Robust Subspace Segmentation by Low-Rank Representation
基于 CT 和磁共振 T2加权图像双模态分类模型的自发性脑出血后脑水肿在 CT 图像上的分割

基于 CT 和磁共振 T2加权图像双模态分类模型的自发性脑出血后脑水肿在 CT 图像上的分割陈明扬;朱时才;贾富仓;李晓东;AhmedElazab;胡庆茂【摘要】Segmentation of cerebral edema from computed tomography (CT) scans for patients with intracr-anial hemorrhage (ICH) is challenging as edema does not show clear boundary on CT. By exploiting the clear boundary on T2-weighted magnetic resonance images, a method was proposed to segment edema on CT images through the model learned from 14 patients with both CT and T2-weighted images using ground truth edema from T2-weighted images to train and classify the features extracted on CT images. By constructing negative samples around the positive samples, employing the feature selection based on common subspace measures, and using support vector machine, the classification model was attained corresponding to the optimum segmentation accuracy. The method has been validated against 36 clinical head CT scans presenting ICH to yield a mean Dice coefficient of 0.859±0.037, which is significantly higher than that of region growing method (0.789±0.036,P<0.000 1), semi-automated level set method (0.712±0.118, P<0.000 1), and threshold based method (0.649±0.147, P<0.000 1). Comparative experiments have been carried out to find that the classifier purely from CT will yield a significantly lower Dice coefficient (0.686±0.136, P<0.000 1). The higher segmentation accuracy may suggest that clear boundaries of edema from T2-weighted images provide implicit constraints on CTimages that could differentiate edema from its neighboring brain tissues more accurately. The proposed method could provide a potential tool to quantify edema, evaluate the severity of pathological changes, and guide therapy of patients with ICH.%自发性脑出血后脑水肿在 CT 图像呈现的模糊边缘是 CT 图像上实现脑水肿自动分割的一个严峻挑战。
图像邻域像素分布分析

图像邻域像素分布分析谭啸,冯久超【摘要】从数字图像的成像原理出发,对邻域像素的分布进行了分析,进而对邻域像素跳变分布提出了2种新的函数模型。
实验采用非线性最小二乘法对从实验图像中得到的分布结果进行函数拟合,并用差值能量函数(DPF)对新提出的函数模型与文献[8,12]的分布函数进行对比分析。
结果表明:新提出的函数模型拥有更高的拟合度,更符合邻域像素值的分布情况。
【期刊名称】吉林大学学报(工学版)【年(卷),期】2011(041)002【总页数】6【关键词】信息处理;分布函数;非线性最小二乘法;邻域;像素;跳变近些年,科研工作者展开了大量的基于邻域性质的研究工作,邻域性质被广泛地应用到图像分割和图像重建等领域[1-5]。
同时,水印和密写工作者们依据密写行为会减弱邻域之间相关性的性质,直接利用邻域像素值跳变关系或者考虑Gray-level co-occurrence matrix(GLCM)和Histogram characteristics function(HCF)的变化达到对密写行为分析的目的[6-11]。
可见,邻域像素之间的关系在图像分析工作中具有重要作用。
有研究者认为[8,12]邻域像素值跳变分布函数服从高斯分布或拉普拉斯分布,并在不同的实验中得到较好的拟合效果。
但是,并没有文献对这种分布的正确性加以分析。
本文对数字邻域像素值跳变分布函数进行了分析。
首先,从数字设备的成像原理出发,分析了一定区域内的像素值分布,并依据大数定理得到这些像素值应服从高斯分布。
然后,分析了不同区域之间的像素分布差异,经过数学分析和推导得到最终的分布函数表达式,并证明了邻域像素值跳变更符合拉普拉斯分布,同时给出了更具一般性的分布函数。
1 数字图像成像原理数字成像设备的成像过程是:通过感光部件(CCD或者CMOS)对自然图像进行采样得到采样信号,再按照一定算法(不同的数字图像设备采用不同的算法)对采样信号进行处理得到相应的数字图像[13]。
信号与数据处理中的低秩模型——理论、算法与应用

min rank( A), s.t.
A
( D) ( A)
2 F
,
(2)
以处理测量数据有噪声的情况。 如果考虑数据有强噪声时如何恢复低秩结构的问题,看似这个问题可以用传统的 PCA 解决,但 实际上传统 PCA 只在噪声是高斯噪声时可以准确恢复潜在的低秩结构。对于非高斯噪声,如果噪声 很强,即使是极少数的噪声,也会使传统的主元分析失败。由于主元分析在应用上的极端重要性, 大量学者付出了很多努力在提高主元分析的鲁棒性上,提出了许多号称“鲁棒”的主元分析方法, 但是没有一个方法被理论上严格证明是能够在一定条件下一定能够精确恢复出低秩结构的。 2009 年, Chandrasekaran 等人[CSPW2009]和 Wright 等人[WGRM2009]同时提出了鲁棒主元分析 (Robust PCA, RPCA) 。他们考虑的是数据中有稀疏大噪声时如何恢复数据的低秩结构:
b) 多子空间模型
RPCA 只能从数据中提取一个子空间,它对数据在此子空间中的精细结构无法刻画。精细结构 的最简单情形是多子空间模型,即数据分布在若干子空间附近,我们需要找到这些子空间。这个问 题马毅等人称为 Generalized PCA (GPCA)问题[VMS2015],之前已有很多算法,如代数法、RANSAC 等,但都没有理论保障。稀疏表示的出现为这个问题提供了新的思路。E. Elhamifar 和 R. Vidal 2009 年利用样本间相互表达,在表达系数矩阵稀疏的目标下提出了 Sparse Subspace Clustering (SSC)模型 [EV2009]((6)中 rank( Z ) 换成 Z
* 本文得到国家自然科学基金(61272341, 61231002)资助。
迭代吉洪诺夫正则化的FCM聚类算法

迭代吉洪诺夫正则化的FCM聚类算法蒋莉芳;苏一丹;覃华【摘要】模糊C均值聚类算法(fuzzy C-means,FCM)存在不适定性问题,数据噪声会引起聚类失真.为此,提出一种迭代Tikhonov正则化模糊C均值聚类算法,对FCM的目标函数引入正则化罚项,推导最优正则化参数的迭代公式,用L曲线法在迭代过程中实现正则化参数的寻优,提高FCM的抗噪声能力,克服不适定问题.在UCI 数据集和人工数据集上的实验结果表明,所提算法的聚类精度较传统FCM高,迭代次数少10倍以上,抗噪声能力更强,用迭代Tikhonov正则化克服传统FCM的不适定问题是可行的.%FCM algorithm has the ill posed problem.Regularization method can improve the distortion of the model solution caused by the fluctuation of the data.And it can improve the precision and robustness of FCM through solving the error estimate of solution caused by ill posed problem.Iterative Tikhonov regularization function was introduced into the proposed problem (ITR-FCM),and L-curve method was used to select the optimal regularization parameter iteratively,and the convergence rate of the algorithm was further improved using the dynamic Tikhonov method.Five UCI datasets and five artificial datasets were chosen for the test.Results of tests show that iterative Tikhonov is an effective solution to the ill posed problem,and ITR-FCM has better convergence speed,accuracy and robustness.【期刊名称】《计算机工程与设计》【年(卷),期】2017(038)009【总页数】5页(P2391-2395)【关键词】模糊C均值聚类;不适定问题;Tikhonov正则化;正则化参数;L曲线【作者】蒋莉芳;苏一丹;覃华【作者单位】广西大学计算机与电子信息学院,广西南宁 530004;广西大学计算机与电子信息学院,广西南宁 530004;广西大学计算机与电子信息学院,广西南宁530004【正文语种】中文【中图分类】TP389.1模糊C均值算法已广泛地应用于图像分割、模式识别、故障诊断等领域[1-6]。
稀疏判别分析
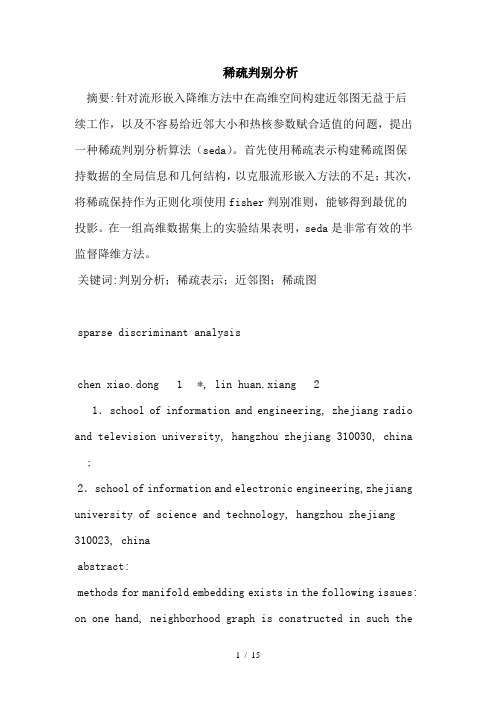
稀疏判别分析摘要:针对流形嵌入降维方法中在高维空间构建近邻图无益于后续工作,以及不容易给近邻大小和热核参数赋合适值的问题,提出一种稀疏判别分析算法(seda)。
首先使用稀疏表示构建稀疏图保持数据的全局信息和几何结构,以克服流形嵌入方法的不足;其次,将稀疏保持作为正则化项使用fisher判别准则,能够得到最优的投影。
在一组高维数据集上的实验结果表明,seda是非常有效的半监督降维方法。
关键词:判别分析;稀疏表示;近邻图;稀疏图sparse discriminant analysischen xiao.dong1*, lin huan.xiang 21.school of information and engineering, zhejiang radio and television university, hangzhou zhejiang 310030, china ;2.school of information and electronic engineering,zhejiang university of science and technology, hangzhou zhejiang 310023, chinaabstract:methods for manifold embedding exists in the following issues: on one hand, neighborhood graph is constructed in such thehigh-dimensionality of original space that it tends to work poorly; on the other hand, appropriate values for the neighborhood size and heat kernel parameter involved in graph construction is generally difficult to be assigned. to address these problems, a novel semi-supervised dimensionality reduction algorithm called sparse discriminant analysis (seda) is proposed. firstly, seda sets up a sparse graph to preserve the global information and geometric structure of the data based on sparse representation. secondly, it applies both sparse graph and fisher criterion to seek the optimal projection. experiments on a broad range of data sets show that seda is superior to many popular dimensionality reduction methods.methods for manifold embedding have the following issues: on one hand, neighborhood graph is constructed in suchhigh.dimensionality of original space that it tends to work poorly; on the other hand, appropriate values for the neighborhood size and heat kernel parameter involved in graph construction are generally difficult to be assigned. to address these problems, a new semi.supervised dimensionality reduction algorithm called sparse discriminant analysis (seda) was proposed. firstly, seda set up a sparse graph topreserve the global information and geometric structure of the data based on sparse representation. secondly, it applied both sparse graph and fisher criterion to seek the optimal projection. the experimental results on a broad range of data sets show that seda is superior to many popular dimensionality reduction methods.key words:discriminant analysis; sparse representation; neighborhood graph; sparse graph0 引言在信息检索、文本分类、图像处理和生物计算等应用中,所面临的数据都是高维的。
