Spectral Clustering for Robust Motion Segmentation
一种新型光谱表面等离子体共振二维探测方法在DNA微阵列中的应用
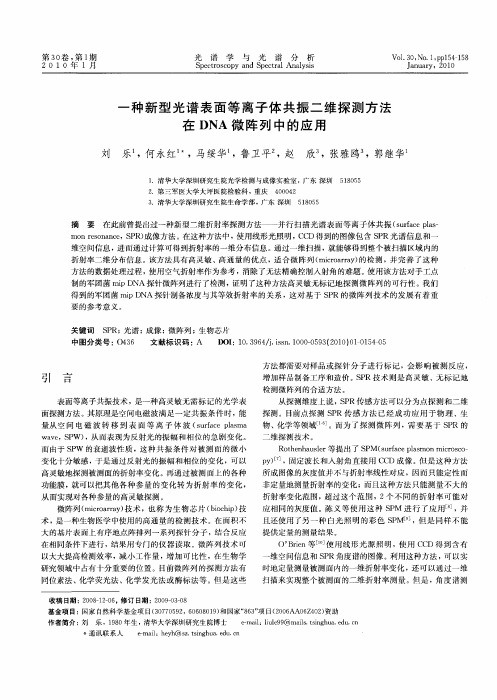
方法 的数 据处 理过程 , 使用空气折射率作 为参考 , 消除 了无法精确控制入射 角的难题 。 使用该方法对手 工点
制 的军团菌 mi NA探针微阵列进行 了检测 , 明了这种 方法高灵敏无标记地探 测微阵列 的可行性 。我们 pD 证
高灵 敏地探测被测 面的折射率变化 。 再通过被 测面上 的各种
所成 图像 的灰度值并不 与折射 率线性对应 ,因而 只能 定性而 非定量地测量折射率 的变 化 ; 而且这种方 法只能测量 不大 的
折射率变化 范围 , 超过 这个范 围 ,2 不 同的折 射率 可能对 个
功能膜 ,就可以把其他 各种参 量 的变化 转为折 射率 的变 化 ,
得到 的军 团菌 mi D p NA探针制备浓度与其等效折射 率的关 系 ,这对基 于 S R的微阵列 技术 的发展 有着重 P
要 的参 考 意 义。
关键词
S R; P 光谱 ; 成像 ; 阵列 ; 微 生物芯片
文 献 标 识 码 :A D :1 .9 4 ji n 10 —5 3 2 1 ) 10 5 5 OI 0 3 6 /.s . 0 00 9 (0 0 O— 140 s
应相 同的灰度值 。陈义 等使 用这 种 S M 进 行 了应用 l ,并 P 8 ]
且还使用 了另一种 白光照 明 的彩色 S I ,但是 同样 不能 PV l  ̄
提 供 定 量 的测 量 结 果 。
o’ r n等l Bi e 1 用线形 光源 照 明 , 用 C D得到 含有 叩使 使 C
一
种 新 型 光谱 表 面等 离 子体 共振 二 维探 测 方 法 在 DNA微 阵 列 中 的应 用
基于改进哈里斯鹰优化算法的光谱特征波段选择模型研究

基于改进哈里斯鹰优化算法的光谱特征波段选择模型研究鲍浩;张艳【期刊名称】《光谱学与光谱分析》【年(卷),期】2024(44)1【摘要】特征波段选择是近红外光谱分析的关键步骤之一,有效的特征波段选择能提高建模效率与模型性能。
传统的特征波段选择算法存在运行时间长、选择特征冗余的缺陷,在实际工程应用中难以达到期望的效果。
哈里斯鹰优化(HHO)算法具有原理简单、参数少的优点,但同时也存在收敛精度低且易陷入局部最优的不足。
在HHO算法的基础上提出了一种基于改进哈里斯鹰优化(IHHO)算法的近红外光谱特征波段选择模型。
针对HHO算法只能用于求解连续空间的优化问题,采用离散化策略对HHO算法进行修正,使其能求解离散形式的特征波段选择问题;考虑到HHO 算法初始种群的质量差,使用混沌映射、反向学习提高初始种群的质量,以增强算法的全局探索能力;由于HHO算法在局部搜索时的收敛精度低,提出了新的猎物能量衰减模型与跳跃策略,以进一步增强算法在局部搜索时的寻优能力;为避免算法在寻优过程中落入局部最优,借鉴了遗传算法的变异方式对HHO算法进行扰动。
使用竞争性自适应重加权采样法(CARS)、连续投影算法(SPA)、粒子群优化(PSO)算法、遗传算法(GA)、 HHO算法与IHHO算法进行比较,并以4个定性分析近红外光谱数据集与2个定量分析近红外光谱数据集分别建立了支持向量机(SVM)识别模型和偏最小二乘回归(PLSR)模型。
在定性分析实验中,IHHO算法得到的平均准确率相对于全波段时分别提高了0.83%、 9.55%、 17.65%以及0%,平均特征波段数仅占全波段的9.97%、 2.59%、 1.36%以及0.59%。
在定量分析实验中,IHHO算法得到的平均决定系数分别较全波段提高了10.57%、 1.47%、 4.41%、 3.66%以及3.06%,平均均方根误差分别较全波段较低了0.162、 1.266 3、 1.868、 1.869 4以及0.408 4,平均特征波段数仅占全波段的9.24%、10.53%、6.54%、6.91%以及7.14%。
无线电发射设备频谱异常检测与干扰定位研究
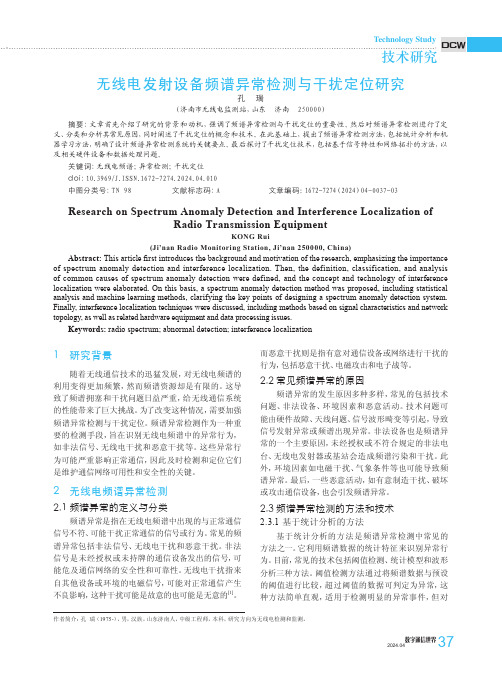
