Maximum-margin matrix factorization
机器学习专业词汇中英文对照
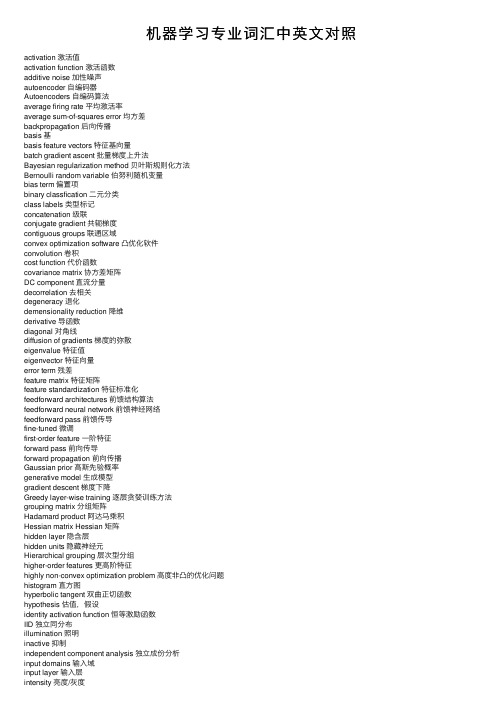
机器学习专业词汇中英⽂对照activation 激活值activation function 激活函数additive noise 加性噪声autoencoder ⾃编码器Autoencoders ⾃编码算法average firing rate 平均激活率average sum-of-squares error 均⽅差backpropagation 后向传播basis 基basis feature vectors 特征基向量batch gradient ascent 批量梯度上升法Bayesian regularization method 贝叶斯规则化⽅法Bernoulli random variable 伯努利随机变量bias term 偏置项binary classfication ⼆元分类class labels 类型标记concatenation 级联conjugate gradient 共轭梯度contiguous groups 联通区域convex optimization software 凸优化软件convolution 卷积cost function 代价函数covariance matrix 协⽅差矩阵DC component 直流分量decorrelation 去相关degeneracy 退化demensionality reduction 降维derivative 导函数diagonal 对⾓线diffusion of gradients 梯度的弥散eigenvalue 特征值eigenvector 特征向量error term 残差feature matrix 特征矩阵feature standardization 特征标准化feedforward architectures 前馈结构算法feedforward neural network 前馈神经⽹络feedforward pass 前馈传导fine-tuned 微调first-order feature ⼀阶特征forward pass 前向传导forward propagation 前向传播Gaussian prior ⾼斯先验概率generative model ⽣成模型gradient descent 梯度下降Greedy layer-wise training 逐层贪婪训练⽅法grouping matrix 分组矩阵Hadamard product 阿达马乘积Hessian matrix Hessian 矩阵hidden layer 隐含层hidden units 隐藏神经元Hierarchical grouping 层次型分组higher-order features 更⾼阶特征highly non-convex optimization problem ⾼度⾮凸的优化问题histogram 直⽅图hyperbolic tangent 双曲正切函数hypothesis 估值,假设identity activation function 恒等激励函数IID 独⽴同分布illumination 照明inactive 抑制independent component analysis 独⽴成份分析input domains 输⼊域input layer 输⼊层intensity 亮度/灰度intercept term 截距KL divergence 相对熵KL divergence KL分散度k-Means K-均值learning rate 学习速率least squares 最⼩⼆乘法linear correspondence 线性响应linear superposition 线性叠加line-search algorithm 线搜索算法local mean subtraction 局部均值消减local optima 局部最优解logistic regression 逻辑回归loss function 损失函数low-pass filtering 低通滤波magnitude 幅值MAP 极⼤后验估计maximum likelihood estimation 极⼤似然估计mean 平均值MFCC Mel 倒频系数multi-class classification 多元分类neural networks 神经⽹络neuron 神经元Newton’s method ⽜顿法non-convex function ⾮凸函数non-linear feature ⾮线性特征norm 范式norm bounded 有界范数norm constrained 范数约束normalization 归⼀化numerical roundoff errors 数值舍⼊误差numerically checking 数值检验numerically reliable 数值计算上稳定object detection 物体检测objective function ⽬标函数off-by-one error 缺位错误orthogonalization 正交化output layer 输出层overall cost function 总体代价函数over-complete basis 超完备基over-fitting 过拟合parts of objects ⽬标的部件part-whole decompostion 部分-整体分解PCA 主元分析penalty term 惩罚因⼦per-example mean subtraction 逐样本均值消减pooling 池化pretrain 预训练principal components analysis 主成份分析quadratic constraints ⼆次约束RBMs 受限Boltzman机reconstruction based models 基于重构的模型reconstruction cost 重建代价reconstruction term 重构项redundant 冗余reflection matrix 反射矩阵regularization 正则化regularization term 正则化项rescaling 缩放robust 鲁棒性run ⾏程second-order feature ⼆阶特征sigmoid activation function S型激励函数significant digits 有效数字singular value 奇异值singular vector 奇异向量smoothed L1 penalty 平滑的L1范数惩罚Smoothed topographic L1 sparsity penalty 平滑地形L1稀疏惩罚函数smoothing 平滑Softmax Regresson Softmax回归sorted in decreasing order 降序排列source features 源特征sparse autoencoder 消减归⼀化Sparsity 稀疏性sparsity parameter 稀疏性参数sparsity penalty 稀疏惩罚square function 平⽅函数squared-error ⽅差stationary 平稳性(不变性)stationary stochastic process 平稳随机过程step-size 步长值supervised learning 监督学习symmetric positive semi-definite matrix 对称半正定矩阵symmetry breaking 对称失效tanh function 双曲正切函数the average activation 平均活跃度the derivative checking method 梯度验证⽅法the empirical distribution 经验分布函数the energy function 能量函数the Lagrange dual 拉格朗⽇对偶函数the log likelihood 对数似然函数the pixel intensity value 像素灰度值the rate of convergence 收敛速度topographic cost term 拓扑代价项topographic ordered 拓扑秩序transformation 变换translation invariant 平移不变性trivial answer 平凡解under-complete basis 不完备基unrolling 组合扩展unsupervised learning ⽆监督学习variance ⽅差vecotrized implementation 向量化实现vectorization ⽮量化visual cortex 视觉⽪层weight decay 权重衰减weighted average 加权平均值whitening ⽩化zero-mean 均值为零Letter AAccumulated error backpropagation 累积误差逆传播Activation Function 激活函数Adaptive Resonance Theory/ART ⾃适应谐振理论Addictive model 加性学习Adversarial Networks 对抗⽹络Affine Layer 仿射层Affinity matrix 亲和矩阵Agent 代理 / 智能体Algorithm 算法Alpha-beta pruning α-β剪枝Anomaly detection 异常检测Approximation 近似Area Under ROC Curve/AUC Roc 曲线下⾯积Artificial General Intelligence/AGI 通⽤⼈⼯智能Artificial Intelligence/AI ⼈⼯智能Association analysis 关联分析Attention mechanism 注意⼒机制Attribute conditional independence assumption 属性条件独⽴性假设Attribute space 属性空间Attribute value 属性值Autoencoder ⾃编码器Automatic speech recognition ⾃动语⾳识别Automatic summarization ⾃动摘要Average gradient 平均梯度Average-Pooling 平均池化Letter BBackpropagation Through Time 通过时间的反向传播Backpropagation/BP 反向传播Base learner 基学习器Base learning algorithm 基学习算法Batch Normalization/BN 批量归⼀化Bayes decision rule 贝叶斯判定准则Bayes Model Averaging/BMA 贝叶斯模型平均Bayes optimal classifier 贝叶斯最优分类器Bayesian decision theory 贝叶斯决策论Bayesian network 贝叶斯⽹络Between-class scatter matrix 类间散度矩阵Bias 偏置 / 偏差Bias-variance decomposition 偏差-⽅差分解Bias-Variance Dilemma 偏差 – ⽅差困境Bi-directional Long-Short Term Memory/Bi-LSTM 双向长短期记忆Binary classification ⼆分类Binomial test ⼆项检验Bi-partition ⼆分法Boltzmann machine 玻尔兹曼机Bootstrap sampling ⾃助采样法/可重复采样/有放回采样Bootstrapping ⾃助法Break-Event Point/BEP 平衡点Letter CCalibration 校准Cascade-Correlation 级联相关Categorical attribute 离散属性Class-conditional probability 类条件概率Classification and regression tree/CART 分类与回归树Classifier 分类器Class-imbalance 类别不平衡Closed -form 闭式Cluster 簇/类/集群Cluster analysis 聚类分析Clustering 聚类Clustering ensemble 聚类集成Co-adapting 共适应Coding matrix 编码矩阵COLT 国际学习理论会议Committee-based learning 基于委员会的学习Competitive learning 竞争型学习Component learner 组件学习器Comprehensibility 可解释性Computation Cost 计算成本Computational Linguistics 计算语⾔学Computer vision 计算机视觉Concept drift 概念漂移Concept Learning System /CLS 概念学习系统Conditional entropy 条件熵Conditional mutual information 条件互信息Conditional Probability Table/CPT 条件概率表Conditional random field/CRF 条件随机场Conditional risk 条件风险Confidence 置信度Confusion matrix 混淆矩阵Connection weight 连接权Connectionism 连结主义Consistency ⼀致性/相合性Contingency table 列联表Continuous attribute 连续属性Convergence 收敛Conversational agent 会话智能体Convex quadratic programming 凸⼆次规划Convexity 凸性Convolutional neural network/CNN 卷积神经⽹络Co-occurrence 同现Correlation coefficient 相关系数Cosine similarity 余弦相似度Cost curve 成本曲线Cost Function 成本函数Cost matrix 成本矩阵Cost-sensitive 成本敏感Cross entropy 交叉熵Cross validation 交叉验证Crowdsourcing 众包Curse of dimensionality 维数灾难Cut point 截断点Cutting plane algorithm 割平⾯法Letter DData mining 数据挖掘Data set 数据集Decision Boundary 决策边界Decision stump 决策树桩Decision tree 决策树/判定树Deduction 演绎Deep Belief Network 深度信念⽹络Deep Convolutional Generative Adversarial Network/DCGAN 深度卷积⽣成对抗⽹络Deep learning 深度学习Deep neural network/DNN 深度神经⽹络Deep Q-Learning 深度 Q 学习Deep Q-Network 深度 Q ⽹络Density estimation 密度估计Density-based clustering 密度聚类Differentiable neural computer 可微分神经计算机Dimensionality reduction algorithm 降维算法Directed edge 有向边Disagreement measure 不合度量Discriminative model 判别模型Discriminator 判别器Distance measure 距离度量Distance metric learning 距离度量学习Distribution 分布Divergence 散度Diversity measure 多样性度量/差异性度量Domain adaption 领域⾃适应Downsampling 下采样D-separation (Directed separation)有向分离Dual problem 对偶问题Dummy node 哑结点Dynamic Fusion 动态融合Dynamic programming 动态规划Letter EEigenvalue decomposition 特征值分解Embedding 嵌⼊Emotional analysis 情绪分析Empirical conditional entropy 经验条件熵Empirical entropy 经验熵Empirical error 经验误差Empirical risk 经验风险End-to-End 端到端Energy-based model 基于能量的模型Ensemble learning 集成学习Ensemble pruning 集成修剪Error Correcting Output Codes/ECOC 纠错输出码Error rate 错误率Error-ambiguity decomposition 误差-分歧分解Euclidean distance 欧⽒距离Evolutionary computation 演化计算Expectation-Maximization 期望最⼤化Expected loss 期望损失Exploding Gradient Problem 梯度爆炸问题Exponential loss function 指数损失函数Extreme Learning Machine/ELM 超限学习机Letter FFactorization 因⼦分解False negative 假负类False positive 假正类False Positive Rate/FPR 假正例率Feature engineering 特征⼯程Feature selection 特征选择Feature vector 特征向量Featured Learning 特征学习Feedforward Neural Networks/FNN 前馈神经⽹络Fine-tuning 微调Flipping output 翻转法Fluctuation 震荡Forward stagewise algorithm 前向分步算法Frequentist 频率主义学派Full-rank matrix 满秩矩阵Functional neuron 功能神经元Letter GGain ratio 增益率Game theory 博弈论Gaussian kernel function ⾼斯核函数Gaussian Mixture Model ⾼斯混合模型General Problem Solving 通⽤问题求解Generalization 泛化Generalization error 泛化误差Generalization error bound 泛化误差上界Generalized Lagrange function ⼴义拉格朗⽇函数Generalized linear model ⼴义线性模型Generalized Rayleigh quotient ⼴义瑞利商Generative Adversarial Networks/GAN ⽣成对抗⽹络Generative Model ⽣成模型Generator ⽣成器Genetic Algorithm/GA 遗传算法Gibbs sampling 吉布斯采样Gini index 基尼指数Global minimum 全局最⼩Global Optimization 全局优化Gradient boosting 梯度提升Gradient Descent 梯度下降Graph theory 图论Ground-truth 真相/真实Letter HHard margin 硬间隔Hard voting 硬投票Harmonic mean 调和平均Hesse matrix 海塞矩阵Hidden dynamic model 隐动态模型Hidden layer 隐藏层Hidden Markov Model/HMM 隐马尔可夫模型Hierarchical clustering 层次聚类Hilbert space 希尔伯特空间Hinge loss function 合页损失函数Hold-out 留出法Homogeneous 同质Hybrid computing 混合计算Hyperparameter 超参数Hypothesis 假设Hypothesis test 假设验证Letter IICML 国际机器学习会议Improved iterative scaling/IIS 改进的迭代尺度法Incremental learning 增量学习Independent and identically distributed/i.i.d. 独⽴同分布Independent Component Analysis/ICA 独⽴成分分析Indicator function 指⽰函数Individual learner 个体学习器Induction 归纳Inductive bias 归纳偏好Inductive learning 归纳学习Inductive Logic Programming/ILP 归纳逻辑程序设计Information entropy 信息熵Information gain 信息增益Input layer 输⼊层Insensitive loss 不敏感损失Inter-cluster similarity 簇间相似度International Conference for Machine Learning/ICML 国际机器学习⼤会Intra-cluster similarity 簇内相似度Intrinsic value 固有值Isometric Mapping/Isomap 等度量映射Isotonic regression 等分回归Iterative Dichotomiser 迭代⼆分器Letter KKernel method 核⽅法Kernel trick 核技巧Kernelized Linear Discriminant Analysis/KLDA 核线性判别分析K-fold cross validation k 折交叉验证/k 倍交叉验证K-Means Clustering K – 均值聚类K-Nearest Neighbours Algorithm/KNN K近邻算法Knowledge base 知识库Knowledge Representation 知识表征Letter LLabel space 标记空间Lagrange duality 拉格朗⽇对偶性Lagrange multiplier 拉格朗⽇乘⼦Laplace smoothing 拉普拉斯平滑Laplacian correction 拉普拉斯修正Latent Dirichlet Allocation 隐狄利克雷分布Latent semantic analysis 潜在语义分析Latent variable 隐变量Lazy learning 懒惰学习Learner 学习器Learning by analogy 类⽐学习Learning rate 学习率Learning Vector Quantization/LVQ 学习向量量化Least squares regression tree 最⼩⼆乘回归树Leave-One-Out/LOO 留⼀法linear chain conditional random field 线性链条件随机场Linear Discriminant Analysis/LDA 线性判别分析Linear model 线性模型Linear Regression 线性回归Link function 联系函数Local Markov property 局部马尔可夫性Local minimum 局部最⼩Log likelihood 对数似然Log odds/logit 对数⼏率Logistic Regression Logistic 回归Log-likelihood 对数似然Log-linear regression 对数线性回归Long-Short Term Memory/LSTM 长短期记忆Loss function 损失函数Letter MMachine translation/MT 机器翻译Macron-P 宏查准率Macron-R 宏查全率Majority voting 绝对多数投票法Manifold assumption 流形假设Manifold learning 流形学习Margin theory 间隔理论Marginal distribution 边际分布Marginal independence 边际独⽴性Marginalization 边际化Markov Chain Monte Carlo/MCMC 马尔可夫链蒙特卡罗⽅法Markov Random Field 马尔可夫随机场Maximal clique 最⼤团Maximum Likelihood Estimation/MLE 极⼤似然估计/极⼤似然法Maximum margin 最⼤间隔Maximum weighted spanning tree 最⼤带权⽣成树Max-Pooling 最⼤池化Mean squared error 均⽅误差Meta-learner 元学习器Metric learning 度量学习Micro-P 微查准率Micro-R 微查全率Minimal Description Length/MDL 最⼩描述长度Minimax game 极⼩极⼤博弈Misclassification cost 误分类成本Mixture of experts 混合专家Momentum 