在twitter中做文本挖掘进行股市预测的论文
基于大数据的股市预测模型研究

基于大数据的股市预测模型研究随着信息技术的不断发展,大数据时代已经来临。
大数据是指规模庞大、结构复杂的数据集合,其中包含了多种类型的数据,如社交媒体数据、搜索引擎数据、金融数据等。
大数据的处理和分析可以帮助我们更好地理解和应对各种社会经济问题。
在金融领域,大数据分析可以用于股市预测。
本文将探讨基于大数据的股市预测模型研究。
一、背景介绍股市的变化是市场需求供给关系变化的结果,受多种因素的影响。
过去,股市预测主要基于技术分析和基本面分析。
但是,随着数据的爆炸性增长,传统的分析方法逐渐显示出了其局限性。
而基于大数据的股市预测模型可以更准确地分析市场需求和供给之间的关系,并帮助投资者做出更明智的投资决策。
二、大数据在股市预测中的应用1.数据来源大数据的来源包括社交媒体、新闻与评论、搜索引擎、金融数据等。
其中,社交媒体数据是一个重要的数据来源,通过社交媒体数据的分析可以了解投资者的看法和观点,而这些看法和观点往往会影响股市的走势。
此外,新闻与评论、搜索引擎数据、金融数据也是股市预测的重要数据来源。
2.数据处理对于庞杂的大数据来说,数据的处理和分析是非常复杂的。
首先需要进行数据清洗,去除无用数据和错误数据。
然后需要进行数据采集,对数据进行分类和标签化。
分析大数据需要使用专业的数据挖掘和分析工具,常见的工具包括Hadoop、Spark和Python等。
利用这些工具,我们可以更准确和高效地对大数据进行处理和分析。
3.数据分析大数据分析的方法包括文本挖掘、聚类分析、情感分析、时间序列分析等。
其中,情感分析是一种比较重要的方法,能够分析出用户对于某个话题的情感及其强度,并进而分析市场情绪对于股市的影响。
时间序列分析则是分析历史数据等综合而来的数列模型。
利用这些方法,我们可以更准确地预测股市的走势。
三、基于大数据的股市预测模型案例1.Twitter情感分析Twitter情感分析是基于社交媒体数据的一种方法。
大多数人在推特上讨论自己的看法和观点,而这些观点和看法往往会对股市产生影响。
文本投资者情绪对股市波动影响与预测研究

文本投资者情绪对股市波动影响与预测研究文本投资者情绪对股市波动影响与预测研究引言:股市波动一直以来都是投资者密切关注的话题,其波动性不仅与市场供需关系有关,还受到投资者情绪的影响。
随着社交媒体和互联网的普及,越来越多的投资者将他们的观点和情绪通过文本表达出来,这为研究文本投资者情绪对股市波动的影响提供了新的机会。
本文将探讨文本投资者情绪对股市波动的影响以及可能的预测方法。
一、文本投资者情绪对股市波动的影响1.1 投资者情绪与股市波动的关系投资者情绪可以看作是投资者对市场的情感和情绪状态的反映。
在股市中,投资者情绪可能受到市场中的各种因素的影响,如市场信息、政治事件和经济数据等。
情绪的变化会导致投资者在买卖股票时产生不同的决策,从而影响股市的波动。
1.2 文本情绪与股市波动的关系文本情绪是指投资者通过文本表达出的情绪状态。
研究发现,文本情绪可以影响股市的波动。
例如,当投资者通过社交媒体发布大量负面文本情绪时,可能会引发市场恐慌情绪,导致股市大幅下跌。
相反,积极的文本情绪可能会提高市场信心,推动股市上涨。
1.3 文本投资者情绪对股市波动的影响机制文本情绪对股市波动的影响机制还存在诸多不确定性。
一方面,投资者情绪的传递可以通过文本媒体的传播实现。
当负面情绪被广泛传播时,其他投资者可能因此而产生恐慌性卖出,导致股市下跌。
另一方面,情绪也可能通过影响投资者的决策行为直接影响股市波动。
例如,当投资者情绪低落时,他们可能会更倾向于出售股票,从而推动股市下跌。
二、文本投资者情绪的预测方法2.1 文本情绪分析及情感词典法文本情绪分析是通过分析文本中的情感词汇来判断情绪状态。
研究者使用情感词典来识别并计算文本中的情感得分,从而预测投资者情绪对股市的影响。
例如,当文本中包含大量负面情感词汇时,可以预测到市场可能会出现下跌。
2.2 机器学习方法机器学习方法通过训练机器学习模型来预测文本情绪对股市的影响。
研究者使用历史数据将文本情绪作为输入,并根据相应的股市波动情况进行训练,通过模型来预测未来的股市波动。
基于文本挖掘和机器学习的股指预测与决策研究
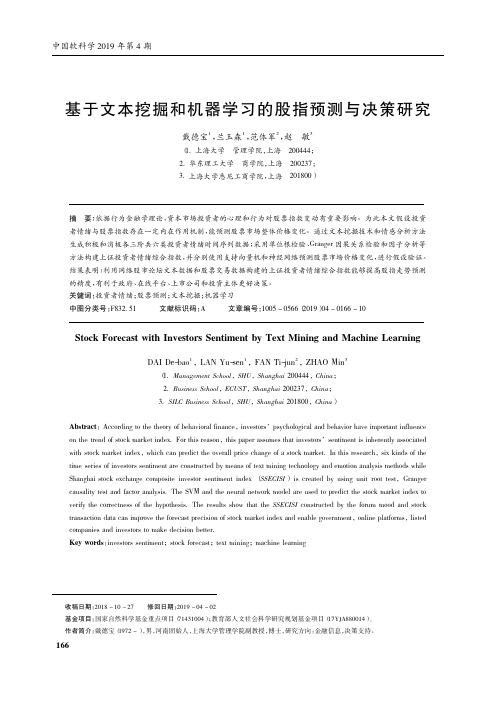
基于文本挖掘和机器学习的股指预测与决策研究戴德宝1,兰玉森1,范体军2,赵敏3(1.上海大学管理学院,上海200444;2.华东理工大学商学院,上海200237;3.上海大学悉尼工商学院,上海201800)摘要:依据行为金融学理论,资本市场投资者的心理和行为对股票指数变动有重要影响。
为此本文假设投资者情绪与股票指数存在一定内在作用机制,能预测股票市场整体价格变化。
通过文本挖掘技术和情感分析方法生成积极和消极各三阶共六类投资者情绪时间序列数据;采用单位根检验、Granger因果关系检验和因子分析等方法构建上证投资者情绪综合指数,并分别使用支持向量机和神经网络预测股票市场价格变化,进行假设验证。
结果表明:利用网络股市论坛文本数据和股票交易数据构建的上证投资者情绪综合指数能够提高股指走势预测的精度,有利于政府、在线平台、上市公司和投资主体更好决策。
关键词:投资者情绪;股票预测;文本挖掘;机器学习中图分类号:F832.51文献标识码:A文章编号:1005-0566(2019)04-0166-10Stock Forecast with Investors Sentiment by Text Mining and Machine LearningDAI De-bao1,LAN Yu-sen1,FAN Ti-jun2,ZHAO Min3(1.Management School,SHU,Shanghai200444,China;2.Business School,ECUST,Shanghai200237,China;3.SILC Business School,SHU,Shanghai201800,China)Abstract:According to the theory of behavioral finance,investors’psychological and behavior have important influence on the trend of stock market index.For this reason,this paper assumes that investors’sentiment is inherently associated with stock market index,which can predict the overall price change of a stock market.In this research,six kinds of the time series of investors sentiment are constructed by means of text mining technology and emotion analysis methods while Shanghai stock exchange composite investor sentiment index(SSECISI)is created by using unit root test,Granger causality test and factor analysis.The SVM and the neural network model are used to predict the stock market index to verify the correctness of the hypothesis.The results show that the SSECISI constructed by the forum mood and stock transaction data can improve the forecast precision of stock market index and enable government,online platforms,listed companies and investors to make decision better.Key words:investors sentiment;stock forecast;text mining;machine learning收稿日期:2018-10-27修回日期:2019-04-02基金项目:国家自然科学基金重点项目(71431004);教育部人文社会科学研究规划基金项目(17YJA880014)。
基于数据挖掘技术的股票预测研究
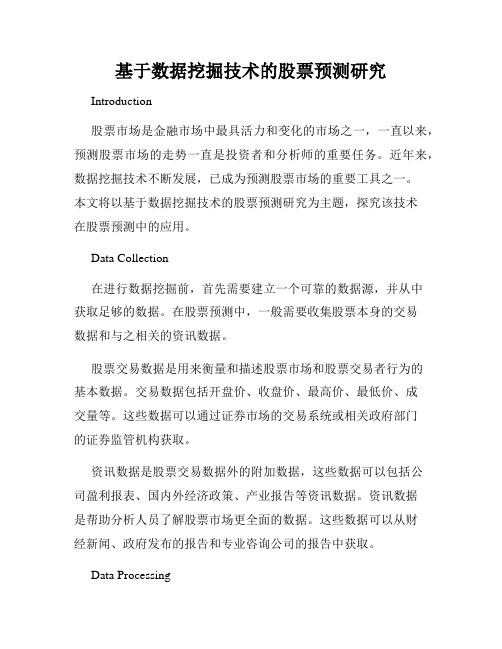
基于数据挖掘技术的股票预测研究Introduction股票市场是金融市场中最具活力和变化的市场之一,一直以来,预测股票市场的走势一直是投资者和分析师的重要任务。
近年来,数据挖掘技术不断发展,已成为预测股票市场的重要工具之一。
本文将以基于数据挖掘技术的股票预测研究为主题,探究该技术在股票预测中的应用。
Data Collection在进行数据挖掘前,首先需要建立一个可靠的数据源,并从中获取足够的数据。
在股票预测中,一般需要收集股票本身的交易数据和与之相关的资讯数据。
股票交易数据是用来衡量和描述股票市场和股票交易者行为的基本数据。
交易数据包括开盘价、收盘价、最高价、最低价、成交量等。
这些数据可以通过证券市场的交易系统或相关政府部门的证券监管机构获取。
资讯数据是股票交易数据外的附加数据,这些数据可以包括公司盈利报表、国内外经济政策、产业报告等资讯数据。
资讯数据是帮助分析人员了解股票市场更全面的数据。
这些数据可以从财经新闻、政府发布的报告和专业咨询公司的报告中获取。
Data Processing在获取到足够的有意义的数据之后,数据挖掘必须进行一些处理以使数据更具可操作性和指导性。
特征选择是数据挖掘的一个重要步骤,它决定着最终预测模型的质量。
股票预测的特征选择主要包括技术指标和基本面指标。
技术指标反映的是股票价格的变化,如移动平均、相对强弱指标等。
基本面指标是用来衡量公司基本面的指标,例如市盈率、市净率等。
在特征选择之后,需要对股票数据进行归一化处理。
数据归一化是指将不同单位或量级的数据转换到同一量级或尺度上,以消除不同数量级对模型预测的影响。
股票数据的归一化一般包括最大最小值归一化、正态分布归一化等。
Data Mining数据挖掘是利用计算机技术从大量不同角度的数据中,通过分析所隐藏的模式,得出有价值的信息的过程。
在股票预测中,数据挖掘涉及分析大量的交易和资讯数据,以识别隐藏在这些数据中的有用信息。
数据挖掘的方法包括回归分析、分类算法、聚类分析等等。
一种基于金融文本情感分析的股票指数预测新方法
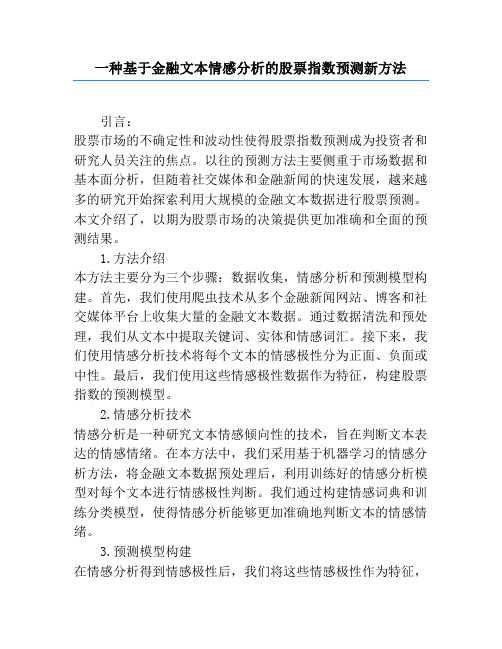
一种基于金融文本情感分析的股票指数预测新方法引言:股票市场的不确定性和波动性使得股票指数预测成为投资者和研究人员关注的焦点。
以往的预测方法主要侧重于市场数据和基本面分析,但随着社交媒体和金融新闻的快速发展,越来越多的研究开始探索利用大规模的金融文本数据进行股票预测。
本文介绍了,以期为股票市场的决策提供更加准确和全面的预测结果。
1.方法介绍本方法主要分为三个步骤:数据收集,情感分析和预测模型构建。
首先,我们使用爬虫技术从多个金融新闻网站、博客和社交媒体平台上收集大量的金融文本数据。
通过数据清洗和预处理,我们从文本中提取关键词、实体和情感词汇。
接下来,我们使用情感分析技术将每个文本的情感极性分为正面、负面或中性。
最后,我们使用这些情感极性数据作为特征,构建股票指数的预测模型。
2.情感分析技术情感分析是一种研究文本情感倾向性的技术,旨在判断文本表达的情感情绪。
在本方法中,我们采用基于机器学习的情感分析方法,将金融文本数据预处理后,利用训练好的情感分析模型对每个文本进行情感极性判断。
我们通过构建情感词典和训练分类模型,使得情感分析能够更加准确地判断文本的情感情绪。
3.预测模型构建在情感分析得到情感极性后,我们将这些情感极性作为特征,结合股票指数的历史数据,构建预测模型。
我们采用机器学习中的监督学习方法,如支持向量机(SVM)、随机森林(RF)等,对情感极性和历史数据进行训练。
通过模型训练,我们可以得到一个预测模型,用于预测未来股票指数的波动情况。