DCWTechnology Study技术研究37数字通信世界2024.041 研究背景随着无线通信技术的迅猛发展,对无线电频谱的利用变得更加频繁,然而频谱资源却是有限的。
这导致了频谱拥塞和干扰问题日益严重,给无线通信系统的性能带来了巨大挑战。
为了改变这种情况,需要加强频谱异常检测与干扰定位。
频谱异常检测作为一种重要的检测手段,旨在识别无线电频谱中的异常行为,如非法信号、无线电干扰和恶意干扰等。
这些异常行为可能严重影响正常通信,因此及时检测和定位它们是维护通信网络可用性和安全性的关键。
2 无线电频谱异常检测2.1 频谱异常的定义与分类频谱异常是指在无线电频谱中出现的与正常通信信号不符、可能干扰正常通信的信号或行为。
常见的频谱异常包括非法信号、无线电干扰和恶意干扰。
非法信号是未经授权或未持牌的通信设备发出的信号,可能危及通信网络的安全性和可靠性。
无线电干扰指来自其他设备或环境的电磁信号,可能对正常通信产生不良影响,这种干扰可能是故意的也可能是无意的[1]。
而恶意干扰则是指有意对通信设备或网络进行干扰的行为,包括恶意干扰、电磁攻击和电子战等。
2.2 常见频谱异常的原因频谱异常的发生原因多种多样,常见的包括技术问题、非法设备、环境因素和恶意活动。
技术问题可能由硬件故障、天线问题、信号波形畸变等引起,导致信号发射异常或频谱出现异常。
非法设备也是频谱异常的一个主要原因,未经授权或不符合规定的非法电台、无线电发射器或基站会造成频谱污染和干扰。
此外,环境因素如电磁干扰、气象条件等也可能导致频谱异常。
最后,一些恶意活动,如有意制造干扰、破坏或攻击通信设备,也会引发频谱异常。
2.3 频谱异常检测的方法和技术2.3.1 基于统计分析的方法基于统计分析的方法是频谱异常检测中常见的方法之一。
它利用频谱数据的统计特征来识别异常行为。
目前,常见的技术包括阈值检测、统计模型和波形分析三种方法。
阈值检测方法通过将频谱数据与预设的阈值进行比较,超过阈值的数据可判定为异常,这种方法简单直观,适用于检测明显的异常事件,但对无线电发射设备频谱异常检测与干扰定位研究孔 瑞(济南市无线电监测站,山东 济南 250000)摘要:文章首先介绍了研究的背景和动机,强调了频谱异常检测与干扰定位的重要性。
用于生成射线跟踪图像的可视化的技术[发明专利]
![用于生成射线跟踪图像的可视化的技术[发明专利]](https://img.taocdn.com/s3/m/6327a0b977232f60dccca133.png)
专利名称:用于生成射线跟踪图像的可视化的技术
专利类型:发明专利
发明人:A·N·金罗斯,S·L·哈格里维斯,A·帕特尔,T·L·戴维松申请号:CN201980016496.2
申请日:20190221
公开号:CN111801713A
公开日:
20201020
专利内容由知识产权出版社提供
摘要:本文中描述的示例总体上涉及生成图像的可视化。
从图形处理单元(GPU)或图形驱动器拦截指定用于使用射线跟踪来生成图像的射线跟踪指令的专有结构。
可以基于辅助信息来将专有结构转换为用于生成图像的可视化的可视化结构。
可以从可视化结构来生成图像的可视化。
申请人:微软技术许可有限责任公司
地址:美国华盛顿州
国籍:US
代理机构:北京市金杜律师事务所
代理人:彭梦晔
更多信息请下载全文后查看。
基于非局部平均的多光谱遥感图像除噪声
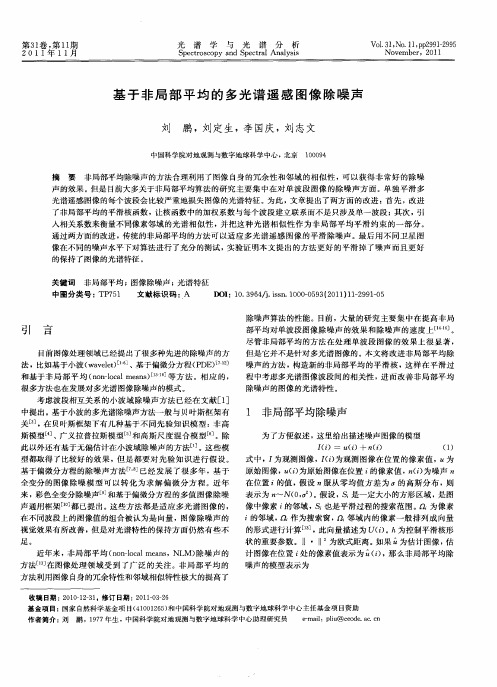
出的 MSNL 方 法 的光 谱 角度 误 差 ( AM) 直都 是 最小 - M S 一
的, 尤其是 噪声 比较大情况下更加 明显一 些 。在噪声 比较小
的 情 况 下 , 文 提 出 方 法 MSNL 的 相 对 整 体 维 数 综 合 误 本 - M 差( R E GAS 总 体 上 也 是 比较 小 的 ,只 有 少 数 情 况 误 差 相 对 )
型都取得了 比较好的效 果 ,但是都 要对 先验 知识进 行假 设 。
