极端环境微生物16S rRNA测序
16S rRNA基因高通量测序分析 牛粪发酵相关细菌多样性
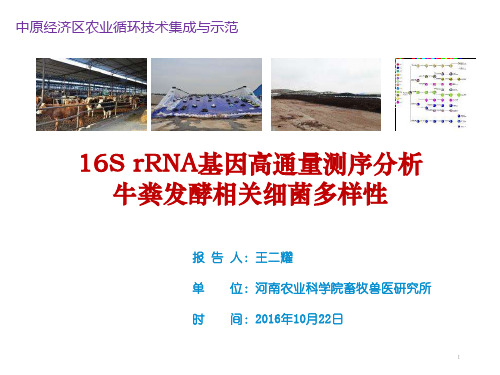
平均值 Mean
0.133 0.096 0.247 0.438
标准差 SD
0.071 0.025 0.058 0.010
P值 P value
0.026 0.004 0.021
-
使用T-test分别分析了门水平上mf与m0、m1、m6的差异物种,结果见表。 mf组与m0、m1、m6组相比具有较多的变形菌门细菌(P < 0.05)和较少的 厚壁菌门细菌(P < 0.05)。
聚类分析
22
组间物种差异分析
分组 (Groups)
m0 m1 m6 mf
平均值 Mean
0.271 0.429 0.313 0.035
厚壁菌门 Firmicutes
标准差 SD
0.046 0.093 0.089 0.008
P值 P value
0.011 0.020 0.026
-
变形菌门 Proteobacteria
Shannon指数(Shannon index)
8.363 8.074 8.417 8.285 7.441 6.683 7.771 7.298 8.256 7.755 5.603 7.205 6.974 7.768 7.91 7.551
Chao1 丰富度估计量 (Chao1 richness estimator)
’s covera
ge) 0.989 0.992 0.988 0.990 0.992 0.99 0.99 0.991 0.989 0.987 0.991 0.989 0.987 0.988 0.988 0.988
注:Shannon指数:用来计算群落多样性,Shannon指数越大,说明群落多样性越高。Chao1 丰 富度估计量:用来计算群落丰度,Chao1值越大代表物种总数越多。测序深度指数:用来计算 测序的覆盖度,其数值越高,则覆盖度越好
16s rdna测序原理
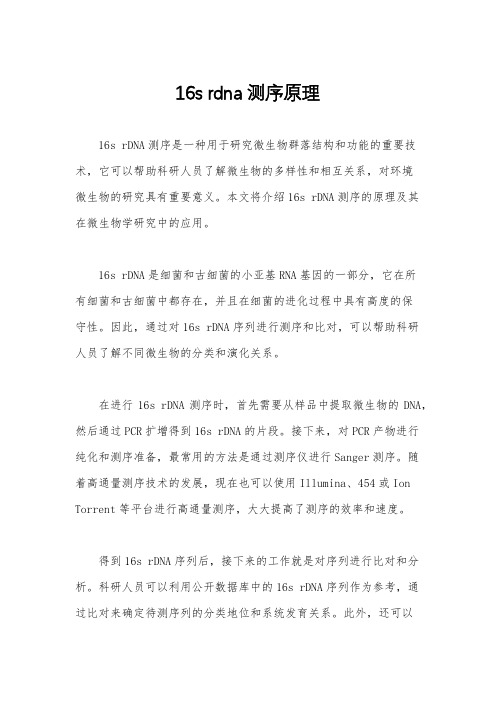
16s rdna测序原理16s rDNA测序是一种用于研究微生物群落结构和功能的重要技术,它可以帮助科研人员了解微生物的多样性和相互关系,对环境微生物的研究具有重要意义。
本文将介绍16s rDNA测序的原理及其在微生物学研究中的应用。
16s rDNA是细菌和古细菌的小亚基RNA基因的一部分,它在所有细菌和古细菌中都存在,并且在细菌的进化过程中具有高度的保守性。
因此,通过对16s rDNA序列进行测序和比对,可以帮助科研人员了解不同微生物的分类和演化关系。
在进行16s rDNA测序时,首先需要从样品中提取微生物的DNA,然后通过PCR扩增得到16s rDNA的片段。
接下来,对PCR产物进行纯化和测序准备,最常用的方法是通过测序仪进行Sanger测序。
随着高通量测序技术的发展,现在也可以使用Illumina、454或Ion Torrent等平台进行高通量测序,大大提高了测序的效率和速度。
得到16s rDNA序列后,接下来的工作就是对序列进行比对和分析。
科研人员可以利用公开数据库中的16s rDNA序列作为参考,通过比对来确定待测序列的分类地位和系统发育关系。
此外,还可以利用一些生物信息学工具对序列进行多样性分析、物种丰度分析等,从而了解微生物群落的结构和功能。
在微生物学研究中,16s rDNA测序被广泛应用于环境微生物群落的研究。
通过对土壤、水体、空气等不同环境中微生物的16s rDNA进行测序和分析,可以揭示微生物的多样性、分布规律以及其对环境的影响。
此外,16s rDNA测序还可以用于研究人体内的微生物群落,例如肠道菌群的研究,有助于了解微生物与宿主健康的关系。
总之,16s rDNA测序是一种重要的技术手段,它为科研人员提供了解微生物多样性、分类和系统发育关系的重要途径,对微生物学、生态学和生物医学研究具有重要意义。
随着测序技术的不断发展和完善,相信16s rDNA测序在微生物学研究中的应用将会更加广泛和深入。
微生物16S测序数据的正确打开方式

微生物16S测序数据的正确打开方式16S rRNA基因测序(也称16S rDNA测序)是最常用的菌群多样性分析的手段。
对于新手,如果收到一份不讲“人话”的16S测序分析报告,很快就会被各种生态学术语、各种指数、各种分析方法弄晕。
7个问题串起16S测序的核心结果怎么办?用你的研究逻辑来梳理16S测序数据(图1)。
简单地说,做16S测序是为了鉴定样本中的微生物(细菌)群组成,找微生物群与疾病或表型的相关性。
详细地说,1)首先想了解在不同组样本中各有哪些微生物存在和丰富度(对应于菌群鉴定和α多样性分析);2)接着想看不同样本组间微生物群组成是否存在差异(对应于β多样性分析);3)如果是,那么就有必要找出引起不同组样本微生物群差异的关键菌。
如果不是,那说明微生物群比如肠道菌群与疾病或表型可能并不相关(基于已有的研究,这种可能性比较小);4)找到了关键菌,在临床上,很自然会想到,这些(个)关键菌是否可以作为Biomarker(对应于疾病诊断模型构建),比如用于区分糖尿病前期患者与健康组的标志物;5)以及这些(个)菌是否与临床指标具有相关性(对应于菌群与临床指标的相关性分析);也会进一步想到,既然不同组的微生物群落存在差异,又与疾病具有相关性,6)那么这些菌群是如何影响宿主的,可能参与了哪些代谢途径(对应于菌群基因功能预测);7)这些预测到的菌群功能是否与疾病有关,通常是肯定的。
最后把这些结果整合起来分析,可以初步得出菌群组成的变化是如何与疾病或表型相关的。
顺着上述7个生物学问题来看16S测序结果,你会轻松拨开迷雾,直达核心结果。
图1 7个问题串起16S测序的核心结果6张图就够发菌群与疾病相关性文章编者对2019发表的数十篇以16S测序为主的肠道菌群与疾病关系研究文章(IF 5至10分)的内容进行了分析和归纳,发现大部分文章的Results部分都是由图1所列的核心结果组成。
以联川生物医学16S测序报告为例,具体讲解16S测序文章中的核心结果及其对应的图表。
16s rdna测序原理
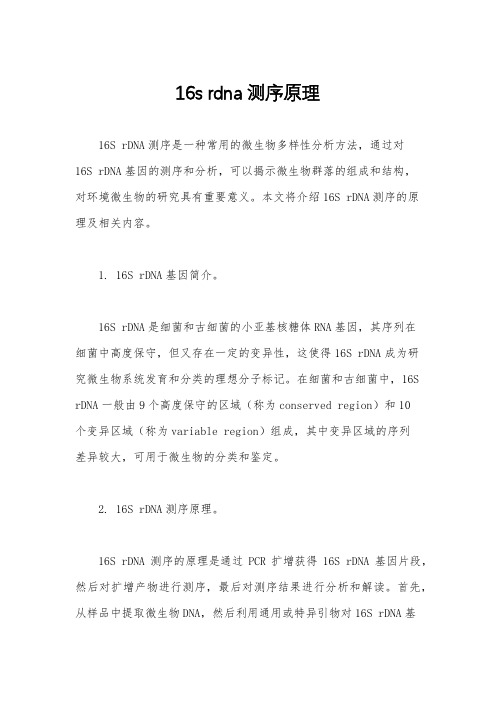
16s rdna测序原理16S rDNA测序是一种常用的微生物多样性分析方法,通过对16S rDNA基因的测序和分析,可以揭示微生物群落的组成和结构,对环境微生物的研究具有重要意义。
本文将介绍16S rDNA测序的原理及相关内容。
1. 16S rDNA基因简介。
16S rDNA是细菌和古细菌的小亚基核糖体RNA基因,其序列在细菌中高度保守,但又存在一定的变异性,这使得16S rDNA成为研究微生物系统发育和分类的理想分子标记。
在细菌和古细菌中,16S rDNA一般由9个高度保守的区域(称为conserved region)和10个变异区域(称为variable region)组成,其中变异区域的序列差异较大,可用于微生物的分类和鉴定。
2. 16S rDNA测序原理。
16S rDNA测序的原理是通过PCR扩增获得16S rDNA基因片段,然后对扩增产物进行测序,最后对测序结果进行分析和解读。
首先,从样品中提取微生物DNA,然后利用通用或特异引物对16S rDNA基因进行PCR扩增,得到所需的16S rDNA片段。
接下来,对PCR产物进行纯化和测序,通常采用Sanger测序法或高通量测序技术(如Illumina、454、Ion Torrent等)。
最后,利用生物信息学方法对测序结果进行分析,包括序列比对、物种注释、多样性分析等。
3. 16S rDNA测序分析。
在16S rDNA测序分析中,首先需要对测序结果进行质控和过滤,去除低质量序列和引物污染,然后进行序列比对和物种注释。
序列比对是将测序结果与16S rDNA数据库进行比对,找到最佳匹配的参考序列,从而确定微生物的分类和系统发育关系。
物种注释是根据比对结果,将未知序列注释为已知的微生物分类单元,如属、种等。
此外,还可以进行多样性分析,如Alpha多样性指数(反映微生物群落的丰富度和多样性)、Beta多样性分析(比较不同样品间的微生物群落差异)等。
菌群16s测序解读
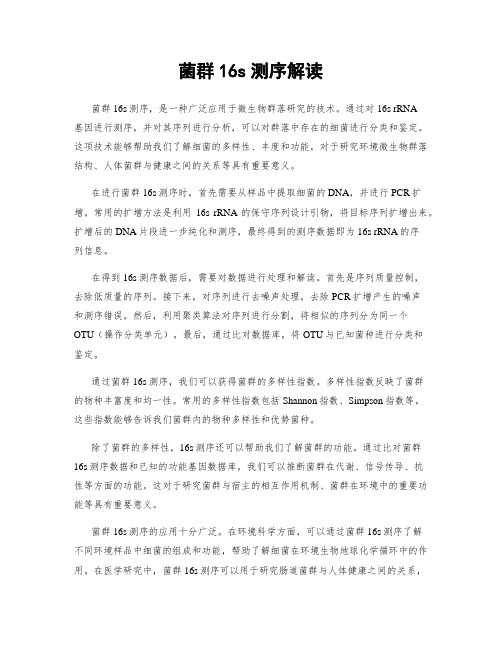
菌群16s测序解读菌群16s测序,是一种广泛应用于微生物群落研究的技术。
通过对16s rRNA基因进行测序,并对其序列进行分析,可以对群落中存在的细菌进行分类和鉴定。
这项技术能够帮助我们了解细菌的多样性、丰度和功能,对于研究环境微生物群落结构、人体菌群与健康之间的关系等具有重要意义。
在进行菌群16s测序时,首先需要从样品中提取细菌的DNA,并进行PCR扩增。
常用的扩增方法是利用16s rRNA的保守序列设计引物,将目标序列扩增出来。
扩增后的DNA片段进一步纯化和测序,最终得到的测序数据即为16s rRNA的序列信息。
在得到16s测序数据后,需要对数据进行处理和解读。
首先是序列质量控制,去除低质量的序列。
接下来,对序列进行去噪声处理,去除PCR扩增产生的噪声和测序错误。
然后,利用聚类算法对序列进行分割,将相似的序列分为同一个OTU(操作分类单元)。
最后,通过比对数据库,将OTU与已知菌种进行分类和鉴定。
通过菌群16s测序,我们可以获得菌群的多样性指数。
多样性指数反映了菌群的物种丰富度和均一性。
常用的多样性指数包括Shannon指数、Simpson指数等。
这些指数能够告诉我们菌群内的物种多样性和优势菌种。
除了菌群的多样性,16s测序还可以帮助我们了解菌群的功能。
通过比对菌群16s测序数据和已知的功能基因数据库,我们可以推断菌群在代谢、信号传导、抗性等方面的功能。
这对于研究菌群与宿主的相互作用机制、菌群在环境中的重要功能等具有重要意义。
菌群16s测序的应用十分广泛。
在环境科学方面,可以通过菌群16s测序了解不同环境样品中细菌的组成和功能,帮助了解细菌在环境生物地球化学循环中的作用。
在医学研究中,菌群16s测序可以用于研究肠道菌群与人体健康之间的关系,探索微生物在疾病发生发展中的作用。
在食品安全检测中,通过菌群16s测序可以追踪食品中可能存在的致病菌,提供相关的病原菌检测和风险评估。
尽管菌群16s测序在微生物群落研究中具有广泛应用,但也存在一些限制。
16s rdna序列 16s rrna基因序列
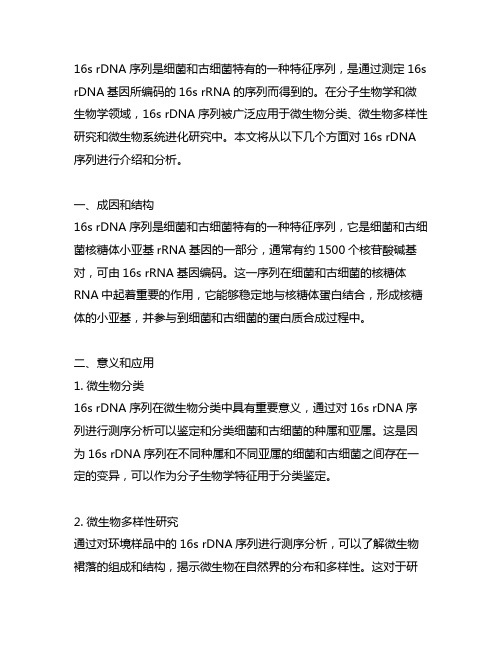
16s rDNA序列是细菌和古细菌特有的一种特征序列,是通过测定16s rDNA基因所编码的16s rRNA的序列而得到的。
在分子生物学和微生物学领域,16s rDNA序列被广泛应用于微生物分类、微生物多样性研究和微生物系统进化研究中。
本文将从以下几个方面对16s rDNA 序列进行介绍和分析。
