Integrating data from biological experiments into metabolic networks with the DBE informati
资料科学基础英文版课件
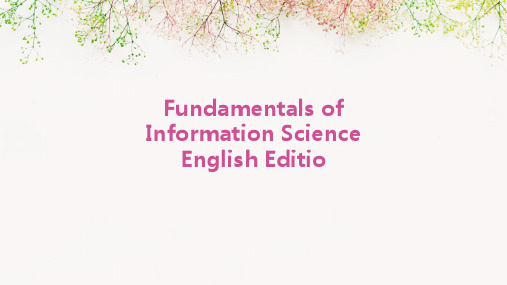
Semi supervised learning is a type of machine learning that combines both labeled and unlabeled data for training
04
Spread: Standard deviation, variation
05
Shape: Skewness, kurtosis
06
Relationships: Correlation, regression
01
02
03
04
05
45%
50%
75%
85%
95%
Purpose: Draw conclusions about population from sample data
Overview of Data Collection and Sampling: In data science, data collection and sampling are important steps in obtaining data for analysis and modeling.
Data Science also helps in identifying risks, reducing uncertainties, and making better predictions about future trends and outcomes
The field of Data Science has evolved over the years, starting from the early days of data management and analysis to the current era of big data, machine learning, and artificial intelligence
生物信息学英文介绍
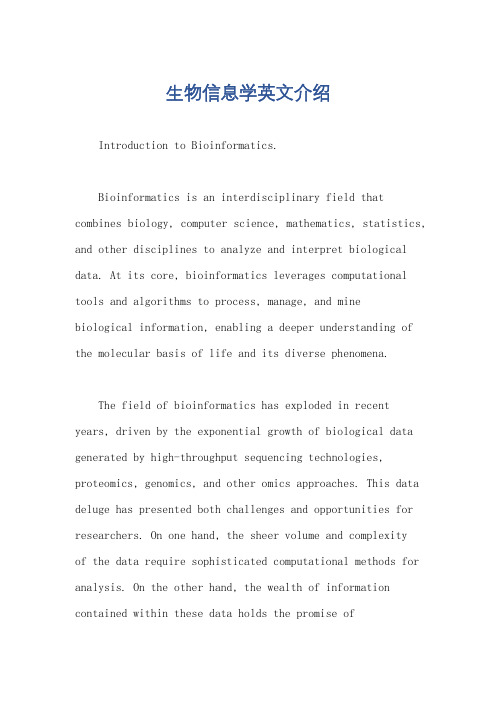
生物信息学英文介绍Introduction to Bioinformatics.Bioinformatics is an interdisciplinary field that combines biology, computer science, mathematics, statistics, and other disciplines to analyze and interpret biological data. At its core, bioinformatics leverages computational tools and algorithms to process, manage, and minebiological information, enabling a deeper understanding of the molecular basis of life and its diverse phenomena.The field of bioinformatics has exploded in recent years, driven by the exponential growth of biological data generated by high-throughput sequencing technologies, proteomics, genomics, and other omics approaches. This data deluge has presented both challenges and opportunities for researchers. On one hand, the sheer volume and complexityof the data require sophisticated computational methods for analysis. On the other hand, the wealth of information contained within these data holds the promise oftransformative insights into the functions, interactions, and evolution of biological systems.The core tasks of bioinformatics encompass genome annotation, sequence alignment and comparison, gene expression analysis, protein structure prediction and function annotation, and the integration of multi-omic data. These tasks require a range of computational tools and algorithms, often developed by bioinformatics experts in collaboration with biologists and other researchers.Genome annotation, for example, involves the identification of genes and other genetic elements within a genome and the prediction of their functions. This process involves the use of bioinformatics algorithms to identify protein-coding genes, non-coding RNAs, and regulatory elements based on sequence patterns and other features. The resulting annotations provide a foundation forunderstanding the genetic basis of traits and diseases.Sequence alignment and comparison are crucial for understanding the evolutionary relationships betweenspecies and for identifying conserved regions within genomes. Bioinformatics algorithms, such as BLAST and multiple sequence alignment tools, are widely used for these purposes. These algorithms enable researchers to compare sequences quickly and accurately, revealing patterns of conservation and divergence that inform our understanding of biological diversity and function.Gene expression analysis is another key area of bioinformatics. It involves the quantification of thelevels of mRNAs, proteins, and other molecules within cells and tissues, and the interpretation of these data to understand the regulation of gene expression and its impact on cellular phenotypes. Bioinformatics tools and algorithms are essential for processing and analyzing the vast amounts of data generated by high-throughput sequencing and other experimental techniques.Protein structure prediction and function annotation are also important areas of bioinformatics. The structure of a protein determines its function, and bioinformatics methods can help predict the three-dimensional structure ofa protein based on its amino acid sequence. These predictions can then be used to infer the protein'sfunction and to understand how it interacts with other molecules within the cell.The integration of multi-omic data is a rapidly emerging area of bioinformatics. It involves theintegration and analysis of data from different omics platforms, such as genomics, transcriptomics, proteomics, and metabolomics. This approach enables researchers to understand the interconnectedness of different biological processes and to identify complex relationships between genes, proteins, and metabolites.In addition to these core tasks, bioinformatics also plays a crucial role in translational research and personalized medicine. It enables the identification of disease-associated genes and the development of targeted therapeutics. By analyzing genetic and other biological data from patients, bioinformatics can help predict disease outcomes and guide treatment decisions.The future of bioinformatics is bright. With the continued development of high-throughput sequencing technologies and other omics approaches, the amount of biological data available for analysis will continue to grow. This will drive the need for more sophisticated computational methods and algorithms to process and interpret these data. At the same time, the integration of bioinformatics with other disciplines, such as artificial intelligence and machine learning, will open up new possibilities for understanding the complex systems that underlie life.In conclusion, bioinformatics is an essential field for understanding the molecular basis of life and its diverse phenomena. It leverages computational tools and algorithms to process, manage, and mine biological information, enabling a deeper understanding of the functions, interactions, and evolution of biological systems. As the amount of biological data continues to grow, the role of bioinformatics in research and medicine will become increasingly important.。
由大数据到智能医学
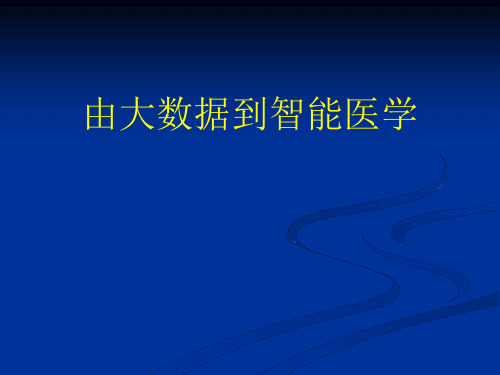
Biomedical big data
Biological data Medical data Real time physiological and pathological
date
Biological Data
Standardizing experimental protocols
Current Opinion in Biotechnology 19:354-359(2008)
Standardization at multiple levels is essential.
