those generated from matched ridges,since local trans-formations are always estimated based on matched minu-tiae,and are better applied in nearer regions.We set the priority value of each CRP generated from matched minutiae to the number of CPPs plus a large positive number.4.1.2.CRPs from matched ridgesGiven a pair of matched ridges,{(SP 1(r 1,p 1,i ),SP 2(r 2,p 2,i ))}N i =1,and the transformation under which these two ridges are matched,we generate CRPs as follows.For each pair of matched points on these two ridges,(SP 1(r 1,p 1,i ),SP 2(r 2,p 2,i )),a pair of neighboring points at the same side is regarded as a CPP,and the pair of ridges that this CPP belongs to is regarded as a CRP.Therefore,from a pair of matched ridges,we may get several CRPs2136J.Feng et al./Pattern Recognition39(2006)2131–2140and each CRP can contain several CPPs.The number of the CPPs contained in a CRP is used as the priority value of this CRP.The transformation of this CRP is the same as the transformation of the matched ridges from which this CRP is generated.4.1.3.Validate a CRPA ridge may be contained in multiple CRPs in CRPQueue. For example,a long ridge in onefingerprint may be paired with two short ridges in anotherfingerprint.During the ridge matching process,the status of points may change. Therefore,before matching a CRP,we check its validity by checking the status of each CPP of the CRP.If the status of any CPP is different from the segment number of this CRP,this CRP is invalid and we divide the orig-inal CRP into several new CRPs that have different seg-ment numbers(note that if all CPPs of the original CRP have been matched,no new CRPs are generated).Then the new CRPs(if have),the transformation of the origi-nal CRP and priority values recomputed are pushed into CRPQueue.4.2.Matching a CRPThe task of comparing two ridges is tofind the longest common sub-curves.Curve matching has been formulated as a string matching problem in[16],where in order to make feature invariant with respect to rotation and translation,cur-vature at sampled points has been used as the characters. In this work,however,ridges have been aligned through lo-cal transformation,so that coordinates of sampled points are directly compared for matching.Two points whose Eu-clidean distance is less than a threshold are regarded as pos-sible matching.The matching procedure accepts a CRP and the corre-sponding transformation as inputs and proceeds as follows (see Fig.6for an example of matching two ridges).(1)Take the two sub-ridges represented by the CRP,de-noted as{a i}M i=1and{b j}N j=1,and align them using the given transformation.(2)A2D array T(i,j),i=1,...,M;j=1,...,N iscreated,and its element T(i,j)is equal to0or1,which, respectively,means that the Euclidean distance between two points,a i and b j,is greater than or less than a threshold.(3)Dynamic programming technique is used tofind thelongest1string in T,which satisfies the following con-ditions:(1)indices monotonously increase;(2)changes between adjacent indices should be less than3.(4)Array T isflipped horizontally,and the operation in step3is performed again to obtain another1string.(5)If the longer1string is longer than3,it is selected asthe matching result;otherwise the two ridges have no matched portion.111213a1a2a3a4a5a6a7a8a9a10a11a12a13b1000 0100000 0 00 00 00 00 0b20 0 0110000b30 0 0 00010 0b40 0 0 00000 0b50 0 00 00000 1 0a1a2a3a4a5a6a7a8a9a10a11a12a13 Before11 1 1 1 1111111After 1111 1 00000 0 222b1b2b3b4b5Before 111 1 1After000 0 01(a)(b)(c)(d)Fig. 6.Matching two ridges,a and b,by using DP matching.(a) Two aligned ridges with points labeled;(b)table T in which the continuous and longest1string is searched.The matching result is {(a5,b1),(a6,b2),(a8,b3),(a9,b4),(a10,b5)};(c)the changes of the status of a before and after matching;and(d)the changes of the status of b before and after matching.4.3.Detecting matched minutiaeWhen a pair of ridges is matched,we check the two pairs of endpoints of these two ridges for matched minutiae.First, select a pair of matched points on the two ridges as reference. If the two minutiae are possibly matched as described in Section3.2,and neither of them is recorded in the matched minutiae list MMList,and the numbers of sampled points between the minutiae and the references differ less than 3pixels,and the sub-ridges between the minutiae and the references are correspondent ridges in the substructure of these two minutiae,then these two minutiae are matched and stored in MMList.4.4.Matching scoreAs twofingerprints may share a small overlapped region, in order to evaluate the similarity degree of them,we should consider only the overlapped pared with the。