动量Moral graph 道德图/端正图Multi-class classification 多分类Multi-document summarization 多⽂档摘要Multi-layer feedforward neural networks 多层前馈神经⽹络Multilayer Perceptron/MLP 多层感知器Multimodal learning 多模态学习Multiple Dimensional Scaling 多维缩放Multiple linear regression 多元线性回归Multi-response Linear Regression /MLR 多响应线性回归Mutual information 互信息Letter NNaive bayes 朴素贝叶斯Naive Bayes Classifier 朴素贝叶斯分类器Named entity recognition 命名实体识别Nash equilibrium 纳什均衡Natural language generation/NLG ⾃然语⾔⽣成Natural language processing ⾃然语⾔处理Negative class 负类Negative correlation 负相关法Negative Log Likelihood 负对数似然Neighbourhood Component Analysis/NCA 近邻成分分析Neural Machine Translation 神经机器翻译Neural Turing Machine 神经图灵机Newton method ⽜顿法NIPS 国际神经信息处理系统会议No Free Lunch Theorem/NFL 没有免费的午餐定理Noise-contrastive estimation 噪⾳对⽐估计Nominal attribute 列名属性Non-convex optimization ⾮凸优化Nonlinear model ⾮线性模型Non-metric distance ⾮度量距离Non-negative matrix factorization ⾮负矩阵分解Non-ordinal attribute ⽆序属性Non-Saturating Game ⾮饱和博弈Norm 范数Normalization 归⼀化Nuclear norm 核范数Numerical attribute 数值属性Letter OObjective function ⽬标函数Oblique decision tree 斜决策树Occam’s razor 奥卡姆剃⼑Odds ⼏率Off-Policy 离策略One shot learning ⼀次性学习One-Dependent Estimator/ODE 独依赖估计On-Policy 在策略Ordinal attribute 有序属性Out-of-bag estimate 包外估计Output layer 输出层Output smearing 输出调制法Overfitting 过拟合/过配Oversampling 过采样Letter PPaired t-test 成对 t 检验Pairwise 成对型Pairwise Markov property 成对马尔可夫性Parameter 参数Parameter estimation 参数估计Parameter tuning 调参Parse tree 解析树Particle Swarm Optimization/PSO 粒⼦群优化算法Part-of-speech tagging 词性标注Perceptron 感知机Performance measure 性能度量Plug and Play Generative Network 即插即⽤⽣成⽹络Plurality voting 相对多数投票法Polarity detection 极性检测Polynomial kernel function 多项式核函数Pooling 池化Positive class 正类Positive definite matrix 正定矩阵Post-hoc test 后续检验Post-pruning 后剪枝potential function 势函数Precision 查准率/准确率Prepruning 预剪枝Principal component analysis/PCA 主成分分析Principle of multiple explanations 多释原则Prior 先验Probability Graphical Model 概率图模型Proximal Gradient Descent/PGD 近端梯度下降Pruning 剪枝Pseudo-label 伪标记Letter QQuantized Neural Network 量⼦化神经⽹络Quantum computer 量⼦计算机Quantum Computing 量⼦计算Quasi Newton method 拟⽜顿法Letter RRadial Basis Function/RBF 径向基函数Random Forest Algorithm 随机森林算法Random walk 随机漫步Recall 查全率/召回率Receiver Operating Characteristic/ROC 受试者⼯作特征Rectified Linear Unit/ReLU 线性修正单元Recurrent Neural Network 循环神经⽹络Recursive neural network 递归神经⽹络Reference model 参考模型Regression 回归Regularization 正则化Reinforcement learning/RL 强化学习Representation learning 表征学习Representer theorem 表⽰定理reproducing kernel Hilbert space/RKHS 再⽣核希尔伯特空间Re-sampling 重采样法Rescaling 再缩放Residual Mapping 残差映射Residual Network 残差⽹络Restricted Boltzmann Machine/RBM 受限玻尔兹曼机Restricted Isometry Property/RIP 限定等距性Re-weighting 重赋权法Robustness 稳健性/鲁棒性Root node 根结点Rule Engine 规则引擎Rule learning 规则学习Letter SSaddle point 鞍点Sample space 样本空间Sampling 采样Score function 评分函数Self-Driving ⾃动驾驶Self-Organizing Map/SOM ⾃组织映射Semi-naive Bayes classifiers 半朴素贝叶斯分类器Semi-Supervised Learning 半监督学习semi-Supervised Support Vector Machine 半监督⽀持向量机Sentiment analysis 情感分析Separating hyperplane 分离超平⾯Sigmoid function Sigmoid 函数Similarity measure 相似度度量Simulated annealing 模拟退⽕Simultaneous localization and mapping 同步定位与地图构建Singular Value Decomposition 奇异值分解Slack variables 松弛变量Smoothing 平滑Soft margin 软间隔Soft margin maximization 软间隔最⼤化Soft voting 软投票Sparse representation 稀疏表征Sparsity 稀疏性Specialization 特化Spectral Clustering 谱聚类Speech Recognition 语⾳识别Splitting variable 切分变量Squashing function 挤压函数Stability-plasticity dilemma 可塑性-稳定性困境Statistical learning 统计学习Status feature function 状态特征函Stochastic gradient descent 随机梯度下降Stratified sampling 分层采样Structural risk 结构风险Structural risk minimization/SRM 结构风险最⼩化Subspace ⼦空间Supervised learning 监督学习/有导师学习support vector expansion ⽀持向量展式Support Vector Machine/SVM ⽀持向量机Surrogat loss 替代损失Surrogate function 替代函数Symbolic learning 符号学习Symbolism 符号主义Synset 同义词集Letter TT-Distribution Stochastic Neighbour Embedding/t-SNE T – 分布随机近邻嵌⼊Tensor 张量Tensor Processing Units/TPU 张量处理单元The least square method 最⼩⼆乘法Threshold 阈值Threshold logic unit 阈值逻辑单元Threshold-moving 阈值移动Time Step 时间步骤Tokenization 标记化Training error 训练误差Training instance 训练⽰例/训练例Transductive learning 直推学习Transfer learning 迁移学习Treebank 树库Tria-by-error 试错法True negative 真负类True positive 真正类True Positive Rate/TPR 真正例率Turing Machine 图灵机Twice-learning ⼆次学习Letter UUnderfitting ⽋拟合/⽋配Undersampling ⽋采样Understandability 可理解性Unequal cost ⾮均等代价Unit-step function 单位阶跃函数Univariate decision tree 单变量决策树Unsupervised learning ⽆监督学习/⽆导师学习Unsupervised layer-wise training ⽆监督逐层训练Upsampling 上采样Letter VVanishing Gradient Problem 梯度消失问题Variational inference 变分推断VC Theory VC维理论Version space 版本空间Viterbi algorithm 维特⽐算法Von Neumann architecture 冯 · 诺伊曼架构Letter WWasserstein GAN/WGAN Wasserstein⽣成对抗⽹络Weak learner 弱学习器Weight 权重Weight sharing 权共享Weighted voting 加权投票法Within-class scatter matrix 类内散度矩阵Word embedding 词嵌⼊Word sense disambiguation 词义消歧Letter ZZero-data learning 零数据学习Zero-shot learning 零次学习Aapproximations近似值arbitrary随意的affine仿射的arbitrary任意的amino acid氨基酸amenable经得起检验的axiom公理,原则abstract提取architecture架构,体系结构;建造业absolute绝对的arsenal军⽕库assignment分配algebra线性代数asymptotically⽆症状的appropriate恰当的Bbias偏差brevity简短,简洁;短暂broader⼴泛briefly简短的batch批量Cconvergence 收敛,集中到⼀点convex凸的contours轮廓constraint约束constant常理commercial商务的complementarity补充coordinate ascent同等级上升clipping剪下物;剪报;修剪component分量;部件continuous连续的covariance协⽅差canonical正规的,正则的concave⾮凸的corresponds相符合;相当;通信corollary推论concrete具体的事物,实在的东西cross validation交叉验证correlation相互关系convention约定cluster⼀簇centroids 质⼼,形⼼converge收敛computationally计算(机)的calculus计算Dderive获得,取得dual⼆元的duality⼆元性;⼆象性;对偶性derivation求导;得到;起源denote预⽰,表⽰,是…的标志;意味着,[逻]指称divergence 散度;发散性dimension尺度,规格;维数dot⼩圆点distortion变形density概率密度函数discrete离散的discriminative有识别能⼒的diagonal对⾓dispersion分散,散开determinant决定因素disjoint不相交的Eencounter遇到ellipses椭圆equality等式extra额外的empirical经验;观察ennmerate例举,计数exceed超过,越出expectation期望efficient⽣效的endow赋予explicitly清楚的exponential family指数家族equivalently等价的Ffeasible可⾏的forary初次尝试finite有限的,限定的forgo摒弃,放弃fliter过滤frequentist最常发⽣的forward search前向式搜索formalize使定形Ggeneralized归纳的generalization概括,归纳;普遍化;判断(根据不⾜)guarantee保证;抵押品generate形成,产⽣geometric margins⼏何边界gap裂⼝generative⽣产的;有⽣产⼒的Hheuristic启发式的;启发法;启发程序hone怀恋;磨hyperplane超平⾯Linitial最初的implement执⾏intuitive凭直觉获知的incremental增加的intercept截距intuitious直觉instantiation例⼦indicator指⽰物,指⽰器interative重复的,迭代的integral积分identical相等的;完全相同的indicate表⽰,指出invariance不变性,恒定性impose把…强加于intermediate中间的interpretation解释,翻译Jjoint distribution联合概率Llieu替代logarithmic对数的,⽤对数表⽰的latent潜在的Leave-one-out cross validation留⼀法交叉验证Mmagnitude巨⼤mapping绘图,制图;映射matrix矩阵mutual相互的,共同的monotonically单调的minor较⼩的,次要的multinomial多项的multi-class classification⼆分类问题Nnasty讨厌的notation标志,注释naïve朴素的Oobtain得到oscillate摆动optimization problem最优化问题objective function⽬标函数optimal最理想的orthogonal(⽮量,矩阵等)正交的orientation⽅向ordinary普通的occasionally偶然的Ppartial derivative偏导数property性质proportional成⽐例的primal原始的,最初的permit允许pseudocode伪代码permissible可允许的polynomial多项式preliminary预备precision精度perturbation 不安,扰乱poist假定,设想positive semi-definite半正定的parentheses圆括号posterior probability后验概率plementarity补充pictorially图像的parameterize确定…的参数poisson distribution柏松分布pertinent相关的Qquadratic⼆次的quantity量,数量;分量query疑问的Rregularization使系统化;调整reoptimize重新优化restrict限制;限定;约束reminiscent回忆往事的;提醒的;使⼈联想…的(of)remark注意random variable随机变量respect考虑respectively各⾃的;分别的redundant过多的;冗余的Ssusceptible敏感的stochastic可能的;随机的symmetric对称的sophisticated复杂的spurious假的;伪造的subtract减去;减法器simultaneously同时发⽣地;同步地suffice满⾜scarce稀有的,难得的split分解,分离subset⼦集statistic统计量successive iteratious连续的迭代scale标度sort of有⼏分的squares平⽅Ttrajectory轨迹temporarily暂时的terminology专⽤名词tolerance容忍;公差thumb翻阅threshold阈,临界theorem定理tangent正弦Uunit-length vector单位向量Vvalid有效的,正确的variance⽅差variable变量;变元vocabulary词汇valued经估价的;宝贵的Wwrapper包装分类:。
初中数学词汇

-初中数学词汇数学mathematics, maths(BrE), math(AmE) 公理axiom[ˈæksiəm]定理theorem [ˈθiərəm]计算calculation运算operation证明prove假设hypothesis, hypotheses(pl.)[hai’pɑθisis]命题(true false) proposition算术arithmeticaddition 加法subtraction 减法multiplication[ˌmʌltəpliˈkeʃən]乘法division 除法加plus(prep.),add(v.)被加数augend,summand加数addend和sum减minus(prep.),subtract(v.)被减数minuend [ˈminjuend]减数subtrahend[ˈsʌbtrəhend]差remainder[riˈmendɚ]乘times,multiply(v.)被乘数multiplicand,faciend乘数multiplicator[ˈmʌltiplikeitə]积product除divided by,divide(v.)被除数dividend[ˈdividend]除数divisor[diˈvaizə]商quotient [ˈkwəʊʃənt]等于equals, is equal to, is equivalent to大于is greater than小于is lesser than 大于等于is equal or greater than小于等于is equal or lesser than运算符operator数字digit数number自然数natural number整数integer小数decimal小数点decimal point分数fraction分子numerator [ˈnuməˌretɚ]分母denominator[diˈnɑməˌnetɚ]比ratio[ˈreʃiəu]正positive负negative零null, zero, nought, nil十进制decimal system二进制binary system[ˈbainəri]进位carry截尾truncation四舍五入round下舍入round down上舍入round up有效数字significant digit无效数字insignificant digit代数algebra公式formula, formulae(pl.)单项式monomial[mɑˈnomiəl]多项式polynomial[ˌpɔliˈnəumjəl], multinomial[ˌmʌltiˈnomiəl]系数coefficient[ˌkəʊˈfiʃənt]未知数unknown, x-factor, y-factor, z-factor等式,方程式equation一次方程simple equation二次方程quadratic equation[kwɑˈdrætik]三次方程cubic equation[ˈkjubik]不等式inequation方程的解solution可解的,可分[溶]解的resoluble [riˈzɔljəbəl]完全平方式plete quadratic form指数,幂exponent乘方power使乘五次方to raise to the power of five二次方,平方square某数的平方x squared三次方,立方cube四次方the power of four, the fourth powern次方the power of n, the nth power开方extraction二次方根,平方根square root三次方根,立方根cube root四次方根the root of four, the fourth rootn次方根the root of n, the nth root集合aggregate[ˈæɡr iɡit]元素element函数function定义域domain, field of definition[dɔˈmen]值域range [rendʒ]常量constant变量variable [ˈvɛriəbəl, ˈvær-]图象image微积分calculus[ˈkælkjələs]微分differential[ˌdifəˈrɛnʃəl]导数derivative[diˈrivətiv]极限limit无穷大infinite(a.) infinity(n.) 无穷小infinitesima l[ˌinfiniˈtɛsəməl]有理数rational number无理数irrational number实数real number虚数imaginary number几何geometry平面几何plane geometry立体几何solid geometry点point线line面plane体solid线段segment射线radial平行、平行线parallel相交intersect角angle角度degree弧度radian锐角acute angle直角right angle钝角obtuse angle [ɔbˈtus]平角straight angle周角perigon底base边side高height三角形triangle锐角三角形acute triangle直角三角形right triangle直角边leg斜边hypotenuse [haiˈpɑtnus]勾股定理Pythagoreantheorem [paiˌθæɡəˈri:ən]钝角三角形obtuse triangle不等边三角形scalene['skeili:n] triangle等腰三角形isosceles[ai'sɒsili:z] triangle等边三角形equilateral triangle[ˌikwəˈlætərəl]四边形quadrilateral[ˌkwɔdrəˈlætərəl]平行四边形parallelogram[ˌpærəˈlɛləˌɡræm]矩形rectangle长length宽width菱形rhomb[rɒm],rhombus,rhombi(pl.), diamond 形square梯形trapezoid[ˈtræp iˌzɔid]直角梯形right trapezoid等腰梯形isosceles trapezoid五边形pentagon [ˈpentəˌgən]多边形polygon正多边形equilateral polygon圆circle圆心centre(BrE), center(AmE)半径radius[ˈrediəs/ˈreidjəs]直径diamete r[daiˈæm itɚ]圆周率pi弧arc[ɑ:k]半圆semicircle扇形sector环ring椭圆ellipse[iˈlips]圆周circumference周长perimeter面积area轨迹locus, loca(pl.)[ˈləʊkəs]相似similar全等congruent[ˈkɑŋɡruənt]四面体tetrahedron[ˌtetrəˈhi:drən]立方体cube多面体polyhedron[ˌpɒliˈhi:drən]棱锥pyramid[ˈpirəmid]棱柱prism[ˈprizəm]旋转rotation轴axis[ˈæks is]圆锥cone /əu/圆柱cylinder [ˈsilində]球sphere半球hemisphere底面undersurface外表积surface area体积volume空间space坐标系coordinates [kəuˈɔ:dineits]坐标轴x-axis, y-axis, z-axis横坐标x-coordinate纵坐标y-coordinate 原点origin正弦sine余弦cosine正切tangent余切cotangent周期period心incentre(BrE), incenter(AmE)外心excentre(BrE), excenter(AmE)旁心escentre(BrE), escenter(AmE)垂心orthocentre(BrE), orthocenter(AmE) 重心barycentre(BrE), barycenter(AmE) 切圆inscribed circle外切圆circumcircle [ˈsə:kəmˈsə:kl]相对的opposite相似的similar等距的equidistant[ˌi:kwiˈdistənt] overlap 重叠统计statistics平均数average比例propotion百分比percent排列permutation组合bination概率,或然率probability分布distribution图表graph条形统计图bar graph柱形统计图histogram折线统计图broken line graph曲线统计图curve diagram扇形统计图pie diagram GRE数学专业术语词汇代数局部1.