一、成因和结构16s rDNA序列是细菌和古细菌特有的一种特征序列,它是细菌和古细菌核糖体小亚基rRNA基因的一部分,通常有约1500个核苷酸碱基对,可由16s rRNA基因编码。
这一序列在细菌和古细菌的核糖体RNA中起着重要的作用,它能够稳定地与核糖体蛋白结合,形成核糖体的小亚基,并参与到细菌和古细菌的蛋白质合成过程中。
二、意义和应用1. 微生物分类16s rDNA序列在微生物分类中具有重要意义,通过对16s rDNA序列进行测序分析可以鉴定和分类细菌和古细菌的种属和亚属。
这是因为16s rDNA序列在不同种属和不同亚属的细菌和古细菌之间存在一定的变异,可以作为分子生物学特征用于分类鉴定。
2. 微生物多样性研究通过对环境样品中的16s rDNA序列进行测序分析,可以了解微生物裙落的组成和结构,揭示微生物在自然界的分布和多样性。
这对于研究微生物的生态学、环境适应性和生态功能具有重要意义。
3. 微生物系统进化研究利用16s rDNA序列进行系统进化研究,可以揭示细菌和古细菌的系统发育关系和演化过程,为了解细菌和古细菌的起源、多样性和进化提供重要的分子学证据。
三、研究方法1. PCR扩增通常情况下,从细菌或古细菌的DNA中提取16s rDNA序列,然后利用PCR技术进行扩增。
通过选择适当的引物和反应条件,可以特异性地扩增出16s rDNA序列,为后续的测序分析做准备。
2. 测序分析测序是获取16s rDNA序列信息的关键步骤,目前常用的测序方法包括Sanger测序和高通量测序。
通过测序分析,可以获得16s rDNA 序列的具体碱基序列信息,用于后续的分类鉴定和系统进化研究。
16s rrna基因测序原理

16s rrna基因测序原理
16S rRNA基因测序是一种常用的微生物分析方法,用于研究
和鉴定微生物的种类和数量。
16S rRNA是细菌和古细菌的核
糖体RNA的一个组成部分,它在不同的微生物中存在一定的
变异性,这种变异性可以用来区分不同的微生物。
16S rRNA基因测序的原理是通过提取样品中的总DNA或总RNA,然后使用聚合酶链式反应(PCR)扩增16S rRNA基因。
PCR反应使用一对通用引物,能扩增大多数细菌和古细菌的
16S rRNA基因片段。
扩增获得的DNA片段可以通过电泳或
其他方法进行分离和纯化。
得到纯化的DNA片段后,可以使用Sanger测序技术或高通量
测序技术对其进行测序。
Sanger测序技术是一种经典的测序方法,通过反复合成和分离DNA链的方式逐个测序碱基。
高通
量测序技术,如Illumina平台,使用一种并行测序原理,可以
同时测序大量的DNA片段。
通过测序获得的16S rRNA序列可以通过比对已知的16S
rRNA数据库进行比对分析,以确定样品中微生物的种类和亲
缘关系。
也可以通过对多个样品的测序结果进行比较,进行微生物群落结构和多样性分析。
总的来说,16S rRNA基因测序是一种通过扩增和测序16S rRNA基因来分析微生物组成和多样性的方法,能够对微生物
进行定性和定量分析。
菌群16s测序解读
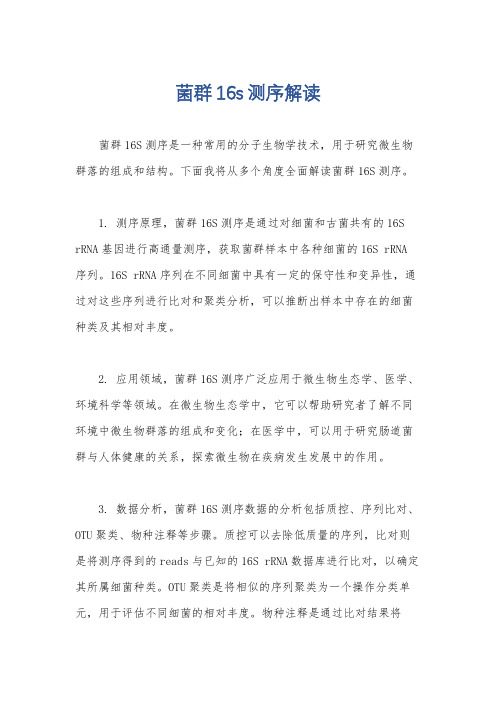
菌群16s测序解读菌群16S测序是一种常用的分子生物学技术,用于研究微生物群落的组成和结构。
下面我将从多个角度全面解读菌群16S测序。
1. 测序原理,菌群16S测序是通过对细菌和古菌共有的16S rRNA基因进行高通量测序,获取菌群样本中各种细菌的16S rRNA 序列。
16S rRNA序列在不同细菌中具有一定的保守性和变异性,通过对这些序列进行比对和聚类分析,可以推断出样本中存在的细菌种类及其相对丰度。
2. 应用领域,菌群16S测序广泛应用于微生物生态学、医学、环境科学等领域。
在微生物生态学中,它可以帮助研究者了解不同环境中微生物群落的组成和变化;在医学中,可以用于研究肠道菌群与人体健康的关系,探索微生物在疾病发生发展中的作用。
3. 数据分析,菌群16S测序数据的分析包括质控、序列比对、OTU聚类、物种注释等步骤。
质控可以去除低质量的序列,比对则是将测序得到的reads与已知的16S rRNA数据库进行比对,以确定其所属细菌种类。
OTU聚类是将相似的序列聚类为一个操作分类单元,用于评估不同细菌的相对丰度。
物种注释是通过比对结果将OTU与已知的细菌物种进行关联。
4. 数据解读,菌群16S测序结果可以通过多种方法进行解读。
首先,可以绘制菌群组成的堆栈柱状图或饼图,展示不同细菌的相对丰度。
其次,可以进行群落结构的比较分析,如聚类分析、PCoA 分析等,帮助研究者了解不同样本之间的相似性或差异性。
此外,还可以进行功能预测,通过将16S rRNA序列与已知功能基因的数据库进行比对,推测菌群的功能潜力。
5. 注意事项,在菌群16S测序的解读过程中,需要注意样本的选择、实验设计的合理性以及数据分析的准确性。
此外,对于菌群16S测序结果的解读,需要结合相关的背景知识和文献,避免过度解读或片面解读。
综上所述,菌群16S测序是一种重要的技术手段,可以帮助研究者了解微生物群落的组成和结构,以及其在不同环境中的功能和作用。
- 1、下载文档前请自行甄别文档内容的完整性,平台不提供额外的编辑、内容补充、找答案等附加服务。
- 2、"仅部分预览"的文档,不可在线预览部分如存在完整性等问题,可反馈申请退款(可完整预览的文档不适用该条件!)。
- 3、如文档侵犯您的权益,请联系客服反馈,我们会尽快为您处理(人工客服工作时间:9:00-18:30)。
ORIGINAL ARTICLEContemporary environmental variation determines microbial diversity patterns in acid mine drainage Jia-Liang Kuang1,Li-Nan Huang1,Lin-Xing Chen,Zheng-Shuang Hua,Sheng-Jin Li, Min Hu,Jin-Tian Li and Wen-Sheng Shu1State Key Laboratory of Biocontrol and Guangdong Key Laboratory of Plant Resources,School of Life Sciences,Sun Yat-sen University,Guangzhou,People’s Republic of ChinaA wide array of microorganisms survive and thrive in extreme environments.However,we know littleabout the patterns of,and controls over,their large-scale ecological distribution.To this end,wehave applied a bar-coded16S rRNA pyrosequencing technology to explore the phylogeneticdifferentiation among59microbial communities from physically and geochemically diverse acidmine drainage(AMD)sites across Southeast China,revealing for the first time environmentalvariation as the major factor explaining community differences in these harsh environments.Our data showed that overall microbial diversity estimates,including phylogenetic diversity,phylotype richness and pairwise UniFrac distance,were largely correlated with pH conditions.Furthermore,multivariate regression tree analysis also identified solution pH as a strong predictorof relative lineage abundance.Betaproteobacteria,mostly affiliated with the‘Ferrovum’genus,wereexplicitly predominant in assemblages under moderate pH conditions,whereas Alphaproteobac-teria,Euryarchaeota,Gammaproteobacteria and Nitrospira exhibited a strong adaptation to moreacidic environments.Strikingly,such pH-dependent patterns could also be observed in asubsequent comprehensive analysis of the environmental distribution of acidophilic microorgan-isms based on16S rRNA gene sequences previously retrieved from globally distributed AMD andassociated environments,regardless of the long-distance isolation and the distinct substrate types.Collectively,our results suggest that microbial diversity patterns are better predicted bycontemporary environmental variation rather than geographical distance in extreme AMD systems.The ISME Journal advance online publication,22November2012;doi:10.1038/ismej.2012.139Subject Category:microbial population and community ecologyKeywords:acid mine drainage;biogeography;contemporary environmental variation;geographicaldistance;microbial