One of the key issues is to obtain highly reproducible quantitative data for mathemn of hypothesis-driven research in systems biology
Current Opinion in Biotechnology 19:354-359(2008)
java英文参考文献
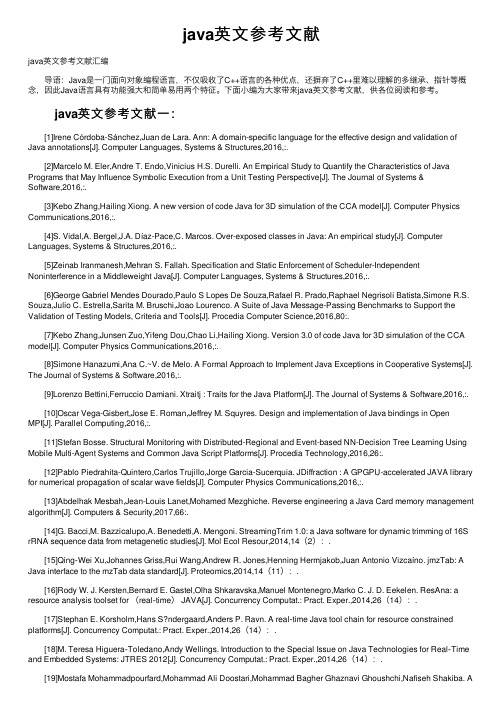
java英⽂参考⽂献java英⽂参考⽂献汇编 导语:Java是⼀门⾯向对象编程语⾔,不仅吸收了C++语⾔的各种优点,还摒弃了C++⾥难以理解的多继承、指针等概念,因此Java语⾔具有功能强⼤和简单易⽤两个特征。
下⾯⼩编为⼤家带来java英⽂参考⽂献,供各位阅读和参考。
java英⽂参考⽂献⼀: [1]Irene Córdoba-Sánchez,Juan de Lara. Ann: A domain-specific language for the effective design and validation of Java annotations[J]. Computer Languages, Systems & Structures,2016,:. [2]Marcelo M. Eler,Andre T. Endo,Vinicius H.S. Durelli. An Empirical Study to Quantify the Characteristics of Java Programs that May Influence Symbolic Execution from a Unit Testing Perspective[J]. The Journal of Systems & Software,2016,:. [3]Kebo Zhang,Hailing Xiong. A new version of code Java for 3D simulation of the CCA model[J]. Computer Physics Communications,2016,:. [4]S. Vidal,A. Bergel,J.A. Díaz-Pace,C. Marcos. Over-exposed classes in Java: An empirical study[J]. Computer Languages, Systems & Structures,2016,:. [5]Zeinab Iranmanesh,Mehran S. Fallah. Specification and Static Enforcement of Scheduler-Independent Noninterference in a Middleweight Java[J]. Computer Languages, Systems & Structures,2016,:. [6]George Gabriel Mendes Dourado,Paulo S Lopes De Souza,Rafael R. Prado,Raphael Negrisoli Batista,Simone R.S. Souza,Julio C. Estrella,Sarita M. Bruschi,Joao Lourenco. A Suite of Java Message-Passing Benchmarks to Support the Validation of Testing Models, Criteria and Tools[J]. Procedia Computer Science,2016,80:. [7]Kebo Zhang,Junsen Zuo,Yifeng Dou,Chao Li,Hailing Xiong. Version 3.0 of code Java for 3D simulation of the CCA model[J]. Computer Physics Communications,2016,:. [8]Simone Hanazumi,Ana C.~V. de Melo. A Formal Approach to Implement Java Exceptions in Cooperative Systems[J]. The Journal of Systems & Software,2016,:. [9]Lorenzo Bettini,Ferruccio Damiani. Xtraitj : Traits for the Java Platform[J]. The Journal of Systems & Software,2016,:. [10]Oscar Vega-Gisbert,Jose E. Roman,Jeffrey M. Squyres. Design and implementation of Java bindings in OpenMPI[J]. Parallel Computing,2016,:. [11]Stefan Bosse. Structural Monitoring with Distributed-Regional and Event-based NN-Decision Tree Learning Using Mobile Multi-Agent Systems and Common Java Script Platforms[J]. Procedia Technology,2016,26:. [12]Pablo Piedrahita-Quintero,Carlos Trujillo,Jorge Garcia-Sucerquia. JDiffraction : A GPGPU-accelerated JAVA library for numerical propagation of scalar wave fields[J]. Computer Physics Communications,2016,:. [13]Abdelhak Mesbah,Jean-Louis Lanet,Mohamed Mezghiche. Reverse engineering a Java Card memory management algorithm[J]. Computers & Security,2017,66:. [14]G. Bacci,M. Bazzicalupo,A. Benedetti,A. Mengoni. StreamingTrim 1.0: a Java software for dynamic trimming of 16S rRNA sequence data from metagenetic studies[J]. Mol Ecol Resour,2014,14(2):. [15]Qing‐Wei Xu,Johannes Griss,Rui Wang,Andrew R. Jones,Henning Hermjakob,Juan Antonio Vizcaíno. jmzTab: A Java interface to the mzTab data standard[J]. Proteomics,2014,14(11):. [16]Rody W. J. Kersten,Bernard E. Gastel,Olha Shkaravska,Manuel Montenegro,Marko C. J. D. Eekelen. ResAna: a resource analysis toolset for (real‐time) JAVA[J]. Concurrency Computat.: Pract. Exper.,2014,26(14):. [17]Stephan E. Korsholm,Hans S?ndergaard,Anders P. Ravn. A real‐time Java tool chain for resource constrained platforms[J]. Concurrency Computat.: Pract. Exper.,2014,26(14):. [18]M. Teresa Higuera‐Toledano,Andy Wellings. Introduction to the Special Issue on Java Technologies for Real‐Time and Embedded Systems: JTRES 2012[J]. Concurrency Computat.: Pract. Exper.,2014,26(14):. [19]Mostafa Mohammadpourfard,Mohammad Ali Doostari,Mohammad Bagher Ghaznavi Ghoushchi,Nafiseh Shakiba. Anew secure Internet voting protocol using Java Card 3 technology and Java information flow concept[J]. Security Comm. Networks,2015,8(2):. [20]Cédric Teyton,Jean‐Rémy Falleri,Marc Palyart,Xavier Blanc. A study of library migrations in Java[J]. J. Softw. Evol. and Proc.,2014,26(11):. [21]Sabela Ramos,Guillermo L. Taboada,Roberto R. Expósito,Juan Touri?o. Nonblocking collectives for scalable Java communications[J]. Concurrency Computat.: Pract. Exper.,2015,27(5):. [22]Dusan Jovanovic,Slobodan Jovanovic. An adaptive e‐learning system for Java programming course, based on Dokeos LE[J]. Comput Appl Eng Educ,2015,23(3):. [23]Yu Lin,Danny Dig. A study and toolkit of CHECK‐THEN‐ACT idioms of Java concurrent collections[J]. Softw. Test. Verif. Reliab.,2015,25(4):. [24]Jonathan Passerat?Palmbach,Claude Mazel,David R. C. Hill. TaskLocalRandom: a statistically sound substitute to pseudorandom number generation in parallel java tasks frameworks[J]. Concurrency Computat.: Pract.Exper.,2015,27(13):. [25]Da Qi,Huaizhong Zhang,Jun Fan,Simon Perkins,Addolorata Pisconti,Deborah M. Simpson,Conrad Bessant,Simon Hubbard,Andrew R. Jones. The mzqLibrary – An open source Java library supporting the HUPO‐PSI quantitative proteomics standard[J]. Proteomics,2015,15(18):. [26]Xiaoyan Zhu,E. James Whitehead,Caitlin Sadowski,Qinbao Song. An analysis of programming language statement frequency in C, C++, and Java source code[J]. Softw. Pract. Exper.,2015,45(11):. [27]Roberto R. Expósito,Guillermo L. Taboada,Sabela Ramos,Juan Touri?o,Ramón Doallo. Low‐latency Java communication devices on RDMA‐enabled networks[J]. Concurrency Computat.: Pract. Exper.,2015,27(17):. [28]V. Serbanescu,K. Azadbakht,F. Boer,C. Nagarajagowda,B. Nobakht. A design pattern for optimizations in data intensive applications using ABS and JAVA 8[J]. Concurrency Computat.: Pract. Exper.,2016,28(2):. [29]E. Tsakalos,J. Christodoulakis,L. Charalambous. The Dose Rate Calculator (DRc) for Luminescence and ESR Dating-a Java Application for Dose Rate and Age Determination[J]. Archaeometry,2016,58(2):. [30]Ronald A. Olsson,Todd Williamson. RJ: a Java package providing JR‐like concurrent programming[J]. Softw. Pract. Exper.,2016,46(5):. java英⽂参考⽂献⼆: [31]Seong‐Won Lee,Soo‐Mook Moon,Seong‐Moo Kim. Flow‐sensitive runtime estimation: an enhanced hot spot detection heuristics for embedded Java just‐in‐time compilers [J]. Softw. Pract. Exper.,2016,46(6):. [32]Davy Landman,Alexander Serebrenik,Eric Bouwers,Jurgen J. Vinju. Empirical analysis of the relationship between CC and SLOC in a large corpus of Java methods and C functions[J]. J. Softw. Evol. and Proc.,2016,28(7):. [33]Renaud Pawlak,Martin Monperrus,Nicolas Petitprez,Carlos Noguera,Lionel Seinturier. SPOON : A library for implementing analyses and transformations of Java source code[J]. Softw. Pract. Exper.,2016,46(9):. [34]Musa Ata?. Open Cezeri Library: A novel java based matrix and computer vision framework[J]. Comput Appl Eng Educ,2016,24(5):. [35]A. Omar Portillo‐Dominguez,Philip Perry,Damien Magoni,Miao Wang,John Murphy. TRINI: an adaptive load balancing strategy based on garbage collection for clustered Java systems[J]. Softw. Pract. Exper.,2016,46(12):. [36]Kim T. Briggs,Baoguo Zhou,Gerhard W. Dueck. Cold object identification in the Java virtual machine[J]. Softw. Pract. Exper.,2017,47(1):. [37]S. Jayaraman,B. Jayaraman,D. Lessa. Compact visualization of Java program execution[J]. Softw. Pract. Exper.,2017,47(2):. [38]Geoffrey Fox. Java Technologies for Real‐Time and Embedded Systems (JTRES2013)[J]. Concurrency Computat.: Pract. Exper.,2017,29(6):. [39]Tórur Biskopst? Str?m,Wolfgang Puffitsch,Martin Schoeberl. Hardware locks for a real‐time Java chip multiprocessor[J]. Concurrency Computat.: Pract. Exper.,2017,29(6):. [40]Serdar Yegulalp. JetBrains' Kotlin JVM language appeals to the Java faithful[J]. ,2016,:. [41]Ortin, Francisco,Conde, Patricia,Fernandez-Lanvin, Daniel,Izquierdo, Raul. The Runtime Performance of invokedynamic: An Evaluation with a Java Library[J]. IEEE Software,2014,31(4):. [42]Johnson, Richard A. JAVA DATABASE CONNECTIVITY USING SQLITE: A TUTORIAL[J]. Allied Academies International Conference. Academy of Information and Management Sciences. Proceedings,2014,18(1):. [43]Trent, Rod. SQL Server Gets PHP Support, Java Support on the Way[J]. SQL Server Pro,2014,:. [44]Foket, C,De Sutter, B,De Bosschere, K. Pushing Java Type Obfuscation to the Limit[J]. IEEE Transactions on Dependable and Secure Computing,2014,11(6):. [45]Parshall, Jon. Rising Sun, Falling Skies: The Disastrous Java Sea Campaign of World War II[J]. United States Naval Institute. Proceedings,2015,141(1):. [46]Brunner, Grant. Java now pollutes your Mac with adware - here's how to uninstall it[J]. ,2015,:. [47]Bell, Jonathan,Melski, Eric,Dattatreya, Mohan,Kaiser, Gail E. Vroom: Faster Build Processes for Java[J]. IEEE Software,2015,32(2):. [48]Chaikalis, T,Chatzigeorgiou, A. Forecasting Java Software Evolution Trends Employing Network Models[J]. IEEE Transactions on Software Engineering,2015,41(6):. [49]Lu, Quan,Liu, Gao,Chen, Jing. Integrating PDF interface into Java application[J]. Library Hi Tech,2014,32(3):. [50]Rashid, Fahmida Y. Oracle fixes critical flaws in Database Server, MySQL, Java[J]. ,2015,:. [51]Rashid, Fahmida Y. Library misuse exposes leading Java platforms to attack[J]. ,2015,:. [52]Rashid, Fahmida Y. Serious bug in widely used Java app library patched[J]. ,2015,:. [53]Odeghero, P,Liu, C,McBurney, PW,McMillan, C. An Eye-Tracking Study of Java Programmers and Application to Source Code Summarization[J]. IEEE Transactions on