Using People and WordNet to Measure Semantic Relatedness
文本情感分析
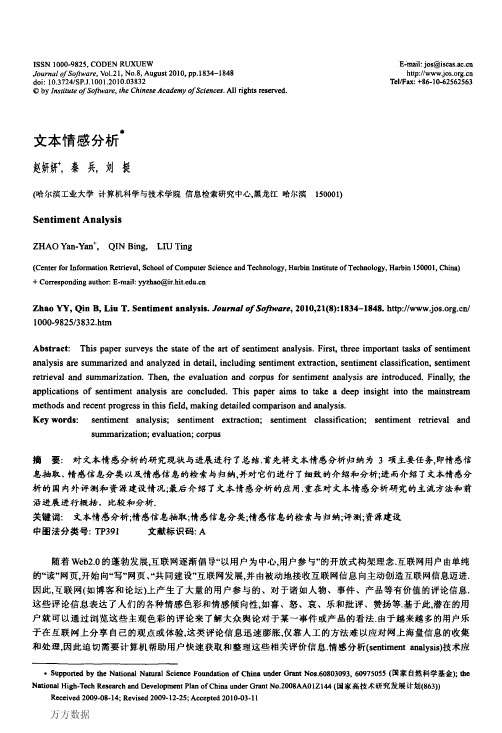
赵妍妍等:文本情感分析1835运而生(本文中提及的情感分析,都是指文本情感分析).文本情感分析又称意见挖掘,简单而言,是对带有情感色彩的丰观性文本进行分析、处理、归纳和推理的过程.最初的情感分析源自前人对带有情感色彩的词语的分析【l】,如,“美好”是带有褒义色彩的词语,而“丑陋”是带有贬义色彩的词语.随着互联网上大量的带有情感色彩的主观性文本的出现,研究者们逐渐从简单的情感词语的分析研究过渡到更为复杂的情感句研究以及情感篇章的研究.基于此,按照处理文本的粒度不同,情感分析可分为词语级、短语级、句子级、篇章级以及多篇章级等几个研究层次【2】.按照处理文本的类别不同。
可分为基于新闻评论的情感分析和基于产品评论的情感分析.其中,前者处理的文本主要是新闻评论,如情感句“他坚定地认为台湾是中国不可分割的一部分”,表明了观点持有者“他”对于事件“台湾归属问题”的立场:后者处理的主要是网络在线的产品评论文本,如“Polo的外观很时尚”。
表明了对评价对象“Polo的外观”的评价“时尚”是褒义的.由于基于产品评论的情感分析可以帮助用户了解某一产品在大众心目中的口碑,因此受到很多消费者和商业网站的青睐.而基于新闻评论的情感分析多用于舆情监控和信息预测中,是国内外评测中重要的评测任务.情感分析涉及多项非常有挑战性的研究任务.本文综合已有的研究成果,将情感分析归纳为3项层层递进的研究任务,即情感信息的抽取、情感信息的分类以及情感信息的检索与归纳,如图1所示.Fig.1Researchframeworkofsentimentanalysis图l情感分析的研究框架情感信息抽取是情感分析的最底层的任务,它旨在抽取情感评论文本中有意义的信息单元.其目的在于将无结构化的情感文本转化为计算机容易识别和处理的结构化文本,继而供情感分析上层的研究和应用服务.如将情感句“我觉得Canon的相片质量不错”转化为如图l所示的结构化文本形式.情感信息分类则利用底层情感信息抽取的结果将情感文本单元分为若干类别,供用户查看,如分为褒、贬两类或者其他更细致的情感类别(如喜、怒、哀、乐等).按照不同的分类目的,可分为主客观分析和褒贬分析;按照不同的分类粒度,可分为词语级、短语级、篇章级等多种情感分类任务.这些分类任务在情感分析初期吸引了大量的研究者.最高层的情感信息的枪索与归纳可以看作与用户直接交互的接口,着重强调检索和!f1纳两项应用.该层次的研究主要在前两项任务即情感信息抽取和分类的结果的基础上进行进一步的加工处理.情感分析是一个新兴的研究课题,具有很大的研究价值和应用价值【3-5】.鉴于此,该研究课题受到国内外越来越多的研究机构的重视.本文在接下来的部分首先分别详细阐述情感分析的3个主要研究任务,重点针对各任务的主流方法和前沿进展进行对比分析;接着介绍国内外主流的评测会议以及现有的资源建设情况:然后介绍情感分析的几个重要应用点;最后,展望情感分析技术的发展趋势.1情感信息抽取情感信息抽取旨在抽取情感文本中有价值的情感信息,它可以看作情感分析的基础任务.一直以来,学术界对它兴趣小减.纵观目前的研究现状,有价值的情感信息单元主要有评价词语(如优秀、好用)、评价对象(如GPS、1848【68】【69】【70】【7l】【72]【73】【74】【75]【76】【78]JournalofSoftware软件学报vol_2l,No.8,August2010TitovI,McDonaldR.Ajointmodeloftextandaspectratingsforsentimentsummarization.In:McKeownKed.Proc.oftheACL2008.MordstOWll:ACL.2008.308—316.BranavanS,ChenH,EisensteinJ.Learningdocument—level8em锄ticpropertiesfromfree-textannotations.In:McKeownKed.proc.oftheACL08:HLT.Morristown:ACL.2008.263-271.KuLW,LiangYT,ChenHH.Opinionextraction,summarizationandtrackinginnewsandBlogcorpora.In:GilY,MooneylU,eds.Proc.oftheAAAI2006SpringSymp.onComputationalApproachestoAnalyzingWeblogs.MenloPark:AAAIPress.2006.OunisI。
中考英语人教版 重难题型突破题型专题三 任务型阅读

4.The scientist did a test and found that trees can grow because of wwwer is a very clean source of energy.This is how wind power works.Wind makes windmills spin(旋转).When the windmills spin,they make electricity.Then we can use the electricity. A lot of people think that wind power is new,but that's not true.For thousands of years,people have used wind to sail boats and move water.We still do those things today,but these days we mostly use wind
Scientists make observations (观察).They may look,or listen.They may read the work of other scientists.↓This gives them ideas.You can do this.You can look around you.You can ask questions. 细节理解题:根据题干的关键词“Paragraph 2”和“make observations”将 第 2 题答案定位此 2 句。从中可知科学家通过看、听或阅读其他科学家 的著作等不同方法来观察物体。故答案为:different ways。
时间计算方法

Architecture and Performance Methods ofA Knowledge Support System ofUbiquitous Time ComputationYinsheng ZhangInstitute of Scientific & Technical Information of China, Beijing, ChinaCity University of Hong Kong,Hong Kong, ChinaEmail: zhangyinshengnet@Abstract— An architecture and main performance methods of a knowledge support system of ubiquitous time computation based on relativity are proposed. As main results, modern time theories are described as certain relations of term-nodes in a tree, and some space-time computation models in a large scale and time computation models in different time measurement systems (institutions) are programmed as interfaces for time computation in complex conditions such as time-anisotropic movement systems or gravity-anisotropic environments.Index Terms—Space-Time, Relativity, Real Time Communication, Time Ontology, Time MeasurementI.I NTRODUCTIONTime computation is so ubiquitous nowadays, not only in analyzing texts with time terms, but also in real time computation even in circumstance across time zones or in quantum application such as satellite positioning systems, time-anisotropic movement systems, gravity-anisotropic environments, or space scale in the cosmos. As the relativity theory and quantum mechanics, which we call modern time theories, have made great advances, time computation is desirable to be made on the new time knowledge. It is well known that an ontology made up of specific terms in relations can succinctly represent knowledge homogeneously structured in syntactic pattern and stratified in entailments or in contents with stem-branch relations, and easily be applied to navigate knowledge by relational calculus, so a time knowledge support system based on time ontology with some computational models is proposed here to suffice requirement of time computation based on modern time theories.II.E XTENSION OF T IME E XPRESSIONTime mostly is expressed in a form of natural number and suitable for a unified time measure system in the Earth. For example, Dan Ionescu & Cristian Lambiri[1], E.-R.Orderog & H.Dierks[2], and Merlin [3] respectively gave time definitions or expressions for the real-time system, which, however, relativity of time, time computation models which define how to calculate time units, are omitted. In contrast to some software application fields’ research, some time science organizations give serial time expressions based on modern time theories, among which the International Astronomical Union (IAU,1991) made time definition widely accepted in a reality frame [4] . Thus we need to integrate these definitions and expressions in a complete and standard form for ubiquitous time. To do this, we give a time expression as follows.The physical quantity of time can be expressed as a 4-tuple:T=< D,U,M,I > (1) where,D: Data about time in quantity, it may be numbers or circle physical signals indicating time, or symbols expressing a time in quantity; that is, D∈{ time reading, tick, time number expression}.U: Unit, the measure unit such as “second”,” day”.M: Model, the mathematical formulae, using which you get a time quantity by mathematical computations.I: Institution, it may be indicated by a code which stipulates what unit U is meaningful, from which start time point S an interval can be fixed, according to what model M about time can be computed. So we use I( ) to indicate determining a time physical quantity by some parameters.For example, you say “2 seconds”, you might refer to two units of the Universal Time i.e., of coordinated universal time (CUT, or UTC) set by IAU and the finally arbitrated by the International Telecommunication Union (ITU). Of course, you probably might not refer to that, but to an atomic time (AT), as it may. Both the quantities can be computed by the corresponding models issued by the related organizations. Here, the institution determines the meanings of the time as a physical quantity and gives the computation methods, so we can give an expression similar with a programming expression as T=I(D,U,M), here, T serves as a return value ,and I, a function for the other parameters.Clearly, to set up a knowledge support system, we need to consider this time expression, its elements in the tuple will constitute the main profiles.© 2013 ACADEMY PUBLISHER doi:10.4304/jsw.8.11.2947-2955Figure 1. The architecture of the knowledge support system ofubiquitous time computation.III. A RCHITECTURE OF THE K NOWLEDGE S UPPORTS YSTEM We designed such an architecture for the knowledge support system developed by the author for the time computation in the complex systems.The system mainly made up of the 4 components that ①Time Knowledge Navigation, ② Time Measurement and Computation Models, ③ Time Expression Semantics Computation Models,④ Time Institution Knowledge Texts.Component ① accepts users’ requests for knowledge relating to the time measuring data, for example, a user requests for a model for computing the derivation between its time readings and a time unit in another space or in a time measurement system. The kernel of Component ① is a tree describing time knowledge profiles, say its branches are classifications of the time knowledge in certain relations. It is a catalogue of classification and relations of time knowledge, and also mappings between the classification and the knowledge in Component ② and Component ③. It contains institutions I in (1), which determines Component ② and Component ③ in logic, however, Component ② and Component ③ are listed for directing call not through the nodes of institutions.Component ② is the mathematical models for time measurement and computation, written in software programs and can be called for other time computation programs.Component ③ and ④ are discussed in number V and VI.IV. T IME ONTOLOGY.4. 