diversity;pyrosequencingIntroductionMicrobial biogeography is increasingly becoming an exciting topic in microbial ecology and a growing number of researches are addressing the spatial scaling and distribution pattern of microorganisms in the environment(Martiny et al.,2006;Green et al., 2008).Despite their tremendous potential for global dispersal,there is accumulating evidence that free-living microorganisms exhibit nonrandom distribu-tion patterns across diverse habitats at various spatial scales.Niche-based processes have been implied as the primary drivers for the widely observed environ-ment-dependent diversity patterns and environmen-tal variables such as salinity(Lozupone and Knight,2007;Auguet et al.,2010),pH(Fierer and Jackson, 2006;Lauber et al.,2009;Rousk et al.,2010;Griffiths et al.,2011)and C:N ratio(Bates et al.,2011) identified as the major determinants of microbial community composition.However,there is also evidence that spatial distance,which may be seen as a proxy variable that represents differential com-munity dynamics related to the past historical events and disturbances(Ramette and Tiedje,2007),have a role in structuring natural microbial assemblages(Cho and Tiedje,2000;McAllister et al.,2011;Martiny et al.,2011).These studies of biogeography have provided initial insights into the processes that generate diversity patterns and improved our under-standing of why organisms live where they do and how they will respond to environmental change. However,systematically exploring the microbial geographical patterns by considering both contem-porary environmental variations and spatial distance simultaneously is limited(Ramette and Tiedje,2007; Ge et al.,2008),and the relative importance of these factors in shaping microbial communities in natural environments remains largely unsolved.Correspondence:W-S Shu,School of Life Sciences,Sun Yat-sen University,No.135,Xin-Gang-Xi Road,Guangzhou510275, People’s Republic of China.E-mail:shuws@1The first two authors contributed equally to this work. Received3July2012;revised1October2012;accepted1October 2012The ISME Journal(2012),1–13&2012International Society for Microbial Ecology All rights reserved1751-7362/12 /ismejThe Earth’s extreme environments harbor a wide array of extraordinary microorganisms that are,in some ways or others,similar to the ancient life forms (Amaral-Zettler et al.,2011).Analyzing the dynamic changes of these microbial communities coupled with physical and geochemical factors will reveal how microbes can adapt and tolerate to different kinds of environmental extremes and increase our understanding of microbial ecology and evolution. Acid mine drainage(AMD)is a widespread envir-onmental problem primarily resulting from the oxidative dissolution of pyrite(FeS2)and other sulfide minerals exposed to oxygen and water during metal ore mining(Nordstrom and Alpers, 1999).Although typically low in overall microbial diversity,these unique environments harbor meta-bolically active,acidophilic microorganisms that are well adapted to the multiple environmental stresses encountered and are mainly responsible for the generation of these hot,sulfuric acid-and toxic metals-rich solutions(Baker and Banfield,2003). While Acidithiobacillus ferrooxidans and Leptospir-illum ferrooxidans(the two iron-oxidizing species most commonly isolated from acidic drainage waters)are widely implicated to be the microorgan-isms that control the rate of AMD generation,more recent molecular-based investigations have revealed that other less known organisms(for example, Ferroplasma spp.in the Archaea and Leptospirillum group III within the Nitrospira)are dominant in certain specific mine environments and they probably have important roles in the pyrite dissolu-tion in situ(Bond et al.,2000;Tan et al.,2007; Huang et al.,2011).Because of their biological and geochemical simplicity,AMD environments have the potential as model systems for quantitative analysis of microbial ecology and evolution and community function(Baker and Banfield,2003; Denef et al.,2010).The first16S rRNA gene-based microbial diversity surveys of AMD systems date back to the mid-1990s(Goebel and Stackebrandt, 1994).Further molecular diversity inventories of AMD microbes have been conducted in a number of acidic environments in diverse geographical loca-tions,including Iron Mountain in California,USA (Bond et al.,2000;Druschel et al.,2004)and the Rio Tinto(RT)in southwestern Spain(Gonzalez-Toril et al.,2003;Garcia-Moyano et al.,2007).Although expanding our knowledge of the biodiversity of extremely acidic systems,these studies have typically examined a limited