Software Engineering,2015,41(11):. [54]Greene, Tim. Oracle settles FTC dispute over Java updates[J]. Network World (Online) [55]Rashid, Fahmida Y. FTC ruling against Oracle shows why it's time to dump Java[J]. ,2015,:. [56]Whitwam, Ryan. Google plans to remove Oracle's Java APIs from Android N[J]. ,2015,:. [57]Saher Manaseer,Warif Manasir,Mohammad Alshraideh,Nabil Abu Hashish,Omar Adwan. Automatic Test Data Generation for Java Card Applications Using Genetic Algorithm[J]. Journal of Software Engineering andApplications,2015,8(12):. [58]Paul Venezia. Prepare now for the death of Flash and Java plug-ins[J]. ,2016,:. [59]PW McBurney,C McMillan. Automatic Source Code Summarization of Context for Java Methods[J]. IEEE Transactions on Software Engineering,2016,42(2):. java英⽂参考⽂献三: [61]Serdar Yegulalp,Serdar Yegulalp. Sputnik automates code review for Java projects on GitHub[J].,2016,:. [62]Fahmida Y Rashid,Fahmida Y Rashid. Oracle security includes Java, MySQL, Oracle Database fixes[J]. ,2016,:. [63]H M Chavez,W Shen,R B France,B A Mechling. An Approach to Checking Consistency between UML Class Model and Its Java Implementation[J]. IEEE Transactions on Software Engineering,2016,42(4):. [64]Serdar Yegulalp,Serdar Yegulalp. Unikernel power comes to Java, Node.js, Go, and Python apps[J]. ,2016,:. [65]Yudi Zheng,Stephen Kell,Lubomír Bulej,Haiyang Sun. Comprehensive Multiplatform Dynamic Program Analysis for Java and Android[J]. IEEE Software,2016,33(4):. [66]Fahmida Y Rashid,Fahmida Y Rashid. Oracle's monster security fixes Java, database bugs[J]. ,2016,:. [67]Damian Wolf,Damian Wolf. The top 5 Java 8 features for developers[J]. ,2016,:. [68]Jifeng Xuan,Matias Martinez,Favio DeMarco,Maxime Clément,Sebastian Lamelas Marcote,Thomas Durieux,Daniel LeBerre. Nopol: Automatic Repair of Conditional Statement Bugs in Java Programs[J]. IEEE Transactions on Software Engineering,2017,43(1):. [69]Loo Kang Wee,Hwee Tiang Ning. Vernier caliper and micrometer computer models using Easy Java Simulation and its pedagogical design features-ideas for augmenting learning with real instruments[J]. Physics Education,2014,49(5):. [70]Loo Kang Wee,Tat Leong Lee,Charles Chew,Darren Wong,Samuel Tan. Understanding resonance graphs using Easy Java Simulations (EJS) and why we use EJS[J]. Physics Education,2015,50(2):.【java英⽂参考⽂献汇编】相关⽂章:1.2.3.4.5.6.7.8.。
Integration of Diverse Knowledge and Data into Biomedical Knowledge Matrices

Integration of Diverse Knowledge and Datainto Biomedical Knowledge MatricesYoshimasa Tsuruoka1Teruyoshi Hishiki2Osamu Ogasawara3Kousaku Okubo4 1CREST,JST(Japan Science and Technology Corporation)tsuruoka@is.s.u-tokyo.ac.jp2Biological Information Research Center,National Institute of Advanced Industrial Science and Technology(AIST)t-hishiki@jbirc.aist.go.jp3Information and Mathematical Science Laboratoryoogasawa@v001.vaio.ne.jp4National Institute of Geneticskousaku@AbstractAfter the accomplishment of human draftsequence,more and more efforts are beingmade in the mapping of the data-drivenpatterns to background knowledge,hop-ing to efficiently produce hypotheses outof theflood of data.Here we propose aframework of biomedical data and knowl-edge that has a high adaptability to theautomated data interpretation.Then,weshow that biomedical databases with het-erogeneous scopes and structures can beconverted to the format,and possible rolesof ontology of biomedical objects com-bined with natural language processingstly,we present applica-tions of formatted biomedical knowledgeto scientific discovery.1IntroductionAfter the accomplishment of human draft genome sequence(Lander,E.S.et al.,2001),systematic pre-diction of gene functions(Marcotte et al.,1999)is one of the major goals in biomedicine.Toward this goal,high-throughput measurement of gene(pro-tein)features such as expression profiles(Iyer,V. R.et al.,99;Velculescu,V.E.et al.,1999;Hsiao, L.L.et al.,2001;Su,2001)and protein-protein in-teractions(Ito,T.et al.,2001;Ho,Y.et al.,2002) has become a trend,and data is generated at an un-precedented rate.It is clear that hypothesis creation on the roles played by genes with systematic and in-tegrative approaches(Scherf,U.et al.,2000;Green-baum,D.et al.,2001)to collected data is anticipated as the next goal.Thefirst step toward that goal was the representa-tion of measurement data,the extraction of global patterns from the data(Eisen,M.B.et al.,1998; Ge et al.,2001;Bussemaker et al.,2001;Rives and Galitski,2003),and the visualization of the results (Gilbert et al.,2000).Here we summarize the mea-surement data into only two formats.One is the‘fea-ture array’,or an array of features and their values for a type of biological object,e.g.genes and cells, and the matrix as a collection of the arrays.For ex-ample,the structural database(Apweiler,R.et al., 2001)is a collection of genes with features such as protein families,domains,and functional sites. Another example is the expression profiles data set, which is a matrix of genes and tissues with each cell representing relative or absolute abundance of cog-nate binations of two types of mea-surement data by making the product of the matri-ces can give a prediction of a new type of relation (Scherf,U.et al.,2000).The other format is the ‘gene-gene correlation/similarity matrix’represent-ing the strength of relations in all-to-all gene pairs. Some of the methods produce directly this type of data;on the other hand,the gene‘feature arrays’can be transformed to this type of data by calculating the correlations between the feature values of all-to-all gene pairs.Now,more and more efforts are being made in the second step,or mapping the data-driven patterns to the integrated background knowledge e.g.bio-chemistry,cell biology,pathology,and medicine.A problem here is to allocate authentic features to biological objects,and the efforts to work col-laboratively to build controlled and classified vo-cabularies(Ogata,H.et al.,1999;Ashburner,M. et al.,2000)for molecular localization,actions, and roles,and then to assign them to genes(Xie, H.,2002;Camon,E.et al.,2003)are being pro-moted.Some of the controlled vocabularies are called‘ontology’because they have manually edited relations representing e.g.‘Is-a’and‘Part-of’re-lations,connecting the objects in a tree or net-work structure.By extending the scope of biolog-ical ontologies,e.g.relations between anatomical structures and tissues constituting them as repre-sented in TissueDB(http://tissuedb.ontology.ims.u-tokyo.ac.jp),more diverse problems like matching tissues between expression profiling platforms will become easier.We argue that there is another aspect of the in-tegration problem:the format of data and knowl-edge to be interrelated with each other.The net-work representation of the relations between objects is widely used,and an experiment(Jenssen et al., 2001)extracted the networks between related genes using the co-occurrence frequency of gene symbols in MEDLINE articles.However,no study using such representation has successfully combined data and knowledge into a global view of a new type of relations as presented in the combination of genome-wide measurement data(Scherf,U.et al.,2000). What we will propose as the common data format will enable calculating the knowledge as well as the genome-wide measurement data,and will integrate the data and knowledge toward a discovery.2Grand DesignThe databases we are planning to integrate are as follows.Major human gene-centred databases(Ref-Seq/Locuslink(Pruitt and Maglott,2001),SWISS-PROT(Boeckmann,B.et al.,2003))will provide structural features of the genes to be extracted with sequence analysis as well as relations to other types of objects(e.g.cells,tissues,and their activi-ties)in the form of text-formatted comments.The gene-centred databases for model organisms(Fly-base(The FlyBase Consortium,2003)for fruitflies) will give information of conserved genes that may also have an important role in human.The dis-ease database(OMIM(Hamosh et al.,2002))may give a basis for molecular-based diagnosis combined with measurement data,e.g.expression patterns. The pathway database(KEGG(Ogata,H.et al., 1999))and Gene Ontology(Ashburner,M.et al., 2000)will be used to enrich functional features of genes,and major textbooks in biomedical sciences will be used to anchor a variety of data and knowl-edge.In addition,we will incorporate a data set of13,543human gene expression profiles across 71normal human tissues(H-invitational data set, an international gene annotation conference held in 2002/8/25-2002/9/3)for integration with those types of knowledge.One of the representational character-istics of the data is the‘tissue distribution pattern’calculated as follows:1,994RNA sources in the data set were classified into10practical tissue cat-egories and the category-mean concentrations were computed.We will also extract relationship as fol-lows from the MEDLINE articles on PubMed:those between diseases and their clinical manifestations and those between genes and cell/tissue types.We introduce appropriate representations of knowledge that can be as computable as that of data,and would work in the integration of data and knowledge.The targets to which they will be ap-plied include,but are not restricted to,the databases listed above.As the studies on diagnostic reason-ing(Joseph and Patel,1990)clarified,experts and novices differ in the way they conceive links