0 General Description sThe tree in Component ① is a time ontology based on modern time theories for logically showing and savingall the knowledge term nodes in certain relations.These relations are potential information for deeper application such as inference based on relational calculus. On time ontology, most studies focus on time expressions and computations of relations between these expressions. For example, Moen’s time ontology is about time concepts in linguistics [5][6].; Frank etc. came up with a plan and principles building space-time in 4 dimensions and 5 tiers [7]. The typical extant time ontology see WordNet in the part of time, DAML time sub-ontology [8],Time Ontology in OWL built by W3C [9] ,and NASASWEET (Semantic Web for Earth an Environmental Terminology)[10]. In addition, ISO 19111 [11] and ISO 19112[12] set out the conceptual schema for spatial references based on geographic identifiers. This work shows various profiles of data structure of time description, yet has the limitations that(1) Time it describes is in the periphery of the Earth, but not in cosmos large scales;(2) The time properties are unraveled only on non-symmetry (non-back as an arrow), a little on relativity, singularity and quantum property.This might lead to difficulties in computations based on modern time theories.In contrast with this work, the time knowledge tree in Component ① is a time ontology based on modern time theories (hereafter “TOboMTT”, the main branches see attachment) .The nodes between any two levels in top-bottom constitute relations which are propositions (note that when we say “A and B in a certain relation”, it just says a proposition) stating the main frame of modern time theories. So, in essence, we have :TOboMTT={N,R }={Propositions} (2)here, N,R refer to nodes and relations respectively.The root (0- level) and the nodes in the next (1-level) are as followingz TimeSpace-Time Type Time Type Time Property Time Measure Time ExpressionThe root “Time” constitutes “has ” relations with the nodes in the 1-level. That is, “Time has the Space-Time Types”, “Time has the Time Types”, “Time has the Time Properties”, “Time has the Time Measures”, “Time has the Time Expressions”. These relations are basic profiles of the up-to-date study on time.The relations of the nodes between the 1 and 2 levels continue such propositions of those relations between 0 and 1 levels, for example, we can say “Time has the Space-Time Types like Euclid Space-Time”, here, “Euclid Space-Time” just is a node in the 2nd level. Thus,© 2013 ACADEMY PUBLISHERthe relations between the 1 and 2 levels are “includes ”, like “Space-Time Type includes Euclid Space-Time”. In the following contexts, we intuitively explain the main nodes which express some important assertions of modern time theories.4. 1 SPACE-Time TYPEAccording to Einstein’s field equation, space andtime are integrated. So we must take space as a parameterof time considering the space-time type. Einstein’s fieldequation see (3) [13]1()+=82R Rg g T αβαβαβαβ−Λπ (3)Here, α and β are space-time dimensions, i.e., α, β=0,1,2,3 and 0 denotes time for the left expression; R αβ is Ricci tensor, it is a 4×4 matrix of the 16 components ofsecond order space-time curvature, R is scalar curvature, g αβ is a 4×4 matrix of metric tensor, Λ is cosmological constant, T αβ is energy-momentum tensor, a 4×4 matrixtoo.From (3), we get (4), i.e., the differentiation of square of space-time intervals:2=ds g dx dy αβαβ (4) here, x,y are curvilineal coordinates, s is space-time interval. (4) adopts Einstein summation convention, normally like in physics, that a repeated index (α or β ) implies summation over all values of that indexed. (3) and (4) are well confirmed by some experiments in the scale 10-13 cm (the radius of a fundamental particle) to 1028 cm (the radius of the universe). A space-time type normally defined by a solution of the equations (3) or (4).See some basic nodes: Space-Time Type Euclidean space-time (absolute time) Riemannian space-time Inertial reference frame space-time Non-inertial reference frame space-time Friedmann- Walke space-time…… If (3) or (4) are determined as the nonlinear partial differential equations about g αβ , we call s is Riemannian space-time, which means space-time is of curvature and might not be flat (flatness is just a special instance, i.e., Minkowski space-time, in which gravity is neglected, it is regarded as inertial). In (3) or (4), if the time in different space places is described as absolutely not different , and independently from its different places and velocities, the space-time is Euclidean space-time or Newton space-time. Friedmann-Lemaître-Robertson-Walker space-time, simply Robertson-Walker space-time [14][15] , put forwarded by Robertson and Walker, and meet the inference of Friedman [16] and Lamaitre [17] , describes homogeneous and isotropic space-time in a non-inertial system, for which, cosmological curvature k and cosmological time t are introduced into (3) or (4). k takes 3 constants 0,1,-1 representing 3 possible space-time types: flatness, positive curvature and negative curvature. If R in (3) is a constant, Robertson-Walker space-time will become some special instance: when R =0, itwill be Minkowski space-time; R >0, de_Sitter space-time;R <0, anti-de_Sitter space-time. Bianchy I space-time is more general than Robertson-Walker that the space-time is homogeneousbut might be anisotropic [18]. Taub-NUT space-time adds magnetic and electric parameters into (3) or (4) [19]. Godel space-time adds rotationally symmetric axis into (3) or (4) [20]. Rindler space-time expresses such space-time determined by inertial system and non-inertial system [21][22]. In some special cases, R is not easy to be determined. To solve (3) or (4), some parameters are given for specialtypes of space-time. These special types include spherical and axial space-time, and time’s elapse may be neglected for a space spot. For (4), Schwarzschild space-time [23] isspherically symmetric beyond a mass sphere. A spherewith great mass and a radius less than Schwarzschild radius is a black hole, which is thought to bear only 3 kinds of information of mass, charge and angular momentum. Schwarzschild black hole is considered as one with only mass, while Ressner-Nordstrom black hole, named as Ressner-Nordstrom space-time, with mass and charge [24][25]; Kerr black hole, named as Kerr space-time with mass and angular momentum [26]; Kerr-Newmanblack hole, named as Kerr-Newman space-time [27], simultaneously have information of mass, charge and angular momentum. Some spherically symmetric space-time like Vaidya space-time [28] and Tolman space-time [29] consider time as the variable of the function of mass and curvature. As an axial metric space-time, Weyl-Levi-Civita space-time [30] is typical. . 4. 