图像处理_Caltech 101(加利福尼亚理工学院101类图像数据库)
2-第2章-图像处理基础(第二版)课件

1,0)
f (M 1,1)
f (0, N 1)
f (1, N 1)
f (M 1, N 1)
(2.7)
二、数字图像的表示
其中:
每个(x,y)对应数字图像中的一个基本单元,称
其为图像元素(picture element),简称为像素
(pixel);且一般取M、N和灰度级L为2的整次幂,
即:
M=2m
在景物大小不变的情况下,采样的空间分辨率 越高,获得的图像阵列M×N就越大;反之,采样的 空间分辨率越低,获得的图像阵列M×N就越小。
在空间分辨率不变的情况下,图像阵列M×N越 大,图像的尺寸就越大;反之,图像阵列M×N越小, 图像的尺寸就越小。
一、空间分辨率和灰度级分辨率
2、灰度分辨率
灰度级分辨率是指在灰度级别中可分辨的最小变 化,通常把灰度级级数 L 称为图像的灰度级分辨率。
对于消色光图像(有些文献称其为单色光图像),
f(x,y)表示图像在坐标点(x,y)的灰度值l,且:
l=f(x,y)
(2.5)
这种只有灰度属性没有彩色属性的图像称为灰
度图像。
由式(2.4),显然有:
Lmin≤l≤Lmxa
(2.6)
区间[Lmn的值为0,Lmax=L-1。这样
1 0 0 I 0 0 1
1 1 0
注意:黑白图像一定是2值图像,但2值图像不一定 是0-黑和1-白两种值。
二、数字图像的表示
4、不同类别数字图像的表示
2)灰度图像(式(2.11) 中当k=8时) 是指图像中每个像素的信息是一个量化了的灰 度级的值,没有彩色信息。
0 150 200 I 120 50 180
◆人眼对从亮突变到暗环境的适应能力称为暗适应性。 ◆人眼对亮度变化跟踪滞后的性质称为视觉惰性(或短
一种基于深度学习的禁飞区无人机目标识别方法

一种基于深度学习的禁飞区无人机目标识别方法虞晓霞;刘智;耿振野;陈思锐【摘要】随着科学技术的迅速发展,中小型无人机逐步走向民用市场.但由于监管技术的缺失,无人机擅闯禁飞区事件屡见不鲜,严重扰乱了空域交通安全.针对传统无人机探测方法成本高、效率低和适应性差等问题,引入深度学习这一新技术,提出了一种基于深度学习的禁飞区无人机目标识别方法.通过对LeNet-5模型进行结构改进,构建一个无人机特征学习网络,经过训练后得到效果良好的模型,实现无人机目标的自主识别.其识别结果可以为禁飞区的监控预警系统提供重要信息,进一步有效保障重要空域的交通安全.实验结果表明,该方法可以有效实现禁飞区无人机目标识别,且误差率比直接应用经典LeNet-5模型减小0.36%.【期刊名称】《长春理工大学学报(自然科学版)》【年(卷),期】2018(041)003【总页数】7页(P95-101)【关键词】无人机;禁飞区;深度学习;自主识别【作者】虞晓霞;刘智;耿振野;陈思锐【作者单位】长春理工大学电子信息工程学院,长春 130022;长春理工大学电子信息工程学院,长春 130022;长春理工大学电子信息工程学院,长春 130022;长春理工大学电子信息工程学院,长春 130022【正文语种】中文【中图分类】TP391近年来,随着科学技术和人工智能技术的发展,各类小型无人机日益增多,其性能不断提升,应用领域不断扩大,在方便人们生活的同时也引发了不良影响。
无人机的无序飞行时有发生,不仅严重扰乱了空域交通秩序,也引发了很多安全事故,如干扰民航机场的客机正常起飞与降落等[1-3]。
为此,空管部门通过设定禁飞区域、无线探测干扰、电磁探测、声音探测、视频监控、轨迹预测等几种主要技术手段对无人机进行管控[4-6]。
但随着无人机技术的发展,低空小型无人机越来越具有体积小、隐身化、飞行噪声低、防电磁干扰等特点,以上几种方法在探测无人机的过程中不仅体现出成本高的缺点,还具有一定程度的局限性。
加利福尼亚理工学院鸟类数据库-2011(Caltech-UCSD Birds-200-2011)

加利福尼亚理工学院鸟类数据库-2011(Caltech-UCSDBirds-200-2011)数据介绍:Caltech-UCSD Birds-200-2011 (CUB-200-2011) is an extended version of the CUB-200 dataset, with roughly double the number of images per class and new part location annotations. For detailed information about the dataset, please see the technical report linked below.■Number of categories: 200■Number of images: 11,788■Annotations per image: 15 Part Locations, 312 Binary Attributes, 1 Bounding Box关键词:边界框,粗分割,属性,鸟类探测, Bounding Box,Rough Segmentation,Attributes,detection birds,数据格式:IMAGE数据详细介绍:Caltech-UCSD Birds-200-2011BrowseClick here to browse the dataset.DetailsCaltech-UCSD Birds-200-2011 (CUB-200-2011) is an extended version of the CUB-200 dataset, with roughly double the number of images per class andnew part location annotations. For detailed information about the dataset, please see the technical report linked below.