大数据外文翻译参考文献综述
大数据时代文献综述(一)2024
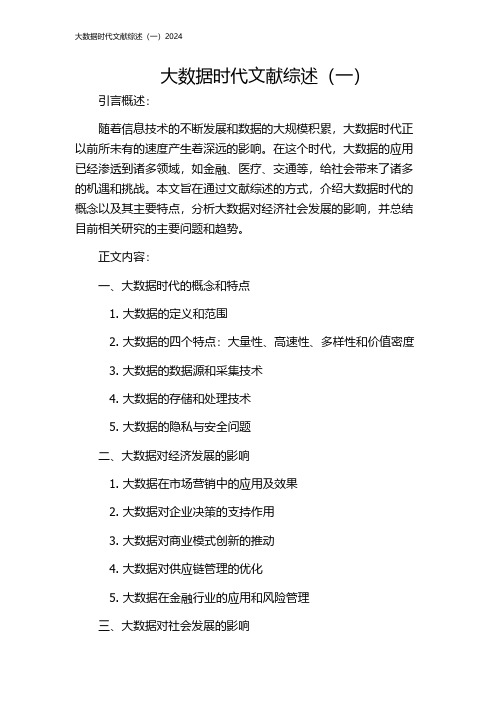
大数据时代文献综述(一)引言概述:随着信息技术的不断发展和数据的大规模积累,大数据时代正以前所未有的速度产生着深远的影响。
在这个时代,大数据的应用已经渗透到诸多领域,如金融、医疗、交通等,给社会带来了诸多的机遇和挑战。
本文旨在通过文献综述的方式,介绍大数据时代的概念以及其主要特点,分析大数据对经济社会发展的影响,并总结目前相关研究的主要问题和趋势。
正文内容:一、大数据时代的概念和特点1. 大数据的定义和范围2. 大数据的四个特点:大量性、高速性、多样性和价值密度3. 大数据的数据源和采集技术4. 大数据的存储和处理技术5. 大数据的隐私与安全问题二、大数据对经济发展的影响1. 大数据在市场营销中的应用及效果2. 大数据对企业决策的支持作用3. 大数据对商业模式创新的推动4. 大数据对供应链管理的优化5. 大数据在金融行业的应用和风险管理三、大数据对社会发展的影响1. 大数据在医疗领域的应用和医疗服务的改进2. 大数据对教育领域的影响和学习模式的改变3. 大数据在城市规划和交通管理中的应用4. 大数据对环境保护与可持续发展的促进5. 大数据对政府决策与治理的影响四、大数据研究的主要问题和趋势1. 大数据的质量与准确性问题2. 大数据融合与共享的难题3. 大数据的处理与分析技术的挑战4. 大数据隐私保护的法律与伦理问题5. 大数据人才培养与研究的跨学科合作五、总结在大数据时代,大数据的产生和应用不仅带来了巨大的机遇,也带来了诸多挑战。
大数据已经对经济社会发展产生了深远影响,但同时也暴露出一系列问题。
未来,需要进一步研究与探索大数据的质量与准确性、处理与分析技术以及隐私保护等方面的问题,加强跨学科合作,培养专业人才,以更好地应对大数据时代的挑战与机遇。
文末总结。
大数据外文翻译参考文献综述
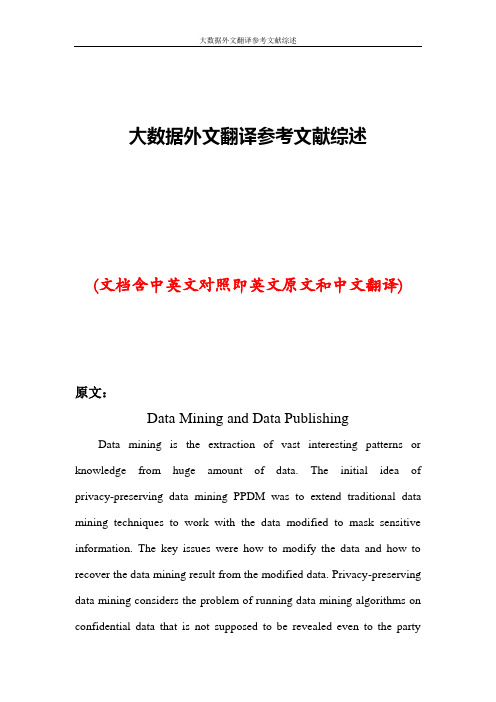
大数据外文翻译参考文献综述(文档含中英文对照即英文原文和中文翻译)原文:Data Mining and Data PublishingData mining is the extraction of vast interesting patterns or knowledge from huge amount of data. The initial idea of privacy-preserving data mining PPDM was to extend traditional data mining techniques to work with the data modified to mask sensitive information. The key issues were how to modify the data and how to recover the data mining result from the modified data. Privacy-preserving data mining considers the problem of running data mining algorithms on confidential data that is not supposed to be revealed even to the partyrunning the algorithm. In contrast, privacy-preserving data publishing (PPDP) may not necessarily be tied to a specific data mining task, and the data mining task may be unknown at the time of data publishing. PPDP studies how to transform raw data into a version that is immunized against privacy attacks but that still supports effective data mining tasks. Privacy-preserving for both data mining (PPDM) and data publishing (PPDP) has become increasingly popular because it allows sharing of privacy sensitive data for analysis purposes. One well studied approach is the k-anonymity model [1] which in turn led to other models such as confidence bounding, l-diversity, t-closeness, (α,k)-anonymity, etc. In particular, all known mechanisms try to minimize information loss and such an attempt provides a loophole for attacks. The aim of this paper is to present a survey for most of the common attacks techniques for anonymization-based PPDM & PPDP and explain their effects on Data Privacy.Although data mining is potentially useful, many data holders are reluctant to provide their data for data mining for the fear of violating individual privacy. In recent years, study has been made to ensure that the sensitive information of individuals cannot be identified easily.Anonymity Models, k-anonymization techniques have been the focus of intense research in the last few years. In order to ensure anonymization of data while at the same time minimizing the informationloss resulting from data modifications, everal extending models are proposed, which are discussed as follows.1.k-Anonymityk-anonymity is one of the most classic models, which technique that prevents joining attacks by generalizing and/or suppressing portions of the released microdata so that no individual can be uniquely distinguished from a group of size k. In the k-anonymous tables, a data set is k-anonymous (k ≥ 1) if each record in the data set is in- distinguishable from at least (k . 1) other records within the same data set. The larger the value of k, the better the privacy is protected. k-anonymity can ensure that individuals cannot be uniquely identified by linking attacks.2. Extending ModelsSince k-anonymity does not provide sufficient protection against attribute disclosure. The notion of l-diversity attempts to solve this problem by requiring that each equivalence class has at least l well-represented value for each sensitive attribute. The technology of l-diversity has some advantages than k-anonymity. Because k-anonymity dataset permits strong attacks due to lack of diversity in the sensitive attributes. In this model, an equivalence class is said to have l-diversity if there are at least l well-represented value for the sensitive attribute. Because there are semantic relationships among the attribute values, and different values have very different levels of sensitivity. Afteranonymization, in any equivalence class, the frequency (in fraction) of