高级数据库系统---L5_IR
ir文件基础知识
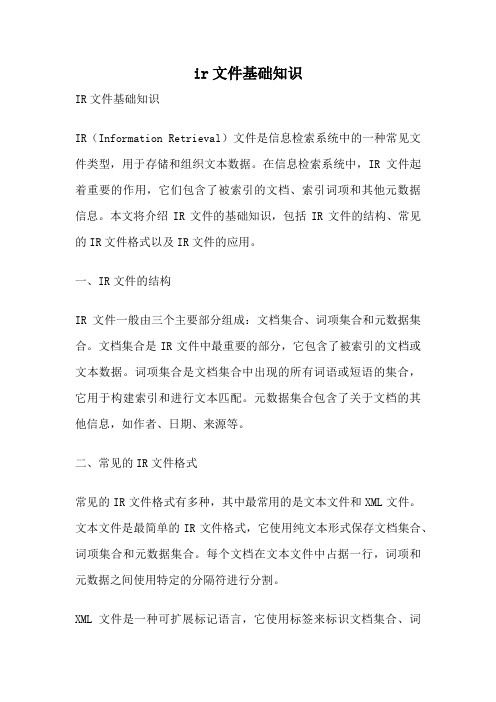
ir文件基础知识IR文件基础知识IR(Information Retrieval)文件是信息检索系统中的一种常见文件类型,用于存储和组织文本数据。
在信息检索系统中,IR文件起着重要的作用,它们包含了被索引的文档、索引词项和其他元数据信息。
本文将介绍IR文件的基础知识,包括IR文件的结构、常见的IR文件格式以及IR文件的应用。
一、IR文件的结构IR文件一般由三个主要部分组成:文档集合、词项集合和元数据集合。
文档集合是IR文件中最重要的部分,它包含了被索引的文档或文本数据。
词项集合是文档集合中出现的所有词语或短语的集合,它用于构建索引和进行文本匹配。
元数据集合包含了关于文档的其他信息,如作者、日期、来源等。
二、常见的IR文件格式常见的IR文件格式有多种,其中最常用的是文本文件和XML文件。
文本文件是最简单的IR文件格式,它使用纯文本形式保存文档集合、词项集合和元数据集合。
每个文档在文本文件中占据一行,词项和元数据之间使用特定的分隔符进行分割。
XML文件是一种可扩展标记语言,它使用标签来标识文档集合、词项集合和元数据集合。
XML文件具有良好的结构性和可读性,方便解析和处理。
每个文档、词项和元数据都以标签的形式出现,标签之间可以嵌套表示层次结构。
三、IR文件的应用IR文件在信息检索系统中有广泛的应用。
首先,IR文件被用于构建索引,以加快文本检索的速度。
索引是一种数据结构,用于存储文档和词项之间的对应关系。
通过索引,可以快速定位包含特定词项的文档。
IR文件被用于计算文本相似度。
文本相似度是衡量两个文本之间相似程度的指标,常用于文本匹配、信息聚类和信息过滤等任务。
通过比较文档集合和词项集合,可以计算文本之间的相似度,并进行相应的处理。
IR文件还被用于构建推荐系统和文本挖掘。
推荐系统根据用户的兴趣和行为,向用户推荐相关的文档或信息。
文本挖掘是从大规模文本数据中提取有用信息的技术,如情感分析、关键词提取和文本分类等。
系统服务
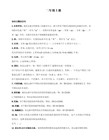
Network Connections 网络连接服务,管理网络和拨号网络,上网和局域网都需要这个服务。 svchost.exe ★★★必须! 手动 |{
3.禁止所有的“不需要”Байду номын сангаас服务将节省12-70M的内存,这将根据你的系统而定。[
第五步:记住先把你的重要资料保存好,跟我来做个实验。在Windows XP下,按下Ctrl+Alt+Del组合键,在弹出的任务管理器里面结束svchost.exe,那么就会弹出一个窗口,开始1分钟倒计时,1分钟后,系统自动重新启动。[O
附表一 显示的服务名称 这是什么东西? 调用的进程 你需要吗? 最安全设置 &}Hp
Alerter 当系统发生故障时向管理员发送错误警报。 services.exe ☆不需要 禁用 Jb2P!