4.实验与结果分析为了验证本方法的有效性,我们对历史股票数据进行实验。
首先,我们收集了大量的金融文本数据,并进行了情感分析。
然后,我们利用得到的情感极性特征和历史股票数据,构建了预测模型。
最后,我们对模型进行了测试和验证,并与传统的股票指数预测方法进行了比较。
实验结果表明,本方法相对于传统方法在股票指数预测的准确性和稳定性方面取得了显著的提高。
5.结论与展望通过本文介绍的基于金融文本情感分析的股票指数预测新方法,我们可以利用金融文本数据提供的情感信息更加准确地预测股票市场的波动。
基于文本分析的股市预测研究

基于文本分析的股市预测研究Introduction在股票市场上,分析和预测是投资者最重要的工作之一。
文本分析是一种研究文本数据的技术,已经被广泛应用于金融领域中的预测和分析。
本文将探讨基于文本分析的股市预测方法和应用,从而提供一个更加准确和可靠的股市预测模型。
文本分析的基本原理文本分析是通过计算机技术对大量非结构化数据进行分析和提取有用信息的过程。
这些非结构化数据包括文本、图片、音频等。
对于金融领域来说,文本分析通常涉及对新闻、公告和财务报表等文本数据的分析,以便提取与股票价格相关的信息。
文本分析有两个主要的技术:自然语言处理和机器学习。
自然语言处理是一种处理自然语言的计算机科学、人工智能和语言学领域的交叉学科。
通过自然语言处理技术,计算机能够分析和理解人类的自然语言,从而处理文本数据。
机器学习是一种人工智能的方法,能够让计算机通过经验学习和训练提高性能。
文本分析在股票市场中的应用文本分析在股票市场中有多种应用,其中包括:1.新闻预测模型新闻预测模型是利用自然语言处理和机器学习技术,通过对大量新闻数据进行分析和处理,从而预测股票价格的走势。
这种方法能够分析新闻数据中的情感、主题和实体等信息,从而预测股票价格的方向和可能的涨跌幅度。
2.投资组合优化投资组合优化是一种文本分析技术,旨在通过分析公司的报表和新闻数据等非结构化数据,以优化资产组合并提高投资回报。
投资组合优化通常包括基于马科维茨理论的资产配置和根据使用最优投资策略的企业股票择时策略。
使用这种技术可以大大提高投资回报和风险管理能力。
3.情感分析情感分析是一种文本分析技术,旨在分析和预测人类的情感态度以及情感状态的变化。
这种技术可以应用于预测股票价格的变化。
4.舆情分析舆情分析是一种文本分析技术,旨在从社交媒体和其他在线渠道中获取消费者或投资者的情感态度和对公司产品或服务的看法。
这种方法可以帮助投资者做出更加明智的投资决策,并评估潜在的风险。
Twitter 能否预测股市

Twitter 能否预测股市?Lithium 发表于 2012-02-15今年 5 月,世界首家基于社交媒体的对冲基金 Derwent Capital Markets 在屡次跳票后 终于上线。
它会即时关注 Twitter 中的公众情绪指导投资。
正如基金创始人保罗•郝汀 (Paul Hawtin)表示:“长期以来,投资者已经广泛地认可金融市场由恐惧和贪婪驱使,但我们从 未拥有一种技术或数据来量化人们的情感。
”一直为金融市场非理性举动所困惑的投资者, 终于有了一扇可以了解心灵世界的窗户——那便是 Twitter 每天浩如烟海的推文[1]。
小试牛刀,成果斐然尽管针对公众情绪的调查耗时耗力, 仍有一些研究另辟蹊径, 揭示出情绪与股市关联中的冰 山一角。
对 39 个国家的调查表明,当在如世界杯、欧洲杯的国际球赛中输球时,当地的股 价指数会受到显著的负面影响[2]。
天气变化也会波及股市。
给一点阳光,股票收益率便会 和人们的心情一样灿烂[3]。
美国印第安纳大学的约翰•博伦(Johan Bollen)等人[4],则将目光投向了新兴的社交网 络 Twitter。
这个反映公众情绪的绝佳晴雨表,在预测电影票房、选举结果等方面,都有不 俗的表现。
研究者采用了两种情绪追踪工具。
一种是开源工具 OpinionFinder,能将推文 二分为正面和负面情绪; 另一种则是研究者在临床上使用的情绪状态量表 (POMS) 基础上, 新开发出的情绪测试工具 GPOMS。
它能将公众的情绪分为冷静、警惕、确信、活力、友善 和幸福这六个类别。
为了验证两个工具的准确性,研究者将公众情绪和社会事件对比,结果十分吻合。
例如,在 总统大选日(2008 年 11 月 4 日)期间,Twitter 在大选日前一天开始紧张,在大选日当天变得冷静、活力、友善、幸福,总体情绪在大选日后又回归平常。
在感恩节(11 月 28 日)当天,整个 Twitter 洋溢着浓浓的幸福味道,过后又恢复正常。
金融领域中基于文本挖掘的事件预测与风险分析

金融领域中基于文本挖掘的事件预测与风险分析Chapter 1: Introduction to Event Prediction and Risk Analysis in the Financial SectorThe financial sector is a dynamic and complex industry that relies on accurate predictions and risk analysis to make informed decisions. One tool that has gained prominence in recent years is text mining, a technique that allows for the extraction of valuable insights from large volumes of unstructured textual data. In this article, we will explore the application of text mining in event prediction and risk analysis within the financial sector.Chapter 2: The Role of Text Mining in Event PredictionText mining involves extracting relevant information from a variety of texts, such as news articles, social media posts, and financial reports. In event prediction, text mining can be used to identify and analyze patterns and trends that may indicate future events or market movements. For example, by analyzing news articles and social media sentiment, text mining algorithms can predict shifts in public opinion that might impact stock prices.Chapter 3: Text Mining Techniques for Event PredictionThere are several text mining techniques that can be employed for event prediction in the financial sector. One popular approach issentiment analysis, which involves analyzing the sentiment expressedin textual data to predict market movements. Another technique is topic modeling, which can categorize news articles or social media posts into different topics and identify trends within each topic. These techniques can aid in predicting events such as market crashes, mergers and acquisitions, or regulatory changes.Chapter 4: Case Study: Event Prediction in Stock MarketTo understand the practical application of text mining in event prediction, let us consider the stock market. By analyzing news articles and social media posts related to specific stocks or companies, text mining algorithms can identify key events or sentiments that may affect stock prices. For instance, if there is a sudden surge in negative sentiment regarding a particular stock, it could indicate a potential decline in its value. Predictive models can also be built based on historical data to forecast future market movements.Chapter 5: Risk Analysis Using Text MiningText mining can also be used for risk analysis in the financial sector. By aggregating and analyzing news articles and financial reports, text mining algorithms can identify potential risks or vulnerabilities within a company or industry. This can include identifying fraud, assessing credit risk, or monitoring compliance with regulations. By identifying risks early on, companies can take proactive measures to mitigate potential losses and minimize their exposure to risk.Chapter 6: Case Study: Fraud DetectionFraud is a significant risk in the financial sector, costing companies billions of dollars each year. Text mining techniques can play a vital role in fraud detection by identifying patterns or anomalies in textual data that might indicate fraudulent activities. By analyzing financial transactions, customer complaints, or employee communications, text mining algorithms can flag suspicious activities, helping companies detect and prevent fraudulent behavior.Chapter 7: Limitations and ChallengesWhile text mining offers valuable insights for event prediction and risk analysis, there are limitations and challenges that should