number of samples from a single mining environment,and the sequen-cing depth provided by a standard clone library analysis is relatively limited.Consequently,a global understanding of the pattern of AMD microbial diversity has not been available,and it is not clear how communities are shaped by the prevailing geochemical factors in these extreme environments and whether the major environmental determinants of microbial community composition differ from those working in‘normal’environments.The advent of high-throughput pyrosequencing technology now affords new opportunities to address these knowledge gaps by comprehensively characterizing microbial communities in large numbers of ecologi-cal samples to examine broad trends of microbial distribution in AMD environments.Here,we applied a massively parallel tag pyro-sequencing of the V4region of the16S rRNA gene to examine in-depth microbial communities from diverse AMD sites across Southeast China to gain insight into the ecological characteristics of these extraordinary microorganisms.We wanted to deter-mine whether AMD microbes exhibit specific biogeographic patterns and which abiotic factors (contemporary environmental factors versus spatial distance)are more important in relating their diversity and composition across a broad range of physical and geochemical gradients.A meta-analysis based on previous molecular inventory studies of AMD environments from diverse geogra-phical locations was subsequently conducted to determine if the patterns observed in our pyrose-quence data set are applicable at broader(global) scales.Materials and methodsSample collection,physicochemical analyses and DNA extractionA total of59AMD samples were collected from 14mining areas(12active and two abandoned) across Southeast China(19.241–31.641N,105.711–118.62o E;Figure1and Supplementary Table1)withFigure1Location of sampling sites of AMD across Southeast China.Detailed site characteristics are listed in Supplementary Tables1and2.Microbial diversity patterns in acid mine drainageJ-L Kuang et al 2The ISME Journaldifferent mineralogy(for example,copper,lead–zinc,pyrite and polymetallic)and representing a broad variety of environmental conditions.Site locations were recorded by global positioning system and the geographical distances between sampling sites ranged from about10m to over 1600km.Water samples were taken from acidic streams,runoff ponds and AMD collection ponds (for storage before treatment)using sterile serum bottles and immediately kept on ice for transport to the laboratory.For DNA extraction,a500ml aliquot of each sample was coarse filtered through a3m m fiber filter(Type A/D Glass;Pall Corporation,Port Washington,NY,USA)and then filtered through a 0.22m m polyethersulfone membrane filter(Supor-200;Pall)using a peristaltic pump.The cell pellets on the polyethersulfone membranes were stored at À401C before nucleic acid extraction,and the filtrates were temporarily stored at41C for the chemical analyses within10days.Temperature,solution pH, dissolved oxygen(DO)and electrical conductivity (EC)were measured on-site by use of specific electrodes.Ferric and ferrous irons were measured by ultraviolet colorimetric assay with1,10-phenan-throline at530nm(Hill et al.,1978).Total organic carbon(TOC)was measured by high-temperature catalytic oxidation and infrared detection with a TOC analyzer(TOC-V CSH;Shimadzu,Kyoto,Japan) and sulfate determined by a BaSO4-based turbidi-metric method(Chesnin and Yien,1951).The element analysis was performed by inductively coupled optical emission spectrometry(Optima2100DV;Per-kin-Elmer,Waltham,MA,USA)after the filtrates were digested at1801C with conc.HNO3and HCl(1:3, v vÀ1).Genomic DNA was extracted from the filters by following the protocol described by Frias-Lopez et al. (2008).As an additional step to facilitate cell lysis,the membranes were placed into the bead tubes and homogenized by shaking with a Fast Prep-24Homo-genization System equipped with QuickPrep Adapter (MP Biomedicals,Seven Hills,NSW,Australia)for 40s at maximum speed.Amplification and bar-coded pyrosequencing of bacterial and archaeal16S rRNA genesPCR amplification,purification,pooling and pyro-sequencing of a region of the16S rRNA gene were performed following the procedure described by Fierer et al.