2014年数学建模美赛ABC_题翻译
2014年美赛C题翻译
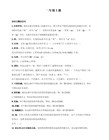
This will take some skilled data extraction and modeling efforts to obtain the correct set of nodes (the Erdös coauthors) and their links (connections with one another as coauthors). 这需要熟练数据提取 并 在建模上下功夫, 以便得到正确的节点和边
Once built, analyze the properties of this network. 建完后分析网络性能(Again, do not include Erdös --- he is the most infnodes in the network. In this case, it’s co-authorship with him that builds the network, but he is not part of the network or the analysis.)
One of the techniques to determine influence of academic research is to build and measure properties of citation or co-author networks.
学术研究的技术来确定影响之一是构建和引文或合著网络的度量属性。
Google Scholar is also a good data tool to use for network influence or impact data collection and analysis.
谷歌学术搜索也是一个好的数据工具用于网络数据收集和分析影响或影响。
2014 数学建模美赛B题
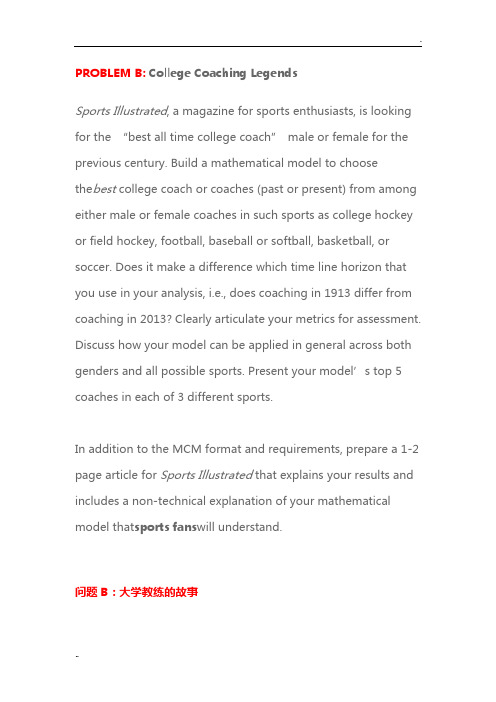
PROBLEM B: College Coaching LegendsSports Illustrated, a magazine for sports enthusiasts, is looking for the “best all time college coach”male or female for the previous century. Build a mathematical model to choosethe best college coach or coaches (past or present) from among either male or female coaches in such sports as college hockey or field hockey, football, baseball or softball, basketball, or soccer. Does it make a difference which time line horizon that you use in your analysis, i.e., does coaching in 1913 differ from coaching in 2013? Clearly articulate your metrics for assessment. Discuss how your model can be applied in general across both genders and all possible sports. Present your model’s top 5 coaches in each of 3 different sports.In addition to the MCM format and requirements, prepare a 1-2 page article for Sports Illustrated that explains your results and includes a non-technical explanation of your mathematical model that sports fans will understand.问题B:大学教练的故事体育画报,为运动爱好者杂志,正在寻找上个世纪堪称“史上最优秀大学教练”的男性或女性。
2014美赛b题 分析

上一篇文章中我们用题意分解的办法详细解读了2014MCM A题的要点,并总结了6个题意的关键点,大家可以对照自己的文章,看看有没有把这些关键问题解释和说明清楚,如果这些问题全部回答到位,M奖就到手啦,在这些部分中有1~2个有所创新,能够令评委眼前一亮的话,那么就有机会得到更高的奖项哦,大家是不是心里更加有底了呢?接下来,我们继续用上篇文章提到的方法来解析B题的关键点,上题:PROBLEM B: CollegeCoaching Legends (bk1: the topic)Sports Illustrated, amagazine for sports enthusiasts, is looking for the “best all time college coach” male or femalefor the previous century. (bk2:briefintroduction of background)Build a mathematical model (spm1) tochoose the best college coach or coaches (past or present)(rsc1) from among either male or female (rsc2)coaches in such sports as college hockey or fieldhockey, football, baseball or softball,basketball, or soccer (rsc3)。
(These 3 restrictiveconditions ask us the range of our evaluation model should contain thedifference above。
)Does it make a difference which time line horizon thatyou use in your analysis, i.e。
2014美赛ICM翻译