Application Layer Gateway Service 给与第三者网络共享/防火墙支持的服务,有些防火墙/网络共享软件需要。占用 1.5mb内存。 ★看情况,可选。 手动 /
DHCP Client 使用静态IP的用户需要,使用Modem的用户就关了它吧。 svchost.exe ★看情况,可选。 自动 ~
Distributed Link Tracking Client 用于局域网更新连接信息,比如在电脑A有个文件,在电脑B做了个连接,如果文件移动了,这个服务将会更新信息。占用4兆内存。 svchost.exe ☆不需要 手动 :%
IPSEC Services IP安全策略代理服务。大部分用户根本用不到! lsass.exe ☆不需要 禁用 vXh>
[VIP专享]关于无法访问拒绝访问的解决方法
![[VIP专享]关于无法访问拒绝访问的解决方法](https://img.taocdn.com/s3/m/9ee39d51c5da50e2534d7f00.png)
安全权限给删了,导致无法打开
选中你当前使用的用户或者Administrators用户组选中下面的“替换子容器及对象的所有者”
点击“确定”
然后系统会覆写安全权限,等覆写完毕之后,你就可以从文件属性的安全页面看到你当前的用户或者Admin用户组了,选中可以看到下面的权限是完全控制权限
然后点击“高级”,添加system和creator owner 两个特殊用户组并修改他们的权限为完全控制,注意一定要做这个,不然你的系统就无法读取或修改这个文件了,这里所说的系统不是你的用户,而是windows 系统,从某一方面来说,系统的权限要比管理员admin组的权限要高,所以必须要添加system用户组的完全控制权限,以确保windows正常读取、运行、修改文件
修改完毕之后点击“确定”,系统可能会出现一段时间的延迟,这是在保存用户安全权限,你就不要管了,
去做别的就可以了。
具体的添加用户权限操作如下:
点击“添加”,在弹出的“选择用户或组”窗口中选择左下角的“高级”,弹出如下图窗口,点击右侧的“立即查找”,系统会在下侧的窗口中显示出目前计算机中的所有用户和组,选中要添加的用户或组,比如这里的
“system”组点击“确定”
然后在权限修改页面中选中你想要的权限,比如这里的“完全控制”,其中注意修改“应用到”选项,如果你只是修改当前文件的权限,而不修改它下层的文件属性,那么就要进行相应的修改,即应该选择为“只有该文件
夹”,修改完毕后点击“确定”
同理添加其他用户或组并修改其相应权限即可。
HS0038红外接受电路设计与应用
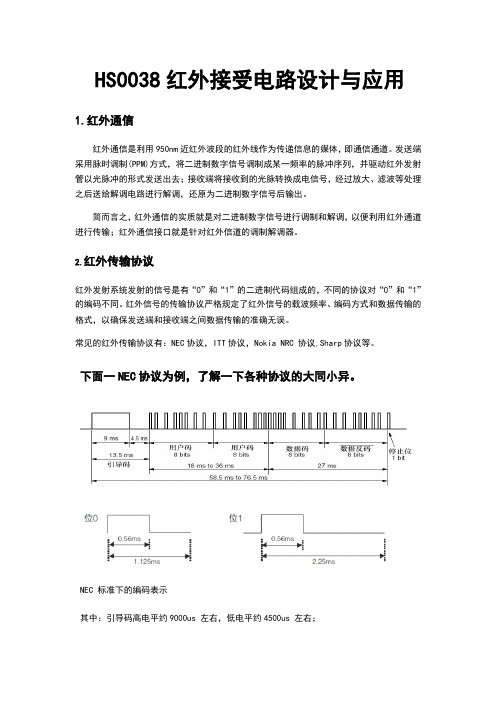
HS0038红外接受电路设计与应用1.红外通信红外通信是利用950nm近红外波段的红外线作为传递信息的媒体,即通信通道。
发送端采用脉时调制(PPM)方式,将二进制数字信号调制成某一频率的脉冲序列,并驱动红外发射管以光脉冲的形式发送出去;接收端将接收到的光脉转换成电信号,经过放大、滤波等处理之后送给解调电路进行解调,还原为二进制数字信号后输出。
简而言之,红外通信的实质就是对二进制数字信号进行调制和解调,以便利用红外通道进行传输;红外通信接口就是针对红外信道的调制解调器。
2.红外传输协议红外发射系统发射的信号是有“0”和“1”的二进制代码组成的,不同的协议对“0”和“1”的编码不同。
红外信号的传输协议严格规定了红外信号的载波频率、编码方式和数据传输的格式,以确保发送端和接收端之间数据传输的准确无误。
常见的红外传输协议有:NEC协议,ITT协议,Nokia NRC 协议,Sharp协议等。