(2008).We used the primer set F515(50-GTGCCAGCMGCCGCGGTAA-30)and R806 (50-GGACTACVSGGGTATCTAAT-30)that was desig-ned to amplify the V4hypervariable region and demonstrated in silico to be universal for nearly all bacterial and archaeal taxa(Bates et al.,2011).This short targeted gene region(B300bp)can provide sufficient resolution for the accurate taxonomic classification of microbial sequences(Liu et al., 2007).An8-bp error-correcting tag(Hamady et al., 2008)was added to the forward primer.Samples were amplified in triplicate following the thermal cycling described previously(Fierer et al.,2008). Replicate PCR reactions for each sample werepooled and purified using a QIAquick Gel Extrac-tion Kit(Qiagen,Chatsworth,CA,USA).A single composite sample for pyrosequencing was preparedby combining approximately equimolar amounts ofPCR products from each sample.Sequencing wascarried out on a454GS FLX Titanium pyrosequen-cer(Roche454Life Sciences,Branford,CT,USA)at Macrogen(Seoul,Korea).Processing of pyrosequencing dataRaw data generated from the454-pyrosequencingrun were processed and analyzed following the pipelines of Mothur(Schloss et al.,2009)and QIIME (Caporaso et al.,2010).Pyrosequences were denoized using the commands of‘shhh.flows’(translation of PyroNoise algorithm;Quince et al., 2009)and‘pre.cluster’(Huse et al.,2010)in Mothur platform.Chimeric sequences were identified and removed using UCHIME with de novo method (Edgar et al.,2011).Quality sequences were subse-quently assigned to samples according to theirunique8-bp barcode and binned into phylotypesusing average clustering algorithm(Huse et al., 2010)at the97%similarity level.Representative sequences were aligned using NAST(DeSantis et al., 2006)and then used to build the neighbor-joining phylogenetic trees using FastTree(Price et al.,2009). Taxonomic classification of phylotypes was deter-mined based on the Ribosomal Database Project atthe80%threshold(Wang et al.,2007).We estimated the relative abundance(%)of individual taxa within each community by compar-ing the number of sequences assigned to a specifictaxon versus the number of total sequences obtainedfor that sample.We also calculated the numberof phylotypes(richness)and the Faith’s index of phylogenetic diversity(Faith’s PD,sums of the totalbranch length in a phylogenetic tree that leads toeach member of a community)to compare the community diversity across all59AMD samples. Weighted UniFrac analyses(Lozupone and Knight, 2005;Lozupone et al.,2006)were applied to calculate the pairwise distance between microbial assemblages.Calculations of diversity indices and UniFrac dissimilarity were based on a randomly selected subset of540sequences per sample. Normalizing the number of sequences per sample allowed us to control the effects of survey effort atsame level in comparing the diversity indices and lineage-specific UniFrac distances across the sam-ples(Lauber et al.,2009).Data collection and beta diversity of microbialcommunities from global AMD and associatedenvironmentsTo reveal broader patterns in the distribution of microorganisms among acidic environmentsMicrobial diversity patterns in acid mine drainageJ-L Kuang et al3The ISME Journalglobally distributed,we searched papers in Web of Science and reviewed molecular inventory studies that explored microbial communities in natural AMD and associated environments(such as acidic biofilm,sediment and tailings)from diverse geogra-phical locations.16S rRNA clone sequences were identified and recovered from GenBank for samples with detailed information of operational taxonomic units and their relative abundance,and environ-mental parameters were extracted and summarized. Community composition and weighted UniFrac dissimilarity were calculated for the subsequent meta-analysis(see detailed methods in Supplemen-tary Information).Statistical analysesAll statistical analyses were implemented using various packages within the R statistical computing environment.Aggregated boosted tree analysis (ABT)(De’ath,2007)was carried out using the gbmplus package(with5000trees used for the boosting,10-folds cross-validation and three-way interactions)to evaluate quantitatively the relative influence of environmental variables to the commu-nity diversity.A sum of squares multivariate regres-sion tree(MRT)(De’ath,2002)was performed using the mvpart package(with default parameters) to relate relative abundance of lineages to the site characteristics.Multiple linear regression(MLR) with stepwise method and Mantel test were con-ducted within the vegan package(Oksanen et al., 2010)to test the significance between diversity indices and the site properties.For data sets of PD,phylotypes richness and relative abundance of individual taxa in the subsequent MLR analyses, independent variables,including pH,EC,DO,TOC, SO42À,Fe3þ,Fe2þ,latitude and longitude,were input into the MLR model,while for the weighted UniFrac dissimilarity data