be considered. One limitation is the inherent subjectivity of textual data, as the same piece of information can be interpreted differently by different individuals. Additionally, there may be biases in the data, such as the underrepresentation of certain demographics or sources. Furthermore, the sheer volume of textual data can pose challenges in terms of computational power and storage.Chapter 8: ConclusionIn conclusion, text mining is a powerful tool for event prediction and risk analysis in the financial sector. By leveraging valuable insights from unstructured textual data, companies can gain a competitive advantage in making informed decisions and mitigating risks. However, it is important to recognize the limitations and challenges associatedwith text mining and continually refine and improve the techniques used to ensure accurate predictions and robust risk analysis in the financial industry.。
- 1、下载文档前请自行甄别文档内容的完整性,平台不提供额外的编辑、内容补充、找答案等附加服务。
- 2、"仅部分预览"的文档,不可在线预览部分如存在完整性等问题,可反馈申请退款(可完整预览的文档不适用该条件!)。
- 3、如文档侵犯您的权益,请联系客服反馈,我们会尽快为您处理(人工客服工作时间:9:00-18:30)。
1Twitter mood predicts the stock market.Johan Bollen 1, ,Huina Mao 1, ,Xiao-Jun Zeng 2.:authors made equal contributions.Abstract —Behavioral economics tells us that emotions can profoundly affect individual behavior and decision-making.Does this also apply to societies at large,i.e.can societies experience mood states that affect their collective decision making?By extension is the public mood correlated or even predictive of economic indicators?Here we investigate whether measurements of collective mood states derived from large-scale Twitter feeds are correlated to the value of the Dow Jones Industrial Average (DJIA)over time.We analyze the text content of daily Twitter feeds by two mood tracking tools,namely OpinionFinder that measures positive vs.negative mood and Google-Profile of Mood States (GPOMS)that measures mood in terms of 6dimensions (Calm,Alert,Sure,Vital,Kind,and Happy).We cross-validate the resulting mood time series by comparing their ability to detect the public’s response to the presidential election and Thanksgiving day in 2008.A Granger causality analysis and a Self-Organizing Fuzzy Neural Network are then used to investigate the hypothesis that public mood states,as measured by the OpinionFinder and GPOMS mood time series,are predictive of changes in DJIA closing values.Our results indicate that the accuracy of DJIA predictions can be significantly improved by the inclusion of specific public mood dimensions but not others.We find an accuracy of 87.6%in predicting the daily up and down changes in the closing values of the DJIA and a reduction of the Mean Average Percentage Error by more than 6%.Index Terms —stock market prediction —twitter —mood analysis.I.I NTRODUCTIONSTOCK market prediction has attracted much attention from academia as well as business.But can the stock market really be predicted?Early research on stock market prediction [1],[2],[3]was based on random walk theory and the Efficient Market Hypothesis (EMH)[4].According to the EMH stock market prices are largely driven by new information,i.e.news,rather than present and past prices.Since news is unpredictable,stock market prices will follow a random walk pattern and cannot be predicted with more than 50percent accuracy [5].There are two problems with EMH.First,numerous studies show that stock market prices do not follow a random walk and can indeed to some degree be predicted [5],[6],[7],[8]thereby calling into question EMH’s basic assumptions.Sec-ond,recent research suggests that news may be unpredictable but that very early indicators can be extracted from online social media (blogs,Twitter feeds,etc)to predict changes in various economic and commercial indicators.This may conceivably also be the case for the stock market.For example,[11]shows how online chat activity predicts book sales.[12]uses assessments of blog sentiment to predict movie sales.[15]predict future product sales using a Probabilistic Latent Semantic Analysis (PLSA)model to extract indicators ofsentiment from blogs.In addition,Google search queries have been shown to provide early indicators of disease infection rates and consumer spending [14].[9]investigates the relations between breaking financial news and stock price changes.Most recently [13]provide a ground-breaking demonstration of how public sentiment related to movies,as expressed on Twitter,can actually predict box office receipts.Although news most certainly influences stock market prices,public mood states or sentiment may play an equally important role.We know from psychological research that emotions,in addition to information,play an significant role in human decision-making [16],[18],[39].Behavioral finance has provided further proof that financial decisions are sig-nificantly driven by emotion and mood [19].It is therefore reasonable to assume that the public mood and sentiment can drive stock market values as much as news.This is supported by recent research by [10]who extract an indicator of public anxiety from LiveJournal posts and investigate whether its variations can predict S&P500values.However,if it is our goal to study how public mood influences the stock markets,we need reliable,scalable and early assessments of the public mood at a time-scale and resolution appropriate