modele-cv-sans-photo-entete1
gtsam 常用因子

gtsam 常用因子English answers:IMU Preintegration.Inertial Measurement Unit (IMU) preintegration is a technique used to integrate the IMU measurements over a period of time, typically between two keyframes. This reduces the computational cost of integrating the measurements online and allows for more efficient optimization. GTSAM provides a robust and accurate implementation of IMU preintegration.Stereo Vision.Stereo vision is a technique used to estimate the depth of a scene using two or more cameras. GTSAM provides a variety of stereo vision factors, including the pinhole model and the fisheye model. These factors can be used to estimate the pose of the cameras and the depth of the scene.Lidar.Lidar (Light Detection and Ranging) is a remote sensing technology that uses laser pulses to measure the distance to objects. GTSAM provides a variety of lidar factors, including the point-to-plane, point-to-line, and plane-to-plane models. These factors can be used to estimate the pose of the lidar sensor and the location of objects in the scene.GPS.Global Positioning System (GPS) is a satellite-based navigation system that provides location and time information. GTSAM provides a variety of GPS factors, including the position-only model and the velocity-aided model. These factors can be used to estimate the pose of the GPS receiver and the velocity of the vehicle.Odomery.Odometry is a technique used to estimate the pose of a vehicle using the measurements from its wheel encoders. GTSAM provides a variety of odometry factors, including the differential drive model and the unicycle model. These factors can be used to estimate the pose of the vehicle and the velocity of the wheels.Chinese answers:IMU预积分。
TG-320 数码相机规格说明书

Specifications TG-320Specifications and appearances are subject to change without any notice or obli-gation on the part of the manufacturer.Image Sensor Effective pixels 14 Megapixels Full resolution 14.5 Megapixels Type1/2.3'' CCD sensor LensOptical zoom 3.6x (WIDE)Focal length5.0 - 18.2mm Focal length (equiv. 35mm)28 - 102mmStructure10 lenses / 8 groups Aspherical glass elements 4ED glass elements 2Maximum aperture 3.5 - 5.1Digital Zoom Enlargement factor 4x / 14.4x Monitor Monitor type TFTMonitor size 6.9cm / 2.7''Resolution230,000 dots Brightness adjustment +/- 2 levels Frame assistance Yes Protection panel YesFocusing System Method TTL iESP auto focus with contrast detection ModesiESP , Face Detection AF, Spot, AF Tracking Standard mode 0.5m - ∞ (wide) / 0.5m - ∞ (tele)Macro mode0.1m - ∞ (wide) / 0.3m - ∞ (tele)Super Macro mode Closest focusing distance: 2cm Light MeteringHistogram in shooting mode YesModesESP light metering, Spot metering Exposure System Shutter speed1/4 - 1/2000s / < 4s(Night scene)Exposure compensation +/- 2EV / 1/3 stepsEnhancement functionMechanical Image StabiliserAdvanced Face Detection Technology Pet DetectionModesi-Auto, Programme automatic, Magic Filter, Panorama, Scene Modes, Movie, 3D Photos Scene ModesNumber of scene modes 17ModesPortrait, Beauty, Landscape, Night Scene, Night Scene with portrait, Sports, Indoor, Candle, Self-portrait, Sunset, Fireworks, Cuisine, Documents, Beach and Snow, Underwater Snapshot, Pet (cat), Pet (dog)Magic Filter Types Pop Art, Pin Hole, Fisheye, Drawing, Soft Focus, Punk, Sparkle, Water colorSensitivity Auto AUTO / High AUTOManual ISO 80, 100, 200, 400, 800, 1600White Balance AUTO WB system YesPreset valuesOvercast, Sunlight, Tungsten, Flourescent 1, UnderwaterSequence ShootingSequential shooting mode (high speed)3 fps / 200 frames (in 5MP mode)Sequential shooting mode 0.6 fps / 200 frames (Full Image Size)Image Processing Pixel mapping Yes Noise reduction YesEngineTruePic III+ Distortion compensation Yes Shading compensation YesImage EditingResize, Trimming, Correction of saturation, Beauty Fix, Red-eye reduction, Shadow AdjustmentMovie Recording System Recording formatMPEG-4Image Stabilisation Mode Digital Image Stabilisation HD Movie quality 720P Recording time: 29min.Movie qualityVGA Recording time: Up to card capacity QVGA Recording time: Up to card capacity Note: maximum file size 4GBWhen shooting 720P movies use SDHC/SDXC class 6 or higher.Magic FilterPop Art, Fisheye, Drawing, Soft Focus, Punk, Water colorSound Recording System Voice playback YesSound recording Yes, format: AAC Image footage 4s SpeakerYesMemoryRemovable MediaSD / SDHC / SDXC (UHS speed class not supported)Eye-Fi Card compatible Yes Internal memory 19.5MB Image Size 14M 4288 x 32168M 3264 x 24485M 2560 x 19203M 2048 x 15362M 1600 x 12001M 1280 x 960VGA 640 x 48016:94288 x 24161920 x 1080View Images Modes Single, Index, Zoom, Slide show, Event, Photo Surfing Index 4x3 / 6x5 frames ZoomYes, 1.1 - 10x Auto rotationYes Image protect modeYes Histogram in playback mode YesView Movie ModesFrame by frame, Fast forward, Reverse playback Internal Flash Working range (wide)0.2 - 4.3 m (ISO 800) Working range (tele)0.3 - 2.8 m (ISO 800)ModesAUTO, Red-eye reduction, Fill-in, Off Power Supply BatteryLI-42B Lithium-Ion Battery Internal ChargingYesInterface HDMI™Yes, Micro connector (Type D)*DC inputYes Combined A/V & USB output Yes USB 2.0 High SpeedYes* "HDMI", the HDMI logo and "High-DefinitionMultimedia Interface" are trademarks or registered trademarks of HDMI Licensing LLC.Other Features Shock resistantShock-proof from heights of up to 1.5m *Nitrogen-filled body forwaterproof/fogproof/dirtproof performance Waterproof up to a water pressure equivalent to 3m depth **FreezeproofFreezeproof up to -10°C ***3D Photo Shooting Mode Yes Perfect Shot Preview YesPanorama function In-Camera Panorama Self timer 2 / 12 s Pet auto shutter Menu guideYes In-camera Manual Yes Date imprint Yes Photo SurfingYes* Equivalent to MIL Standard (Olympus test conditions)** According to IEC standard publication 529 IPX8*** According to Olympus test conditions SizeDimensions (W x H x D)96.3 x 63.4 x 22.7mmWeight155g (including battery and memory card)。
瞰景Smart3D建模软件镜像的使用流程说明书
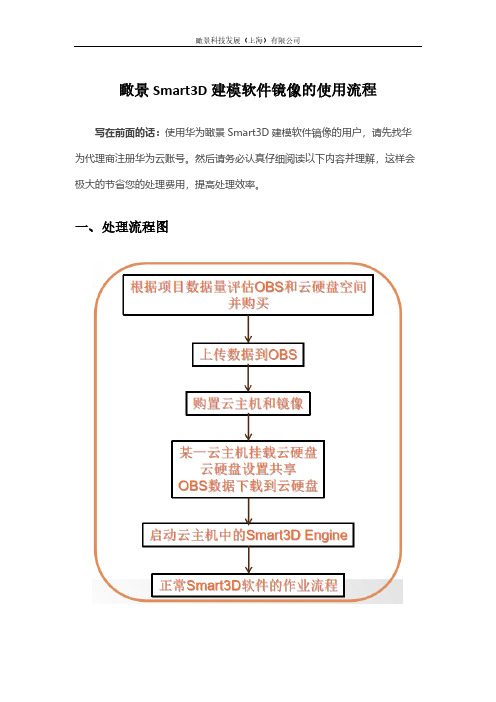
瞰景Smart3D建模软件镜像的使用流程写在前面的话:使用华为瞰景Smart3D建模软件镜像的用户,请先找华为代理商注册华为云账号。
然后请务必认真仔细阅读以下内容并理解,这样会极大的节省您的处理费用,提高处理效率。
一、处理流程图二、关于推荐的云主机说明注意:选择实例时,务必先阅读本手册内容。
界面上的推荐配置包括:空三云主机和建模云主机。
对于按需购买的用户,推荐空三云主机和建模云主机分开使用。
因为空三云主机不需要用到显卡,价格会更便宜。
所以分开使用成本更低。
空三云主机:“超高I/O型ir3.xlarge.4”主要用于空三流程中的特征提取和特征匹配的计算;“内存优化型m6系列”因内存有64GB和128GB所以推荐用于空三的平差处理,当然为了充分利用其算力,引擎能力全开能够实现空三全流程处理。
如果需要更大内存的云主机,可以在界面上选择“自定义云主机”进行选择更大内存如196GB、256GB的m6系列。
由于推荐的空三云主机中不带GPU,所以无法在Smart3D Master的三维界面下查看航点数据和空三结果。
因此如果要在Smart3D Master中可视化分块、刺点、三维浏览等步骤,就需要启动一台带GPU的云主机。
可以选择一台建模主机如“GPU加速型g5r.4xlarge.2”。
因为g5r的云主机的内存只有32GB所以,在引擎能力设置时需要关闭“图像相似性计算”和“光束法平差”的能力。
以上就是一套的空三计算的资源配置(1个m6+5个ir3+1个g5r)适合处理1-2万张照片的工程,处理时间3-4小时。
超过2万张照片的数据可以参考瞰景Smart3D软件的数据分块处理,详情查看中的分块操作。
同样根据项目的处理周期的需要,可以增加和减少云主机。
另外需要注意的是空三过程中的g5r可以用于空三也可以仅用于三维显示,如果是仅用于三维显示,可以在显示完后,将g5r的主机关机以节省成本。
(瞰景Smart3D 在10月份发布的版本支持64GB内存处理5万张照片,128GB内存处理10万张照片左右,可以减少分块处理。
犀牛指令集
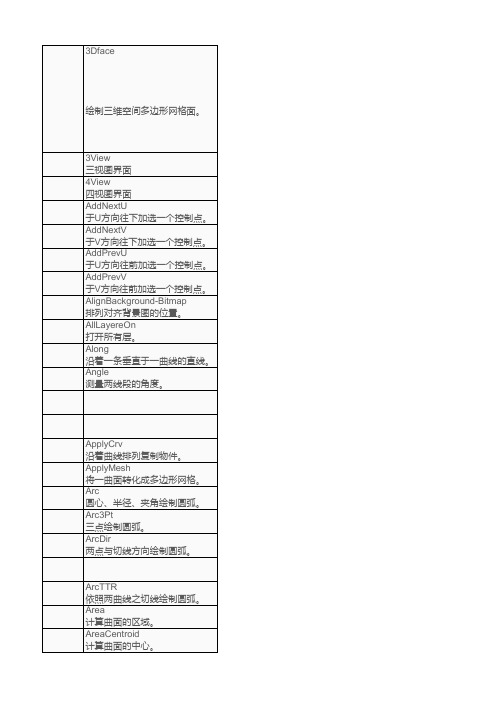
绘制与一曲线成切线的线段。 LineTT 绘制与二次曲线成切线的线段。 LineV 绘制与设计平面垂直的线段。 List 列出物件的设计资料。 Lock 锁定物体。 LockOsnap 锁住物体的捕捉功能。 Loft 放样曲面。 Make2D 将立体物体转绘成平面图。 MakeCrvPeriodic 将开放的线段封闭,或者将已封 闭曲线平滑化 MakeSrfNonPeriodic 将曲面变成可以产生节点的曲面 。akeSrfPeriodic M 将开放的曲面封闭,或者将已封 闭曲面平滑化 Match 两曲线端点靠齐。 MatchLayer 将选择的对象移动到另一层。 MatchSrf 将两个不相连曲面的边缘拉靠在 一起。 MaxViewport 最大化视图显示。 MergeEdge 连接曲面上相邻的两边缘。 MergeSrf 连接二相邻未经修剪得曲面。 Mesh 将NURBS对象转换成网格物体 。eshBox M 绘制多边形网格立方体。 MeshCone 绘制多边形网格圆锥体。 MeshCylinder 绘制多边形网格圆柱体。 MeshDensity 改变多边形网格的密度。 MeshPlane 绘制多边形网格平面。 MeshPolyline 从一封闭多边形曲线建立多边形 网格。
Polygon 绘制多边形。 PolygonEdge 以一边绘制多边形。 Polyline 以选择的点建立线段。 PolylineOnMesh PolylineThroughPt 以选择的点建立线段。 PrevU 选择U坐标上的一个控制点。 PrevV 选择V坐标上的一个控制点。 Print 打印。 PrintSetup 打印摄制。 Project 投射一曲线至曲面上。 Projection 切换视图为等角视图或透视图。 ProjectOsnap 投射物体捕捉的点至设计平面上 。 ProjectToCPlane 投射物体至设计平面上。 Properties 编辑物体属性。 Pt 捕捉点。 PtOff 关闭显示控制点和编辑点。 PtOn 打开显示控制点和编辑点。 Pull 依靠近的部位将曲线拉至曲面上 。 Quad 捕捉圆或椭圆的四个点。 Radius 测量弧的半径。 RaliRevolve 将一曲线沿着一轨道与轴心旋转 成型。 ReadCommandFile 从外部文档读取命令文件。 ReadNamedCPlanesFromFile 读取已命名的视图或设计平面。 ReadViewportsFromFile 从外部3DM文件读进视区。 RebuildEdges 重建曲面边缘的组成架构。 RebuildSrf
DIRART (Deformable Image Registration and Adaptive Radiotherapy) Software Suite

1
Table of Content DIRART (Deformable Image Registration and Adaptive Radiotherapy) Software Suite.............. 1 (Version 1.0a) ................................................................................................................................. 1 User Instruction Manual ................................................................................................................. 1 Version 0.1...................................................................................................................................... 1 Deshan Yang, PhD...................................................................................................................... 1 Issam El Naqa, PhD .................................................................................................