set Bray–Curtis dissim-ilarities of these environmental variables and geo-graphical distance were used.ABT and MRT analyses are statistical techniques that fundamen-tally aim to perform accurate prediction and explanation between complex ecological data(as we used diversity indices and dissimilarity matrices in ABT and relative lineage abundance in MRT)and environmental characteristics(De’ath,2002,2007). More importantly,the application of these methods allowed us to quantify and visualize the different contribution of environmental variables and geogra-phical distance to the community diversity.ResultsSite characteristics and environmental conditions The AMD samples captured a wide range of physical and geochemical gradients(Supplementary Tables1 and2)and were characterized by extremely acidic pH values ranging from 1.9to 4.1(2.6±0.45, mean±s.d.)and high concentrations of dissolved solids(measured as EC)ranging from134to20000 (4528±3786)m S cmÀ1.Concentrations of sulfate and ferric and ferrous irons were highly variable across the samples,averaging at3787±2129,1317±3915 and130±354mg lÀ1,respectively.Additionally,DO (3.3±3.6mg lÀ1)and TOC(8.9±12.4mg lÀ1)were also subjected to considerable fluctuations.Composition and diversity of AMD microbial communitiesThe bar-coded pyrosequencing generated131720 quality sequences from the59AMD samples,with an average of2234±1756sequences and a range of 542to9263sequences per sample.All but436of the 131720sequences could be classified at the domain level(Bacteria or Archaea)by the RDP classifier (80%threshold).A total of2198phylotypes were defined at the97%similarity level,with the majority(B54%of the phylotypes)represented by a single sequence,whereas all of these singletons could be assigned to the taxa that were identified in the whole pyrosequence data set.The number of phylotypes detected in each sample ranged from 10to244,with an average of61±49according to a subset of540randomly selected sequences.Of the classifiable sequences,18phyla were identified, with Proteobacteria,Nitrospira and Euryarchaeota representing the most dominant lineages and accounting for72%,12%and5.1%of all sequences, respectively.Some other phyla were less abundant but still detected in most of the samples;these included Firmicutes(3.4%),Actinobacteria(1.1%) and Acidobacteria(1.1%).Down to the genus level, the most abundant phylotypes were affiliated with the‘Ferrovum’(59333sequences),Acidithiobacillus (13744sequences),Acidiphilium(9461sequences) and Leptospirillum(7756sequences);these collec-tively accounted for69%of the total sequences. Specifically,the Acidithiobacillus sequences were composed predominantly(495%)of A.ferrooxi-dans-like organisms,with an approximately4.3%of A.caldus.The largest portion of the Leptospirillum reads was affiliated with Leptospirillum ferrodiazo-trophum(60%),with the remaining being phylo-genetically affiliated with L.ferrooxidans(439%) and L.ferriphilum(0.34%).Additionally,almost all of the Acidiphilium sequences(498%)were affiliated with Acidiphilium cryptum.The relative abundance of different lineages varied considerably across the AMD communities(Figures2and3and Supplementary Table3a,also see the variances of the measured averages of individual lineages com-prising the defined pH levels in Supplementary Tables3b and4).Relative influence of environmental conditions on microbial diversityABT models were conducted to interpret the relative importance of environmental conditions and spatialMicrobial diversity patterns in acid mine drainageJ-L Kuang et al 4The ISME Journalisolation to the diversity patterns of AMD microbial communities in Southeast China.ABT analysis indicated that pH was the major factor for the patterns of both PD and phylotypes,accounting for approximately 23%and 21%of the relative influ-ence,respectively (Figures 4a and b).Partial depen-dency plots of pH from the fitted model revealed that high values of diversity index were most likely to be observed at higher pH conditions (Supplementary Figures 1a and b).These results were in good agreement with the significantly positive correlations between solution pH and over-all diversity determined by MLR analysis (Faith’s PD:r ¼0.349,P ¼0.008;Phylotypes:r ¼0.359,P ¼0.006;for both PD and Phylotypes,environmen-tal variables other than pH were all eliminated in the predicted MLR model,see Statistical analyses).Additionally,the ABT analysis also revealed a moderate effect for EC and weaker effects for otherenvironmental variables such as TOC and DO on both diversity estimates.In comparison,the spatial isolation represented by the gradients of