a sensitive value is no more than α.3. Related Research AreasSeveral polls show that the public has an in- creased sense of privacy loss. Since data mining is often a key component of information systems, homeland security systems, and monitoring and surveillance systems, it gives a wrong impression that data mining is a technique for privacy intrusion. This lack of trust has become an obstacle to the benefit of the technology. For example, the potentially beneficial data mining re- search project, Terrorism Information Awareness (TIA), was terminated by the US Congress due to its controversial procedures of collecting, sharing, and analyzing the trails left by individuals. Motivated by the privacy concerns on data mining tools, a research area called privacy-reserving data mining (PPDM) emerged in 2000. The initial idea of PPDM was to extend traditional data mining techniques to work with the data modified to mask sensitive information. The key issues were how to modify the data and how to recover the data mining result from the modified data. The solutions were often tightly coupled with the data mining algorithms under consideration. In contrast, privacy-preserving data publishing (PPDP) may not necessarily tie to a specific data mining task, and the data mining task is sometimes unknown at the time of data publishing. Furthermore, some PPDP solutions emphasize preserving the datatruthfulness at the record level, but PPDM solutions often do not preserve such property. PPDP Differs from PPDM in Several Major Ways as Follows :1) PPDP focuses on techniques for publishing data, not techniques for data mining. In fact, it is expected that standard data mining techniques are applied on the published data. In contrast, the data holder in PPDM needs to randomize the data in such a way that data mining results can be recovered from the randomized data. To do so, the data holder must understand the data mining tasks and algorithms involved. This level of involvement is not expected of the data holder in PPDP who usually is not an expert in data mining.2) Both randomization and encryption do not preserve the truthfulness of values at the record level; therefore, the released data are basically meaningless to the recipients. In such a case, the data holder in PPDM may consider releasing the data mining results rather than the scrambled data.3) PPDP primarily “anonymizes” the data by hiding the identity of record owners, whereas PPDM seeks to directly hide the sensitive data. Excellent surveys and books in randomization and cryptographic techniques for PPDM can be found in the existing literature. A family of research work called privacy-preserving distributed data mining (PPDDM) aims at performing some data mining task on a set of private databasesowned by different parties. It follows the principle of Secure Multiparty Computation (SMC), and prohibits any data sharing other than the final data mining result. Clifton et al. present a suite of SMC operations, like secure sum, secure set union, secure size of set intersection, and scalar product, that are useful for many data mining tasks. In contrast, PPDP does not perform the actual data mining task, but concerns with how to publish the data so that the anonymous data are useful for data mining. We can say that PPDP protects privacy at the data level while PPDDM protects privacy at the process level. They address different privacy models and data mining scenarios. In the field of statistical disclosure control (SDC), the research works focus on privacy-preserving publishing methods for statistical tables. SDC focuses on three types of disclosures, namely identity disclosure, attribute disclosure, and inferential disclosure. Identity disclosure occurs if an adversary can identify a respondent from the published data. Revealing that an individual is a respondent of a data collection may or may not violate confidentiality requirements. Attribute disclosure occurs when confidential information about a respondent is revealed and can be attributed to the respondent. Attribute disclosure is the primary concern of most statistical agencies in deciding whether to publish tabular data. Inferential disclosure occurs when individual information can be inferred with high confidence from statistical information of the published data.Some other works of SDC focus on the study of the non-interactive query model, in which the