ICML_NIPS_ICCV_CVPR(14~18)

ICML2014ICML20151. An embarrassingly simple approach to zero-shot learning2. Learning Transferable Features with Deep Adaptation Networks3. A Theoretical Analysis of Metric Hypothesis Transfer Learning4. Gradient-based hyperparameter optimization through reversible learningICML20161. One-Shot Generalization in Deep Generative Models2. Meta-Learning with Memory-Augmented Neural Networks3. Meta-gradient boosted decision tree model for weight and target learning4. Asymmetric Multi-task Learning based on Task Relatedness and ConfidenceICML20171. DARLA: Improving Zero-Shot Transfer in Reinforcement Learning2. Model-Agnostic Meta-Learning for Fast Adaptation of Deep Networks3. Meta Networks4. Learning to learn without gradient descent by gradient descentICML20181. MSplit LBI: Realizing Feature Selection and Dense Estimation Simultaneously in Few-shotand Zero-shot Learning2. Understanding and Simplifying One-Shot Architecture Search3. One-Shot Segmentation in Clutter4. Meta-Learning by Adjusting Priors Based on Extended PAC-Bayes Theory5. Bilevel Programming for Hyperparameter Optimization and Meta-Learning6. Gradient-Based Meta-Learning with Learned Layerwise Metric and Subspace7. Been There, Done That: Meta-Learning with Episodic Recall8. Learning to Explore via Meta-Policy Gradient9. Transfer Learning via Learning to Transfer10. Rapid adaptation with conditionally shifted neuronsNIPS20141. Zero-shot recognition with unreliable attributesNIPS2015NIPS20161. Learning feed-forward one-shot learners2. Matching Networks for One Shot Learning3. Learning from Small Sample Sets by Combining Unsupervised Meta-Training with CNNs NIPS20171. One-Shot Imitation Learning2. Few-Shot Learning Through an Information Retrieval Lens3. Prototypical Networks for Few-shot Learning4. Few-Shot Adversarial Domain Adaptation5. A Meta-Learning Perspective on Cold-Start Recommendations for Items6. Neural Program Meta-InductionNIPS20181. Bayesian Model-Agnostic Meta-Learning2. The Importance of Sampling inMeta-Reinforcement Learning3. MetaAnchor: Learning to Detect Objects with Customized Anchors4. MetaGAN: An Adversarial Approach to Few-Shot Learning5. Regret bounds for meta Bayesian optimization with an unknown Gaussian process prior6. Meta-Gradient Reinforcement Learning7. Meta-Reinforcement Learning of Structured Exploration Strategies8. Meta-Learning MCMC Proposals9. Probabilistic Model-Agnostic Meta-Learning10. MetaReg: Towards Domain Generalization using Meta-Regularization11. Zero-Shot Transfer with Deictic Object-Oriented Representation in Reinforcement Learning12. Uncertainty-Aware Few-Shot Learning with Probabilistic Model-Agnostic Meta-Learning13. Multitask Reinforcement Learning for Zero-shot Generalization with Subtask Dependencies14. Stacked Semantics-Guided Attention Model for Fine-Grained Zero-Shot Learning15. Delta-encoder: an effective sample synthesis method for few-shot object recognition16. One-Shot Unsupervised Cross Domain Translation17. Generalized Zero-Shot Learning with Deep Calibration Network18. Domain-Invariant Projection Learning for Zero-Shot Recognition19. Low-shot Learning via Covariance-Preserving Adversarial Augmentation Network20. Improved few-shot learning with task conditioning and metric scaling21. Adapted Deep Embeddings: A Synthesis of Methods for k-Shot Inductive Transfer Learning22. Learning to Play with Intrinsically-Motivated Self-Aware Agents23. Learning to Teach with Dynamic Loss Functiaons24. Memory Replay GANs: learning to generate images from new categories without forgettingICCV20151. One Shot Learning via Compositions of Meaningful Patches2. Unsupervised Domain Adaptation for Zero-Shot Learning3. Active Transfer Learning With Zero-Shot Priors: Reusing Past Datasets for Future Tasks4. Zero-Shot Learning via Semantic Similarity Embedding5. Semi-Supervised Zero-Shot Classification With Label Representation Learning6. Predicting Deep Zero-Shot Convolutional Neural Networks Using Textual Descriptions7. Learning to Transfer: Transferring Latent Task Structures and Its Application to Person-Specific Facial Action Unit DetectionICCV20171. Supplementary Meta-Learning: Towards a Dynamic Model for Deep Neural Networks2. Attributes2Classname: A Discriminative Model for Attribute-Based Unsupervised Zero-ShotLearning3. Low-Shot Visual Recognition by Shrinking and Hallucinating Features4. Predicting Visual Exemplars of Unseen Classes for Zero-Shot Learning5. Learning Discriminative Latent Attributes for Zero-Shot Classification6. Spatial-Aware Object Embeddings for Zero-Shot Localization and Classification of ActionsCVPR20141. COSTA: Co-Occurrence Statistics for Zero-Shot Classification2. Zero-shot Event Detection using Multi-modal Fusion of Weakly Supervised Concepts3. Learning to Learn, from Transfer Learning to Domain Adaptation: A Unifying Perspective CVPR20151. Zero-Shot Object Recognition by Semantic Manifold DistanceCVPR20162. Multi-Cue Zero-Shot Learning With Strong Supervision3. Latent Embeddings for Zero-Shot Classification4. One-Shot Learning of Scene Locations via Feature Trajectory Transfer5. Less Is More: Zero-Shot Learning From Online Textual Documents With Noise Suppression6. Synthesized Classifiers for Zero-Shot Learning7. Recovering the Missing Link: Predicting Class-Attribute Associations for UnsupervisedZero-Shot Learning8. Fast Zero-Shot Image Tagging9. Zero-Shot Learning via Joint Latent Similarity Embedding10. Learning to Read Chest X-Rays: Recurrent Neural Cascade Model for Automated ImageAnnotation11. Learning to Co-Generate Object Proposals With a Deep Structured Network12. Learning to Select