基 于偏微 分方 程的除噪声 方法 _ ] 7 已经 发展 了很多 年 ,基于 全变分的图像 除 噪模 型 可 以转化 为 求解 偏微 分 方程 。近年 来 ,彩色全变分除噪声[ 和基于偏微分方程 的多值 图像 除噪 9 ] 声通用框架 『 都 已提 出。这些方法 都是适应 多光谱 图像 的 , 】 叩 在不 同波段上 的图像值 的组合被认 为是 向量 ,图像除 噪声的
通过 两方 面的改进 , 传统的非局部平均 的方法可 以适应 多光谱遥 感 图像 的平 滑除噪声 。最后 用不 同卫星 图 像在不 同的噪声水 平下 对算法进行 了充分的测 试 , 实验 证明本文 提出的方法 更好 的平滑掉 了噪声 而且更好
的保 持 了 图像 的光 谱 特 征 。
关键词 非局部平均 ; 图像除噪声 ; 光谱特 征
进, 传统 的非局部平 均的方法更加适应多 光谱遥感 像除 噪
4 结 论
本文改进 了非局部平 均的平滑核函数 ,让核 函数 中 的加 权 系数与每个波段建立联 系而不是 只涉及单一 波段 ,引入相
声的情况 。 文用不同卫星的图像在 同的噪声水 平下对算 本
法进 行 了 充 分 的验 证 ,实 验 证 明 本 文 提 出 的 方 法 比 较 好 的平 滑 掉 了噪 声 ,不 但 获 得 更 好 的 视 觉 效 果 而 且 更 好 的 保 持 _ r图
Spectral
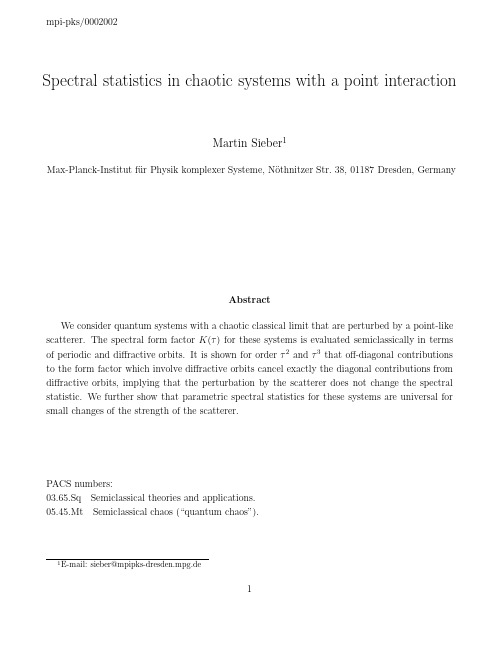
Introduction
Semiclassical theories for spectral statistics have been developed [1, 2, 3] to find an explanation for the observed universality in energy spectra of quantum systems with a chaotic classical limit, the agreement of correlations in energy spectra with those between eigenvalues of random matrices [4]. They are based on semiclassical trace formulas that approximate the density of states in terms of classical trajectories [5]. It has been shown by these theories that in the asymptotic limit of long-range correlations two-point correlation functions do coincide with those of random matrix theory [2, 3]. These results are based on mean properties of periodic orbits [1]. To go beyond the leading asymptotic term requires information about correlations between periodic orbits which are presently not available [6]. One of the expectations, on basis of the random matrix hypothesis [7], is that a perturbation of a chaotic system should not change the statistical distribution of the energy levels of the quantum system, if it does not change the chaotic nature of the classical motion. In the present article we investigate, on the level of the semiclassical approximation, whether the