data recipients can submit one query to the system. This type of non-interactive query model may not fully address the information needs of data recipients because, in some cases, it is very difficult for a data recipient to accurately construct a query for a data mining task in one shot. Consequently, there are a series of studies on the interactive query model, in which the data recipients, including adversaries, can submit a sequence of queries based on previously received query results. The database server is responsible to keep track of all queries of each user and determine whether or not the currently received query has violated the privacy requirement with respect to all previous queries. One limitation of any interactive privacy-preserving query system is that it can only answer a sublinear number of queries in total; otherwise, an adversary (or a group of corrupted data recipients) will be able to reconstruct all but 1 . o(1) fraction of the original data, which is a very strong violation of privacy. When the maximum number of queries is reached, the query service must be closed to avoid privacy leak. In the case of the non-interactive query model, the adversary can issue only one query and, therefore, the non-interactive query model cannot achieve the same degree of privacy defined by Introduction the interactive model. One may consider that privacy-reserving data publishing is a special case of the non-interactivequery model.This paper presents a survey for most of the common attacks techniques for anonymization-based PPDM & PPDP and explains their effects on Data Privacy. k-anonymity is used for security of respondents identity and decreases linking attack in the case of homogeneity attack a simple k-anonymity model fails and we need a concept which prevent from this attack solution is l-diversity. All tuples are arranged in well represented form and adversary will divert to l places or on l sensitive attributes. l-diversity limits in case of background knowledge attack because no one predicts knowledge level of an adversary. It is observe that using generalization and suppression we also apply these techniques on those attributes which doesn’t need th is extent of privacy and this leads to reduce the precision of publishing table. e-NSTAM (extended Sensitive Tuples Anonymity Method) is applied on sensitive tuples only and reduces information loss, this method also fails in the case of multiple sensitive tuples.Generalization with suppression is also the causes of data lose because suppression emphasize on not releasing values which are not suited for k factor. Future works in this front can include defining a new privacy measure along with l-diversity for multiple sensitive attribute and we will focus to generalize attributes without suppression using other techniques which are used to achieve k-anonymity because suppression leads to reduce the precision ofpublishing table.译文:数据挖掘和数据发布数据挖掘中提取出大量有趣的模式从大量的数据或知识。
文献综述外文翻译写作规范及要求
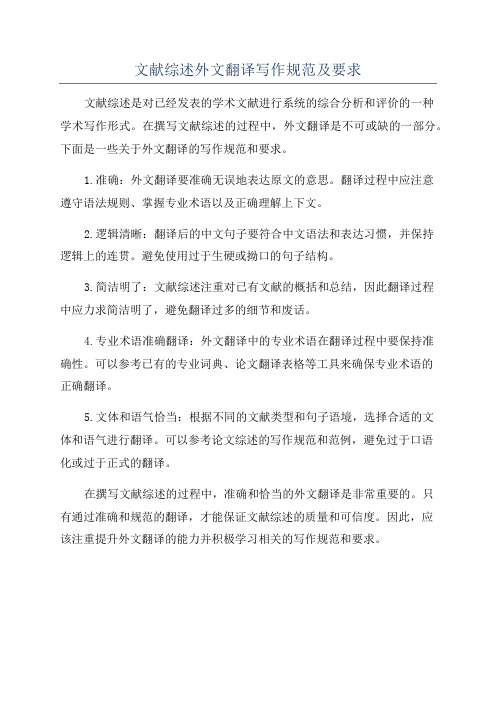
文献综述外文翻译写作规范及要求
文献综述是对已经发表的学术文献进行系统的综合分析和评价的一种
学术写作形式。
在撰写文献综述的过程中,外文翻译是不可或缺的一部分。
下面是一些关于外文翻译的写作规范和要求。
1.准确:外文翻译要准确无误地表达原文的意思。
翻译过程中应注意
遵守语法规则、掌握专业术语以及正确理解上下文。
2.逻辑清晰:翻译后的中文句子要符合中文语法和表达习惯,并保持
逻辑上的连贯。
避免使用过于生硬或拗口的句子结构。
3.简洁明了:文献综述注重对已有文献的概括和总结,因此翻译过程
中应力求简洁明了,避免翻译过多的细节和废话。
4.专业术语准确翻译:外文翻译中的专业术语在翻译过程中要保持准
确性。
可以参考已有的专业词典、论文翻译表格等工具来确保专业术语的
正确翻译。
5.文体和语气恰当:根据不同的文献类型和句子语境,选择合适的文
体和语气进行翻译。
可以参考论文综述的写作规范和范例,避免过于口语
化或过于正式的翻译。
在撰写文献综述的过程中,准确和恰当的外文翻译是非常重要的。
只
有通过准确和规范的翻译,才能保证文献综述的质量和可信度。
因此,应
该注重提升外文翻译的能力并积极学习相关的写作规范和要求。
外文文献综述
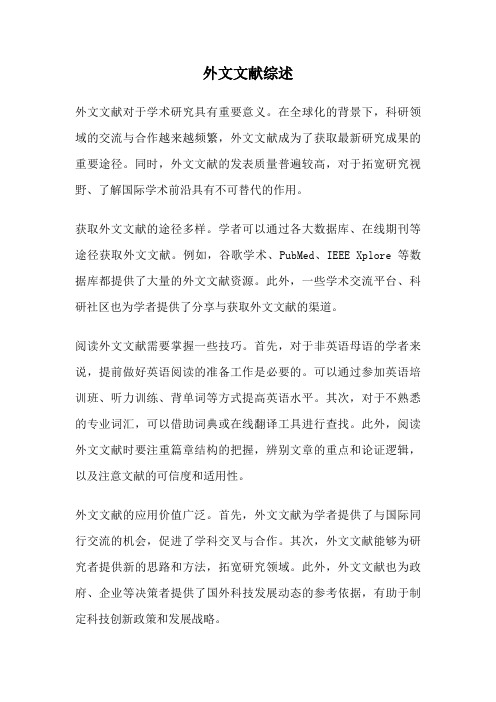
外文文献综述
外文文献对于学术研究具有重要意义。
在全球化的背景下,科研领域的交流与合作越来越频繁,外文文献成为了获取最新研究成果的重要途径。
同时,外文文献的发表质量普遍较高,对于拓宽研究视野、了解国际学术前沿具有不可替代的作用。
获取外文文献的途径多样。
学者可以通过各大数据库、在线期刊等途径获取外文文献。
例如,谷歌学术、PubMed、IEEE Xplore等数据库都提供了大量的外文文献资源。
此外,一些学术交流平台、科研社区也为学者提供了分享与获取外文文献的渠道。
阅读外文文献需要掌握一些技巧。
首先,对于非英语母语的学者来说,提前做好英语阅读的准备工作是必要的。
可以通过参加英语培训班、听力训练、背单词等方式提高英语水平。
其次,对于不熟悉的专业词汇,可以借助词典或在线翻译工具进行查找。
此外,阅读外文文献时要注重篇章结构的把握,辨别文章的重点和论证逻辑,以及注意文献的可信度和适用性。
外文文献的应用价值广泛。
首先,外文文献为学者提供了与国际同行交流的机会,促进了学科交叉与合作。
其次,外文文献能够为研究者提供新的思路和方法,拓宽研究领域。
此外,外文文献也为政府、企业等决策者提供了国外科技发展动态的参考依据,有助于制定科技创新政策和发展战略。
外文文献对于学术研究具有重要意义,了解外文文献的获取途径和阅读技巧对于扩大研究视野、提高学术水平至关重要。
外文文献的应用价值也不容忽视,对于促进学科交流与合作、拓宽研究领域、指导科技发展具有积极作用。
因此,学者们应该重视外文文献的阅读和应用,并不断提升自己的外文文献获取与阅读能力。
大数据时代 文献综述
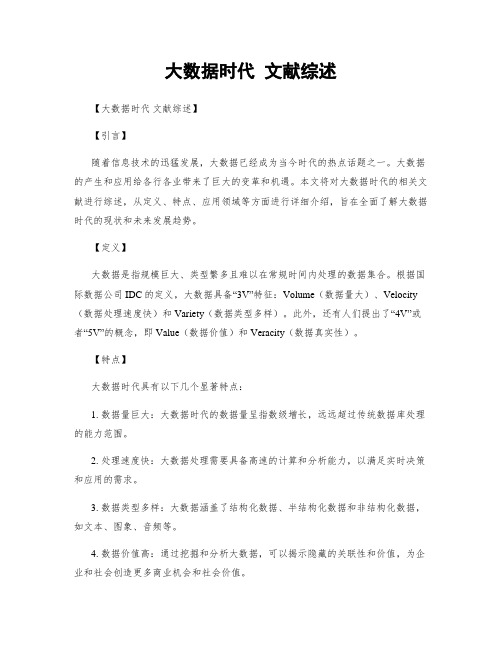
大数据时代文献综述【大数据时代文献综述】【引言】随着信息技术的迅猛发展,大数据已经成为当今时代的热点话题之一。
大数据的产生和应用给各行各业带来了巨大的变革和机遇。
