integrate-and-fire neurons fi
视网膜功能启发的边缘检测层级模型
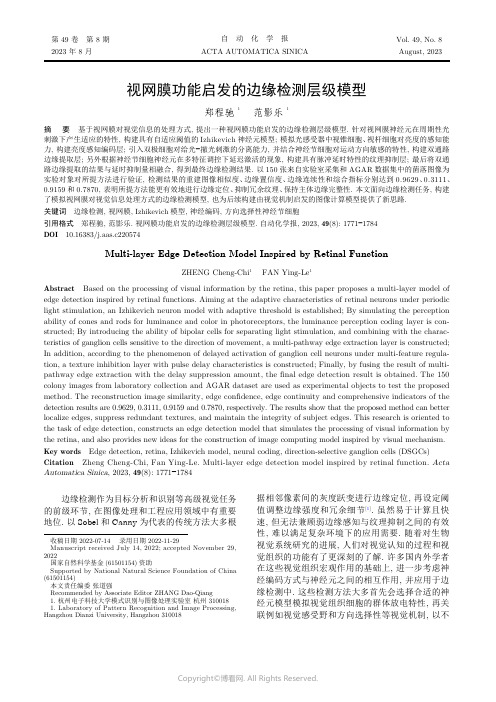
视网膜功能启发的边缘检测层级模型郑程驰 1范影乐1摘 要 基于视网膜对视觉信息的处理方式, 提出一种视网膜功能启发的边缘检测层级模型. 针对视网膜神经元在周期性光刺激下产生适应的特性, 构建具有自适应阈值的Izhikevich 神经元模型; 模拟光感受器中视锥细胞、视杆细胞对亮度的感知能力, 构建亮度感知编码层; 引入双极细胞对给光−撤光刺激的分离能力, 并结合神经节细胞对运动方向敏感的特性, 构建双通路边缘提取层; 另外根据神经节细胞神经元在多特征调控下延迟激活的现象, 构建具有脉冲延时特性的纹理抑制层; 最后将双通路边缘提取的结果与延时抑制量相融合, 得到最终边缘检测结果. 以150张来自实验室采集和AGAR 数据集中的菌落图像为实验对象对所提方法进行验证, 检测结果的重建图像相似度、边缘置信度、边缘连续性和综合指标分别达到0.9629、0.3111、0.9159和0.7870, 表明所提方法能更有效地进行边缘定位、抑制冗余纹理、保持主体边缘完整性. 本文面向边缘检测任务, 构建了模拟视网膜对视觉信息处理方式的边缘检测模型, 也为后续构建由视觉机制启发的图像计算模型提供了新思路.关键词 边缘检测, 视网膜, Izhikevich 模型, 神经编码, 方向选择性神经节细胞引用格式 郑程驰, 范影乐. 视网膜功能启发的边缘检测层级模型. 自动化学报, 2023, 49(8): 1771−1784DOI 10.16383/j.aas.c220574Multi-layer Edge Detection Model Inspired by Retinal FunctionZHENG Cheng-Chi 1 FAN Ying-Le 1Abstract Based on the processing of visual information by the retina, this paper proposes a multi-layer model of edge detection inspired by retinal functions. Aiming at the adaptive characteristics of retinal neurons under periodic light stimulation, an Izhikevich neuron model with adaptive threshold is established; By simulating the perception ability of cones and rods for luminance and color in photoreceptors, the luminance perception coding layer is con-structed; By introducing the ability of bipolar cells for separating light stimulation, and combining with the charac-teristics of ganglion cells sensitive to the direction of movement, a multi-pathway edge extraction layer is constructed;In addition, according to the phenomenon of delayed activation of ganglion cell neurons under multi-feature regula-tion, a texture inhibition layer with pulse delay characteristics is constructed; Finally, by fusing the result of multi-pathway edge extraction with the delay suppression amount, the final edge detection result is obtained. The 150colony images from laboratory collection and AGAR dataset are used as experimental objects to test the proposed method. The reconstruction image similarity, edge confidence, edge continuity and comprehensive indicators of the detection results are 0.9629, 0.3111, 0.9159 and 0.7870, respectively. The results show that the proposed method can better localize edges, suppress redundant textures, and maintain the integrity of subject edges. This research is oriented to the task of edge detection, constructs an edge detection model that simulates the processing of visual information by the retina, and also provides new ideas for the construction of image computing model inspired by visual mechanism.Key words Edge detection, retina, Izhikevich model, neural coding, direction-selective ganglion cells (DSGCs)Citation Zheng Cheng-Chi, Fan Ying-Le. Multi-layer edge detection model inspired by retinal function. Acta Automatica Sinica , 2023, 49(8): 1771−1784边缘检测作为目标分析和识别等高级视觉任务的前级环节, 在图像处理和工程应用领域中有重要地位. 以Sobel 和Canny 为代表的传统方法大多根据相邻像素间的灰度跃变进行边缘定位, 再设定阈值调整边缘强度和冗余细节[1]. 虽然易于计算且快速, 但无法兼顾弱边缘感知与纹理抑制之间的有效性, 难以满足复杂环境下的应用需要. 随着对生物视觉系统研究的进展, 人们对视觉认知的过程和视觉组织的功能有了更深刻的了解. 许多国内外学者在这些视觉组织宏观作用的基础上, 进一步考虑神经编码方式与神经元之间的相互作用, 并应用于边缘检测中. 这些检测方法大多首先会选择合适的神经元模型模拟视觉组织细胞的群体放电特性, 再关联例如视觉感受野和方向选择性等视觉机制, 以不收稿日期 2022-07-14 录用日期 2022-11-29Manuscript received July 14, 2022; accepted November 29,2022国家自然科学基金(61501154)资助Supported by National Natural Science Foundation of China (61501154)本文责任编委 张道强Recommended by Associate Editor ZHANG Dao-Qiang1. 杭州电子科技大学模式识别与图像处理实验室 杭州 3100181. Laboratory of Pattern Recognition and Image Processing,Hangzhou Dianzi University, Hangzhou 310018第 49 卷 第 8 期自 动 化 学 报Vol. 49, No. 82023 年 8 月ACTA AUTOMATICA SINICAAugust, 2023同的编码方式将输入的图像转化为脉冲信号, 经过多级功能区块处理和传递后提取出图像的边缘. 其中, 频率编码和时间编码是视觉系统编码光刺激的重要方式, 在一些计算模型中被广泛使用. 例如,文献[2]以HH (Hodgkin-Huxley)神经元模型为基础, 使用多方向Gabor滤波器模拟神经元感受野的方向选择性, 实现神经元间连接强度关联边缘方向,将每个神经元的脉冲发放频率作为边缘检测的结果输出, 实验结果表明其比传统方法更有效; 文献[3]在 LIF (Leaky integrate-and-fire) 神经元模型的基础上进行改进, 引入根据神经元响应对外界输入进行调整的权值, 在编码的过程中将空间的脉冲发放转化为时序上的激励强度, 实现强弱边缘分类, 对梯度变化幅度小的弱边缘具有良好的检测能力. 除此之外, 也有关注神经元突触间的相互作用, 通过引入使突触的连接权值产生自适应调节的机制来提取边缘信息的计算方法. 例如, 文献 [4] 构建具有STDP (Spike-timing-dependent plasticity) 性质的神经元模型, 根据突触前后神经元首次脉冲发放时间顺序来增强或减弱突触连接, 对真伪边缘具有较强的辨别能力; 文献 [5] 则在构建神经元模型时考虑了具有时间不对称性的STDP机制, 再融合方向特征和侧抑制机制重建图像的主要边缘信息, 其计算过程对神经元突触间的动态特性描述更加准确.更进一步, 神经编码也被应用于实际的工程需要.例如, 文献 [6]针对现有的红外图像边缘检测算法中存在的缺陷, 构建一种新式的脉冲神经网络, 增强了对红外图像中弱边缘的感知; 文献 [7] 则通过模拟视皮层的处理机制, 使用包含左侧、右侧和前向3条并行处理支路的脉冲神经网络模型提取脑核磁共振图像的边缘, 并将提取的结果用于异常检测,同样具有较好的效果. 上述方法都在一定程度上考虑了视觉组织中神经元的编码特性以及视觉机制,与传统方法相比, 在对复杂环境的适应性更强的同时也有较高的计算效率. 但这些方法都未能考虑到神经元自身也会随着外界刺激产生适应, 从而使活动特性发生改变. 此外, 上述方法大多也只选择了频率编码、时间编码等编码方式中的一种, 并不能完整地体现视觉组织中多种编码方式的共同作用.事实上, 在对神经生理实验和理论的持续探索中发现, 视觉组织(以视网膜为例)在对视觉刺激的加工中就存在着丰富的动态特性和编码机制[8−9]. 视网膜作为视觉系统中的初级组织结构, 由多种不同类型的细胞构成, 共同组成一个纵横相连、具有层级结构的复杂网络, 能够针对不同类型的刺激性选择相应的编码方式进行有效处理. 因此, 本文面向图像的边缘检测任务, 以菌落图像处理为例, 模拟视网膜中各成分对视觉信息的处理方式, 构建基于视网膜动态编码机制的多层边缘检测模型, 以适应具有多种形态结构差异的菌落图像边缘检测任务.1 材料和方法本文提出的算法流程如图1所示. 首先, 根据视网膜神经元在周期性光刺激下脉冲发放频率发生改变的特性, 构建具有自适应阈值特性的Izhikevich 神经元模型, 改善神经元的同步发放能力; 其次, 考虑光感受器对强弱光和颜色信息的不同处理方式编码亮度信息, 实现不同亮度水平目标与背景的区分;然后, 引入固视微动机制, 结合神经节细胞的方向选择性和给光−撤光通路的传递特性, 将首发脉冲时间编码的结果作为双通路的初级边缘响应输出;随后, 模拟神经节细胞的延迟发放特性, 融入对比度和突触前后偏好方向差异, 计算各神经元的延时抑制量, 对双通路的计算结果进行纹理抑制; 最后,整合双通路边缘信息, 将二者融合为最终的边缘检测结果.1.1 亮度感知编码层构建神经元模型时, 本文综合考虑对神经元生理特性模拟的合理性和进行仿真计算的高效性, 以Izhikevich模型[10]为基础构建神经元模型. Izhike-vich模型由Izhikevich在HH模型的基础上简化而来, 在保留原模型对神经元放电模式描述的准确性的同时, 也具有较低的时间复杂度, 适合神经元群体计算时应用, 其表达式如下式所示v thv th 其中, v为神经元的膜电位, 其初始值设置为 −70; u为细胞膜恢复变量, 设置为14; I为接收的图像亮度刺激; 为神经元脉冲发放的阈值, 设置为30; a描述恢复变量u的时间尺度, b描述恢复变量u 对膜电位在阈值下波动的敏感性, c和d分别描述产生脉冲发放后膜电位v的重置值和恢复变量u的增加程度, a, b, c, d这4个模型参数的典型值分别为0.02、0.2、−65和6. 若某时刻膜电位v达到,则进行一次脉冲发放, 同时该神经元对应的v被重置为c, u被重置为u + d.适应是神经系统中广泛存在的现象, 具体表现为神经元会根据外界的刺激不断地调节自身的性质. 其中, 视网膜能够适应昼夜环境中万亿倍范围的光照变化, 这种适应能够帮助其在避免饱和的同时保持对光照的敏感性[11]. 研究表明, 视网膜持续1772自 动 化 学 报49 卷接受外界周期性光刺激时, 光感受器会使神经元细胞的活动特性发生改变, 导致单个神经元的发放阈值上升, 放电频率下降; 没有脉冲发放时, 对应阈值又会以指数形式衰减, 同时放电频率逐渐恢复[12].