2014 ICM ProblemUsing Networks to Measure Influence and Impact One of the techniques to determine influence of academic research is to build and measure properties of citation or co-author networks. Co-authoring a manuscript usually connotes a strong influential connection between researchers. One of the most famous academic co-authors was the 20th-century mathematician Paul Erdös who had over 500co-authors and published over 1400 technical research papers. It is ironic, or perhapsnot, that Erdös is also one of the influencers in building the foundation for the emerging interdisciplinary science of networks, particularly, through his publication with AlfredRényi of the paper “On Random Graphs” in 1959. Erdös’s role as a collaborator was sosignificant in the field of mathematics that mathematicians often measure their closeness to Erdös through analysis of Erdös’s amazingly large and robust co-authornetwork (see the website /enp/ ). The unusual and fascinatingstory of Paul Erdös as a gifted mathematician, talented problem solver, and mastercollaborator is provided in many books and on-line websites(e.g.,/Biographies/Erd os.html). Perhaps his itinerantlifestyle, frequently staying with or residing with his collaborators, and giving much of hismoney to students as prizes for solving problems, enabled his co-authorships to flourishand helped build his astounding network of influence in several areas of mathematics.In order to measure such influence as Erdös produced, there are network-basedevaluation tools that use co-author and citation data to determine impact factor ofresearchers, publications, and journals. Some of these are Science Citation Index, Hfactor,Impact factor, Eigenfactor, etc. Google Scholar is also a good data tool to use fornetwork influence or impact data collection and analysis. Your team’s goal for ICM2014 is to analyze influence and impact in research networks and other areas of society. Your tasks to do this include:1) Build the co-author network of the Erdos1 authors (you can use the file from thewebsitehttps:///users/grossman/enp/Erdos1. html or the one weinclude at Erdos1.htm ). You should build a co-author network of theapproximately 510 researchers from the file Erdos1, who coauthored a paperwith Erdös, but do not include Erdös. This will take some skilled data extractionand modeling efforts to obtain the correct set of nodes (the Erdös coauthors) andtheir links (connections with one another as coauthors). There are over 18,000lines of raw data in Erdos1 file, but many of them will not be used since they arelinks to people outside the Erdos1 network. If necessary, you can limit the size ofyour network to analyze in order to calibrate your influence measurementalgorithm. Once built, analyze the properties of this network. (Again, do notinclude Erdös --- he is the most influential and would be connected to