本文将对大数据时代的相关文献进行综述,从定义、特点、应用领域等方面进行详细介绍,旨在全面了解大数据时代的现状和未来发展趋势。
【定义】大数据是指规模巨大、类型繁多且难以在常规时间内处理的数据集合。
根据国际数据公司IDC的定义,大数据具备“3V”特征:Volume(数据量大)、Velocity (数据处理速度快)和Variety(数据类型多样)。
此外,还有人们提出了“4V”或者“5V”的概念,即Value(数据价值)和Veracity(数据真实性)。
【特点】大数据时代具有以下几个显著特点:1. 数据量巨大:大数据时代的数据量呈指数级增长,远远超过传统数据库处理的能力范围。
2. 处理速度快:大数据处理需要具备高速的计算和分析能力,以满足实时决策和应用的需求。
3. 数据类型多样:大数据涵盖了结构化数据、半结构化数据和非结构化数据,如文本、图象、音频等。
4. 数据价值高:通过挖掘和分析大数据,可以揭示隐藏的关联性和价值,为企业和社会创造更多商业机会和社会价值。
5. 数据真实性要求高:大数据的真实性对于决策和应用至关重要,因此数据质量和数据安全成为大数据时代的重要问题。
【应用领域】大数据时代的应用领域广泛,以下是几个典型的应用领域:1. 商业智能和市场营销:通过大数据分析,企业可以深入了解消费者的需求和行为,提供个性化的产品和服务,从而提高市场竞争力。
2. 金融风控和欺诈检测:大数据分析可以匡助金融机构及时发现风险和欺诈行为,提高风险管理和客户信任度。
3. 医疗健康:通过大数据分析,可以实现个性化的医疗诊断和治疗方案,提高医疗效果和患者满意度。
4. 城市管理和智慧城市:大数据分析可以匡助城市管理者更好地了解城市运行情况,提供智慧交通、智慧能源等解决方案,提升城市管理效率和居民生活质量。
大数据文献综述英文版

The development and tendency of Big DataAbstract: "Big Data" is the most popular IT word after the "Internet of things" and "Cloud computing". From the source, development, status quo and tendency of big data, we can understand every aspect of it. Big data is one of the most important technologies around the world and every country has their own way to develop the technology.Key words: big data; IT; technology1 The source of big dataDespite the famous futurist Toffler propose the conception of “Big Data” in 1980, for a long time, because the primary stage is still in the development of IT industry and uses of information sources, “Big Data” is not get enough attention by the people in that age[1].2 The development of big dataUntil the financial crisis in 2008 force the IBM ( multi-national corporation of IT industry) proposing conception of “Smart City”and vigorously promote Internet of Things and Cloud computing so that information data has been in a massive growth meanwhile the need for the technology is very urgent. Under this condition, some American data processing companies have focused on developing large-scale concurrent processing system, then the “Big Data”technology become available sooner and Hadoop mass data concurrent processing system has received wide attention. Since 2010, IT giants have proposed their products in big data area. Big companies such as EMC、HP、IBM、Microsoft all purchase other manufacturer relating to big data in order to achieve technical integration[1]. Based on this, we can learn how important the big data strategy is. Development of big data thanks to some big IT companies such as Google、Amazon、China mobile、Alibaba and so on, because they need a optimization way to store and analysis data. Besides, there are also demands of health systems、geographic space remote sensing and digital media[2].3 The status quo of big dataNowadays America is in the lead of big data technology and market application. USA federal government announced a “Big Data’s research and development” plan in March,2012, which involved six federal government department the National Science Foundation, Health Research Institute, Department of Energy, Department of Defense, Advanced Research Projects Agency and Geological Survey in order to improve the ability to extract information and viewpoint of big data[1]. Thus, it can speed science and engineering discovery up, and it is a major move to push some research institutions making innovations.The federal government put big data development into a strategy place, which hasa big impact on every country. At present, many big European institutions is still at the primary stage to use big data and seriously lack technology about big data. Most improvements and technology of big data are come from America. Therefore, there are kind of challenges of Europe to keep in step with the development of big data. But, in the financial service industry especially investment banking in London is one of the earliest industries in Europe. The experiment and technology of big data is as good as the giant institution of America. And, the investment of big data has been maintained promising efforts. January 2013, British government announced 1.89 million pound will be invested in big data and calculation of energy saving technology in earth observation and health care[3].Japanese government timely takes the challenge of big data strategy. July 2013, Japan’s communications ministry proposed a synthesize strategy called “Energy ICT of Japan” which focused on big data application. June 2013, the abe cabinet formally announced the new IT strategy----“The announcement of creating the most advanced IT country”. This announcement comprehensively expounded that Japanese new IT national strategy is with the core of developing opening public data and big data in 2013 to 2020[4].Big data has also drawn attention of China government.