ae滤镜中英文对照文库
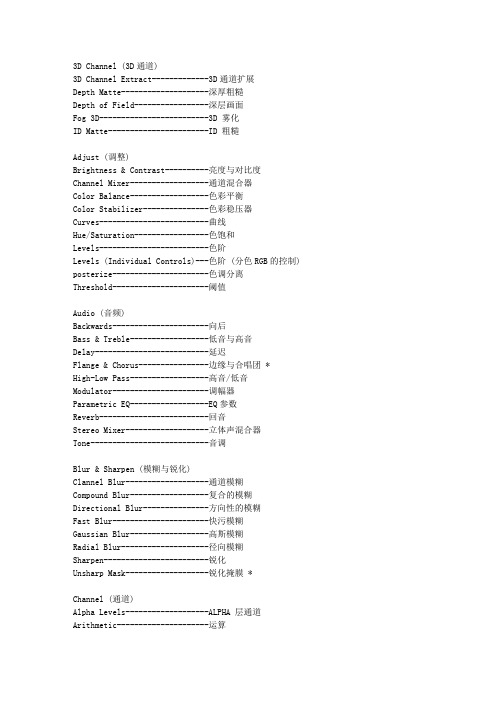
3D Channel (3D通道)3D Channel Extract-------------3D通道扩展Depth Matte--------------------深厚粗糙Depth of Field-----------------深层画面Fog 3D-------------------------3D 雾化ID Matte-----------------------ID 粗糙Adjust (调整)Brightness & Contrast----------亮度与对比度Channel Mixer------------------通道混合器Color Balance------------------色彩平衡Color Stabilizer---------------色彩稳压器Curves-------------------------曲线Hue/Saturation-----------------色饱和Levels-------------------------色阶Levels (Individual Controls)---色阶 (分色RGB的控制) posterize----------------------色调分离Threshold----------------------阈值Audio (音频)Backwards----------------------向后Bass & Treble------------------低音与高音Delay--------------------------延迟Flange & Chorus----------------边缘与合唱团 *High-Low Pass------------------高音/低音Modulator----------------------调幅器Parametric EQ------------------EQ参数Reverb-------------------------回音Stereo Mixer-------------------立体声混合器Tone---------------------------音调Blur & Sharpen (模糊与锐化)Clannel Blur-------------------通道模糊Compound Blur------------------复合的模糊Directional Blur---------------方向性的模糊Fast Blur----------------------快污模糊Gaussian Blur------------------高斯模糊Radial Blur--------------------径向模糊Sharpen------------------------锐化Unsharp Mask-------------------锐化掩膜 *Channel (通道)Alpha Levels-------------------ALPHA 层通道Arithmetic---------------------运算Bland--------------------------柔化Cineon Converter---------------间距转换器Compound Arithmetic------------复合运算Invert-------------------------反向Minimax------------------------像素化Remove Color Matting-----------去除粗颗粒颜色 *Set Channels-------------------调节通道Set Matte----------------------调节粗糙度Shift Channels-----------------转换通道Distort (变型)Bezier Warp--------------------Bezier 变型Bulge--------------------------鱼眼Displacement Map---------------画面偏移Mesh Warp----------------------网状变形Mirror-------------------------镜像Offset-------------------------偏移量Optics Compensation------------光学替换 (可制作球体滚动效果) Polar Coordinates--------------极坐标Reshape------------------------重塑Ripple-------------------------涟漪Smear--------------------------涂片Spherize-----------------------球型变形Transform----------------------变换Twirl--------------------------旋转变形Wave Warp----------------------波型变形Expression Controls (表达式控制)Angle Control------------------角度控制Checkbox Control---------------复选框控制Color Control------------------颜色控制Layer Control------------------图层控制Point Control------------------锐化控制Slider Control-----------------滑块控制Image Control (图像控制)Chaner Color-------------------改变颜色Color Balance (HLS)------------色彩平衡 (HLS)Colorama-----------------------着色剂Equalize-----------------------平衡Gamma/Pedestal/Gain------------GAMMA/电平/增益Median-------------------------中线PS Arbitrary MapPS-------------任意的映射Tint---------------------------去色Keying (键控制)Color Difference Key-----------差异的色键Color Key----------------------色键Color Range--------------------色键幅度Difference Matte---------------不同粗粗糙 (以粗颗粒渐变到下一张图) Extract------------------------扩展Inner Outer Key----------------内部、外部色键Linear Color Key---------------线性色键Luma Key-----------------------LUMA键Spill Suppressor---------------溢出抑制器Matte Tools (粗糙工具)Matte Cloker-------------------粗糙窒息物 *Simple Choker------------------简单的窒息物 *Paint (油漆)Vector Paint-------------------矢量油漆Perspective (透视)Basic 3D-----------------------基本的3DBevel Alpha--------------------倾斜 ALPHABevel Edges--------------------倾斜边Drop Shadow--------------------垂直阴影Render (渲染)4-Color Gradient---------------4色倾斜度Advanced Lightning-------------高级闪电Audio Spectrum-----------------音频光谱Audio Waveform-----------------音频波形Beam---------------------------射线Cell Pattern-------------------单元模式Ellipse------------------------椭圆Fill---------------------------填充Fractal------------------------分数维Fractal Noise------------------粗糙的分数维Grid---------------------------网格Lens Flare---------------------镜头光晕Lightning----------------------闪电Radio Waves--------------------音波Ramp---------------------------斜面Stroke-------------------------笔划 (与stylize-write on功能类似) Vegas--------------------------维加斯Simulation (模拟)Particle Playground------------粒子运动场Shatter------------------------粉碎Stylize (风格化)Brush Strokes------------------笔刷Color Emboss-------------------颜色浮雕Emboss-------------------------浮雕Find Edges---------------------查找边缘Glow---------------------------照亮边缘Leave Color--------------------离开颜色Mosaic-------------------------马赛克Motion Tile--------------------运动平铺Noise--------------------------噪音Roughen Edges------------------变粗糙边Scatter------------------------分散Strobe Light-------------------匣门光 *Texturize----------------------基底凸现Write-on-----------------------在.....上写 (与render-stroke功能类似)Text (文本)Basic Text---------------------基本的文本Numbers------------------------数字文本Path Text----------------------路径文本Time (时间)Echo---------------------------回响Posterize Time-----------------发布时间Time Difference----------------时间差别 *Time Displacement--------------时间偏移Transition (转场)Block Dissolve-----------------块溶解Gradient Wipe------------------斜角转场Iris Wipe----------------------爱丽斯转场 (三角形转场)Linear Wipe--------------------线性转场Radial Wipe--------------------半径转场Venetian Blinds----------------直贡呢的遮掩 (百叶窗式转场)Video (视频)Broadcast Colors---------------广播色Reduce Interlace Flicker-------降低频闪Timecode-----------------------时间码。
AXIS P13网络摄像头系列说明书
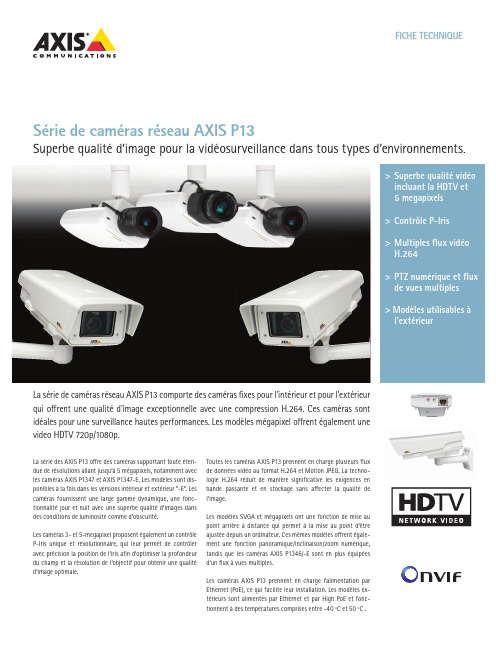
FICHE TECHNIQUELa série de caméras réseau AXIS P13 comporte des caméras fixes pour l’intérieur et pour l’extérieur qui offrent une qualité d’image exceptionnelle avec une compression H.264. Ces caméras sont idéales pour une surveillance hautes performances. Les modèles mégapixel offrent également une video HDTV 720p/1080p.Série de caméras réseau AXIS P13Superbe qualité d’image pour la vidéosurveillance dans tous types d’environnements.> Superbe qualité vidéo incluant la HDTV et 5 megapixels > Contrôle P-Iris > Multiples flux vidéo H.264> PTZ numérique et flux de vues multiples > Modèles utilisables à l’extérieurLa série des AXIS P13 offre des caméras supportant toute éten-due de résolutions allant jusqu’à 5 mégapixels, notamment avec les caméras AXIS P1347 et AXIS P1347-E. Les modèles sont dis-ponibles à la fois dans les versions intérieur et extérieur ”-E ”. Les caméras fournissent une large gamme dynamique, une fonc-tionnalité jour et nuit avec une superbe qualité d’images dans des conditions de luminosité comme d’obscurité.Les caméras 3- et 5-megapixel proposent également un contrôle P-I ris unique et révolutionnaire, qui leur permet de contrôler avec précision la position de l’iris afin d’optimiser la profondeur du champ et la résolution de l’objectif pour obtenir une qualité d’image optimale.Toutes les caméras AXIS P13 prennent en charge plusieurs flux de données vidéo au format H.264 et Motion JPEG. La techno-logie H.264 réduit de manière significative les exigences en bande passante et en stockage sans affecter la qualité de l’image.Les modèles SVGA et mégapixels ont une fonction de mise au point arrière à distance qui permet à la mise au point d’êtreajustée depuis un ordinateur. Ces mêmes modèles offrent égale-ment une fonction panoramique/inclinaison/zoom numérique, tandis que les caméras AXI S P1346/-E sont en plus équipées d’un flux à vues multiples. Les caméras AXI S P13 prennent en charge l’alimentation par Ethernet (PoE), ce qui facilite leur installation. Les modèles ex-térieurs sont alimentés par Ethernet et par High PoE et fonc-tionnent à des températures comprises entre -40 ºC et 50 ºC .La série AXIS P13 comprend des caméras réseau fixes conçues pour l’intérieur et l’extérieur qui conviennent à un large éventail d’applications de vidéosurveillance, bâtiments publics ou industriels, commerces, aéroports, gares et écoles. Caméras hautes performances pour l’intérieur/l’extérieurInstallation facile grâce à l’assistance à la mise au point, la mise au point à distance et le compteur de pixelsL’assistance à la mise au point simplifie le réglage de la mise au point de toutes les caméras AXIS P13, grâce au clignotement d’un voyant vert lorsqu’une image est au point après un réglage manuel de l’objectif. De plus, les modèles SVGA et mégapixel/HDTV sont équipés d’une fonction de mise au point à distance du foyer arrière qui permet l’ajustement de la mise au point à partir d’un ordina-teur. Le compteur de pixels permet de vérifier que la résolution en pixels d’un objet est conforme aux règlementations en vigueur ou aux besoins du client (ex. : reconnaissance faciale).Modèles utilisables àl’extérieurLes caméras réseau AXI S P13-E permet-tent de gagner du temps et de réaliser deséconomies puisqu’elles sont immédiate-ment prêtes pour un montage en exté-rieur. Certifiées IP66, elles sont protégéescontre