all nodes inthe network. In this case, it’s co-authorship with him that builds the network, buthe is not part of the network or the analysis.)2) Develop influence measure(s) to determine who in this Erdos1 network hassignificant influence within the network. Consider who has published importantworks or connects important researchers within Erdos1. Again, assume Erdös isnot there to play these roles.3) Another type of influence measure might be to compare the significance of aresearch paper by analyzing the important works that follow from its publication.Choose some set of foundational papers in the emerging field of network scienceeither from the attached list (NetSciFoundation.pdf) or papers you discover.Use these papers to analyze and develop a model to determine their relativeinfluence. Build the influence (coauthor or citation) networks and calculateappropriate measures for your analysis. Which of the papers in your set do youconsider is the most influential in network science and why? Is there a similarway to determine the role or influence measure of an individual networkresearcher? Consider how you would measure the role, influence, or impact of aspecific university, department, or a journal in network science? Discussmethodology to develop such measures and the data that would need to becollected.4) Implement your algorithm on a completely different set of network influence data--- for instance, influential songwriters, music bands, performers, movie actors,directors, movies, TV shows, columnists, journalists, newspapers, magazines,novelists, novels, bloggers, tweeters, or any data set you care to analyze. Youmay wish to restrict the network to a specific genre or geographic location orpredetermined size.5) Finally, discuss the science, understanding and utility of modeling influence andimpact within networks. Could individuals, organizations, nations, and society useinfluence methodology to improve relationships, conduct business, and makewise decisions? For instance, at the individual level, describe how you could useyour measures and algorithms to choose who to try to co-author with in order toboost your mathematical influence as rapidly as possible. Or how can you useyour models and results to help decide on a graduate school or thesis advisor toselect for your future academic work?6) Write a report explaining your modeling methodology, your network-basedinfluence and impact measures, and your progress and results for the previousfive tasks. The report must not exceed 20 pages (not including your summarysheet) and should present solid analysis of your network data; strengths,weaknesses, and sensitivity of your methodology; and the power of modelingthese phenomena using network science.