《Guiding opinions of the State Council on promoting the healthy and orderly development of the Internet of things》promote to quicken the core technology including sensor network、intelligent terminal、big data processing、intelligent analysis and service integration. December 2012, the national development and reform commission add data analysis software into special guide, in the beginning of 2013 ministry of science and technology announced that big data research is one of the most important content of “973 program”[1]. This program requests that we need to research the expression, measure and semantic understanding of multi-source heterogeneous data, research modeling theory and computational model, promote hardware and software system architecture by energy optimal distributed storage and processing, analysis the relationship of complexity、calculability and treatment efficiency[1]. Above all, we can provide theory evidence for setting up scientific system of big data.4 The tendency of big data4.1 See the future by big dataIn the beginning of 2008, Alibaba found that the whole number of sellers were on a slippery slope by mining analyzing user-behavior data meanwhile the procurement to Europe and America was also glide. They accurately predicting the trend of world economic trade unfold half year earlier so they avoid the financial crisis[2]. Document [3] cite an example which turned out can predict a cholera one year earlier by mining and analysis the data of storm, drought and other natural disaster[3].4.2 Great changes and business opportunitiesWith the approval of big data values, giants of every industry all spend more money in big data industry. Then great changes and business opportunity comes[4].In hardware industry, big data are facing the challenges of manage, storage and real-time analysis. Big data will have an important impact of chip and storage industry,besides, some new industry will be created because of big data[4].In software and service area, the urgent demand of fast data processing will bring great boom to data mining and business intelligence industry.The hidden value of big data can create a lot of new companies, new products, new technology and new projects[2].4.3 Development direction of big dataThe storage technology of big data is relational database at primary. But due to the canonical design, friendly query language, efficient ability dealing with online affair, Big data dominate the market a long term. However, its strict design pattern, it ensures consistency to give up function, its poor expansibility these problems are exposed in big data analysis. Then, NoSQL data storage model and Bigtable propsed by Google start to be in fashion[5].Big data analysis technology which uses MapReduce technological frame proposed by Google is used to deal with large scale concurrent batch transaction. Using file system to store unstructured data is not lost function but also win the expansilility. Later, there are big data analysis platform like HA VEn proposed by HP and Fusion Insight proposed by Huawei . Beyond doubt, this situation will be continued, new technology and measures will come out such as next generation data warehouse, Hadoop distribute and so on[6].ConclusionThis paper we analysis the development and tendency of big data. Based on this, we know that the big data is still at a primary stage, there are too many problems need to deal with. But the commercial value and market value of big data are the direction of development to information age.忽略此处..[1] Li Chunwei, Development report of China’s E-Commerce enterprises, Beijing , 2013,pp.268-270[2] Li Fen, Zhu Zhixiang, Liu Shenghui, The development status and the problems of large data, Journal of Xi’an University of Posts and Telecommunications, 18 volume, pp. 102-103,sep.2013 [3] Kira Radinsky, Eric Horivtz, Mining the Web to Predict Future Events[C]//Proceedings of the 6th ACM International Conference on Web Search and Data Mining, WSDM 2013: New York: Association for Computing Machinery,2013,pp.255-264[4] Chapman A, Allen M D, Blaustein B. It’s About the Data: Provenance as a Toll for Assessing Data Fitness[C]//Proc of the 4th USENIX Workshop on the Theory and Practice of Provenance, Berkely, CA: USENIX Association, 2012:8[5] Li Ruiqin, Zheng Janguo, Big data Research: Status quo, Problems and Tendency[J],Network Application,Shanghai,1994,pp.107-108[6] Meng Xiaofeng, Wang Huiju, Du Xiaoyong, Big Daya Analysis: Competition and Survival of RDBMS and ManReduce[J], Journal of software, 2012,23(1): 32-45。