有关数学运算add,plus 加subtract 减difference 差multiply, times 乘product 积divide 除divisible 可被整除的divided evenly 被整除dividend被除数,divisor因子,除数quotient 商remainder余数factorial 阶乘power 乘方radical sign/ root sign 根号round to四舍五入increase 增加reduce减少3.有关代数式、方程和不等式value值equation 方程algebraic term 代数项like terms, similar terms 同类项satisfy 满足numerical coefficient数字系数literal coefficient 字母系数inequality 不等式triangle inequality 三角不等式range 值域original equation 原方程equivalent equation 同解方程,等价方程linear equation线性方程(e.g.5x+6=22)4.有关分数和小数fraction 分数proper fraction 真分数improper fraction 假分数mixed number 带分数vulgar fraction,mon fraction 普通分数simple fraction 简分数plex fraction繁分数numerator 分子denominator 分母(least)mon denominator 〔最小〕公分母quarter 四分之一decimal fraction纯小数infinite decimal 无穷小数recurring decimal循环小数percent 百分之5.根本数学概念average平均数maximum/ minimum number 最大/ 最小数square 平方arithmetic mean 算术平均值weighted average 加权平均值geometric mean 几何平均数exponent指数,幂base乘幂的底数,底边cube 立方数,立方体square root 平方根cube root 立方根mon logarithm 常用对数digit数字not more than不大于less than 小于units’ digit个位数字sum 和represent 代表adjacent 相邻的constant 常数variable 变量inversefunction反函数plementary function余函数linear一次的,线性的factorization因式分解absolute value绝对值,e.g.|-32|=32round off四舍五入6.有关数论number数natural number自然数positive number正数negative number负数odd integer, odd number奇数even integer, even number偶数integer, whole number整数positive integer正整数positive whole number正整数negative whole number负整数consecutive number 连续整数real number, rational number实数,有理数irrational〔number〕无理数inverse倒数posite number合数prime number质数reciprocal倒数mon divisor公约数multiple倍数(least)mon multiple(最小)公倍数(prime)factor(质)因子mon factor公因子decimal system十进制nonnegative非负的tens十位units个位mode众数median中数mon ratio公比7.数列sequence 数列arithmetic progression(sequence)等差数列geometric progression(sequence)等比数列consecutive连续的8.其它approximate近似(anti)clockwise(逆)顺时针方向direct proportion 、direct ratio正比distinct不同的estimation估计,近似parentheses括号proportion比例permutation排列bination组合table表格trigonometric function三角函数unit单位,位figure 图表几何局部1.所有的角angle 角alternate angle错角corresponding angle同位角vertical angle对顶角central angle圆心角interior angle角exterior angle外角 a supplementary angle补角plementary angle余角adjacent angle邻角acute angle锐角obtuse angle钝角right angle直角round angle周角straight angle平角included angle夹角2.所有的三角形triangle三角形regular triangle 正三角形equilateral triangle等边三角形scalene triangle不等边三角形isosceles triangle等腰三角形right triangle直角三角形oblique斜三角形inscribed triangle 接三角形3.有关收敛的平面图形,除三角形外semicircle半圆concentric circles同心圆quadrilateral 四边形pentagon五边形polygon多边形parallelogram平行四边形equilateral等边形plane 平面square形,平方rectangle长方形regular polygon正多边形rhombus菱形trapezoid梯形4.其它平面图形arc弧line, straight line 、beeline直线line segment线段parallel lines平行线segment of a circle 弧形5.有关立体图形cube立方体,立方数rectangular solid长方体regular solid/regular polyhedron正多面体circular cylinder圆柱体cone圆锥sphere球体solid立体的octahedron 八面体6.有关图形上的附属物perimeter 周长area 面积altitude高depth深度side边长circumference, perimeter周长radian 弧度surface area外表积volume体积arm直角三角形的股cross section横截面centre of a circle圆心chord弦radius半径angle bisector角平分线diagonal对角线diameter直径edge棱face of a solid立体的面hypotenuse斜边included side夹边leg三角形的直角边median of a triangle三角形的中线base底边,底数〔e.g.2的5次方,2就是底数〕opposite直角三角形中的对边midpoint中点endpoint端点vertex(复数形式vertices)顶点tangent切线的transversal截线intercept截距shaded region 阴影区7.有关坐标coordinate system坐标系rectangular coordinate直角坐标系origin原点abscissa横坐标ordinate纵坐标number line数轴quadrant象限slope斜率plex plane复平面8.其它plane geometry平面几何trigonometry三角学bisect平分circumscribe外切inscribe切intersect相交perpendicular垂直parallel 平行Pythagorean proposition勾股定理congruent全等的multilateral多边的measure 度量其它1.单位类cent美分penny 一美分硬币nickel5美分硬币dime一角硬币dozen打〔12个〕score廿(20个)Centigrade摄氏Fahrenheit华氏quart夸脱gallon加仑(1gallon=4quart)yard 码meter米micron微米inch英寸foot英尺minute分(角度的度量单位,60分=1度)square measure平方单位制cubic meter立方米pint品脱(干量或液量的单位)2.有关文字表达题,主要是有关商业intercalary year(leap year)闰年(366天)mon year平年(365天)depreciation折旧down payment预付定金discount打折margin利润profit利润interest 利息simple interest单利pounded interest复利dividend红利decrease to减少到decrease by减少了increase to增加到increase by增加了denote表示list price标价markup涨价per capita每人ratio比率retail price零售价tie打平to the nearest最接近的velocity速度[vəˈlɑs iti]3.chapter 章section 节paragraph段落textbook教材, 课本course , curriculum[kəˈrikjələm]课程,courseware课件examination, exam考试test 测验fill in the blanks填空simple select 单项选择grade 等级score分数成绩results book成绩册test paper试卷total score 总分。
AI术语

人工智能专业重要词汇表1、A开头的词汇:Artificial General Intelligence/AGI通用人工智能Artificial Intelligence/AI人工智能Association analysis关联分析Attention mechanism注意力机制Attribute conditional independence assumption属性条件独立性假设Attribute space属性空间Attribute value属性值Autoencoder自编码器Automatic speech recognition自动语音识别Automatic summarization自动摘要Average gradient平均梯度Average-Pooling平均池化Accumulated error backpropagation累积误差逆传播Activation Function激活函数Adaptive Resonance Theory/ART自适应谐振理论Addictive model加性学习Adversarial Networks对抗网络Affine Layer仿射层Affinity matrix亲和矩阵Agent代理/ 智能体Algorithm算法Alpha-beta pruningα-β剪枝Anomaly detection异常检测Approximation近似Area Under ROC Curve/AUC R oc 曲线下面积2、B开头的词汇Backpropagation Through Time通过时间的反向传播Backpropagation/BP反向传播Base learner基学习器Base learning algorithm基学习算法Batch Normalization/BN批量归一化Bayes decision rule贝叶斯判定准则Bayes Model Averaging/BMA贝叶斯模型平均Bayes optimal classifier贝叶斯最优分类器Bayesian decision theory贝叶斯决策论Bayesian network贝叶斯网络Between-class scatter matrix类间散度矩阵Bias偏置/ 偏差Bias-variance decomposition偏差-方差分解Bias-Variance Dilemma偏差–方差困境Bi-directional Long-Short Term Memory/Bi-LSTM双向长短期记忆Binary classification二分类Binomial test二项检验Bi-partition二分法Boltzmann machine玻尔兹曼机Bootstrap sampling自助采样法/可重复采样/有放回采样Bootstrapping自助法Break-Event Point/BEP平衡点3、C开头的词汇Calibration校准Cascade-Correlation级联相关Categorical attribute离散属性Class-conditional probability类条件概率Classification and regression tree/CART分类与回归树Classifier分类器Class-imbalance类别不平衡Closed -form闭式Cluster簇/类/集群Cluster analysis聚类分析Clustering聚类Clustering ensemble聚类集成Co-adapting共适应Coding matrix编码矩阵COLT国际学习理论会议Committee-based learning基于委员会的学习Competitive learning竞争型学习Component learner组件学习器Comprehensibility可解释性Computation Cost计算成本Computational Linguistics计算语言学Computer vision计算机视觉Concept drift概念漂移Concept Learning System /CLS概念学习系统Conditional entropy条件熵Conditional mutual information条件互信息Conditional Probability Table/CPT条件概率表Conditional random field/CRF条件随机场Conditional risk条件风险Confidence置信度Confusion matrix混淆矩阵Connection weight连接权Connectionism连结主义Consistency一致性/相合性Contingency table列联表Continuous attribute连续属性Convergence收敛Conversational agent会话智能体Convex quadratic programming凸二次规划Convexity凸性Convolutional neural network/CNN卷积神经网络Co-occurrence同现Correlation coefficient相关系数Cosine similarity余弦相似度Cost curve成本曲线Cost Function成本函数Cost matrix成本矩阵Cost-sensitive成本敏感Cross entropy交叉熵Cross validation交叉验证Crowdsourcing众包Curse of dimensionality维数灾难Cut point截断点Cutting plane algorithm割平面法4、D开头的词汇Data mining数据挖掘Data set数据集Decision Boundary决策边界Decision stump决策树桩Decision tree决策树/判定树Deduction演绎Deep Belief Network深度信念网络Deep Convolutional Generative Adversarial Network/DCGAN深度卷积生成对抗网络Deep learning深度学习Deep neural network/DNN深度神经网络Deep Q-Learning深度Q 学习Deep Q-Network深度Q 网络Density estimation密度估计Density-based clustering密度聚类Differentiable neural computer可微分神经计算机Dimensionality reduction algorithm降维算法Directed edge有向边Disagreement measure不合度量Discriminative model判别模型Discriminator判别器Distance measure距离度量Distance metric learning距离度量学习Distribution分布Divergence散度Diversity measure多样性度量/差异性度量Domain adaption领域自适应Downsampling下采样D-separation (Directed separation)有向分离Dual problem对偶问题Dummy node哑结点Dynamic Fusion动态融合Dynamic programming动态规划5、E开头的词汇Eigenvalue decomposition特征值分解Embedding嵌入Emotional analysis情绪分析Empirical conditional entropy经验条件熵Empirical entropy经验熵Empirical error经验误差Empirical risk经验风险End-to-End端到端Energy-based model基于能量的模型Ensemble learning集成学习Ensemble pruning集成修剪Error Correcting Output Codes/ECOC纠错输出码Error rate错误率Error-ambiguity decomposition误差-分歧分解Euclidean distance欧氏距离Evolutionary computation演化计算Expectation-Maximization期望最大化Expected loss期望损失Exploding Gradient Problem梯度爆炸问题Exponential loss function指数损失函数Extreme Learning Machine/ELM超限学习机6、F开头的词汇Factorization因子分解False negative假负类False positive假正类False Positive Rate/FPR假正例率Feature engineering特征工程Feature selection特征选择Feature vector特征向量Featured Learning特征学习Feedforward Neural Networks/FNN前馈神经网络Fine-tuning微调Flipping output翻转法Fluctuation震荡Forward stagewise algorithm前向分步算法Frequentist频率主义学派Full-rank matrix满秩矩阵Functional neuron功能神经元7、G开头的词汇Gain ratio增益率Game theory博弈论Gaussian kernel function高斯核函数Gaussian Mixture Model高斯混合模型General Problem Solving通用问题求解Generalization泛化Generalization error泛化误差Generalization error bound泛化误差上界Generalized Lagrange function广义拉格朗日函数Generalized linear model广义线性模型Generalized Rayleigh quotient广义瑞利商Generative Adversarial Networks/GAN生成对抗网络Generative Model生成模型Generator生成器Genetic Algorithm/GA遗传算法Gibbs sampling吉布斯采样Gini index基尼指数Global minimum全局最小Global Optimization全局优化Gradient boosting梯度提升Gradient Descent梯度下降Graph theory图论Ground-truth真相/真实8、H开头的词汇Hard margin硬间隔Hard voting硬投票Harmonic mean调和平均Hesse matrix海塞矩阵Hidden dynamic model隐动态模型Hidden layer隐藏层Hidden Markov Model/HMM隐马尔可夫模型Hierarchical clustering层次聚类Hilbert space希尔伯特空间Hinge loss function合页损失函数Hold-out留出法Homogeneous同质Hybrid computing混合计算Hyperparameter超参数Hypothesis假设Hypothesis test假设验证9、I开头的词汇ICML国际机器学习会议Improved iterative scaling/IIS改进的迭代尺度法Incremental learning增量学习Independent and identically distributed/i.i.d.