Pre-Trained Deep Representations With Bayesian Evidence Framework13. DeepStereo: Learning to Predict New Views From the World’s ImageryCVPR20171. One-Shot Video Object Segmentation2. FastMask: Segment Multi-Scale Object Candidates in One Shot3. Few-Shot Object Recognition From Machine-Labeled Web Images4. From Zero-Shot Learning to Conventional Supervised Classification: Unseen Visual DataSynthesis5. Learning a Deep Embedding Model for Zero-Shot Learning6. Low-Rank Embedded Ensemble Semantic Dictionary for Zero-Shot Learning7. Multi-Attention Network for One Shot Learning8. Zero-Shot Action Recognition With Error-Correcting Output Codes9. One-Shot Metric Learning for Person Re-Identification10. Semantic Autoencoder for Zero-Shot Learning11. Zero-Shot Recognition Using Dual Visual-Semantic Mapping Paths12. Matrix Tri-Factorization With Manifold Regularizations for Zero-Shot Learning13. One-Shot Hyperspectral Imaging Using Faced Reflectors14. Gaze Embeddings for Zero-Shot Image Classification15. Zero-Shot Learning - the Good, the Bad and the Ugly16. Link the Head to the “Beak”: Zero Shot Learning From Noisy Text Description at PartPrecision17. Semantically Consistent Regularization for Zero-Shot Recognition18. Semantically Consistent Regularization for Zero-Shot Recognition19. Zero-Shot Classification With Discriminative Semantic Representation Learning20. Learning to Detect Salient Objects With Image-Level Supervision21. Quad-Networks: Unsupervised Learning to Rank for Interest Point DetectionCVPR20181. A Generative Adversarial Approach for Zero-Shot Learning From Noisy Texts2. Transductive Unbiased Embedding for Zero-Shot Learning3. Zero-Shot Visual Recognition Using Semantics-Preserving Adversarial EmbeddingNetworks4. Learning to Compare: Relation Network for Few-Shot Learning5. One-Shot Action Localization by Learning Sequence Matching Network6. Multi-Label Zero-Shot Learning With Structured Knowledge Graphs7. “Zero-Shot” Super-Resolution Using Deep Internal Learning8. Low-Shot Learning With Large-Scale Diffusion9. CLEAR: Cumulative LEARning for One-Shot One-Class Image Recognition10. Zero-Shot Sketch-Image Hashing11. Structured Set Matching Networks for One-Shot Part Labeling12. Memory Matching Networks for One-Shot Image Recognition13. Generalized Zero-Shot Learning via Synthesized Examples14. Dynamic Few-Shot Visual Learning Without Forgetting15. Exploit the Unknown Gradually: One-Shot Video-Based Person Re-Identification byStepwise Learning16. Feature Generating Networks for Zero-Shot Learning17. Low-Shot Learning With Imprinted Weights18. Zero-Shot Recognition via Semantic Embeddings and Knowledge Graphs19. Webly Supervised Learning Meets Zero-Shot Learning: A Hybrid Approach for Fine-Grained Classification20. Few-Shot Image Recognition by Predicting Parameters From Activations21. Low-Shot Learning From Imaginary Data22. Discriminative Learning of Latent Features for Zero-Shot Recognition23. Multi-Content GAN for Few-Shot Font Style Transfer24. Preserving Semantic Relations for Zero-Shot Learning25. Zero-Shot Kernel Learning26. Neural Style Transfer via Meta Networks27. Learning to Estimate 3D Human Pose and Shape From a Single Color Image28. Learning to Segment Every Thing29. Leveraging Unlabeled Data for Crowd Counting by Learning to Rank。
Subspace Clustering
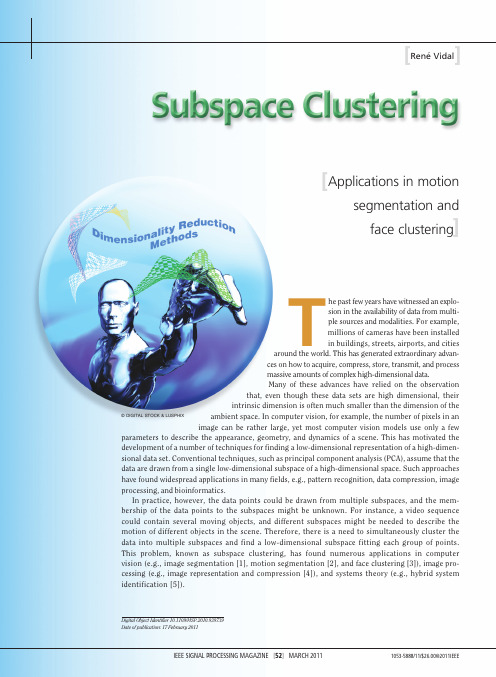
[Ren e Vidal][Applications in motionsegmentation andface clustering]T he past few years have witnessed an explo-sion in the availability of data from multi-ple sources and modalities.For example,millions of cameras have been installedin buildings,streets,airports,and citiesaround the world.This has generated extraordinary advan-ces on how to acquire,compress,store,transmit,and processmassive amounts of complex high-dimensional data.Many of these advances have relied on the observationthat,even though these data sets are high dimensional,theirintrinsic dimension is often much smaller than the dimension of theambient space.In computer vision,for example,the number of pixels in an ©DIGITAL STOCK&LUSPHIXimage can be rather large,yet most computer vision models use only a few parameters to describe the appearance,geometry,and dynamics of a scene.This has motivated the development of a number of techniques for finding a low-dimensional representation of a high-dimen-sional data set.Conventional techniques,such as principal component analysis(PCA),assume that the data are drawn from a single low-dimensional subspace of a high-dimensional space.Such approaches have found widespread applications in many fields,e.g.,pattern recognition,data compression,image processing,and bioinformatics.In practice,however,the data points could be drawn from multiple subspaces,and the mem-bership of the data points to the subspaces might be unknown.For instance,a video sequence could contain several moving objects,and different subspaces might be needed to describe the motion of different objects in the scene.Therefore,there is a need to simultaneously cluster the data into multiple subspaces and find a low-dimensional subspace fitting each group of points. This problem,known as subspace clustering,has found numerous applications in computer vision(e.g.,image segmentation[1],motion segmentation[2],and face clustering[3]),image pro-cessing(e.g.,image representation and compression[4]),and systems theory(e.g.,hybrid system identification[5]).Digital Object Identifier10.1109/MSP.2010.939739Date of publication:17February2011methods from the machine learningand computer vision communities,including algebraic methods[7]–[10],iterative methods [11]–[15],statistical methods [16]–[20],and spectral clustering-based methods [7],[21]–[27].We review these methods,discuss their advantages and disadvantages,and evaluate their performance on the motion segmentation and face-clustering problems.THE SUBSPACE CLUSTERING PROBLEM Consider the problem of modeling a collection of data points with a union of subspaces,as illustrated in Figure 1.Specifically,let f x j 2R D g Nj ¼1be a given set of points drawn from an unknown union of n 1linear or affine subspaces f S i g ni ¼1of unknowndimensions d i ¼dim (S i ),05d i 5D ,i ¼1;...;n .The subspa-ces can be described as S i ¼f x 2R D:x ¼l i þU i y g ,i ¼1,...,n ,(1)where l i 2R Dis an arbitrary point in subspace S i that can bechosen as l i ¼0for linear subspaces,U i 2R D 3d i is a basis forsubspace S i ,and y 2R d iis a low-dimensional representation forpoint x .The goal of subspace clustering is to find the number ofsubspaces n ,their dimensions f d i g ni ¼1,the subspace basesf U ig n i ¼1,the points f l i g ni ¼1,and the segmentation of the pointsaccording to the subspaces.When the number of subspaces is equal to one,this problemreduces to finding a vector l 2R D ,a basis U 2R D 3d,a low-dimensional representation Y ¼½y 1;...;y N 2R d 3N ,and the dimension d .This problem is known as PCA [28].