图像处理
图像处理技术的应用
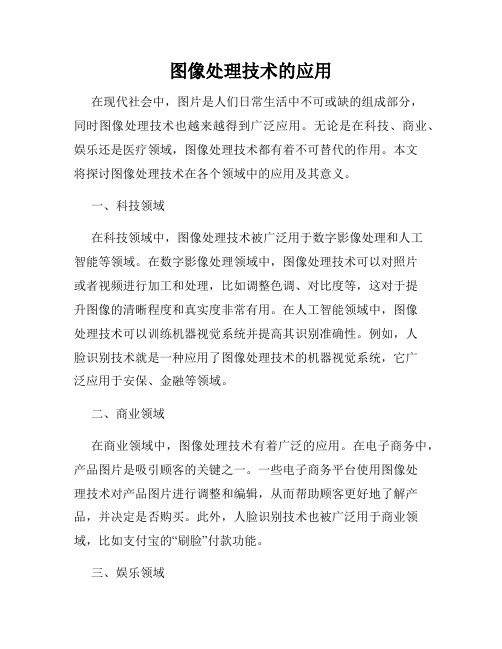
图像处理技术的应用在现代社会中,图片是人们日常生活中不可或缺的组成部分,同时图像处理技术也越来越得到广泛应用。
无论是在科技、商业、娱乐还是医疗领域,图像处理技术都有着不可替代的作用。
本文将探讨图像处理技术在各个领域中的应用及其意义。
一、科技领域在科技领域中,图像处理技术被广泛用于数字影像处理和人工智能等领域。
在数字影像处理领域中,图像处理技术可以对照片或者视频进行加工和处理,比如调整色调、对比度等,这对于提升图像的清晰程度和真实度非常有用。
在人工智能领域中,图像处理技术可以训练机器视觉系统并提高其识别准确性。
例如,人脸识别技术就是一种应用了图像处理技术的机器视觉系统,它广泛应用于安保、金融等领域。
二、商业领域在商业领域中,图像处理技术有着广泛的应用。
在电子商务中,产品图片是吸引顾客的关键之一。
一些电子商务平台使用图像处理技术对产品图片进行调整和编辑,从而帮助顾客更好地了解产品,并决定是否购买。
此外,人脸识别技术也被广泛用于商业领域,比如支付宝的“刷脸”付款功能。
三、娱乐领域在娱乐领域中,图像处理技术被广泛使用于电影、游戏制作以及虚拟现实技术中。
例如在电影制作中,图像处理技术可以让特效更加真实,让观众完全沉浸于电影中。
在游戏制作中,图像处理技术也能够让游戏画面更加逼真,提高游戏体验度。
虚拟现实技术则需要依赖于图像处理技术,这种技术可以创建出真实世界的虚拟场景,让人们在场景中感受到身临其境的感觉,这对于实现沉浸式体验至关重要。
四、医疗领域在医疗领域中,图像处理技术可以被应用于医学影像处理中。
医学影像处理是一种通过计算机来处理医学影像数据,并提取出对医生诊断、治疗具有指导意义的信息的技术。
医学影像处理可以帮助医生对患者进行更准确的诊断,并有助于医疗工作的高效率。
例如,在肿瘤检测中,医学影像处理可以使用算法进行肿瘤的分割、定位和检测等工作,这有助于医生准确诊断和治疗患者。
综上所述,图像处理技术在科技、商业、娱乐和医疗领域都有着广泛的应用。
医学图像处理

性。
03
CATALOGUE
医学图像处理的应用案例
医学影像诊断辅助系统
总结词
通过医学图像处理技术,辅助医生进行疾病诊断,提高诊断 准确率。
详细描述
医学影像诊断辅助系统利用计算机技术和图像处理算法,对 医学影像(如X光片、CT扫描、MRI等)进行分析和解读, 帮助医生快速准确地识别病变和异常情况,为临床诊断提供 有力支持。
医学图像处理涉及的领域包括医学影 像学、计算机科学、数学和物理学等 ,是医学和工程学交叉学科的一个重 要分支。
医学图像处理的重要性
提高诊断准确性和可靠性
优化治疗过程
通过医学图像处理,医生可以更准确 地识别病变和异常,减少人为因素导 致的误诊和漏诊。
通过医学图像处理,医生可以制定更 加精确的治疗计划,提高治疗效果, 减少对患者的创伤和副作用。
合并等算法。
边缘分割
03
利用边缘检测算法识别图像中的边图像识别技术
01
特征提取
从医学图像中提取出与疾病或病变相关的特征,如形状、大小、纹理等
。
02
分类器设计
利用提取的特征训练分类器,实现对医学图像的自动识别和分类。
03
深度学习在医学图像识别中的应用
利用深度学习算法对医学图像进行自动识别和分类,提高准确率和可靠
CATALOGUE
医学图像处理的挑战与未来发展
数据安全与隐私保护
数据加密存储与传输
采用高级加密算法对医学图像数据进行加密,确保数据在存储和传 输过程中的安全性。
访问控制与权限管理
建立严格的访问控制和权限管理机制,限制对医学图像数据的访问 和使用权限,防止未经授权的访问和泄露。
匿名化处理
对医学图像数据进行匿名化处理,去除患者身份信息,保护患者隐 私。
计算机图像处理学习报告
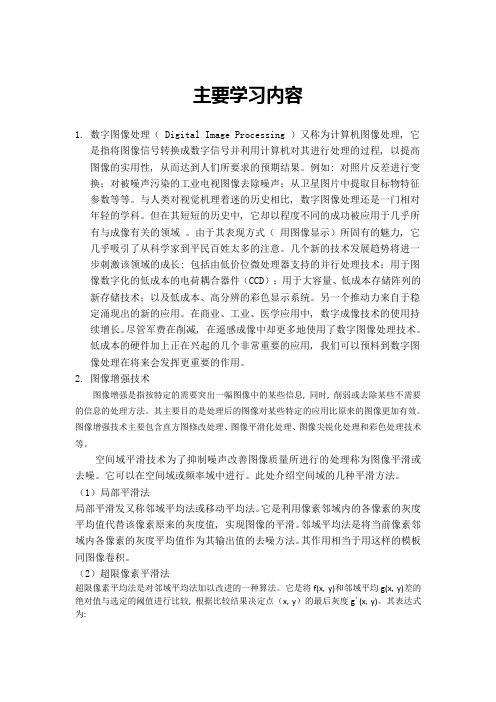
主要学习内容1.数字图像处理( Digital Image Processing )又称为计算机图像处理, 它是指将图像信号转换成数字信号并利用计算机对其进行处理的过程, 以提高图像的实用性, 从而达到人们所要求的预期结果。
例如: 对照片反差进行变换;对被噪声污染的工业电视图像去除噪声;从卫星图片中提取目标物特征参数等等。
与人类对视觉机理着迷的历史相比, 数字图像处理还是一门相对年轻的学科。
但在其短短的历史中, 它却以程度不同的成功被应用于几乎所有与成像有关的领域。
由于其表现方式(用图像显示)所固有的魅力, 它几乎吸引了从科学家到平民百姓太多的注意。
几个新的技术发展趋势将进一步刺激该领域的成长: 包括由低价位微处理器支持的并行处理技术;用于图像数字化的低成本的电荷耦合器件(CCD);用于大容量、低成本存储阵列的新存储技术;以及低成本、高分辨的彩色显示系统。
另一个推动力来自于稳定涌现出的新的应用。
在商业、工业、医学应用中, 数字成像技术的使用持续增长。
尽管军费在削减, 在遥感成像中却更多地使用了数字图像处理技术。
低成本的硬件加上正在兴起的几个非常重要的应用, 我们可以预料到数字图像处理在将来会发挥更重要的作用。
2.图像增强技术图像增强是指按特定的需要突出一幅图像中的某些信息, 同时, 削弱或去除某些不需要的信息的处理方法。
其主要目的是处理后的图像对某些特定的应用比原来的图像更加有效。
图像增强技术主要包含直方图修改处理、图像平滑化处理、图像尖锐化处理和彩色处理技术等。
空间域平滑技术为了抑制噪声改善图像质量所进行的处理称为图像平滑或去噪。
它可以在空间域或频率域中进行。
此处介绍空间域的几种平滑方法。
(1)局部平滑法局部平滑发又称邻域平均法或移动平均法。
它是利用像素邻域内的各像素的灰度平均值代替该像素原来的灰度值, 实现图像的平滑。
邻域平均法是将当前像素邻域内各像素的灰度平均值作为其输出值的去噪方法。
其作用相当于用这样的模板同图像卷积。
图像处理技术的特点和应用场景

图像处理技术的特点和应用场景随着时代的进步和科技的发展,图像处理技术在各个领域中扮演着愈加重要的角色。
它能够将数字图像处理成为更易懂、更有意义的信息,让人们更好地理解和应用它们。
在非常多的领域中,图像处理技术的应用已经逐渐走向成熟,成为社会和经济发展的新引擎,同时它也有自身的独特性质和特点。
第一特点:图像处理技术的非线性在数字图像处理中,图像是一种复杂的、非线性的数据结构。
这意味着,在一定条件下,图像处理技术可以通过线性过滤方法对其进行处理。
但在更多的情况下,图像处理需要采用非线性方法,以得到更精确的结果。
微分算子、纹理特征、形态学等非线性的技术轻而易举解决了许多线性算法无法解决的问题。
非线性算法也能够加强像素表达的具体细节和局部性,更好地反映物体特征,同时还能够实现更加稳健的算法,拥有强大的适用性。
第二特点:图像处理技术的实时性随着科技的发展和计算机硬件性能的提升,当今的图像处理技术可以实现实时处理,即当数据产生时就能够及时响应和处理。
例如,实时视频图像流处理技术可以逐一检测视频图像中的特定物体,实现实时的警报并群众准确化。
实时图像处理技术能够显著提高人们的行动速度和生产效率,在实时控制和决策方面发挥更大的作用。
第三特点:图像处理技术的可扩展性图像处理技术具有很强的可扩展性,在不断发展中逐渐形成了一个庞大的技术库。
这意味着,图像处理技术可以适应不同的领域、不同的应用场景和不同的问题。
同时,图像处理还可以通过不同的算法和技术进行优化和改进,以应对新的挑战。
一种算法可以被分解成一个操作序列,每个操作都可以用比它更基础、更强大的操作替换。
在底层操作的规范和组合下,算法能够不断发展,逐渐深入优化。
应用场景:医学影像图像处理技术在医学领域中有着很大的应用。
如CT、MRI等图像逐渐被医生用来进行诊断。
珍贵的医学影像数据中蕴含着大量的信息,如果不进行图像处理,解读这些影像就会显得困难。
因此,图像处理技术可以进行图像增强、小波变换、分割和分类等操作,提供高清晰度的影像,将潜在的病情清晰地呈现。
图像处理技术的方法和应用

图像处理技术的方法和应用随着科技的不断发展,我们的生活已经离不开图像。
我们每天在手机、电视、网络、广告等等各个方面都会看到图像。
而图像处理技术的发展,就是为了更好地满足这些需求。
图像处理技术是一种将数字信号处理和数字图像处理相结合的技术。
其主要任务是对图像进行优化,更好地呈现出图像本身所包含的信息。
在近年来,图像处理技术的应用已经涉及到了很多领域,如医学、车辆、智能设备等等。
图像处理技术的方法1. 数字信号处理方法在图像处理技术中,数字信号处理方法充分考虑到数字信号的特点,对图像进行分析、处理和识别,从而达到更好的效果。
例如,数字信号处理可以对图像进行去噪、压缩、增强等。
去噪:图像信号是由噪声和图像本身所构成的混杂信号,对其进行去噪处理可以将图像中的难以分辨的细节信息恢复出来。
压缩:图像压缩是将图像信号进行压缩和恢复处理,将大规模的图像数据变成小而有用的数据,从而方便存储和传输。
增强:图像增强是利用一系列的技术方法,增强图像的对比度、亮度以及色彩等方面的特征,使得图像更加清晰、明亮。
2. 计算机视觉方法计算机视觉是一种应用数学、计算机科学和机器学习等技术,将图像中的信息转化为数学模型,以实现图像的识别、分类、目标检测等任务。
例如,计算机视觉可以实现火车识别、人脸检测、自动驾驶等。
火车识别:利用计算机视觉技术,对图像进行识别,可以有效地实现火车识别的任务,从而实现自动化的铁路监测和安全防护。
人脸检测:利用计算机视觉技术,基于图像中的特征点信息,可以完成人脸检测的任务,从而应用于人脸识别场景。
自动驾驶:利用计算机视觉技术,结合深度学习算法,实时地获取车辆周围的信息,对路况进行分析,最终实现自动驾驶的功能。
图像处理技术的应用1. 医学领域图像处理技术在医学领域应用广泛,例如医学影像的处理、医学诊断等等。
医学影像处理技术能够对医学图像进行处理和优化,增强图像的对比度和清晰度,更好地表达患者的病情。