独立同分布Independent Component Analysis/ICA独立成分分析Indicator function指示函数Individual learner个体学习器Induction归纳Inductive bias归纳偏好Inductive learning归纳学习Inductive Logic Programming/ILP归纳逻辑程序设计Information entropy信息熵Information gain信息增益Input layer输入层Insensitive loss不敏感损失Inter-cluster similarity簇间相似度International Conference for Machine Learning/ICML国际机器学习大会Intra-cluster similarity簇内相似度Intrinsic value固有值Isometric Mapping/Isomap等度量映射Isotonic regression等分回归Iterative Dichotomiser迭代二分器10、K开头的词汇Kernel method核方法Kernel trick核技巧Kernelized Linear Discriminant Analysis/KLDA核线性判别分析K-fold cross validation k 折交叉验证/k 倍交叉验证K-Means Clustering K –均值聚类K-Nearest Neighbours Algorithm/KNN K近邻算法Knowledge base知识库Knowledge Representation知识表征11、L开头的词汇Label space标记空间Lagrange duality拉格朗日对偶性Lagrange multiplier拉格朗日乘子Laplace smoothing拉普拉斯平滑Laplacian correction拉普拉斯修正Latent Dirichlet Allocation隐狄利克雷分布Latent semantic analysis潜在语义分析Latent variable隐变量Lazy learning懒惰学习Learner学习器Learning by analogy类比学习Learning rate学习率Learning Vector Quantization/LVQ学习向量量化Least squares regression tree最小二乘回归树Leave-One-Out/LOO留一法linear chain conditional random field线性链条件随机场Linear Discriminant Analysis/LDA线性判别分析Linear model线性模型Linear Regression线性回归Link function联系函数Local Markov property局部马尔可夫性Local minimum局部最小Log likelihood对数似然Log odds/logit对数几率Logistic Regression Logistic 回归Log-likelihood对数似然Log-linear regression对数线性回归Long-Short Term Memory/LSTM长短期记忆Loss function损失函数12、M开头的词汇Machine translation/MT机器翻译Macron-P宏查准率Macron-R宏查全率Majority voting绝对多数投票法Manifold assumption流形假设Manifold learning流形学习Margin theory间隔理论Marginal distribution边际分布Marginal independence边际独立性Marginalization边际化Markov Chain Monte Carlo/MCMC马尔可夫链蒙特卡罗方法Markov Random Field马尔可夫随机场Maximal clique最大团Maximum Likelihood Estimation/MLE极大似然估计/极大似然法Maximum margin最大间隔Maximum weighted spanning tree最大带权生成树Max-Pooling最大池化Mean squared error均方误差Meta-learner元学习器Metric learning度量学习Micro-P微查准率Micro-R微查全率Minimal Description Length/MDL最小描述长度Minimax game极小极大博弈Misclassification cost误分类成本Mixture of experts混合专家Momentum动量Moral graph道德图/端正图Multi-class classification多分类Multi-document summarization多文档摘要Multi-layer feedforward neural networks多层前馈神经网络Multilayer Perceptron/MLP多层感知器Multimodal learning多模态学习Multiple Dimensional Scaling多维缩放Multiple linear regression多元线性回归Multi-response Linear Regression /MLR多响应线性回归Mutual information互信息13、N开头的词汇Naive bayes朴素贝叶斯Naive Bayes Classifier朴素贝叶斯分类器Named entity recognition命名实体识别Nash equilibrium纳什均衡Natural language generation/NLG自然语言生成Natural language processing自然语言处理Negative class负类Negative correlation负相关法Negative Log Likelihood负对数似然Neighbourhood Component Analysis/NCA近邻成分分析Neural Machine Translation神经机器翻译Neural Turing Machine神经图灵机Newton method牛顿法NIPS国际神经信息处理系统会议No Free Lunch Theorem/NFL没有免费的午餐定理Noise-contrastive estimation噪音对比估计Nominal attribute列名属性Non-convex optimization非凸优化Nonlinear model非线性模型Non-metric distance非度量距离Non-negative matrix factorization非负矩阵分解Non-ordinal attribute无序属性Non-Saturating Game非饱和博弈Norm范数Normalization归一化Nuclear norm核范数Numerical attribute数值属性14、O开头的词汇Objective function目标函数Oblique decision tree斜决策树Occam’s razor奥卡姆剃刀Odds几率Off-Policy离策略One shot learning一次性学习One-Dependent Estimator/ODE独依赖估计On-Policy在策略Ordinal attribute有序属性Out-of-bag estimate包外估计Output layer输出层Output smearing输出调制法Overfitting过拟合/过配Oversampling过采样15、P开头的词汇Paired t-test成对t 检验Pairwise成对型Pairwise Markov property成对马尔可夫性Parameter参数Parameter estimation参数估计Parameter tuning调参Parse tree解析树Particle Swarm Optimization/PSO粒子群优化算法Part-of-speech tagging词性标注Perceptron感知机Performance measure性能度量Plug and Play Generative Network即插即用生成网络Plurality voting相对多数投票法Polarity detection极性检测Polynomial kernel function多项式核函数Pooling池化Positive class正类Positive definite matrix正定矩阵Post-hoc test后续检验Post-pruning后剪枝potential function势函数Precision查准率/准确率Prepruning预剪枝Principal component analysis/PCA主成分分析Principle of multiple explanations多释原则Prior先验Probability Graphical Model概率图模型Proximal Gradient Descent/PGD近端梯度下降Pruning剪枝Pseudo-label伪标记16、Q开头的词汇Quantized Neural Network量子化神经网络Quantum computer量子计算机Quantum Computing量子计算Quasi Newton method拟牛顿法17、R开头的词汇Radial Basis Function/RBF径向基函数Random Forest Algorithm随机森林算法Random walk随机漫步Recall查全率/召回率Receiver Operating Characteristic/ROC受试者工作特征Rectified Linear Unit/ReLU线性修正单元Recurrent Neural Network循环神经网络Recursive neural network递归神经网络Reference model参考模型Regression回归Regularization正则化Reinforcement learning/RL强化学习Representation learning表征学习Representer theorem表示定理reproducing kernel Hilbert space/RKHS再生核希尔伯特空间Re-sampling重采样法Rescaling再缩放Residual Mapping残差映射Residual Network残差网络Restricted Boltzmann Machine/RBM受限玻尔兹曼机Restricted Isometry Property/RIP限定等距性Re-weighting重赋权法Robustness稳健性/鲁棒性Root node根结点Rule Engine规则引擎Rule learning规则学习18、S开头的词汇Saddle point鞍点Sample space样本空间Sampling采样Score function评分函数Self-Driving自动驾驶Self-Organizing Map/SOM自组织映射Semi-naive Bayes classifiers半朴素贝叶斯分类器Semi-Supervised Learning半监督学习semi-Supervised Support Vector Machine半监督支持向量机Sentiment analysis情感分析Separating hyperplane分离超平面Sigmoid function Sigmoid 函数Similarity measure相似度度量Simulated annealing模拟退火Simultaneous localization and mapping同步定位与地图构建Singular Value Decomposition奇异值分解Slack variables松弛变量Smoothing平滑Soft margin软间隔Soft margin maximization软间隔最大化Soft voting软投票Sparse representation稀疏表征Sparsity稀疏性Specialization特化Spectral Clustering谱聚类Speech Recognition语音识别Splitting variable切分变量Squashing function挤压函数Stability-plasticity dilemma可塑性-稳定性困境Statistical learning统计学习Status feature function状态特征函Stochastic gradient descent随机梯度下降Stratified sampling分层采样Structural risk结构风险Structural risk minimization/SRM结构风险最小化Subspace子空间Supervised learning监督学习/有导师学习support vector expansion支持向量展式Support Vector Machine/SVM支持向量机Surrogat loss替代损失Surrogate function替代函数Symbolic learning符号学习Symbolism符号主义Synset同义词集19、T开头的词汇T-Distribution Stochastic Neighbour Embedding/t-SNE T–分布随机近邻嵌入Tensor张量Tensor Processing Units/TPU张量处理单元The least square method最小二乘法Threshold阈值Threshold logic unit阈值逻辑单元Threshold-moving阈值移动Time Step时间步骤Tokenization标记化Training error训练误差Training instance训练示例/训练例Transductive learning直推学习Transfer learning迁移学习Treebank树库Tria-by-error试错法True negative真负类True positive真正类True Positive Rate/TPR真正例率Turing Machine图灵机Twice-learning二次学习20、U开头的词汇Underfitting欠拟合/欠配Undersampling欠采样Understandability可理解性Unequal cost非均等代价Unit-step function单位阶跃函数Univariate decision tree单变量决策树Unsupervised learning无监督学习/无导师学习Unsupervised layer-wise training无监督逐层训练Upsampling上采样21、V开头的词汇Vanishing Gradient Problem梯度消失问题Variational inference变分推断VC Theory VC维理论Version space版本空间Viterbi algorithm维特比算法Von Neumann architecture冯·诺伊曼架构22、W开头的词汇Wasserstein GAN/WGAN Wasserstein生成对抗网络Weak learner弱学习器Weight权重Weight sharing权共享Weighted voting加权投票法Within-class scatter matrix类内散度矩阵Word embedding词嵌入Word sense disambiguation词义消歧23、Z开头的词汇Zero-data learning零数据学习Zero-shot learning零次学习。
局部和稀疏保持无监督特征选择法

局部和稀疏保持无监督特征选择法简彩仁;陈晓云【摘要】By locality preserving projection and sparsity preserving projection to represent the intrinsic geometrical struc-ture of the data set and use the group sparse of L2 ,1 norm,one new unsupervised feature selection method for high-dimen-sionality small sample data set is proposed.Experimental results show that the method is effective and sensitive to balance parameter.%利用局部保持投影和稀疏保持投影来刻画数据的本质结构,结合 L2,1范数的组稀疏性来选择特征,提出一种新的针对高维小样本数据集的无监督特征选择算法。
实验表明:局部和稀疏保持无监督特征选择法是一种有效的无监督特征选择方法;平衡参数对实验结果有较大的影响。
【期刊名称】《华侨大学学报(自然科学版)》【年(卷),期】2015(000)001【总页数】5页(P111-115)【关键词】局部保持投影;稀疏保持投影;高维小样本;无监督;特征选择;聚类【作者】简彩仁;陈晓云【作者单位】福州大学数学与计算机科学学院,福建福州 350116;福州大学数学与计算机科学学院,福建福州 350116【正文语种】中文【中图分类】TP311;TP371数据维数灾难普遍存在于模式识别的许多应用中.高维数据集不仅限制传统模式识别方法的应用,还会显著地增加内存和时间开销.特征选择是解决这些问题的有效手段之一[1].特征选择旨在选择一些相关的特征代表原始的高维数据,而剔除一些不相关的特征.基于聚类的特征选择法[2],利用聚类算法将数据聚类,用得到的类别信息指导特征选择.然而,由此获得的判别信息是不可靠的.近年来,随着流形学习的兴起,学者提出了新的无监督特征选择方法,如拉普拉斯得分[3]、多簇特征选择方法[4]等.利用L2,1范数的组稀疏性,学者提出了许多嵌入型的特征选择方法,如稀疏限制的无监督最大化边缘的特征选择法[5]、局部和相似保持嵌入特征选择法[6].这些方法应用在高维小样本数据时,需要求解大规模的特征值问题,不利于问题的求解.而联合特征选择和子空间学习法[7]、联合局部保持投影和L2,1范数构造的特征选择,可以克服大规模特征值的问题.本文提出一种基于局部保持投影和稀疏保持投影的无监督特征选择方法,并利用L2,1范数的组稀疏性质,通过正则化L2,1范数来选择特征.局部和稀疏保持无监督特征选择法利用局部保持投影和稀疏保持投影来刻画数据的本质结构.给定数据集X∈Rm×n,局部保持投影(LPP)[8]的目标函数定义为式(1)中:yi=VTxi;W=Wi,j为相似矩阵.最小化目标函数可以使降维后的样本保持原空间的距离.常见的相似矩阵定义是热核函数.经过简单的代数运算,LPP求解如下优化问题,即式(2)中:V为投影矩阵;D为对角矩阵,为图拉普拉斯矩阵,L=D-W.稀疏保持投影(SPP)[9]用样本稀疏重构每一个样本xi∈X,得到求解稀疏表示系数的模型为式(3)中:‖·‖1为1-范数;si=[si,1,…,si,n] ,si,j反映了xi和xj之间的关系.因此,将S=(si,j)n×n视为仿射权矩阵是合理的.SPP旨在寻找保持稀疏关系的投影,SPP的目标函数为式(4)中:V为投影矩阵.为避免平凡解,通常引入正交约束VTXXTV,V可以通过求解广义特征值问题X(I-S-ST+STS)XTv=λXXTv得到.将式(2)的局部保持项和式(4)的稀疏保持项相结合,得到目标函数为引入L2,1范数来选择有利于保持局部性和稀疏性的相关特征,且为避免平凡解,引入正交约束VTXXTV,得到局部和稀疏保持投影特征选择模型,即式(6)中:α为平衡参数,用于平衡局部性和稀疏性;λ为正则参数;‖V‖2.1定义为|Vi,j|2)1/2.当获得投影矩阵V后,可以利用vi的2范数,即‖vi‖2来选择特征,其值越大表示该特征越重要.式(6)可以写为式(7)中:A=αL+(1-α)(I-S-ST+STS).式(7)可以利用广义特征值问题求解.但是,当X 是高维小样本数据时,式(7)需要求解大规模的特征值问题,且会造成矩阵不可逆的问题.类似于文献[7],采用分步求解的方法避免上述困难.令Y=XTV,有式(8)的解为Y*=[y1,…,yd],其为A的最小d个特征值对应的特征向量.求解如下的问题得到式(7)的解,即该问题可用拉格朗日乘子法迭代求解[7].拉格朗日函数为对V求导得式(11)中:Di,i=1/(2‖vi‖2),vi≠0,当Di,i=0时,用一个较小的正数替代,确保D可逆[8].不难求得将式(12)代入式(11),可得由式(12),(13)交替迭代直至收敛,可得投影矩阵V.通过上述讨论可以得到局部和稀疏保持投影无监督特征选择法(LSP).Input:数据矩阵X;Output:特征子集.1) 计算拉普拉斯矩阵L和稀疏表示矩阵S;2) 通过式(8)计算最小d个广义特征值对应的特征向量Y*;3) 求解式(9)得到投影矩阵V;4) 将‖vi‖2降序排列,选取前p个特征构成特征子集.相似矩阵通过W=(|S|+|ST|)/2计算.其中:S为稀疏矩阵,可以避免近邻数量的选择;平衡参数α=0.8.选用数据方差(DV)、拉普拉斯得分(LS)[3]、多簇特征选择方法(MCFS)[4]、联合特征选择和子空间学习法(JFSSL)[7]作为对比方法.LS,MCFS 和JFSSL的近邻数量取5,MCFS,JFSSL和LSP的降维维数d取类别个数.通过对选取的特征子集进行聚类分析,对比聚类准确率(ACC)来验证特征选择的有效性.实验环境为Windows 7系统,内存为2 G,用Matlab 2010b编程实现.对给定样本,令ri和si分别为聚类算法得到的类标签和样本自带的类标签,则聚类准确率[10]为式(14)中:n为样本总数;δ(x,y)为函,当x=y时,其值为1,否则,为0;map(ri)为正交函数,将每一个类标签ri映射成与样本自带的类标签等价的类标签.选用6个公开数据集进行实验,如表1所示.表1中:DLBCL,LUNGCANCER,LEUKEMIA,TOX为基因表达数据集;ORL,PIE为图像数据集.应用每种方法选取特征子集,选取的特征个数依次设为{5,10,15,…,95,100},采用K-means对选取的特征子集进行聚类分析,运行20次.各种方法的平均聚类准确率,如表2所示.聚类准确率与特征数量(n)的关系,如图1所示.由表2,图1可知:局部和稀疏保持投影无监督特征选择法具有良好的特征选择能力,除TOX数据集外,其聚类准确率的平均值最高.与MCFS和JFSSL相比,LSP 的聚类效果更为理想,这说明稀疏保持性质也可以刻画数据的本质结构.与DV和LS相比,因为DV和LS只考虑独立的计算每个特征的得分,而忽略了特征之间的相互作用,所以考虑特征之间的关系可以提高聚类准确率.此外,用LSP进行特征选择与保留全部特征(ALL)可以明显地提高聚类的准确率.因此,利用局部和稀疏保持投影构造的无监督特征选择法是有效的.平衡参数α在{0,0.1,0.2,…,0.9,1.0}变化时,平均聚类准确率的情况,如图2所示.由图2可知:总体上,平衡参数α对LSP的影响是明显的;当α为0.6~0.9时,LSP的聚类准确率在较高的水平上保持相对稳定.在这一范围内稀疏保持项的比重较大,说明稀疏保持项可以提高特征选择的能力.提出局部和稀疏保持无监督特征选择法,利用局部保持投影和稀疏保持投影来刻画数据的本质结构,利用L2,1范数的组稀疏性来筛选特征.实验结果表明:LSP是一种有效的无监督特征选择方法.LSP方法的平衡参数α对实验结果有较大的影响,如何自适应地选取该参数将在今后的研究中给出.【相关文献】[1] 徐峻岭,周毓明,陈林,等.基于互信息的无监督特征选择[J].计算机研究与发展,2012,49(2):372-382.[2] 张莉,孙钢,郭军.基于 K-均值聚类的无监督的特征选择方法[J].计算机应用研究,2005,22(3):23-24.[3] HE Xiao-fei,CAI Deng,NIYOGI placian score for feature selection[C]∥Advances in Neural Inform ation Processing Systems.Vancouver:[s.n.],2005:507-514.[4] CAI Deng,ZHANG Chi-yuan,HE Xiao-fei.Unsupervised feature selection for multi-cluster data[C]∥Proceedings of the 16th ACM SIGKDD International Conference on Knowl edge Discovery and Data Mining.Washington DC:ACM,2010:333-342.[5] YANG Shi-zhun,HOU Chen-ping,NIE Fei-ping,et al.Unsupervised maximum margin feature selection via L2,1-norm minimization[J].Neural Computing and Applications,2012,21(7):1791-1799.[6] FANG Xiao-zhao,XU Yong,LI Xue-long,et al.Locality and similarity preserving embedding for feature selection[J].Neurocomp uting,2014,128:304-315.[7] GU Quan-quan,LI Zhen-hui,HAN Jia-wei.Joint feature selection and subspace learning[C]∥The 22nd International Joint Confere nce on Artificial Intelligence.Barcelona:[s.n.],2011:1294-1299.[8] HE Xiao-hui,NIYOGI P.Locality preserving projections[C]∥Proceedings of the 17th Annual Conference on Neural Information Processing Systems.Columbia:[s.n.],2003:153-160.[9] QIAO Li-shan,CHEN Song-can,TAN Xiao-yang.Sparsity preserving projections with applications to face recognition[J].Pattern Recog nition,2010,43(1):331-341.[10] CAI Deng,HE Xiao-fei,WU Xiao-yun,et al.Non-negative matrix factorization on manifold[C]∥Proceedings of International Conference on Data Mining.Pisa:IEEE Press,2008:63-72.。
数学英文术语
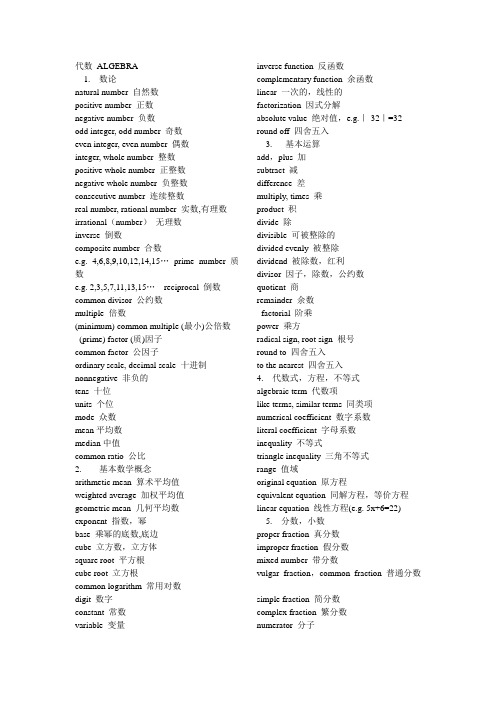