for practical stock market rge surveys of public mood over representative samples of the population are generally expensive and time-consuming to conduct,cf.Gallup’s opinion polls and various consumer and well-being indices.Some have therefore proposed indirect assessment of public mood or sentiment from the results of soccer games [20]and from weather conditions [21].The accuracy of these methods is however limited by the low degree to which the chosen indicators are expected to be correlated with public mood.Over the past 5years significant progress has been made in sentiment tracking techniques that extract indicators of public mood directly from social media content such as blog content [10],[12],[15],[17]and in particular large-scale Twitter feeds [22].Although each so-called tweet ,i.e.an individual user post,is limited to only 140characters,the aggregate of millions of tweets submitted to Twitter at any given time may provide an accurate representation of public mood and sentiment.This has led to the development of real-time sentiment-tracking indicators such as [17]and “Pulse of Nation”1.In this paper we investigate whether public sentiment,as expressed in large-scale collections of daily Twitter posts,can be used to predict the stock market.We use two tools to measure variations in the public mood from tweets submitted1/home/amislove/twittermood/a r X i v :1010.3003v 1 [c s .C E ] 14 O c t 2010to the Twitter service from February28,2008to December19, 2008.Thefirst tool,OpinionFinder,analyses the text content of tweets submitted on a given day to provide a positive vs.negative daily time series of public mood.The second tool,GPOMS,similarly analyses the text content of tweets to generate a six-dimensional daily time series of public mood to provide a more detailed view of changes in public alonga variety of different mood dimensions.The resulting public mood time series are correlated to the Dow Jones Industrial Average(DJIA)to assess their ability to predict changes in the DJIA over time.Our results indicate that the prediction accuracy of standard stock market prediction models is signif-icantly improved when certain mood dimensions are included, but not others.In particular variations along the public mood dimensions of Calm and Happiness as measured by GPOMS seem to have a predictive effect,but not general happiness as measured by the OpinionFinder tool.II.R ESULTSA.Data and methods overviewWe obtained a collection of public tweets that was recorded from February28to December19th,2008(9,853,498tweets posted by approximately2.7M users).For each tweet these records provide a tweet identifier,the date-time of the sub-mission(GMT+0),its submission type,and the text content of the Tweet which is by design limited to140characters. After removal of stop-words and punctuation,we group all tweets that were submitted on the same date.We only take into account tweets that contain explicit statements of their author’s mood states,i.e.those that match the expressions“i feel”,“i am feeling”,“i’m feeling”,“i dont feel”,“I’m”,“Im”,“I am”,and“makes me”.In order to avoid spam messages and other information-oriented tweets,we alsofilter out tweets that match the regular expressions”http:”or”www.”As shown in Fig.1we then proceed in three phases.In the first phase,we subject the collections of daily tweets to2mood assessment tools:(1)OpinionFinder which measures positive vs.negative mood from text content,and(2)GPOMS which measures6different mood dimensions from text content. This results in a total of7public mood time series,one generated by OpinionFinder and six generated by GPOMS, each representing a potentially different aspect of the public’s mood on a given day.In addition,we extract a time series of daily DJIA closing-values from Yahoo!Finance.In the second phase,we investigate the hypothesis that public mood as measured by GPOMS and OpinionFinder is predictive of future DJIA values.We use a Granger causality analysis in which we correlate DJIA values to GPOMs and OF values of the past n days.In the third phase,we deploy a Self-Organizing Fuzzy Neural Network model to test the hypothesis that the prediction accuracy of DJIA prediction models can be improved by including measurements of public mood.We are not interested in proposing an optimal DJIA prediction model, but to assess the effects of including public mood information on the accuracy of a“baseline”prediction model.Stock market (daily)t-1t-2t-3t=0value(2) Granger Causality analysisbetween DJIA,OpinionFinder and GPOMS public mood from August2008to December2008,and(3)training of a Self-Organizing Fuzzy Neural Network to predict DJIA values on the basis of various combinations of past DJIA values and OF and GPOMS public mood data from March2008to December 2008.B.Generating public mood time series:OpinionFinder and GPOMSOpinionFinder(OF)2is a publicly available software pack-age for sentiment analysis that can be applied to determine sentence-level subjectivity[25],i.e.to identify the emo-tional polarity(positive or negative)of sentences.It has been successfully used to analyze the emotional content of large collections of tweets[26]by using the OF lexicon to determine the ratio of positive versus negative tweets on a given day.The resulting time series were shown to correlate with the Consumer Confidence