同时,也能够通过自动化的医学识别和自动定位等技术,实现医学诊断和治疗的智能化。
图像处理基础课程设计
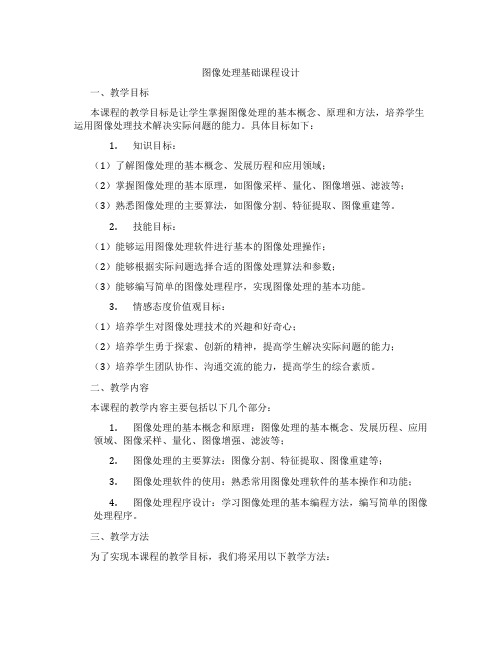
图像处理基础课程设计一、教学目标本课程的教学目标是让学生掌握图像处理的基本概念、原理和方法,培养学生运用图像处理技术解决实际问题的能力。
具体目标如下:1.知识目标:(1)了解图像处理的基本概念、发展历程和应用领域;(2)掌握图像处理的基本原理,如图像采样、量化、图像增强、滤波等;(3)熟悉图像处理的主要算法,如图像分割、特征提取、图像重建等。
2.技能目标:(1)能够运用图像处理软件进行基本的图像处理操作;(2)能够根据实际问题选择合适的图像处理算法和参数;(3)能够编写简单的图像处理程序,实现图像处理的基本功能。
3.情感态度价值观目标:(1)培养学生对图像处理技术的兴趣和好奇心;(2)培养学生勇于探索、创新的精神,提高学生解决实际问题的能力;(3)培养学生团队协作、沟通交流的能力,提高学生的综合素质。
二、教学内容本课程的教学内容主要包括以下几个部分:1.图像处理的基本概念和原理:图像处理的基本概念、发展历程、应用领域、图像采样、量化、图像增强、滤波等;2.图像处理的主要算法:图像分割、特征提取、图像重建等;3.图像处理软件的使用:熟悉常用图像处理软件的基本操作和功能;4.图像处理程序设计:学习图像处理的基本编程方法,编写简单的图像处理程序。
三、教学方法为了实现本课程的教学目标,我们将采用以下教学方法:1.讲授法:通过讲解图像处理的基本概念、原理和算法,使学生掌握相关知识;2.讨论法:引导学生分组讨论实际问题,培养学生解决问题的能力;3.案例分析法:分析典型的图像处理案例,使学生更好地理解图像处理技术的应用;4.实验法:让学生动手实践,熟悉图像处理软件和编程方法,提高学生的实际操作能力。
四、教学资源为了支持本课程的教学内容和教学方法,我们将准备以下教学资源:1.教材:选用权威、实用的图像处理教材,为学生提供系统、全面的知识体系;2.参考书:提供相关的参考书籍,丰富学生的知识储备;3.多媒体资料:制作课件、演示视频等,增强课堂教学的趣味性和生动性;4.实验设备:准备计算机、图像处理软件、编程环境等,为学生提供实践操作的机会。
图象处理基本方法
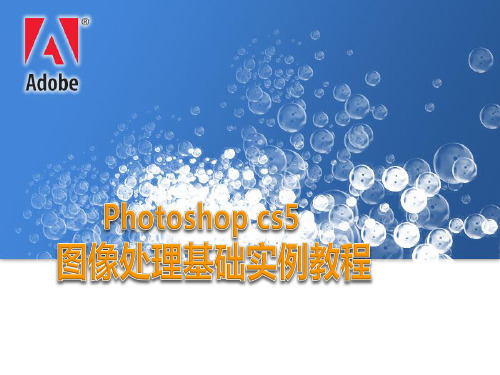
cs5
四、常用工具及文件的操作方法
磁性套索工具
磁性套索工具是一个半自动化地选区工具,其优点是能 够非常迅速,方便地选择边缘颜色对比度强的图像。
在工具选项栏“宽度”数值中输入数值,用于设置磁性 套索工具自动查寻颜色边缘地宽度范围,数值越大,所 要查寻地颜色越相似。
“边对比度”数值框中地百分比数用于设置边缘地对比 度。数值越大,磁性套索工具对颜色对比反差地敏感度 越低。
cs5
二、图形图象的基本概念
JPEG文件格式
JPEG格式是互联网中最为常用的图像格式之一,JPEG 格式支持CMYK, RGB和灰度颜色模式,也可以保存图 像中的路径,但无法保存Alpha通道。
最大优点是能够大幅度降低文件的大小,但由于降低文 件的途径是通过有选择地删除图像数据进行的,因此图 像质量有一定的损失。在将图像文件保存为JPEG格式 时,可以选择压缩的级别,级别越低得到的图像品质越 低,但文件也越小。
cs5
四、常用工具及文件的操作方法
多边形套索工具
如果要将不规则对象从复杂地背景中选择出来,多边形套索 工具是最佳选择。
使用方法:选择”套索工具”按钮后,在图像中先单击鼠标 左键确定第一点,然后围绕需要选择的图像边缘不断进行点 击,点与点之间将出现连接线,在结束绘制选区的地方双击 完成完成创建多边形选区;也可以将最后一点的光标放在第 一点上,当工具图标右下角出现一个小圆时单击鼠标即可。
cs5
四、常用工具及文件的操作方法
羽化选区
羽化是专门针对选择区域的一个参数,当一个选区具有 羽化值时,其边缘处将发生变化,选区外部的一部分像 素被选中,而选区内部的一部分像素被取消选择,具体 有多少外部的像素被选中,多少内部的像素被取消选择 ,将视羽化值的大小而定。
图形图像处理
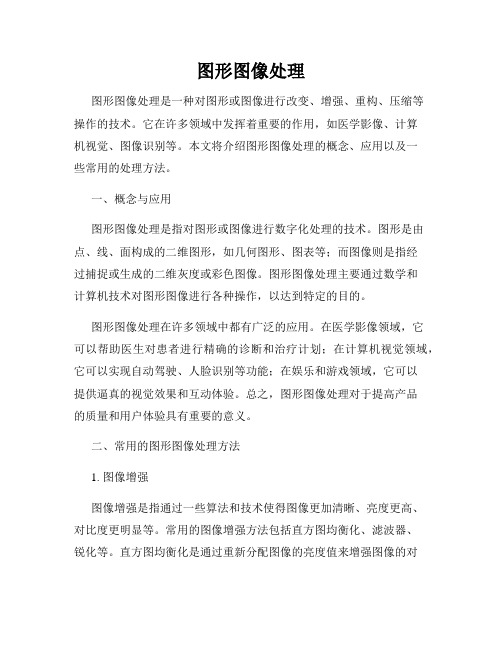
图形图像处理图形图像处理是一种对图形或图像进行改变、增强、重构、压缩等操作的技术。
它在许多领域中发挥着重要的作用,如医学影像、计算机视觉、图像识别等。
本文将介绍图形图像处理的概念、应用以及一些常用的处理方法。
一、概念与应用图形图像处理是指对图形或图像进行数字化处理的技术。
图形是由点、线、面构成的二维图形,如几何图形、图表等;而图像则是指经过捕捉或生成的二维灰度或彩色图像。
图形图像处理主要通过数学和计算机技术对图形图像进行各种操作,以达到特定的目的。
图形图像处理在许多领域中都有广泛的应用。
在医学影像领域,它可以帮助医生对患者进行精确的诊断和治疗计划;在计算机视觉领域,它可以实现自动驾驶、人脸识别等功能;在娱乐和游戏领域,它可以提供逼真的视觉效果和互动体验。
总之,图形图像处理对于提高产品的质量和用户体验具有重要的意义。
二、常用的图形图像处理方法1. 图像增强图像增强是指通过一些算法和技术使得图像更加清晰、亮度更高、对比度更明显等。
常用的图像增强方法包括直方图均衡化、滤波器、锐化等。
直方图均衡化是通过重新分配图像的亮度值来增强图像的对比度;滤波器可以消除图像中的噪声;锐化则可以使得图像的边缘更加清晰。
2. 图像处理图像处理是指对图像进行一系列的数学运算和变换,以提取出图像中的特征、进行识别和分析。
常用的图像处理方法包括图像滤波、边缘检测、形态学运算等。
图像滤波可以平滑图像,去除噪声和不必要的细节;边缘检测可以将图像中的边缘提取出来,帮助进行目标检测和识别;形态学运算可以对图像进行形状分析和重构。
3. 图像压缩图像压缩是将图像的数据进行编码,以减少存储和传输所需的空间和时间。
常用的图像压缩方法包括有损压缩和无损压缩。
有损压缩是指在压缩过程中会丢失一部分图像信息,但可以获得更高的压缩比,如JPEG压缩;无损压缩是指在压缩过程中不会丢失任何图像信息,但压缩比较低,如PNG压缩。
三、图形图像处理的挑战和发展趋势图形图像处理面临着一些挑战,如图像质量的提升、图像识别和分析的准确性等。
- 1、下载文档前请自行甄别文档内容的完整性,平台不提供额外的编辑、内容补充、找答案等附加服务。
- 2、"仅部分预览"的文档,不可在线预览部分如存在完整性等问题,可反馈申请退款(可完整预览的文档不适用该条件!)。
- 3、如文档侵犯您的权益,请联系客服反馈,我们会尽快为您处理(人工客服工作时间:9:00-18:30)。
SIAM J.I MAGING S CIENCES c2013Society for Industrial and Applied Mathematics Vol.6,No.3,pp.1345–1366A Variational Approach for Image Stitching II:Using Image Gradients ∗Michael K.Ng †and Wei Wang ‡Abstract.In [W.Wang and M.K.Ng,SIAM J.Imaging Sci.,6(2013),pp.1318–1344],we proposed and developed an image stitching algorithm by studying a variational model for automatically computing weighting mask functions on input images and stitching them together.The main aim of this paper is to further develop an image stitching algorithm using the gradients of input images.Our idea is to study a variational method for computing a stitched image by using an energy functional containing the data-fitting term based on the difference between the gradients of the stitched image and the input images,and the Laplacian regularization term based on the smoothness of weighting mask functions.The use of image gradient information allows us to automatically adjust the stitched image to handle color inconsistency across input images.In the model,we incorporate both boundary conditions of the stitched image and the weighting mask functions.The existence of a solution of the proposed energy functional is shown.We also present an alternating minimizing algorithm for solvingthe variational model numerically,and we show the convergence of this algorithm.Experimental results show that the performance