因此, 本文在Izhikevich 模型的基础上作出改进,加入根据脉冲发放频率对阈值进行自适应调节的机制, 如下式所示τ1τ2τ1τ2v th τ1v th τ2其中, 和 分别为脉冲发放和未发放时阈值变化的时间常数, 其值越小, 阈值变化的幅度越大, 神经元敏感性变化的过程越快; 反之, 则表示阈值变化的幅度越小, 神经元敏感性变化的过程也就越慢.生理学实验表明, 在外界持续光刺激下, 神经元对刺激产生适应导致放电频率降低后, 这种适应衰退的过程比产生适应的过程通常要长数倍[13]. 因此,为了在准确模拟生理特性的同时保证计算模型的性能, 本文将 和 分别设置为20和40. 这样, 当某时刻某个神经元产生脉冲发放时, 则对应阈值 根据 的值升高, 神经元产生适应, 活跃度降低; 反之, 对应阈值 根据 的值下降, 神经元的适应衰退, 活跃度提升. 实现限制活跃神经元的脉冲发放频率, 促进不活跃神经元的脉冲发放, 改善神经元群体的同步发放能力, 减少检测目标内部冗余. 图2边缘检测结果图 1 边缘检测算法原理图Fig. 1 Principle of edge detection algorithm8 期郑程驰等: 视网膜功能启发的边缘检测层级模型1773显示了改进前后的Izhikevich 模型对图像进行处理后目标内部冗余情况.0∼255为了规范检测目标图像的亮度范围, 本文将输入的彩色图像Img 各通路的亮度映射到 区间内, 如下式所示Img (;i )I (;i )其中, 和 表示经亮度映射前和映射后的R 、G 、B 三种颜色分量图像; max(·) 和min(·)分别计算对应分量图像中的最大和最小像素值.光感受器分两类, 分别为视锥细胞和视杆细胞[14], 都能将接收到的视觉刺激转化为电信号, 实现信息的编码和传递. 其中, 视锥细胞能够根据外界光刺激的波长来分解为三个不同的颜色通道[15].考虑到人眼对颜色信息的敏感性能有效区分离散目标与背景, 令图像中的每个像素点对应一个神经元,将R 、G 、B 三种颜色分量图像分别输入上文构建的神经元模型中, 在一定时间范围内进行脉冲发放,如下式所示fires (x,y ;i )其中, 为每个神经元的脉冲发放次数,函数Izhikevich(·)表示式(2)给出的神经元模型.视杆细胞对光线敏感, 主要负责弱光环境下的外界刺激感知. 当光刺激足够强时, 视杆细胞的感知能力达到饱和, 视觉系统转为使用视锥细胞负责亮度信息的处理[16]. 因此, 除了对颜色信息敏感外,视锥细胞对强光也有高度辨别能力. 考虑到作为检测对象的图像中, 目标与背景具有不同的亮度水平,本文构建一种综合视锥细胞和视杆细胞亮度感知能力的编码方法, 以适应目标与背景不同亮度对比的多种情况, 如下式所示I base I base (x,y )fires Res (x,y )其中, var(·) 计算图像亮度方差; ave(·) 计算图像亮度均值. 本文取三种颜色分量图像中方差最大的一幅作为基准图像 , 对于其中的像素值 ,将其中亮度低于平均亮度的部分设置为三种颜色分量脉冲发放结果的最小值, 反之设置为最大值, 最终得到模型的亮度编码结果 , 实现在图像局部亮度相对较低的区域由视杆细胞进行弱光感知, 亮度较高区域由视锥细胞处理, 强化计算模型对不同亮度目标和背景的区分能力, 凸显具有弱边缘的对象. 图3显示了亮度感知编码对存在弱边缘的对象的感知能力.1.2 基于固视微动的多方向双通路边缘提取层Img gray 人眼注视目标时, 接收的图像并非是静止的,而是眼球以每秒2至3次的微动使投射在视网膜上的图像发生持续运动, 不断地改变照射在光感受器上的光刺激[17]. 本文考虑人眼的固视微动机制,在原图像的灰度图像 上构建大小为3×3的微动作用窗口temp , 使窗口接收到的亮度信息朝8个方向进行微动, 如下式所示p i q i θi temp θi d x d y 其中, 和 是用于决定微动方向 的参数, 其值被设置为 −1、0或1, 通过计算反正切函数能够得到以45° 为单位、从0° 到315° 的8个角度的微动方向, 对应8个微动结果窗口 ; 和 分别表示水平和竖直方向的微动尺度; Dir 为计算得到(a) 原图(a) Original image (b) Izhikevich 模型(b) Izhikevich model (c) 改进的 Izhikevich 模型(c) Improved Izhikevich model图 2 改进前后的Izhikevich 模型对图像进行脉冲发放的结果对比图Fig. 2 Comparison of the image processing results of the Izhikevich model before and after improvement1774自 动 化 学 报49 卷Dir (x,y )的微动方向矩阵, 其中每个像素点的值为 ;sum(·) 计算窗口中像素值的和. 本文取每个微动窗口前后差异最大的方向作为该点的偏好方向, 分别用数字1 ~ 8表示.视网膜存在一类负责对运动刺激编码、具有方向选择性的神经节细胞 (Direction-selective gangli-on cells, DSGCs)[18]. 经过光感受器处理, 转化为电信号的视觉信息, 通过双极细胞处理后传递给神经节细胞. 双极细胞可分为由光照增强 (ON) 激发的细胞和由光照减弱 (OFF) 激发的细胞[19], 分别将信号输入给光通路 (ON-pathway)和撤光通路 (OFF-pathways) 两条并行通路[20], 传递给光运动和撤光运动产生的刺激. 而神经节细胞同样包括ON 和OFF 两种, 会对给光和撤光所产生的运动方向做出反应[21]. 因此, 本文构造5×5大小的对特定方向微动敏感的神经节细胞感受野窗口, 将其对偏好方向和反方向微动所产生的响应分别作为给光通路和撤光通路的输入. 以偏好方向为45° 的方向选择性神θi fires Res S xy ∗通过上述定义, 可以形成以45° 为单位、从0°到315° 的8个方向的感受野窗口, 与上文 的8个方向对应. 之后本文在亮度编码结果 上构筑与感受野相同大小的局部窗口 , 根据最优方向矩阵Dir 对应窗口中心点的方向, 取与其相同和相反方向的感受野窗口和亮度编码结果进行卷积运算 (本文用符号 表示卷积运算), 分别作为ON 和OFF 通道的输入, 如下式所示T ON T OFF 考虑到眼球微动能够将静止的空间场景转变为视网膜上的时间信息流, 激活视网膜神经元的发放,同时ON 和OFF 两通路也只在光刺激的呈现和撤去的瞬时产生电位发放, 因此本文采用首发脉冲时间作为编码方式, 将 和 定义为两通路首次脉冲发放时间构成的时间矩阵, 并作为初级边缘响应的结果. 将1个单位的发放时间设置为0.25, 当总发放时间大于30时停止计算, 此时还未进行发放的神经元即被判断为非边缘.1.3 多特征脉冲延时纹理抑制层视网膜神经节细胞在对光刺激编码的过程中,外界刺激特征的变化会显著影响神经元的反应时间. 研究发现, 当刺激对比度增大时, 神经元反应延时会减小, 更快速地进行脉冲发放; 反之, 则反应延时增大, 抑制神经元的活性[22]. 除此之外, 方向差异也会影响神经元活动, 突触前后偏好方向相似的神经元更倾向于优先连接, 在受到外界刺激时能够更快被同步激活[23]. 因此, 本文引入视网膜的神经元延时发放机制, 考虑方向和对比度对神经元敏感性的影响, 构造脉冲延时抑制模型. 首先结合局部窗口权重函数计算图像对比度, 如下式所示ω(x i ,y i )其中, 为窗口权重函数, L 为亮度图像, Con(a) 原图(a) Original image (b) Izhikevich 模型(b) Izhikevich model (c) 改进的 Izhikevich 模型(c) Improved Izhikevich model (d) 亮度感知编码(d) Luminance perception coding图 3 不同方式对存在弱边缘的菌落图像的处理结果Fig. 3 Different ways to process the image of colonies with weak edges8 期郑程驰等: 视网膜功能启发的边缘检测层级模型1775S xy x i y i µ=∑x i ,y i ∈S xy ω(x i ,y i )为对比度图像, 为以(x , y )为中心的局部窗口,( , ) 为方窗中除中心外的周边像素, ws 为局部方窗的窗长, . 之后考虑局部方窗中心神经元和周边神经元方向差异, 同时用高斯函数模拟对比度大小与延时作用强度之间的关系, 构建脉冲延时抑制模型, 如下式所示D Dir (x,y )D Con (x,y )D (x,y )∆Dir (x i ,y i )min {|θ(x i ,y i )−θ(x,y )|,2π−|θ(x i ,y i )−θ(x,y )|}δ其中, 和 分别表示方向延时抑制量和对比度延时抑制量; 为计算得到的综合延时抑制量; 为突触前后神经元微动方向的差异, 被定义为 ; 用于调节对比度延时抑制量.T ON T OFFRes ON Res OFF 将上文计算得到的两个时间矩阵 和 中进行过脉冲发放的神经元与综合延时抑制量相加, 同样设置1个单位的发放时间为0.25, 将经延时作用后总发放时间大于30的神经元设置为不发放, 即判定为非边缘, 反之则判定为边缘. 根据式(19)和式(20) 得到两通道边缘检测结果 和. 最后, 将两通道得到的结果融合, 得到最终边缘响应结果Res ,如下式所示2 算法流程基于视网膜对视觉信息的处理顺序和编码特性, 本文构建图4所示的算法流程, 具体步骤如下:1) 根据视网膜在外界持续周期性光刺激下产生的适应现象, 在式(1)所示的Izhikevich 模型上作出改进, 构建如式(2)所示的具有自适应阈值的Izhikevich 模型.2) 根据式(3)将作为检测目标的图像映射到0 ~ 255区间规范亮度范围, 接着分离3种通道的颜色分量, 根据式(4)输入到改进的Izhikevich 模型中进行脉冲发放.3) 根据式(5)的方差计算提取出基准图像, 再结合基准图像根据式(6)对三通道脉冲发放的结果进行亮度感知编码, 得到亮度编码结果.4) 考虑人眼的固视微动机制, 根据式(7)和式(8)通过原图的灰度图像提取每个神经元的偏好方向, 得到微动方向矩阵, 接着根据式(9)和式(10)构筑8个方向的方向选择性神经节细胞感受野窗口.5) 根据式(11)和式(12), 将感受野窗口与亮度编码图像作卷积运算, 并输入Izhikevich 模型中得到ON 和OFF 通路的首发脉冲时间矩阵, 作为两通道的初级边缘响应.6) 根据式(13) ~ 式 (15), 结合局部窗口权重计算图像对比度.7) 考虑对比度和突触前后偏好方向对脉冲发放的延时作用, 根据式(16) ~ 式 (18)构建延时纹理抑制模型, 并根据式(19)和式(20)将纹理抑制模型和两通道的初级边缘响应相融合.8) 根据式(21)将两通路纹理抑制后的结果在神经节细胞处进行整合, 得到最终边缘响应结果.3 结果为了验证本文方法用于菌落边缘检测的有效性, 本文选择Canny 方法和其他3种同样基于神经元编码的边缘检测方法作为横向对比, 并进行定性、定量分析. 首先, 选择文献[4]提出的基于神经元突触可塑性的边缘检测方法(Synaptic plasticity model, SPM), 用于对比本文方法对弱边缘的增强效果; 其次, 选择文献[24]提出的基于抑制性突触的多层神经元群放电编码的边缘检测方法 (Inhibit-ory synapse model, ISM), 验证本文的延时抑制层在抑制冗余纹理方面的有效性; 然后, 选择文献[25]提出的基于突触连接视通路方向敏感的分级边缘检测方法(Orientation sensitivity model, OSM), 对比本文方法在抑制冗余纹理的同时保持边缘提取完整性上的优势; 最后, 还以本文方法为基础, 选择去除亮度感知编码后的方法(No luminance coding,NLC)作为消融实验, 以验证本文方法模拟光感受器功能的亮度感知编码模块的有效性.本文使用实验室在微生物学实验中采集的菌落图像和AGAR 数据集[26]作为实验对象. 前者具有丰富的颜色和形态结构, 用于检验算法对复杂检测环境的适应性; 后者则存在更多层次强度的边缘信息, 菌落本身与背景的颜色和亮度水平也较为相近,用于检测算法对颜色、亮度特征和弱边缘的敏感性.本文通过局部采样生成150张512×512像素大小的测试图像, 其中38张来自实验室采集, 112张来自AGAR 数据集. 然后分别使用上文的6种边缘1776自 动 化 学 报49 卷检测算法提取图像边缘, 使每种算法得到150张边缘检测结果, 其中部分检测结果如图5所示.定性分析图5可知, Canny 、SPM 和ISM 方法在Colony4和Colony5等存在弱边缘的图像中往往会出现大面积的边缘丢失. OSM 方法对弱边缘的敏感性强于以上3种方法, 但仍然会出现不同程度的边缘断裂, 且在调整阈值时难以均衡边缘连续性和目标菌落内部冗余. NLC 方法同样丢失了Colony4和Colony5中几乎所有的边缘, 对于Colony3也只能检出其中亮度较低的菌落内部, 对于梯度变化不明显的边缘辨别力差. 与其他方法相比, 本文方法检出的边缘更加显著且完整性更高, 对于弱边缘也有很强的检测能力, 在Colony3、Colony4和Colony5等存在多层次水平强弱边缘的菌落图像中能够取得较好的检测结果. 为了对检测结果进行定量分析并客观评价各方法的优劣, 计算边缘图像重建相似度MSSIM [27]对检测结果进行重建, 并计算重建图像与原图像的相似度作为边缘定位的准确性RGfires (R)fires (G)亮度编码结果Luminance codingresult方差计算Variance1 2 3ON-result对比度Contrast脉冲延时抑制量Neuron spiking delay感受野窗口感受野窗口DSGC templateOFF-通路输出OFF-result 5)6)7)图 4 边缘检测算法流程图Fig. 