la poussière, la pluie, la neige et lesrayons du soleil et peuvent fonctionnerjusqu’à une température de -40 °C. Lescaméras sont alimentées par Ethernet, cequi facilite l’installation puisqu’elles nenécessitent pas de câble d’alimentation séparé. Une membrane de déshumidifi-cation intégrée permet d’éliminer toute l’humidité le boîtier de la caméra lors de l’installation. Ces caméras permettent l’installation facile d’une lampe à infrarouge sous le boîtier. Ils arrivent avec un support de montage mural, un pare-soleil et les presse-étoupes.PTZ numérique et flux à vue multipleLes modèles de caméras SVGA et mégapixel sont dotés de fonctions pan-oramiques, d’inclinaison et de zoom numériques qui permettent de sélectionnerune vue détourée de la vue d’ensemble pour l’afficher ou l’enregistrer, ce quiréduit ainsi le débit binaire et l’espace de stockage requis. Les caméras 3- et5-megapixel sont également dotées de la fonction de flux à vues multiplesqui permet de transmettre simultanément plusieurs zones détourées de la vuecomplète, simulant jusqu’à huit caméras virtuelles.Flux à vues multiples avec les cameras réseau AXIS P1346/-E et AXIS P1347/-EUne caméra Vue panoramique complète offrant des zonesdétourées de la vue complètePlusieurs champs de vision virtuels dela caméra (jusqu’à huit vues possibles)Contrôle P-IrisLes cameras 3-megapixel AXI S P1346/-E et 5-megapixel AXI S P1347/-E of-frent un nouveau contrôle précis de l’iris avancé, P-Iris, qui établit de nouvelles normes de qualité d’image pour les caméras fixes. Ce contrôle comporte un objectif P-Iris spécial associé à un logiciel spécialisé de la caméra qui fournit la meilleure position de l’iris pour un contraste, une clarté, une résolution et une profondeur de champ améliorés de l’image. Une bonne profondeur de champ, où des objets situés à différentes distances de la caméra sont simultanément mis au point, permet d’obtenir une meilleure visibilité de scène.Le P-Iris est particulièrement utile aux caméras mégapixel/HDTV, car il permet de continuer à obtenir des images haute résolution nettes, même dans des conditions d’éclairage difficiles. Il utilise le même type de connecteur et de câble que l’iris DC classique qui est également pris en charge par les caméras 3- et 5-megapixel pour la rétrocompatibilité.Pour en savoir plus sur P-Iris et ses contrôles, cliquez sur le lien :/corporate/corp/tech_papers.htmAXIS P1343/P1344/P1346/P1347 :Microphone intégréPour plus d’informations, visitez le site ** Ce produit inclut un logiciel développé par le projet OpenSSLpour une utilisation dans la boîte à outils OpenSSL. ()©2012 Axis Communications AB. AXIS COMMUNICATIONS, AXIS, ETRAX, ARTPEC et VAPIX sont des marques déposées d’Axis AB ou en cours de dépôt par Axis AB dans différentes juridictions. Tous les autres noms, produits ou services sont la propriété de leurs détenteurs respectifs. Document sujet à modification sans préavis.A x i s C o m m u n i c a t i o n s S A S -R C S B 4 0 8 9 6 9 9 9 8 4 7 2 9 2 / F R / R 1 / 1 2 0 4AXIS T8123 à 1 port。
VoxelNet_ End-to-End Learning for Point Cloud Base
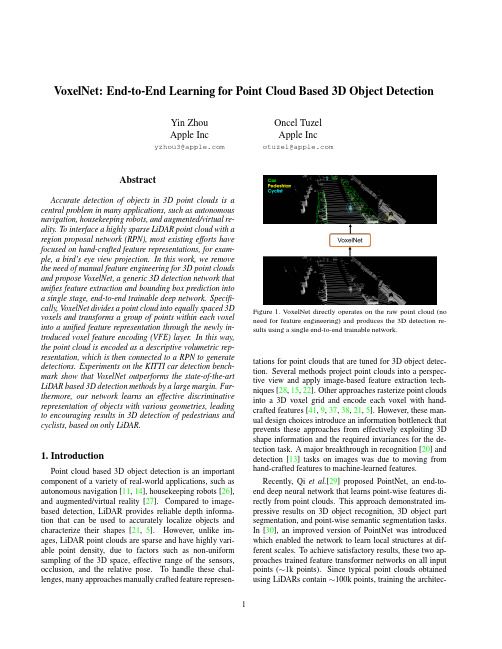
VoxelNet:End-to-End Learning for Point Cloud Based3D Object DetectionYin ZhouApple Inc****************Oncel TuzelApple Inc****************AbstractAccurate detection of objects in3D point clouds is a central problem in many applications,such as autonomous navigation,housekeeping robots,and augmented/virtual re-ality.To interface a highly sparse LiDAR point cloud with a region proposal network(RPN),most existing efforts have focused on hand-crafted feature representations,for exam-ple,a bird’s eye view projection.In this work,we remove the need of manual feature engineering for3D point clouds and propose VoxelNet,a generic3D detection network that unifies feature extraction and bounding box prediction into a single stage,end-to-end trainable deep network.Specifi-cally,VoxelNet divides a point cloud into equally spaced3D voxels and transforms a group of points within each voxel into a unified feature representation through the newly in-troduced voxel feature encoding(VFE)layer.In this way, the point cloud is encoded as a descriptive volumetric rep-resentation,which is then connected to a RPN to generate detections.Experiments on the KITTI car detection bench-mark show that VoxelNet outperforms the state-of-the-art LiDAR based3D detection methods by a large margin.Fur-thermore,our network learns an effective discriminative representation of objects with various geometries,leading to encouraging results in3D detection of pedestrians and cyclists,based on only LiDAR.1.IntroductionPoint cloud based3D object detection is an important component of a variety of real-world applications,such as autonomous navigation[11,14],housekeeping robots[26], and augmented/virtual reality[27].Compared to image-based detection,LiDAR provides reliable depth informa-tion that can be used to accurately localize objects and characterize their shapes[21,5].However,unlike im-ages,LiDAR point clouds are sparse and have highly vari-able point density,due to factors such as non-uniform sampling of the3D space,effective range of the sensors, occlusion,and the relative pose.To handle these chal-lenges,many approaches manually crafted featurerepresen-Figure1.V oxelNet directly operates on the raw point cloud(no need for feature engineering)and produces the3D detection re-sults using a single end-to-end trainable network.tations for point clouds that are tuned for3D object detec-tion.Several methods project point clouds into a perspec-tive view and apply image-based feature extraction tech-niques[28,15,22].Other approaches rasterize point clouds into a3D voxel grid and encode each voxel with hand-crafted features[41,9,37,38,21,5].However,these man-ual design choices introduce an information bottleneck that prevents these approaches from effectively exploiting3D shape information and the required invariances for the de-tection task.A major breakthrough in recognition[20]and detection[13]tasks on images was due to moving from hand-crafted features to machine-learned features.Recently,Qi et al.[29]proposed PointNet,an end-to-end deep neural network that learns point-wise features di-rectly from point clouds.This approach demonstrated im-pressive results on3D object recognition,3D object part segmentation,and point-wise semantic segmentation tasks.In[30],an improved version of PointNet was introduced which enabled the network to learn local structures at dif-ferent scales.To achieve satisfactory results,these two ap-proaches trained feature transformer networks on all input points(∼1k points).Since typical point clouds obtained using LiDARs contain∼100k points,training the architec-1Figure2.V oxelNet architecture.The feature learning network takes a raw point cloud as input,partitions the space into voxels,and transforms points within each voxel to a vector representation characterizing the shape information.The space is represented as a sparse 4D tensor.The convolutional middle layers processes the4D tensor to aggregate spatial context.Finally,a RPN generates the3D detection.tures as in[29,30]results in high computational and mem-ory requirements.Scaling up3D feature learning networks to orders of magnitude more points and to3D detection tasks are the main challenges that we address in this paper.Region proposal network(RPN)[32]is a highly opti-mized algorithm for efficient object detection[17,5,31, 24].However,this approach requires data to be dense and organized in a tensor structure(e.g.image,video)which is not the case for typical LiDAR point clouds.In this pa-per,we close the gap between point set feature learning and RPN for3D detection task.We present V oxelNet,a generic3D detection framework that simultaneously learns a discriminative feature represen-tation from point clouds and predicts accurate3D bounding boxes,in an end-to-end fashion,as shown in Figure2.We design a novel voxel feature encoding(VFE)layer,which enables inter-point interaction within a voxel,by combin-ing point-wise features with a locally aggregated feature. Stacking multiple VFE layers allows learning complex fea-tures for characterizing local3D shape information.Specif-ically,V oxelNet divides the point cloud into equally spaced 3D voxels,encodes each voxel via stacked VFE layers,and then3D convolution further aggregates local voxel features, transforming the point cloud into a high-dimensional volu-metric representation.Finally,a RPN consumes the vol-umetric representation and yields the detection result.This efficient algorithm benefits both from the sparse point struc-ture and efficient parallel processing on the voxel grid.We evaluate V oxelNet on the bird’s eye view detection and the full3D detection tasks,provided by the KITTI benchmark[11].Experimental results show that V oxelNet outperforms the state-of-the-art LiDAR based3D detection methods by a large margin.We also demonstrate that V oxel-Net achieves highly encouraging results in detecting pedes-trians and cyclists from LiDAR point cloud.1.1.Related WorkRapid development of3D sensor technology has moti-vated researchers to develop efficient representations to de-tect and localize objects in point clouds.Some of the earlier methods for feature representation are[39,8,7,19,40,33, 6,25,1,34,2].These hand-crafted features yield satisfac-tory results when rich and detailed3D shape information is available.However their inability to adapt to more complex shapes and scenes,and learn required invariances from data resulted in limited success for uncontrolled scenarios such as autonomous navigation.Given that images provide detailed texture information, many algorithms infered the3D bounding boxes from2D images[4,3,42,43,44,36].However,the accuracy of image-based3D detection approaches are bounded by the accuracy of the depth estimation.Several LIDAR based3D object detection techniques utilize a voxel grid representation.