of the proposed method is better than the other testing methods proposed in the literature for input images with color inconsistency.Key words.image stitching,variational model,image gradients,algorithm,weighting mask functions AMS subject classifications.65K10,68U10,91-08DOI.10.1137/rge objects often cannot be captured in a single picture under the view of camera phones or Planetary Data System cameras.Image stitching is a process that combines two or more images and blends them into one.It is the main step in the generation of panoramic images,and it is widely used in remote sensing [1,2],superresolution [3],andtexture synthesis [4].The main aim of image stitching is to find a visually acceptable orseamless blending from the input images with overlapping regions.Stitching problems usually contains two steps:image alignment and image blending.The goal of image alignment is to find corresponding point pairs in the overlapping region of two images;see,for instance,[5,6,7,8,9].Image blending combines the two aligned images seamlessly.There are two main approaches for image stitching in the literature.Optimal seam meth-ods [4,10,11,12,13,14]search for a curve in the overlapping region on which the differencesbetween two original images are minimal.Then each image is copied to the corresponding side of the curve.When the difference between two input images is zero on the curve,there will∗Received by the editors April 2,2012;accepted for publication April 10,2013;published electronically July 11,2013./journals/siims/6-3/87214.html †Centre for Mathematical Imaging and Vision and Department of Mathematics,Hong Kong Baptist University,Kowloon Tong,Hong Kong (mng@.hk ).This author’s research was supported in part by an HKRGC grant and HKBU FRG grant.‡Corresponding author.Department of Mathematics,Tongji University,Shanghai 200442,China (weiwamng@ ).This author’s research was supported by National Natural Science Foundation of China grant 11201341and China Postdoctoral Science Foundation grants 2012M511126and 2013T60459.1345D o w n l o a d e d 10/31/13 t o 218.201.115.245. R e d i s t r i b u t i o n s u b j e c t t o S I A M l i c e n s e o r c o p y r i g h t ; s e e h t t p ://w w w .s i a m .o r g /j o u r n a l s /o j s a .p h p1346MICHAEL K.NG AND WEI WANGnot be visual seams on the curve.However,the seam is visible when there is no such curve in practice.This case happens when there is a global intensity difference between the input images.The second approach uses a weighting mask over the overlapping area to smooth thetransition between the input images.In feathering [15],the stitching image is a weighted com-bination of the input images.Other image blending methods based on combined features in different resolutions are introduced in [16,17,18].The weighting mask functions vary spatiallyas a function of the distance from the seam.Pyramid blending [19]smooths the transition in each frequency band independently.The key step of this kind of approach is how to choose a weighting mask function properly.Similarly,Zomet et al.[20]and Levin et al.[21]proposed an energy functional based on image intensity and gradient for computing image stitching.In [22,23],Suen,Lam,and Wong considered optimization problems for image stitching based on image intensity,gradient,and curvature.Thebasic idea is to find a stitching image whose pixel intensity,gradient,and curvature values are close to those of the inputs.The methodcan stitch seamlessly when uniform photometric inconsistency exists.However,the weightingmask functions associated with these energy functionals are still required to choose properly in order to obtain high visual quality of image stitching ually,suitable weighting mask functions are unknown in advance.In [24],we have proposed and developed an image stitching algorithm by studying avariational method for automatically computing weighting mask functions on input images and stitching them together.In order to enhance visual quality of stitched images where inputimages have color inconsistency or different lighting conditions,a color correction procedure is required in their postprocessing step.The main aim of this paper is to further develop an imagestitching