代数ALGEBRA1.数论natural number 自然数positive number 正数negative number 负数odd integer, odd number 奇数even integer, even number 偶数integer, whole number 整数positive whole number 正整数negative whole number 负整数consecutive number 连续整数real number, rational number 实数,有理数irrational(number)无理数inverse 倒数composite number 合数e.g. 4,6,8,9,10,12,14,15…prime number 质数e.g. 2,3,5,7,11,13,15…reciprocal 倒数common divisor 公约数multiple 倍数(minimum) common multiple (最小)公倍数(prime) factor (质)因子common factor 公因子ordinary scale, decimal scale 十进制nonnegative 非负的tens 十位units 个位mode 众数mean平均数median中值common ratio 公比2. 基本数学概念arithmetic mean 算术平均值weighted average 加权平均值geometric mean 几何平均数exponent 指数,幂base 乘幂的底数,底边cube 立方数,立方体square root 平方根cube root 立方根common logarithm 常用对数digit 数字constant 常数variable 变量inverse function 反函数complementary function 余函数linear 一次的,线性的factorization 因式分解absolute value 绝对值,e.g.|-32|=32 round off 四舍五入3. 基本运算add,plus 加subtract 减difference 差multiply, times 乘product 积divide 除divisible 可被整除的divided evenly 被整除dividend 被除数,红利divisor 因子,除数,公约数quotient 商remainder 余数factorial 阶乘power 乘方radical sign, root sign 根号round to 四舍五入to the nearest 四舍五入4.代数式,方程,不等式algebraic term 代数项like terms, similar terms 同类项numerical coefficient 数字系数literal coefficient 字母系数inequality 不等式triangle inequality 三角不等式range 值域original equation 原方程equivalent equation 同解方程,等价方程linear equation 线性方程(e.g. 5x+6=22)5.分数,小数proper fraction 真分数improper fraction 假分数mixed number 带分数vulgar fraction,common fraction 普通分数simple fraction 简分数complex fraction 繁分数numerator 分子denominator 分母(least) common denominator (最小)公分母quarter 四分之一decimal fraction 纯小数infinite decimal 无穷小数recurring decimal 循环小数tenths unit 十分位6. 集合union 并集proper subset 真子集solution set 解集7.数列arithmetic progression(sequence) 等差数列geometric progression(sequence) 等比数列8.其它approximate 近似(anti)clockwise (逆) 顺时针方向cardinal 基数ordinal 序数direct proportion 正比distinct 不同的estimation 估计,近似parentheses 括号proportion 比例permutation 排列combination 组合table 表格trigonometric function 三角函数unit 单位,位几何GEOMETRY1. 角alternate angle 内错角corresponding angle 同位角vertical angle 对顶角central angle 圆心角interior angle 内角exterior angle 外角supplementary angles 补角complementary angle 余角adjacent angle 邻角acute angle 锐角obtuse angle 钝角right angle 直角round angle 周角straight angle 平角included angle 夹角2.三角形equilateral triangle 等边三角形scalene triangle 不等边三角形isosceles triangle 等腰三角形right triangle 直角三角形oblique 斜三角形inscribed triangle 内接三角形3.收敛的平面图形,除三角形外semicircle 半圆concentric circles 同心圆quadrilateral 四边形pentagon 五边形hexagon 六边形heptagon 七边形octagon 八边形nonagon 九边形decagon 十边形polygon 多边形parallelogram 平行四边形equilateral 等边形plane 平面square 正方形,平方rectangle 长方形regular polygon 正多边形rhombus 菱形trapezoid 梯形4.其它平面图形arc 弧line, straight line 直线line segment 线段parallel lines 平行线segment of a circle 弧形5.立体图形cube 立方体,立方数rectangular solid 长方体regular solid/regular polyhedron 正多面体circular cylinder 圆柱体cone 圆锥sphere 球体solid 立体的6.图形的附属概念plane geometry 平面几何trigonometry 三角学bisect 平分circumscribe 外切inscribe 内切intersect 相交perpendicular 垂直Pythagorean theorem 勾股定理(毕达哥拉斯定理)congruent 全等的multilateral 多边的altitude 高depth 深度side 边长circumference, perimeter 周长radian 弧度surface area 表面积volume 体积arm 直角三角形的股cross section 横截面center of a circle 圆心chord 弦diameter 直径radius 半径angle bisector 角平分线diagonal 对角线化edge 棱face of a solid 立体的面hypotenuse 斜边included side 夹边leg 三角形的直角边median (三角形的)中线base 底边,底数(e.g. 2的5次方,2就是底数)opposite 直角三角形中的对边midpoint 中点endpoint 端点vertex (复数形式vertices)顶点tangent 切线的transversal 截线intercept 截距7.坐标coordinate system 坐标系rectangular coordinate 直角坐标系origin 原点abscissa 横坐标ordinate 纵坐标number line 数轴quadrant 象限slope 斜率complex plane 复平面8.计量单位cent 美分penny 一美分硬币nickel 5美分硬币dime 一角硬币dozen 打(12个)score 廿(20个)Centigrade 摄氏Fahrenheit 华氏quart 夸脱gallon 加仑(1 gallon = 4 quart) yard 码meter 米micron 微米inch 英寸foot 英尺minute 分(角度的度量单位,60分=1度) square measure 平方单位制cubic meter 立方米pint 品脱(干量或液量的单位)数学专业术语词汇代数部分1.有关数学运算add,plus 加subtract 减difference 差multiply, times 乘product 积divide 除divisible 可被整除的divided evenly 被整除dividend 被除数,divisor 因子,除数quotient 商remainder余数factorial 阶乘power 乘方radical sign, root sign 根号round to 四舍五入 increase 增加 reduce减少3.有关代数式、方程和不等式value值equation 方程algebraic term代数项like terms, similar terms 同类项satisfy 满足numerical coefficient数字系数literal coefficient 字母系数inequality 不等式triangle inequality 三角不等式range 值域original equation 原方程equivalent equation 同解方程,等价方程linear equation线性方程(e.g.5x +6=22)4.有关分数和小数fraction 分数proper fraction 真分数improper fraction 假分数mixed number 带分数vulgar fraction,common fraction 普通分数simple fraction简分数complex fraction 繁分数numerator 分子denominator 分母(least)common denominator(最小)公分母quarter 四分之一decimal fraction 纯小数infinite decimal 无穷小数recurring decimal循环小数percent 百分之5.基本数学概念average 平均数 maximum/ minimum number 最大/ 最小数 square 平方arithmetic mean 算术平均值weighted average 加权平均值geometric mean几何平均数exponent指数,幂base乘幂的底数,底边cube立方数,立方体square root 平方根cube root立方根common logarithm 常用对数digit数字 not more than不大于less than 小于units’ digit个位数字 sum和 represent 代表adjacent 相邻的 constant 常数variable 变量inverse function 反函数complementary function余函数linear 一次的,线性的factorization因式分解absolute value绝对值,e.g.|-32|=32round off四舍五入6.有关数论number 数natural number 自然数positive number 正数negative number负数odd integer, odd number 奇数even integer, even number 偶数integer, whole number整数positiveinteger 正整数positive whole number 正整数negative whole number 负整数consecutive number连续整数real number, rational number实数,有理数irrational(number)无理数inverse 倒数composite number合数e.g.4,6,8,9,10,12,14,15……prime number质数e.g.2,3,5,7,11,13,15……注意:所有的质数(2除外)都是奇数,但奇数不一定是质数reciprocal 倒数common divisor 公约数multiple 倍数(least)common multiple(最小)公倍数(prime)factor(质)因子common factor 公因子 decimal system十进制nonnegative 非负的tens 十位units 个位mode 众数median 中数common ratio公比7.数列sequence 数列arithmetic progression(sequence)等差数列geometric progression(sequence)等比数列consecutive 连续的8.其它approximate 近似(anti)clockwise(逆)顺时针方向cardinal 基数ordinal序数direct proportion 、direct ratio 正比distinct 不同的estimation估计,近似parentheses 括号proportion 比例permutation 排列combination组合table 表格trigonometric function 三角函数unit单位,位figure 图表几何部分1.所有的角corner 角alternate angle 内错角corresponding angle 同位角vertical angle 对顶角central angle 圆心角interior angle 内角exterior angle外角 a supplementary angle补角complementary angle 余角adjacent angle 邻角acute angle锐角obtuse angle 钝角right angle直角round angle 周角straight angle 平角included angle 夹角2.所有的三角形triangle三角形regulartriangle 正三角形equilateral triangle 等边三角形scalene triangle 不等边三角形isosceles triangle 等腰三角形right triangle直角三角形oblique 斜三角形inscribed triangle 内接三角形3.有关收敛的平面图形,除三角形外semicircle 半圆concentric circles同心圆quadrilateral 四边形pentagon 五边形hexagon 六边形heptagon七边形octagon 八边形nonagon 九边形decagon十边形 icosagon 正二十边形polygon 多边形parallelogram平行四边形equilateral 等边形plane平面square正方形,平方rectangle 长方形regular polygon 正多边形rhombus 菱形trapezoid梯形4.其它平面图形arc 弧line, straight line 、beeline 直线line segment 线段parallel lines 平行线segment of a circle 弧形5.有关立体图形cube 立方体,立方数rectangular solid 长方体regular solid/regular polyhedron正多面体circular cylinder 圆柱体cone 圆锥sphere 球体solid 立体的octahedron 八面体6.有关图形上的附属物perimeter 周长area 面积altitude 高depth 深度side 边长circumference, perimeter 周长radian 弧度surface area表面积volume 体积arm直角三角形的股cross section 横截面centre of a circle 圆心chord 弦radius 半径angle bisector 角平分线diagonal对角线diameter 直径edge 棱face of a solid 立体的面hypotenuse 斜边included side 夹边leg 三角形的直角边median of a triangle 三角形的中线base底边,底数(e.g.2的5次方,2就是底数)opposite 直角三角形中的对边midpoint 中点endpoint 端点vertex(复数形式vertices)顶点tangent 切线的transversal 截线intercept截距shaded region 阴影区7.有关坐标coordinate system 坐标系rectangular coordinate 直角坐标系origin 原点abscissa 横坐标ordinate 纵坐标number line 数轴quadrant 象限slope 斜率complex plane 复平面8.其它plane geometry 平面几何trigonometry 三角学bisect 平分circumscribe 外切inscribe 内切intersect 相交perpendicular 垂直parallel 平行Pythagorean proposition 勾股定理congruent 全等的multilateral 多边的measure 度量其它1.单位类cent 美分penny 一美分硬币nickel5美分硬币dime 一角硬币dozen打(12个)score廿(20个)Centigrade 摄氏Fahrenheit 华氏quart 夸脱gallon 加仑(1gallon=4quart)yard 码meter 米micron 微米inch 英寸foot 英尺minute分(角度的度量单位,60分=1度) square measure 平方单位制cubic meter 立方米pint品脱(干量或液量的单位)2.有关文字叙述题,主要是有关商业intercalary year(leap year)闰年(366天) common year平年(365天) depreciation 折旧down payment直接付款discount打折margin 利润profit 利润interest 利息simple interest 单利compounded interest 复利dividend 红利decrease to减少到decrease by 减少了increase to 增加到increase by 增加了denote 表示list price 标价markup 涨价per capita 每人ratio 比率retail price 零售价。
中科院机器学习题库-new
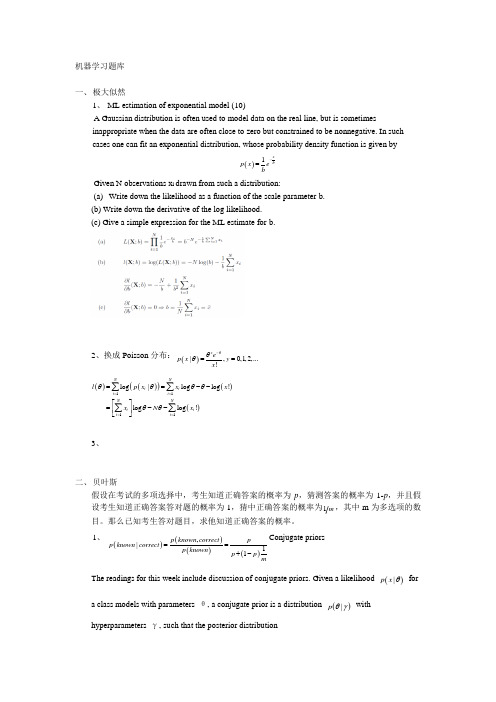
机器学习题库一、 极大似然1、 ML estimation of exponential model (10)A Gaussian distribution is often used to model data on the real line, but is sometimesinappropriate when the data are often close to zero but constrained to be nonnegative. In such cases one can fit an exponential distribution, whose probability density function is given by()1xb p x e b-=Given N observations x i drawn from such a distribution:(a) Write down the likelihood as a function of the scale parameter b.(b) Write down the derivative of the log likelihood.(c) Give a simple expression for the ML estimate for b.2、换成Poisson 分布:()|,0,1,2,...!x e p x y x θθθ-==()()()()()1111log |log log !log log !N Ni i i i N N i i i i l p x x x x N x θθθθθθ======--⎡⎤=--⎢⎥⎣⎦∑∑∑∑3、二、 贝叶斯假设在考试的多项选择中,考生知道正确答案的概率为p ,猜测答案的概率为1-p ,并且假设考生知道正确答案答对题的概率为1,猜中正确答案的概率为1,其中m 为多选项的数目。
自动化英语专业英语词汇表

自动化英语专业英语词汇表文章摘要:本文介绍了自动化英语专业的一些常用的英语词汇,包括自动化技术、控制理论、系统工程、人工智能、模糊逻辑等方面的专业术语。
本文按照字母顺序,将这些词汇分为26个表格,每个表格包含了以相应字母开头的词汇及其中文释义。
本文旨在帮助自动化专业的学习者和从业者掌握和使用这些专业英语词汇,提高他们的英语水平和专业素养。
A英文中文acceleration transducer加速度传感器acceptance testing验收测试accessibility可及性accumulated error累积误差AC-DC-AC frequency converter交-直-交变频器AC (alternating current) electric drive交流电子传动active attitude stabilization主动姿态稳定actuator驱动器,执行机构adaline线性适应元adaptation layer适应层adaptive telemeter system适应遥测系统adjoint operator伴随算子admissible error容许误差aggregation matrix集结矩阵AHP (analytic hierarchy process)层次分析法amplifying element放大环节analog-digital conversion模数转换annunciator信号器antenna pointing control天线指向控制anti-integral windup抗积分饱卷aperiodic decomposition非周期分解a posteriori estimate后验估计approximate reasoning近似推理a priori estimate先验估计articulated robot关节型机器人assignment problem配置问题,分配问题associative memory model联想记忆模型associatron联想机asymptotic stability渐进稳定性attained pose drift实际位姿漂移B英文中文attitude acquisition姿态捕获AOCS (attritude and orbit control system)姿态轨道控制系统attitude angular velocity姿态角速度attitude disturbance姿态扰动attitude maneuver姿态机动attractor吸引子augment ability可扩充性augmented system增广系统automatic manual station自动-手动操作器automaton自动机autonomous system自治系统backlash characteristics间隙特性base coordinate system基座坐标系Bayes classifier贝叶斯分类器bearing alignment方位对准bellows pressure gauge波纹管压力表benefit-cost analysis收益成本分析bilinear system双线性系统biocybernetics生物控制论biological feedback system生物反馈系统C英文中文calibration校准,定标canonical form标准形式canonical realization标准实现capacity coefficient容量系数cascade control级联控制causal system因果系统cell单元,元胞cellular automaton元胞自动机central processing unit (CPU)中央处理器certainty factor确信因子characteristic equation特征方程characteristic function特征函数characteristic polynomial特征多项式characteristic root特征根英文中文charge-coupled device (CCD)电荷耦合器件chaotic system混沌系统check valve单向阀,止回阀chattering phenomenon颤振现象closed-loop control system闭环控制系统closed-loop gain闭环增益cluster analysis聚类分析coefficient of variation变异系数cogging torque齿槽转矩,卡齿转矩cognitive map认知图,认知地图coherency matrix相干矩阵collocation method配点法,配置法combinatorial optimization problem组合优化问题common mode rejection ratio (CMRR)共模抑制比,共模抑制率commutation circuit换相电路,换向电路commutator motor换向电动机D英文中文damping coefficient阻尼系数damping ratio阻尼比data acquisition system (DAS)数据采集系统data fusion数据融合dead zone死区decision analysis决策分析decision feedback equalizer (DFE)决策反馈均衡器decision making决策,决策制定decision support system (DSS)决策支持系统decision table决策表decision tree决策树decentralized control system分散控制系统decoupling control解耦控制defuzzification去模糊化,反模糊化delay element延时环节,滞后环节delta robot德尔塔机器人demodulation解调,检波density function密度函数,概率密度函数derivative action微分作用,微分动作design matrix设计矩阵E英文中文eigenvalue特征值,本征值eigenvector特征向量,本征向量elastic element弹性环节electric drive电子传动electric potential电势electro-hydraulic servo system电液伺服系统electro-mechanical