be-tween given information and between invoked ideas, leading to different ability in generating and elimi-nating alternative hypotheses.Therefore,we may well define biomedical knowledge as recognition of relations between biomedical terms.This definition will justify the adoption of the second data format, the‘object-object correlation/similarity matrix’.We may well adopt thefirst format,the‘feature array’to form the basis of the correlation matrix.With those formats,various types of knowledge buried in existing databases can be represented. Some of the knowledge can be extracted with no advanced processing(e.g.sequence elements an-notated in the database,and reference to other databases’objects),and others need specific pro-cessing(e.g.sequence elements yet to be anno-tated in the database).However,most of the rela-Figure1:Representation and computation of data and knowledge.See‘Grand Design’section for detailstions are written in the text format with various lev-els of abstraction.An example of the higher-level abstraction is the statement like‘SUBCELLULAR LOCA TION Secreted’,a part of Comment section in SWISS-PROT,consisting of a feature(SUBCEL-LULAR LOCATION)and the value(Secreted).In this case,both the feature and the value are from a controlled vocabulary.The OMIM Clinical Synop-sis(CS)field is a feature for a phenotype having a hierarchy consisting of sub-fields categorized by the type of heredity,the affected organs,or the affected systems(119categories written in loosely controlled terms,and can be merged to about40categories), and under the sub-fields are about22,000leaf de-scriptions for clinical manifestations.They are com-posite phrase of medical terms,with about18,000 descriptions appear only once,and the analysis of the structure and the summarization of the descrip-tions would be useful.An example of the lower-level abstraction is‘FUNCTION:it induces nerve cells differentiation’,also taken seen in the Com-ment section of SWISS-PROT.Very basic natural language processing(NLP)would be necessary to extract the possible feature value(‘nerve cells differ-entiation’)for the feature(FUNCTION);moreover, the feature value may not be included in a controlled vocabulary,requiring matching of the meanings for stly,the lowest-level abstraction is the free text format including most of the OMIM fields and MEDLINE articles.To cope with the textual descriptions,we will apply NLP resources as follows to the tasks: vocabularies from Unified Medical Language System(UMLS(Lindberg,1990))subsum-ing International Classification of Diseases (ICD)10(http://www.who.int/whosis/icd10/), a disease classification;textbook index terms;GENA(http://gena.ontology.ims.u-tokyo.ac.jp/search/servlet/gena),a vocabulary of gene names;Gene Ontology as one of the controlled vocabularies of gene functions;and GE-NIA(http://www-tsujii.is.u-tokyo.ac.jp/GENIA/), a biomedical and linguistic tagged corpus,to test rules to be applied to the extraction of relations between objects.These vocabularies combined with NLP techniques will extract the target objects,and co-occurrence relations between features for an object and the feature value,or between two types of objects will be calculated;then,those relations will be converted to either format:feature array or object-object correlation matrix.Once the feature arrays are calculated,they can be converted to the object-object correlation matrix.For the calculation of matrices,several sets of ontology will be neces-sary to match the items in rows or columns between the matrices.In addition to TissueDB,we may useGene Ontology to match terms representing gene functions,and UMLS to match terms representing diseases.All those processes are described in Figure 1.3Encoding3.1Disease vs Clinical ManifestationsWe describe extracted relationships between dis-eases and their symptoms(clinical manifestations) from the MEDLINE database.In this study,we adopt a simply assumption that the frequency of the co-occurrence between disease names in titles and symptom names in abstracts indicates their strength of association.We have conducted experiments using the whole MEDLINE database as of August2002containing about12,000,000abstracts.The dictionaries were constructed from the UMLS Metathesaurus.The disease and symptom name dictionary were con-structed by gathering the terms having the semantice type of“Disease or Syndrome”and“Sign or Symp-tom”respectively.Since we adopt a simple longest matching algorithm for term detection,Common Engligh words such as“signs”and“other”were ex-cluded from the dictinoaries to avoid false recogni-tions.The number of unique diseases that appeared in the titles was6,586and that of unique symptoms was1,083.We can thus construct a6,586x1,083 matrix from the extracted pairs.Each element in the matrix represents the frequency of the co-occurrence between the corresponding disease and symptom.A disease can be represented in the form of a vector on the corresponding row.In order to evaluate the validity of the represen-tation of diseases by our method,we compared the similarities between diseases,which are computed as the cosine value between the vectors,with those computed by using International Classification of Disease(ICD10),which is a manually constructed disease classification.Since diseases are classified in a tree-like structure in ICD10,we define the dis-tance of two disease on ICD10as the number of steps along the shortest path from one disease to the other.Table1shows the relationship between the distance measured on ICD10and the average simi-larity of vectors.The results show a clear negative Table1:Relationship between Distance on ICD10 and Vector SimilarityVector Similarity0.7620.4040.2060.1680.18Figure2:Result of Clustering correlation between them,which suggests that these two data provide similar information as for the sim-ilarity(or dissimilarity)of two diseases.We next performed hierarchical clustering using the average-linkage criterion.Figure2shows the re-sult in the form of a visualization of the similarity matrix.Both the rows and the columns corresond to the diseases which are sorted in the order of the clustering.The intensity of each point represents the similarity of the two corresponding diseases.The more similary they are,the brighter the point is. Therefore,the points on the diagonal are of max-imal intensity because every point on the diagonal represents the similarity of identical diseases.The vague squares scattered along the diagonal indicate the existence of clusters of similar diseases.A part of the clustering result is shown in Figure 3in the form of a dendrogram.We can see that the diseases accompanied by seizures are merged by the clustering method.Epilepsy Epilepsy empo l es Epilept ic sEpilepsies,ia lplex pa ia l seiz es lsio s ine ewio en ic eiz esen st syn eEplepsy,seneFigure 3:Part of Clustering Result3.2Extracting OMIM vs CS relationship from OMIMWe downloaded a text-formatted OMIM file as of August 2002,and extracted from each record the ID,title,and the Clinical Synopsis (CS)fields.Out of the 14,316records,4418records had CS descriptions.We selected the subset of sub-fields for the CS field that represent affected body parts/organs/systems and the resultant pathophysiol-ogy (e.g.‘Oncology’,standing for the malignancy caused by the mutation or accompanied by the phe-notype)for ing the mapping infor-mation of monogenic diseases on the chromosomes provided by NCBI,we identified 990Locuslink en-tries,or identified and located genes,causing 987of the diseases.Because we found that their represen-tation seems to be controlled only loosely (e.g.there are headings with both upper and lower case repre-sentations),we merged the headings for the selected sub-fields into 30types.To make a vector representation,we assumed that the pattern of the clinical manifestations of diseases could be compared in terms of how the CS cate-gories defined here are filled.Moreover,we as-sumed that the comparison of each CS category was possible based on the number of descriptions in that category.In other words,a CS category may be null,filled with only one description,or filled with many descriptions,and we focused on just the tendency of descriptions distributed in the CS categories without caring for the content of the descriptions.The vec-tors were hierarchically clustered (Eisen,M.B.et al.,1998),and we obtained 31tight and large clus-ters.4Application4.1Application of OMIM-derived disease vs clinical manifestation tableWe used the H-invitational set as the source of 13,543gene expression profiles in human adult nor-mal tissues.They were also clustered hierarchically and 29tight and large clusters were identified.We developed a ‘cross-bar’representation that visual-izes the interaction between diseases and genes (Fig-ure 4);the rows represent diseases clustered with the patterns in affected organs/systems,while the columns represent genes clustered with the expres-sion profiles;these patterns are represented as col-ored cells representing the coordinate values (for rows)or as the stacked bars representing the relative strength of expression (for columns).The intersec-tion of a row and a column represents that the gene corresponding to the column causes the disease cor-responding to the row.One may imagine that affected organs for a dis-ease may match with the prominent organ in the causing gene’s expression