algorithm using the gradients of inputimages.Our idea is to study a variational model to compute a stitched image by using an energy functional containing the data-fitting term based on the difference between the gradients of the stitched image and the input images,and the Laplacian regularization term based onthe smoothness of weighting mask functions.The use of image gradient information allows us to automatically adjust the stitched imageto handle color inconsistency across input images.In the model,we incorporate boundary conditions of both the stitched image and the weighting mask functions.The existence of the solution of the proposed energy functional is shown.We also present an alternating minimizing algorithm to solve the variational model numerically,and we show the convergence of this algorithm.Experimental results show that the performance of the proposed method is betterthan that of the other testing methods proposed in the literature when input images have color inconsistency or different lighting conditions.This paper is organized as follows.In section 2,we describe the proposed model and show the existence of the solution of the proposed energy functional.In section 3,we present an alternating minimizing algorithm to solve the proposed model numerically,and we show theconvergence of this algorithm.In section 4,we present numerical examples which demonstrate the effectiveness of the proposed model.Finally,the concluding remarks are given in section 5.2.The proposed model.2.1.The motivation.Let us consider the following example to demonstrate the proposed model.In Figures 1(a)–1(b),there are two images to be stitched.Assume that the input images have been aligned,i.e.,we know exactly where the overlapping region is in each inputD o w n l o a d e d 10/31/13 t o 218.201.115.245. R e d i s t r i b u t i o n s u b j e c t t o S I A M l i c e n s e o r c o p y r i g h t ; s e e h t t p ://w w w .s i a m .o r g /j o u r n a l s /o j s a .p h pVARIATIONAL IMAGE STITCHING USING GRADIENTS 13471Ω0N (a)(b)(c)Figure 1.The two input images (a)I 1and (b)I 2;(c)the overlapping region Ω.image.The overlapping region Ωis shown in Figure 1(c).In [24],we study a variational approach containing an energy functional based on image intensity to determine both weighting mask functions and a stitched image.Let I 1and I 2represent the two input images.We want to determine a weighting mask function in theoverlapping region such that it is smooth based on the goal of perfect blending and also variesregularly based on our assumption of transition direction from one image to another image.The following optimization problem is studied in [24]:(2.1)min(α,u )∈Λold E old (α,u )= Ω|∇α|2dx + Ωα(u −I 1)2dx +Ω(1−α)(u −I 2)2dx ,where Λold ={(α,u )|α∈W 1,2(Ω),α∈˜Λ,0≤α≤1,u ∈L 2(Ω)}is our admissible set containing the constraints of two variables:the weighting mask functionαand the stitched image u .Here we define the gradient operator to be ∇:= ∂x ∂y .We note that the first term in (2.1)ensures thesmoothness of α,and the next two terms are interpreted as fidelity terms.When αtends to 1,u is forced to get closer to I 1.When αtendsto 0,u is forced to get closer to I 2.On the other hand,the set ˜Λfor αcoincides with the transition direction which is based on the overlapping situation of the input images in practice.As an example of the overlapping region Ωin Figure 1(c),the weighting mask function varies smoothly from 1to 0along the left-to-right direction.Then we consider Dirichlet boundary condition α=1on the vertical line marked with blue color and α=0on the vertical line marked with red color,and the Neumann boundary condition ∂α∂ n =0on the twohorizontal lines marked with green color.In particular,the set ˜Λin this example is given as follows:˜Λ= α|∂x α≤0,α=f on D,∂α∂ n =0on N ,and D ∪N =∂Ω ,where D and N refer to the boundaries for the Dirichlet and Neumann boundary conditions in ∂Ω,respectively.The situations where we want to smooth the transition from right to left D o w n l o a d e d 10/31/13 t o 218.201.115.245. R e d i s t r i b u t i o n s u b j e c t t o S I A M l i c e n s e o r c o p y r i g h t ; s e e h t t p ://w w w .s i a m .o r g /j o u r n a l s /o j s a .p h p1348MICHAEL K.NG AND WEI WANGFigure2.The stitched image by using(left)the old model in(2.1)and(right)the proposed method.