4 The procedure of edge detection algorithm8 期郑程驰等: 视网膜功能启发的边缘检测层级模型1777图 5 Colony1 ~ Colony5的边缘检测结果(第1行为原图; 第2行为Canny 检测的结果; 第3行为SPM 检测的结果; 第4行为ISM 检测的结果; 第5行为OSM 检测的结果; 第6行为NLC 检测的结果; 第7行为本文方法检测的结果)Fig. 5 Edge detection results of Colony1 to Colony5 (The first line is original images; The second line is the results of Canny; The third line is the results of SPM; The fourth line is the results of ISM; The fifth line is the results of OSM;The sixth line is the results of NLC; The seventh line is the results of the proposed method)1778自 动 化 学 报49 卷指标. 首先对检测出的边缘图像做膨胀处理, 之后将原图像上的像素值赋给膨胀后边缘的对应位置,得到的图像记为ET , 则边缘重建如下式所示T k ET d k 其中, 为图像 上3×3窗口中8个方向的周边像素, 为窗口中心像素点与周边像素的距离, 计算得到重建图像R . 重建图像的相似度指标如下式所示µA µB σA σB σAB 其中, 和 为原图像和重建图像的灰度均值, 和 为其各自的标准差, 为原图像与重建图像之间的协方差. 将原图像和重建图像各自分为N 个子图, 并分别计算相似度指标SSIM , 得到平均相似度指标MSSIM . 除此之外, 为了验证边缘检测方法检出边缘的真实性和对菌落内部冗余纹理的抑制能力, 本文计算边缘置信度BIdx [28], 根据边缘两侧灰度值的跃变程度判断边缘的真伪. 边缘置信度指标如下式所示σij E (x i k ,y ik )(x i ,y i )d i其中, 为边缘像素在原图像对应位置的邻域标准差, EdgeNum 为边缘像素数量. 另外, 本文进一步计算边缘连续性 CIdx [29]来验证检出目标的边缘完整性. 首先将得到的边缘图像E 分割为m 个区域, 分别计算每个区域中的边缘像素 到其空间中心 的距离 ,则连续性指标如下式所示c i k C i n i 其中, 为边缘连续性的贡献值, D 为阈值, 为第i 个区域的像素点的连续性贡献值之和,为第i 个区域边缘像素点数量. 最后, 将计算得到的3个指标根据下式融合, 得到综合评价指标EIdx [21]其中, row 和col 分别为原图像的行数和列数. 于是, 检测图像的各项性能指标如表1 ~ 表5所示, 图像重建的结果如图6所示.表 1 不同检测方法下的重建相似度MSSIM Table 1 MSSIM of different methodsSerial number MSSIMCanny SPMISMOSMNLC本文方法Colony10.74520.77250.83570.92650.91750.9371Colony20.79510.79710.84900.95280.94470.9725Colony30.85760.86620.83140.91490.83370.9278Colony40.96900.98270.98380.98870.98930.9972Colony50.96340.97580.97800.97710.98830.9933表 2 不同检测方法下的边缘置信度BIdx Table 2 BIdx of different methodsSerial number BIdxCanny SPMISMOSMNLC本文方法Colony10.49880.46180.43070.58010.50580.6026Colony20.18210.15370.15530.33650.46150.4479Colony30.19830.15100.16100.26340.12630.3257Colony40.16310.14880.19060.14370.15210.2016Colony50.16200.18960.19020.18820.17350.1654表 3 不同检测方法下的边缘连续性CIdxTable 3 CIdx of different methodsSerial numberCIdxCanny SPMISMOSMNLC本文方法Colony10.83770.85300.86010.86760.97490.9652Colony20.80690.86550.85330.82930.91770.9518Colony30.80640.74080.72930.82690.77640.9406Colony40.81430.86110.90440.84300.90150.9776Colony50.90470.84480.86320.85920.87090.95718 期郑程驰等: 视网膜功能启发的边缘检测层级模型1779。
integrate and fire modle
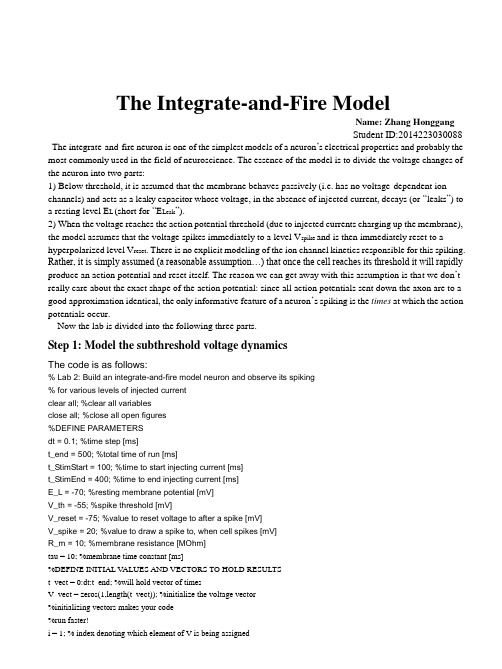
The Integrate-and-Fire ModelName: Zhang HonggangStudent ID:2014223030088 The integrate-and-fire neuron is one of the simplest models of a neuron’s electrical properties and probably the most commonly used in the field of neuroscience. The essence of the model is to divide the voltage changes of the neuron into two parts:1) Below threshold, it is assumed that the membrane behaves passively (i.e. has no voltage-dependent ion channels) and acts as a leaky capacitor whose voltage, in the absence of injected current, decays (or “leaks”) toa resting level E L (short for “E Leak”).2) When the voltage reaches the action potential threshold (due to injected currents charging up the membrane), the model assumes that the voltage spikes immediately to a level V spike and is then immediately reset to a hyperpolarized level V reset. There is no explicit modeling of the ion channel kinetics responsible for this spiking. Rather, it is simply assumed (a reasonable assumption…) that once the cell reaches its threshold it will rapidly produce an action potential and reset itself. The reason we can get away with this assumption is that we don’t really care about the exact shape of the action potential: since all action potentials sent down the axon are to a good approximation identical, the only informative feature of a neuron’s spiking is the times at which the action potentials occur.Now the lab is divided into the following three parts.Step 1: Model the subthreshold voltage dynamicsThe code is as follows:% Lab 2: Build an integrate-and-fire model neuron and observe its spiking% for various levels of injected currentclear all; %clear all variablesclose all; %close all open figures%DEFINE PARAMETERSdt = 0.1; %time step [ms]t_end = 500; %total time of run [ms]t_StimStart = 100; %time to start injecting current [ms]t_StimEnd = 400; %time to end injecting current [ms]E_L = -70; %resting membrane potential [mV]V_th = -55; %spike threshold [mV]V_reset = -75; %value to reset voltage to after a spike [mV]V_spike = 20; %value to draw a spike to, when cell spikes [mV]R_m = 10; %membrane resistance [MOhm]tau = 10; %membrane time constant [ms]%DEFINE INITIAL VALUES AND VECTORS TO HOLD RESULTSt_vect = 0:dt:t_end; %will hold vector of timesV_vect = zeros(1,length(t_vect)); %initialize the voltage vector%initializing vectors makes your code%run faster!i = 1; % index denoting which element of V is being assignedV_vect(i)=E_L; %first element of V, i.e. value of V at t=0%INTEGRATE THE EQUATION tau*dV/dt = -V + E_L + I_e*R_mI_Stim = 1; %magnitude of pulse of injected current [nA]I_e_vect = zeros(1,t_StimStart/dt); %portion of I_e_vect from t=0 to t=t_StimStartI_e_vect = [I_e_vect I_Stim*ones(1,1+((t_StimEnd-t_StimStart)/dt))]; %add portion from % t=t_StimStart to t=t_StimEndI_e_vect = [I_e_vect zeros(1,(t_end-t_StimEnd)/dt)]; %add portion from% t=t_StimEnd to t=t_endfor t=dt:dt:t_end %loop through values of t in steps of dt msV_inf = E_L + I_e_vect(i)*R_m; %value that V_vect is exponentially%decaying towards at this time step%next line does the integration update ruleV_vect(i+1) = V_inf + (V_vect(i) - V_inf)*exp(-dt/tau);i = i + 1; %add 1 to index, corresponding to moving forward 1 time stepend%MAKE PLOTSfigure(1)plot(t_vect, V_vect);title('V oltage vs. time');xlabel('Time in ms');ylabel('V oltage in mV');The matlab operating results as shown below:Step 2: Add the spiking to the model and calculate the firing rateThe code is as follows:% Lab 2: Build an integrate-and-fire