2 Time TYPEWhen we solely study time, we can primarily dividetime into the 3 types: Proper time Coordinate time Cosmological time Proper time is the elapsed between two events as measured by a clock that passes through both events. In other words, proper time value is from the real readings of the clock set by an observer in a definite space spot (ifthe measured body moves, then the clock spot and the moved body’s end spot are considered as one area for the two spots are so near for a large scale space). © 2013 ACADEMY PUBLISHERCoordinate time is integrated time under a coordinate system. It is not a real readings for a special spot (the difference between the different spots in the system is neglected), but a stipulated (calculated that it should be) time in the system. Proper time multiplied by (1- v2/c2)-2 is coordinate time (v is the velocity of the body, in which an implied observer is, c is light velocity). If we set a clock in a universe coordinate system indicating the integrated time, it would indicate the universal time (t in Robertson-Walker equation).The proper time in the Earth can be expressed in various forms as the follows.Ephemeris Time (ET) [31] was defined in principle by the orbital motion of the Earth around the Sun. Here, ephemeris is based on Julian calendar which had been reformed to be Gregorian calendar lasted to the nowadays.True solar time (apparent solar time) is given by the daily apparent motion of the true, or observed, Sun. It is based on the apparent solar day, which is the interval between two successive returns of the Sun to the local meridian [32].Mean solar time is the mean values of measured time of the intervals between two Sun passing an identical meridian [33].Sidereal Time is based on a sidereal day; a sidereal day is a time scale that is based on the Earth's rate of rotation measured relative to the fixed stars, normally to the Sun [34]. Sidereal time may be Greenwich Sidereal Time (GST) which calculated by Greenwich Royal Observatory in mean data or Local Sidereal Time (LST) which is computed by adding or subtracting the numbers of timezone [35] .Universal Time (UT) is computed by truly measured time data based on rotation of the Earth, it is a Greenwich Mean Time (GMT) and computed from the start of a midnight of Prime Meridian at Greenwich, and it has different versions such as UT0,UT1,UT2 and Coordinated Universal Time (UTC) for the computations from varying data on non-exact time scales of the Earth rotation. UT0 is Universal Time determined at an observatory by observing the diurnal motion of stars or extragalactic radio sources. It is uncorrected for the displacement of Earth's geographic pole from its rotational pole. This displacement, called polar motion, causes the geographic position of any place on Earth to vary by several metres, and different observatories will find a different value for UT0 at the same moment.UT1 is the principal form of Universal Time. While conceptually it is mean solar time at 0° longitude, precise measurements of the Sun are difficult. UT1R is a smoothly tuned version of UT1, filtering out periodic variations due to tides. UT2 is a smoothed version of UT1, filtering out periodic seasonal variations. UTC is an atomic timescale that approximates UT1. It is the international standard on which civil time is based [36].Atomic time applies the principle of stimulated atom radiation in a constant frequency. The Thirteenth General Conference of Weights and Measures define a second that "the duration of 9,192,631,770 periods of the radiation corresponding to the transition between the two hyperfine levels of the ground state of the caesium 133 atom [37] ". That is a unit of International Atomic Time (ATI).The results of atomic time computed by different local laboratories are called local atomic time.Dynamical Time (DT) [38] is inferred from the observed position of an astronomical object via a theory of its motion, ET is a DT based on revolution of the Earth in replace of UT based on rotation of the Earth meet Newton’s time theory; to meet Einstein’s time theory IAU builds two versions of ET respectively in the system of Terrestrial Dynamic Time (TDT) Barycentric Dynamical Time (TDB).Local civil time is the corrected version of UTC by adding timezone numbers and adjusting daylight saving time [35] .Coordinate time includes centroid coordinate time and Earth-centered coordinate time, they are set by IAU.4. 3 Time propertyThe time properties are divided into 4 kinds as follows.Time PropertyAsymmetryRelativitySingularityQuantum propertyAsymmetry is the property human first discovered, it refers to what seems to be an arrow went out in one direction and not back.Relativity means anisotropy against gravity or in a light-like velocity.Singularity is the property of some places, where the present physical laws break down, or it can be thought of as the property of edge of space-time [39].The quantum property of time refers to that of time in the particle-scale, where time appears the stranger phenomena far from the macro-scale as we see. For example, the former -latter sequence in macro-scale might be isochronous in the quantum –scale [40].4. 4 Time measure4. 4.1 CoordinatorThe space-time expressed in (3) or (4) can’t always be indicated by Cartesian system, mostly due to some properties which are difficult to be indicated by Cartesian system, and also due to the singularity in the space-time which normally cannot be indicated by the real number system. So two kinds of coordinates are mainly introduced, they are general coordinates and special coordinates. The former are popular in common sense, and transforming them for a special purpose we get the latter----special coordinates, which mainly for describing some new metrics -solutions of (3), (4) with some© 2013 ACADEMY PUBLISHERsingularity variables, or for some particular space-time areas.The coordinates special for the metrics are introduced as follows.Schwarzschild coordinate indicates spherical symmetry, it sometimes becomes degeneratation of some more general conditions. Schwarzschild coordinate uses sphere coordinate with the radius r≠2GM/C2 and r≠0, here , G is universal gravitational constant, M is the mass. The coordinate is divided into two areas by r >2GM/C2 and r <2GM/C2 and leads to the two metrics in (3): g00= - (1-2GM/rC2) and g11= (1-2GM/rC2) -1.In Schwarzschild coordinate, there is not the expression that r=2GM/C2 (this is a singularity), but tortoise coordinate covers this singularity.Eddington coordinate does not diverge in r=2GM/C2 and r=0 by the linear transformation of the variables.Kruskal coordinate covers r=2GM/C2 and r=0 too, and more general in indicating space-time than tortoise and Eddington coordinate .Lemaitre coordinate covers r=2GM/C2 with a different method to Kruskal coordinate.Rindler coordinate indicates the space-time determined by both inertial and non-inertial system.Weyl coordinate indicates the function of metric and allows to indicate imaginary numbers.Fermi normal coordinate indicates space-like geodesic which is the trajectory that its covariant differential is 0 for (4). “space-like” denotes the velocity in the area is far less than light speed. And its time axis indicates proper time for a non-inertial or locally inertial conditions.Harmonic coordinate indicates harmonic conditions that coordinates in curved space satisfy a D' Alembert equation, it is a Cartesian-coordinate-like one in curved space.Local inertial coordinate indicates Minkowski space-time.The special coordinates for the particular space-time areas are introduced as follows.Centroid coordinate (center-of-mass coordinate system) is one taking the centre of a space area as the origin of coordinate. These coordinates include non-rotating geocentric reference system, rotating geocentric reference system, Barycentric Celestial Reference System (BCRS), International Celestial Reference System (ICRS).Non-rotating geocentric reference system takes the Earth centre as the origin of coordinate . IAU provides the metric and methods for computing proper time.Rotating geocentric reference system is supposed as rotated with the Earth together, its X3 axis is the rotation axis of the Earth, and it is taken as International Terrestrial Reference System (ITRS) by IAU. For the rotation direction is not considered, the time in non-rotating geocentric reference system and rotating geocentric reference system is the same.Barycentric Celestial Reference System (BCRS) is recommended by IAU, its origin is the mass centre of the solar system,its third axis is approximately the rotation axis of the Earth.International Celestial Reference System is a centroid coordinate, it is made up of circle of right ascension and circle of declination of approximate 600 quasars, the coordinates are provided by International Earth Rotation and Reference Systems Service (IERS) Most general coordinates are introduced by the mathematical textbooks, so they are omitted here.4. 