(The problem ofmatrix factorization dates back to the work of Beltrami [29]and Jordan [30].In the context of stochastic signal process-ing,PCA is also known as Karhunen-Loeve transform [31].In the applied statistics literature,PCA is also known as Eck-art-Young decomposition [32].)PCA can be solved in a remark-ably simple way:l ¼(1=N )P Nj ¼1x j is the mean of the data points (U ;Y )can be obtained from the rank-d singular value decomposition (SVD)of the (mean-subtracted)data matrix X ¼½x 1Àl ,x 2Àl ,...,x N Àl 2R D 3N asU ¼UandY ¼R V >,whereX ¼U R V >,(2)and d can be obtained as d ¼rank(X )with noise-free data or using model-selection techniques when the data are noisy [28].When n 41,the subspace clustering problem becomes sig-nificantly more difficult due to a number of challenges.n First,there is a strong coupling between data segmenta-tion and model estimation.Specifically,if the segmentation of the data is known,one could easily fit a single subspaceneither the segmentation of the data nor the subspace parameters are known,and one needs to solve both problems simultaneously.n Second,the distribution of the data inside the subspaces is generally unknown.If the data within each subspace are distributed around a cluster center and the cluster centersfor different subspaces are far apart,the subspace clusteringproblem reduces to the simpler and well-studied centralclustering problem.However,if the distribution of the data points in the subspaces is arbitrary,the subspace clustering problem cannot be solved by central clustering techniques.In addition,the problem becomes more difficult when manypoints lie close to the intersection of two or more subspaces.n Third,the position and orientation of the subspaces rela-tive to each other can be arbitrary.As we will show later,when the subspaces are disjoint or independent,the sub-space clustering problem can be solved more easily.How-ever,when the subspaces are dependent,the subspaceclustering problem becomes much harder.(n linear sub-spaces are disjoint if every two subspaces intersect only at the origin.n linear subspaces are independent if the dimension of their sum is equal to the sum of their dimen-sions.Independent subspaces are disjoint,but the converse is not always true.n affine subspaces are disjoint,inde-pendent,if so are the corresponding linear subspaces in homogeneous coordinates.)n The fourth challenge is that the data can be corrupted by noise,missing entries,and outliers.Although robust estima-tion techniques for handling such nuisances have been devel-oped for the case of a single subspace,the case of multiple subspaces is not well understood.n The fifth challenge is model selection.In classical PCA,the only parameter is subspace dimension,which can befound by searching for the subspace of the smallest dimension[FIG1]A set of sample points in R drawn from a union of three subspaces:two lines and a plane.that fits the data with a given accuracy.In the case of multiple subspaces,one can fit the data with N different subspaces of dimension one,i.e.,one subspace per data point,or with a single subspace of dimension D .Obviously,neither solution is satisfactory.The challenge is to find a model-selection criteria that favors a small number of sub-spaces of small dimensions.In what follows,we present a number of subspace clustering algorithms and show how they try to address these challenges.SUBSPACE CLUSTERING ALGORITHMSALGEBRAIC ALGORITHMSWe first review two algebraic algorithms for clustering noise-free data drawn from multiple linear subspaces,i.e.,l i ¼0.The first algorithm is based on linear algebra,specifically matrix factorization,and is provably correct for independent sub-spaces.The second one is based on polynomial algebra and is provably correct for both dependent and independent subspaces.Although these algorithms are designed for linear subspaces,in the case of noiseless data,they can also be applied to affine subspaces by using homogeneous coordinates,thus interpreting an affine subspace of dimension d in R D as a linear subspace of dimension d þ1in R D þ1.(The homogeneous coordinates of x 2R D are given by ½x >1 >2R D þ1.)Also,while these algorithms operate under the assumption of noise-free data,they provide great insights into the geometry and algebra of the subspace clustering problem.Moreover,they can be extended to handle moderate amounts of noise.MATRIX FACTORIZATION-BASED ALGORITHMSThese algorithms obtain the segmentation of the data from a low-rank factorization of the data matri X .Hence,they are a natural extension of PCA from one to multiple independent linear subspaces.Specifically,let X i 2R D 3N i be the matrix containing the N i points in subspace i .The columns of the data matrix can be sorted according to the n subspaces as ½X 1,X 2,...,X n ¼X C ,where C 2R N 3N is an unknown permutation matrix.Because each matrix X i is of rank d i ,it can be factorized asX i ¼U i Y ii ¼1,...,n ,(3)where U i 2R D 3d i is an orthogonal basis for subspace i and Y i 2R d i 3N i is the low-dimensional representation of the points with respect to U i .Therefore,if the subspaces are independent,then r ¼Drank(X )¼P n i ¼1d i min f D ,N g andX C ¼U 1,U 2,ÁÁÁ,U n ½ Y 1Y 2...Y n2666437775¼DUY ,(4)where U 2R D 3r and Y 2R r 3N .The subspace clustering prob-lem is then equivalent to finding a permutation matrix C ,suchthat X C admits a rank-r factorization into a matrix U and a block diagonal matrix Y .This idea is the basis for the algorithms of Boult and Brown [7],Costeira and Kanade [8],and Gear [9],which compute C from the SVD of X [7],[8]or from the row echelon canonical form of X [9].Specifically,the Costeira and Kanade algorithm proceeds as follows.Let X ¼U R V >be the rank-r SVD of the data matrix,i.e.,U 2R D 3r ,R 2R r 3r ,and V 2R N 3r .Also,letQ ¼VV >2R N 3N :ð5ÞAs shown in [2]and [33],the matrix Q is such thatQ jk ¼0if points j and k are in different subspaces :(6)In the absence of noise,(6)can be used to obtain the segmenta-tion of the data by applying spectral clustering to the eigenvectors of Q [7](see the ‘‘Spectral Clustering-Based Methods’’section)or by sorting and thresholding the entries of Q [8],[34].For instance,[8]obtains the segmentation by maximizing the sum of the squared entries of Q in different groups,while [34]finds the groups by thresholding a subset of the rows of Q .However,as noted in [33]and [35],this thresholding process is very sensitive to noise.Also,the construction of Q requires knowledge of the rank of X ,and using the wrong rank can lead to very poor results [9].Wu et al.