pattern;however,in most of the cases,it is not true.For example,the expres-sions cluster #21consisting of genes almost specific to the ‘muscle/heart’tissue category causes diseases not only muscles and cardiovasucular systems,but also diseases with neurological disorders.The ex-pression cluster #28consisting of genes almost spe-cific to the liver causes few diseases of the liver;rather,they cause haematological,immunological,neural,and cardiovascular diseases.It is of note that genes causing neural diseases have a wide range of expression patterns,as well as the genes causing ma-lignancies.4.2Application of MEDLINE-derived disease vs clinical manifestation tableA preliminary analysis of Figure 4showed that with the knowledge of disease cluster the prediction of the gene cluster increases by 15%.This result ap-pears not so striking.However,we noticed in the figure that if we treat each disease cluster as one entity,the expression patterns for the causal genes are not randomly or equally distributed over the di-verse ranges of expression patterns,but tend to be aggregated on some of,if not one of,the patterns;the extent of the aggregation seems to differ amongFigure4:Anatomical gene expression patterns and the patterns in affected organs/systems for the diseases caused by the genes.The rows represent the diseases and the columns represent the genes.The squares placed at their intersections indicate that the gene causes the disease,and indicate the absolute abundance with the intensity of the color.Genes are clustered with their expression profiles among the tissue types,and the diseases are clustered with their patterns in affected organs/systems.The areas for tight and large clusters for either genes or diseases are colored.the disease clusters.This implies that diseases with similar patterns in clinical manifestations may not be caused by genes with similar expression patterns, but may be caused by genes within a range of ex-pression patterns.This property might be used to infer a set of causative genes for diseases not shown in thefigure.As thefirst step,we are comparing the disease x clinical manifestation matrix made out of MEDLINE articles with one made from OMIM CS field in terms of their similarity in indexing.4.3Flybase-derived Table and Its Application To investigate the relationship between gene func-tion and expression pattern of the gene,we investi-gated association between mutant phenotype classes of Drosophila melanogaster(fruitsfly)and expres-sion pattern of human homologues to thefly gene. The structure,expression and function of human genes can be studied by comparing to homologous genes of experimental animals.The homologous gene is defined as the gene of significant local se-quence matching with a test sequence.The function of experimental animal genes can be determined by random or directed mutagenesis and experiments in crossbreeding.Because such information can not be acquired about human,comparison of homologues can be a powerful tool.In addition,it is well known that selecting candidate human disease genes by ho-mology is often more successful using model or-ganism than by considering human paralogs.In this study,we used fruitsfly as a model organism, because the Drosophila has been used mutagenesis studies extensively for many decades,and genomic sequence data is available.We made a correspondence table of Drosophila mutant phenotype with the tissue distribution pat-terns of the human homologues contained in H-invitational data set.To construct this table,we converted phenotypic information of alleles in the FlyBase into a‘Knowl-edge matrix’.The conversion was carried out based on“phe-notypic class:”and“phenotype manifest in:”fea-ture in the FlyBase allele dataset.The“phenotypic class:”and the“phenotype manifest in:”featureFigure5:Association between Drosophila phenotype classes and expression patterns of human homo-logues.We used FlyBase version3.1dataset for our analysis.This dataset contained42,143alleles.1,168 alleles had information of MIM number of human homologues.The phenotype of alleles was classified into 11classes.The expression pattern of each gene is shown by the stacked column representing each of10 tissue category by different colors.Numbered boxes represent‘tight’clusters e1though e29and colored as follows:red for‘tissue specific’and blue for‘even’.The blue bars show associations between Drosophila phenotype class and expression pattern of human homologue.The aggregation in blue bars suggests that genes for corresponding biological functions are dense in the corresponding expression clusters.were subcategories of“phenotypic information of alleles”(*k)field.Because,in the42,143FlyBase allele entry,only 1,168entries were homologous to OMIM gene en-tries(2),some“phenotypic class”of FlyBase should be merged into larger classes for further analysis. However description format of“phenotypic class”and“phenotype manifest in:”was rather free and hi-erarchical ontology was lacked.So we re-classified the allele phenotypes into11major classes based on “phenotypic class:”and“phenotype manifest in:”feature(Figure5).Each major class contained some 40to160OMIM gene entries.Human homologue of the Drosophila mu-tant alleles were obtained using MIM num-ber in a“cross-reference to non-Drosophila ho-molog(s)/analogs”(*j)field in the FlyBase.The ho-mologues were associated to the tissue distribution pattern(Figure5).Lots of Drosophila mutant strain had been con-structed by random or directed mutagenesis ex-periments and many lethal alleles were known in Drosophila.Because wild type gene products of lethal alleles should have essential biological func-tion,one may infer that the transcripts of such genes may distribute evenly across the tissues.But,from the Figure5,we can see this is not true.The expression pattern of Drosophila lethal allele was widespread.That is,the expression of some lethal gene have tissue specificity,and others have not.In the human homologues of Drosophila mutant alleles,few genes had endocrine/exocrine specific and Placenta/ovary/testis specific expression pattern, compared with others.The result was consistent with the fact that such organ was unique to verte-brates and mammals.We emphasize that our method is suitable for providing a whole view and clarifying such tendencies.5Future DirectionsWe have shown three types of seemingly qualita-tive data represented in the text format with dif-ferent degree of structures successfully transformed into the array of object features,and have demon-strated that the matrix representation gives new in-sight into the global understanding of large-scalemeasurement data and knowledge.We also pre-sented a practical use of NLP techniques and pos-sible targets to which the ontology of biological ob-jects may be applied.We will test these resources for NLP in the process of extracting more types of relations between objects and the features. ReferencesApweiler,R.et al.2001.The interpro database,an integrated documentation resource for protein fami-lies,domains and functional sites.Nucleic Acids Res, 29:37–40.Ashburner,M.et al.2000.Gene ontology:tool for the unification of biology.the gene ontology consortium.Nat Genet,25:25–9.Boeckmann,B.et al.2003.The swiss-prot protein knowledgebase and its supplement trembl in2003.Nucleic Acids Res,31:365–70.H.J.Bussemaker,H.Li,and E.D.Siggia.2001.Regu-latory element detection using correlation with expres-sion.Nat Genet,27:167–71.Camon,E.et al.2003.The gene ontology annota-tion(goa)project:Implementation of go in swiss-prot, trembl,and interpro.Genome Res,13:662–72. Eisen,M.B.et al.1998.Cluster analysis and display of genome-wide expression patterns.Proc Natl Acad Sci U S A,1998:14863–8.H.Ge,Z.Liu,G.M.Church,and M.Vidal.2001.Cor-relation between transcriptome and interactome map-ping data from saccharomyces cerevisiae.Nat Genet, 29:482–6.D.R.Gilbert,M.Schroeder,and J.van Helden.2000.Interactive visualization and exploration of relation-ships between biological objects.Trends Biotechnol, 18:487–94.Greenbaum,D.et al.2001.Interrelating different types of genomic data,from proteome to secretome:’oming in on function.Genome Res,11:1463–8.A.Hamosh, A. F.Scott,J.Amberger, C.Bocchini,D.Valle,and V. A.McKusick.2002.Onlinemendelian inheritance in man(omim),a knowledge-base of human genes and genetic disorders.Nucleic Acids Res,30:52–55.Ho,Y.et al.2002.Systematic identification of protein complexes in saccharomyces cerevisiae by mass spec-trometry.Nature,415:180–3.Hsiao,L.L.et al.2001.A compendium of gene ex-pression in normal human tissues.Physiol Genomics, 7:97–104.Ito,T.et al.2001.A comprehensive two-hybrid analysis to explore the yeast protein interactome.Proc Natl Acad Sci U S A,98:4569–74.Iyer,V.R.et al.99.The transcriptional program in the response of humanfibroblasts to serum.Science, 283:83–87.T.K.Jenssen,egreid,J.Komorowski,and E.Hovig.2001.A literature network of human genes for high-throughput analysis of gene expression.Nat Genet, 28:21–8.G.M.Joseph and V.L.Patel.1990.Domain knowl-edge and hypothesis generation in diagnostic reason-ing.Med Decis Making,10:31–46.Lander,E.S.et al.2001.Initial sequencing and analysis of the human genome.Nature,409:860–921.C Lindberg.1990.The unified medical language system(umls)of the national library of medicine.J Am Med Rec Assoc,61:40–42.E.M.Marcotte,M.Pellegrini,M.J.Thompson,T.O.Yeates,and D.Eisenberg.1999.A combined algo-rithm for genome-wide prediction of protein function.Nature,402:83–86.Ogata,H.et al.1999.Kegg:Kyoto encyclopedia of genes and genomes.Nucleic Acids Res,27:29–34. K.D.Pruitt and D.R.Maglott.2001.Refseq and lo-cuslink:Ncbi gene-centered resources.Nucleic Acids Res,29:137–40.A.W.Rives and T.Galitski.2003.Modular organiza-tion of cellular networks.Proc Natl Acad Sci U S A, 100:1128–33.Scherf,U.et al.2000.A gene expression database for the molecular pharmacology of cancer.Nat Genet, 24:236–44.rge-scale analysis of the human andmouse transcriptomes.Proc Natl Acad Sci U S A, 99:4465–70.The FlyBase Consortium.2003.Theflybase database of the drosophila genome projects and community litera-ture.Nucleic Acids Research,31:172–175. Velculescu,V.E.et al.1999.Analysis of human tran-scriptomes.Nat Genet,23:387–8.Xie,rge-scale protein annotation through gene ontology.Genome Res,12:785–94.。