[41,9]encode each nonempty voxel with6statistical quantities that are de-rived from all the points contained within the voxel.[37] fuses multiple local statistics to represent each voxel.[38] computes the truncated signed distance on the voxel grid.[21]uses binary encoding for the3D voxel grid.[5]in-troduces a multi-view representation for a LiDAR point cloud by computing a multi-channel feature map in the bird’s eye view and the cylindral coordinates in the frontal view.Several other studies project point clouds onto a per-spective view and then use image-based feature encoding公众号DASOU-整理schemes[28,15,22].There are also several multi-modal fusion methods that combine images and LiDAR to improve detection accu-racy[10,16,5].These methods provide improved perfor-mance compared to LiDAR-only3D detection,particularly for small objects(pedestrians,cyclists)or when the objectsare far,since cameras provide an order of magnitude more measurements than LiDAR.However the need for an addi-tional camera that is time synchronized and calibrated with the LiDAR restricts their use and makes the solution more sensitive to sensor failure modes.In this work we focus on LiDAR-only detection.1.2.Contributions•We propose a novel end-to-end trainable deep archi-tecture for point-cloud-based3D detection,V oxelNet, that directly operates on sparse3D points and avoids information bottlenecks introduced by manual feature engineering.•We present an efficient method to implement V oxelNet which benefits both from the sparse point structure and efficient parallel processing on the voxel grid.•We conduct experiments on KITTI benchmark and show that V oxelNet produces state-of-the-art results in LiDAR-based car,pedestrian,and cyclist detection benchmarks.2.VoxelNetIn this section we explain the architecture of V oxelNet, the loss function used for training,and an efficient algo-rithm to implement the network.2.1.VoxelNet ArchitectureThe proposed V oxelNet consists of three functional blocks:(1)Feature learning network,(2)Convolutional middle layers,and(3)Region proposal network[32],as il-lustrated in Figure2.We provide a detailed introduction of V oxelNet in the following sections.2.1.1Feature Learning NetworkVoxel Partition Given a point cloud,we subdivide the3D space into equally spaced voxels as shown in Figure2.Sup-pose the point cloud encompasses3D space with range D, H,W along the Z,Y,X axes respectively.We define each voxel of size v D,v H,and v W accordingly.The resulting 3D voxel grid is of size D =D/v D,H =H/v H,W = W/v W.Here,for simplicity,we assume D,H,W are a multiple of v D,v H,v W.Grouping We group the points according to the voxel they reside in.Due to factors such as distance,occlusion,ob-ject’s relative pose,and non-uniform sampling,the LiDARFullyConnectedNeuralNetPoint-wiseInputPoint-wiseFeatureElement-wiseMaxpoolPoint-wiseConcatenateLocallyAggregatedFeaturePoint-wiseconcatenatedFeatureFigure3.V oxel feature encoding layer.point cloud is sparse and has highly variable point density throughout the space.Therefore,after grouping,a voxel will contain a variable number of points.An illustration is shown in Figure2,where V oxel-1has significantly more points than V oxel-2and V oxel-4,while V oxel-3contains no point.Random Sampling Typically a high-definition LiDAR point cloud is composed of∼100k points.Directly pro-cessing all the points not only imposes increased mem-ory/efficiency burdens on the computing platform,but also highly variable point density throughout the space might bias the detection.To this end,we randomly sample afixed number,T,of points from those voxels containing more than T points.This sampling strategy has two purposes,(1)computational savings(see Section2.3for details);and(2)decreases the imbalance of points between the voxels which reduces the sampling bias,and adds more variation to training.Stacked Voxel Feature Encoding The key innovation is the chain of VFE layers.For simplicity,Figure2illustrates the hierarchical feature encoding process for one voxel. Without loss of generality,we use VFE Layer-1to describe the details in the following paragraph.Figure3shows the architecture for VFE Layer-1.Denote V={p i=[x i,y i,z i,r i]T∈R4}i=1...t as a non-empty voxel containing t≤T LiDAR points,where p i contains XYZ coordinates for the i-th point and r i is the received reflectance.Wefirst compute the local mean as the centroid of all the points in V,denoted as(v x,v y,v z). Then we augment each point p i with the relative offset w.r.t. the centroid and obtain the input feature set V in={ˆp i= [x i,y i,z i,r i,x i−v x,y i−v y,z i−v z]T∈R7}i=1...t.Next, eachˆp i is transformed through the fully connected network (FCN)into a feature space,where we can aggregate in-formation from the point features f i∈R m to encode the shape of the surface contained within the voxel.The FCN is composed of a linear layer,a batch normalization(BN) layer,and a rectified linear unit(ReLU)layer.After obtain-ing point-wise feature representations,we use element-wise MaxPooling across all f i associated to V to get the locally aggregated feature˜f∈R m for V.Finally,we augmenteach f i with˜f to form the point-wise concatenated featureas f outi =[f T i,˜f T]T∈R2m.Thus we obtain the outputfeature set V out={f outi }i...t.All non-empty voxels areencoded in the same way and they share the same set of parameters in FCN.We use VFE-i(c in,c out)to represent the i-th VFE layer that transforms input features of dimension c in into output features of dimension c out.The linear layer learns a ma-trix of size c in×(c out/2),and the point-wise concatenation yields the output of dimension c out.Because the output feature combines both point-wise features and locally aggregated feature,stacking VFE lay-ers encodes point interactions within a voxel and enables thefinal feature representation to learn descriptive shape information.The voxel-wise feature is obtained by trans-forming the output of VFE-n into R C via FCN and apply-ing element-wise Maxpool where C is the dimension of the voxel-wise feature,as shown in Figure2.Sparse Tensor Representation By processing only the non-empty voxels,we obtain a list of voxel features,each uniquely associated to the spatial coordinates of a particu-lar non-empty voxel.The obtained list of voxel-wise fea-tures can be represented as a sparse4D tensor,of size C×D ×H ×W as shown in Figure2.Although the point cloud contains∼100k points,more than90%of vox-els typically are empty.Representing non-empty voxel fea-tures as a sparse tensor greatly reduces the memory usage and computation cost during backpropagation,and it is a critical step in our efficient implementation.2.1.2Convolutional Middle LayersWe use Conv M D(c in,c out,k,s,p)to represent an M-dimensional convolution operator where c in and c out are the number of input and output channels,k,s,and p are the M-dimensional vectors corresponding to kernel size,stride size and padding size respectively.When the size across the M-dimensions are the same,we use a scalar to represent the size e.g.k for k=(k,k,k).Each convolutional middle layer applies3D convolution,BN layer,and ReLU layer sequentially.The convolutional middle layers aggregate voxel-wise features within a pro-gressively expanding receptivefield,adding more context to the shape description.The detailed sizes of thefilters in the convolutional middle layers are explained in Section3.2.1.3Region Proposal NetworkRecently,region proposal networks[32]have become an important building block of top-performing object detec-tion frameworks[38,5,23].In this work,we make several key modifications to the RPN architecture proposed in[32], and combine it with