*Your submission should consist of a 1 page Summary Sheet and your solution cannotexceed 20 pages for a maximum of 21 pages.This is a listing of possible papers that could be included in a foundational set ofinfluential publications in network science. Network science is a new, emerging, diverse, interdisciplinary field so there is no large, concentrated set of journals that are easy touse to find network papers even though several new journals were recently establishedand new academic programs in network science are beginning to be offered inuniversities throughout the world. You can use some of these papers or others of yourown choice for your team’s set to analyze and compare for influence or impact innetwork science for task #3.Erdös, P. and Rényi, A., On Random Graphs, Publicationes Mathematicae, 6: 290-297,1959.Albert, R. and Barabási, A-L. Statistical mechanics of complex networks. Reviews ofModern Physics, 74:47-97, 2002.Bonacich, P.F., Power and Centrality: A family of measures, Am J. Sociology. 92: 1170-1182, 1987.Barabási, A-L, and Albert, R. Emergence of scaling in random networks. Science, 286:509-512, 1999.Borgatti, S. Identifying sets of key players in a network. Computational andMathematical Organization Theory, 12: 21-34, 2006. Borgatti, S. and Everett, M. Models of core/periphery structures. Social Networks, 21:375-395, October 2000Graham, R. On properties of a well-known graph, or, What is your Ramseynumber? Annals of the New York Academy of Sciences, 328:166-172, June 1979.Kleinberg, J. Navigation in a small world. Nature, 406: 845, 2000.Newman, M. Scientific collaboration networks: II. Shortest paths, weightednetworks, and centrality. Physical Review E, 64:016132, 2001.Newman, M. The structure of scientific collaboration networks. Proc. Natl.Acad. Sci. USA, 98: 404-409, January 2001. Newman, M. The structure and function of complex networks. SIAM Review,45:167-256, 2003.Watts, D. and Dodds, P. Networks, influence, and public opinion formation. Journal ofConsumer Research, 34: 441-458, 2007.Watts, D., Dodds, P., and Newman, M. Identity and search in social networks. Science,296:1302-1305, May 2002.Watts, D. and Strogatz, S. Collective dynamics of `small-world' networks. Nature, 393:440-442, 1998.Snijders, T. Statistical models for social networks. Annual Review of Sociology, 37:131–153, 2011.Valente, T. Social network thresholds in the diffusion of innovations, Social Networks,18: 69-89, 1996.Erdos1, V ersion 2010, October 20, 2010This is a list of the 511 coauthors of Paul Erdos, together with their coauthors listed beneath them. The date of first joint paper with Erdos is given, followed by the number of joint publications (ifit is more than one). An asterisk following the name indicates that this Erdos coauthor is known to be deceased; additional information about the status of Erdos coauthors would be most welcomed. (This convention is not used for those with Erdos number 2, as to do so would involve