人类基因组计划名词解释生物信息学
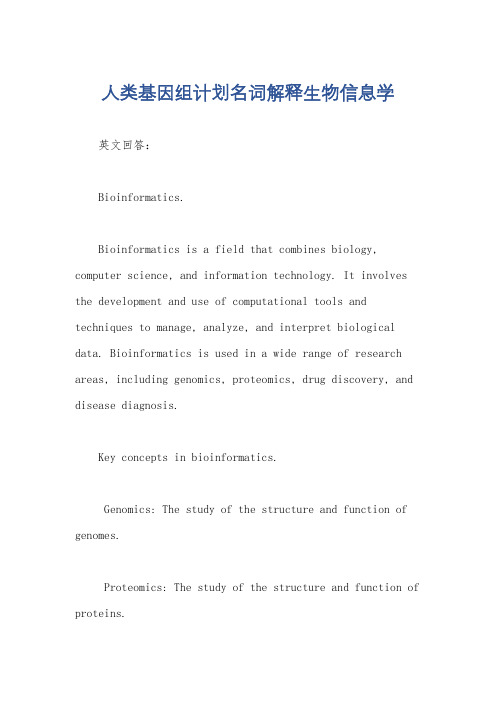
人类基因组计划名词解释生物信息学英文回答:Bioinformatics.Bioinformatics is a field that combines biology, computer science, and information technology. It involves the development and use of computational tools and techniques to manage, analyze, and interpret biological data. Bioinformatics is used in a wide range of research areas, including genomics, proteomics, drug discovery, and disease diagnosis.Key concepts in bioinformatics.Genomics: The study of the structure and function of genomes.Proteomics: The study of the structure and function of proteins.Transcriptomics: The study of the structure and function of transcripts.Metabolomics: The study of the structure and function of metabolites.Bioinformatics databases: Databases that store and manage biological data.Bioinformatics tools: Software tools that are used to analyze and interpret biological data.Applications of bioinformatics.Drug discovery: Bioinformatics is used to identify new drug targets and to design new drugs.Disease diagnosis: Bioinformatics is used to develop new diagnostic tests for diseases.Personalized medicine: Bioinformatics is used todevelop personalized treatment plans for patients.Evolutionary biology: Bioinformatics is used to study the evolution of species.Challenges in bioinformatics.Data explosion: The amount of biological data is growing rapidly, making it difficult to manage and analyze.Data integration: Biological data is often stored in different formats and in different databases, making it difficult to integrate and analyze.Algorithm development: New algorithms are needed to analyze and interpret complex biological data.Despite these challenges, bioinformatics is a rapidly growing field with the potential to revolutionize the way we understand and treat diseases.中文回答:生物信息学。
2024年新课标全国ⅰ卷英语高考真题文档版(含答案)

2024年普通高等学校招生全国统一考试(新课标Ⅰ卷)英语学科姓名________________准考证号________________全卷共12页,满分150分,考试时间120分钟。
考生注意:1.答题前,请务必将自己的姓名、准考证号用黑色字迹的签字笔或钢笔分别填写在试题卷和答题纸规定的位置上。
2.答题时,请按照答题纸上“注意事项”的要求,在答题纸相应的位置上规范作答,在本试题卷上的作答一律无效。
第一部分听力(共两节,满分30分)做题时,先将答案标在试卷上。
录音内容结束后,你将有两分钟的时间将试卷上的答案转涂到答题纸上。
第一节(共5小题;每小题1.5分,满分7.5分)听下面5段对话。
每段对话后有一个小题,从题中所给的A、B、C三个选项中选出最佳选项。
听完每段对话后,你都有10秒钟的时间来回答有关小题和阅读下一小题。
每段对话仅读一遍。
例:How much is the shirt?A.£19.15.B.£9.18.C.£9.15.答案是C。
1.【此处可播放相关音频,请去附件查看】What is Kate doing?A.Boarding a flight.B.Arranging a trip.C.Seeing a friend off.2.【此处可播放相关音频,请去附件查看】What are the speakers talking about?A.A pop star.B.An old song.C.A radio program.3.【此处可播放相关音频,请去附件查看】What will the speakers do today?A.Go to an art show.B.Meet the man's aunt.C.Eat out with Mark.4.【此处可播放相关音频,请去附件查看】What does the man want to do?A.Cancel an order.B.Ask for a receipt.C.Reschedule a delivery.5.【此处可播放相关音频,请去附件查看】When will the next train to Bedford leave?A.At9:45.B.At10:15.C.At11:00.第二节(共15小题;每小题1.5分,满分22.5分)听下面5段对话或独白。
蛋白质组学 生物芯片 壁垒

蛋白质组学生物芯片壁垒英文回答:Proteomics and Biochips: Breaking Barriers and Unlocking New Possibilities.Proteomics, the study of proteins, is a vital fieldthat has revolutionized our understanding of biological processes and disease mechanisms. Biochips, miniaturized devices that can analyze multiple proteins simultaneously, have played a critical role in advancing proteomics research. However, the integration of proteomics and biochips has faced several barriers, limiting their full potential.One major barrier has been the complexity of proteomes. With thousands of proteins present in a single biological sample, it has been challenging to develop biochips that can capture and analyze a comprehensive range of proteins. Additionally, post-translational modifications (PTMs) andprotein isoforms can further increase the complexity of proteomes, making it difficult to detect and quantify all relevant protein species.Another barrier has been the lack of standardized protocols and data analysis methods. This has hindered the reproducibility and comparability of proteomics data across different laboratories and studies. As a result, it has been difficult to draw robust conclusions from proteomics studies and translate findings into clinical applications.To overcome these barriers, researchers have explored various innovative approaches. One strategy has been to develop biochips with high capture efficiency andspecificity for target proteins. This has been achieved through the optimization of surface chemistry, the use of affinity ligands, and the incorporation of microfluidic technologies.Advances in mass spectrometry-based proteomics have also played a crucial role in improving the accuracy and sensitivity of biochip-based protein analysis. By couplingbiochips with mass spectrometers, researchers can identify and quantify proteins with high throughput and precision.Furthermore, the development of standardized data analysis pipelines and repositories has facilitated the sharing and comparison of proteomics data. This has enabled researchers to pool their resources and leverage the collective knowledge of the scientific community.As these barriers continue to be addressed, the integration of proteomics and biochips holds immense promise for advancing our understanding of protein functions, cellular processes, and disease mechanisms. By providing high-throughput, multiplexed, and sensitive protein analysis, biochip-based proteomics is poised to revolutionize healthcare, personalized medicine, and drug discovery.中文回答:蛋白质组学与生物芯片,突破障碍,释放新可能。
- 1、下载文档前请自行甄别文档内容的完整性,平台不提供额外的编辑、内容补充、找答案等附加服务。
- 2、"仅部分预览"的文档,不可在线预览部分如存在完整性等问题,可反馈申请退款(可完整预览的文档不适用该条件!)。
- 3、如文档侵犯您的权益,请联系客服反馈,我们会尽快为您处理(人工客服工作时间:9:00-18:30)。
erscheint in: In Silico Biology 5(1), 2005Integrating Data from Biological Experiments intoMetabolic Networks with the DBE InformationSystemLjudmilla Borisjuk Mohammad-Reza HajirezaeiChristian Klukas∗Hardy RolletschekFalk SchreiberInstitute of Plant Genetics and Crop Plant ResearchCorrensstr.3,D-06466Gatersleben,GermanySummaryModern’omics’-technologies result in huge amounts of data about life processes.For analysis and data mining purposes this data has to be considered in the context of the underlying biological networks.This work presents an approach for integrating data from biological experiments into metabolic networks by mapping the data onto network elements and visualising the data enriched networks automatically.This methodology is implemented in DBE,an information system that supports the analysis and visualisation of experimental data in the context of metabolic networks.It consists offive parts:(1)the DBE-Database for consistent data storage,(2)the Excel-Importer application for the data import,(3)the DBE-Website as the interface for the system,(4)the DBE-Pictures application for the up-and download of binary(e.g.image)files,and(5)DBE-Gravisto,a network analysis and graph visualisationsystem.The usability of this approach is demonstrated in two examples.Keywords:Metabolic networks,Visualisation,Metabolic profiling,Information sys-tem,Data integration1IntroductionMetabolic pathways or more generally biological networks provide the basis for every living organism.In order to understand the interactions between different metabolic ∗To whom correspondence should be addressed(klukas@ipk-gatersleben.de)1pathways,biochemical experiments are carried out.These experiments produce a large amount of data such as expression profiles and metabolic time series.Experiments are often repeated multiple times to compare the influence of different growth conditions or genetic modifications,and often many substances are detected at once.For data analysis purposes the biological context(e.g.the metabolic network)needs to be considered.We discuss an approach for the mapping of data onto metabolic networks for their dynamic visualisation and analysis.This methodology is implemented in a compre-hensive information system called DBE(D ata analysis and visualisation system for B iological E xperiments),which helps biologists in managing and analysing their ex-perimental data,especially metabolic data.In addition to the data mapping,the DBE data storage layer makes it possible to store experimental results in a single place and in a consistent form.Mapping experimental data onto metabolic networks opens new possibilities in analysing and visualising this data in the context of networks.Contrary to other systems which map such data onto static pictures[13,14],network-mapping allows new analysis methods(e.g.the computation of metabolic paths with the highest data changes)and advanced interactive visualisation and navigation techniques.2Methods2.1Mapping of experimental data onto dynamic networks Biological experiments are carried out in order to gain a deeper understanding of the metabolism of an organism,under changing conditions or while comparing different lines or mutants.Modern techniques like mass spectrometry allow biologists to analyse metabolite concentrations of up to100-200substances at once.This helps to gain a more complete view of the changes in the metabolism.But even more important than the number of analysed substances is the knowledge of the underlying processes.The measured metabolites are part of a huge network of metabolic reactions.This is the foundation for the presented integration method.During the analysis of experimental metabolic data an integrated view of the underlying network and the corresponding measured values needs to be considered.Our approach consists of three parts:(1)dynamic networks,(2)data mapping and(3)charting for the display of the measured values.