[35]use an agglomerative process to reduce the effect of noise.The entries of Q are first thresholded to obtain an initial oversegmentation of the data.A subspace is then fit to each group G i ,and two groups are merged when the distance between their subspaces is below a threshold.A similar approach is followed by Kanatani et al.[33],[36],except that the geometric Akaike informa-tion criterion [37]is used to decide when to merge the two groups.Although these approaches indeed reduce the effect of noise,in practice,they are not effective because the equation Q jk ¼0holds only when the subspaces are independent.In the case of dependent subspaces,one can use the subset of the columns of V that do not span the intersections of the subspaces.Unfortunately,we do not know which columns to choose a priori.Zelnik-Manor and Irani [38]propose to use the top columns of V to define Q .However,this heuristic is not provably correct.Another issue with factorization-based algorithms is that,with a few exceptions,they do not provide a method for computing the number of subspaces,n ,and their dimensions,f d i g n i ¼1.The first exception is when n is known.In this case,d i can be computed from each group after the segmenta-tion has been obtained.The second exception is for independent subspaces of equal dimension d .In this case rank(X )¼nd ,hence we may determine n when d is known or vice versa.GENERALIZED PCAGeneralized PCA (GPCA;see [10]and [39])is an algebraic-geometric method for clustering data lying in (not necessarily independent)linear subspaces.The main idea behind GPCA is that one can fit a union of n subspaces with a set of polynomials of degree n ,whose derivatives at a point give a vector normal to the subspace containing that point.The segmentation of thedata is then obtained by grouping these normal vectors using several possible techniques.The first step of GPCA,which is not strictly needed,is to project the data points onto a subspace of R D of dimension r¼d maxþ1,where d max¼max f d1,...,d n g.(The value of r is determined using model-selection techniques when the subspace dimensions are unknown.)The rationale behind this step is as fol-lows.Since the maximum dimension of each subspace is d max,a projection onto a generic subspace of R D of dimension d maxþ1 preserves the number and dimensions of the subspaces with probabilty one.As a by-product,the subspace clustering problem is reduced to clustering subspa-ces of dimension at most d maxin R d maxþ1.As we shall see,thisstep is very important to reducethe computational complexityof the GPCA algorithm.With anabuse of notation,we will denotethe original and projected sub-spaces as S i,and the original and projected data matrix asX¼½x1,...,x N 2R D3N or R r3N:(7)The second step is to fit a homogeneous polynomial of degree n to the(projected)data.The rationale behind this step is as fol-lows.Imagine,for instance,that the data came from the union of two planes in R3,each one with normal vector b i2R3.The union of the two planes can be represented as a set of points, such that p(x)¼(b>1x)(b>2x)¼0.This equation is nothing but the equation of a conic of the formc1x21þc2x1x2þc3x1x3þc4x22þc5x2x3þc6x23¼0:(8)Imagine now that the data came from the plane b>x¼0or the line b>1x¼b>2x¼0.The union of the plane and the line is the set of points,such that p1(x)¼(b>x)(b>1x)¼0and p2(x)¼(b>x)(b>2x)¼0.More generally,data drawn from the union of n subspaces of R r can be represented with polynomialsof the form p(x)¼(b>1x)ÁÁÁ(b>n x)¼0,where the vector b i2R r is orthogonal to S i.Each polynomial is of degree n in x and can be written as c>m n(x),where c is the vector of coefficients and m n(x) is the vector of all monomials of degree n in x.There areM n(r)¼nþrÀ1nindependent monomials;hence,c2R M n(r).In the case of noiseless data,the vector of coefficients c of each polynomial can be computed fromc>½m n(x1),m n(x2),ÁÁÁ,m n(x N) ¼D c>V n¼0>(9)and the number of polynomials is simply the dimension of the null space of V n.While in general the relationship between the number of subspaces,n,their dimensions,f d i g n i¼1,and the number of polynomials involves the theory of Hilbert functions[40],in the particular case where all the dimensions are equal tod and r¼dþ1,there is a unique polynomial that fits the data. This fact can be exploited to determine both n and d.For exam-ple,given d,n can be computed asn¼min f i:rank(V i)¼M i(r)À1g:(10)In the case of data contaminated with small-to-moderate amounts of noise,the polynomial coefficients(9)can be found using least squares—the vectors c are the left singular vectors ofV n corresponding to the small-est singular values.To handlelarger amounts of noise in theestimation of the polynomialcoefficients,one can resort totechniques from robust statis-tics[20]or rank minimization[41].Model-selection techni-ques can be used to determine the rank of V n and,hence,the number of polynomials,as shown in[42].Model-selection techniques can also be used to determine the number of sub-spaces of equal dimensions in(10),as shown in[10].However, determining n and f d i g n i¼1for subspaces of different dimen-sions from noisy data remains a challenge.The reader is referred to[43]for a model-selection criteria called minimum effective dimension,which measures the complexity of fitting n subspaces of dimensions f d i g n i¼1to a given data set within a certain tolerance,and to[40]and[42]for algebraic relation-ships among n,f d i g n i¼1and the number of polynomials,which can be used for model-selection purposes.The last step is to compute the normal vectors b i from the vec-tor of coefficients c.This can be done by taking the derivatives of the polynomials at a data point.For example,if n¼2,we have r