coupling system电机耦合系统electro-pneumatic servo system电气伺服系统electronic governor电子调速器encoder编码器,编码装置end effector末端执行器,末端效应器entropy熵equivalent circuit等效电路error analysis误差分析error bound误差界,误差限error signal误差信号estimation theory估计理论Euclidean distance欧几里得距离,欧氏距离Euler angle欧拉角Euler equation欧拉方程F英文中文factor analysis因子分析factorization method因子法,因式分解法feedback反馈,反馈作用feedback control反馈控制feedback linearization反馈线性化feedforward前馈,前馈作用feedforward control前馈控制field effect transistor (FET)场效应晶体管filter滤波器,滤波环节finite automaton有限自动机finite difference method有限差分法finite element method (FEM)有限元法finite impulse response (FIR) filter有限冲激响应滤波器first-order system一阶系统fixed-point iteration method不动点迭代法flag register标志寄存器flip-flop circuit触发器电路floating-point number浮点数flow chart流程图,流程表fluid power system流体动力系统G英文中文gain增益gain margin增益裕度Galerkin method伽辽金法game theory博弈论Gauss elimination method高斯消元法Gauss-Jordan method高斯-约当法Gauss-Markov process高斯-马尔可夫过程Gauss-Seidel iteration method高斯-赛德尔迭代法genetic algorithm (GA)遗传算法gradient method梯度法,梯度下降法graph theory图论gravity gradient stabilization重力梯度稳定gray code格雷码,反向码gray level灰度,灰阶grid search method网格搜索法ground station地面站,地面控制站guidance system制导系统,导航系统gyroscope陀螺仪,陀螺仪器H英文中文H∞ control H无穷控制Hamiltonian function哈密顿函数harmonic analysis谐波分析harmonic oscillator谐振子,谐振环节Hartley transform哈特利变换Hebb learning rule赫布学习规则Heisenberg uncertainty principle海森堡不确定性原理hidden layer隐层,隐含层hidden Markov model (HMM)隐马尔可夫模型hierarchical control system分层控制系统high-pass filter高通滤波器Hilbert transform希尔伯特变换Hopfield network霍普菲尔德网络hysteresis滞后,迟滞,磁滞I英文中文identification识别,辨识identity matrix单位矩阵,恒等矩阵image processing图像处理impulse response冲激响应impulse response function冲激响应函数inadmissible control不可接受控制incremental encoder增量式编码器indefinite integral不定积分index of controllability可控性指标index of observability可观测性指标induction motor感应电动机inertial navigation system (INS)惯性导航系统inference engine推理引擎,推理机inference rule推理规则infinite impulse response (IIR) filter无限冲激响应滤波器information entropy信息熵information theory信息论input-output linearization输入输出线性化input-output model输入输出模型input-output stability输入输出稳定性J英文中文Jacobian matrix雅可比矩阵jerk加加速度,冲击joint coordinate system关节坐标系joint space关节空间Joule's law焦耳定律jump resonance跳跃共振K英文中文Kalman filter卡尔曼滤波器Karhunen-Loeve transform卡尔胡南-洛维变换kernel function核函数,核心函数kinematic chain运动链,运动链条kinematic equation运动方程,运动学方程kinematic pair运动副,运动对kinematics运动学kinetic energy动能L英文中文Lagrange equation拉格朗日方程Lagrange multiplier拉格朗日乘子Laplace transform拉普拉斯变换Laplacian operator拉普拉斯算子laser激光,激光器latent root潜根,隐根latent vector潜向量,隐向量learning rate学习率,学习速度least squares method最小二乘法Lebesgue integral勒贝格积分Legendre polynomial勒让德多项式Lennard-Jones potential莱纳德-琼斯势level set method水平集方法Liapunov equation李雅普诺夫方程Liapunov function李雅普诺夫函数Liapunov stability李雅普诺夫稳定性limit cycle极限环,极限圈linear programming线性规划linear quadratic regulator (LQR)线性二次型调节器linear system线性系统M英文中文machine learning机器学习machine vision机器视觉magnetic circuit磁路,磁电路英文中文magnetic flux磁通量magnetic levitation磁悬浮magnetization curve磁化曲线magnetoresistance磁阻,磁阻效应manipulability可操作性,可操纵性manipulator操纵器,机械手Markov chain马尔可夫链Markov decision process (MDP)马尔可夫决策过程Markov property马尔可夫性质mass matrix质量矩阵master-slave control system主从控制系统matrix inversion lemma矩阵求逆引理maximum likelihood estimation (MLE)最大似然估计mean square error (MSE)均方误差measurement noise测量噪声,观测噪声mechanical impedance机械阻抗membership function隶属函数N英文中文natural frequency固有频率,自然频率natural language processing (NLP)自然语言处理navigation导航,航行negative feedback负反馈,负反馈作用neural network神经网络neuron神经元,神经细胞Newton method牛顿法,牛顿迭代法Newton-Raphson method牛顿-拉夫逊法noise噪声,噪音nonlinear programming非线性规划nonlinear system非线性系统norm范数,模,标准normal distribution正态分布,高斯分布notch filter凹槽滤波器,陷波滤波器null space零空间,核空间O英文中文observability可观测性英文中文observer观测器,观察器optimal control最优控制optimal estimation最优估计optimal filter最优滤波器optimization优化,最优化orthogonal matrix正交矩阵oscillation振荡,振动output feedback输出反馈output regulation输出调节P英文中文parallel connection并联,并联连接parameter estimation参数估计parity bit奇偶校验位partial differential equation (PDE)偏微分方程passive attitude stabilization被动姿态稳定pattern recognition模式识别PD (proportional-derivative) control比例-微分控制peak value峰值,峰值幅度perceptron感知器,感知机performance index性能指标,性能函数period周期,周期时间periodic signal周期信号phase angle相角,相位角phase margin相位裕度phase plane analysis相平面分析phase portrait相轨迹,相图像PID (proportional-integral-derivative) control比例-积分-微分控制piezoelectric effect压电效应pitch angle俯仰角pixel像素,像元Q英文中文quadratic programming二次规划quantization量化,量子化quantum computer量子计算机quantum control量子控制英文中文queueing theory排队论quiescent point静态工作点,静止点R英文中文radial basis function (RBF) network径向基函数网络radiation pressure辐射压random variable随机变量random walk随机游走range范围,区间,距离rank秩,等级rate of change变化率,变化速率rational function有理函数Rayleigh quotient瑞利商real-time control system实时控制系统recursive algorithm递归算法recursive estimation递归估计reference input参考输入,期望输入reference model参考模型,期望模型reinforcement learning强化学习relay control system继电器控制系统reliability可靠性,可信度remote control system遥控系统,远程控制系统residual error残差误差,残余误差resonance frequency共振频率S英文中文sampling采样,取样sampling frequency采样频率sampling theorem采样定理saturation饱和,饱和度scalar product标量积,点积scaling factor缩放因子,比例系数Schmitt trigger施密特触发器Schur complement舒尔补second-order system二阶系统self-learning自学习,自我学习self-organizing map (SOM)自组织映射sensitivity灵敏度,敏感性sensitivity analysis灵敏度分析,敏感性分析sensor传感器,感应器sensor fusion传感器融合servo amplifier伺服放大器servo motor伺服电机,伺服马达servo valve伺服阀,伺服阀门set point设定值,给定值settling time定常时间,稳定时间T英文中文tabu search禁忌搜索,禁忌表搜索Taylor series泰勒级数,泰勒展开式teleoperation遥操作,远程操作temperature sensor温度传感器terminal终端,端子testability可测试性,可检测性thermal noise热噪声,热噪音thermocouple热电偶,热偶threshold阈值,门槛time constant时间常数time delay时延,延时time domain时域time-invariant system时不变系统time-optimal control时间最优控制time series analysis时间序列分析toggle switch拨动开关,切换开关tolerance analysis公差分析torque sensor扭矩传感器transfer function传递函数,迁移函数transient response瞬态响应U英文中文uncertainty不确定性,不确定度underdamped system欠阻尼系统undershoot低于量,低于值unit impulse function单位冲激函数unit step function单位阶跃函数unstable equilibrium point不稳定平衡点unsupervised learning无监督学习upper bound上界,上限utility function效用函数,效益函数V英文中文variable structure control变结构控制variance方差,变异vector product向量积,叉积velocity sensor速度传感器verification验证,校验virtual reality虚拟现实viscosity粘度,黏度vision sensor视觉传感器voltage电压,电位差voltage-controlled oscillator (VCO)电压控制振荡器W英文中文wavelet transform小波变换weighting function加权函数Wiener filter维纳滤波器Wiener process维纳过程work envelope工作空间,工作范围worst-case analysis最坏情况分析X英文中文XOR (exclusive OR) gate异或门,异或逻辑门Y英文中文yaw angle偏航角Z英文中文Z transform Z变换zero-order hold (ZOH)零阶保持器zero-order system零阶系统zero-pole cancellation零极点抵消。
MetricLearning度量学习
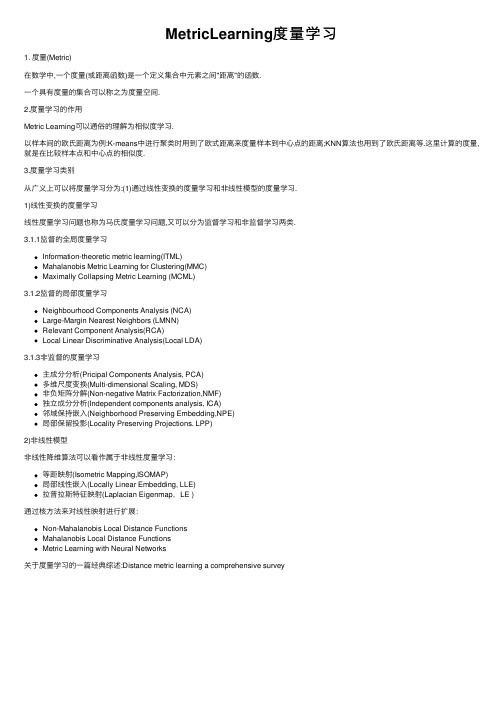
MetricLearning度量学习1. 度量(Metric)在数学中,⼀个度量(或距离函数)是⼀个定义集合中元素之间"距离"的函数.⼀个具有度量的集合可以称之为度量空间.2.度量学习的作⽤Metric Learning可以通俗的理解为相似度学习.以样本间的欧⽒距离为例:K-means中进⾏聚类时⽤到了欧式距离来度量样本到中⼼点的距离;KNN算法也⽤到了欧⽒距离等.这⾥计算的度量,就是在⽐较样本点和中⼼点的相似度.3.度量学习类别从⼴义上可以将度量学习分为:(1)通过线性变换的度量学习和⾮线性模型的度量学习.1)线性变换的度量学习线性度量学习问题也称为马⽒度量学习问题,⼜可以分为监督学习和⾮监督学习两类.3.1.1监督的全局度量学习Information-theoretic metric learning(ITML)Mahalanobis Metric Learning for Clustering(MMC)Maximally Collapsing Metric Learning (MCML)3.1.2监督的局部度量学习Neighbourhood Components Analysis (NCA)Large-Margin Nearest Neighbors (LMNN)Relevant Component Analysis(RCA)Local Linear Discriminative Analysis(Local LDA)3.1.3⾮监督的度量学习主成分分析(Pricipal Components Analysis, PCA)多维尺度变换(Multi-dimensional Scaling, MDS)⾮负矩阵分解(Non-negative Matrix Factorization,NMF)独⽴成分分析(Independent components analysis, ICA)邻域保持嵌⼊(Neighborhood Preserving Embedding,NPE)局部保留投影(Locality Preserving Projections. LPP)2)⾮线性模型⾮线性降维算法可以看作属于⾮线性度量学习:等距映射(Isometric Mapping,ISOMAP)局部线性嵌⼊(Locally Linear Embedding, LLE)拉普拉斯特征映射(Laplacian Eigenmap,LE )通过核⽅法来对线性映射进⾏扩展:Non-Mahalanobis Local Distance FunctionsMahalanobis Local Distance FunctionsMetric Learning with Neural Networks关于度量学习的⼀篇经典综述:Distance metric learning a comprehensive survey。
- 1、下载文档前请自行甄别文档内容的完整性,平台不提供额外的编辑、内容补充、找答案等附加服务。
- 2、"仅部分预览"的文档,不可在线预览部分如存在完整性等问题,可反馈申请退款(可完整预览的文档不适用该条件!)。
- 3、如文档侵犯您的权益,请联系客服反馈,我们会尽快为您处理(人工客服工作时间:9:00-18:30)。
Maximum-Margin Matrix FactorizationNathan Srebro Dept.of Computer Science University of Toronto Toronto,ON,CANADA nati@ Jason D.M.Rennie Tommi S.Jaakkola Computer Science and Artificial Intelligence Lab Massachusetts Institute of TechnologyCambridge,MA,USAjrennie,tommi@ AbstractWe present a novel approach to collaborative prediction,using low-norminstead of low-rank factorizations.The approach is inspired by,and hasstrong connections to,large-margin linear discrimination.We show howto learn low-norm factorizations by solving a semi-definite program,anddiscuss generalization error bounds for them.1IntroductionFitting a target matrix Y with a low-rank matrix X by minimizing the sum-squared error is a common approach to modeling tabulated data,and can be done explicitly in terms of the singular value decomposition of Y.It is often desirable,though,to minimize a different loss function:loss corresponding to a specific probabilistic model(where X are the mean parameters,as in pLSA[1],or the natural parameters[2]);or loss functions such as hinge loss appropriate for binary or discrete ordinal data.Loss functions other than squared-error yield non-convex optimization problems with multiple local minima.Even with a squared-error loss,when only some of the entries in Y are observed,as is the case for collaborative filtering,local minima arise and SVD techniques are no longer applicable[3].Low-rank approximations constrain the dimensionality of the factorization X=UV . Other constraints,such as sparsity and non-negativity[4],have also been suggested for better capturing the structure in Y,and also lead to non-convex optimization problems.In this paper we suggest regularizing the factorization by constraining the norm of U and V—constraints that arise naturally when matrix factorizations are viewed as feature learn-ing for large-margin linear prediction(Section2).Unlike low-rank factorizations,such constraints lead to convex optimization problems that can be formulated as semi-definite programs(Section4).Throughout the paper,we focus on using low-norm factorizations for“collaborative prediction”:predicting unobserved entries of a target matrix Y,based on a subset S of observed entries Y S.In Section5,we present generalization error bounds for collaborative prediction using low-norm factorizations.2Matrix Factorization as Feature LearningUsing a low-rank model for collaborative prediction[5,6,3]is straightforward:A low-rank matrix X is sought that minimizes a loss versus the observed entries Y S.Unobservedentries in Y are predicted according to X.Matrices of rank at most k are those that can be factored into X=UV ,U∈R n×k,V∈R m×k,and so seeking a low-rank matrix is equivalent to seeking a low-dimensional factorization.If one of the matrices,say U,isfixed,and only the other matrix V needs to be learned,then fitting each column of the target matrix Y is a separate linear prediction problem.Each row of U functions as a“feature vector”,and each column of V is a linear predictor,predicting the entries in the corresponding column of Y based on the“features”in U.In collaborative prediction,both U and V are unknown and need to be estimated.This can be thought of as learning feature vectors(rows in U)for each