(1)Contrary to mapping of data onto static pictures[13,14]we consider dynamic networks.Dynamic networks are networks that are either derived from databases(e.g. Proton/MARGBench[4,5],KEGG[7])or given by the user.They may,for example, change depending on the set of substances analysed in a particular experiment.(2)Data mapping is the assignment of data from experiments onto network el-ements.Here we consider the assignment of metabolite measurements to metabolic networks,but in general different experimental data can be mapped onto corresponding network elements of biological networks:protein levels onto protein-protein-interaction networks,transcriptomic data onto gene regulatory networks,and so on.Such a map-2Figure1:Components of the DBE information system and the external data source Proton/MARGBench.ping can be carried out automatically if there exists a function which assigns every data value to a network element.(3)Charting techniques(e.g.bar or line charts)are used to display measured values in the context of the network elements.Metabolite levels are shown inside the corresponding network nodes(metabolites),elucidating the interpretation of the data and the interaction between various metabolic routes.2.2The DBE information systemThe DBE information system consists of a number of components,see Fig.1:(1)the DBE-Database,(2)the Excel-Importer application which extracts biological experi-mental data out of Excel-files and stores this data in the database,(3)the DBE-Website as the interface of the system,(4)the DBE-Pictures application,which supports the up-and download of binary(image)files and(5)DBE-Gravisto,a network analysis and visualisation system.The Proton/MARGBench system[4,5]allows the integration of biological network data from different relational databases(e.g.KEGG,BRENDA). It is not a component of the DBE system but closely coupled to it.Although different techniques are used to implement the individual components of the information system,they are all uniformly accessible from the DBE-Website.In the following the different components are described in more detail:(1)DBE-Database:The focus of this database,implemented as an Oracle9i3Figure2:Entity-Relationship-Model of the DBE-Databasedatabase,is the storage of metabolite data connected to biological experiments.The Entity-Relationship-Model[12]is shown in Fig.2.The top entity is the“experiment”-table,which stores information about the import(e.g.time,username,name of experi-ment).For each experiment a number of“plants”can be stored.Genotype,variety and growth-conditions of these plants are saved in this entity.From these plants biologists take“samples”.For each sample a number of“measurements”can be stored in the database.Two entities for user management,the“account”and“usergroup”tables, are used to specify the accessibility of an experiment for different users.Additional ref-erence information can be assigned to the“substance”and“substancegroup”entities. These tables store reference information so that the users use a defined vocabulary for substances and measurement-units.(2)Excel-Importer:While examining different laboratory PCs it became clear, that these PCs run different Windows versions and special laboratory software that is suited for the special types of analysis apparatus that are in use by the biologists.The common export format from these software packages is Microsoft Excel.A solution that can process Excelfiles enables the biologists to copy the experimental data tables from the analysis software directly into the import template.A number of additional fields in the template,such as start of the experiment,notes,plant names and growth conditions can be added too.This way it is possible to enter all relevant data at one place into onefile.(3)DBE-Pictures:In order to be able to compare raw data and the corresponding pictures and chromatograms it is of great importance to include all this information in the database.This enables the users to identify known and unknown substances even after a very long period.To manage this type of data the DBE-Pictures application was designed.This application makes it possible to upload and assign imagefiles to experiments,plants and to individual measurements.Additional commands make it4possible to remove individualfiles or allfiles that are assigned to experiments,plants or measurements.It is also possible to download,save or view uploaded images and binary files.Therefore the user can also store experiment relatedfiles(e.g.documentation) in one place,which makes it easy to share experiment related data.(4)DBE-Website:The web interface makes it possible to access the different com-ponents of the information system.It can be used to initiate the import of experimental data into the database,to do basic data retrieval tasks,and to manage the experimen-tal data stored in the database.The DBE-Pictures and DBE-Gravisto applications can be started directly from the DBE website by using Java Web Start[8].(5)DBE-Gravisto:This system is based on Gravisto[1],an extensible graph-library and-editor.We developed several Gravisto-plugins(application extensions)to access the DBE-Database,to map experimental data onto given networks,to visualise the data-enriched networks,and to perform network analysis tasks.Visualisations created with DBE-Gravisto can be exported into standard graphics formats such as JPG,PNG,SVG or PDF.Examples for such visualisations are shown in the following section.The visualisation can be enhanced by using different levels of detail.A simple drawing of the chart without labels or captions is well suited for a larger view of the biological network.For a high zoom level where only few network elements are visible, more details(captions,legend and label)can be shown.3Results3.1Example1-comparison of different bean plant linesTo demonstrate the utility of our integrative bioinformatics approach we used metabo-lite data from the seed development of beans(Vicia narbonensis).Beans and other legume species are an economically important plant-derived protein source in the worldwide feed and food industry.In this case transgenic technology was used to increase protein accumulation by introducing the bacterial enzyme PEPC into the seed.The enzyme re-fixes HCO3-deliberated by respiration and together with phos-phoenolpyruvate yields oxaloacetate that can either be converted to aspartate or into malate and other intermediates of the citric acid cycle.PEPC controls the anaplerotic carbonflow and may improve seed carbon economy[6].Analysis of mature seeds revealed that transgenic seeds have a significant increase in crude protein content up to20%per gram and a higher dry bining both effects reveals that protein content per seed increases by40to50%[10].Tracer experiments could further show a clear stimulation of both[14C]-CO2uptake and incorporation into proteins.This corresponds to higher in vivofluxes via the PEPC-catalysed pathway.Because the transgenic effect appeared with different intensities and varied in many trangenic lines,visualisation of multiple changes for a snap overview was needed to recognize general tendencies.To characterise the responsible metabolic shift within seeds from sugars/starch into organic acids/amino acids/proteins,the metabolite pattern for gylcolysis,citrate cycle as well as related sugars and free amino acids was analysed.Metabolites were5Figure3:Visualisation of experimental data in the context of a metabolic network: Relative substance levels of different Vicia narbonensis lines(wild type in dark-grey, transgenic lines in light-grey),mapped onto the glycolysis and the citric acid cycle.6measured by liquid chromatography coupled to mass spectrometry(LC-MS).This technique allowed the separation according to retention times and molecular masses, and enabled parallel quantitative determinations with very low detection limits(sub-picomolar range).A detailed description of this metabolite profiling technique can be found in[10].Visualisation of metabolites within their pathways(Fig.3)gives an immediate overview of specific changes in metabolism within transgenic seeds.There was a clear trend towards the decrease of sucrose and phosphorylated sugars of the glycolytic pathway(Glucose-6-P,Glucose-1-P,Fructose-1,6-diP),but increases in the pool size of certain free amino acids due to transgene expression.Concentration of