∙Number of categories: 200∙Number of images: 11,788∙Annotations per image: 15 Part Locations, 312 Binary Attributes, 1 Bounding BoxSome related datasets are Caltech-256, the Oxford Flower Dataset, and Animals with Attributes. More datasets are available at the Caltech Vision Dataset Archive.CitationIf you use CUB-200-2011 in your work, please cite the technical report:∙Wah C., Branson S., Welinder P., Perona P., Belongie S. “The Caltech-UCSD Birds-200-2011 Dataset.” Computation & NeuralSystems Technical Report, CNS-TR-2011-001. download pdfBibTeX@techreport{WahCUB_200_2011,Title = {{The Caltech-UCSD Birds-200-2011 Dataset}},Author = {Wah, C. and Branson, S. and Welinder, P. and Perona, P. and Belongie, S.},Year = {2011}Institution = {California Institute of Technology},Number = {CNS-TR-2011-001}}DownloadYou can download the dataset using the links below:∙All Images and Annotations, 1.1 gigabytes∙READMEContactContact Catherine Wah for questions about the dataset.PublicationsThe following publications use the dataset. Please contact us if you are using the dataset, and we will add your paper to the list.∙Wah C., Branson S., Perona P., Belongie S., “Intera ctive Localization and Recognition of Fine-Grained Visual Categories”, IEEE International Conference on Computer Vision (ICCV), Barcelona, 2011. pdf ∙Branson S., Perona P., Belongie S. “Strong Supervision From Weak Annotation: Interactive Training of Deformable Part Models”, IEEEInternational Conference on Computer Vision (ICCV), Barcelona, 2011.pdf, supplementary material∙Branson S., Wah C., Babenko B., Schroff F., Welinder P., Perona P., Belongie S., “Visual Recognition with Humans in the Loop”, EuropeanConference on Computer Vision (ECCV), Heraklion, Crete, Sept., 2010.pdf∙Welinder, P., Perona, P. Online crowdsourcing: rating annotators and obtaining cost-effective labels. Workshop on Advancing ComputerVision with Humans in the Loop at CVPR. 2010. pdf数据预览:点此下载完整数据集。
美国加利福尼亚州理工学院(California Institute of Technology)介绍
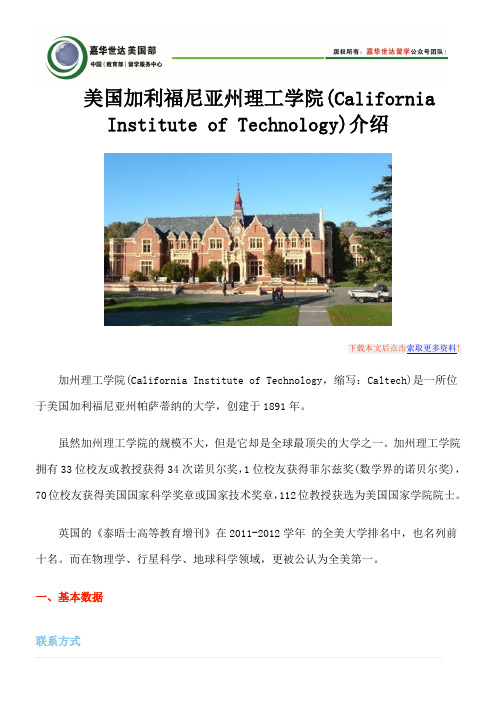
在职本科生: 男性本科生: 女性本科生: 研究生总人数: 全日制研究生: 在职研究生:
0 615 382 1,246 1,246 0
二、助学金统计数据
基本信息 助学金申请表提交截止日期: 学费担保计划: 预付学费计划: 学费分期付款计划: 州内学费: 州外学费: 助学金联系方式 电话: 电子邮箱: 网站: (626) 395-6280 finaid@ March 2 (priority) 不提供 提供 提供 $39,588 $39,588
34 - 36 暂无数据 33 - 35 (36)
五、专业介绍
结业证 副学士 学士学 硕士学 博士学 专业 书 生物科学 生物学 细胞生物学 微生物学 分子生物学 神经科学和神经生物学 学位 位 24 21 3 位 3 1 1 1 位 32 14 0 0 9 9
商务学 企业管理
-
-
1 1
-
-
计算机与信息科学 信息研究学
美国加利福尼亚州理工学院(California Institute of Technology)介绍
下载本文后点击索取更多资料!