perturbation by a pointlike scatterer has this property. One argument in favour of this invariance is that the semiclassical approximation for the density of states is not changed in leading order of ~ for this perturbation. The influence of the scatterer is described semiclassically by a certain class of trajectories, so-called diffractive orbits that start from the scatterer and return to it. They contribute to the density of states in higher order of ~ than the leading order contribution from periodic orbits. The present article is motivated by the observation in [8] that a scatterer could nevertheless have an influence on spectral statistics. When spectral correlation functions are calculated by using mean properties of diffractive orbits, the so-called diagonal approximation, they show modifications which, in general, do not vanish in the semiclassical limit (~ → 0). In order that this does not lead to deviations from random matrix statistics, these terms have to be cancelled by off-diagonal terms which contain information about correlations between different trajectories. As remarked above, the calculation of correlations between trajectories is an unsolved problem in general systems. For the diffractive orbits that describe the influence of a scatterer, however, off-diagonal terms can be calculated explicitly. This is done in the following sections. The results show that diagonal and off-diagonal terms indeed cancel each other. Furthermore, the results can be used to investigate parametric spectral correlations, i. e. correlations between spectra of the system for different parameter values, where the parameter is the strength of the scatterer. It is shown that the parametric spectral correlations are universal for small changes of the parameter.