the feature learning network and con-volutional middle layers to form an end-to-end trainable pipeline.The input to our RPN is the feature map provided by the convolutional middle layers.The architecture of this network is illustrated in Figure4.The network has three blocks of fully convolutional layers.Thefirst layer of each block downsamples the feature map by half via a convolu-tion with a stride size of2,followed by a sequence of con-volutions of stride1(×q means q applications of thefilter). After each convolution layer,BN and ReLU operations are applied.We then upsample the output of every block to a fixed size and concatanate to construct the high resolution feature map.Finally,this feature map is mapped to the de-sired learning targets:(1)a probability score map and(2)a regression map.2.2.Loss FunctionLet{a pos i}i=1...N pos be the set of N pos positive an-chors and{a neg j}j=1...N neg be the set of N neg negative anchors.We parameterize a3D ground truth box as (x g c,y g c,z g c,l g,w g,h g,θg),where x g c,y g c,z g c represent the center location,l g,w g,h g are length,width,height of the box,andθg is the yaw rotation around Z-axis.To re-trieve the ground truth box from a matching positive anchor parameterized as(x a c,y a c,z a c,l a,w a,h a,θa),we define the residual vector u∗∈R7containing the7regression tar-gets corresponding to center location∆x,∆y,∆z,three di-Voxel Input Feature BufferVoxel CoordinateBufferK T7Sparse TensorK31Voxel-wise FeatureK C 1Point CloudIndexingMemory CopyS t a c k e d V F EFigure 5.Illustration of efficient implementation.mensions ∆l,∆w,∆h ,and the rotation ∆θ,which are com-puted as:∆x =x g c −x a cd a ,∆y =y g c −y a c d a ,∆z =z gc −z a c h a ,∆l =log(l g l a ),∆w =log(w g w a ),∆h =log(h gh a ),(1)∆θ=θg −θawhere d a =(l a )2+(w a )2is the diagonal of the base of the anchor box.Here,we aim to directly estimate the oriented 3D box and normalize ∆x and ∆y homogeneously with the diagonal d a ,which is different from [32,38,22,21,4,3,5].We define the loss function as follows:L =α1N pos i L cls (p posi ,1)+β1N neg jL cls (p neg j ,0)+1N posiL reg (u i ,u ∗i )(2)where p pos i and p neg j represent the softmax output for posi-tive anchor a posi and negative anchor a neg j respectively,whileu i ∈R 7and u ∗i ∈R 7are the regression output and ground truth for positive anchor a pos i .The first two terms are the normalized classification loss for {a pos i }i =1...N pos and {a negj }j =1...N neg ,where the L cls stands for binary cross en-tropy loss and α,βare postive constants balancing the rel-ative importance.The last term L reg is the regression loss,where we use the SmoothL1function [12,32].2.3.Efficient ImplementationGPUs are optimized for processing dense tensor struc-tures.The problem with working directly with the point cloud is that the points are sparsely distributed across space and each voxel has a variable number of points.We devised a method that converts the point cloud into a dense tensor structure where stacked VFE operations can be processed in parallel across points and voxels.The method is summarized in Figure 5.We initialize aK ×T ×7dimensional tensor structure to store the voxel input feature buffer where K is the maximum number of non-empty voxels,T is the maximum number of points per voxel,and 7is the input encoding dimension for each point.The points are randomized before processing.For each point in the point cloud,we check if the corresponding voxel already exists.This lookup operation is done effi-ciently in O (1)using a hash table where the voxel coordi-nate is used as the hash key.If the voxel is already initial-ized we insert the point to the voxel location if there are less than T points,otherwise the point is ignored.If the voxel is not initialized,we initialize a new voxel,store its coordi-nate in the voxel coordinate buffer,and insert the point to this voxel location.The voxel input feature and coordinate buffers can be constructed via a single pass over the point list,therefore its complexity is O (n ).To further improve the memory/compute efficiency it is possible to only store a limited number of voxels (K )and ignore points coming from voxels with few points.After the voxel input buffer is constructed,the stacked VFE only involves point level and voxel level dense oper-ations which can be computed on a GPU in parallel.Note that,after concatenation operations in VFE,we reset the features corresponding to empty points to zero such that they do not affect the computed voxel features.Finally,using the stored coordinate buffer we reorganize the com-puted sparse voxel-wise structures to the dense voxel grid.The following convolutional middle layers and RPN oper-ations work on a dense voxel grid which can be efficiently implemented on a GPU.3.Training DetailsIn this section,we explain the implementation details of the V oxelNet and the training procedure.work DetailsOur experimental setup is based on the LiDAR specifi-cations of the KITTI dataset [11].Car Detection For this task,we consider point clouds within the range of [−3,1]×[−40,40]×[0,70.4]meters along Z,Y ,X axis respectively.Points that are projected outside of image boundaries are removed [5].We choose a voxel size of v D =0.4,v H =0.2,v W =0.2meters,which leads to D =10,H =400,W =352.We set T =35as the maximum number of randomly sam-pled points in each non-empty voxel.We use two VFE layers VFE-1(7,32)and VFE-2(32,128).The final FCN maps VFE-2output to R 128.Thus our feature learning net generates a sparse tensor of shape 128×10×400×352.To aggregate voxel-wise features,we employ three convo-lution middle layers sequentially as Conv3D(128,64,3,(2,1,1),(1,1,1)),Conv3D(64,64,3,(1,1,1),(0,1,1)),andConv3D(64,64,3,(2,1,1),(1,1,1)),which yields a4D ten-sor of size64×2×400×352.After reshaping,the input to RPN is a feature map of size128×400×352,where the dimensions correspond to channel,height,and width of the3D tensor.Figure4illustrates the detailed network ar-chitecture for this task.Unlike[5],we use only one anchor size,l a=3.9,w a=1.6,h a=1.56meters,centered at z a c=−1.0meters with two rotations,0and90degrees. Our anchor matching criteria is as follows:An anchor is considered as positive if it has the highest Intersection over Union(IoU)with a ground truth or its IoU with ground truth is above0.6(in bird’s eye view).An anchor is considered as negative if the IoU between it and all ground truth boxes is less than0.45.We treat anchors as don’t care if they have 0.45≤IoU≤0.6with any ground truth.We setα=1.5 andβ=1in Eqn.2.Pedestrian and Cyclist Detection The input range1is [−3,1]×[−20,20]×[0,48]meters along Z,Y,X axis re-spectively.We use the same voxel size as for car detection, which yields D=10,H=200,W=240.We set T=45 in order to obtain more LiDAR points for better capturing shape information.The feature learning network and con-volutional middle layers are identical to the networks used in the car detection task.For the RPN,we make one mod-ification to block1in Figure4by changing the stride size in thefirst2D convolution from2to1.This allowsfiner resolution in anchor matching,which is necessary for de-tecting pedestrians and cyclists.We use anchor size l a= 0.8,w a=0.6,h a=1.73meters centered at z a c=−0.6 meters with0and90degrees rotation for pedestrian detec-tion and use anchor size l a=1.76,w a=0.6,h a=1.73 meters centered at z a c=−0.6with0and90degrees rota-tion for cyclist detection.The specific anchor matching cri-teria is as follows:We assign an anchor as postive if it has the highest IoU with a ground truth,or its IoU with ground truth is above0.5.An anchor is considered as negative if its IoU with every ground truth is less than0.35.For anchors having0.35≤IoU≤0.5with any ground truth,we treat them as don’t care.During training,we use stochastic gradient descent (SGD)with learning rate0.01for thefirst150epochs and decrease the learning rate to0.001for the last10epochs. We use a batchsize of16point clouds.3.2.Data AugmentationWith less than4000training point clouds,training our network from scratch will inevitably suffer from overfitting. To reduce this issue,we introduce three different forms of data augmentation.The augmented training data are gener-ated on-the-fly without the need to be stored on disk[20].1Our empirical observation suggests that beyond this range,LiDAR returns from pedestrians and cyclists become very sparse and therefore detection results will be unreliable.Define set M={p i=[x i,y i,z i,r i]T∈R4}i=1,...,N as the whole point cloud,consisting of N points.We parame-terize a3D bouding box b i as(x c,y c,z c,l,w,h,θ),where x c,y c,z c are center locations,l,w,h are length,width, height,andθis the yaw rotation around Z-axis.We de-fineΩi={p|x∈[x c−l/2,x c+l/2],y∈[y c−w/2,y c+ w/2],z∈[z c−h/2,z c+h/2],p∈M}as the set con-taining