Index from Gallup3and the Reuters/University of Michigan Surveys of Consumers4over a given period of time.We adopt OF’s subjective lexicon that has been established upon previous work[37],[38],[24].We select positive and negative words that are marked as either “weak”and“strong”from the OF sentiment lexicon resulting in a list of2718positive and4912negative words.For each tweet we determine whether it contains any number of negative and positive terms from the OF lexicon.For each occurrence we increase the score of either negative or positive tweets by 1and calculate the ratio of positive vs.negative messages for the tweets posted on the same day t.Like many sentiment analysis tools OF adheres to a unidi-mensional model of mood,making binary distinctions between positive and negative sentiment[23].This may however ignore the rich,multi-dimensional structure of human mood.To capture additional dimensions of public mood we created a second mood analysis tools,labeled GPOMS,that can measure human mood states in terms of6different mood dimensions, namely Calm,Alert,Sure,Vital,Kind and Happy.GPOMS’2/mpqa/opinionfinderrelease/3/poll/122840/Gallup-Daily-Economic-Indexes.aspx 4/3mood dimensions and lexicon are derived from an existing and well-vetted psychometric instrument,namely the Profile of Mood States (POMS-bi)[32],[33].To make it applicable to Twitter mood analysis we expanded the original 72terms of the POMS questionnaire to a lexicon of 964associated terms by analyzing word co-occurrences in a collection of 2.5billion 4-and 5-grams 5computed by Google in 2006from approximately 1trillion word tokens observed in publicly accessible Webpages [35],[36].The enlarged lexicon of 964terms thus allows GPOMS to capture a much wider variety of naturally occurring mood terms in Tweets and map them to their respective POMS mood dimensions.We match the terms used in each tweet against this lexicon.Each tweet term that The GPOMS results reveal a more differentiated public mood response to the events in the three-day period surround-ing the election day (November 4,2008).November 3,2008is5n-gramsare frequently occurring sequences of terms in text of length n ,for example “we are the robots”could be a frequent 4-gram.6/datacharacterized by a significant drop in Calm indicating highly elevated levels of public anxiety.Election Day itself is char-acterized by a reversal of Calm scores indicating a significant reduction in public anxiety,in conjunction with a significant increases of Vital,Happy as well as Kind scores.The latter indicates a public that is energized,happy and friendly on election day.On November 5,these GPOMS dimensions con-tinue to indicate positive mood levels,in particular high levels of Calm,Sure,Vital and Happy.After November 5,all mood dimensions gradually return to the baseline.The public mood response to Thanksgiving on November 27,2008provides a counterpart to the differentiated response to the Presidential election.On Thanksgiving day we find a spike in Happy regression. 2.Y OF =α+n iβi X i + t (2)where X 1,X 2,X 3,X 4,X 5and X 6represent the mood timeseries obtained from the 6GPOMS dimensions,respectively4TABLE IM ULTIPLE R EGRESSION R ESULTS FOR O PINION F INDER VS .6GPOMSMOOD DIMENSIONS .Parameters Coeff.Std.Err.t p Calm (X 1) 1.731 1.348 1.2840.20460Alert (X 2)0.199 2.3190.0860.932Sure (X 3) 3.8970.613 6.356 4.25e-08 Vital (X 4) 1.7630.595 2.9650.004 Kind (X 5) 1.687 1.377 1.2260.226Happy (X 6) 2.7700.578 4.790 1.30e-05 SummaryResidual Std.Err Adj.R 2F 6,55p 0.0780.68322.93 2.382e-13(p-value <0.001: ,p-value <0.05: ,p-value <0.1: )Calm,Alert,Sure,Vital,Kind and Happy.The multiple linear regression results are provided in Table I (coefficient andp-values),and indicate that Y OF is significantly correlated with X 3(Sure),X 4(Vital)and X 6(Happy),but not with X 1(Calm),X 2(Alert)and X 5(Kind).We therefore conclude that certain GPOMS mood dimension partially overlap with the mood values provided by OpinionFinder,but not necessarily all mood dimensions that may be important in describing the various components of GPOMS and OpinionFinderWe perform the Granger causality analysis according to model L 1and L 2shown in Eq.3and 4for the period of time between February 28to November 3,2008to exclude the exceptional public mood response to the Presidential Election and Thanksgiving from the comparison.GPOMS and OpinionFinder time series were produced for 342,255tweets in that period,and the daily Dow Jones Industrial Average (DJIA)was retrieved from Yahoo!Finance for each day 8.L 1:D t =α+n i =1βi D t −i + t(3)L 2:D t =α+n i =1βi D t −i +n i =1γi X t −i + t (4)Based on the results of our Granger causality (shown in Table II),we can reject the null hypothesis that the mood time series do not predict DJIA values,i.e.