A Tutorial on Spectral Clustering
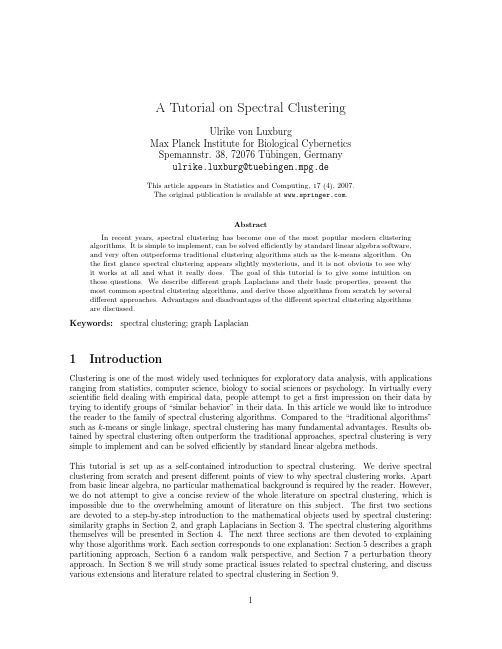
Ulrike von Luxburg Max Planck Institute for Biological Cybernetics Spemannstr. 38, 72076 T¨ ubingen, Germany ulrike.luxburg@tuebingen.mpg.de
2
Similarity graphs
Given a set of data points x1 , . . . xn and some notion of similarity sij ≥ 0 between all pairs of data points xi and xj , the intuitive goal of clustering is to divide the data points into several groups such that points in the same group are similar and points in different groups are dissimilar to each other. If we do not have more information than similarities between data points, a nice way of representing the data is in form of the similarity graph G = (V, E ). Each vertex vi in this graph represents a data point xi . Two vertices are connected if the similarity sij between the corresponding data points xi and xj is positive or larger than a certain threshold, and the edge is weighted by sij . The problem of clustering can now be reformulated using the similarity graph: we want to find a partition of the graph such that the edges between different groups have very low weights (which means that points in different clusters are dissimilar from each other) and the edges within a group have high weights (which means that points within the same cluster are similar to each other). To be able to formalize this intuition we first want to introduce some basic graph notation and briefly discuss the kind of graphs we are going to study.