4.2 Measure UNITThe frame of time measure unit is as follows:Measure of timeUnits of measureTime intervalDynamical time intervalDuration fixed time intervalTime interval with the duration fixedby an ephemerisIntegral time scaleDynamical time scale is referred to as measured values of time parameters by physical quantities in a physical system. Basically, a proper time interval is a dynamical time scale.The main units of dynamical time scales in the ontology are concerned with ephemeris time units. A second in ephemeris time is defined as the fraction 1/31,556,925.9747 of the tropical year in Julian calendar for 1900 January 0 at 12 hours ephemeris time by International Committee for Weights and Measures (CIPM), from this unit, Julian century, year, week and day can be worked out.An integral time scale is accumulated value copied from a contracted time start point, for example, atomic time scale. So it may be proper time or coordinate time.V. T IME MEASURE AND COMPUTATION MODELSComponent ②is the set of the measure and computation models, which are from two resources: one is from the institutions put forward by some organizations such as IAU stipulating how to measure and computation, another resource is from the exact solutions of the (3) or (4).The models are programmed in Mathematica as the Application Programming Interface (API) so that a users’ programs can call these API.EXAMPLE 1[41]: a model (group) to compute a coordinated universal timeUTC (t) – TAI(t) = ns (5)UTC (t) –UT 1(t)=<0.9s; (6) Here, UTC(t) is a time expressed in coordinated universal time’ institution unit, TAI(t) means a time of Atomic Time International, n is natural number; s is the second, UT 1(t) is a time expressed in UT 1.© 2013 ACADEMY PUBLISHEREXAMPLE 2 is calling from a user’s application for the interface of a model, which is drawn from reference [42] and re-wrote by the author, to get an exact solution of Einstein’s field equation given Roberson-Walker Metric:1 /*An application from users in pseudo-code callingthe model-interface. See the tree in the attachment*/2 e num Space-Time in non- inertial system3 {4 B ianchi I Space-Time,5……6R obertson-WalkerSpace-Time7 /*Here, all the 16 Space-Time in non- inertialsystem in the tree enumerated */8 } Metric[16];9 for(i=0;i<16;i++){10 switch(Metric [i])11 case Robertson-Walker Space-Time:12 input and assign vector:13 v = {t, r, e, phi};141516 M = {-1, R[t]^2/(1 - K (r^2), (r^2) (R [t]^2), (r^2) (Sin[e]^2) (R [t]^2)};Call Einstein [M, v]}“Einstein.m”1 E instein [g_, v_] := Block[2 {invsg, dg1, dg2, dg3, Christf2, dChristf2, Ruv1,Ruv2, Ruv3, Ruv4, RicciTensor, R, EMTensor} 3 EMTensor = {}; (*Save return value.*)(*Calculate the inverse metric of g.*)4 g=DiagonalMatrix[M];5 invsg = Inverse[g];(*Calculate the affine connection.*)6 dg1 = Outer[D, g, v];7 dg2 = Transpose[dg1, {1, 3, 2}];8 dg3 = Transpose[dg1, {2, 3, 1}];9 Christf2 = (1/2) invsg.(dg1 + dg2 - dg3);(*Calculate the Ricci tensor.*)10 dChristf2 = Outer[D, Christf2, v];11 Ruv1 = Table[Sum[dChristf2[[k, i, k, j]], {k, 4}],{i, 4}, {j, 4}];12 Ruv2 = Table[Sum[dChristf2[[k, i, j, k]], {k, 4}],{i, 4}, {j, 4}];13 R uv3 = Table[Sum[Christf2[[k, i, j]] Christf2[[h,k, h]], {k, 4}, {h, 4}], {i, 4}, {j, 4}];14 Ruv4 = Table[ Sum[Christf2[[k, i, h]] Christf2[[h,j, k]], {k, 4}, {h, 4}], {i, 4}, {j, 4}];15 RicciTensor = Ruv1 - Ruv2 - Ruv3 + Ruv4;(*Calculate the Curvature Scalar.*)16R = Sum[invsg[[i, i]] RicciTensor[[i, i]], {i, 4}];(*Calculate the field equation left part.*)17EMTensor = RicciTensor - (1/2) g R ;18return [EMTensor]19]20End[]21EndPackage[]This program is divided into two parts: the first part is user’s input for computation, which is space-time dimensions v in a spherical coordinator, in which, t is the cosmological time (see 4. 2 Time Type), M is Roberson-Walker Metric. Users can input similar metrics for calling the function Einstein[ ],which is saved in the second part, a document Einstein.m, starting from the sentence BeginPackage["Einstein`"]. mathlink.h in VC++ enables to run Mathematica programs in VC++ environment The section Block[] is a function of local variables for calling.Outer[] is to give the partial derivative ∂f/∂x.Transpose[dg1, {1, 3, 2}] is to transposes dg1 so that the k th level in dg1 is the n k th level in the result.D [] is to get partial differential.Table [] is to generate a list of the expression Sum[].Sum[] is to get sum.The line 19 is the computation result of left part of (3), yet the cosmological constant is omitted. The right part of (3) is considered as zero.VI. M ECHANISM AND R UNNING OF T HE A RCHITECTURETOboMTT is designed to be a tree not only for satisfying the structure and classification of knowledge of time, but also for developing the knowledge in Web Ontology Language (OWL), which is based on Resource Description Framework (RDF) in a tree. Thus we can divide TOboMTT into some sub-trees and further expressed them in OWL or RDF. Figure 2 is a sample of Class—SubClass relation in RDF. As a result, navigation of knowledge of time, based on TOboMTT, become navigation of resources and serves, based on eXtensible Markup Language (XML) compatible with both OWL and RDF.A query for a sub-class or property value will give the corresponding answer by rational calculus on a XML scheme. For the example in Figure 2, “Space-Time Type includes Euclid Space-Time ” will be the answer for the query “What kind does the Space-Time Type include?” Therefore, query and answer is the first and direct results of navigation of knowledge of time by TOboMTT.<?xml version="1.0"?>© 2013 ACADEMY PUBLISHER。
高二英语科技成果单选题50题
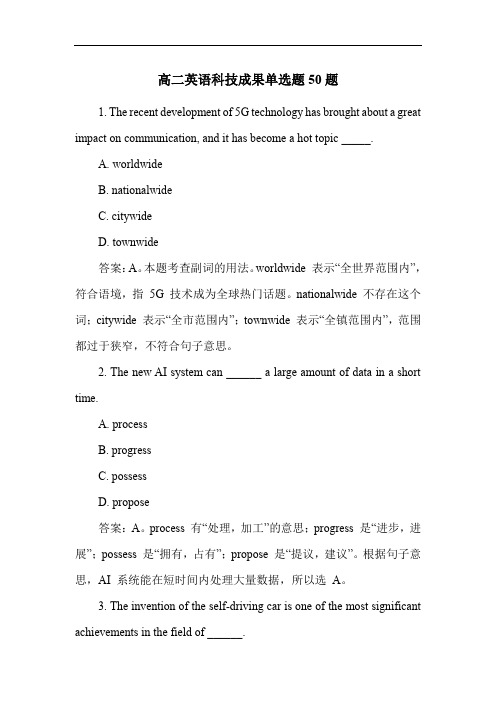
高二英语科技成果单选题50题1. The recent development of 5G technology has brought about a great impact on communication, and it has become a hot topic _____.A. worldwideB. nationalwideC. citywideD. townwide答案:A。
本题考查副词的用法。
worldwide 表示“全世界范围内”,符合语境,指5G 技术成为全球热门话题。
nationalwide 不存在这个词;citywide 表示“全市范围内”;townwide 表示“全镇范围内”,范围都过于狭窄,不符合句子意思。
2. The new AI system can ______ a large amount of data in a short time.A. processB. progressC. possessD. propose答案:A。
process 有“处理,加工”的意思;progress 是“进步,进展”;possess 是“拥有,占有”;propose 是“提议,建议”。
根据句子意思,AI 系统能在短时间内处理大量数据,所以选A。
3. The invention of the self-driving car is one of the most significant achievements in the field of ______.A. transportationB. educationC. entertainmentD. agriculture答案:A。
self-driving car 自动驾驶汽车属于交通运输领域,transportation 表示“交通运输”;education 是“教育”;entertainment 是“娱乐”;agriculture 是“农业”,均不符合题意,故选A。
Theemissionmeasureofthequietcorona,denedby
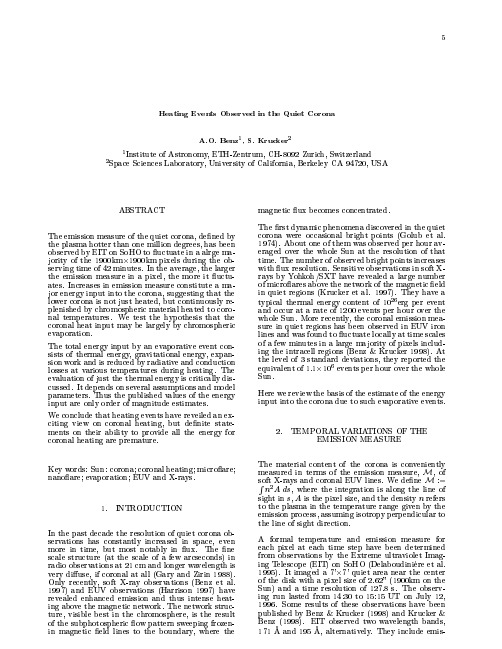
6
sion lines of Fe IX/X and Fe XII, respectively, with diagnostic capabilities for temperatures in the range of 1.1-1.9 106K. These coronal lines dominate the observed parts of the EUV spectrum, and their large photon uxes provide higher sensitivity than previous observations in soft X-rays. The derived parameters are to be taken as formal values, representing weighted means over the sensitive temperature range. Figure 1 displays the resulting emission measure of one pixel vs. time. It is an arbitary example of a total array of 23716 pixels of quiet corona. The average level of emission measure of the pixel is relatively low, indicating that it is not above the network. Note that the emission measure and thus the material content of the corona is signi cantly changing during the 42 minutes of observation. The square of the density integrated along the line of sight peaked round 15:00 UT after an increase lasting 10 minutes. The decay has a similar time constant. This is a very common property of quiet sun coronal observations by EIT. In fact, only 15% of all pixel do not change signi cantly in emission measure (Krucker & Benz 1998).