加州理工学院(California Institute of Technology,缩写:Caltech)是一所位 于美国加利福尼亚州帕萨蒂纳的大学,创建于 1891 年。 虽然加州理工学院的规模不大,但是它却是全球最顶尖的大学之一。加州理工学院 拥有 33 位校友或教授获得 34 次诺贝尔奖, 1 位校友获得菲尔兹奖(数学界的诺贝尔奖), 70 位校友获得美国国家科学奖章或国家技术奖章, 112 位教授获选为美国国家学院院士。 英国的《泰晤士高等教育增刊》在 2011-2012 学年 的全美大学排名中,也名列前 十名。而在物理学、行星科学、地球科学领域,更被公认为全美第一。 一、基本数据
caltech101特征

caltech101特征Caltech101是一个包含101个不同类别的图像数据集,用于计算机视觉的研究和算法开发。
这个数据集由加州理工学院(Caltech)的计算机科学系创建,旨在帮助研究人员开发和评估图像分类算法。
在这个数据集中,每个类别都包含大约50到800张图像,总共有大约9,000张图像。
这些图像涵盖了各种不同的物体、动物、场景和人物,如飞机、汽车、猫、狗、椅子、花朵等等。
每个图像都有相应的标签,用于指示图像所属的类别。
对于研究人员来说,Caltech101是一个非常有用的数据集,可以用于测试和评估各种图像分类算法的性能。
通过使用这个数据集,研究人员可以比较不同算法的准确性和效率,并进一步改进他们的算法。
除了用于研究和算法开发,Caltech101也可以用于教育目的。
学生可以通过使用这个数据集来学习图像分类的基本概念和技术。
他们可以尝试不同的特征提取和分类方法,并通过与标签进行比较来评估他们的算法。
虽然Caltech101是一个非常有用的数据集,但它也有一些限制。
首先,由于数据集中每个类别的图像数量不一致,可能会导致一些类别的性能评估不准确。
其次,该数据集仅包含静态图像,而没有包含视频或动态图像。
最后,由于该数据集是由特定机构创建的,可能存在一些主观性和偏见,例如标签的选择和图像的质量。
总的来说,Caltech101是一个非常有用的图像数据集,可以用于各种计算机视觉任务。
无论是用于研究和算法开发,还是用于教育目的,这个数据集都能提供丰富多样的图像样本,有助于进一步推动计算机视觉的发展和应用。
希望未来能够有更多的数据集出现,以满足不断增长的研究和教育需求。
caltech数据集格式
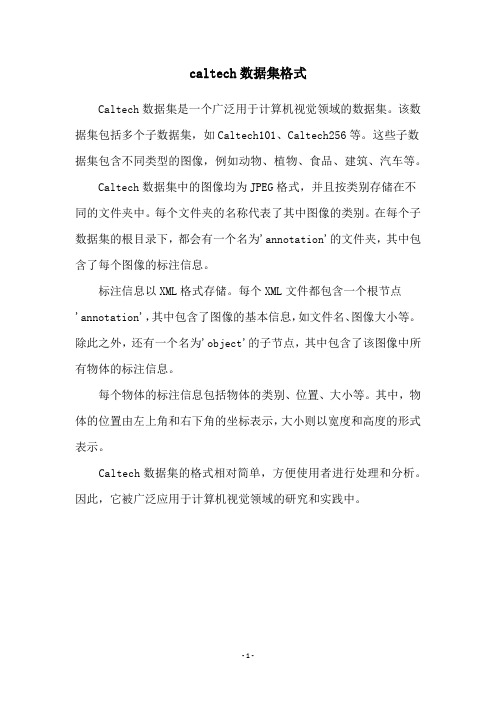
caltech数据集格式
Caltech数据集是一个广泛用于计算机视觉领域的数据集。
该数据集包括多个子数据集,如Caltech101、Caltech256等。
这些子数据集包含不同类型的图像,例如动物、植物、食品、建筑、汽车等。
Caltech数据集中的图像均为JPEG格式,并且按类别存储在不同的文件夹中。
每个文件夹的名称代表了其中图像的类别。
在每个子数据集的根目录下,都会有一个名为'annotation'的文件夹,其中包含了每个图像的标注信息。
标注信息以XML格式存储。
每个XML文件都包含一个根节点
'annotation',其中包含了图像的基本信息,如文件名、图像大小等。
除此之外,还有一个名为'object'的子节点,其中包含了该图像中所有物体的标注信息。
每个物体的标注信息包括物体的类别、位置、大小等。
其中,物体的位置由左上角和右下角的坐标表示,大小则以宽度和高度的形式表示。
Caltech数据集的格式相对简单,方便使用者进行处理和分析。
因此,它被广泛应用于计算机视觉领域的研究和实践中。
- 1 -。
caltech101 transfer learning -回复
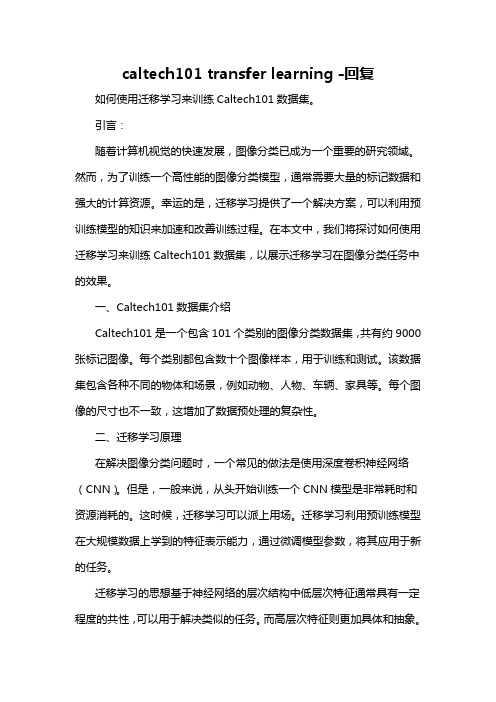
caltech101 transfer learning -回复如何使用迁移学习来训练Caltech101数据集。
引言:随着计算机视觉的快速发展,图像分类已成为一个重要的研究领域。
然而,为了训练一个高性能的图像分类模型,通常需要大量的标记数据和强大的计算资源。
幸运的是,迁移学习提供了一个解决方案,可以利用预训练模型的知识来加速和改善训练过程。
在本文中,我们将探讨如何使用迁移学习来训练Caltech101数据集,以展示迁移学习在图像分类任务中的效果。
一、Caltech101数据集介绍Caltech101是一个包含101个类别的图像分类数据集,共有约9000张标记图像。
每个类别都包含数十个图像样本,用于训练和测试。
该数据集包含各种不同的物体和场景,例如动物、人物、车辆、家具等。
每个图像的尺寸也不一致,这增加了数据预处理的复杂性。
二、迁移学习原理在解决图像分类问题时,一个常见的做法是使用深度卷积神经网络(CNN)。
但是,一般来说,从头开始训练一个CNN模型是非常耗时和资源消耗的。
这时候,迁移学习可以派上用场。
迁移学习利用预训练模型在大规模数据上学到的特征表示能力,通过微调模型参数,将其应用于新的任务。
迁移学习的思想基于神经网络的层次结构中低层次特征通常具有一定程度的共性,可以用于解决类似的任务。
而高层次特征则更加具体和抽象。
因此,我们可以将预训练的卷积部分保持不变,而仅训练密集连接层以适应新任务。
三、准备工作在开始使用迁移学习之前,我们需要完成以下准备工作:1. 下载Caltech101数据集:从官方网站或其他合法来源下载Caltech101数据集,并解压到合适的文件夹中。
2. 预处理数据:由于Caltech101数据集的图像尺寸不一致,我们需要将所有图像调整为固定的尺寸,例如224x224。
此外,还可以进行数据增强操作,例如随机剪裁、水平翻转等,以增加数据的多样性。
四、构建模型在使用迁移学习时,我们可以选择使用已经在大规模数据集上训练好的模型,例如ImageNet上的模型。
图像处理_Caltech 101(加利福尼亚理工学院101类图像数据库)