星载高光谱成像系统发展综述

航天返回与遥感第44卷第6期12 SPACECRAFT RECOVERY & REMOTE SENSING2023年12月星载高光谱成像系统发展综述刘思田卢慧王栋李晓兰朱春丽边丽蘅*(北京理工大学复杂环境智能感测技术工信部重点实验室,北京100081)摘要高光谱遥感技术通过记录地表物体在多个连续波段下的光谱信息,实现高精度的地球观测与分析。
为了获取更多地物目标的细节信息,研究人员提出了对高光谱成像系统各项参数指标的新要求,国内外开展了大量相关研究。
随着卫星技术的成熟,高光谱遥感平台从最初的机载平台逐渐发展到星载平台,促进了高光谱遥感图像在地质、农林业、环境监测等领域的广泛应用。
目前,多数光谱成像系统选用传统的光学器件来实现分光,将计算光学与高光谱遥感结合,有利于集成更紧凑便捷的成像系统。
文章首先介绍了高光谱成像系统的主要类型和原理,随后对近30年来典型的星载高光谱成像系统及载荷进行了综述,梳理了典型国内外星载高光谱成像系统的发展现状,并对不同国家成像系统的性能指标进行了对比分析,总结了相应的发展历程,并对未来星载高光谱成像系统的发展作出了展望。
关键词高光谱成像系统遥感载荷星载光谱成像仪发展趋势中图分类号:V248.1;TP391.41文献标志码: A 文章编号: 1009-8518(2023)06-0012-15 DOI: 10.3969/j.issn.1009-8518.2023.06.002Overview of Development Analysis of Space-Borne HyperspectralImaging SystemLIU Sitian LU Hui WANG Dong LI Xiaolan ZHU Chunli BIAN Liheng*(MIIT Key Laboratory of Complex-Field Intelligent Sensing, Beijing Institute of Technology, Beijing 100081, China)Abstract Hyperspectral remote sensing technology provides high-precision earth observation and analysis by recording the spectral information of ground objects over multiple continuous wavelength bands. To obtain more detailed information about the earth’s surface, researchers worldwide have conducted extensive studies on various parameters of hyperspectral remote sensing payloads. With satellite technology maturing, hyperspectral remote sensing has shifted from airborne platforms to satellites, broadening applications in geology, agriculture, forestry, and environmental monitoring. Most spectral imaging systems now rely on traditional optical components for spectral separation. Combining computational optics with hyperspectral remote sensing is conducive to integrating more compact and convenient imaging systems. This paper initiates by introducing the principal types and principles of hyperspectral imaging instruments. It subsequently offers an overview of conventional space-borne hyperspectral remote sensing payloads spanning the last three decades. It analyses the present status of typical hyperspectral payloads on both domestic and international fronts, undertaking a comparative evaluation of their performance metrics. Finally, the paper concludes by encapsulating the associated trends in development. Furthermore, this paper offers insights into the future收稿日期:2023-09-25基金项目:国家自然科学基金面上项目(61971045);国家优秀青年科学基金项目(62322502)引用格式:刘思田, 卢慧, 王栋, 等. 星载高光谱成像系统发展综述[J]. 航天返回与遥感, 2023, 44(6): 12-26.LIU Sitian, LU Hui, WANG Dong, et al. Development Analysis of Spaceborne Hyperspectral Imaging System[J].Spacecraft Recovery & Remote Sensing, 2023, 44(6): 12-26. (in Chinese)第6期刘思田等: 星载高光谱成像系统发展综述 13trends of hyperspectral remote sensing payloads, providing valuable references for advancing research and applications of hyperspectral remote sensing payloads in China.Keywords hyperspectral imager; remote sensing payload; space-borne spectral imager; development trend0 引言高光谱遥感是一种基于成像光谱理论的遥感方法,旨在同时捕获地物的光谱信息和空间位置关系,以实现对地球表面的精确观测和深入分析。
- 1、下载文档前请自行甄别文档内容的完整性,平台不提供额外的编辑、内容补充、找答案等附加服务。
- 2、"仅部分预览"的文档,不可在线预览部分如存在完整性等问题,可反馈申请退款(可完整预览的文档不适用该条件!)。
- 3、如文档侵犯您的权益,请联系客服反馈,我们会尽快为您处理(人工客服工作时间:9:00-18:30)。
Paper ID:627
No Institute Given
Abstract. In this paper, we propose a robust motion segmentation method based on the matrix factorization and subspace separation. We, first, mathematically prove that the shape interaction matrix can be derived using QR decomposition rather than Singular Value Decomposition(SVD). Using shape interaction matrix, we solve the motion segmentation problem using spectral graph clustering technique. We exploit K-way Min-Max cut clustering method. This method yields a good performance for noise-free data, but it is sensitive to noise. We combine a cluster refinement method based on subspace separation to the spectral graph clustering to deal with noise. The proposed method yields very good performance for both synthetic and real image sequences.