all LiDAR points within b i,where p=[x,y,z,r] denotes a particular LiDAR point in the whole set M.Thefirst form of data augmentation applies perturbation independently to each ground truth3D bounding box to-gether with those LiDAR points within the box.Specifi-cally,around Z-axis we rotate b i and the associatedΩi with respect to(x c,y c,z c)by a uniformally distributed random variable∆θ∈[−π/10,+π/10].Then we add a translation (∆x,∆y,∆z)to the XYZ components of b i and to each point inΩi,where∆x,∆y,∆z are drawn independently from a Gaussian distribution with mean zero and standard deviation1.0.To avoid physically impossible outcomes,we perform a collision test between any two boxes after the per-turbation and revert to the original if a collision is detected. Since the perturbation is applied to each ground truth box and the associated LiDAR points independently,the net-work is able to learn from substantially more variations than from the original training data.Secondly,we apply global scaling to all ground truth boxes b i and to the whole point cloud M.Specifically, we multiply the XYZ coordinates and the three dimen-sions of each b i,and the XYZ coordinates of all points in M with a random variable drawn from uniform distri-bution[0.95,1.05].Introducing global scale augmentation improves robustness of the network for detecting objects with various sizes and distances as shown in image-based classification[35,18]and detection tasks[12,17].Finally,we apply global rotation to all ground truth boxes b i and to the whole point cloud M.The rotation is applied along Z-axis and around(0,0,0).The global ro-tation offset is determined by sampling from uniform dis-tribution[−π/4,+π/4].By rotating the entire point cloud, we simulate the vehicle making a turn.4.ExperimentsWe evaluate V oxelNet on the KITTI3D object detection benchmark[11]which contains7,481training images/point clouds and7,518test images/point clouds,covering three categories:Car,Pedestrian,and Cyclist.For each class, detection outcomes are evaluated based on three difficulty levels:easy,moderate,and hard,which are determined ac-cording to the object size,occlusion state,and truncation level.Since the ground truth for the test set is not avail-able and the access to the test server is limited,we con-duct comprehensive evaluation using the protocol described in[4,3,5]and subdivide the training data into a training setMethod ModalityCar Pedestrian CyclistEasy Moderate Hard Easy Moderate Hard Easy Moderate HardMono3D[3]Mono 5.22 5.19 4.13N/A N/A N/A N/A N/A N/A 3DOP[4]Stereo12.639.497.59N/A N/A N/A N/A N/A N/A VeloFCN[22]LiDAR40.1432.0830.47N/A N/A N/A N/A N/A N/A MV(BV+FV)[5]LiDAR86.1877.3276.33N/A N/A N/A N/A N/A N/A MV(BV+FV+RGB)[5]LiDAR+Mono86.5578.1076.67N/A N/A N/A N/A N/A N/A HC-baseline LiDAR88.2678.4277.6658.9653.7951.4763.6342.7541.06 V oxelNet LiDAR89.6084.8178.5765.9561.0556.9874.4152.1850.49 Table1.Performance comparison in bird’s eye view detection:average precision(in%)on KITTI validation set.Method ModalityCar Pedestrian CyclistEasy Moderate Hard Easy Moderate Hard Easy Moderate HardMono3D[3]Mono 2.53 2.31 2.31N/A N/A N/A N/A N/A N/A 3DOP[4]Stereo 6.55 5.07 4.10N/A N/A N/A N/A N/A N/A VeloFCN[22]LiDAR15.2013.6615.98N/A N/A N/A N/A N/A N/A MV(BV+FV)[5]LiDAR71.1956.6055.30N/A N/A N/A N/A N/A N/A MV(BV+FV+RGB)[5]LiDAR+Mono71.2962.6856.56N/A N/A N/A N/A N/A N/A HC-baseline LiDAR71.7359.7555.6943.9540.1837.4855.3536.0734.15 V oxelNet LiDAR81.9765.4662.8557.8653.4248.8767.1747.6545.11 Table2.Performance comparison in3D detection:average precision(in%)on KITTI validation set.and a validation set,which results in3,712data samples for training and3,769data samples for validation.The split avoids samples from the same sequence being included in both the training and the validation set[3].Finally we also present the test results using the KITTI server.For the Car category,we compare the proposed method with several top-performing algorithms,including image based approaches:Mono3D[3]and3DOP[4];LiDAR based approaches:VeloFCN[22]and3D-FCN[21];and a multi-modal approach MV[5].Mono3D[3],3DOP[4]and MV[5]use a pre-trained model for initialization whereas we train V oxelNet from scratch using only the LiDAR data provided in KITTI.To analyze the importance of end-to-end learning,we implement a strong baseline that is derived from the V ox-elNet architecture but uses hand-crafted features instead of the proposed feature learning network.We call this model the hand-crafted baseline(HC-baseline).HC-baseline uses the bird’s eye view features described in[5]which are computed at0.1m resolution.Different from[5],we in-crease the number of height channels from4to16to cap-ture more detailed shape information–further increasing the number of height channels did not lead to performance improvement.We replace the convolutional middle lay-ers of V oxelNet with similar size2D convolutional layers, which are Conv2D(16,32,3,1,1),Conv2D(32,64,3,2, 1),Conv2D(64,128,3,1,1).Finally RPN is identical in V oxelNet and HC-baseline.The total number of parame-ters in HC-baseline and V oxelNet are very similar.We train the HC-baseline using the same training procedure and data augmentation described in Section3.4.1.Evaluation on KITTI Validation SetMetrics We follow the official KITTI evaluation protocol, where the IoU threshold is0.7for class Car and is0.5for class Pedestrian and Cyclist.The IoU threshold is the same for both bird’s eye view and full3D evaluation.We compare the methods using the average precision(AP)metric. Evaluation in Bird’s Eye View The evaluation result is presented in Table1.V oxelNet consistently outperforms all the competing approaches across all three difficulty levels. HC-baseline also achieves satisfactory performance com-pared to the state-of-the-art[5],which shows that our base region proposal network(RPN)is effective.For Pedestrian and Cyclist detection tasks in bird’s eye view,we compare the proposed V oxelNet with HC-baseline.V oxelNet yields substantially higher AP than the HC-baseline for these more challenging categories,which shows that end-to-end learn-ing is essential for point-cloud based detection.We would like to note that[21]reported88.9%,77.3%, and72.7%for easy,moderate,and hard levels respectively, but these results are obtained based on a different split of 6,000training frames and∼1,500validation frames,and they are not directly comparable with algorithms in Table1. Therefore,we do not include these results in the table. Evaluation in3D Compared to the bird’s eye view de-tection,which requires only accurate localization of ob-jects in the2D plane,3D detection is a more challeng-ing task as it requiresfiner localization of shapes in3D space.Table2summarizes the comparison.For the class Car,V oxelNet significantly outperforms all other ap-proaches in AP across all difficulty levels.Specifically, using only LiDAR,V oxelNet significantly outperforms the。
- 1、下载文档前请自行甄别文档内容的完整性,平台不提供额外的编辑、内容补充、找答案等附加服务。
- 2、"仅部分预览"的文档,不可在线预览部分如存在完整性等问题,可反馈申请退款(可完整预览的文档不适用该条件!)。
- 3、如文档侵犯您的权益,请联系客服反馈,我们会尽快为您处理(人工客服工作时间:9:00-18:30)。
TITRE DE VOTRE CV - POSTE SOUHAITEMois AA - Mois AA Nom de l'Entreprise - Titre du poste occupé- Description brève d'un point clé- Responsabilité, tâche, réalisation...- Autre responsabilité, autre tâche, autre réalisation...Mois AA - Mois AA Nom de l'Entreprise - Titre du poste occupé- Description brève d'un point clé- Responsabilité, tâche, réalisation...- Autre responsabilité, autre tâche, autre réalisation...Mois AA - Mois AA Nom de l'Entreprise - Titre du poste occupé- Description brève d'un point clé- Responsabilité, tâche, réalisation...- Autre responsabilité, autre tâche, autre réalisation...Début - Fin Titre de votre Formation - Ecole / Université- Spécialisation éventuelle choisieDébut - Fin Titre de votre Formation - Ecole / Université- Spécialisation éventuelle choisieAnglais Courant (TOEIC : 805)Informatique Word, Excel, Internet, Access, PowerPointAssociation Description de votre activité associative / culturelle Sport Description du sport pratiqué, niveauTITRE DE VOTRE CV - POSTE SOUHAITEMois AA - Mois AA Nom de l'Entreprise - Titre du poste occupé- Description brève d'un point clé- Responsabilité, tâche, réalisation...- Autre responsabilité, autre tâche, autre réalisation...Mois AA - Mois AA Nom de l'Entreprise - Titre du poste occupé- Description brève d'un point clé- Responsabilité, tâche, réalisation...- Autre responsabilité, autre tâche, autre réalisation...Mois AA - Mois AA Nom de l'Entreprise - Titre du poste occupé- Description brève d'un point clé- Responsabilité, tâche, réalisation...