小学上册第8次英语第1单元期末试卷
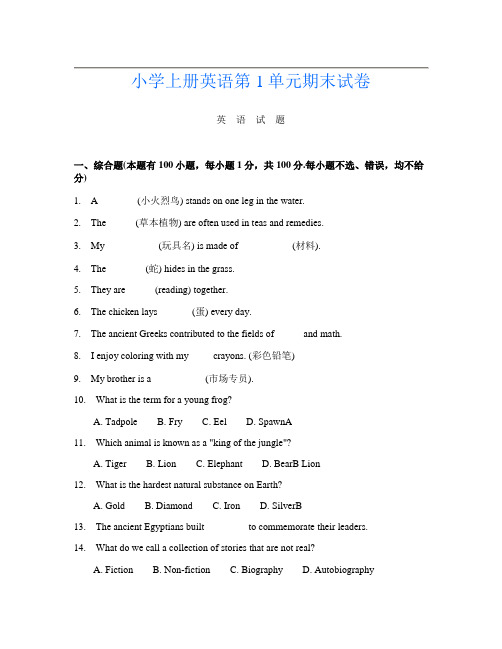
小学上册英语第1单元期末试卷英语试题一、综合题(本题有100小题,每小题1分,共100分.每小题不选、错误,均不给分)1. A _______ (小火烈鸟) stands on one leg in the water.2.The _____ (草本植物) are often used in teas and remedies.3.My __________ (玩具名) is made of __________ (材料).4.The _______ (蛇) hides in the grass.5.They are _____ (reading) together.6.The chicken lays ______ (蛋) every day.7.The ancient Greeks contributed to the fields of _____ and math.8.I enjoy coloring with my ____ crayons. (彩色铅笔)9.My brother is a __________ (市场专员).10.What is the term for a young frog?A. TadpoleB. FryC. EelD. SpawnA11.Which animal is known as a "king of the jungle"?A. TigerB. LionC. ElephantD. BearB Lion12.What is the hardest natural substance on Earth?A. GoldB. DiamondC. IronD. SilverB13.The ancient Egyptians built ________ to commemorate their leaders.14.What do we call a collection of stories that are not real?A. FictionB. Non-fictionC. BiographyD. Autobiography15.What is the name of the famous ship that is a symbol of love?A. TitanicB. Love BoatC. Queen MaryD. USS EnterpriseA16.She has a _____ (colorful) backpack.17.My mom teaches me to be __________ (善良的) to others.18. A lion is a type of ______ that lives in groups called prides.19.What do you call the force that pulls objects toward the Earth?A. MagnetismB. GravityC. FrictionD. PressureB20.I can _____ (count/sing) to twenty.21.What do we call the process of learning through experience?A. EducationB. TrainingC. PracticeD. ApprenticeshipA22.River flows northward through _____ (埃及). The Nile23.What is the process of breathing in called?A. ExhalationB. InhalationC. RespirationD. CirculationB24.What is the primary color of a strawberry?A. BlueB. RedC. YellowD. GreenB25.The ________ (柳树) sways gently in the wind by the river.26.I love to play _______ (篮球) after school.27.What do you call the person who teaches students?A. DoctorB. TeacherC. EngineerD. ChefB28.What do we call a person who studies the stars and planets?A. AstronomerB. GeologistC. MeteorologistD. Biologist29.What color is the grass?A. BlueB. GreenC. RedD. Yellow30.Plants grow from ______ (种子).31.My favorite _____ is a little kitten.32.The library is full of _______ (books).33.I like to play with _____ (积木) in the afternoon.34.What do you call the process of changing a liquid to a gas?A. MeltingB. EvaporationC. CondensationD. Freezing35.How many days are in a leap year?A. 365B. 366C. 364D. 36036.What do we call a large vehicle that carries people?A. CarB. BusC. BikeD. Train37.What do we call the process by which plants make their food?A. DigestionB. TranspirationC. PhotosynthesisD. FermentationC38.The __________ is the temperature at which a substance changes from a liquid to a solid.39.He is a _____ (商人) who sells products online.40.The chef prepares _____ (美味的) meals.41.What is the name of the process plants use to make food?A. PhotosynthesisB. RespirationC. DigestionD. FermentationA42.__________ can live both in water and on land.43.My favorite season is __________ because it brings __________. During this time,I enjoy __________ with my family and friends. One of the best activities in this season is __________, where we can __________.44.How many players are there in a soccer team?A. 7B. 9C. 11D. 13C45.The _______ (马) is often used for riding.46.My favorite sport is ________.47.What do we call a scientist who studies insects?A. EntomologistB. BiologistC. ChemistD. EcologistA48.The _______ of sound can be amplified with a speaker.49.What do we call the study of the universe beyond Earth?A. BiologyB. AstronomyC. GeologyD. Meteorology50.The French Revolution began in the year _______.51.The stars are _____ (bright/dim) in the sky.52.What do you call a book containing words and their meanings?A. EncyclopediaB. DictionaryC. AtlasD. ManualB53. A ______ is a geological feature that can provide insights into the past.54.We are learning about ______ (space) in class.55.What do we call a person who studies the impact of climate on society?A. ClimatologistB. SociologistC. AnthropologistD. Environmental ScientistA56.I want to learn ________ (新语言).57.My sister wants a pet ______ (小狗).58.The first written laws were established by ________ (汉谟拉比).59.What is the color of grass?A. GreenB. YellowC. BlueD. Red60.What is the capital city of Spain?A. BarcelonaB. MadridC. SevilleD. ValenciaB61.The chemical formula for potassium iodide is ______.62.What do we call a young eagle?A. EagletB. ChickC. CalfD. Pup63.How many sides does a hexagon have?A. 4B. 5C. 6D. 7答案:C64.What is the capital of Tuvalu?A. FunafutiB. NukufetauC. NuiD. NanumeaA65.What is the opposite of 'heavy'?A. LightB. StrongC. ThickD. Large66.What do we call something that we use to measure weight?A. RulerB. ScaleC. Tape MeasureD. ThermometerB67.The _______ (猴子) is very intelligent and playful.68.In Canada, the official languages are English and ________ (在加拿大,官方语言是英语和________).69.What do we call the art of folding paper?A. OrigamiB. CollageC. SculptureD. Painting70.The iguana basks in the ______ (阳光) to stay warm.71. A toy car moves when we _______ it.72.The sun is shining and the sky is __________. (晴朗的)73.We are having a ___. (birthday party) for him.74.I love exploring new hobbies. Recently, I tried __________.75.I feel ______ when I play sports with my friends.76. A ________ (植物研究支持) fosters innovation.77.What is the main ingredient in pizza?A. BreadB. RiceC. PastaD. CheeseA Bread78.I built a race track for my toy ____. (玩具名称)79.What do you call a large body of saltwater?A. OceanB. LakeC. RiverD. PondA80.What is the name of the famous English playwright?A. Charles DickensB. J.K. RowlingC. William ShakespeareD. Mark TwainC81.The _______ is very tall and green.82.The ancient Greeks introduced the idea of _______. (民主)83.What do we call the study of fungi?A. MycologyB. BotanyC. ZoologyD. EcologyA84.I love ______ (chocolate) cookies.85.What is the main source of light during the day?A. MoonB. StarsC. SunD. FlashlightC86. A _____ (花瓣) can be different shapes and sizes.87.What is the name of the popular board game where you buy properties?A. ChessB. MonopolyC. ScrabbleD. ClueB88.The boy has a cool ________.89.The ________ loves to explore and discover new things.90.The smallest unit of an element is called an _______.91.Plants can be grown from ______ (扦插) or seeds.92.What do we call the place where you can see many animals in a controlled environment?A. FarmB. ZooC. AquariumD. SanctuaryB Zoo93.I enjoy _____ (聊天) with friends.94.The process of evaporation involves heat and ______.95.What type of animal is a shark?A. MammalB. ReptileC. FishD. AmphibianC96.My dad loves to play ____ (poker) with friends.97. A ladybug is ______ (小) and often red with spots.98.I enjoy helping my ______ (朋友) with their homework. It feels good to support each other.99.What do we call a person who studies the ocean?A. OceanographerB. BiologistC. GeologistD. Meteorologist 100. A __________ is a type of reaction where new substances are formed.。
中考英语科技单选题50题
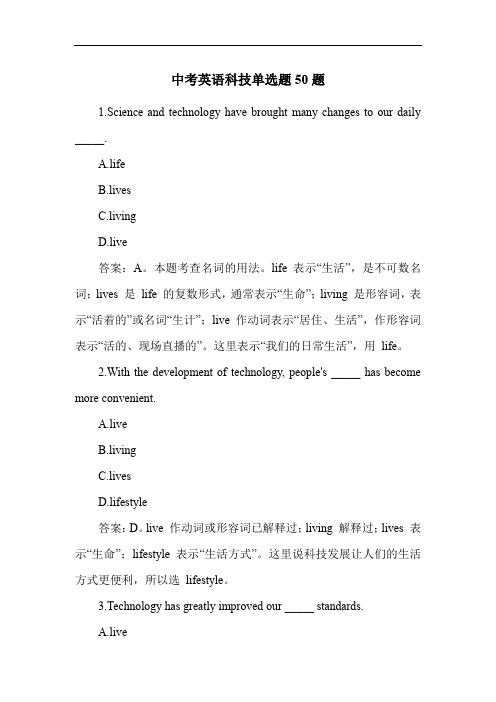
中考英语科技单选题50题1.Science and technology have brought many changes to our daily _____.A.lifeB.livesC.livingD.live答案:A。
本题考查名词的用法。
life 表示“生活”,是不可数名词;lives 是life 的复数形式,通常表示“生命”;living 是形容词,表示“活着的”或名词“生计”;live 作动词表示“居住、生活”,作形容词表示“活的、现场直播的”。
这里表示“我们的日常生活”,用life。
2.With the development of technology, people's _____ has become more convenient.A.liveB.livingC.livesD.lifestyle答案:D。
live 作动词或形容词已解释过;living 解释过;lives 表示“生命”;lifestyle 表示“生活方式”。
这里说科技发展让人们的生活方式更便利,所以选lifestyle。
3.Technology has greatly improved our _____ standards.A.liveB.livingC.lifeD.lives答案:B。
live 和life 解释过;lives 表示“生命”;living 作为名词表示“生活,生计”,living standards 是“生活标准”的固定搭配。
4.New technologies have had a huge impact on people's _____ habits.A.liveB.livingC.lifeD.lives答案:B。
live 和life 解释过;lives 表示“生命”;living 作为名词有“生活方式、习惯”的意思,living habits 是“生活习惯”的固定搭配。
小学上册第11次英语第4单元期末试卷
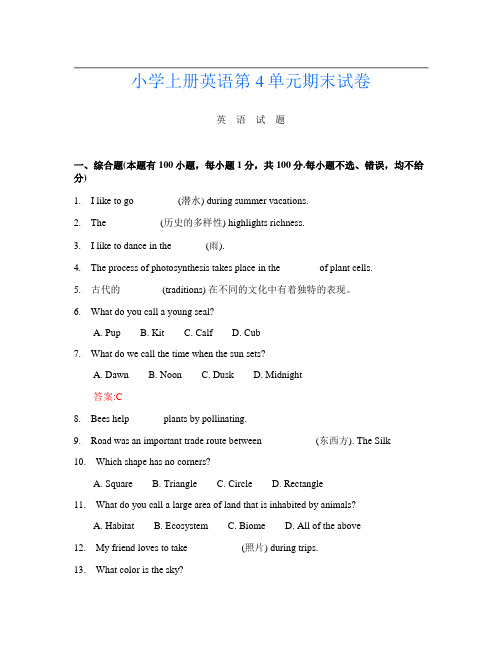
小学上册英语第4单元期末试卷英语试题一、综合题(本题有100小题,每小题1分,共100分.每小题不选、错误,均不给分)1.I like to go ________ (潜水) during summer vacations.2.The __________ (历史的多样性) highlights richness.3.I like to dance in the ______ (雨).4.The process of photosynthesis takes place in the _______ of plant cells.5.古代的________ (traditions) 在不同的文化中有着独特的表现。