大数据时代 文献综述(二)2024
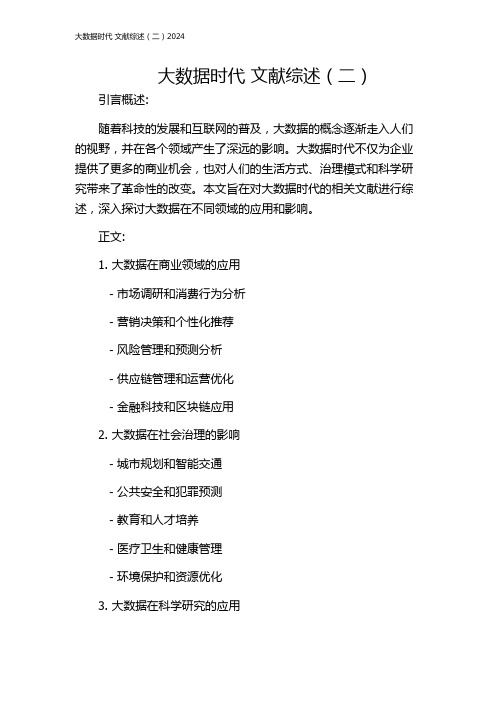
大数据时代文献综述(二)引言概述:随着科技的发展和互联网的普及,大数据的概念逐渐走入人们的视野,并在各个领域产生了深远的影响。
大数据时代不仅为企业提供了更多的商业机会,也对人们的生活方式、治理模式和科学研究带来了革命性的改变。
本文旨在对大数据时代的相关文献进行综述,深入探讨大数据在不同领域的应用和影响。
正文:1. 大数据在商业领域的应用- 市场调研和消费行为分析- 营销决策和个性化推荐- 风险管理和预测分析- 供应链管理和运营优化- 金融科技和区块链应用2. 大数据在社会治理的影响- 城市规划和智能交通- 公共安全和犯罪预测- 教育和人才培养- 医疗卫生和健康管理- 环境保护和资源优化3. 大数据在科学研究的应用- 生物医学研究和药物开发- 天文学和宇宙探索- 地球科学和气候变化研究- 材料科学和新材料开发- 社会科学和行为分析4. 大数据时代的挑战与问题- 数据隐私和安全保护- 数据质量和准确性- 数据治理和标准化- 技术能力和人才短缺- 法律法规和伦理问题5. 大数据时代的机遇与未来发展- 人工智能与大数据融合- 数据共享和合作机制- 数据开放和开放创新- 数据驱动的决策和智能化服务- 数据智能化的社会发展和治理总结:大数据时代带来了商业、社会和科学各个领域的巨大机遇和挑战。
在商业领域,大数据应用的深入推进将进一步提高企业的竞争力和效率;在社会治理方面,大数据将为城市发展和公共服务提供更精准的决策支持;在科学研究领域,大数据将推动科学家们的发现和创新。
然而,我们也需要面对数据隐私保护、数据治理以及技术人才短缺等问题。
未来,随着人工智能与大数据的深度融合,数据驱动的决策和智能化服务将成为大数据时代的新趋势,为社会发展和治理带来更多想象空间。
外文翻译与文献综述模板格式以及要求说明
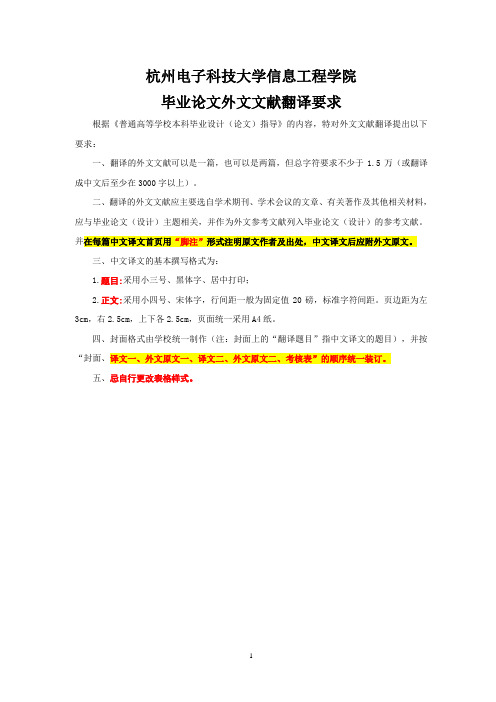
杭州电子科技大学信息工程学院毕业论文外文文献翻译要求根据《普通高等学校本科毕业设计(论文)指导》的内容,特对外文文献翻译提出以下要求:一、翻译的外文文献可以是一篇,也可以是两篇,但总字符要求不少于1.5万(或翻译成中文后至少在3000字以上)。
二、翻译的外文文献应主要选自学术期刊、学术会议的文章、有关著作及其他相关材料,应与毕业论文(设计)主题相关,并作为外文参考文献列入毕业论文(设计)的参考文献。
并在每篇中文译文首页用“脚注”形式注明原文作者及出处,中文译文后应附外文原文。
三、中文译文的基本撰写格式为:1.题目:采用小三号、黑体字、居中打印;2.正文:采用小四号、宋体字,行间距一般为固定值20磅,标准字符间距。
页边距为左3cm,右2.5cm,上下各2.5cm,页面统一采用A4纸。
四、封面格式由学校统一制作(注:封面上的“翻译题目”指中文译文的题目),并按“封面、译文一、外文原文一、译文二、外文原文二、考核表”的顺序统一装订。
五、忌自行更改表格样式。
毕业论文外文文献翻译毕业设计(论文)题目Xxx翻译(1)题目指翻译后的中文译文的题目翻译(2)题目指翻译后的中文译文的题目系会计系以本模板为准)专业XXXXXX(以本模板为准)姓名XXXXXX(以本模板为准)班级XXXXXX(以本模板为准)学号XXXXXX(以本模板为准)指导教师XXXXXX(以本模板为准)正文指导教师对外文翻译的评语:指导教师(签名)年月日建议成绩(百分制)评阅小组或评阅人对外文翻译的评语:评阅小组负责人或评阅人(签名)年月日建议成绩(百分制)杭州电子科技大学信息工程学院本科毕业论文文献综述的写作要求为了促使学生熟悉更多的专业文献资料,进一步强化学生搜集文献资料的能力,提高对文献资料的归纳、分析、综合运用能力及独立开展科研活动的能力,现对本科学生的毕业设计(论文)提出文献综述的写作要求,具体要求如下:一、文献综述的概念文献综述是针对某一研究领域或专题搜集大量文献资料的基础上,就国内外在该领域或专题的主要研究成果、最新进展、研究动态、前沿问题等进行综合分析而写成的、能比较全面地反映相关领域或专题历史背景、前人工作、争论焦点、研究现状和发展前景等内容的综述性文章。
- 1、下载文档前请自行甄别文档内容的完整性,平台不提供额外的编辑、内容补充、找答案等附加服务。
- 2、"仅部分预览"的文档,不可在线预览部分如存在完整性等问题,可反馈申请退款(可完整预览的文档不适用该条件!)。
- 3、如文档侵犯您的权益,请联系客服反馈,我们会尽快为您处理(人工客服工作时间:9:00-18:30)。
大数据外文翻译参考文献综述(文档含中英文对照即英文原文和中文翻译)原文:Data Mining and Data PublishingData mining is the extraction of vast interesting patterns or knowledge from huge amount of data. The initial idea of privacy-preserving data mining PPDM was to extend traditional data mining techniques to work with the data modified to mask sensitive information. The key issues were how to modify the data and how to recover the data mining result from the modified data. Privacy-preserving data mining considers the problem of running data mining algorithms on confidential data that is not supposed to be revealed even to the partyrunning the algorithm. In contrast, privacy-preserving data publishing (PPDP) may not necessarily be tied to a specific data mining task, and the data mining task may be unknown at the time of data publishing. PPDP studies how to transform raw data into a version that is immunized against privacy attacks but that still supports effective data mining tasks. Privacy-preserving for both data mining (PPDM) and data publishing (PPDP) has become increasingly popular because it allows sharing of privacy sensitive data for analysis purposes. One well studied approach is the k-anonymity model [1] which in turn led to other models such as confidence bounding, l-diversity, t-closeness, (α,k)-anonymity, etc. In particular, all known mechanisms try to minimize information loss and such an attempt provides a loophole for attacks. The aim of this paper is to present a survey for most of the common attacks techniques for anonymization-based PPDM & PPDP and explain their effects on Data Privacy.Although data mining is potentially useful, many data holders are reluctant to provide their data for data mining for the fear of violating individual privacy. In recent years, study has been made to ensure that the sensitive information of individuals cannot be identified easily.Anonymity Models, k-anonymization techniques have been the focus of intense research in the last few years. In order to ensure anonymization of data while at the same time minimizing the informationloss resulting from data modifications, everal extending models are proposed, which are discussed as follows.1.k-Anonymityk-anonymity is one of the most classic models, which technique that prevents joining attacks by generalizing and/or suppressing portions of the released microdata so that no individual can be uniquely distinguished from a group of size k. In the k-anonymous tables, a data set is k-anonymous (k ≥ 1) if each record in the data set is in- distinguishable from at least (k . 