- Autre responsabilité, autre tâche, autre réalisation...Début - Fin Titre de votre Formation - Ecole / Université- Spécialisation éventuelle choisieDébut - Fin Titre de votre Formation - Ecole / Université- Spécialisation éventuelle choisieAnglais Courant (TOEIC : 805)Informatique Word, Excel, Internet, Access, PowerPointAssociation Description de votre activité associative / culturelle Sport Description du sport pratiqué, niveauTITRE DE VOTRE CV - POSTE SOUHAITEMois AA - Mois AA Nom de l'Entreprise - Titre du poste occupé- Description brève d'un point clé- Responsabilité, tâche, réalisation...- Autre responsabilité, autre tâche, autre réalisation...Mois AA - Mois AA Nom de l'Entreprise - Titre du poste occupé- Description brève d'un point clé- Responsabilité, tâche, réalisation...- Autre responsabilité, autre tâche, autre réalisation...Mois AA - Mois AA Nom de l'Entreprise - Titre du poste occupé- Description brève d'un point clé- Responsabilité, tâche, réalisation...- Autre responsabilité, autre tâche, autre réalisation...Début - Fin Titre de votre Formation - Ecole / Université- Spécialisation éventuelle choisieDébut - Fin Titre de votre Formation - Ecole / Université- Spécialisation éventuelle choisieAnglais Courant (TOEIC : 805)Informatique Word, Excel, Internet, Access, PowerPointAssociation Description de votre activité associative / culturelleSport Description du sport pratiqué, niveauTITRE DE VOTRE CV - POSTE SOUHAITEMois AA - Mois AA Nom de l'Entreprise - Titre du poste occupé- Description brève d'un point cléPoints Clés, Chiffres- Responsabilité, tâche, réalisation...- Autre responsabilité, autre tâche, autre réalisation...Mois AA - Mois AA Nom de l'Entreprise - Titre du poste occupé- Description brève d'un point clé- Responsabilité, tâche, réalisation...Points Clés, Chiffres- Autre responsabilité, autre tâche, autre réalisation...Mois AA - Mois AA Nom de l'Entreprise - Titre du poste occupé- Description brève d'un point clé- Responsabilité, tâche, réalisation...Points Clés, Chiffres- Autre responsabilité, autre tâche, autre réalisation...Début - Fin Titre de votre Formation - Ecole / Université- Spécialisation éventuelle choisieDébut - Fin Titre de votre Formation - Ecole / Université- Spécialisation éventuelle choisieAnglais Courant (TOEIC : 805)Informatique Word, Excel, Internet, Access, PowerPointAssociation Description de votre activité associative / culturelleSport Description du sport pratiqué, niveauTITRE DE VOTRE CV - POSTE SOUHAITEMois AA - Mois AA Nom de l'Entreprise - Titre du poste occupé- Description brève d'un point clé- Responsabilité, tâche, réalisation...- Autre responsabilité, autre tâche, autre réalisation...Mois AA - Mois AA Nom de l'Entreprise - Titre du poste occupé- Description brève d'un point clé- Responsabilité, tâche, réalisation...- Autre responsabilité, autre tâche, autre réalisation...Mois AA - Mois AA Nom de l'Entreprise - Titre du poste occupé- Description brève d'un point clé- Responsabilité, tâche, réalisation...- Autre responsabilité, autre tâche, autre réalisation...Début - Fin Titre de votre Formation - Ecole / Université- Spécialisation éventuelle choisieDébut - Fin Titre de votre Formation - Ecole / Université- Spécialisation éventuelle choisieAnglais Courant (TOEIC : 805)Informatique Word, Excel, Internet, Access, PowerPointAssociation Description de votre activité associative / culturelle Sport Description du sport pratiqué, niveauTITRE DE VOTRE CV - POSTE SOUHAITEMois AA - Mois AA Nom de l'Entreprise - Titre du poste occupé- Description brève d'un point clé- Responsabilité, tâche, réalisation...- Autre responsabilité, autre tâche, autre réalisation...Mois AA - Mois AA Nom de l'Entreprise - Titre du poste occupé- Description brève d'un point clé- Responsabilité, tâche, réalisation...- Autre responsabilité, autre tâche, autre réalisation...Mois AA - Mois AA Nom de l'Entreprise - Titre du poste occupé- Description brève d'un point clé- Responsabilité, tâche, réalisation...- Autre responsabilité, autre tâche, autre réalisation...Début - Fin Titre de votre Formation - Ecole / Université- Spécialisation éventuelle choisieDébut - Fin Titre de votre Formation - Ecole / Université- Spécialisation éventuelle choisieAnglais Courant (TOEIC : 805)Informatique Word, Excel, Internet, Access, PowerPointAssociation Description de votre activité associative / culturelle Sport Description du sport pratiqué, niveauTITRE DE VOTRE CV - POSTE SOUHAITEMois AA - Mois AA Nom de l'Entreprise - Titre du poste occupé- Description brève d'un point clé- Responsabilité, tâche, réalisation...- Autre responsabilité, autre tâche, autre réalisation...Mois AA - Mois AA Nom de l'Entreprise - Titre du poste occupé- Description brève d'un point clé- Responsabilité, tâche, réalisation...- Autre responsabilité, autre tâche, autre réalisation...Mois AA - Mois AA Nom de l'Entreprise - Titre du poste occupé- Description brève d'un point clé- Responsabilité, tâche, réalisation...- Autre responsabilité, autre tâche, autre réalisation...Début - Fin Titre de votre Formation - Ecole / Université- Spécialisation éventuelle choisieDébut - Fin Titre de votre Formation - Ecole / Université- Spécialisation éventuelle choisieAnglais Courant (TOEIC : 805)Informatique Word, Excel, Internet, Access, PowerPointAssociation Description de votre activité associative / culturelleSport Description du sport pratiqué, niveauTITRE DE VOTRE CV - POSTE SOUHAITEMois AA - Mois AA Nom de l'Entreprise - Titre du poste occupé- Description brève d'un point cléPoints Clés, Chiffres- Responsabilité, tâche, réalisation...- Autre responsabilité, autre tâche, autre réalisation...Mois AA - Mois AA Nom de l'Entreprise - Titre du poste occupé- Description brève d'un point clé- Responsabilité, tâche, réalisation...Points Clés, Chiffres- Autre responsabilité, autre tâche, autre réalisation...Mois AA - Mois AA Nom de l'Entreprise - Titre du poste occupé- Description brève d'un point clé- Responsabilité, tâche, réalisation...Points Clés, Chiffres- Autre responsabilité, autre tâche, autre réalisation...Début - Fin Titre de votre Formation - Ecole / Université- Spécialisation éventuelle choisieDébut - Fin Titre de votre Formation - Ecole / Université- Spécialisation éventuelle choisieAnglais Courant (TOEIC : 805)Informatique Word, Excel, Internet, Access, PowerPointAssociation Description de votre activité associative / culturelleSport Description du sport pratiqué, niveauTITRE DE VOTRE CV - POSTE SOUHAITE EXPERIENCE PROFESSIONNELLEMois AA - Mois AA Nom de l'Entreprise - Titre du poste occupé- Description brève d'un point clé- Responsabilité, tâche, réalisation...- Autre responsabilité, autre tâche, autre réalisation... Mois AA - Mois AA Nom de l'Entreprise - Titre du poste occupé- Description brève d'un point clé- Responsabilité, tâche, réalisation...- Autre responsabilité, autre tâche, autre réalisation... Mois AA - Mois AA Nom de l'Entreprise - Titre du poste occupé- Description brève d'un point clé- Responsabilité, tâche, réalisation...- Autre responsabilité, autre tâche, autre réalisation... FORMATIONDébut - Fin Titre de votre Formation - Ecole / Université- Spécialisation éventuelle choisieDébut - Fin Titre de votre Formation - Ecole / Université- Spécialisation éventuelle choisieLANGUES ET INFORMATIQUEAnglais Courant (TOEIC : 805)Informatique Word, Excel, Internet, Access, PowerPoint CENTRES D'INTERETAssociation Description de votre activité associative / culturelle Sport Description du sport pratiqué, niveauTITRE DE VOTRE CV - POSTE SOUHAITE EXPERIENCE PROFESSIONNELLEMois AA - Mois AA Nom de l'Entreprise - Titre du poste occupé- Description brève d'un point clé- Responsabilité, tâche, réalisation...- Autre responsabilité, autre tâche, autre réalisation... Mois AA - Mois AA Nom de l'Entreprise - Titre du poste occupé- Description brève d'un point clé- Responsabilité, tâche, réalisation...- Autre responsabilité, autre tâche, autre réalisation... Mois AA - Mois AA Nom de l'Entreprise - Titre du poste occupé- Description brève d'un point clé- Responsabilité, tâche, réalisation...- Autre responsabilité, autre tâche, autre réalisation... FORMATIONDébut - Fin Titre de votre Formation - Ecole / Université- Spécialisation éventuelle choisieDébut - Fin Titre de votre Formation - Ecole / Université- Spécialisation éventuelle choisieLANGUES ET INFORMATIQUEAnglais Courant (TOEIC : 805)Informatique Word, Excel, Internet, Access, PowerPoint CENTRES D'INTERETAssociation Description de votre activité associative / culturelle Sport Description du sport pratiqué, niveauTITRE DE VOTRE CV - POSTE SOUHAITEEXPERIENCE PROFESSIONNELLEMois AA - Mois AA Nom de l'Entreprise - Titre du poste occupé- Description brève d'un point clé- Responsabilité, tâche, réalisation...- Autre responsabilité, autre tâche, autre réalisation...Mois AA - Mois AA Nom de l'Entreprise - Titre du poste occupé- Description brève d'un point clé- Responsabilité, tâche, réalisation...