of the rows of Y,enabling good linear prediction across all of the prediction problems(columns of Y)concurrently, each with a different linear predictor(columns of V ).The features are learned without any external information or constraints which is impossible for a single prediction task(we would use the labels as features).The underlying assumption that enables us to do this in a collaborativefiltering situation is that the prediction tasks(columns of Y)are related,in that the same features can be used for all of them,though possibly in different ways.Low-rank collaborative prediction corresponds to regularizing by limiting the dimensional-ity of the feature space—each column is a linear prediction problem in a low-dimensional space.Instead,we suggest allowing an unbounded dimensionality for the feature space,and regularizing by requiring a low-norm factorization,while predicting with large-margin. Consider adding to the loss a penalty term which is the sum of squares of entries in U andV,i.e. U 2Fro + V 2Fro(Frodenotes the Frobenius norm).Each“conditional”problem(fitting U given V and vice versa)again decomposes into a collection of standard,this time regularized,linear prediction problems.With an appropriate loss function,or constraints on the observed entries,these correspond to large-margin linear discrimination problems. For example,if we learn a binary observation matrix by minimizing a hinge loss plus such a regularization term,each conditional problem decomposes into a collection of SVMs.3Maximum-Margin Matrix FactorizationsMatrices with a factorization X=UV ,where U and V have low Frobenius norm(recall that the dimensionality of U and V is no longer bounded!),can be characterized in several equivalent ways,and are known as low trace norm matrices:Definition1.The trace norm1 XΣis the sum of the singular values of X.Lemma1. XΣ=min X=UV UFroVFro=min X=UV 12( U 2Fro+ V 2Fro)The characterization in terms of the singular value decomposition allows us to characterize low trace norm matrices as the convex hull of bounded-norm rank-one matrices:Lemma2.{X| XΣ≤B}=convuv |u∈R n,v∈R m,|u|2=|v|2=BIn particular,the trace norm is a convex function,and the set of bounded trace norm ma-trices is a convex set.For convex loss functions,seeking a bounded trace norm matrix minimizing the loss versus some target matrix is a convex optimization problem.This contrasts sharply with minimizing loss over low-rank matrices—a non-convex prob-lem.Although the sum-squared error versus a fully observed target matrix can be min-imized efficiently using the SVD(despite the optimization problem being non-convex!), minimizing other loss functions,or even minimizing a squared loss versus a partially ob-served matrix,is a difficult optimization problem with multiple local minima[3].1Also known as the nuclear norm and the Ky-Fan n-norm.In fact,the trace norm has been suggested as a convex surrogate to the rank for various rank-minimization problems [7].Here,we justify the trace norm directly,both as a natural extension of large-margin methods and by providing generalization error bounds.To simplify presentation,we focus on binary labels,Y ∈{±1}n ×m .We consider hard-margin matrix factorization ,where we seek a minimum trace norm matrix X that matches the observed labels with a margin of one:Y ia X ia ≥1for all ia ∈S .We also consider soft-margin learning,where we minimize a trade-off between the trace norm of X and its hinge-loss relative to Y S :minimize X Σ+c ia ∈Smax(0,1−Y ia X ia ).(1)As in maximum-margin linear discrimination,there is an inverse dependence between the norm and the margin.Fixing the margin and minimizing the trace norm is equivalent to fixing the trace norm and maximizing the margin.As in large-margin discrimination with certain infinite dimensional (e.g.radial)kernels,the data is always separable with sufficiently high trace norm (a trace norm of n |S |is sufficient to attain a margin of one).The max-norm variant Instead of constraining the norms of rows in U and V on aver-age,we can constrain all rows of U and V to have small L 2norm,replacing the trace norm with X max =min X =UV (max i |U i |)(max a |V a |)where U i ,V a are rows of U,V .Low-max-norm discrimination has a clean geometric interpretation.First,note that predicting the target matrix with the signs of a rank-k matrix corresponds to mapping the “items”(columns)to points in R k ,and the “users”(rows)to homogeneous hyperplanes,such that each user’s hyperplane separates his positive items from his negative items.Hard-margin low-max-norm prediction corresponds to mapping the users and items to points and hy-perplanes in a high-dimensional unit sphere such that each user’s hyperplane separates his positive and negative items with a large-margin (the margin being the inverse of the max-norm).4Learning Maximum-Margin Matrix FactorizationsIn this section we investigate the optimization problem of learning a MMMF,i.e.a low norm factorization UV ,given a binary target matrix.Bounding the trace norm of UV by 12( U 2Fro + V 2Fro ),we can characterize the trace norm in terms of the trace of a positive semi-definite matrix:Lemma 3([7,Lemma 1]).For any X ∈R n ×m and t ∈R : X Σ≤t iff there existsA ∈R n ×n andB ∈R m ×m such that 2 A X X B0and tr A +tr B ≤2t .Proof.Note that for any matrix W , W Fro =tr W W .If A X X B 0,we can write it as a product [U V ][U V ].We have X =UV and 12( U 2Fro + V 2Fro )=12(tr A +tr B )≤t ,establishing X Σ≤t .Conversely,if X Σ≤t we can write it as X =UV with tr UU +tr V V ≤2t and consider the p.s.d.matrix UU XX V V .Lemma 3can be used in order to formulate minimizing the trace norm as a semi-definite optimization problem (SDP).Soft-margin matrix factorization (1),can be written as:min 12(tr A +tr B )+c ia ∈Sξia s.t. A X X B 0,y ia X ia ≥1−ξia ξia ≥0∀ia ∈S (2)2A 0denotes A is positive semi-definiteAssociating a dual variable Q ia with each constraint on X ia,the dual of(2)is[8,Section 5.4.2]:maxia∈S Q ia s.t.I(−Q⊗Y)(−Q⊗Y) I0,0≤Q ia≤c(3)where Q⊗Y denotes the sparse matrix(Q⊗Y)ia=Q ia Y ia for ia∈S and zeros elsewhere.The problem is strictly feasible,and there is no duality gap.The p.s.d.constraint in the dual(3)is equivalent to bounding the spectral norm of Q⊗Y,and the dual can also be written as an optimization problem subject to a bound on the spectral norm,i.e.a bound on the singular values of Q⊗Y:maxia∈S Q ia s.t.Q⊗Y2≤10≤Q ia≤c∀ia∈S(4)In typical collaborative prediction problems,we observe only a small fraction of the entries in a large target matrix.Such a situation translates to a sparse dual semi-definite program, with the number of variables equal to the number of observed rge-scale SDP solvers can take advantage of such sparsity.The prediction matrix X∗minimizing(1)is part of the primal optimal solution of(2),and can be extracted from it directly.Nevertheless,it is interesting to study how the optimal prediction matrix X∗can be directly recovered from a dual optimal solution Q∗alone. Although unnecessary when relying on interior point methods used by most SDP solvers(as these return a primal/dual optimal pair),this can enable us to use specialized optimization methods,taking advantage of the simple structure of the dual.Recovering X∗from Q∗As for linear programming,recovering a primal optimal solu-tion directly from a dual optimal solution is not always possible for SDPs.However,at least for the hard-margin problem(no slack)this is possible,and we describe below how an optimal prediction matrix X∗can be recovered from a dual optimal solution Q∗by calculating a singular value decomposition and solving linear equations.Given a dual optimal Q∗,consider its singular value decomposition Q∗⊗Y=UΛV . Recall that all singular values of Q∗⊗Y are bounded by one,and consider only the columns ˜U∈R n×p of U and˜V∈R m×p of V with singular value one.It is possible to show[8,Section5.4.3],using complimentary slackness,that for some matrix R∈R p×p,X∗=˜URR ˜V is an optimal solution to the maximum margin matrix factorization problem(1).Furthermore,p(p+1)2is bounded above by the number of non-zero Q∗ia.When Q∗ia>0,and assuming hard-margin constraints,i.e.no box constraints in the dual,complimentary slackness dictates that X∗ia=˜U i RR ˜V a=Y ia,providing us with a linear equation onthe p(p+1)2entries in the symmetric RR .For hard-margin matrix factorization,we cantherefore recover the entries of RR by solving a system of linear equations,with a number of variables bounded by the number of observed entries.Recovering specific entries The approach described above requires solving a large sys-tem of linear equations(with as many variables as observations).Furthermore,especially when the observations are very sparse(only a small fraction of the entries in the target matrix are observed),the dual solution is much more compact then the prediction matrix: the dual involves a single number for each observed entry.It might be desirable to avoidstoring the prediction matrix X∗explicitly,and calculate a desired entry X∗i0a0,or at leastits sign,directly from the dual optimal solution Q∗.Consider adding the constraint X i0a0>0to the primal SDP(2).If there exists an optimalsolution X∗to the original SDP with X∗i0a0>0,then this is also an optimal solution tothe modified SDP,with the same objective value.Otherwise,the optimal solution of the modified SDP is not optimal for the original SDP,and the optimal value of the modified SDP is higher(worse)than the optimal value of the original SDP.Introducing the constraint X i0a0>0to the primal SDP(2)corresponds to introducing anew variable Q i0a0to the dual SDP(3),appearing in Q⊗Y(with Y ia0=1)but not in theobjective.In this modified dual,the optimal solution Q∗of the original dual would alwaysbe feasible.But,if X∗i0a0<0in all primal optimal solutions,then the modified primalSDP has a higher value,and so does the dual,and Q∗is no longer optimal for the new dual. By checking the optimality of Q∗for the modified dual,e.g.by attempting to re-optimizeit,we can recover the sign of X∗i0a0 .We can repeat this test once with Y i0a0=1and once with Y ia0=−1,correspondingto X i0a0<0.If Y ia0X∗ia0<0(in all optimal solutions),then the dual solution can beimproved by introducing Q i0a0with a sign of Y ia0.Predictions for new users So far,we assumed that learning is done on the known entries in all rows.It is commonly desirable to predict entries in a new partially observed row of Y(a new user),not included in the original training set.This essentially requires solving a“conditional”problem,where V is already known,and a new row of U is learned(the predictor for the new user)based on a new partially observed row of ing maximum-margin matrix factorization,this is a standard SVM problem.Max-norm MMMF as a SDP The max-norm variant can also be written as a SDP,with the primal and dual taking the forms:min t+cia∈S ξia s.t.A XX BA ii,B aa≤t∀i,ay ia X ia≥1−ξiaξia≥0∀ia∈S(5)maxia∈S Q ia s.t.