(∂xα≥0),top to bottom(∂yα≤0),or bottom to top(∂yα≥0)can be handled similarly;see[24]for a detailed discussion.In Figure2(left),we give the stitched image by using themodel in(2.1).Although wefind that both input images can be stitched smoothly together,the visual quality of the stitched image is not good where the two input images have differentlighting conditions;see Figures1(a)–1(b).In this paper,our main aim is to further develop an image stitching algorithm using the gradients of input images so that the stitched image can be adjusted automatically to handlecolor inconsistency across input images;see the stitched image computed by the new modelin Figure2(right).2.2.The gradient stitching.LetΩ1(Ω2,respectively)be the region viewed exclusively inimage I1(I2,respectively)satisfyingΩ1∩Ω2=Ω1∩Ω=Ω2∩Ω=∅,and letΩc=Ω1∪Ω2∪Ωbe the combined domain(see Figure3for the example in Figure1).First,we define a newweighting mask function in the combined domainΩc based on the weighting maskαgiven inthe overlapping regionΩas follows:(2.2)ω(x,y):=⎧⎨⎩1,(x,y)∈Ω1,α(x,y),(x,y)∈Ω,0,(x,y)∈Ω2.Then we extend I1and I2into the combined domainΩc by setting proper values inΩ2andΩ1,respectively.We consider the following minimization problem:(2.3)min(α,u)∈ΛE(α,u)=Ω|∇α|2dx+Ωcω|∇u−∇I1|2dx+Ωc(1−ω)|∇u−∇I2|2dx, whereΛ=(α,u)|α∈W1,2(Ω),α∈˜Λ,0≤α≤1,u∈W1,2(Ωc),u=I1on∂Ω1∩∂Ωc,u=I2on∂Ω2∩∂Ωc,∂u∂ n=0on N,I min≤u≤I max(2.4)is our admissible set containing the constraints.Here I min and I max are the minimum andmaximum values,respectively,in I1and I2.We remark that the gradients of the stitchedimage and the weighting mask functions are involved in(2.3);thus their boundary conditionsmust be included in(2.4)in order to guarantee the existence of the solution of(2.3).Downloaded1/31/13to218.21.115.245.RedistributionsubjecttoSIAMlicenseorcopyright;seehttp://www.siam.org/journals/ojsa.phpVARIATIONAL IMAGE STITCHING USING GRADIENTS 1349Ω1ΩΩ2Figure 3.The combined domain Ωc for the example in Figure 1.In the previous subsection,we have discussed how to set the boundary condition of α.The boundary condition for u can be defined similarly.As an example,the new weighting mask function ωin Figure 3varies smoothly from 1to 0along the left-to-right direction for thetwo input images in Figures 1(a)–1(b).Moreover,we have the following Dirichlet boundary condition:the values of the stitched image u are equal to the values of the input image I 1on the line marked with blue color,and the values of the stitched image u are equal to the values of the input image I 2on the line marked with red color.The two horizontal lines marked with green color are set for the Neumann boundary condition ∂u ∂ n =0.According to this setting,we keep the colors of I 1and I 2in the left-and right-hand sides of the stitchedimage,respectively,and fuse their colors smoothly in the overlapping region of the stitched image.We see from Figure 2(c)that the new model can provide better visual quality of the stitched image than that of the previous model.In section 4,we will show more examples ofhow to set the boundary condition of u to stitch input images together.2.3.Mathematical analysis.In this subsection,we analyze the existence of a solution for (2.3).Let us consider the unconstrained form of the problem (2.3)as follows:(2.5)min (α,u,v )∈Λ0{E1(α,u,v )},whereE 1(α,u,v )≡ Ω|∇α|2dx+Ωc ω|∇u −∇I 1|2dx+ Ωc(1−ω)|∇u −∇I 2|2dx+μ2 Ω|∂x α+v |2dx ,Λ0=(α,u )|α∈W 1,2(Ω),0≤α≤1,α=f on D,∂α∂ n =0on N ,u ∈W 1,2(Ωc ),u =I 1on ∂Ω1∩∂Ωc ,u =I 2on ∂Ω2∩∂Ωc ,∂u∂ n =0on N ,I min ≤u ≤I max ,v ≥0.We introduce a new variable v and add a penalty term to handle the constraint ∂x α≤0.If μis large enough,v ≥0guarantees ∂x α≤0.Then we have the following theorem for problem (2.5).Theorem 2.1.Assume f is continuous on ∂Ω,and 0≤f ≤1.Assume I 1,I 2∈W 1,2(Ωc ),and I 1and I 2are continuous on ∂Ω1∩∂Ωc and ∂Ω2∩∂Ωc ,respectively.Then the problem in (2.5)has at least one solution.D o w n l o a d e d 10/31/13 t o 218.201.115.245. R e d i s t r i b u t i o n s u b j e c t t o S I A M l i c e n s e o r c o p y r i g h t ; s e e h t t p ://w w w .s i a m .o r g /j o u r n a l s /o j