Caltech 101(加利福尼亚理工学院101类图像数据库)数据摘要:Pictures of objects belonging to 101 categories. About 40 to 800 images per category. Most categories have about 50 images. Collected in September 2003 by Fei-Fei Li, Marco Andreetto, and Marc 'Aurelio Ranzato. The size of each image is roughly 300 x 200 pixels.We have carefully clicked outlines of each object in these pictures, these are included under the 'Annotations.tar'. There is also a matlab script to view the annotaitons, 'show_annotations.m'.The Caltech 101 dataset consists of a total of 9146 images, split between 101 different object categories, as well as an additionalbackground/clutter category.Each object category contains between 40 and 800 images on average. Common and popular categories such as faces tend to have a larger number of images than less used categories. Each image is about 300x200 pixels in dimension. Images of oriented objects such as airplanes and motorcycles were mirrored to be left-right aligned, and vertically oriented structures such as buildings were rotated to be off axis.中文关键词:识别,多类,分类,标注,轮廓,英文关键词:Recognition,Multi class,Categories,Annotations,Outline,数据格式:IMAGE数据用途:To train and test several Computer Vision recognition and classification algorithms.数据详细介绍:Caltech 101DescriptionPictures of objects belonging to 101 categories. About 40 to 800 images per category. Most categories have about 50 images. Collected in September 2003 by Fei-Fei Li, Marco Andreetto, and Marc 'Aurelio Ranzato. The size of each image is roughly 300 x 200 pixels.We have carefully clicked outlines of each object in these pictures, these are included under the 'Annotations.tar'. There is also a matlab script to view the annotaitons, 'show_annotations.m'.How to use the datasetIf you are using the Caltech 101 dataset for testing your recognition algorithm you should try and make your results comparable to the results of others. We suggest training and testing on fixed number of pictures and repeating theexperiment with different random selections of pictures in order to obtain error bars. Popular number of training images: 1, 3, 5, 10, 15, 20, 30. Popular numbers of testing images: 20, 30. See also the discussion below.When you report your results please keep track of which images you used and which were misclassified. We will soon publish a more detailed experimental protocol that allows you to report those details. See the Discussion section for more details.LiteraturePapers reporting experiments on Caltech 101 images:1. Learning generative visual models from few training examples: an incremental Bayesian approach tested on 101 object categories. L. Fei-Fei, R. Fergus, and P. Perona. CVPR 2004, Workshop on Generative-Model Based Vision. 