p(x)¼(b>2x)b1þ(b>1x)b2.Thus,if x belongs to the first sub-space,then r p(x)$b1.More generally,in the case of n subspaces,we have p(x)¼(b>1x)ÁÁÁ(b>n x)and r p(x)$b i if x2S i.We can use this result to obtain the set of all normal vectors to S i from the derivatives of all the polynomials at x2S i.This gives us a basis for the orthogonal complement of S i from which we can obtain a basis U i for S i.Therefore,if we knew one point per sub-space,f y i2S i g n i¼1,we could compute the n subspace basesf U ig ni¼1from the gradient of the polynomials at f y i g n i¼1and then obtain the segmentation by assigning each point f x j g N j¼1to its clos-est subspace.A simple method for choosing the points f y i g n i¼1is to select any data point as y1to obtain the basis U1for the first sub-space S1.After removing the points that belong to S1from the data set,we can choose any of the remaining data points as y2to obtain U2,hence S2,and then repeat this process until all the subspaces are found.In the‘‘Spectral Clustering-Based Methods’’section,we will describe an alternative method based on spectral clustering.The first advantage of GPCA is that it is an algebraic algo-rithm;thus,it is computationally cheap when n and d are small.Second,intersections between subspaces are automati-cally allowed;hence,GPCA can deal with both independent andGENERALIZED PCA IS AN ALGEBRAIC-GEOMETRIC METHOD FORCLUSTERING DATA LYING IN (NOT NECESSARILY INDEPENDENT)LINEAR SUBSPACES.dependent subspaces.Third,in the noiseless case,it does notrequire the number of subspaces or their dimensions to be known beforehand.Specifically,the theory of Hilbert functions may be used to determine n and f d i g ,as shown in [40].The first drawback of GPCA is that its complexity increases expo-nentially with n and f d i g .Specifically,each vector c is of dimension O(M n (r )),while there are only O(r P ni ¼1ðr Àd i )Þunknowns inthe n sets of normal vectors.Second,the vector c is computed usingleast squares;thus,the computation of c is sensitive to outliers.Third,the least-squares fit does not take into account nonlinearconstraints among the entries of c (recall that p ðx )must factorize as a product of linear factors).These issues cause the performance of GPCA to deteriorate as n increases.Fourth,the method in [40]to determine n and f d i g ni ¼1does not handle noisy data.Fifth,while GPCA can be applied to affinesubspaces by using homogene-ous coordinates,in our experi-ence,this does not work very well when the data are conta-minated with noise.ITERATIVE METHODSA very simple way of improvingthe performance of algebraic algorithms in the case of noisy datais to use iterative refinement.Intuitively,given an initial seg-mentation,we can fit a subspace to each group using classicalPCA.Then,given a PCA model for each subspace,we can assigneach data point to its closest subspace.By iterating these two steps,we can obtain a refined estimate of the subspaces and seg-mentation.This is the basic idea behind the K -planes [11]algo-rithm,which generalizes the K -means algorithm [44]from data distributed around multiple cluster centers to data drawn from multiple hyperplanes.The K -subspaces algorithm [12],[13]further generalizes K -planes from multiple hyperplanes to multi-ple affine subspaces of any dimensions and proceeds as follows.Let w ij ¼1if point j belongs to subspace i and w ij ¼0otherwise.Referring back to (1),assume that the number of subspaces n andthe subspace dimensions f d i g ni ¼1are known.Our goal is to findthe points f l i 2R D g n i ¼1,the subspace bases f U i 2RD 3d i g ni ¼1,the low-dimensional representations f Y i 2R d i 3N i g ni ¼1,and thesegmentation of the data f w ij g j ¼1,...,Ni ¼1,...,n .We can do so by minimiz-ing the sum of the squared distances from each data point toits own subspacemin f l i g ,f U i g ,f y i g ,f w ij gXn i ¼1X N j ¼1w ij k x j Àl i ÀU i y j k 2subject to w ij 2f 0,1g and Xn i ¼1w ij ¼1:(11)Given f l i g ,f U i g ,and f y j g ,the optimal value for w ij isw ij ¼1if i ¼arg min k ¼1,...,nk x j Àl k ÀU k y j k 20else:((12)Given f w ij g ,the cost function in (11)decouples as the sum of n cost functions,one per subspace.Since each cost function is identical to that minimized by standard PCA,the optimal values for l i ,U i ,and y j are obtained by applying PCA to each group of points.The K -subspaces algorithm then proceeds by alternatingbetween assigning points to subspaces and reestimating the sub-spaces.Since the number of possible assignments of points to subspaces is finite,the algorithm is guaranteed to converge to a local minimum in a finite number of iterations.The main advantage of K -subspaces is its simplicity since it alternates between assigning points to subspaces and estimating the subspaces via PCA.Another advantage is that it can handle both linear and affine subspaces explicitly.The third advantageis that it converges to a local optimum in a finite number ofiterations.However,K -subspaces suffers from a number of draw-backs.First,its convergence to the global optimum depends on a good initialization.If a random initialization is used,several restarts are often neededto find the global optimum.In practice,one may use any of the algorithms described in this article to reduce the number of restarts needed.We refer the reader to [22]and [45]for two addi-tional initialization methods.Second,K -subspaces is sensitive to outliers,partly due to the use of the ‘2-norm.This issue can be addressed using a robust norm,such as the ‘1-norm,as done by the median K -flat algorithm [15].However,this results in a morecomplex algorithm,which requires solving a robust PCA problemat each iteration.Alternatively,one can resort to nonlinear mini-mization techniques,which are only guaranteed to converge to a local minimum.Third,K -subspaces requires n and f d i g n i ¼1to be known beforehand.One possible avenue to be explored is to usethe model-selection criteria for mixtures of subspaces proposed in [43].We refer the reader to [45]and [46]for a more detailed analy-sis of some of the aforementioned issues.STATISTICAL METHODS The approaches described so far seek to cluster the data according to multiple subspaces using mostly algebraic and geometric proper-ties of a union of subspaces.While these approaches can handle noise in the data,they do not make explicit assumptions about the distribution of data inside the subspaces or about