model neuron and observe its spiking% for various levels of injected currentclear all; %clear all variablesclose all; %close all open figures%DEFINE PARAMETERSdt = 0.1; %time step [ms]t_end = 500; %total time of run [ms]t_StimStart = 100; %time to start injecting current [ms]t_StimEnd = 400; %time to end injecting current [ms]E_L = -70; %resting membrane potential [mV]V_th = -55; %spike threshold [mV]V_reset = -75; %value to reset voltage to after a spike [mV]V_spike = 20; %value to draw a spike to, when cell spikes [mV]R_m = 10; %membrane resistance [MOhm]tau = 10; %membrane time constant [ms]%DEFINE INITIAL VALUES AND VECTORS TO HOLD RESULTSt_vect = 0:dt:t_end; %will hold vector of timesV_vect = zeros(1,length(t_vect)); %initialize the voltage vector%initializing vectors makes your code run faster!V_plot_vect = zeros(1,length(t_vect)); %pretty version of V_vect to be plotted, that displays a spike % whenever voltage reaches thresholdi = 1; % index denoting which element of V is being assignedV_vect(i)= E_L; %first element of V, i.e. value of V at t=0V_plot_vect(i) = V_vect(i); %if no spike, then just plot the actual voltage V%INTEGRATE THE EQUATION tau*dV/dt = -V + E_L + I_e*R_mI_Stim = 1.55; %magnitude of pulse of injected current [nA]I_e_vect = zeros(1,t_StimStart/dt); %portion of I_e_vect from t=0 to t=t_StimStartI_e_vect = [I_e_vect I_Stim*ones(1,1+((t_StimEnd-t_StimStart)/dt))]; %add portion from% t=t_StimStart to t=t_StimEndI_e_vect = [I_e_vect zeros(1,(t_end-t_StimEnd)/dt)]; %add portion from% t=t_StimEnd to t=t_endNumSpikes = 0; %holds number of spikes that have occurredfor t=dt:dt:t_end %loop through values of t in steps of dt msV_inf = E_L + I_e_vect(i)*R_m; %value that V_vect is exponentially%decaying towards at this time step%next line does the integration update ruleV_vect(i+1) = V_inf + (V_vect(i) - V_inf)*exp(-dt/tau);%if statement below says what to do if voltage crosses thresholdif (V_vect(i+1) > V_th) %cell spikedV_vect(i+1) = V_reset; %set voltage back to V_resetV_plot_vect(i+1) = V_spike; %set vector that will be plotted to show a spike hereNumSpikes = NumSpikes + 1; %add 1 to the total spike countelse %voltage didn't cross threshold so cell does not spikeV_plot_vect(i+1) = V_vect(i+1); %plot the actual voltageendi = i + 1; %add 1 to index, corresponding to moving forward 1 time stependAveRate = 1000*NumSpikes/(t_StimEnd - t_StimStart) %gives average firing rate in [#/sec = Hz] %MAKE PLOTSfigure(1)plot(t_vect, V_plot_vect);title('V oltage vs. time');xlabel('Time in ms');ylabel('V oltage in mV');The matlab operating results as shown below:Step 3: Compare r isi,theory to r aveThe code is as follows:The final code from this lab should be:% Lab 2: Build an integrate-and-fire model neuron and observe its spiking% for various levels of injected currentclear all; %clear all variablesclose all; %close all open figures%DEFINE PARAMETERSdt = 0.1; %time step [ms]t_end = 500; %total time of run [ms]t_StimStart = 100; %time to start injecting current [ms]t_StimEnd = 400; %time to end injecting current [ms]E_L = -70; %resting membrane potential [mV]V_th = -55; %spike threshold [mV]V_reset = -75; %value to reset voltage to after a spike [mV]V_spike = 20; %value to draw a spike to, when cell spikes [mV]R_m = 10; %membrane resistance [MOhm]tau = 10; %membrane time constant [ms]%DEFINE INITIAL VALUES AND VECTORS TO HOLD RESULTSt_vect = 0:dt:t_end; %will hold vector of timesV_vect = zeros(1,length(t_vect)); %initialize the voltage vector%initializing vectors makes your code run faster!V_plot_vect = zeros(1,length(t_vect)); %pretty version of V_vect to be plotted, that displays a spike % whenever voltage reaches threshold%INTEGRATE THE EQUATION tau*dV/dt = -V + E_L + I_e*R_mPlotNum=0;I_Stim_vect = 1.43:0.04:1.63; %magnitudes of pulse of injected current [nA]for I_Stim = I_Stim_vect; %loop over different I_Stim valuesPlotNum = PlotNum + 1;i = 1; % index denoting which element of V is being assignedV_vect(i)= E_L; %first element of V, i.e. value of V at t=0V_plot_vect(i) = V_vect(i); %if no spike, then just plot the actual voltage VI_e_vect = zeros(1,t_StimStart/dt); %portion of I_e_vect from t=0 to t=t_StimStartI_e_vect = [I_e_vect I_Stim*ones(1,1+((t_StimEnd-t_StimStart)/dt))]; %add portion from% t=t_StimStart to t=t_StimEndI_e_vect = [I_e_vect zeros(1,(t_end-t_StimEnd)/dt)]; %add portion from% t=t_StimEnd to t=t_endNumSpikes = 0; %holds number of spikes that have occurredfor t=dt:dt:t_end %loop through values of t in steps of dt msV_inf = E_L + I_e_vect(i)*R_m; %value that V_vect is exponentially%decaying towards at this time step%next line does the integration update ruleV_vect(i+1) = V_inf + (V_vect(i) - V_inf)*exp(-dt/tau);%if statement below says what to do if voltage crosses thresholdif (V_vect(i+1) > V_th) %cell spikedV_vect(i+1) = V_reset; %set voltage back to V_resetV_plot_vect(i+1) = V_spike; %set vector that will be plotted to show a spike hereNumSpikes = NumSpikes + 1; %add 1 to the total spike countelse %voltage didn't cross threshold so cell does not spikeV_plot_vect(i+1) = V_vect(i+1); %plot the actual voltageendi = i + 1; %add 1 to index, corresponding to moving forward 1 time stependAveRate_vect(PlotNum) = 1000*NumSpikes/(t_StimEnd - t_StimStart) %gives average firing%rate in [#/sec = Hz]%MAKE PLOTSfigure(1)subplot(length(I_Stim_vect),1,PlotNum)plot(t_vect, V_plot_vect);if (PlotNum == 1)title('Voltage vs. time');endif (PlotNum == length(I_Stim_vect))xlabel('Time in ms');endylabel('Voltage in mV');end %for I_Stim%COMPARE R_AVE TO R_ISII_threshold = (V_th - E_L)/R_m; %current below which cell does not fireI_vect_long = (I_threshold+0.001):0.001:1.8; %vector of injected current for producing theory plot r_isi = 1000./(tau*log((V_reset - E_L - I_vect_long*R_m)./(V_th - E_L - I_vect_long*R_m))); figure(2)plot(I_vect_long,r_isi)hold onplot(I_Stim_vect,AveRate_vect,'ro')title('Comparison of r_{isi} vs. I_e and r_{ave} vs. I_e')xlabel('Injected current (nA)')ylabel('r_{isi} or r_{ave} (Hz)')hold off %to ensure that doesn't keep this data the next time you want to plot somethingThe matlab operating results as shown below:( Explanation: The above test may have many ambiguities,For in-depth interpretation,please refer to the following link address, thanks~~~/goldman/Tutorials_files/Integrate&Fire.pdf)。