too much work.) Numbers preceded by carets follow the convention used by Mathematical Reviews in MathSciNet to distinguish people with the same names.Please send corrections and comments to grossman@The Erdos Number Project Web site can be found at the following URL:/enpABBOTT, HARVEY LESLIE 1974Aull, Charles E.Brown, Ezra A.Dierker, Paul F.Exoo, GeoffreyGardner, BenHANSON, DENISHare, Donovan R. Katchalski, MeirLiu, Andy C. F.MEIR, AMRAMMOON, JOHN W.MOSER, LEO*Pareek, Chandra MohanRiddell, JamesSAUER, NORBERT W.SIMMONS, GUSTA VUS J.Smuga-Otto, M. J.SUBBARAO, MA TUKUMALLI VENKATA* Suryanarayana, D.Toft, BjarneWang, Edward Tzu HsiaWilliams, Emlyn R.Zhou, BingACZEL, JANOS D. 1965Abbas, Ali E.Aczel, S.Alsina Catala, ClaudiBaker, John A.Beckenbach, Edwin F.Beda, GyulaBelousov, Valentin DanilovichBenz, WalterBerg, LotharBoros, ZoltanChudziak, JacekDaroczy, ZoltanDhombres, Jean G.Djokovic, Dragomir Z.Egervary, Jeno2014 ICM问题使用网络来测量的影响和冲击其中一项技术来确定学术研究的影响力是建立和测量引文或合著者网络的性能。
2014美国数学建模竞赛赛题翻译
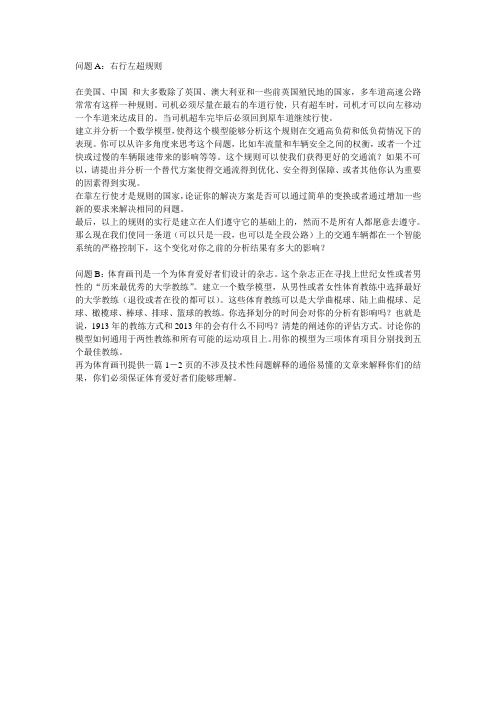
问题A:右行左超规则在美国、中国和大多数除了英国、澳大利亚和一些前英国殖民地的国家,多车道高速公路常常有这样一种规则。
司机必须尽量在最右的车道行使,只有超车时,司机才可以向左移动一个车道来达成目的。
当司机超车完毕后必须回到原车道继续行使。
建立并分析一个数学模型,使得这个模型能够分析这个规则在交通高负荷和低负荷情况下的表现。
你可以从许多角度来思考这个问题,比如车流量和车辆安全之间的权衡,或者一个过快或过慢的车辆限速带来的影响等等。
这个规则可以使我们获得更好的交通流?如果不可以,请提出并分析一个替代方案使得交通流得到优化、安全得到保障、或者其他你认为重要的因素得到实现。
在靠左行使才是规则的国家,论证你的解决方案是否可以通过简单的变换或者通过增加一些新的要求来解决相同的问题。
最后,以上的规则的实行是建立在人们遵守它的基础上的,然而不是所有人都愿意去遵守。
那么现在我们使同一条道(可以只是一段,也可以是全段公路)上的交通车辆都在一个智能系统的严格控制下,这个变化对你之前的分析结果有多大的影响?问题B:体育画刊是一个为体育爱好者们设计的杂志。
这个杂志正在寻找上世纪女性或者男性的“历来最优秀的大学教练”。
建立一个数学模型,从男性或者女性体育教练中选择最好的大学教练(退役或者在役的都可以)。
这些体育教练可以是大学曲棍球、陆上曲棍球、足球、橄榄球、棒球、排球、篮球的教练。
你选择划分的时间会对你的分析有影响吗?也就是说,1913年的教练方式和2013年的会有什么不同吗?清楚的阐述你的评估方式。
讨论你的模型如何通用于两性教练和所有可能的运动项目上。
用你的模型为三项体育项目分别找到五个最佳教练。
再为体育画刊提供一篇1-2页的不涉及技术性问题解释的通俗易懂的文章来解释你们的结果,你们必须保证体育爱好者们能够理解。
2014年数学建模美赛ABC 题翻译

问题A:除非超车否则靠右行驶的交通规则在一些汽车靠右行驶的国家(比如美国,中国等等),多车道的高速公路常常遵循以下原则:司机必须在最右侧驾驶,除非他们正在超车,超车时必须先移到左侧车道在超车后再返回。
建立数学模型来分析这条规则在低负荷和高负荷状态下的交通路况的表现。
你不妨考察一下流量和安全的权衡问题,车速过高过低的限制,或者这个问题陈述中可能出现的其他因素。
这条规则在提升车流量的方面是否有效?如果不是,提出能够提升车流量、安全系数或其他因素的替代品(包括完全没有这种规律)并加以分析。
在一些国家,汽车靠左形式是常态,探讨你的解决方案是否稍作修改即可适用,或者需要一些额外的需要。
最后,以上规则依赖于人的判断,如果相同规则的交通运输完全在智能系统的控制下,无论是部分网络还是嵌入使用的车辆的设计,在何种程度上会修改你前面的结果?问题B:大学传奇教练体育画报是一个为运动爱好者服务的杂志,正在寻找在整个上个世纪的“史上最好的大学教练”。
建立数学模型选择大学中在一下体育项目中最好的教练:曲棍球或场地曲棍球,足球,棒球或垒球,篮球,足球。
时间轴在你的分析中是否会有影响?比如1913年的教练和2013年的教练是否会有所不同?清晰的对你的指标进行评估,讨论一下你的模型应用在跨越性别和所有可能对的体育项目中的效果。
展示你的模型中的在三种不同体育项目中的前五名教练。
除了传统的MCM格式,准备一个1到2页的文章给体育画报,解释你的结果和包括一个体育迷都明白的数学模型的非技术性解释。
使用网络测量的影响和冲击学术研究的技术来确定影响之一是构建和引文或合著网络的度量属性。
与人合写一手稿通常意味着一个强大的影响力的研究人员之间的联系。
最著名的学术合作者是20世纪的数学家保罗鄂尔多斯曾超过500的合作者和超过1400个技术研究论文发表。
讽刺的是,或者不是,鄂尔多斯也是影响者在构建网络的新兴交叉学科的基础科学,尤其是,尽管他与Alfred Rényi的出版物“随即图标”在1959年。
2014美赛题目(翻译版)