1) other records within the same data set. The larger the value of k, the better the privacy is protected. k-anonymity can ensure that individuals cannot be uniquely identified by linking attacks.2. Extending ModelsSince k-anonymity does not provide sufficient protection against attribute disclosure. The notion of l-diversity attempts to solve this problem by requiring that each equivalence class has at least l well-represented value for each sensitive attribute. The technology of l-diversity has some advantages than k-anonymity. Because k-anonymity dataset permits strong attacks due to lack of diversity in the sensitive attributes. In this model, an equivalence class is said to have l-diversity if there are at least l well-represented value for the sensitive attribute. Because there are semantic relationships among the attribute values, and different values have very different levels of sensitivity. Afteranonymization, in any equivalence class, the frequency (in fraction) of a sensitive value is no more than α.3. Related Research AreasSeveral polls show that the public has an in- creased sense of privacy loss. Since data mining is often a key component of information systems, homeland security systems, and monitoring and surveillance systems, it gives a wrong impression that data mining is a technique for privacy intrusion. This lack of trust has become an obstacle to the benefit of the technology. For example, the potentially beneficial data mining re- search project, Terrorism Information Awareness (TIA), was terminated by the US Congress due to its controversial procedures of collecting, sharing, and analyzing the trails left by individuals. Motivated by the privacy concerns on data mining tools, a research area called privacy-reserving data mining (PPDM) emerged in 2000. The initial idea of PPDM was to extend traditional data mining techniques to work with the data modified to mask sensitive information. The key issues were how to modify the data and how to recover the data mining result from the modified data. The solutions were often tightly coupled with the data mining algorithms under consideration. In contrast, privacy-preserving data publishing (PPDP) may not necessarily tie to a specific data mining task, and the data mining task is sometimes unknown at the time of data publishing. Furthermore, some PPDP solutions emphasize preserving the datatruthfulness at the record level, but PPDM solutions often do not preserve such property. PPDP Differs from PPDM in Several Major Ways as Follows :1) PPDP focuses on techniques for publishing data, not techniques for data mining. In fact, it is expected that standard data mining techniques are applied on the published data. In contrast, the data holder in PPDM needs to randomize the data in such a way that data mining results can be recovered from the randomized data. To do so, the data holder must understand the data mining tasks and algorithms involved. This level of involvement is not expected of the data holder in PPDP who usually is not an expert in data mining.2) Both randomization and encryption do not preserve the truthfulness of values at the record level; therefore, the released data are basically meaningless to the recipients. In such a case, the data holder in PPDM may consider releasing the data mining results rather than the scrambled data.3) PPDP primarily “anonymizes” the data by hiding the identity of record owners, whereas PPDM seeks to directly hide the sensitive data. Excellent surveys and books in randomization and cryptographic techniques for PPDM can be found in the existing literature. A family of research work called privacy-preserving distributed data mining (PPDDM) aims at performing some data mining task on a set of private databasesowned by different parties. It follows the principle of Secure Multiparty Computation (SMC), and prohibits any data sharing other than the final data mining result. Clifton et al. present