β{1,2,···,n }=0with a high level of confidence.However,this result only applies to 1GPOMS mood dimension.We observe that X 1(i.e.Calm)has the highest Granger causality relation with DJIA for lags the gaps.This results in a time series of 64days.5TABLE IIS TATISTICAL SIGNIFICANCE (P -VALUES )OF BIVARIATE G RANGER -CAUSALITY CORRELATION BETWEEN MOODS AND DJIA IN PERIOD F EBRUARY 28,2008TO N OVEMBER 3,2008.Lag OF Calm Alert Sure Vital Kind Happy 1day 0.085 0.2720.9520.6480.1200.8480.3882days 0.2680.013 0.9730.8110.3690.9910.70613days 0.4360.022 0.9810.3490.4180.9910.7234days 0.2180.030 0.9980.4150.4750.9890.7505days 0.3000.036 0.9890.5440.5530.9960.1736days 0.4460.065 0.9960.6910.6820.9940.081 7days 0.6200.1570.9990.3810.7130.9990.150(p-value<0.05: ,p-value <0.1: )0.The Calm mood dimension thus has predictive value with regards to the DJIA.In fact the p-value for this shorter period,i.e.August 1,2008to October 302008,issignificantly lower (lag n =3,p =0.009)than that listed in Table II for the period February 28,2008to November 3,2008.The cases in which the t −3mood time series fails to track changes in the DJIA are nearly equally informative as where it doesn’t.In particular we point to a significant deviation between the two graphs on October 13th where the DJIA surges by more than 3standard deviations trough-to-peak.The Calm curve however remains relatively flat at that time after which it starts to again track changes in the DJIA again.This discrepancy may be the result of the the Federal Reserve’s announcement on October 13th of a major bank bailout initiative which unexpectedly increase DJIA values that day.The deviation between Calm values and the DJIA on that day illustrates that unexpected news is not anticipated by the public mood yet remains a significant factor in modeling the stock E.Non-linear models for emotion-based stock prediction Our Granger causality analysis suggests a predictive re-lation between certain mood dimensions and DJIA.How-ever,Granger causality analysis is based on linear regression whereas the relation between public mood and stock market values is almost certainly non-linear.To better address these non-linear effects and assess the contribution that public mood assessments can make in predictive models of DJIA values,we compare the performance of a Self-organizing Fuzzy Neural Network (SOFNN)model [30]that predicts DJIA values on the basis of two sets of inputs:(1)the past 3days of DJIA values,and (2)the same combined with various permutations of our mood time series (explained below).Statistically signif-icant performance differences will allow us to either confirm or reject the null hypothesis that public mood measurement do not improve predictive models of DJIA values.We use a SOFNN as our prediction model since they have previously been used to decode nonlinear time series data which describe the characteristics of the stock market [28]and predict its values [29].Our SOFNN in particular is a five-layer hybrid neural network with the ability to self-organize its own neurons in the learning process.A similar organization has been successfully used for electricial load forecasting in our previous work [31].To predict the DJIA value on day t ,the input attributes of our SOFNN include combinations of DJIA values andmood values of the past n days.We choose n =3since the results shown in Table II indicate that past n =4the Granger causal relation between Calm and DJIA decreases significantly.All historical load values are linearly scaled to [0,1].This causes every input be with similar importance since they are processed within a uniform range.SOFNN models require the tuning of a number of pa-rameters that can influence the performance of the model.We maintain the same parameter values across our vari-ous input combinations to allow an unbiased comparison of model performance,namely δ=0.04,σ=0.01,k rmse =0.05,k d (i ),(i =1,...,r )=0.1where r is the dimension of input variables and k rmse is the expected training root mean squared error which is a predefined value.To properly evaluate the SOFNN model’s ability to predict daily DJIA prices,we extend the period under consideration to February 28,2008to December 19,2008for training and testing.February 28,2008to November 28,2008is chosen as the longest possible training period while Dec 1to Dec 19,2008was chosen as the test period because it was characterized by stabilization of DJIA values after considerable volatility in previous months and the absence of any unusual or significant socio-cultural events.Fig.4shows that the Fall of 2008is an unusual period for the DJIA due to a sudden dramatic decline of stock prices.This variability may in fact render stock market prediction more difficult than in other periods.DJIA daily closing value (March 2008−December 2008MarAprMayJunJulAugSepOctNovDec 20088000900010000110001200013000Fig.4.Daily Dow Jones Industrial Average values between February 28,2008and December 19,2008.The Granger causality analysis indicates that only Calm (and to some degree Happy)is Granger-causative of DJIA values.However,the other mood dimensions still con-tain predictive information of DJIA values when combined6with other mood dimensions.For example,Happy may not be independently linearly related with DJIA,but it may nevertheless improve the SOFNN prediction accuracy when combined with Calm.To clarify these questions,we investigate seven permutations of input variables to the SOFNN model, thefirst of which,denoted I0,represents a naive,baseline model that has been trained to predict DJIA values at time t from the historical values at time{t−1,t−2,t−3}: I0={DJIA t−3,2,1}I1={DJIA t−3,2,1,X1,t−3,2,1}I1,2={DJIA t−3,2,1,X1,t−3,2,1,X2,t−3,2,1}I1,3={DJIA t−3,2,1,X1,t−3,2,1,X3,t−3,2,1}···DJIA t−3,2,1represents the DJIA values and X1,t−3,2,1 represents the values of the GPOMS mood dimension1,at time t−3,t−2,and t−1.According