20042. Shape Matching and Object Recognition using Low Distortion Correspondence. Alexander C. Berg, Tamara L. Berg, Jitendra Malik. CVPR 20053. The Pyramid Match Kernel:Discriminative Classification with Sets of Image Features. K. Grauman and T. Darrell. International Conference on Computer Vision (ICCV), 2005.4. Combining Generative Models and Fisher Kernels for Object Class Recognition Holub, AD. Welling, M. Perona, P. International Conference on Computer Vision (ICCV), 2005.5. Object Recognition with Features Inspired by Visual Cortex. T. Serre, L. Wolf and T. Poggio. Proceedings of 2005 IEEE Computer Society Conference on Computer Vision and Pattern Recognition (CVPR 2005), IEEE Computer Society Press, San Diego, June 2005.6. SVM-KNN: Discriminative Nearest Neighbor Classification for Visual Category Recognition. Hao Zhang, Alex Berg, Michael Maire, Jitendra Malik. CVPR, 2006.7. Beyond Bags of Features: Spatial Pyramid Matching for Recognizing Natural Scene Categories. Svetlana Lazebnik, Cordelia Schmid, and Jean Ponce. CVPR, 2006 (accepted).8. Empirical study of multi-scale filter banks for object categorization, M.J. Marín-Jiménez, and N. Pérez de la Blanca. December 2005. Tech Report.9. Multiclass Object Recognition with Sparse, Localized Features, Jim Mutch and David G. Lowe. , pg. 11-18, CVPR 2006, IEEE Computer Society Press, New York, June 2006.10. Using Dependant Regions or Object Categorization in a Generative Framework, G. Wang, Y. Zhang, and L. Fei-Fei. IEEE Comp. Vis. Patt. Recog. 2006数据预览:点此下载完整数据集。
- 1、下载文档前请自行甄别文档内容的完整性,平台不提供额外的编辑、内容补充、找答案等附加服务。
- 2、"仅部分预览"的文档,不可在线预览部分如存在完整性等问题,可反馈申请退款(可完整预览的文档不适用该条件!)。
- 3、如文档侵犯您的权益,请联系客服反馈,我们会尽快为您处理(人工客服工作时间:9:00-18:30)。
Caltech 101(加利福尼亚理工学院101类图像数据库)数据摘要:Pictures of objects belonging to 101 categories. About 40 to 800 images per category. Most categories have about 50 images. Collected in September 2003 by Fei-Fei Li, Marco Andreetto, and Marc 'Aurelio Ranzato. The size of each image is roughly 300 x 200 pixels.We have carefully clicked outlines of each object in these pictures, these are included under the 'Annotations.tar'. There is also a matlab script to view the annotaitons, 'show_annotations.m'.The Caltech 101 dataset consists of a total of 9146 images, split between 101 different object categories, as well as an additionalbackground/clutter category.Each object category contains between 40 and 800 images on average. Common and popular categories such as faces tend to have a larger number of images than less used categories. Each image is about 300x200 pixels in dimension. Images of oriented objects such as airplanes and motorcycles were mirrored to be left-right aligned, and vertically oriented structures such as buildings were rotated to be off axis.中文关键词:识别,多类,分类,标注,轮廓,英文关键词:Recognition,Multi class,Categories,Annotations,Outline,数据格式:IMAGE数据用途:To train and test several Computer Vision recognition and classification algorithms.