纳米管制作皮肤感应器 翻译 中英
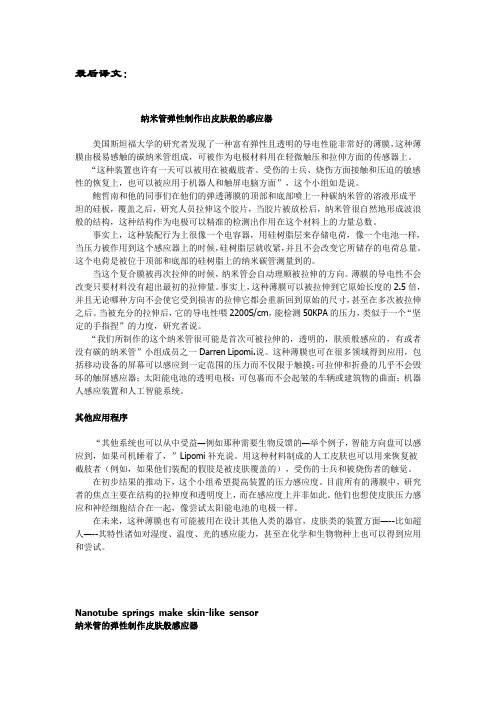
最后译文:纳米管弹性制作出皮肤般的感应器美国斯坦福大学的研究者发现了一种富有弹性且透明的导电性能非常好的薄膜,这种薄膜由极易感触的碳纳米管组成,可被作为电极材料用在轻微触压和拉伸方面的传感器上。
“这种装置也许有一天可以被用在被截肢者、受伤的士兵、烧伤方面接触和压迫的敏感性的恢复上,也可以被应用于机器人和触屏电脑方面”,这个小组如是说。
鲍哲南和他的同事们在他们的弹透薄膜的顶部和底部喷上一种碳纳米管的溶液形成平坦的硅板,覆盖之后,研究人员拉伸这个胶片,当胶片被放松后,纳米管很自然地形成波浪般的结构,这种结构作为电极可以精准的检测出作用在这个材料上的力量总数。
事实上,这种装配行为上很像一个电容器,用硅树脂层来存储电荷,像一个电池一样,当压力被作用到这个感应器上的时候,硅树脂层就收紧,并且不会改变它所储存的电荷总量。
这个电荷是被位于顶部和底部的硅树脂上的纳米碳管测量到的。
当这个复合膜被再次拉伸的时候,纳米管会自动理顺被拉伸的方向。
薄膜的导电性不会改变只要材料没有超出最初的拉伸量。
事实上,这种薄膜可以被拉伸到它原始长度的2.5倍,并且无论哪种方向不会使它受到损害的拉伸它都会重新回到原始的尺寸,甚至在多次被拉伸之后。
当被充分的拉伸后,它的导电性喂2200S/cm,能检测50KPA的压力,类似于一个“坚定的手指捏”的力度,研究者说。
“我们所制作的这个纳米管很可能是首次可被拉伸的,透明的,肤质般感应的,有或者没有碳的纳米管”小组成员之一Darren Lipomi.说。
这种薄膜也可在很多领域得到应用,包括移动设备的屏幕可以感应到一定范围的压力而不仅限于触摸;可拉伸和折叠的几乎不会毁坏的触屏感应器;太阳能电池的透明电极;可包裹而不会起皱的车辆或建筑物的曲面;机器人感应装置和人工智能系统。
其他应用程序“其他系统也可以从中受益—例如那种需要生物反馈的—举个例子,智能方向盘可以感应到,如果司机睡着了,”Lipomi补充说。
神经科学热点 神经元的分类

神经科学热点神经元的分类Neurons are the basic building blocks of the nervous system. They play a crucial role in transmitting information throughout the body, allowing us to think, feel, move, and perform various functions. Despite their inherent complexity, neurons can be classified based on different criteria, including their structure, function, and neurotransmitter type.神经元是神经系统的基本组成部分。
它们在整个身体中传递信息起着关键作用,使我们能够思考、感受、运动和执行各种功能。
尽管神经元本身非常复杂,但可以根据结构、功能和神经递质类型等不同的标准对其进行分类。
One common way to classify neurons is based on their structure. Neurons can be broadly divided into three categories: multipolar neurons, bipolar neurons, andunipolar neurons.根据结构将神经元进行分类是一种常见的方法。
神经元可以大致分为三类:多极神经元、双极神经元和单极神经元。
Multipolar neurons are the most common type of neuron. They have multiple processes or extensions called dendrites that receive signals from other neurons and one long axon that transmits signals away from the cell body to other neurons or muscles.多极神经元是最常见的一种类型。
基于FPGA的脉冲神经网络加速器设计

基于FPGA的脉冲神经网络加速器设计沈阳靖;沈君成;叶俊;马琪【摘要】脉冲神经网络是一种基于离散神经脉冲原理进行信息处理的人工神经网络,文中提出了一种基于FPGA的灵活可配的脉冲神经网络加速器架构,能够支持神经网络拓扑结构、连接权值的灵活配置.该设计首先在算法层对LIF神经元模型进行公式分解和浮点转定点两个层次的优化,并在硬件实现中采用时分复用技术将硬件中实现的8个物理神经元复用为256个逻辑神经元.神经元模电压计算采用三级流水线架构,以提高神经元数据处理效率.通过采用Xilinx XC6SLX45 FPGA实现整个神经网络加速器,工作频率可达50 MHz,并基于该加速器构建手写数字识别网络架构,实验结果表明,采用MNIST数据集作为测试样例,该网络架构准确率可达93%.【期刊名称】《电子科技》【年(卷),期】2017(030)010【总页数】5页(P89-92,96)【关键词】脉冲神经网络;LIF模型;时分复用;分类【作者】沈阳靖;沈君成;叶俊;马琪【作者单位】杭州电子科技大学微电子CAD研究所,浙江杭州310018;浙江大学超大规模集成电路研究所,浙江杭州310007;杭州士兰微电子股份有限公司,浙江杭州310007;杭州电子科技大学微电子CAD研究所,浙江杭州310018【正文语种】中文【中图分类】TN912.11;TP183Abstract Spiking neural network is a kind of biologically-inspired neural networks that perform information processing based on discrete-time spikes. This paper proposes a FPGA based hardware accelerator, which supports the flexible configuration of topology and synapse weights. First, LIF(Leaky Integrate-and- Fire, LIF) model is optimized for hardware implementation, and then 8 physical LIF neurons are implemented, which could be extended to 256 neurons by using time-multiplexing technology. To improve the data processing efficiency of the spiking neuron, the design adopts three-stage pipeline architecture to calculate the neuron voltage. At last, the design is implemented on XC6SLX45 FPGA running over 50 MHz operation frequency. MINST database is used as an application example to demonstrate the configurability and efficiency of the proposed implementation. The experimental results show the accuracy of handwritten number classification could be achieved as high as 93%. Keywords spiking neuron network; LIF model; time-multiplexing technology; classification.脉冲神经网络[1](Spiking Neuron Network,SNN)是一种基于离散神经脉冲进行信息处理的人工神经网络,采用可塑的突触和基于脉冲模式的编码,能够同时模拟神经网络的时空特性,具有更高的生物真实性, 可达到更好的性能功耗比,被称为第三代人工神经网络[3]。
计算神经科学讲义
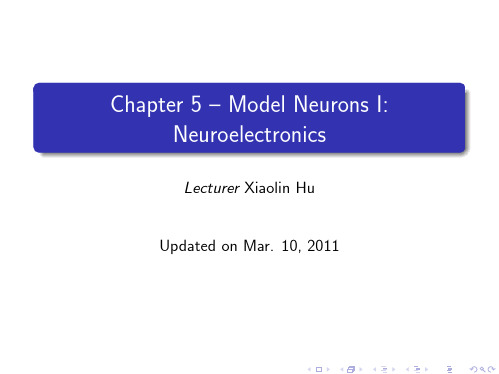
ENa = 61.54 log10 ECa =
[Na+ ]o [Na+ ]i [Ca2+ ]o 30.77 log10 [Ca2+ ] i
mV mV
Goldman Equation and Reversal Potential
Example Calculate the longitudinal resistance of a segment 100 µm long with a radius of 2 µm): RL = rL L 1kΩmm × 100µm = ≈ 8MΩ. 2 πa π × 4µm2
Example Calculate a single channel’s conductance (6 nm long with a cross-sectional area 0.15 nm2 ): g= πa2 0.15nm2 = ≈ 25 × 10−12 S = 25pS. rL L 1kΩmm × 6nm
dV = −V + EL + Rm Ie dt where Rm = rm /A is the total membrane resistance and τm is the time constant. τm
Firing Rate w.r.t Constant Input
The subthreshold potential V (t) is obtained by solving the basic model as V (t) = EL + Rm Ie + (V (0) − EL − Rm Ie ) exp(−t/τm ) Suppose V (0) = Vreset and the neuron will fire an action potential at time t = tisi again, then V (tisi ) = Vth = EL + Rm Ie + (Vreset − EL − Rm Ie ) exp(−tisi /τm ) 1 = ⇒risi = tisi
The Leaky Integrate-and-fire Neuron
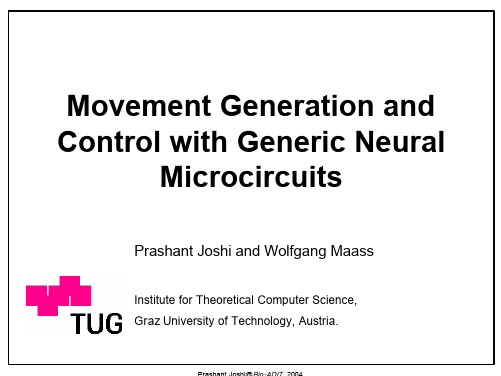
[2] D.V. Buonomano and M.M. Merzenich. Temporal information transformed into a spatial code by a neural network with realistic properties. Science, 267:1028-1030
Movement Generation and Control with Generic Neural Microcircuits
Prashant Joshi and Wolfgang Maass
Institute for Theoretical Computer Science, Graz University of Technology, Austria.
[4] W. Maass, T. Natschläger and H. Markram. Computational models for generic cortical microcircuits. In J. Feng, editor, Computational Neuroscience: A Comprehensive Approach, chap. 18. CRC-Press.
• Multiple combinations of joint motions at shoulder, elbow and wrist for each trajectory.
• Multiple muscle combinations can result in same joint torques.
Overview Task:
• 2-joint robot arm control with generic spiking neural microcircuits.