2014 MCM ProblemsPROBLEM A: The Keep-Right-Except-To-Pass RuleIn countries where driving automobiles on the right is the rule (that is, USA, China and most other countries except for Great Britain, Australia, and some former British colonies), multi-lane freeways often employ a rule that requires drivers to drive in the right-most lane unless they are passing another vehicle, in which case they move one lane to the left, pass, and return to their former travel lane.在一些以行车靠右为规则的国家中(比如美国、中国以及除了大不列颠、澳大利亚和一些前英属殖民国家以外的其他国家),多行道的高速公路经常采用要求驾驶人在除超车以外时都靠右行驶的交通规则。
Build and analyze a mathematical model to analyze the performance of this rule in light and heavy traffic. You may wish to examine tradeoffs between traffic flow and safety, the role of under- or over-posted speed limits (that is, speed limits that are too low or too high), and/or other factors that may not be explicitly called out in this problem statement. Is this rule effective in promoting better traffic flow? If not, suggest and analyze alternatives (to include possibly no rule of this kind at all) that might promote greater traffic flow, safety, and/or other factors that you deem important.1、请你建立和分析一个数学模型来分析这个规则在交通畅通和交通堵塞条件下的表现。
建模美赛C题带翻译

Problem C: “Cooperate and navigate”Traffic capacity is limited in many regions of the United States due to the number of lanes of roads. For example, in the Greater Seattle area drivers experience long delays during peak traffic hours because the volume of traffic exceeds the designed capacity of the road networks. This is particularly pronounced on Interstates 5, 90, and 405, as well as State Route 520, the roads of particular interest for this problem.Self-driving, cooperating cars have been proposed as a solution to increase capacity of highways without increasing number of lanes or roads. The behavior of these cars interacting with the existing traffic flow and each other is not well understood at this point.The Governor of the state of Washington has asked for analysis of the effects of allowing self-driving, cooperating cars on the roads listed above in Thurston, Pierce, King, and Snohomish counties. (See the provided map and Excel spreadsheet). In particular, how do the effects change as the percentage of self-driving cars increases from 10% to 50% to 90%? Do equilibria exist? Is there a tipping point where performance changes markedly? Under what conditions, if any, should lanes be dedicated to these cars? Does your analysis of your model suggest any other policy changes?Your answer should include a model of the effects on traffic flow of the number of lanes, peak and/or average traffic volume, and percentage of vehicles using self-driving, cooperating systems. Your model should address cooperation between self-driving cars as well as the interaction between self- driving and non-self-driving vehicles. Your model should then be applied to the data for the roads of interest, provided in the attached Excel spreadsheet.Your MCM submission should consist of a 1 page Summary Sheet, a 1-2 page letter to the Governor’s office, and your solution (not to exceed 20 pages) for a maximum of 23 pages. Note: The appendix and references do not count toward the 23 page limit. Some useful background information:On average, 8% of the daily traffic volume occurs during peak travel hours.