1
Introduction
The Matrix factorization methods proposed by Tomasi, Costeira and Kanade [2] [3] have been widely used for solving the motion segmentation problems [4] [5] [6] [7] [8] [9] and the 3D shape recovering problems [10] [11] [12]. The basic idea of the methods is to factorize the feature trajectory matrix into the motion matrix and the shape matrix, providing the separation of the feature point trajectories into independent motions. In this paper, we develop a novel robust factorization method using the techniques of spectral clustering. Given a set of N feature points tracked through F frames, we can construct a feature trajectory matrix P ∈ R2F ×N where the rows correspond to the x or y coordinates of the feature points in the image plane and the columns correspond to the individual feature points. Motion segmentation algorithms based on matrix factorization [7] first construct a shape interaction matrix, Q by applying the singular value decomposition (SVD) to the feature trajectory matrix P. Under the noise-free situation, the shape interaction matrix Q can be transformed to a block diagonal matrix by a symmetric row and column permutation thereby grouping the feature points of the same object into a diagonal block. If the trajectory matrix P is contaminated by noise, however, the block diagonal form of Q no longer holds, and the methods such as the greedy technique proposed in [3] tend to perform rather poorly. Recently there have been several research proposed specifically addressing this problem [8] [6] [4] [5] [6] [7] [9]. We will give a brief review of these methods in Section 2. In this paper we deal with the issues related to the robustness of the factorization methods. We first show that the shape interaction matrix can be
2
Previous Work
The factorization method was originally introduced by Tomasi and Kanade [2]. The method decomposes a matrix of image coordinates of N feature points tracked through F frames into two matrices which, respectively, represent object shape and camera motion. The method deals with a single static object viewed by a moving camera. Extending this method, Costerira and Kanade [3] proposed a multibody factorization method which separates and recovers the shape and motion of multiple independently moving objects in a sequence of images. To achieve this, they introduce a shape interaction matrix which is invariant to both the object motions and the selection of coordinate systems, and suggest a greedy algorithm to permute the shape interaction matrix into block diagonal form. Gear [4] exploited the reduced row echelon form of the shape interaction matrix to group the feature points into the linearly independent subspaces. For Gear’s method, in the noise-free case, any two columns of the echelon form which have nonzero elements in the same row correspond to feature points belonging to the same rigid body. The echelon form matrix can be represented by a weighted bipartite graph. Gear also used a statistical approach to estimate the grouping
2
Paper ID:627来自extracted from the trajectory matrix using QR decomposition with pivoting, an idea that was briefly mentioned in [3]. As a by-product we give a simple and clean proof of the subspace separation theorem described in [7]. We then observe that the shape interaction matrix is very similar to the weight matrix used for graph partitioning and clustering [13] [1] [14], and the motion segmentation problem can be cast as an optimal graph partitioning problem. To this end, we apply the spectral k-way clustering method ?? to the shape interaction matrix to transform it into near-block diagonal form. In particular, we propose a novel QR decomposition based technique for cluster assignment. The technique at the same time also provides confidence levels of the cluster membership for each feature point trajectory. The confidence levels are explored to provide a more robust cluster assignment strategy: we assign a feature point directly to a cluster when it has a very confidence level for the cluster compared to those for other clusters. Using the assigned feature points in each cluster, we compute a linear subspace in the trajectory space. The cluster memberships of other feature points having lower confidence levels, and are therefore not assigned to a cluster, are determined by their distances to each of the linear subspaces. Our experiments on both synthetic data sets and real video images have shown that this method are very reliable for motion segmentation even in the presence of severe noise. The rest of the paper is organized a s follows: Previous works are discussed in Section 2 discusses. Section 3 is devoted to a simple proof that the shape interaction matrix can be computed using QR decomposition. Motion segmentation based on spectral relaxation k-way clustering and subspace separation is followed in Section 4. Experiment results are shown in Section 5 and conclusion is given in 6.