Integrate&Fire

The Integrate-and-Fire ModelThe integrate-and-fire neuron is one of the simplest models of a neuron’s electrical properties and probably the most commonly used in the field of neuroscience. The essence of the model is to divide the voltage changes of the neuron into two parts:1) Below threshold, it is assumed that the membrane behaves passively (i.e. has no voltage-dependent ion channels) and acts as a leaky capacitor whose voltage, in the absence of injected current, decays (or “leaks”) to a resting level E L (short for “E Leak ”).2) When the voltage reaches the action potential threshold (due to injected currents charging up the membrane), the model assumes that the voltage spikes immediately to a level V spike and is then immediately reset to a hyperpolarized level V reset . There is no explicitmodeling of the ion channel kinetics responsible for this spiking. Rather, it is simply assumed (a reasonable assumption…) that once the cell reaches its threshold it will rapidly produce an action potential and reset itself. The reason we can get away with this assumption is that we don’treally care about the exact shape of the action potential: since all action potentials sent down the axon are to a good approximation identical, the only informative feature of a neuron’s spiking is the times at which the action potentials occur.I. Mathematics of the integrate-and-fire neuronConsider a neuron modeled as a leaky capacitor with membrane resistance R m , time constant τm =R m C m (where C m is the membrane’s capacitance), and resting potential E L . Below the action potential threshold, the equation for the voltage of this cell when it receives current injection I e is: m L dV E V R I dtτ=−+m e (1)Exercise: When the current injection I e is constant over time, verify(i) that the solution to this equation is:()0()(())exp ()/L m e L m e o m V t E R I V t E R I t t τ=++−−−− (2)where the constant t 0 is any reference time. (Do this by inserting the solution on both sides of equation (1). Recall that ()()()()f t f t d d e e dt dt=f t e ).(ii) that when t=t 0, the left and right sides of equation (2) agree (and both equal what value?), and(iii) that when t → ∞, V(t) → .L m V E R I ∞≡+Setting t 0 equal to the current time in a computer simulation and t equal to the time a single time-step Δt later gives the one-time-step update rule we will use for simulating theintegrate-and-fire neuron (and which we will use more generally for simulating any equation of the form of equation (1) above, e.g. in your homework for the spike-rate-adaptation conductance if we substitute g sra for V and set E L =I e =0):()()exp L m e L m e m V E R I V E R I t /τ→++−−−Δ,where this notation means that, in the time step Δt, the voltage gets updated from its old value to the value on the right of the arrow, i.e. (in mathematics notation, in terms of the actual time t) ()()()(()())exp /L m e L m e m V t t E R I t V t E R I t t τ+Δ=++−−−Δ (3) or (in MATLAB notation, in terms of values at time step i)()(1)()(()())exp /L m e L m e m V i E R I i V i E R I i t τ+=++−−−Δ (4)Now let’s see how to implement this…II. Today’s model: the integrate-and-fire neuronIn the following sections, our goal will be to verify the firing rate r vs. (constant) injected current relationship for the integrate-and-fire model neuron: 10000ln ,if 1 0, if m L reset th L m threshold m L th m theory isi th L threshold m R I E V V E I I R I E V R r t V E I I R τ−⎧⎡⎤⎛⎞+−−⎪>=⎢⎥⎜⎟+−⎪⎝⎠⎣⎦==⎨−⎪≤=⎪⎩(5) where t isi is the interspike interval for an integrate-and-fire neuron receiving constant current input I e =I 0=constant and I threshold is the minimum level of current injection needed to make the neuron fire.To confirm this relation, we will build a model integrate-and-fire neuron that obeys the equation (equation (1)) m L dV E V R I dtτ=−+m e for voltages below the action potential threshold and spikes whenever it reaches the action potential threshold. For concreteness, we will use parameter values E L = -70 mV, R m = 10 M Ω, and τm =10 ms. We will assume that, initially (i.e. at t=0), V = E L .To model the spiking of the neuron when it reaches threshold, we will assume that when the membrane potential reaches V th = -55 mV, the neuron fires a spike and then resets its membrane potential to V reset = -75 mV.We will inject various levels of current I e into the neuron and, to calculate the firing rate, we will count the number of action potentials in a fixed amount of time. For starters, we will assume that the neuron receives a 300-ms-long current pulse of magnitude I 0 beginning at time t pulse =100 ms and plot several representative values of I e that produce firing rates between 1 and 100 Hz. We will run our our simulations for 500 ms total (i.e. 100 ms with I e =0; 300 ms with I e =I 0 > 0; and another 100 ms with I e =0). We will run our simulation with a time step dt = 0.1 ms.As the final output, we will produce graphs of voltage vs. time for several levels of I 0 and a summary graph comparing r theory to the average firing rate r ave of the neuron over ameasurement time T, defined as: # of AP's in time T T Tave N r == (6)III. Step 1: Model the subthreshold voltage dynamicsAs a first step, we will model just the subthreshold dynamics of the model governed by equation (1). In the next section, we will add the spiking. Our general overall strategy is to break our code into the following sections:1. Define the parameters in the model2. Define the vectors that will hold our final results such as the time, voltage, and current;and assign their initial values corresponding to t=0.3. Integrate the equation(s) of the model to obtain the values of the above vectors at latertimes by updating the values at the previous time step with the update rule.4. Make pretty plots of our results.First, let’s open a new m-file and name it something memorable like “IntAndFire1.m”.Let’s put a comment right at the top:% Lab 2: Build an integrate-and-fire model neuron and observe its spiking% for various levels of injected currentNow let’s ensure (as we should always do) that all variables are cleared and figures are closed by adding:clear all; %clear all variablesclose all; %close all open figuresIt is good programming style to next assign values to all model parameters in a well-marked section of your code. We need to assign values to the following parameters (type this code):%DEFINE PARAMETERSdt = 0.1; %time step [ms]t_end = 500; %total time of run [ms]t_StimStart = 100; %time to start injecting current [ms]t_StimEnd = 400; %time to end injecting current [ms]E_L = -70; %resting membrane potential [mV]V_th = -55; %spike threshold [mV]V_reset = -75; %value to reset voltage to after a spike [mV]V_spike = 20; %value to draw a spike to, when cell spikes [mV]R_m = 10; %membrane resistance [MOhm]tau = 10; %membrane time constant [ms]Notice that we have made a comment describing each parameter and noting its units. Checking that your units make sense (i.e. that both sides of any equation have the same units) is very important and a good way to find errors.Next, it is good to set up the initial conditions for the run (i.e. specify what the values of the relevant variables will be at time t=0) and to define and initialize variables (often vectors) that will hold all of the information we eventually might want to plot or use for other purposes. In our case, we are certainly going to plot voltage vs. time so let’s define a time vector running from t=0 to t=t_end in time steps of size dt; and a corresponding voltage vector that will hold the voltage at each of these times. As a placeholder, let’s initially assign the voltage vector to be all zeros. Note below that I put “_vect” on the end of the names of all vector variables – this notation helps to keep track of which variables are simply numbers (scalars) versus vectors.%DEFINE INITIAL VALUES AND VECTORS TO HOLD RESULTSt_vect = 0:dt:t_end; %will hold vector of timesV_vect = zeros(1,length(t_vect)); %initialize the voltage vector%initializing vectors makes your code%run faster!Aside: this initial setting up of the voltage vector to be the correct size is not strictly necessary but makes your code run faster. This is because it takes MATLAB a long time to create new vectors or change the size of old vectors (for the computer science whizzes, this is because creating or changing the size of vectors requires MATLAB to ask the computer for memory in which to store the vector, which is a slow and complicated process).One more thing to add to the above section: We said that we initially want V = E_L so let’s set the first element of V to this value (recall that the first element of the t_vector is t=0). It will be useful to have a variable corresponding to the index of the array so let’s also define the variable i as the current element of V being assigned:i = 1; % index denoting which element of V is being assignedV_vect(i)=E_L; %first element of V, i.e. value of V at t=0Good! Now we’re ready to integrate equation (1). First, let’s define the current injected at all times as I_e_vect. For now, set I_e_vect=zeros for all time. We’ll try a few more interesting values soon. (Note: you may wonder why we didn’t define I_e_vect in the parameters section. We could and maybe even should have, but here I am anticipating that we will later do a loop over various I_e_vect values. Stay tuned…).