Γ(−Q⊗Y)(−Q⊗Y) ∆Γ,∆are diagonaltrΓ+tr∆=10≤Q ia≤c∀ia∈S(6)5Generalization Error Bounds for Low Norm Matrix Factorizations Similarly to standard feature-based prediction approaches,collaborative prediction meth-ods can also be analyzed in terms of their generalization ability:How confidently can we predict entries of Y based on our error on the observed entries Y S?We present here gen-eralization error bounds that holds for any target matrix Y,and for a random subset of observations S,and bound the average error across all entries in terms of the observed margin error3.The central assumption,paralleling the i.i.d.source assumption for standard feature-based prediction,is that the observed subset S is picked uniformly at random. Theorem4.For all target matrices Y∈{±1}n×m and sample sizes|S|>n log n,and for a uniformly selected sample S of|S|entries in Y,with probability at least1−δover 3The bounds presented here are special cases of bounds for general loss functions that we present and prove elsewhere[8,Section6.2].To prove the bounds we bound the Rademacher complexity of bounded trace norm and bounded max-norm matrices(i.e.balls w.r.t.these norms).The unit trace norm ball is the convex hull of outer products of unit norm vectors.It is therefore enough to bound the Rademacher complexity of such outer products,which boils down to analyzing the spectral norm of random matrices.As a consequence of Grothendiek’s inequality,the unit max-norm ball is within a factor of two of the convex hull of outer products of sign vectors.The Rademacher complexity of such outer products can be bounded by considering their cardinality.the sample selection,the following holds for all matrices X ∈R n ×m and all γ>0:1nm |{ia |X ia Y ia ≤0}|<1|S ||{ia ∈S |X ia Y ia ≤γ}|+K X Σγ√nm4√ln m (n +m )ln n |S |+ ln(1+|log X Σ/γ|)|S |+ ln(4/δ)2|S |(7)and1nm |{ia |X ia Y ia ≤0}|<1|S ||{ia ∈S |X ia Y ia ≤γ}|+12 X max γ n +m |S |+ ln(1+|log X Σ/γ|)|S |+ ln(4/δ)2|S |(8)Where K is a universal constant that does not depend on Y ,n ,m ,γor any other quantity.To understand the scaling of these bounds,consider n ×m matrices X =UV where the norms of rows of U and V are bounded by r ,i.e.matrices with X max ≤r 2.The trace norm of such matrices is bounded by r 2/√nm ,and so the two bounds agree up to log-factors—the cost of allowing the norm to be low on-average but not uniformly.Recall that the conditional problem,where V is fixed and only U is learned,is a collection of low-norm (large-margin)linear prediction problems.When the norms of rows in U and V are bounded by r ,a similar generalization error bound on the conditional problem would include the term r2γ n |S |,matching the bounds of Theorem 4up to log-factors—learningboth U and V does not introduce significantly more error than learning just one of them.Also of interest is the comparison with bounds for low-rank matrices,for which X Σ≤√rank X X Fro .In particular,for n ×m rank-k X with entries bounded by B , X Σ≤√knmB ,and the second term in the right-hand side of (7)becomes:K B γ4√ln m k (n +m )ln n |S |(9)Although this is the best (up to log factors)that can be expected from scale-sensitive bounds 4,taking a combinatorial approach,the dependence on the magnitude of the entries in X (and the margin)can be avoided [9].6Implementation and ExperimentsRatings In many collaborative prediction tasks,the labels are not binary,but rather are discrete “ratings”in several ordered levels (e.g.one star through five stars).Separating R levels by thresholds −∞=θ0<θ1<···<θR =∞,and generalizing hard-margin constraints for binary labels,one can require θY ia +1≤X ia ≤θY ia +1−1.A soft-margin version of these constraints,with slack variables for the two constraints on each observed rating,corresponds to a generalization of the hinge loss which is a convex bound on the zero/one level-agreement error (ZOE)[10].To obtain a loss which is a convex bound on the mean-absolute-error (MAE—the difference,in levels,between the predicted level and the true level),we introduce R −1slack variables for each observed rating—one for each4For general loss functions,bounds as in Theorem 4depend only on the Lipschitz constant of the loss,and (9)is the best (up to log factors)that can be achieved without explicitly bounding the magnitude of the loss function.of the R−1constraints X ia≥θr for r<Y ia and X ia≤θr for r≥Y ia.Both of these soft-margin problems(“immediate-threshold”and“all-threshold”)can be formulated as SDPs similar to(2)-(3).Furthermore,it is straightforward to learn also the thresholds (they appear as variables in the primal,and correspond to constraints in the dual)—either a single set of thresholds for the entire matrix,or a separate threshold vector for each row of the matrix(each“user”).Doing the latter allows users to“use ratings differently”and alleviates the need to normalize the data.Experiments We conducted preliminary experiments on a subset of the100K MovieLens Dataset5,consisting of the100users and100movies with the most ratings.We used CSDP [11]to solve the resulting SDPs6.The ratings are on a discrete scale of one throughfive, and we experimented with both generalizations of the hinge loss above,allowing per-user thresholds.We compared against WLRA and K-Medians(described in[12])as“Baseline”learners.We randomly split the data into four sets.For each of the four possible test sets, we used the remaining sets to calculate a3-fold cross-validation(CV)error for each method (WLRA,K-medians,trace norm and max-norm MMMF with immediate-threshold and all-threshold hinge loss)using a range of parameters(rank for WLRA,number of centers for K-medians,slack cost for MMMF).For each of the four splits,we selected the two MMMF learners with lowest CV ZOE and MAE and the two Baseline learners with lowest CV ZOE and MAE,and measured their error on the held-out test data.Table1lists these CV and test errors,and the average test error across all four test sets.On average and on three of the four test sets,MMMF achieves lower MAE than the Baseline learners;on all four of the test sets,MMMF achieves lower ZOE than the Baseline learners.Test ZOE MAESet Method CV Test Method CV Test 1WLRA rank20.5470.575K-Medians K=20.6780.691 2WLRA rank20.5500.562K-Medians K=20.6860.681 3WLRA rank10.5620.543K-Medians K=20.7000.681 4WLRA rank20.5570.553K-Medians K=20.6850.696 Avg.0.5580.687 1max-norm C=0.00120.5430.562max-norm C=0.00120.6690.677 2trace norm C=0.240.5500.552max-norm C=0.00110.6750.683 3max-norm C=0.00120.5510.527max-norm C=0.00120.6680.646 4max-norm C=0.00120.5440.550max-norm C=0.00120.6670.686 Avg.0.5480.673 Table1:Baseline(top)and MMMF(bottom)methods and parameters that achieved the lowest cross validation error(on the training data)for each train/test split,and the error for this predictor on the test data.All listed MMMF learners use the“all-threshold”objective. 7DiscussionLearning maximum-margin matrix factorizations requires solving a sparse semi-definite program.We experimented with generic SDP solvers,and were able to learn with up to tens of thousands of labels.We propose that just as generic QP solvers do not perform well on SVM problems,special purpose techniques,taking advantage of the very simple structure of the dual(3),are necessary in order to solve large-scale MMMF problems. SDPs were recently suggested for a related,but different,problem:learning the features 5/Research/GroupLens/6Solving with immediate-threshold loss took about30minutes on a3.06GHz Intel Xeon. Solving with all-threshold loss took eight to nine hours.The MATLAB code is available at /˜nati/mmmf(or equivalently,kernel)that are best for a single prediction task[13].This task is hopeless if the features are completely unconstrained,as they are in our nckriet et al suggest constraining the allowed features,e.g.to a linear combination of a few“base fea-ture spaces”(or base kernels),which represent the external information necessary to solve a single prediction problem.It is possible to combine the two approaches,seeking con-strained features for multiple related prediction problems,as a way of combining external information(e.g.details of users and of items)and collaborative information.An alternate method for introducing external information into our formulation is by adding to U and/or V additionalfixed(non-learned)columns representing the external features. This method degenerates to standard SVM learning when Y is a vector rather than a matrix. An important limitation of the approach we have described,is that observed entries are assumed to be uniformly sampled.This is made explicit in the generalization error bounds. Such an assumption is typically unrealistic,as,e.g.,users tend to rate items they like.At an extreme,it is often desirable to make predictions based only on positive samples.Even in such situations,it is still possible to learn a low-norm factorization,by using appropriate loss functions,e.g.derived from probabilistic models incorporating the observation pro-cess.However,obtaining generalization error bounds in this case is much harder.Simply allowing an arbitrary sampling distribution and calculating the expected loss based on this distribution(which is not possible with the trace norm,but is possible with the max-norm [8])is not satisfying,as this would guarantee low error on items the user is likely to want anyway,but not on items we predict he would like.Acknowledgments We would like to thank Sam Roweis for pointing out[7]. References[1]T.Hofmann.Unsupervised learning by probabilistic latent semantic analysis.Machine Learn-ing Journal,42(1):177–196,2001.[2]M.Collins,S.Dasgupta,and R.Schapire.A generalization of principal component analysis tothe exponential family.In Advances in Neural Information Processing Systems14,2002. [3]Nathan Srebro and Tommi Jaakkola.Weighted low rank approximation.In20th InternationalConference on Machine Learning,2003.[4] D.D.Lee and H.S.Seung.Learning the parts of objects by non-negative matrix factorization.Nature,401:788–791,1999.[5]tent semantic models for collaborativefiltering.ACM Trans.Inf.Syst.,22(1):89–115,2004.[6]Benjamin Marlin.Modeling user rating profiles for collaborativefiltering.In Advances inNeural Information Processing Systems,volume16,2004.[7]Maryam Fazel,Haitham Hindi,and Stephen P.Boyd.A rank minimization heuristic with appli-cation to minimum order system approximation.In Proceedings American Control Conference, volume6,2001.[8]Nathan Srebro.Learning with Matrix Factorization.PhD thesis,Massachusetts Institute ofTechnology,2004.[9]N.Srebro,N.Alon,and T.Jaakkola.Generalization error bounds for collaborative predictionwith low-rank matrices.In Advances In Neural Information Processing Systems17,2005. [10]Amnon Shashua and Anat Levin.Ranking with large margin principle:Two approaches.InAdvances in Neural Information Proceedings Systems,volume15,2003.[11] B.Borchers.CSDP,a C library for semidefinite programming.Optimization Methods andSoftware,11(1):613–623,1999.[12] B.Marlin.Collaborativefiltering:A machine learning perspective.Master’s thesis,Universityof Toronto,2004.[13]nckriet,N.Cristianini,P.Bartlett,L.El Ghaoui,and M.Jordan.Learning the kernel matrixwith semidefinite programming.Journal of Machine Learning Research,5:27–72,2004.。