数据详细介绍:Caltech 101DescriptionPictures of objects belonging to 101 categories. About 40 to 800 images per category. Most categories have about 50 images. Collected in September 2003 by Fei-Fei Li, Marco Andreetto, and Marc 'Aurelio Ranzato. The size of each image is roughly 300 x 200 pixels.We have carefully clicked outlines of each object in these pictures, these are included under the 'Annotations.tar'. There is also a matlab script to view the annotaitons, 'show_annotations.m'.How to use the datasetIf you are using the Caltech 101 dataset for testing your recognition algorithm you should try and make your results comparable to the results of others. We suggest training and testing on fixed number of pictures and repeating theexperiment with different random selections of pictures in order to obtain error bars. Popular number of training images: 1, 3, 5, 10, 15, 20, 30. Popular numbers of testing images: 20, 30. See also the discussion below.When you report your results please keep track of which images you used and which were misclassified. We will soon publish a more detailed experimental protocol that allows you to report those details. See the Discussion section for more details.LiteraturePapers reporting experiments on Caltech 101 images:1. Learning generative visual models from few training examples: an incremental Bayesian approach tested on 101 object categories. L. Fei-Fei, R. Fergus, and P. Perona. CVPR 2004, Workshop on Generative-Model Based Vision. 20042. Shape Matching and Object Recognition using Low Distortion Correspondence. Alexander C. Berg, Tamara L. Berg, Jitendra Malik. CVPR 20053. The Pyramid Match Kernel:Discriminative Classification with Sets of Image Features. K. Grauman and T. Darrell. International Conference on Computer Vision (ICCV), 2005.4. Combining Generative Models and Fisher Kernels for Object Class Recognition Holub, AD. Welling, M. Perona, P. International Conference on Computer Vision (ICCV), 2005.5. Object Recognition with Features Inspired by Visual Cortex. T. Serre, L. Wolf and T. Poggio. Proceedings of 2005 IEEE Computer Society Conference on Computer Vision and Pattern Recognition (CVPR 2005), IEEE Computer Society Press, San Diego, June 2005.6. SVM-KNN: Discriminative Nearest Neighbor Classification for Visual Category Recognition. Hao Zhang, Alex Berg, Michael Maire, Jitendra Malik. CVPR, 2006.7. Beyond Bags of Features: Spatial Pyramid Matching for Recognizing Natural Scene Categories. Svetlana Lazebnik, Cordelia Schmid, and Jean Ponce. CVPR, 2006 (accepted).8. Empirical study of multi-scale filter banks for object categorization, M.J. Marín-Jiménez, and N. Pérez de la Blanca. December 2005. Tech Report.9. Multiclass Object Recognition with Sparse, Localized Features, Jim Mutch and David G. Lowe. , pg. 11-18, CVPR 2006, IEEE Computer Society Press, New York, June 2006.10. Using Dependant Regions or Object Categorization in a Generative Framework, G. Wang, Y. Zhang, and L. Fei-Fei. IEEE Comp. Vis. Patt. Recog. 2006数据预览:点此下载完整数据集。