s a .p h p1350MICHAEL K.NG AND WEI WANGProof .It is well known that,with the requirements that the length of D be nonzero and f be continuous,the solution of the problem min α=f on D,∂α∂ n=0on NΩ|∇α|2dx exists and can be derived by solving the following equation:⎧⎨⎩Δα=0in Ω,α=fon D,∂α∂ n =0on N .The above statement can be found in Chapter 2of [25].Then we denote the solution as ˆα,and by the maximum principle,we can easily obtain 0≤ˆα≤1.We can also get ˆu satisfying the constraints in Λ0by using the same arguments.Then we choose α=ˆα,u =ˆu ,and v =1,and our energy E 1(α,u,v )will be finite.This implies that the infimum of the energy E 1must be finite.Suppose {(αn ,˜u n ,v n )}⊂Λ0is a minimizing sequence of problem (2.5).Then there existsa constant M >0such that E 1(αn ,˜u n ,v n )≤M,which implies Ω|∇αn |2dx ≤M and Ω|∂x αn +v n |2dx ≤M.Associated with the boundedness of α,we derive that {αn }is uniformly bounded in W 1,2(Ω),and {v n }is uniformly bounded in L 2(Ω).Noting that W 1,2(Ω)is compactly embedded in L 2(Ω),we deduce that up to a subsequence αn converges to α∗∈W 1,2(Ω),and v n weakly converges to v ∗∈L 2(Ω);i.e.,(2.6)αn −−−−→L 2(Ω)α∗,and v n v ∗∈L 2(Ω).Now we can derive the corresponding ωn based on αn ,and we can easily deduce ωn −−−−→L 2(Ωc )ω∗,where ω∗=⎧⎨⎩1,x ∈Ω1,α∗,x ∈Ω,0,x ∈Ω2.Then we fix αn and v n to consider the following problem:min (αn ,u,v n )∈Λ0{E 1(αn ,u,v n )}.We denote the solution of the above problem as u n .Thus,E 1(αn ,u n ,v n )≤E 1(αn ,˜u n ,v n ).This means {(αn ,u n ,v n )}is also a minimizing sequence of problem (2.5).From Theorem 2in [21],we know that the minimum of E 1(αn ,u,v n)is obtained with the image having the closest D o w n l o a d e d 10/31/13 t o 218.201.115.245. R e d i s t r i b u t i o n s u b j e c t t o S I A M l i c e n s e o r c o p y r i g h t ; s e e h t t p ://w w w .s i a m .o r g /j o u r n a l s /o j s a .p h pVARIATIONAL IMAGE STITCHING USING GRADIENTS 1351derivatives to ωn ∇I 1+(1−ωn )∇I 2under the L 2norm.Noting that I 1,I 2∈W 1,2(Ωc ),we have that Ω|∇u n |2dx is uniformly bounded,and I min ≤u n ≤I max ;i.e.,{u n }is uniformly bounded in W 1,2(Ωc ).We deduce that up to a subsequence u n converges to I min ≤u ∗∈W 1,2(Ωc )≤I max ,and ∇u n weakly converges to∇u ∗∈L 2(Ωc );i.e.,(2.7)u n −−−−→L 2(Ωc )u ∗,and ∇u n ∇u ∗∈L 2(Ωc ).The last convergence result can be derived in the same way as ∇αn converges;see the following discussion.Then we can rewrite the first three terms of E 1(αn ,u n ,v n ),associated with (2.6),(2.7),and from Theorem 2.1.3in [26],the dominated convergence theorem,and the convexity of the three terms in ∇α,∇u ,we have the following lower semicontinuity:lim infn →∞ Ω|∇αn |2dx + Ωc ωn |∇u n −∇I 1|2dx + Ωc(1−ωn )|∇u n −∇I 2|2dx =lim infn →∞ Ω|∇αn |2dx + Ωc ωn |∇I 1|2dx + Ωc(1−ωn )|∇I 2|2dx +Ωc |∇u n |2dx −2 Ωc ωn ∇u n ·∇I 1dx −2 Ωc(1−ωn )∇u n ·∇I 2dx ≥Ω|∇α∗|2dx + Ωc ω∗|∇I 1|2dx + Ωc (1−ω∗)|∇I 2|2dx)+Ωc |∇u ∗|2dx −2 Ωc ω∗∇u ∗·∇I 1dx −2 Ωc(1−ω∗)∇u ∗·∇I 2dx =Ω|∇α∗|2dx + Ωcω∗|∇u ∗−∇I 1|2dx +Ωc (1−ω∗)|∇u ∗−∇I 2|2dx .By using (2.6),we know that αn →α∗,a .e .in Ω.Noting that 0≤αn ≤1,we deduce0≤α∗≤1,a .e .in Ω.Then we claim thatα∗=f on D,∂α∗∂ n =0on N .Indeed,αn is bounded in BV (Ω).Then up to a subsequence we have ∇αn ∗−→∇α∗in a measure sense;i.e.,for any ϕ∈C ∞0(Ω)2,the following convergence holds:(2.8)Ωϕ·∇αn dx →Ωϕ·∇α∗dx .Noting that ∇αn is bounded in L 2(Ω),we havethat up to a subsequence it weakly converges in L 2(Ω).By combining (2.8)and the property that a function in L 2(Ω)can be approximatedby smooth functions,we obtain the following result:∇αn ∇α∗in L 2(Ω).Then we choose any φ∈C ∞(¯Ω)2,where ¯Ωmeans the closure of Ω,and we have Ωφ·∇αn dx →Ωφ·∇α∗dx .D o w n l o a d e d 10/31/13 t o 218.201.115.245. R e d i s t r i b u t i o n s u b j e c t t o S I A M l i c e n s e o r c o p y r i g h t ; s e e h t t p ://w w w .s i a m .o r g /j o u r n a l s /o j s a .p h p1352MICHAEL K.NG AND WEI WANGBy using the formula of integration by parts, ∂Ωαn φ· n d H 1− Ωαn div φdx →∂Ωα∗φ· n d H 1−Ωα∗div φdx ,where n is the normal vector of ∂Ω,and H 1is the one-dimensional Hausdorffmeasure.Noting (2.6),we have Ωαn div φdx → Ωα∗div φdx .Then combining the boundary condition,we deriveD fφ· n d H 1= D α∗φ· n d H 