latitude and longitude made less contributions,indicating that there is no obvious effect of geographical distance on the AMD microbial diversity.When considering the pairwise community distances between microbial assemblages,the variation of pH calculated by Bray–Curtis distance again revealed the most important influence on the weighted UniFrac dissimilarity (Figure 4c).This relationship was corroborated by the Mantel test (Spearman’s r ¼0.329,P o 0.001)and MLR analysis (r ¼0.337,P o 0.001,with Bray–Curtis dissimilarity of pH as the only variable remained in the MLR model),with higher divergence of solution pH likely leading to higher UniFrac dissimilarity (Supplementary Figure 1c).In contrast,no significant correlation between geographical distance and the UniFrac distance was detected by the Mantel test (Spear-man’s r ¼0.106,P ¼0.072),implying that the contribution of spatial isolation to the community dissimilarity was limited (Figure 4c).Relationship between relative abundance of dominant lineages and environmental conditionsTaxonomy-supervised analysis has the advantages of less computation requirement and more tolerance of sequencing errors (Sul et al.,2011).We further conducted an MRT analysis using our AMD field data,which interpreted the relationship between the relative abundance of dominant lineages and envir-onmental conditions by providing a tree with seven terminal nodes based on pH,TOC and concentra-tions of sulfate,ferric and ferrous irons,collectively explaining 70%of the standardized abundance variance (Figure 5).The results suggested that spatial isolation represented by sampling location (measuring longitude and latitude as two variables in the MRT model)was less of a factor than environmental variables in explaining thevariationFigure 2Relative abundances (%)of dominant lineages (phylum level)in overall communities and in different groups of AMD samples along the gradient of pH levels.The numbers above the columns indicate the number of samples in each group.Others include 12phyla:Bacteroidetes ,Chlamydiae ,Chloroflexi ,Crenarchaeota ,Cyanobacteria ,Deinococcus-Thermus ,Gemmatimonadetes ,OD1,OP11,Planctomycetes ,TM7and Verrucomicrobia ;and two subphyla for Proteobacteria :Deltaproteobacteria and Epsilonproteobacteria.Figure 3Relative abundances of Ferrovum spp.,Leptospirillum groups and A.ferrooxidans in different groups of microbial assemblages along the gradient of pH levels in AMD.The numbers within the parentheses indicate the number of samples in each group.Microbial diversity patterns in acid mine drainage J-L Kuang et al5The ISME Journalin microbial community composition,and pH appeared to be a strong predictor of relative lineage abundance with samples with low pH levels (pHo 2.3)clustering separately from those with moder-ate pH values.Betaproteobacteria ,the most abun-dant lineage (44±34%)across allcommunities,Figure 4Relative influence (%)of environmental properties and spatial distance for phylogenetic diversity (a ),phylotypes (b ),weighted UniFrac dissimilarity of field data (pyrosequecing)(c )and weighted UniFrac dissimilarity of metadata (meta-analysis)(d )evaluated by ABTmodels.Figure 5Multivariate regression tree analysis of the relation between relative abundance of dominant lineages and environmental parameters in microbial communities of AMD.The bar plots show the mean relative abundance of specific lineages at each terminal nodes and the distribution patterns of relative abundance represent the dynamics of community composition among each split.The numbers under the bar plots indicate the number (n )of samples within each group.All values are in mg l À1,except pH,which is in standard units.Microbial diversity patterns in acid mine drainageJ-L Kuang et al6The ISME Journalshowed an intense response to solution pH,with low relative abundance(4.2±9.1%,n¼15)in extremely acidic environments,but explicitly pre-dominant(48±32%,n¼44)in the microbial assem-blages under moderate pH conditions.Such a trend was largely attributed to the distribution pattern of ‘Ferrovum’-related organisms(Figure3).In contrast, other lineages such as Alphaproteobacteria, Euryarchaeota,Gammaproteobacteria and Nitros-pira exhibited a distinct adaptation to more acidic environments with an increase of relative abun-dance.These results were coincident with the overall dynamics of community composition along the pH gradient(Figure2).Similar patterns were found for other environmental variables in the MRT analysis,with groups uniquely dominated by Beta-proteobacteria generally separating from those with notable increase of relative abundance of