6.What do you call a young seal?A. PupB. KitC. CalfD. Cub7.What do we call the time when the sun sets?A. DawnB. NoonC. DuskD. Midnight答案:C8.Bees help ______ plants by pollinating.9.Road was an important trade route between __________ (东西方). The Silk10.Which shape has no corners?A. SquareB. TriangleC. CircleD. Rectangle11.What do you call a large area of land that is inhabited by animals?A. HabitatB. EcosystemC. BiomeD. All of the above12.My friend loves to take __________ (照片) during trips.13.What color is the sky?A. GreenB. BlueC. YellowD. Red答案:B14.__________ are used to measure the temperature of substances.15.I have a ________ (玩具名称) that sings songs.16.I found a __________ on the ground.17.The ancient Romans utilized ________ to enhance their architecture.18.What do you call the sound a dog makes?A. MeowB. BarkC. RoarD. Moo答案:B19.What is the primary gas that plants take in during photosynthesis?A. OxygenB. NitrogenC. Carbon DioxideD. Hydrogen答案:C20.My _____ (舅舅) is a firefighter.21.Which part of the body allows you to see?A. EarsB. EyesC. NoseD. Mouth22.What is the name of the famous American actor known for "Forrest Gump"?A. Tom HanksB. Brad PittC. Johnny DeppD. Leonardo DiCaprio答案:A23.The __________ was a significant document in the history of democracy. (权利法案)24.The ______ teaches us about digital marketing.25.What is the opposite of "young"?A. OldB. NewC. FreshD. Modern答案:A26.We will _______ (go) hiking in the hills.27.The artist, ______ (艺术家), creates beautiful paintings.28.The _____ (植物世界) is full of wonders and discoveries.29.Some frogs can change ______.30.The country famous for its spices is ________ (印度).31.What is the main ingredient in ice cream?A. WaterB. MilkC. SugarD. Flour32.I see a __ in the garden. (bug)33.What do you wear on your feet?A. HatB. GlovesC. ShoesD. Scarf34.What is the name of the famous mountain in South America?A. KilimanjaroB. AndesC. RockiesD. Alps答案:B Andes35.I love to watch ________ (体育比赛).36.I keep a journal to write down my ______ (梦想) and goals for the future. It motivates me to work hard.37.I see a _____ (水桶) by the door.38.The _____ (火烈鸟) feeds on small shrimp and algae.39.What do you call a baby dog?A. KittenB. PuppyC. CubD. Calf答案:B40. A chemical reaction can absorb or release ______.41.What do we call a person who studies the structure and function of proteins?A. BiochemistB. Molecular BiologistC. GeneticistD. Microbiologist答案:A42.The __________ (古代王国) often waged wars for territory.43.The park is _______ (适合家庭) activities.44.What does a thermometer measure?A. TimeB. TemperatureC. SpeedD. Distance45.We played ________ in the playground.46.She wears a pretty ___. (dress)47.What do we call the time of year when leaves fall from trees?A. SpringB. SummerC. AutumnD. Winter答案:C48.Metals are usually _____ at room temperature.49.What do we call the solid form of water?A. SteamB. IceC. LiquidD. Vapor答案:B50.The tree has green ________.51.What is the capital of the Central African Republic?A. BanguiB. LibrevilleC. YaoundéD. Kinshasa答案:A52.The __________ is the part of a flower that produces seeds.53.She has a big ________.54.The first successful composite transplant was performed in ________.55.Many _______ are used in traditional medicine.56.The _____ (field) is green.57.The _______ (Great Wall) was built over several dynasties in China.58.What is the capital of the United Kingdom?A. LondonB. EdinburghC. CardiffD. Belfast答案:A59.What is the term for a baby goat?A. LambB. KidC. CalfD. Foal答案:B60.The _____ (basket) is full of fruits.61.What do we call the process of a liquid turning into a gas?A. FreezingB. MeltingC. EvaporationD. Condensation答案:C62.The _______ (小青蛙) croaks by the pond.63.Which is a large body of water?A. LakeB. PondC. RiverD. Ocean答案:D64.Which instrument has keys and makes music?A. GuitarB. ViolinC. PianoD. Drum答案:C65.What do you call the process of water turning into vapor?A. FreezingB. MeltingC. EvaporationD. Condensation66.Matter is anything that has ______.67.What is the function of a thermometer?A. To measure speedB. To measure temperatureC. To measure weightD. To measure distance答案:B68. A ferret is a curious little ________________ (动物).69.What do you call a house for birds?A. NestB. CoopC. CageD. Aviary70.The __________ are beautiful in the spring garden. (花儿)71. A pendulum's swing is an example of ______ motion.72.Which animal is known for its intelligence and problem-solving skills?A. DogB. CatC. DolphinD. Bird73.What do you call a baby cow?A. CalfB. KidC. PuppyD. Chick答案:A74.The first person to climb Mount Everest was _______ Hillary.75.I enjoy reading ________ (故事书) before bedtime.76.What is the main ingredient in chocolate?A. SugarB. CocoaC. MilkD. Vanilla77.My dad is a __________ (公共关系经理).78.The boat is ___ (sailing) on the water.79.My favorite type of ________ (音乐) is rock.80.The _____ (小型植物) can enhance indoor spaces.81.My family often has fun by calling each other funny names like . (我的家人经常通过称呼对方有趣的名字,比如,来增添乐趣。
- 1、下载文档前请自行甄别文档内容的完整性,平台不提供额外的编辑、内容补充、找答案等附加服务。
- 2、"仅部分预览"的文档,不可在线预览部分如存在完整性等问题,可反馈申请退款(可完整预览的文档不适用该条件!)。
- 3、如文档侵犯您的权益,请联系客服反馈,我们会尽快为您处理(人工客服工作时间:9:00-18:30)。
Using People and WordNet to MeasureSemantic RelatednessBeata Beigman KlebanovApril16,2006AbstractThis technical report describes in some detail(1)the creation ofa dataset for testing the degree of relatedness between concepts outof the data from Beigman Klebanov and Shamir’s lexical cohesionexperiment[3,5,6],and(2)a new measure of semantic relatednessbased on WordNet.We welcome comments on this manuscript;how-ever,please refrain from citing it,but rather the concise publishedversion[4].This report is intended to accompany the published pa-per with more thorough technical detail to enable replication of themethod.1IntroductionEstimating the degree of semantic relatedness between words in a text is deemed important in numerous applications:word-sense disambiguation[1], story segmentation[20],error correction[12],summarization[2,9].Furthermore,Budanitsky and Hirst(2006)noted that various applica-tions tend to pick the same measures of relatedness,which suggests a certain commonality in what is required from such a measure by the different ap-plications.It thus seems worthwhile to develop such measures intrinsically, before putting them to application-based utility tests.The most popular,by-now-standard testbed is Rubenstein and Goode-nough’s(1965)list of65noun pairs,ranked by similarity of meaning.A 30-pair subset(henceforth,MC)passed a number of replications[15,19], and is thus highly reliable.1Rubenstein and Goodenough view similarity of meaning as degree of sy-nonymy.Researchers have long recognized,however,that synonymy is only one kind of semantic affinity between words in a text[10],and expressed a wish for a dataset for testing a more general notion of semantic relatedness.1 This paper proposes and explores a new relatedness dataset.In sections2-3,we briefly introduce the experiment by Beigman Klebanov and Shamir (henceforth,BS),and show how to use it to induce relatedness scores,in-cluding estimation of their subjects’homogeneity with respect to the scores.In section4,we propose a new WordNet-based measure of relatedness, and use it to explore the new dataset.We show that it usually does better than competing WordNet-based measures(sections5,6).We discuss future directions in section7.2DataAiming at reader-based exploration of lexical cohesion in texts,Beigman Kle-banov and Shamir conducted an experiment with22students,each reading 10texts:3news stories,4journalistic and3fiction pieces[6].People were instructed to read the textfirst,and then go over a separately attached list of words in order of their appearance in the text,and ask themselves,for every newly mentioned concept,“which previously mentioned concepts help the easy accommodation of the current concept into the evolving story,if indeed it is easily accommodated,based on the common knowledge as perceived by the annotator”[5];this preceding helper concept is called an anchor.People were asked to mark all anchoring relations they couldfind.The rendering of relatedness between two concepts is not tied to any specific lexical relation,but rather to common-sense knowledge,which,ac-cording to[11](cited in the guidelines),has to do with“knowledge of kinds, of associations,of typical situations,and even typical utterances”.The phe-nomenon is thus clearly construed as much broader than degree-of-synonymy.Analyzing the experimental data,Beigman Klebanov and Shamir[3,6] exclude2outliers,and provide reliability estimation using statistical analysis. They show that items that are given an anchor by>12people are reliably anchored in the text;items left bare by all20people are reliably un-anchored.1“...there is at present no large dataset of human judgments of semantic related-ness”[12];“To our knowledge,no datasets are available for validating the results of se-mantic relatedness metric”[8].2Strong anchors for the reliably anchored items are identified and validated experimentally.Their analysis provides high-validity data for classification; however,much of the data regarding intermediate degrees of relatedness is left out.3Relatedness ScoresOur idea is to induce scores for pairs of anchored items with their anchors (henceforth,AApairs)using the cumulative annotations by20people.Thus, AApairs written by all20people would score20,and those written by just one person score1.The scores would correspond to the perceived relatedness of the pair of concepts