%INTEGRATE THE EQUATION tau*dV/dt = -V + E_L + I_e*R_mI_e_vect = zeros(1,length(t_vect)); %injected current [nA]To do this integration, we now use the rule described in equation (3). We’re clearly going to need to iterate this rule many times. That should be a clear signal to us that it’s time to use a for loop that iterates over the values of t. Let’s set that up and then fill in the inside of the loop later: for t=dt:dt:t_end % loop through values of t in steps of dt msendNote that we start the loop at time dt because we already have the initial values t=0 andV(t=0)=E_L defined. Now let’s fill in the inside of the loop. We need to first denote which element of V_vect is being updated (this should be one more than the last time through the loop, i.e. set i = i + 1) and then run our update rule to assign the appropriate value of V to this element of V_vect. The loop should read:for t=dt:dt:t_end %loop through values of t in steps of dt msV_inf = E_L + I_e_vect(i)*R_m; %value that V_vect is exponentially%decaying towards at this time step%next line does the integration update ruleV_vect(i+1) = V_inf + (V_vect(i) - V_inf)*exp(-dt/tau);i = i + 1; %add 1 to index, corresponding to moving forward 1 time stependGreat! Now that we’ve assigned v, we’re ready to plot. Add some plotting code next:%MAKE PLOTSfigure(1)plot(t_vect, V_vect);title('Voltage vs. time');xlabel('Time in ms');ylabel('Voltage in mV');Now go to your MATLAB command window and run your file. You should see a solid trace at -70 mV (if you don’t, peek ahead and the code you should have typed will be summarized). This is exactly right: you assigned the voltage to start at rest and then didn’t inject any current so the voltage stayed at rest. If you like, try playing with changing your initial voltage and see what happens (please remember to set it back to E_L before continuing on!).Next, we said that we wanted to start stimulating at time t_StimStart = 100 ms and end at timet_StimEnd = 400 ms. Between 100 and 400 ms, let’s set the elements of I_e_vect = I_StimPulse where I_StimPulse is the amplitude of the injected current during the stimulation. Let’s set this to a value I_StimPulse = 1 nA. We could do this in 2 ways:1) Within the loop use an if statement that says: if (t<100 || t>400) then assign the elements of I_e_vect = 0; else assign I_e_vect = I_StimPulse. (Note: || is MATLAB’s symbol for the logical word OR; MATLAB’s symbol for the logic word AND is &&). This is the most conceptually straightforward way but is not particularly efficient.2) The more efficient way of doing the assignment is just to replace the line I_e_vect = zeros(1,length(t_vect)); by an appropriate line defining the vector. Since dt=0.1 ms, we really want the first 1000 elements (from t=0.0 to t=99.9 ms) to equal zero; the next 3001 elements (from t=100.0 to t=400.0 ms) to equal I_StimPulse; and the final 1000 elements (from t=400.1 to t=500 ms) to equal zero.We can do the latter by the following lines (replace the previous I_e_vect line by this, and see next paragraph for detailed explanation):I_Stim = 1; %magnitude of pulse of injected current [nA]I_e_vect = zeros(1,t_StimStart/dt); %portion of I_e_vect from t=0 to t=t_StimStartI_e_vect = [I_e_vect I_Stim*ones(1,1+((t_StimEnd-t_StimStart)/dt))]; %add portion from% t=t_StimStart to t=t_StimEndI_e_vect = [I_e_vect zeros(1,(t_end-t_StimEnd)/dt)]; %add portion from% t=t_StimEnd to t=t_endIn the second line, we set up the first portion of the vector as a row of zeros with the number of elements equal to t_StimStart/dt, which is the number of time points between zero and the stimulus start time. In the next line, we append to this vector a row of values I_Stim for all time point between t_StimStart and t_StimEnd (including the points t=tStimStart and the pointt=tStimEnd. The “1+” covers this. For example, if tStimStart = 10, tStimEnd = 11, and dt = 0.1, then there would be eleven 5’s appended to I_e_vector here.). In the final line above, we append to I_e_vect another row of zeros corresponding to times from t=tStimEnd to t=t_end. We couldactually have done this all in one line but it would have made our code harder to read without a major savings in efficiency. If you want to see the vector output from any of these lines, just remove the semicolon and see the print out (Warning: there are a lot of entries here! Use Control-C, which makes MATLAB stop whatever it is doing and return to the Command Prompt, to stop the output if you get tired of it scrolling across your screen). You could also type a length() statement to just check the lengths of these vectors. Checking lengths of vectors and size’s of arrays is a very useful tool in debugging your code.Now run your code. It should rise exponentially towards -60mV starting at t = 100, then decay back down exponentially at t = 400 (both rise and decay with time constants τ = 10 ms) as shown below. Try out some other values of I_Stim on your own to get a feeling for how big a voltage change you get for different values of I_Stim.To summarize, your code at this stage should read:% Lab 2: Build an integrate-and-fire model neuron and observe its spiking% for various levels of injected currentclear all; %clear all variablesclose all; %close all open figures%DEFINE PARAMETERSdt = 0.1; %time step [ms]t_end = 500; %total time of run [ms]t_StimStart = 100; %time to start injecting current [ms]t_StimEnd = 400; %time to end injecting current [ms]E_L = -70; %resting membrane potential [mV]V_th = -55; %spike threshold [mV]V_reset = -75; %value to reset voltage to after a spike [mV]V_spike = 20; %value to draw a spike to, when cell spikes [mV]R_m = 10; %membrane resistance [MOhm]tau = 10; %membrane time constant [ms]%DEFINE INITIAL VALUES AND VECTORS TO HOLD RESULTSt_vect = 0:dt:t_end; %will hold vector of timesV_vect = zeros(1,length(t_vect)); %initialize the voltage vector%initializing vectors makes your code%run faster!i = 1; % index denoting which element of V is being assignedV_vect(i)=E_L; %first element of V, i.e. value of V at t=0%INTEGRATE THE EQUATION tau*dV/dt = -V + E_L + I_e*R_mI_Stim = 1; %magnitude of pulse of injected current [nA]I_e_vect = zeros(1,t_StimStart/dt); %portion of I_e_vect from t=0 to t=t_StimStartI_e_vect = [I_e_vect I_Stim*ones(1,1+((t_StimEnd-t_StimStart)/dt))]; %add portion from% t=t_StimStart to t=t_StimEndI_e_vect = [I_e_vect zeros(1,(t_end-t_StimEnd)/dt)]; %add portion from% t=t_StimEnd to t=t_endfor t=dt:dt:t_end %loop through values of t in steps of dt msV_inf = E_L + I_e_vect(i)*R_m; %value that V_vect is exponentially%decaying towards at this time step%next line does the integration update ruleV_vect(i+1) = V_inf + (V_vect(i) - V_inf)*exp(-dt/tau);i = i + 1; %add 1 to index, corresponding to moving forward 1 time stepend%MAKE PLOTSfigure(1)plot(t_vect, V_vect);title('Voltage vs. time');xlabel('Time in ms');ylabel('Voltage in mV');IV. Step 2: Add the spiking to the model and calculate the firing rate Hopefully you noticed that, no matter how large you made I_Stim, your neuron did not spike. Next, we will add the code to make the neuron spike and then reset each time its voltage reaches the threshold value V_th. To do this, we need an if statement to detect when the voltage reaches V_th, and if so, we need to then reset the voltage back to V_reset. This can be done by adding the following immediately after the assignment V_vect(i+1) = V_inf + (V_vect(i) - V_inf)*exp(-dt/tau); %if statement below says what to do if voltage crosses thresholdif (V_vect(i+1) > V_th) %cell spikedV_vect(i+1) = V_reset; %set voltage back to V_resetendTry running this code with I_Stim = 1.55. You should see the neuron reset its voltage each time it reaches V_th = -55 mV. This should occur 8 times. However, you are probably wondering, “Where are the beautiful spikes going up to some high voltage?” Well, in truth, the integrate-and-fire model never really assigns a voltage above V_th. Every time threshold is reached, it immediately resets the voltage to V_reset (which is our signal that a spike occurred at this time, if we were trying to count the number of spikes).Well, we certainly want to make our plots prettier than that so let’s “by hand” (well, aided by the computer…) assign a new vector we’ll call V_plot_vect which replaces the first time point after threshold by a beautiful point at a voltage V=V_spike = 20 mV (or whatever value you find to be aesthetically appealing). We can do this by creating this vector in the “Define initial values…” section and also there assigning its first value to be equal to the value of the Voltage vector. Do this by modifying that section of the code to read:%DEFINE INITIAL VALUES AND VECTORS TO HOLD RESULTSt_vect = 0:dt:t_end; %will hold vector of timesV_vect = zeros(1,length(t_vect)); %initialize the voltage vector%initializing vectors makes your code run faster!V_plot_vect = zeros(1,length(t_vect)); %pretty version of V_vect to be plotted, that displays a spike% whenever voltage reaches thresholdi = 1; % index denoting which element of V is being assignedV_vect(i)= E_L; %first element of V, i.e. value of V at t=0V_plot_vect(i) = V_vect(i); %if no spike, then just plot the actual voltage VThen modify the integration loop to assign this vector by replacing the if statement above by:if (V_vect(i+1) > V_th) %cell spikedV_vect(i+1) = V_reset; %set voltage back to V_resetV_plot_vect(i+1) = V_spike; %set vector that will be plotted to show a spike hereelse %voltage didn't cross threshold so cell does not spikeV_plot_vect(i+1) = V_vect(i+1); %plot the actual voltageendNow also change your plotting command to plot V_plot_vect rather than V_vect and run your program. You should see 8 beautiful spikes(!) like the following:Finally, we would like to compute the average firing rate of the cell during the time of stimulation. A cell’s average firing rate over a specified period of time is the number of spikesproduced over the specified time period: r ave=(# of spikes)/(time period). A special situation is when we choose the period of time to be from immediately after one spike’s occurrence to immediately after the next spike’s occurrence. This time period between spikes is known as the interspike interval and is denoted by t isi. The corresponding firing rate is r isi = 1/t isi, and this is what we calculated exactly in class for the integrate-and-fire model neuron receiving a constant stimulating current. Here, we will more simply calculate r ave by counting the number of spikes that occurred during the stimulation period and then dividing by this time period. In the next section, we compare this value to the value of r isi that we calculated in class.To count the number of spikes, we add a new variable to our code called NumSpikes that we set initially to zero (since no spikes have occurred at the beginning of the simulation) and that we increase in value by 1 every time a spike occurs. We then divide this number by the duration of stimulation to get the firing rate in # spikes/ms. To convert from # spikes/ms to # spikes/sec we then multiply by 1000.To do this, add just before your for loop the line:NumSpikes = 0 %holds number of spikes that have occurredThen add the following code within the if statement that identifies a spike:NumSpikes = NumSpikes + 1 %add 1 to the total spike countFinally, just after the end of your for loop add the line defining the average firing rate:AveRate = 1000*NumSpikes/(t_StimEnd - t_StimStart) %gives average firing rate in [#/sec = Hz]Leave off the semicolons so that the values output to your screen. Try this for a few values of I_Stim (to be realistic try to keep the firing rate between 0 and 100 Hz). For IStim=1.55 you should get a rate of 26.6667 Hz. [After trying this, add semicolons after the first 2 lines you assigned above so that NumSpikes doesn’t keep printing to your screen.]Your code for this section should now read:% Lab 2: Build an integrate-and-fire model neuron and observe its spiking% for various levels of injected currentclear all; %clear all variablesclose all; %close all open figures%DEFINE PARAMETERSdt = 0.1; %time step [ms]t_end = 500; %total time of run [ms]t_StimStart = 100; %time to start injecting current [ms]t_StimEnd = 400; %time to end injecting current [ms]E_L = -70; %resting membrane potential [mV]V_th = -55; %spike threshold [mV]V_reset = -75; %value to reset voltage to after a spike [mV]V_spike = 20; %value to draw a spike to, when cell spikes [mV]R_m = 10; %membrane resistance [MOhm]tau = 10; %membrane time constant [ms]%DEFINE INITIAL VALUES AND VECTORS TO HOLD RESULTSt_vect = 0:dt:t_end; %will