other lineages(Figure5),implying that the optimal conditions for the growth of Betaproteobacteria (mainly‘Ferrovum’spp.)were apparently different from those for the other lineages.Global distribution patterns of microbial diversityin AMD and associated environmentsFrom the25molecular inventory studies that met our literature searching criteria,66samples with overall community composition were extracted for the taxon-based analysis in MRT model,and 45of them(which had detailed information of OTUs and their relative abundance)were retained for the phylogenetic-based analysis in ABT model(Supple-mentary Tables5a and b).It should be noted, however,that some parameters were missing from individual studies,but this problem could be over-come in the ABT and MRT model analyses as these models can deal with different types of response variables(for example,numeric or categorical)with missing values(De’ath,2002,2007).Overall,similar patterns of microbial composition were found in the global AMD and associated systems,with Proteobacteria,Nitrospira and Eur-yarchaeota as the major groups despite considerable fluctuations in their relative recovery in the16S rRNA gene libraries(Supplementary Table6).Most strikingly,pairwise UniFrac distances between the 45samples were still largely affected by environ-mental pH as revealed by the ABT model (Figure4d),implying that microbial assemblages from different substrates may have similar commu-nity composition under a similar pH condition. Furthermore,the geographical distance between the globally distributed samples(up to18000km)still had less influence to the community dissimilarity than pH,supporting environmental variation as the major factor relating microbial communities as observed in our pyrosequence data set.Likewise, the MRT analysis using the metadata of66samples indicated that microbial community composition was mainly shaped by pH level,with relatively little influence from spatial isolation(Figure6).However,in comparison with the significant contributionof Betaproteobacteria to the overall microbial distribution in the Chinese AMD environments,the global-scale pH-dependent pattern was largely attributed by the predominant distribution of Euryarchaeota and Nitrospira under relatively lowpH conditions(pH o1.9).DiscussionA comprehensive survey of AMD microbial diversityWe characterized the diversity and compositionof microbial communities from diverse and geogra-phically separated acidic mining environments in Southeast China.The large number of samples surveyed and the sequencing depth provided bythe bar-coded pyrosequencing generated an unpre-cedented number of AMD microbial16S rRNA gene sequence data that far exceed the total number of sequences reported in previous clone library stu-dies,significantly expanding our knowledge of thebroad trends of microbial distribution in extremelyacidic environments.Although most of the AMD communities have been sufficiently sampled by the pyrosequencing(as suggested by the rarefaction analyses;Supplementary Figure2),the full extentof microbial diversity in a few samples has not been captured.It is not likely that this is due to inflationof biodiversity estimate by sequencing errors gener-ated by noise introduced during pyrosequencingand the PCR amplification stage(Reeder and Knight, 2010),as such bias should have been limited afterour stringent denoizing of data.Similar results havebeen reported in other extreme habitats such as hydrothermal chimneys(Brazelton et al.,2010)andacidic hot spring(Bohorquez et al.,2012),where numerous rare taxa account for most of the observed diversity,implying that microbial diversity could behigher than expected in some specific sites with complex interactions among environmental vari-ables and microorganisms.Interestingly,althoughthe microbial diversity(Faith’s PD and Phylotypes) generally increased along the solution pH gradient,a moderately higher diversity and relatively uniform distribution pattern were found in the lowest pHlevel(pH o2.0;Figure2and SupplementaryTable7).This may be related to the significantlyhigher organic carbon contents in a few samples inthis pH group,as high carbon contents with heterogeneous resource condition have previouslybeen found to promote high species diversity insoil(Zhou et al.,2002)and marine sediments (Stach et al.,2003).Better prediction of microbial diversity patterns bysolution pHPrevious molecular investigations have documentedspatial and seasonal variations in microbial populations in specific AMD environments,andMicrobial diversity patterns in acid mine drainageJ-L Kuang et al7The ISME Journal。