•The nominal speed limit for all these roads is 60 miles per hour.•Mileposts are numbered from south to north, and west to east.•Lane widths are the standard 12 feet.•Highway 90 is classified as a state route until it intersects Interstate 5.•In case of any conflict between the data provided in this problem and any other source, use the data provided in this problem.Definitions:milepost: A marker on the road that measures distance in miles from either the start of the route or astate boundary.average daily traffic: The average number of cars per day driving on the road.interstate: A limited access highway, part of a national system.state route: A state highway that may or may not be limited access.route ID: The number of the highway.increasing direction: Northbound for N-S roads, Eastbound for E-W roads.decreasing direction: Southbound for N-S roads, Westbound for E-W roads.问题C:“合作和导航”由于道路的数量,美国许多地区的交通容量有限。
- 1、下载文档前请自行甄别文档内容的完整性,平台不提供额外的编辑、内容补充、找答案等附加服务。
- 2、"仅部分预览"的文档,不可在线预览部分如存在完整性等问题,可反馈申请退款(可完整预览的文档不适用该条件!)。
- 3、如文档侵犯您的权益,请联系客服反馈,我们会尽快为您处理(人工客服工作时间:9:00-18:30)。
问题A:除非超车否则靠右行驶的交通规则在一些汽车靠右行驶的国家(比如美国,中国等等),多车道的高速公路常常遵循以下原则:司机必须在最右侧驾驶,除非他们正在超车,超车时必须先移到左侧车道在超车后再返回。
建立数学模型来分析这条规则在低负荷和高负荷状态下的交通路况的表现。
你不妨考察一下流量和安全的权衡问题,车速过高过低的限制,或者这个问题陈述中可能出现的其他因素。
这条规则在提升车流量的方面是否有效?如果不是,提出能够提升车流量、安全系数或其他因素的替代品(包括完全没有这种规律)并加以分析。
在一些国家,汽车靠左形式是常态,探讨你的解决方案是否稍作修改即可适用,或者需要一些额外的需要。
最后,以上规则依赖于人的判断,如果相同规则的交通运输完全在智能系统的控制下,无论是部分网络还是嵌入使用的车辆的设计,在何种程度上会修改你前面的结果?问题B:大学传奇教练体育画报是一个为运动爱好者服务的杂志,正在寻找在整个上个世纪的“史上最好的大学教练”。
建立数学模型选择大学中在一下体育项目中最好的教练:曲棍球或场地曲棍球,足球,棒球或垒球,篮球,足球。
时间轴在你的分析中是否会有影响?比如1913年的教练和2013年的教练是否会有所不同?清晰的对你的指标进行评估,讨论一下你的模型应用在跨越性别和所有可能对的体育项目中的效果。
展示你的模型中的在三种不同体育项目中的前五名教练。
除了传统的MCM格式,准备一个1到2页的文章给体育画报,解释你的结果和包括一个体育迷都明白的数学模型的非技术性解释。
使用网络测量的影响和冲击学术研究的技术来确定影响之一是构建和引文或合著网络的度量属性。
与人合写一手稿通常意味着一个强大的影响力的研究人员之间的联系。
最著名的学术合作者是20世纪的数学家保罗鄂尔多斯曾超过500的合作者和超过1400个技术研究论文发表。
讽刺的是,或者不是,鄂尔多斯也是影响者在构建网络的新兴交叉学科的基础科学,尤其是,尽管他与Alfred Rényi的出版物“随即图标”在1959年。
鄂尔多斯作为合作者的角色非常重要领域的数学,数学家通常衡量他们亲近鄂尔多斯通过分析鄂尔多斯的令人惊讶的是大型和健壮的合著网络网站(见http:// /enp/)。
保罗的与众不同、引人入胜的故事鄂尔多斯作为一个天才的数学家,才华横溢的problemsolver,掌握合作者提供了许多书籍和在线网站(如。
,/Biographies/Erdos.html)。
也许他流动的生活方式,经常住在带着合作者或居住,并给他的钱来解决问题学生奖,使他co-authorships蓬勃发展并帮助构建了惊人的网络在几个数学领域的影响力。
为了衡量这种影响asErdos生产,有基于网络的评价工具,使用作者和引文数据来确定影响因素的研究,出版物和期刊。
一些科学引文索引,Hfactor、影响因素,特征因子等。
谷歌学术搜索也是一个好的数据工具用于网络数据收集和分析影响或影响。
ICM 2014你的团队的目标是分析研究网络和其他地区的影响力和影响社会。
你这样做的任务包括:1)构建networkof Erdos1作者合著者(你可以使用我们网站https://files.oak /users/grossman/enp/Erdos1.htmlor的文件包括Erdos1.htm)。
你应该建立一个合作者网络Erdos1大约有510名研究人员的文件,与鄂尔多斯的一篇论文的合著者,他但不包括鄂尔多斯。
这将需要一些技术数据提取和建模工作获得的节点correctset(鄂尔多斯合作者)和他们的链接(彼此连接ascoauthors)。