in the given text.3.1Multi-Word ItemsIn the core data for classification[3,6],no distinctions were retained between pairs marked by19or13people,as long as the number of people passed the established threshold.Now,however,we are interested in the relative relatedness,so it is important to handle cases where the BS data might under-rate a given pair.One such case are multi-word(henceforth,complex)anchored items;the instructions asked for judgements on the given wordlist,but some subjects added complex items they perceived as a unit,and gave them anchors.Those are most often proper names,like yasser arafat/palestinian,or phrasal verbs,like passed away/died.Such items received an unsystematic treat-ment,some people inserting complex anchored items,others anchoring one part of the item in the other(arafat/yasser,away/passed),and yet others skipping the issue altogether.To exclude such cases,we remove all AA-pairs with a complex anchored item;we make a note of all complex an-chored items produced by at least2people,and remove AApairs that have parts of those complex constructs as anchored items(like yasser/palestinian, passed/died),fearing that such annotations pertain to the complex item rather than to its parts(a particularly telling example is bank/ramallah,in a text mentioning the West Bank).Another issue concerns complex anchors to single-word anchored items. The instructions ask for the minimal word sequence that is sufficient for3anchoring to occur.2However,the data shows that such minimality decision is not always straightforward:as anchors for aged,people produced old, 69years,years,69years old.The number of“votes”each version received is small,possibly undermining their actual degree of relatedness.Thus,for a given anchored item,we exclude AApairs with anchors that contain words in common with other anchors of the same anchored item;in this case,all the mentioned anchors for aged will be excluded.Of the remaining data,we retain only pairs where the anchored item and the anchor belong to open-class parts of speech;this is based on the obser-vation that functional categories contribute little to the lexical texture[10].The Size column of table1shows the sizes of the datasets for all BS texts, after the aforementioned exclusions.3.2Group Homogeneity EstimationThe induced scores correspond to the cumulative judgements of a certain group of people.How well do they represent the people’s ideas?One way to measure group homogeneity is leave-one-out estimation,as performed by Resnik(1995)for his experiment on Miller&Charles noun pairs,attaining the high average correlation of r=0.88.In the current case,however,every specific person made a binary decision,whereas a group is represented by scores1to20;such difference in granularity is problematic for correlation or rank order analysis.Another way to measure group homogeneity is to split it into subgroups and compare ranks emerging from the different subgroups.We know from Beigman Klebanov and Shamir[3,6]that it is not the case that the20-subject group clusters into subgroups that systematically produced different patterns of answers.This leads us to expect relative lack of sensitivity to the exact splits into subgroups.To validate this reasoning,we performed100random choices of two9-subject3groups,calculated the ranks induced on the AApairs by the two 2“...prefer University over The Hebrew University of Jerusalem as an anchor for a subsequent lecturer”p.5in Beigman Klebanov and Shamir(2005).3To use correlation statistics faithfully,we must make sure that there is no dependence between the judgments of the two groups.In a10/10split,there is a certain amount of dependence:if one group gives a rank of0to an AApair(none of the group members marked it),it is not possible for the second group to mark this pair0,since a pair that nobody marked would not have been included in the dataset.Choosing9/9split allows4groups,and computed Pearson correlation between the two lists.4 Thus,for every BS text,we have a distribution of100coefficients,which is approximately normal.Estimations ofµandσof these distributions are µ=.69toµ=.82,σ=.02toσ=.03for the different BS texts.Theµ(r) column in table1lists thefigures for each of the BS texts.To summarize:although the homogeneity is lower than for MC data,we observe good average inter-group correlations with little deviation across the 100splits.We now turn to discussion of a relatedness measure,which we will evaluate using the data.4GIC:WordNet-based MeasureMeasures using WordNet taxonomy are state-of-the-art in capturing semantic similarity,attaining r=.85–.89correlations with the MC dataset[13,7]. However,they fall short of measuring relatedness,as,operating within a single-POS taxonomy,they cannot meaningfully compare kill to death.This is a major limitation with respect to BS data,where only about40%of pairs are nominal,and less than10%are verbal.We develop a WordNet-based measure that would allow cross-POS comparisons,using WordNet glosses in addition to the taxonomy.One family of WordNet measures are methods based on estimation of in-formation content(henceforth,IC)of concepts,as proposed in[19].Resnik’s key idea in corpus-based information content induction using a taxonomy is to count every appearance of a concept as mentions of all its hypernyms as well.This way,artifact#n#1,although rarely mentioned explicitly,receives high frequency and low IC value.We will count a concept’s mention towards all its hypernyms AND all words5that appear in its own and its hypernyms’glosses.Analogously to artifact,we expect properties mentioned in glosses of more general concepts to be less informative,as those pertain to more things (ex.,visible,a property of anything that is-a physical object).The details both comparable scale(0-9in each),and full freedom of choice on any AApair.4We chose Pearson correlation over Spearman’s rank order correlation since the datasets contain many tied ranks,and rank order correlation tends to show higher-than-real values in such cases.5We induce IC values on(POS-tagged base form)words rather than senses.Ongoing gloss sense-tagging projects like eXtended WordNet (/links.html)would allow sense-based calculation in the future.5of the algorithm for information content induction from taxonomy and gloss information(IC GT)are given in appendix A.To estimate the semantic affinity between two senses A and B,we average the IC GT values of the3words with the highest IC GT in the overlap of A’s and B’s expanded glosses(the expansion follows the algorithm in appendix A).6 If A∗(the word of which A is a sense)appears in the expanded gloss of B,we the maximum between the IC GT(A∗)and the value returned by the 3-smoothed calculation.To compare two words,we take the maximum value returned by pairwise comparisons of their WordNet senses.7The performance of this measure is shown under GIC in table1.GIC manages robust but weak correlations,never reaching the r=.40threshold. 5Previous Work:RelatednessWe compare GIC to another WordNet based measure that can handle cross-POS comparisons,proposed by Banerjee and Pedersen(2003).To compare word senses A and B,the algorithm compares not only their glosses,but also glosses of items standing in various WordNet relations with A and B.For example,it compares the gloss of A’s meronym to that of B’s hyponym.We use the default configuration of the measure in WordNet::Similarity-0.12package[16],and,with a single exception,the measure performed below GIC;see column BP in table1.Experimenting with this measure,we tried applying to it the following transformation.Suppose BP is the score returned by the original BP algo-rithm on a certain pair;then:EBP=e BP−e−1×BP e BP+e−1×BPThe transformation was proposed in[14],and applied to linear mea-sures used therein.If,for the original measure,0means no similarity,and scores close and above1mean much similarity,the transformation scales the 6The number3is empirically-based;the idea is to counter-balance(a)the effect of an accidental match of a single word which is relatively rarely used in glosses;(b)the multitude of low-IC items in many of the overlaps that tend to downplay the impact of the few higher-IC members of the overlap.7To speed the processing up,we usefirst5WordNet senses of each item for results reported here.6Dataset Sizeµ(r)Gic BP EBPBS-11007.71.29.19.19BS-2776.79.37.16.20BS-31015.73.22.09.11BS-4512.73.34.39.37BS-51020.75.25.11.17BS-6536.69.24.19.22BS-7917.72.22.10.11BS-8529.76.24.12.11BS-9509.76.31.16.20BS-10417.82.36.19.20Av BS724.75.28.17.19Table1:Dataset sizes,homogeneity and correlations of GIC,BP with human ratings.r>0.16is significant at p<.05;r>.23is significant at p<.01. scores such that the differences between higher scores are diminished.So, BP=0.20and BP=0.30turn into EBP=0.20and EBP=0.29,respec-tively,nearly preserving the