- Autre responsabilité, autre tâche, autre réalisation...Mois AA - Mois AA Nom de l'Entreprise - Titre du poste occupé- Description brève d'un point clé- Responsabilité, tâche, réalisation...- Autre responsabilité, autre tâche, autre réalisation...FORMATIONDébut - Fin Titre de votre Formation - Ecole / Université- Spécialisation éventuelle choisieDébut - Fin Titre de votre Formation - Ecole / Université- Spécialisation éventuelle choisieLANGUES ET INFORMATIQUEAnglais Courant (TOEIC : 805) Informatique Word, Excel, Internet, Access, PowerPoint CENTRES D'INTERETAssociation Description de votre activité associative / culturelle Sport Description du sport pratiqué, niveauTITRE DE VOTRE CV - POSTE SOUHAITEEXPERIENCE PROFESSIONNELLEMois AA - Mois AA Nom de l'Entreprise - Titre du poste occupé- Description brève d'un point cléPoints Clés, Chiffres- Responsabilité, tâche, réalisation...- Autre responsabilité, autre tâche, autre réalisation...Mois AA - Mois AA Nom de l'Entreprise - Titre du poste occupé- Description brève d'un point clé- Responsabilité, tâche, réalisation...Points Clés, Chiffres- Autre responsabilité, autre tâche, autre réalisation...Mois AA - Mois AA Nom de l'Entreprise - Titre du poste occupé- Description brève d'un point clé- Responsabilité, tâche, réalisation...Points Clés, Chiffres- Autre responsabilité, autre tâche, autre réalisation...FORMATIONDébut - Fin Titre de votre Formation - Ecole / Université- Spécialisation éventuelle choisieDébut - Fin Titre de votre Formation - Ecole / Université- Spécialisation éventuelle choisieLANGUES ET INFORMATIQUEAnglais Courant (TOEIC : 805)Informatique Word, Excel, Internet, Access, PowerPointCENTRES D'INTERETAssociation Description de votre activité associative / culturelleSport Description du sport pratiqué, niveauTITRE DE VOTRE CV - POSTE SOUHAITEEXPERIENCE PROFESSIONNELLE__________________________________________Mois AA - Mois AA Nom de l'Entreprise - Titre du poste occupé- Description brève d'un point clé- Responsabilité, tâche, réalisation...- Autre responsabilité, autre tâche, autre réalisation...Mois AA - Mois AA Nom de l'Entreprise - Titre du poste occupé- Description brève d'un point clé- Responsabilité, tâche, réalisation...- Autre responsabilité, autre tâche, autre réalisation...Mois AA - Mois AA Nom de l'Entreprise - Titre du poste occupé- Description brève d'un point clé- Responsabilité, tâche, réalisation...- Autre responsabilité, autre tâche, autre réalisation...FORMATION_________________________________________________________Début - Fin Titre de votre Formation - Ecole / Université- Spécialisation éventuelle choisieDébut - Fin Titre de votre Formation - Ecole / Université- Spécialisation éventuelle choisieLANGUES ET INFORMATIQUE____________________________________________ Anglais Courant (TOEIC : 805)Informatique Word, Excel, Internet, Access, PowerPointCENTRES D'INTERET___________________________________________________ Association Description de votre activité associative / culturelleSport Description du sport pratiqué, niveauTITRE DE VOTRE CV - POSTE SOUHAITEEXPERIENCE PROFESSIONNELLE__________________________________________Mois AA - Mois AA Nom de l'Entreprise - Titre du poste occupé- Description brève d'un point clé- Responsabilité, tâche, réalisation...- Autre responsabilité, autre tâche, autre réalisation...Mois AA - Mois AA Nom de l'Entreprise - Titre du poste occupé- Description brève d'un point clé- Responsabilité, tâche, réalisation...- Autre responsabilité, autre tâche, autre réalisation...Mois AA - Mois AA Nom de l'Entreprise - Titre du poste occupé- Description brève d'un point clé- Responsabilité, tâche, réalisation...- Autre responsabilité, autre tâche, autre réalisation...FORMATION_________________________________________________________Début - Fin Titre de votre Formation - Ecole / Université- Spécialisation éventuelle choisieDébut - Fin Titre de votre Formation - Ecole / Université- Spécialisation éventuelle choisieLANGUES ET INFORMATIQUE____________________________________________ Anglais Courant (TOEIC : 805)Informatique Word, Excel, Internet, Access, PowerPointCENTRES D'INTERET___________________________________________________ Association Description de votre activité associative / culturelleSport Description du sport pratiqué, niveauTITRE DE VOTRE CV - POSTE SOUHAITEEXPERIENCE PROFESSIONNELLE__________________________________________Mois AA - Mois AA Nom de l'Entreprise - Titre du poste occupé- Description brève d'un point clé- Responsabilité, tâche, réalisation...- Autre responsabilité, autre tâche, autre réalisation...Mois AA - Mois AA Nom de l'Entreprise - Titre du poste occupé- Description brève d'un point clé- Responsabilité, tâche, réalisation...- Autre responsabilité, autre tâche, autre réalisation...Mois AA - Mois AA Nom de l'Entreprise - Titre du poste occupé- Description brève d'un point clé- Responsabilité, tâche, réalisation...- Autre responsabilité, autre tâche, autre réalisation...FORMATION_________________________________________________________ Début - Fin Titre de votre Formation - Ecole / Université- Spécialisation éventuelle choisieDébut - Fin Titre de votre Formation - Ecole / Université- Spécialisation éventuelle choisieLANGUES ET INFORMATIQUE____________________________________________ Anglais Courant (TOEIC : 805) Informatique Word, Excel, Internet, Access, PowerPoint CENTRES D'INTERET___________________________________________________ Association Description de votre activité associative / culturelle Sport Description du sport pratiqué, niveauTITRE DE VOTRE CV - POSTE SOUHAITEEXPERIENCE PROFESSIONNELLE__________________________________________Mois AA - Mois AA Nom de l'Entreprise - Titre du poste occupé- Description brève d'un point cléPoints Clés, Chiffres- Responsabilité, tâche, réalisation...- Autre responsabilité, autre tâche, autre réalisation...Mois AA - Mois AA Nom de l'Entreprise - Titre du poste occupé- Description brève d'un point clé- Responsabilité, tâche, réalisation...Points Clés, Chiffres- Autre responsabilité, autre tâche, autre réalisation...Mois AA - Mois AA Nom de l'Entreprise - Titre du poste occupé- Description brève d'un point clé- Responsabilité, tâche, réalisation...Points Clés, Chiffres- Autre responsabilité, autre tâche, autre réalisation...FORMATION_________________________________________________________Début - Fin Titre de votre Formation - Ecole / Université- Spécialisation éventuelle choisieDébut - Fin Titre de votre Formation - Ecole / Université- Spécialisation éventuelle choisieLANGUES ET INFORMATIQUE____________________________________________ Anglais Courant (TOEIC : 805)Informatique Word, Excel, Internet, Access, PowerPointCENTRES D'INTERET___________________________________________________ Association Description de votre activité associative / culturelleSport Description du sport pratiqué, niveauTITRE DE VOTRE CV - POSTE SOUHAITEEXPERIENCE PROFESSIONNELLE Nom de l'Entreprise Mois AA - Mois AA Titre du poste occupé- Description brève d'un point clé- Responsabilité, tâche, réalisation...- Autre responsabilité, autre tâche, autre réalisation...Nom de l'Entreprise Mois AA - Mois AA Titre du poste occupé- Description brève d'un point clé- Responsabilité, tâche, réalisation...- Autre responsabilité, autre tâche, autre réalisation...Nom de l'Entreprise Mois AA - Mois AA Titre du poste occupé- Description brève d'un point clé- Responsabilité, tâche, réalisation...- Autre responsabilité, autre tâche, autre réalisation...FORMATION Ecole / UniversitéMois AA - Mois AATitre de votre Formation- Spécialisation éventuelle choisieEcole / UniversitéMois AA - Mois AATitre de votre Formation- Spécialisation éventuelle choisieLANGUES INFORMATIQUE Anglais Courant (TOEIC : 805)Informatique Word, Excel, Internet, Access, PowerPointCENTRE D'INTERET Association Description de votre activité culturelleSport Description du sport pratiqué, niveauTITRE DE VOTRE CV - POSTE SOUHAITENom de l'Entreprise Mois AA - Mois AA Titre du poste occupé- Description brève d'un point clé - Responsabilité, tâche, réalisation...- Autre responsabilité, autre tâche, autre réalisation...Nom de l'Entreprise Mois AA - Mois AA Titre du poste occupé- Description brève d'un point clé - Responsabilité, tâche, réalisation...- Autre responsabilité, autre tâche, autre réalisation...Nom de l'Entreprise Mois AA - Mois AA Titre du poste occupé- Description brève d'un point clé - Responsabilité, tâche, réalisation...- Autre responsabilité, autre tâche, autre réalisation...Ecole / Université Mois AA - Mois AATitre de votre Formation- Spécialisation éventuelle choisieEcole / Université Mois AA - Mois AATitre de votre Formation- Spécialisation éventuelle choisieAnglais Courant (TOEIC : 805)InformatiqueWord, Excel, Internet, Access, PowerPointAssociation Description de votre activité culturelle SportDescription du sport pratiqué, niveau。