the distribution ofnoise.Therefore,the estimates they provide are not optimal,e.g.,in a maximum likelihood (ML)sense.This issue can be addressed by defining a proper generative model for the data,as described next.MIXTURE OF PROBABILISTIC PCA Resorting back to the geometric PCA model (1),probabilistic PCA (PPCA)[47]assumes that the data within a subspace S is generated as x ¼l þU y þ ,(13)where y and are independent zero-mean Gaussian random vec-tors with covariance matrices I and r 2I ,respectively.Therefore,A VERY SIMPLE WAY OF IMPROVINGTHE PERFORMANCE OF ALGEBRAICALGORITHMS IN THE CASE OF NOISYDATA IS TO USE ITERATIVEREFINEMENT.x is also Gaussian with mean l and covariance matrix R¼UU>þr2I.It can be shown that the ML estimate of l is the mean of the data,and ML estimates of U and r can be obtained from the SVD of the data matrix X.PPCA can be naturally extended to a generative model for a union of subspaces[ni¼1S i by using a mixture of PPCA(MPPCA) model[16].Let G(x;l,R)be the probability density function of a D-dimensional Gaussian with mean l and covariance matrix R. MPPCA uses a mixture of Gaussians modelp(x)¼X ni¼1p i G(x;l i,U i U>iþr2i I),X ni¼1p i¼1,(14)where the parameter p i,called the mixing proportion,represents the a priori probability of drawinga point from subspace S i.TheML estimates of the parametersof this mixture model can befound using expectation maxi-mization(EM)[48].EM is aniterative procedure that alter-nates between data segmenta-tion and model estimation.Specifically,given initial values (e li,~U i,~r i,~p i)for the model parameters,in the E-step,the proba-bility that x j belongs to subspace i is estimated as~p ij¼G(x j;l i,~Ui~U>iþ~r2iI)~p ip(x j),(15)and in the M-step,the~p ij s are used to recompute the subspace parameters using PPCA.Specifically,p i and liare updated as~p i¼1N X Nj¼1~p ij and e li¼1N~p iX Nj¼1~p ij x j,(16)and r i and U i are updated from the SVD of~R i ¼1N~p iX Nj¼1~p ij(x jÀe li)(x jÀe l i)>:(17)These two steps are iterated until convergence to a local max-ima of the log-likelihood.Notice that MPPCA can be seen as a probabilistic version of K-subspaces that uses soft assignments p ij2½0;1 rather than hard assignments w ij¼f0;1g.As in the case of K-subspaces,the main advantage of MPPCA is that it is a simple and intuitive method,where each iteration can be computed in closed form by using PPCA.More-over,the MPPCA model is applicable to both linear and affine subspaces and can be extended to accommodate outliers[49] and missing entries in the data points[50].However,an impor-tant drawback of MPPCA is that the number and dimensions of the subspaces need to be known beforehand.One way to address this issue is to put a prior on these parameters,as shown in[51].A second drawback is that MPPCA is not optimal when the data inside each subspace or the noise is not Gaussian.A third drawback is that MPPCA often converges to a local maximum;hence,a good initialization is critical.The initiali-zation problem can be addressed by using any of the methods described earlier for K-subspaces.For example,the multistage learning(MSL)algorithm[17]uses the factorization method of [8]followed by the agglomerative refinement steps of[33]and [36]for initialization.AGGLOMERATIVE LOSSY COMPRESSIONThe agglomerative lossy compression(ALC)algorithm[18] assumes that the data are drawn from a mixture of degenerate Gaus-sians.However,unlike MPPCA,ALC does not aim to obtain an ML estimate of the parameters of the mixture model.Instead,it looks for the segmentation of the data that minimizes the coding lengthneeded to fit the points with amixture of degenerate Gaussiansup to a given distortion.Specifically,the number ofbits needed to optimally code Nindependent identically distrib-uted(i.i.d.)samples from a zero-mean D-dimensional Gaussian, i.e.,X2R D3N,up to a distortion d can be approximated as ½(NþD)=2 log2det(Iþ(D=d2N)XX>).Thus,the total number of bits for coding a mixture of Gaussians can be approximated asX ni¼1N iþD2log2det IþDd2N iX i X>iÀN i log2N iN,(18)where X i2R D3N i is the data from subspace i,and the last term is the number of bits needed to code(losslessly)the membership of the N samples to the n groups.The minimization of(18)over all possible segmentations of the data is,in general,an intractable problem.ALC deals with this issue by using an agglomerative clustering method.Ini-tially,each data point is considered as a separate group.At each iteration,two groups are merged if doing so results in the great-est decrease of the coding length.The algorithm terminates when the coding length cannot be further decreased.Similar agglomerative techniques have been used[52],[53],though with a different criterion for merging subspaces.ALC can naturally handle noise and outliers in the data.Specif-ically,it is shown in[18]that outliers tend to cluster either as a single group or as small separate groups depending on the dimen-sion of the ambient space.Also,in principle,ALC does not need to know the number of subspaces and their dimensions.In practice, however,the number of subspaces is directly related to the param-eter d.When d is chosen to be very large,all the points could be merged into a single group.Conversely,when d is very small,each point could end up as a separate group.Since d is related to the variance of the noise,one can use statistics on the data to deter-mine d(see[22]and[33]for possible methods).When the number of subspaces is known,one can run ALC for several values of d,dis-card the values of d that give the wrong number of subspaces,and choose the d that results in the segmentation with the smallestAN IMPORTANT DRAWBACK OF MPPCA IS THAT THE NUMBER AND DIMENSIONS OF THE SUBSPACES NEED TO BE KNOWN BEFOREHAND.。
图像分割的改进稀疏子空间聚类方法

图像分割的改进稀疏子空间聚类方法李小平;王卫卫;罗亮;王斯琪【期刊名称】《系统工程与电子技术》【年(卷),期】2015(000)010【摘要】提出一种基于改进稀疏子空间聚类的图像分割方法。
首先将图像进行过分割得到一些均匀区域称为超像素,并提取超像素的颜色直方图作为其特征;然后建立特征数据的改进稀疏子空间表示并由此构造图相似度矩阵,最后利用谱聚类算法得到超像素的聚类结果并作为图像分割结果。
实验结果表明,本文提出的改进稀疏子空间聚类方法具有良好的聚类性能,对噪声具有一定的鲁棒性;用于自然图像能够得到更好的分割效果。