Acetyl-CoA was significantly higher in all transgenic lines as well as an overall trend towards higher levels of intermediates of the citric acid cycle.Thus,the PEPC expression in Vicia narbonensis seeds leads to changes in the metabolite pattern,indicating a shift of metabolicfluxes from sugars/starch into organic acids/amino acids/proteins.The metabolite profiling approach combined with bioinformatics tools and visu-alisation techniques used here enables the identification of the effects of transgene expression on plant metabolism in a fast and efficient way.In certain types of experi-ments it may help scientists tofind new targets for transgenic invasions.3.2Example2-seed development time series analysisIn this example we investigated the metabolite pattern of growing barley caryopses (Hordeum vulgare).The agronomical importance of cereal seeds is principally based on their accumulation of storage products,mainly starch and proteins.Despite exten-sive studies on the structure,biochemistry and genetics of developing grains[2,3,9] the regulatory mechanisms underlying their high storage capacity are largely unknown. During their growth,caryopses undergo distinct differentiation events.These in turn are reflected in changes of the metabolic state and biosyntheticfluxes.To investigate their specific temporal patterns,time series analyses of metabolites are required.Seed development includes the pre-storage,intermediate and storage phase.Within the pre-storage phase caryopsis consists mainly of pericarp tissue,embedding the liquid endosperm.Increase in the fresh weight and starch accumulation is low.The sub-sequent intermediate phase begins after endosperm cellularisation at4-5days post anthesis(DPA)and proceeds with the differentiation of endosperm tissues.Starch accumulation starts,although with low synthesis rates.The endosperm enlarges,be-coming the main storage organ of cereal seeds.During the main storage phase(from 10-11DPA onwards),the high starch synthesis rate is evident(Fig.4).Caryopses were harvested every2days over a growth period of about20DPA.Dynamic changes of about70metabolites were characterised.A typical example visualisation of time series data is given in Fig.5.In this case we used two line charts for the display of the time series data,the chart at the top of each network element shows the development of the metabolite concentrations for samples taken at day,the chart below shows the experimental data from samples taken at night.Such representation allows to observe not only developmental changes, but also metabolic responses on day/night conditions.Effect of light and darkness7Figure4:Structural changes of growing barley caryopsis and localisation of starch ac-cumulation during pre-storage-,intermediate-and main storage stage of development. Starch deposition is visualised within the cross sections through caryopsis(shown in dark colour,after iodine staining,upper panel)and tissues structures shown in dark-field images(lower panel):1-pericarp,2-endosperm.8Figure5:Visualisation of experimental data in the context of an metabolic network: Relative substance levels of Hordeum vulgare seeds sampled during day(top diagram inside the network elements)and night(bottom diagram),respectively.9on accumulation of storage products and metabolicfluxes is recently under investiga-tion[11].It is shown,that the amplitude of this response changes during development and visualisation of these changes in developmental scale is of big importance.4DiscussionWe presented an approach for integrating data of biological experiments into metabolic networks by mapping the data onto network elements and visualising the data enriched networks automatically.The developed information system allows the user to store the results of biochemical experiments and digital images of plants,chromatograms and experiment related binaryfiles consistently in one place.Because of the built-in user-management and access-system biologists can easily share their work results(measured values and visualisations)within their group,between different departments or even with the public.Our approach has already proved it’s usefulness as biologists use the system to support their scientific work,as shown in the application examples.Different charting techniques are useful in various applications.In thefirst example a condensed bar chart is used,where each displayed data point is based on a number of repeated measurements.The standard error of these measurements,represented by a line of variable length,makes it easier to estimate the relevance of differences.A future task is the integration of statistical methods to allow a more comprehensive data analysis.In the second example line charts are well suited for the display of time series data.The stacking of different result sets(here day and night),gives an immediate overview of the data.Because of high quality output of the visualisations and the export functionality (graphfile export in GML format,and image export in JPG,PNG,SVG and PDF format)the system is also in use for presentation purposes such as the creation of images for posters or papers.In the future we plan to develop network-search andfilter algorithms,which allow the user to analyse and visualise parts of metabolic pathways that are of interest or for which experimental data is available.Along with that we plan to develop interactive network layout and navigation methods.The DBE information system has significant potential as a powerful tool in ex-perimental biology and biotechnology.Its visualisation and modelling of metabolic pathways allows better understanding of the systems and consequences of experimen-tal manipulation.This leads to more efficient,targeted and successful experimental design,and promotes better achievement of biological and biotechnological goals.Taken together we present a tool combining bioinformatics and biochemistry in order to facilitate for biochemists the storage,management and visualisation of all processed results.Further work is still needed tofind out whether it might be possible to make predictions about any interaction between metabolite channelling through various compartments and how an efficient modification of a pathway can be prepared to increase and/or decrease an endproduct.Currently the DBE information system is already in use by scientists at the IPK. After implementation of the discussed extensions the components of the system will10be available for public users.AcknowledgementsWe would like to thank Prof.Franz J.Brandenburg,Michael Forster,Andreas Pick, and Paul Holleis(all University of Passau)for excellent cooperation and for granting usage of Gravisto;Prof.Ralf Hofest¨a dt and Andreas Freier(Bielefeld University)for fruitful cooperation and permission to use the PROTON/MARGBench system.For helpful discussion and support we thank Prof.Ulrich Wobus and Prof.Uwe Sonnewald (IPK Gatersleben).This work was supported by the German Ministry of Education and Research(BMBF)under grant0312706A.We acknowledge funding by the Land Sachsen-Anhalt(MK-LSA0031KL/1002L).References[1]C.Bachmaier,F.J.Brandenburg,M.Forster,M.Raitner,and P.Holleis.Grav-isto:Graph visualization toolkit.In Proc.Intl.Symposium on Graph Drawing (GD’04),2004,to appear.[2]J.D.Bewley and M.Black.Seeds-Physiology of Development and Germination.Plenum Press,New York,London,1994.[3]C.M.Duffus and M.P.Cochrane.Carbohydrate metabolism during cereal graindevelopment,pages43–66.Elsevier Biomedical press,1982.[4]A.Freier,R.Hofest¨a dt,nge,and U.Scholz.MARGBench-an approachfor integration,modeling and animation of metabolic networks.In Proceedings of the German Conference on Bioinformatics(GCB’99),pages190–194,1999. [5]A.Freier,nge,and R.Hofest¨a dt.Integrative analysis of gene networks usingdynamic process pattern modelling.In Bioinformatics of Genome Regulation and Structure,pages257–264.Kluwer Academic Publishers,2004.[6]S.Golombek,U.Heim,C.Horstmann,U.Wobus,and H.Weber.Phospho-enolpyruvate carboxylase in developing seeds on Vicia faba.gene expression and metabolic regulation.Planta,208:66–72,1999.[7]M.Kanehisa and S.Goto.KEGG:kyoto encyclopedia of genes and genomes.Nucleic Acids Research,28(1):27–30,2000.[8]M.Marinilli.Java Deployment with JNLP and WebStart.Sams Publishing,2001.[9]O.A.Olsen,R.H.Potter,and R.Kalla.Histo-differentiation and molecularbiology of developing cereal endosperm.Seed Science Research,2:117–131,1992.11[10]H.Rolletschek,L.Borisjuk,R.Radchuk,M.Miranda,U.Heim,U.Wobus,andH.Weber.Seed-specific expression of a bacterial phosphoenolpyruvate carboxylasein Vicia narbonensis increases protein content and improves carbon economy.Plant Biotechnology Journal,2:211–219,2004.[11]H.Rolletschek,W.Weschke,H.Weber,U.Wobus,and L.Boriskuk.Energy stateand its control on seed development:starch accumulation is associated with high atp and steep oxygen gradients within barley grains.Journal of Experimental Botany,55:1351–1359,2004.[12]G.Saake and A.Heuer.Datenbanken,Konzepte und Sprachen.Thomson Pub-lishing,1997.[13]O.Thimm,O.Bl¨a sing,Y.Gibon, A.Nagel,S.Meyer,P.Kr¨u ger,J.Selbig,L.A.M¨u ller,S.Y.Rhee,and M.Stitt.MAPMAN:a user-driven tool to display genomics data sets onto diagrams of metabolic pathways and other biological processes.The Plant Journal,37:914–939,2004.[14]G.Wolf.Visualising gene expression in its metabolic context.Briefings in Bioin-formatics,1(3):297–304,2000.12。