to the same notation I1,3,I1,4,I1,5,I1,6represent a combination of historical DJIA with mood dimensions3,4,5and6at time t−3,t−2,and t−1.For example,I1,6represents a set of inputs that includes the DJIA values t−3,t−2,and t−1,and mood dimensions 1and6at the same times.In order to compare the performance of the GPOMS mood data with the positive/negative sentiment values given by OpinionFinder,we additionally define the input combination:I OF={DJIA t−3,2,1,X OF,t−3,2,1} Forecasting accuracy is measured in terms of the average Mean Absolute Percentage Error(MAPE)and the direction accuracy(up or down)during the test period(December1 to December19,2008).The prediction results are shown in Table III.We can draw several conclusions from these results.First, adding positive/negative sentiment obtained from OF(I OF) has no effect on prediction accuracy compared to using only historical DJIA values(I0).This confirms the results of our Granger causuality analysis.Second,adding Calm,i.e.input I1,wefind the highest prediction pared to I0and all other input com-binations,adding input I1leads to significant improvements in MAPE values(1.83%vs.the maximum of2.13%and 1.95%for I OF)and direction accuracy(86.7%compared to 73.3%for I OF and46.7%for I1,3).Thirdly,I1,3and I1,4 actually reduce prediction accuracy significantly both in terms of MAPE and direction%,most likely because X3(Sure) and X4(Vital)do not contain information that is useful for prediction of DJIA values causing prediction accuracy to regress to chance levels.It is notable that I1,6,i.e.a combination of X6and X1does significantly reduce average MAPE,and provides good direction accuracy(80%).This is surprising since X6(i.e.Happy)does not have a good Granger causality relation with DJIA at a lag of n=3days(see Table II,p=0.723).However in combination with Calm,it produces a more accurate SOFNN prediction(MAPE=1.79%) and direction accuracy(80%).To assess the statistical significance of the SOFNN achiev-ing the above accuracy of87.6%in predicting the up and down movement of the DJIA we calculate the odds of this result occurring by chance.The binomial distribution in-dicates that the probability of exactly87.6%correct guesses over15trials(20days minus weekends)with a50% chance of success on each single trial equals0.32%.Taken over the entire length of our data set(February28to December 20,excluding weekends)wefind approximately10.9of such 20day periods.The odds that the mentioned probability would hold by chance for a random period of20days within that period is then estimated to be1−(1−0.0032)10.9=0.0343or 3.4%.The SOFNN direction accuracy is thus most likely not the result of chance nor our selecting a specifically favorable test period.In addition,we test the linear effect of both Calm(X1)and Happy(X6)on DJIA,with a nested F−test between the full model F and reduced model R shown as follows:F:D t=α+ni=1βi D t−i+3i=1γi X1,t−i+3i=1γi X6,t−i+ tR:D t=α+ni=1βi D t−i+3i=1γi X1,t−i+ tWefind a p-value of0.66and an F-statistic of0.53 indicating that a linear combination of X1and X6produces worse results than X1alone.Since the SOFNN prediction is more accurate when using a combination of X1and X6,we conclude that this confirms a nonlinear relation among the different dimensions of moods.III.D ISCUSSIONIn this paper,we investigate whether public mood as measured from large-scale collection of tweets posted on is correlated or even predictive of DJIA values. Our results show that changes in the public mood state can indeed be tracked from the content of large-scale Twitter feeds by means of rather simple text processing techniques and that such changes respond to a variety of socio-cultural drivers in a highly differentiated manner.Among the7observed mood dimensions only some are Granger causative of the DJIA; changes of the public mood along these mood dimensions match shifts in the DJIA values that occur3to4days later. Surprisingly we do not observe this effect for OpinionFinder’s assessment of public mood states in terms of positive vs.neg-ative mood but rather for the GPOMS dimension labeled “Calm”.The calmness of the public(measured by GPOMS) is thus predictive of the DJIA rather than general levels of positive sentiment as measured by OpinionFinder.A Self-Organizing Fuzzy Neural Network trained on the basis of past DJIA values and our public mood time series furthermore demonstrated the ability of the latter to significantly improve the accuracy of even the most basic models to predict DJIA closing values.Given the performance increase for a relatively basic model such as the SOFNN we are hopeful tofind equal or better improvements for more sophisticated market models that may in fact include other information derived from newsTABLE IIIDJIA D AILY P REDICTION U SING SOFNNEvaluation I OF I0I1I1,2I1,3I1,4I1,5I1,6 MAPE(%) 1.95 1.94 1.83 2.03 2.13 2.05 1.851.79 Direction(%)73.373.386.7 60.046.760.073.380.0sources,and a variety of relevant economic indicators.These results have implications for existing sentiment tracking tools as well as surveys of“self-reported subjective well-being”in which individuals evaluate the extent to which they experience positive and negative affect,happiness,or satisfaction with life[40].Such surveys are relatively expensive and time-consuming,and may nevertheless not allow the measurement of public