1+ Nα∗φ· n d H 1.Because this is valid for any φ,and f is continuous,we obtain that α∗=f on D and ∂α∗∂ n =0on N .We remark here that we can get u ∗|∂Ω1∩∂Ωc =I 1,u ∗|∂Ω2∩∂Ω=I 2,∂u ∗∂ n =0on N byusing similar arguments.As a consequence of the lower semicontinuity for the L 2norm,lim infn →∞ Ω|∂x αn +v n |2dx ≥ Ω|∂x α∗+v ∗|2dx .Therefore,min (α,u,v )∈Λ0{E 1(α,u,v )}=lim inf n →∞E 1(αn ,u n ,v n )≥E 1(α∗,u ∗,v ∗),and we have α∗=f on D ,∂α∗∂ n =0on N ,0≤α∗≤1;u ∗|∂Ω1∩∂Ωc =I 1,u ∗|∂Ω2∩∂Ωc =I 2,∂u ∗∂ n =0on N ,I min ≤u ∗∈W1,2(Ωc )≤I max ;and v ∗≥0.This completes the proof.3.The alternating minimization algorithm.In this section,we develop a numerical method for solving problem (2.3).We first transform problem (2.3)into the following equiv-alent problem:(3.1)min α∈W 1,2(Ω),u ∈W 1,2(Ωc ),v ∈L 2(Ω){E 2(α,u,v )}subject to ∂x α+v =0,v ≥0,0≤α≤1,α=f on D,∂α∂ n=0on N ,u |∂Ω1∩∂Ωc =I 1,u |∂Ω2∩∂Ωc =I 2,∂u∂ n =0on N ,I min ≤u ≤I max ,whereE 2(α,u,v )≡ Ω|∇α|2dx + Ωc ω|∇u −∇I 1|2dx + Ωc (1−ω)|∇u −∇I 2|2dx .There are three variables α,u ,and v in the optimization problem.A simple but effectivestrategy is to use an alternating minimization scheme for solving the optimization problem as follows.D o w n l o a d e d 10/31/13 t o 218.201.115.245. R e d i s t r i b u t i o n s u b j e c t t o S I A M l i c e n s e o r c o p y r i g h t ; s e e h t t p ://w w w .s i a m .o r g /j o u r n a l s /o j s a .p h pVARIATIONAL IMAGE STITCHING USING GRADIENTS 1353Algorithm 1.(i)Let α0=α0be the initial mask function.(ii)At the k th iteration:•Given αk ,generate ωk based on (2.2),and compute u k +1by solving (3.2)min uE 3(u )≡Ωcωk |∇u −∇I 1|2dx + Ωc(1−ωk )|∇u −∇I 2|2dx subject tou |∂Ω1∩∂Ωc =I 1,u |∂Ω2∩∂Ωc =I 2,∂u ∂ n =0on N ,I min ≤u ≤I max .•Given u k +1,compute αk +1,vk +1by solving min α∈W 1,2(Ω),v ∈L 2(Ω)E 4(α,v )(3.3)≡Ω|∇α|2dx + Ωα(|∇u k +1−∇I 1|2−|∇u k +1−∇I 2|2)dx ,subject to∂x α+v =0,v ≥0,0≤α≤1,α=f on D,∂α∂ n =0on N .(iii)Go back to step (ii)until ||αk +1−αk ||||αk +1||≤ αand ||u k +1−u k ||||u k +1||≤ u .3.1.Solution of u -subproblem.For the minimization subproblem in (3.2),we first in-troduce notation ι1(w ):=0,I min ≤w ≤I max ,+∞otherwise ,H 1(u )=Ωc ωk |∇u −∇I 1|2dx + Ωc (1−ωk )|∇u −∇I 2|2dx .Then we can rewrite problem (3.2)as follows:(3.4)min u,w{E 5(u,w )≡ι1(w )+H 1(u )}subject to u −w =0,u |∂Ω1∩∂Ωc =I 1,u |∂Ω2∩∂Ωc =I 2,∂u ∂ n=0on N .For this constrained optimization problem,we can employ the alternating direction methodof multipliers (ADMM),which is introduced in [27,28,29]to solve this problem.By using the Lagrangian multiplier λ1to the linear constraint,the augmented Lagrangian function of (3.4)is given by L 1(u,w,λ1)=ι1(w )+H 1(u )+ λ1,u −w +μ12||u −w ||2.D o w n l o a d e d 10/31/13 t o 218.201.115.245. R e d i s t r i b u t i o n s u b j e c t t o S I A M l i c e n s e o r c o p y r i g h t ; s e e h t t p ://w w w .s i a m .o r g /j o u r n a l s /o j s a .p h p1354MICHAEL K.NG AND WEI WANGHere the scalar product ·,· is the inner product of L 2(Ωc ).Then the ADMM iterations are described in the following steps:(3.5)w i +1=argmin wL 1(u i ,w,λi1),(3.6)u i +1=argmin u |∂Ω1∩∂Ωc =I 1,u |∂Ω2∩∂Ωc =I 2,∂u∂ n =0on N L 1(u,w i +1,λi1),(3.7)λi +11=λi 1+μ1(u i +1−w i +1).We rewrite problem (3.5)as follows:w i +1=argmin w ι1(w )+μ12w −u i −λi 1μ1 2 .It is obvious that w i +1is equal to the following projection:w i +1=max min u i +λi 1μ1,I max ,I min .The Euler–Lagrange equation for problem (3.6)is given by the following equation:(∂T x ∂x +∂T y ∂y +μ1I )u =∂T x ωk ∂x I 1+∂T y ωk ∂y I 1+∂T x (1−ωk )∂x I 2+∂Ty (1−ωk )∂y I 2+μ1w i +1−λi 1.Here we note that ∂x and ∂y are the matrix forms of the corresponding discrete convolution operators,and I is the identity matrix.As the coefficient matrix of the above linear system isgiven by the second-order differential operator with Dirichlet boundary conditions,this matrix equation can be solved by many efficient numerical solvers and preconditioning techniques;see,for instance,the book by Saad [30].3.2.Solution of α-subproblem.For the minimization subproblem in (3.3),we make use of the variable-splitting technique to solve the constrained problem.Similarly,let’s firstintroduce some notation.For simplicity,we denote g =|∇u k +1−∇I 1|2−|∇uk +1−∇I 