difference.If the originals are.85and.95,the transformed ones are.69and.74,with only.05difference.Finally,had the originals been3and10,the transformed values are0.995054753686731and 0.999999995877693,hardly different at all.If used for a similarity measure, this means that a pair of very similar things should score closer to another pair of very similar things than the original measure would predict.The last column in table1shows the correlations attained by the trans-formed version EBP.We note that it usually improves slightly on the original BP measure;still,it is doing worse than Gic in all but one cases.6Previous Work:SimilarityAs mentioned before,taxonomy-based similarity measures cannot fully han-dle BS data.Table2uses nominal-only subsets of BS data and the MC nominal similarity dataset to show that(a)state-of-the-art WordNet-based similarity measure JC8[13,7]does very poorly on the relatedness data,sug-gesting that nominal similarity and relatedness are rather different things;8See appendix B.7(b)GIC does better on average,and is more robust;(c)GIC yields on MC to gain performance on BS,whereas BP is no more inclined towards relatedness than JC.Dataset Size Gic BP EBP JCMC30.78.80.83.86BS-1328.38.18.19.21BS-2249.53.18.22.37BS-3259.21.04.05.01BS-4125.28.38.33.33BS-5505.12.07.11.16BS-6286.25.16.20.22BS-7309.23.10.09.04BS-8257.32.10.08.00BS-9178.24.17.18.27BS-10186.41.25.24.25Av BS268.30.16.17.19Table2:MC and Nominal-only subsets of BS:correlations of various mea-sures with the human ratings.Table3gives an example of relatedness vs.similarity distinction.Whereas, taxonomically speaking,son is more similar to man,as reflected in JC scores, people marked family and mother as much stronger anchors for son in BS-2; GIC follows suit.AApair Human Gic JCson–man20.35522.3son–family130.37516.9son–mother160.37020.1Table3:Relatendess vs.Similarity Example87Conclusion and Future WorkWe proposed a dataset of relatedness judgements that differs from the exist-ing ones9as follows:•size:about7000items,as opposed to up to350in existing datasets;•cross-POS data,as opposed to purely nominal or verbal;•a broad approach to semantic relatedness,not focussing on any particular relation,but grounding it in the reader’s(idea of)common knowledge;this as opposed to synonymy-based similarity prevalent in existing databases.We explored the data with WordNet-based measures,showing that(1) the data is different in character from a standard similarity dataset,and very challenging for state-of-the-art methods;(2)the proposed novel WordNet-based measure of relatedness usually outperforms its competitor,as well as a state-of-the-art similarity measure when the latter applies.In future work,we plan to explore distributional methods for modeling relatedness,as well as the use of text-based information to improve corre-lations with the human data,as judgments are situated in specific textual contexts.References[1]Satanjeev Banerjee and Ted Pedersen.Extended gloss overlaps as ameasure of semantic relatedness.In Proceedings of IJCAI,2003.[2]Regina Barzilay and Michael ing lexical chains for textsummarization.In Proceedings of ACL Intelligent Scalable Text Sum-marization Workshop,1997.[3]Beata Beigman ing readers to identify lexical cohesivestructures in texts.In Proceedings of ACL Student Research Workshop, 2005.9MC is not the only available dataset;we will address other datasets in a subsequent paper.9[4]Beata Beigman Klebanov.Measuring semantic relatedness using peopleand WordNet.In Proceedings of NAACL Short Papers,2006.[5]Beata Beigman Klebanov and Eli Shamir.Guidelines for annotation ofconcept mention patterns.Technical Report2005-8,Leibniz Center for Research in Computer Science,The Hebrew University of Jerusalem, Israel,2005.[6]Beata Beigman Klebanov and Eli Shamir.Reader-based exploration oflexical nguage Resources and Evaluation,to appear,2006.Springer,Netherlands.[7]Alexander Budanitsky and Graeme Hirst.Evaluating WordNet-basedmeasures of semantic putational Linguistics,32(1):13–47, 2006.[8]Iryna ing the structure of a conceptual network in com-puting semantic relatedness.In Proceedings of IJCNLP,2005.[9]Iryna Gurevych and Michael Strube.Semantic similarity applied tospoken dialogue summarization.In Proceedings of COLING,2004. [10]M.A.K.Halliday and Ruqaiya Hasan.Cohesion in English.LongmanGroup Ltd.,1976.[11]Graeme Hirst.Context as a spurious concept.In Proceedings of CI-CLING,2000.[12]Graeme Hirst and Alexander Budanitsky.Correcting real-word spellingerrors by restoring lexical cohesion.Natural Language Engineering, 11(1):87–111,2005.[13]Jay Jiang and David Conrath.Semantic similarity based on corpusstatistics and lexical taxonomy.In Proceedings on International Confer-ence on Research in Computational Linguistics,1997.[14]Yuhua Li,Zygaur Bandar,and David McLean.An approach to mea-suring semantic similarity between words using multiple information sources.IEEE Transactions on Knowledge and Data Engineering, 15(4):871–882,2003.10[15]George Miller and Walter Charles.Contextual correlates of semanticnguage and Cognitive Processes,6(1):1–28,1991.[16]Ted Pedersen,Siddharth Patwardhan,and Jason Michelizzi.WordNet::Similarity-measuring the relatedness of concepts.In Proceedings of NAACL,2004.[17]Adwait Ratnaparkhi.A maximum entropy model for part-of-speechtagging.In Proceedings of EMNLP,1996.[18]Jason Rennie.WordNet::QueryData:a Perl module for accessingthe WordNet database./~jrennie/WordNet, 2000.[19]Philip ing information content to evaluate semantic similarityin a taxonomy.In Proceedings of IJCAI,1995.[20]Nicola Stokes,Joe Carthy,and Alan F.Smeaton.SeLeCT:A lexicalcohesion based news story segmentation system.Journal of AI Com-munications,17(1):3–12,2004.A Gloss&Taxonomy IC(IC GT)We now detail the method for IC induction from taxonomy and gloss infor-mation.First,we part-of-speech-tag[17]and bring to the base form all words in WordNet glosses10;we refer to such items when we say“words”through-out this section.Then,for every word-sense W in WordNet database for a given part of speech:1.Collect all content words from the gloss of W,excluding examples,including W∗-the part-of-speech-tagged word of which W is a sense.2.If W is part of a taxonomy,expand its gloss,without repetitions,withwords appearing in the glosses of all its super-ordinate concepts,up to the top of the hierarchy.Thus,the expanded gloss for airplane#n#1 would contain words from the glosses of the relevant senses of aircraft ,vehicle,transport,etc.The idea behind this step is that mention of 10We use WordNet::QueryData[18]package version1.38to access WordNet-2.0.11properties in a taxonomic database is often organized in an inheritance hierarchy-a property is mentioned at the most general level it applies.Thus,the rather salientflying property of airplane pertains already to its super-super-ordinate,aircraft.3.Add sense count of W to all words in its expanded gloss.11Each part-of-speech database induces its own counts on each word that appeared in the gloss of at least one of its bining raw counts would effectively correspond to the data in the nominal database,by far the largest.While acceptable in general,it downplays the fact that,adverbs,for example,often describe the manner in which some action is performed.Thus, the concept of manner would be relatively un-informative as far as adverbs are concerned,whereas it would seem more informative from the point of view of nominal definitions.Hence,when merging the information from the different parts of speech,we scale the aggregated counts,such that they correspond to the proportion of the given word in the part-of-speech database where it was the least informative.The standard log-frequency calculation transforms these counts into taxonomy-and-gloss based information content (IC GT)values.B JC measure of similarityIn the formula below,IC is taxonomy-only based information content,as articulated in[19],LS is the lowest common subsumer of the two concepts in the WordNet hierarchy,and Max is the maximum distance between any two concepts in the given hierarchy.For two concepts c1and c2:JC(c1,c2)=Max−(IC(c1)+IC(c2)−2×IC(LS(c1,c2)) To make JC scores comparable to Gic’s[0,1]range,the score can be divided by Max.Normalization has no effect on correlations.We use[16]implementation of JC measure,with the following alteration: instead of turning distance into similarity by1/Distance,as done in[16],we follow Jiang and Conrath’s(1997)proposal and subtract it from Max–the longest possible distance between two concepts in a hierarchy.The longest 11We do add-1smoothing on WordNet sense counts.12possible distance is between two concepts with highest possible IC(a single appearance count)that share only the most general concept(topmost level of the nominal hierarchy);this value is about26for WordNet-2.0and the add-1smoothed SemCor database.13。