mood along mood dimensions that are relevant to assess particular socio-economic indicators.Public mood analysis from Twitter feeds on the other hand offers an automatic,fast,free and large-scale addition to this toolkit that may in addition be optimized to measure a variety of dimensions of the public mood state.Our analysis does not acknowledge a number of important factors that will form the basis of future research.First,we note that our analysis is not designed to be limited to any particular geographical location nor subset of the world’s population.This approach may be appropriate since the US stock markets are affected by individuals worldwide,but for the particular period under observation users were de facto predominantly English speaking and located in the US.As ’s user base becomes increasingly interna-tional and the use of smartphones equipped with geo-location increases,future analysis will have to factor in location and language to avoid geographical and cultural sampling errors. Second,although we have cross-validated the results of2 different tools to assess public mood states,we have no knowledge of the“ground truth”for public mood states nor in fact for the particular subsample of the population represented by the community of users.This problem can only be addressed by increased research into direct assessments of public mood states vs.those derived from online communities such as Twitter.Third,these results are strongly indicative of a predictive correlation between measurements of the public mood states from Twitter feeds,but offer no information on the causative mechanisms that may connect public mood states with DJIA values in this manner.One could speculate that the general public is presently as strongly invested in the DJIA asfinancial experts,and that therefore their mood states will directly affect their investment decisions and thus stock market values,but this too remains an area of future research.A CKNOWLEDGMENTThis research was supported by NSF Grant BCS#1032101. We thank David Crandell and Michael Nelson for their helpful comments on earlier versions of this manuscript.We are also grateful to Daniel Kahneman for his early comments on our work.R EFERENCES[1]Fama,Eugene F,e.a.(1969)International Economic Review10,1–21.[2]Fama,E.F.(1991)Journal of Finance46,1575–617.[3]H.Cootner,P.(1964)The random character of stock market prices.(MIT).[4]Fama,E.F.(1965)The Journal of Business38,34–105.[5]Qian,Bo,Rasheed,&Khaled.(2007)Applied Intelligence26,25–33.[6]Gallagher,L.A&Taylor,M.P.(2002)Southern Economic Journal69,345–362.[7]Kavussanos,M&Dockery,E.(2001)Applied Financial Economics11,573–79.[8]Butler,K.C&Malaikah,S.J.(1992)Journal of Banking and Finance16,197–210.[9]Schumaker,R.P&Chen,H.(2009)ACM Trans.Inf.Syst.27,12:1–12:19.[10]Gilbert,E&Karahalios,K.(2010)Widespread worry and the stockmarket.[11]Gruhl,D,Guha,R,Kumar,R,Novak,J,&Tomkins,A.(2005)Thepredictive power of online chatter.(ACM,New York,NY,USA),pp.78–87.[12]Mishne,G&Glance,N.(2006)Predicting Movie Sales from BloggerSentiment.AAAI2006Spring Symposium on Computational Ap-proaches to Analysing Weblogs[13]S.Asur and B.A.Huberman2010Predicting the Future with SocialMedia arXiv:1003.5699v1[14]Choi,H&Varian,H.(2009)Predicting the present with google trends.,(Google),Technical report.[15]Liu,Y,Huang,X,An,A,&Yu,X.(2007)ARSA:a sentiment-awaremodel for predicting sales performance using blogs.(ACM,New York, NY,USA),pp.607–614.[16]Dolan,R.J.(2002)Science298,1191–1194.[17]Dodds,Peter.(2009)Journal of Happiness July,doi:10.1007/s10902-009-9150-9[18]Damasio,A.R.(1994)Descartes’error:emotion,reason,and thehuman brain.(Putnam),pp.xix,312p.+.[19]Nofsinger,J.(2005)Journal of Behaviour Finance.6,144–160.[20]Edmans,A,Garca,D,&Norli,.(2007)Journal of Finance62,1967–1998.[21]Hirshleifer,D&Shumway,T.(2003)Journal of Finance58,1009–1032.[22]Pak,A&Paroubek,P.(2010)Twitter as a Corpus for Sentiment Analysisand Opinion Mining.(European Language Resources Association (ELRA),Valletta,Malta).[23]Pang,B&Lee,L.(2008)Foundations and Trends in InformationRetrieval2,1–135.[24]Wilson,T,Wiebe,J,&Hoffmann,P.(2005)Recognizing ContextualPolarity in Phrase-Level Sentiment Analysis.(Vancouver,CA). [25]Wilson,T,Hoffmann,P,Somasundaran,S,Kessler,J,Wiebe,J,Choi,Y,Cardie,C,Riloff,E,&Patwardhan,S.(2005)OpinionFinder:A system for subjectivity analysis.pp.34–35.[26]O’Connor,B,Balasubramanyan,R,Routledge,B.R,&Smith,N.A.(2010)From Tweets to Polls:Linking Text Sentiment to Public Opinion Time Series.[27]Diener E,Diener M&Diener D(2009)Factors Predicting the SubjectiveWell-Being of Nations.Social Indicators Research Series38:43-70 [28]Lapedes,A&Farber,R.(1987)Nonlinear signal processing using neuralnetwork:Prediction and system modeling,(Los Alamos National Lab Technical Report),Technical report.[29]Zhu,X,Wang,H,Xu,L,&Li,H.(2008)Expert Syst.Appl.34,3043–3054.[30]Leng,G,Prasad,G,&McGinnity,T.M.(2004)Neural Netw.17,1477–1493.[31]Mao,H,Zeng,X.-J,Leng,G,Zhai,Y,&Keane,A.J.(2009)IEEETransaction on Power System.24,1080–1090.[32]Norcross,J.C,Guadagnoli,E,&Prochaska,J.O.(2006)Journal ofClinical Psychology40,1270–1277.[33]McNair,D,Heuchert,J.P,&Shilony,E.(2003)Profile of mood states.Bibliography1964–2002.(Multi-Health Systems).[34]Pepe,A&Bollen,J.(2008)Between conjecture and memento:shapinga collective emotional perception of the future.。