2|2and denote ι2,ι3as the indicator functions for the sets 0≤α≤1,v ≥0,respectively.Moreprecisely,they are defined as follows:ι2(α):=0,0≤α≤1,+∞otherwiseand ι3(v ):=0,v ≥0,+∞otherwise .D o w n l o a d e d 10/31/13 t o 218.201.115.245. R e d i s t r i b u t i o n s u b j e c t t o S I A M l i c e n s e o r c o p y r i g h t ; s e e h t t p ://w w w .s i a m .o r g /j o u r n a l s /o j s a .p h pOn the other hand,we denoteH 2(α)= Ω|∇α|2dx + Ωαg dx;then we can rewrite problem (3.3)as follows:(3.8)min α,β,v{E 6(α,β,v )≡ι1(β)+ι2(v )+H 2(α)},subject to ∂x α+v =0,α−β=0,α=f on D,∂α∂ n =0on N .For this constrained optimization problem,we can also employ ADMM to solve this problem.By using the Lagrangian multiplier λ2,λ3to the linear constraint,the augmented Lagrangianfunction of (3.8)is given byL 2(α,β,v,λ2,λ3)=ι2(β)+ι3(v )+H 2(α)+ λ2,α−β + λ3,∂x α+v +μ22||∂x α+v ||2+μ22||α−β||2.We penalize the last two norms equally usingthe same parameter μ2.Then the ADMM iterations are described in the following steps:(3.9)(βi +1,v i +1)=argmin β,v L 2(αi ,β,v,λi 2,λi3),(3.10)αi +1=argmin α=f on D,∂α∂ n=0on N L 2(α,βi +1,v i +1,λi 2,λi3),(3.11)λi +12=λi2+μ2(αi +1−βi +1),(3.12)λi +13=λi 3+μ2(∂x αi +1+v i +1).We rewrite problem (3.9)in detail as follows:(βi +1,v i +1)=argmin β,vι2(β)+μ22 β−αi −λi 2μ2 2+ι3(v )+μ22 v +∂x αi +λi 3μ2 2 .It is obvious that βi +1and v i +1are equal to the following two projections:βi +1=max min αi +λi 2μ2,1 ,0 ,D o w n l o a d e d 10/31/13 t o 218.201.115.245. R e d i s t r i b u t i o n s u b j e c t t o S I A M l i c e n s e o r c o p y r i g h t ; s e e h t t p ://w w w .s i a m .o r g /j o u r n a l s /o j s a .p h pv i +1=max −∂x αi −λi 3μ2,0 .The Euler–Lagrange equation for problem (3.10)is given by the following equation:(3.13)((2+μ2)∂T x∂x +2∂Ty ∂y+μ2I )α=−∂T x (μ2v i +1+λ3)−λ2−g +μ2βi +1.The coefficient matrix of the above linear system is given by the second-order differential operator with the Dirichlet and Neumann boundary conditions.This matrix equation can also be solved by many efficient numerical solvers and preconditioning techniques;see the book by Saad [30].In particular,we consider the overlapping region to be rectangular like the example inFigure 1.In this case,the Dirichlet boundary conditions are on the blue line and the red line,and the Neumann boundary conditions are on the green lines.In [31],it was mentionedthat the linear system in (3.13)arising from the differential operator with the Dirichlet and Neumann boundary conditions can be diagonalized by using the discrete sine transform matrix in the x -direction and the discrete cosine transform matrix in the y -direction.As we can make use of fast sine and cosine transforms to implement these computation tasks,the cost of solving such a linear system is O (N log N ),where N is the number of pixels in the overlapping region.3.3.Convergence analysis.In this subsection,we study the convergence of Algorithm 1.We first note that the convergence for ADMM [32]can be used here for the proposed method-ology for solving the problem in (3.4)and (3.8).We conclude the convergence for ADMM asthe following theorem.Theorem 3.1.For the problem in (3.4)and (3.8),let u 0,λ01and α0,λ02,λ03be arbitrary,andlet μ1,μ2>0.Then the sequences {u k ,w k ,λk 1}and {(αk ,βk ,v k ,λk 2,λk 3)}generated by (3.5)–(3.7)and (3.9)–(3.12)converge to (u ∗,w ∗,λ∗1)and (α∗,β∗,v ∗,λ∗2,λ∗3),which are saddle points of L 1and L 2.Now we can show the convergence of Algorithm 1.Theorem 3.2.Suppose I 1,I 2∈W 1,2(Ωc ).Let ˆΛ= (α,u,v )|(α,u,v )∈W 1,2(Ω)×W 1,2(Ωc )×L 2(Ω),0≤α≤1,α=f on D,∂α∂ n =0on N ,∂x α+v =0,u |∂Ω1∩∂Ωc =I 1,u |∂Ω2∩∂Ωc =I 2,∂u∂ n =0on N ,I min ≤u ≤I max ,v ≥0 .Let {(αk ,u k ,v k )}be the sequence generated by Algorithm 1.Then {(αk ,u k ,v k )}converges to(α∗,u ∗,v ∗)∈ˆΛ(up to a subsequence),and for any (α,u,v )∈ˆΛ,we have E 2(α∗,u ∗,v ∗)≤E 2(α,u ∗,v ),E 2(α∗,u ∗,v ∗)≤E 2(α∗,u,v ∗).Proof .First,we deduce the following inequality from Algorithm 1:E 2(αk +1,u k +1,v k +1)≤E 2(αk ,uk +1,v k)≤E 2(αk ,u k ,v k ).D o w n l o a d e d 10/31/13 t o 218.201.115.245. R e d i s t r i b u t i o n s u b j e c t t o S I A M l i c e n s e o r c o p y r i g h t ; s e e h t t p ://w w w .s i a m .o r g /j o u r n a l s /o j s a .p h p。