有超过18000行Erdos1的原始数据文件,但是很多人不会用因为它们链接Erdos 1网络之外的人。
如果有必要,你可以限制你的网络的规模分析,以校准你的影响力度量算法。
一旦建立,分析该网络的属性。
(不包括鄂尔多斯——他是最有影响力的,将连接到网络中的所有节点。
在这种情况下,它的co-authorship营造网络与他,但他不属于网络或分析。
)2)开发影响措施(s)决定谁在这个Erdos1网络在网络中有显著的影响。
考虑谁发表了重要的作品在Erdos1或连接重要人员。
同样,假设没有鄂尔多斯扮演这些角色。
3)另一种类型的影响测量)比较研究论文通过分析的意义重要的作品,从其出版。
选择一些新兴领域的基础性文件网络科学从附表(NetSciFoundation.pdf)或论文你发现。
使用这些文件来分析和开发一个模型来确定它们的相对影响力。
构建的影响(合著者或引用)网络和计算分析适当措施。
论文在你设定你认为是最具影响力的网络科学,为什么? 有类似的方式来确定个体的作用或影响测量网络研究员? 考虑如何测量作用、影响或影响特定大学的部门,或在网络科学杂志吗? 讨论开发这些措施和方法需要收集的数据。
4)一组完全不同的网络上实现算法影响的数据——例如,影响力的作曲家,音乐乐队,表演者,电影演员、导演、电影、电视节目、专栏作家、记者、报纸、杂志、小说,小说,博客,推特,或者任何你愿意分析的数据集。
您可能希望限制网络特定类型或地理位置或预定的大小。
5)最后,讨论科学、理解和建模的影响和影响在网络的效用。
可以个人、组织、国家和社会使用影响方法改善人际关系,做生意,和做出明智的决定吗? 例如,在个体层面,描述如何使用你的措施和算法选择谁试图与合著者为了尽快提高你的数学的影响。
或你如何使用你的模型和结果来帮助决定毕业学校或导师的选择为你的未来学术工作吗?6)写报告解释您的建模方法,基于网络的影响和影响的措施,和之前的五项任务的进程和结果。
报告不能exceed20页(不包括你的汇总表),应该提供确凿的网络数据的分析,优势,劣势,和灵敏度的方法,建模这些现象使用网络科学的力量。
你的提交应该由一个1页汇总表和您的解决方案不能超过20页最长21页。
这是一个可能的论文清单,可以包含在一组基本的有影响力的网络科学出版物。
网络科学是一个新的、新兴、多样化、跨学科领域所以没有大型、集中组易于使用找到的期刊网络报纸,尽管一些新的期刊最近网络科学的建立和新的学术项目正开始在世界各地被提供在大学。
您可以使用其中的一些文件或其他你的选择你的团队的设置来分析和比较影响或影响在网络科学任务# 3。
Erdös, P. and Rényi, A., On Random Graphs, Publicationes Mathematicae, 6: 290-297, 1959.Albert, R. and Barabási, A-L. Statistical mechanics of complex networks. Reviews of Modern Physics, 74:47-97, 2002.Bonacich, P.F., Power and Centrality: A family of measures, Am J. Sociology. 92: 1170-1182, 1987.Barabási, A-L, and Albert, R. Emergence of scaling in random networks. Science, 286:509-512, 1999.Borgatti, S. Identifying sets of key players in a network. Computationaland Mathematical Organization Theory, 12: 21-34, 2006.Borgatti, S. and Everett, M. Models of core/periphery structures. Social Networks, 21:375-395, October 2000Graham, R. On properties of a well-known graph, or, What is your Ramseynumber? Annals of the New York Academy of Sciences, 328:166-172, June 1979.Kleinberg, J. Navigation in a small world. Nature, 406: 845, 2000. Newman, M. Scientific collaboration networks: II. Shortest paths, weightednetworks, and centrality. Physical Review E, 64:016132, 2001. Newman, M. The structure of scientific collaboration networks. Proc. Natl.Acad. Sci. USA, 98: 404-409, January 2001.Newman, M. The structure and function of complex networks. SIAM Review,45:167-256, 2003.Watts, D. and Dodds, P. Networks, influence, and public opinion formation. Journal of Consumer Research, 34: 441-458, 2007.Watts, D., Dodds, P., and Newman, M. Identity and search in social networks. Science, 296:1302-1305, May 2002.Watts, D. and Strogatz, S. Collective dynamics of `small-world' networks. Nature, 393:440-442, 1998.Snijders, T. Statistical models for social networks. Annual Review of Sociology, 37:131–153, 2011.Valente, T. Social network thresholds in the diffusion of innovations, Social Networks, 18: 69-89, 1996.。