%A novel image segmentation method based on improved sparse subspace clustering is presented. The image to be segmented is over-partitioned into some uniform sub-regions called superpixels,and color histo-gram of each superpixel is computed as its feature data.Then by employing an improved sparse subspace repre-sentation model,the sparse representation coefficient matrix is computed and used to construct the affinity ma-trix of a graph.Finally,the spectral clustering algorithm is used to obtain the image segmentation result.Ex-periments show that the proposed improved sparse subspace clustering method performs well in clustering and is robust to noise.It can obtain good segmentation results for natural color images.【总页数】7页(P2418-2424)【作者】李小平;王卫卫;罗亮;王斯琪【作者单位】西安电子科技大学数学与统计学院,陕西西安 710171;西安电子科技大学数学与统计学院,陕西西安 710171;西安电子科技大学数学与统计学院,陕西西安 710171;西安电子科技大学数学与统计学院,陕西西安 710171【正文语种】中文【中图分类】TP391【相关文献】1.改进的稀疏子空间聚类算法 [J], 张彩霞;胡红萍;白艳萍2.一种改进的稀疏子空间聚类算法 [J], 欧阳佩佩;赵志刚;刘桂峰3.改进重加权稀疏子空间聚类算法 [J], 赵晓晓;周治平;贾旋4.基于加权稀疏子空间聚类多特征融合图像分割 [J], 岳温川;王卫卫;李小平5.图像分割的加权稀疏子空间聚类方法 [J], 李涛;王卫卫;翟栋;贾西西因版权原因,仅展示原文概要,查看原文内容请购买。
- 1、下载文档前请自行甄别文档内容的完整性,平台不提供额外的编辑、内容补充、找答案等附加服务。
- 2、"仅部分预览"的文档,不可在线预览部分如存在完整性等问题,可反馈申请退款(可完整预览的文档不适用该条件!)。
- 3、如文档侵犯您的权益,请联系客服反馈,我们会尽快为您处理(人工客服工作时间:9:00-18:30)。
several efforts have been made for improving their robustness, e.g., the Median K-flats (Zhang et al., 2009) for K-subspaces, the work of (Yang et al., 2006) for RANSAC, and (Ma et al., 2008a; Wright et al., 2008a) use a coding length to characterize a mixture of Gaussian, which may have some robustness. Nevertheless, the problem is still not well solved due to the optimization difficulty, which is a bottleneck for these methods to achieve robustness. Factorization based methods (Costeira & Kanade, 1998; Gruber & Weiss, 2004) seek to represent the given data matrix as a product of two matrices, so that the support pattern of one of the factors reveals the grouping of the points. These methods aim at modifying popular factor analysis algorithms (often based on alternating minimization or EM-style algorithms) to produce such factorizations. Nevertheless, these methods are sensitive to noise and outliers, and it is not easy to modify them to be robust because they usually need iterative optimization algorithms to obtain the factorizations. Generalized Principal Component Analysis (GPCA) (Ma et al., 2008b) presents an algebraic way to model the data drawn from a union of multiple subspaces. By describing a subspace containing a data point by using the gradient of a polynomial at that point, subspace segmentation is then equivalent to fitting the data with polynomials. GPCA can guarantee the success of the segmentation under certain conditions, and it does not impose any restriction on the subspaces. However, this method is sensitive to noise and outliers due to the difficulty of estimating the polynomials from real data, which also causes the high computation cost of GPCA. Recently, Robust Algebraic Segmentation (RAS)(Rao et al., 2010) is proposed to resolve the robustness issue of GPCA. However, the computation difficulty for fitting polynomials is unfathomed. So RAS can make sense only when the data dimension is low and the number of subspaces is small. Recently, the work of (Rao et al., 2009) and Sparse Subspace Clustering (SSC) (Elhamifar & Vidal, 2009) introduced compressed sensing techniques to subspace segmentation. SSC uses the sparsest representation produced by 1 -minimization (Wright et al., 2008b; Eldar & Mishali, 2008) to define the affinity matrix of an undirected graph. Then subspace segmentation is performed by spectral clustering algorithms such as the Normalized Cuts (NCut) (Shi & Malik, 2000). Under the assumption that the subspaces are independent, SSC shows that the sparsest representation is also “block-sparse”. Namely, the within-cluster affinities are sparse (but nonzero) and the between-cluster
‡
Microsoft Research Asia, NO. 49, Zhichun Road, Hai Dian District, Beijing, China, 100190
Abstract
We propose low-rank representation (LRR) to segment data drawn from a union of multiple linear (or affine) subspaces. Given a set of data vectors, LRR seeks the lowestrank representation among all the candidates that represent all vectors as the linear combination of the bases in a dictionary. Unlike the well-known sparse representat sparsest representation of each data vector individually, LRR aims at finding the lowest-rank representation of a collection of vectors jointly. LRR better captures the global structure of data, giving a more effective tool for robust subspace segmentation from corrupted data. Both theoretical and experimental results show that LRR is a promising tool for subspace segmentation.
Robust Subspace Segmentation by Low-Rank Representation
Guangcan Liu † roth@ Zhouchen Lin ‡ zhoulin@ Yong Yu † yyu@ † Shanghai Jiao Tong University, NO. 800, Dongchuan Road, Min Hang District, Shanghai, China, 200240
subspaces have been gaining much attention in recent years. For example, the hotly discussed matrix compees & Recht, 2009; Keshavan et al., 2009; tition (Cand` Cand` es et al., 2009) problem is essentially based on the hypothesis that the data is drawn from a low-rank subspace. However, a given data set is seldom well described by a single subspace. A more reasonable model is to consider data as lying near several subspaces, leading to the challenging problem of subspace segmentation. Here, the goal is to segment (or cluster) data into clusters with each cluster corresponding to a subspace. Subspace segmentation is an important data clustering problem as it arises in numerous research areas, including machine learning (Lu & Vidal, 2006), computer vision (Ho et al., 2003), image processing (Fischler & Bolles, 1981) and system identification. Previous Work. According to their mechanisms of representing the subspaces, existing works can be roughly divided into four main categories: mixture of Gaussian, factorization, algebraic and compressed sensing. In statistical learning, mixed data are typically modeled as a set of independent samples drawn from a mixture of probabilistic distributions. As a single subspace can be well modeled by a Gaussian distribution, it is straightforward to assume that each probabilistic distribution is Gaussian, so known as the mixture of Gaussian model. Then the problem of segmenting the data is converted to a model estimation problem. The estimation can be performed either by using the Expectation Maximization (EM) algorithm to find a maximum likelihood estimate, as done in (Gruber & Weiss, 2004), or by iteratively finding a min-max estimate, as adopted by Ksubspaces (Ho et al., 2003) and Random Sample Consensus (RANSAC) (Fischler & Bolles, 1981). These methods are sensitive to the noise and outliers. So