hold vector of timesV_vect = zeros(1,length(t_vect)); %initialize the voltage vector%initializing vectors makes your code run faster!V_plot_vect = zeros(1,length(t_vect)); %pretty version of V_vect to be plotted, that displays a spike % whenever voltage reaches thresholdi = 1; % index denoting which element of V is being assignedV_vect(i)= E_L; %first element of V, i.e. value of V at t=0V_plot_vect(i) = V_vect(i); %if no spike, then just plot the actual voltage V%INTEGRATE THE EQUATION tau*dV/dt = -V + E_L + I_e*R_mI_Stim = 1.55; %magnitude of pulse of injected current [nA]I_e_vect = zeros(1,t_StimStart/dt); %portion of I_e_vect from t=0 to t=t_StimStartI_e_vect = [I_e_vect I_Stim*ones(1,1+((t_StimEnd-t_StimStart)/dt))]; %add portion from% t=t_StimStart to t=t_StimEndI_e_vect = [I_e_vect zeros(1,(t_end-t_StimEnd)/dt)]; %add portion from% t=t_StimEnd to t=t_endNumSpikes = 0; %holds number of spikes that have occurredfor t=dt:dt:t_end %loop through values of t in steps of dt msV_inf = E_L + I_e_vect(i)*R_m; %value that V_vect is exponentially%decaying towards at this time step%next line does the integration update ruleV_vect(i+1) = V_inf + (V_vect(i) - V_inf)*exp(-dt/tau);%if statement below says what to do if voltage crosses thresholdif (V_vect(i+1) > V_th) %cell spikedV_vect(i+1) = V_reset; %set voltage back to V_resetV_plot_vect(i+1) = V_spike; %set vector that will be plotted to show a spike hereNumSpikes = NumSpikes + 1; %add 1 to the total spike countelse%voltage didn't cross threshold so cell does not spikeV_plot_vect(i+1) = V_vect(i+1); %plot the actual voltageendi = i + 1; %add 1 to index, corresponding to moving forward 1 time stependAveRate = 1000*NumSpikes/(t_StimEnd - t_StimStart) %gives average firing rate in [#/sec = Hz]%MAKE PLOTSfigure(1)plot(t_vect, V_plot_vect);title('Voltage vs. time');xlabel('Time in ms');ylabel('Voltage in mV');V. Step 3: Compare r isi,theory to r aveSave your work from the last section and then use Save As… to rename the file you are working on to something new (e.g. to IntAndFire3.m).Next we’d like to compare the theoretical value for the firing rate of the integrate-and-fire neuron r isi=1/t isi (equation (5)) to the value of r ave we calculated above. We’ll do this for several values of I_Stim (i.e. of I_e in equation (5)). How are we going to efficiently run our code for several different values of I_Stim? You guessed it…use another for loop!First, take a moment to indent all the lines below (but not including) I_Stim = 1.55 by selecting them and then clicking Text >> Increase Indent. This will make the following code more readable.Now let’s turn our code into a for loop by defining a vector of stimuli and then looping over it. Erase the I_Stim = 1.55 line and replace it by:I_Stim_vect = 1.43:0.04:1.63; %magnitudes of pulse of injected current [nA]for I_Stim = I_Stim_vect; %loop over different I_Stim valuesAlso add as the last line of your entire code:end %for I_StimThis is needed to finish the for loop. The code you just added will allow you to loop over 6 values of I_Stim from 1.43 to 1.63. Each time we loop we’re going to want to re-initialize the voltage vector and voltage plotting vector so cut and paste your previously typed lines:i = 1; % index denoting which element of V is being assignedV_vect(i)= E_L; %first element of V, i.e. value of V at t=0V_plot_vect(i) = V_vect(i); %if no spike, then just plot the actual voltage Vto be the first lines of your new for loop. Now we’re also going to want to make separate plots for each run so let’s define a variable PlotNum corresponding to the number of plots. Initialize PlotNum to zero above the for loop, and then have it increase by 1 every time we step through the for loop. Your %INTEGRATE THE EQUATION code should now start with:PlotNum=0;I_Stim_vect = 1.43:0.04:1.63;for I_Stim = I_Stim_vect; %magnitude of pulse of injected current [nA]PlotNum = PlotNum + 1;i = 1; % index denoting which element of V is being assignedV_vect(i)= E_L; %first element of V, i.e. value of V at t=0V_plot_vect(i) = V_vect(i); %if no spike, then just plot the actual voltage VNow let’s set up to make an array of plots: Below the command figure(1), make your code now read:subplot(length(I_Stim_vect),1,PlotNum)plot(t_vect, V_plot_vect);if (PlotNum == 1)title('Voltage vs. time');endif (PlotNum == length(I_Stim_vect))xlabel('Time in ms');endylabel('Voltage in mV');end %for I_StimThe subplot command sets up an array of plots with length(I_Stim_vect) rows and 1 column. The if statements make the title only plot above the first subplot and the x-axis label only plot below the last plot. Try running your code now. This should produce the following panels, the first 2 with no spikes and the latter ones with increasing numbers of spikes:This should look like the effect of increasing light intensity on the spiking of neurons of the eye in Hartline’s paper! In this context, we are assuming that the effect of increasing light is simply to increase the current injected into the neuron and thereby to increase its firing rate.Now let’s add another figure that plots the theoretical firing rate vs. I_e curve for values of I_e above firing rate threshold I_threshold = (V_th - E_L)/R_m. This is easily done by defining a vector of injected currents (let’s call it I_vect_long since it will contain many points) and then typing in the ugly formula for r isi from equation (5)). If we want to plot from just aboveI_threshold to I_e = 1.8 in fine steps of 0.001, the code is (add to end of your code):%COMPARE R_AVE TO R_ISII_threshold = (V_th - E_L)/R_m; %current below which cell does not fireI_vect_long = (I_threshold+0.001):0.001:1.8; %vector of injected current for producing theory plotr_isi = 1000./(tau*log((V_reset - E_L - I_vect_long*R_m)./(V_th - E_L - I_vect_long*R_m)));figure(2)plot(I_vect_long,r_isi)title('Comparison of r_{isi} vs. I_e and r_{ave} vs. I_e')xlabel('Injected current (nA)')ylabel('r_{isi} or r_{ave} (Hz)')。
- 1、下载文档前请自行甄别文档内容的完整性,平台不提供额外的编辑、内容补充、找答案等附加服务。
- 2、"仅部分预览"的文档,不可在线预览部分如存在完整性等问题,可反馈申请退款(可完整预览的文档不适用该条件!)。
- 3、如文档侵犯您的权益,请联系客服反馈,我们会尽快为您处理(人工客服工作时间:9:00-18:30)。
Abstract
The response of model neurons driven by a periodic current coi~vergesonto mode-locked attractors. Reliability, defined as the noise stability of the attractor, was studied as a function of the drive frequency and noise strength. For weak noise, the neuron remained on one attractor and reliability was high. For intermediate noise strength, transitioas between attractors occurred. For strong noise, mode locking became unstable. The attractor was most stable for frequencies for which the neuron produced one spike on each cycle. The prediction of a reliability resonance as a function of drive frequency has been observed in vitro in cortical neurons. @ 2002 Published by Elsevier Science B.V.
+
+
0925-2312/02/$-see front matter @ 2002 Published by Elsevier Science B.V. Pll: S O 9 2 5 - 2 3 1 2 ( 0 2 ) 0 0 3 9 0 - 9
If a feature is present in the spike train response to one stiinulus across multiple trials it can fo1-111 the basis of a neuroi~alcode. Spike-time reliability is a measure for the reproducibility of individual spike times [6]. Neurons produce a reliable sequence of spike times in response to some inputs and respond uixeliably to others. In vitro, neurons fire reliably when injected with a raildoin current containing high-frequency components, but they fire unreliably when driven with a low-pass or constant current [2,6]. Sinusoidally driven neurons show resonances in reliability as a fuilction of drive frequency [3,4]. Our aim is to understand these experimental results within the framework of noise stability of attractors.
+ +
+
3. Results
The paraineters of an integrate-adfire neuron driven by a sinusoidal current were chosen such that the neuron produced on average one spike per two cycles of the driving current (Fig. 1). From different initial voltages the neuron converged to one of the two different voltage trajectories (Fig. IA and B), yielding two different sequences of spike times (not shown). The two solutioils are related: when one solution is shifted by one cycle, the other solution is obtained. There were two attractors. Attractor 1, when the neuron spikes on odd cycles, was obtained starting from 0.78 d Knit < 0.98, here Villit was the voltage at the start of the simulation. Attractor 2, when the neuron spikes on even cycles, was obtained starting from 0.0 < Vinit < 0.78 and 0.98 d 6,it < 1.0.
ELSEVIER
Spike-time reliability of periodically driven integrate-and-fire neurons fi
P.H.E. Tiesinga*, J.-M. Fellous, T.J. Sejnowski
Sloun-Swurtz Center jor Theoreticul Neurobiology, Conzpututionul Neurobiology Lub, und Ho~vurd Hughes Medicul Institute, The Sulk Institute, 10010 N. Torrey Pines Rd, Lu Jollu, CA 92037, USA
Kejwords: Neural code; Oscillation; Phase locking; Precisiou
1. Introduction
Although spike trains in the cerebral cortex are highly variable (for a review see [lo]), neurons can fire with high temporal precision and reliability in vitro [1,5,8]. Precision is defined here as the inverse of the temporal jitter in the spike time and reliability as the reprod~~cibility spikes across trials. Infoimation-theoretical analyses of of the neuronal spike trains in the lateral geniculate nucleus indicate that precise spike times contain more infomation about the input than firing ratห้องสมุดไป่ตู้ alone [9]. It is u11know11 how these precise spike times are used in the cortex.
2. Methods
The membrane potential V of an integrate-aidfire neuron driven by a fluctuating current satisfied dV/dt = -V I f (t) t(t), where I was the time-independent driving cussent, f (t) the fluctuating current and t was a white noise current, wit11 zero mean and variance D, that represented the effects of intrinsic noise. When the voltage V reached tllreshold, V(t) = 1, a spike was emitted and the voltage was reset to zero. Diinensioilless units were used: 1 voltage unit was the distance between resting membrane potential and action potential threshold, approximately 20 inV; one time unit corresponded to the membrane tiine constant, approximately 10-40 ins. The periodic current was either sinusoidal, f (t) = A sin o t , or a periodic piecewise coilstant current equal to f ( t ) = -A when 0 < inod(t, T) < T/2 and f(t)=A otherwise. Here, A was the amplitude of the diive, T the period, and the frequency w = 2nlT. For the sinusoidal cussent, the differential equation was integrated directly using the fourth-order RungeKutta algorithm [7] with step size dt = 0.01. For the piecewise constant current an ai~alytical spike-time map, t' =M(t), was derived [ll], here t and t' were the previous and new spike time, respectively. Spike times were generated by iterating this map. The spike phase of the nth spike tiine t,, was $,, = inod(t,,, T)/T. The mean phase, Y,,, = ( / ,~!:~ pN) r!was deternilled for periodic (!,, time series, here p was the period, nz = 1, . . ., y , and N, was the total number of spikes.