a suite of SMC operations, like secure sum, secure set union, secure size of set intersection, and scalar product, that are useful for many data mining tasks. In contrast, PPDP does not perform the actual data mining task, but concerns with how to publish the data so that the anonymous data are useful for data mining. We can say that PPDP protects privacy at the data level while PPDDM protects privacy at the process level. They address different privacy models and data mining scenarios. In the field of statistical disclosure control (SDC), the research works focus on privacy-preserving publishing methods for statistical tables. SDC focuses on three types of disclosures, namely identity disclosure, attribute disclosure, and inferential disclosure. Identity disclosure occurs if an adversary can identify a respondent from the published data. Revealing that an individual is a respondent of a data collection may or may not violate confidentiality requirements. Attribute disclosure occurs when confidential information about a respondent is revealed and can be attributed to the respondent. Attribute disclosure is the primary concern of most statistical agencies in deciding whether to publish tabular data. Inferential disclosure occurs when individual information can be inferred with high confidence from statistical information of the published data.Some other works of SDC focus on the study of the non-interactive query model, in which the data recipients can submit one query to the system. This type of non-interactive query model may not fully address the information needs of data recipients because, in some cases, it is very difficult for a data recipient to accurately construct a query for a data mining task in one shot. Consequently, there are a series of studies on the interactive query model, in which the data recipients, including adversaries, can submit a sequence of queries based on previously received query results. The database server is responsible to keep track of all queries of each user and determine whether or not the currently received query has violated the privacy requirement with respect to all previous queries. One limitation of any interactive privacy-preserving query system is that it can only answer a sublinear number of queries in total; otherwise, an adversary (or a group of corrupted data recipients) will be able to reconstruct all but 1 . o(1) fraction of the original data, which is a very strong violation of privacy. When the maximum number of queries is reached, the query service must be closed to avoid privacy leak. In the case of the non-interactive query model, the adversary can issue only one query and, therefore, the non-interactive query model cannot achieve the same degree of privacy defined by Introduction the interactive model. One may consider that privacy-reserving data publishing is a special case of the non-interactivequery model.This paper presents a survey for most of the common attacks techniques for anonymization-based PPDM & PPDP and explains their effects on Data Privacy. k-anonymity is used for security of respondents identity and decreases linking attack in the case of homogeneity attack a simple k-anonymity model fails and we need a concept which prevent from this attack solution is l-diversity. All tuples are arranged in well represented form and adversary will divert to l places or on l sensitive attributes. l-diversity limits in case of background knowledge attack because no one predicts knowledge level of an adversary. It is observe that using generalization and suppression we also apply these techniques on those attributes which doesn’t need th is extent of privacy and this leads to reduce the precision of publishing table. e-NSTAM (extended Sensitive Tuples Anonymity Method) is applied on sensitive tuples only and reduces information loss, this method also fails in the case of multiple sensitive tuples.Generalization with suppression is also the causes of data lose because suppression emphasize on not releasing values which are not suited for k factor. Future works in this front can include defining a new privacy measure along with l-diversity for multiple sensitive attribute and we will focus to generalize attributes without suppression using other techniques which are used to achieve k-anonymity because suppression leads to reduce the precision ofpublishing table.译文:数据挖掘和数据发布数据挖掘中提取出大量有趣的模式从大量的数据或知识。