下面一NEC协议为例,了解一下各种协议的大同小异。
NEC 标准下的编码表示其中:引导码高电平约9000us 左右,低电平约4500us 左右;用户码16 位,数据码16 位,共32位;数据0 是用“高电平约560us +低电平约560us”表示。
数据1 可用“高电平约560us+低电平约1680us”表示。
*其实自己在做红外系统时,借助示波器,可以编写自己独特的红外协议。
但要尊守一点,要以38KHz的方波来驱动红外发射LED,同时要把这38KHz的波形斩断,也就是编码。
对应的接收管会在接收到38KHz的红外信号时输出低电平,没有信号就输出高电平。
简介:红外线接收器 HS0038参数参数符号测试条件Min Typ Max单位工作电压Vcc V接收距离L L5IR=300mA1214M 测试信号载波频率f038K HZ 接收角度O1/2距离衰减1/2十/-45Deg BMP宽度F BW一3Db andwidth25KHz静态电流Icc无信号输入时mA 低电平输出Vo L Vin=OV Vcc=5V V 高电平输出Vo H Vcc=5V V 输出脉冲Tpw L Vin=500μ Vp-p500600700μS 宽度Tpw H Vin=50μ Vp-p500600700μS 光轴上测试,以宽度为600/900μS为发射脉冲,在5CM之接收范围内,取50次接脉冲之平均值1).特性a)光电检测和前置放大器集成在同一封装上。
浅谈数据库的建设与应用毕业论文
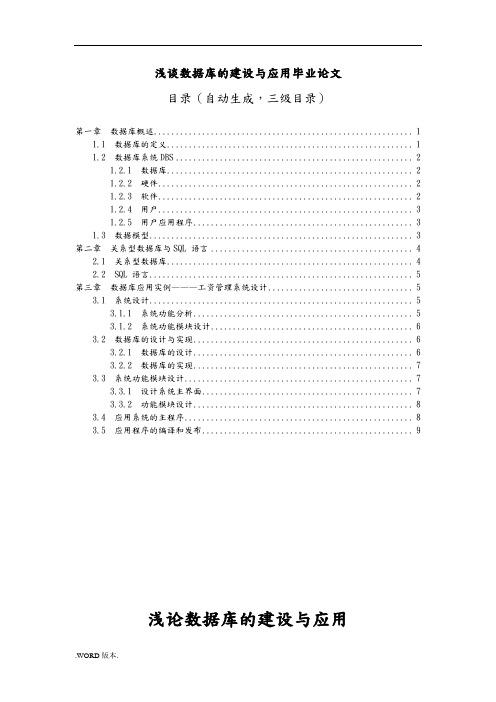
浅谈数据库的建设与应用毕业论文目录(自动生成,三级目录)第一章数据库概述 (1)1.1 数据库的定义 (1)1.2 数据库系统DBS (2)1.2.1 数据库 (2)1.2.2 硬件 (2)1.2.3 软件 (2)1.2.4 用户 (3)1.2.5 用户应用程序 (3)1.3 数据模型 (3)第二章关系型数据库与SQL 语言 (4)2.1 关系型数据库 (4)2.2 SQL 语言 (5)第三章数据库应用实例———工资管理系统设计 (5)3.1 系统设计 (5)3.1.1 系统功能分析 (5)3.1.2 系统功能模块设计 (6)3.2 数据库的设计与实现 (6)3.2.1 数据库的设计 (6)3.2.2 数据库的实现 (7)3.3 系统功能模块设计 (7)3.3.1 设计系统主界面 (7)3.3.2 功能模块设计 (8)3.4 应用系统的主程序 (8)3.5 应用程序的编译和发布 (9)浅论数据库的建设与应用摘要:随着信息技术的不断发展,数据库已经成为信息化社会中信息资源管理与开发利用的基础。
文章从对数据库的基本概念开始,介绍了目前最为流行的关系型数据库以及被称为“公共数据库语言”的SQL ,同时,给出应用实例并对其作了详细的分析,最后阐述了数据库技术发展的趋势。
关键词:数据库;数据库管理系统;数据库系统英文摘要:限优秀论文随着生产技术的发展,计算机已广泛应用于社会的各个方面,信息管理也发展到自动化、网络化和社会化的阶段。
计算机从科学计算领域诞生,随着计算机技术的蓬勃发展,在计算机的三大主要应用(科学计算、过程控制和数据处理) 领域中,数据处理已成为计算机应用的主要方面。
数据库技术的应用围不断扩大,不仅应用于数据库处理,而且进一步应用到情报检索、人工智能、专家系统、计算机辅助设计等,涉及到非数值计算机各个方面。
可以说,数据库系统已成为计算机应用系统的重要组成部分之一。
第一章数据库概述1.1 数据库的定义数据库是存储在一起的相关数据的集合。
Microsoft证书分类
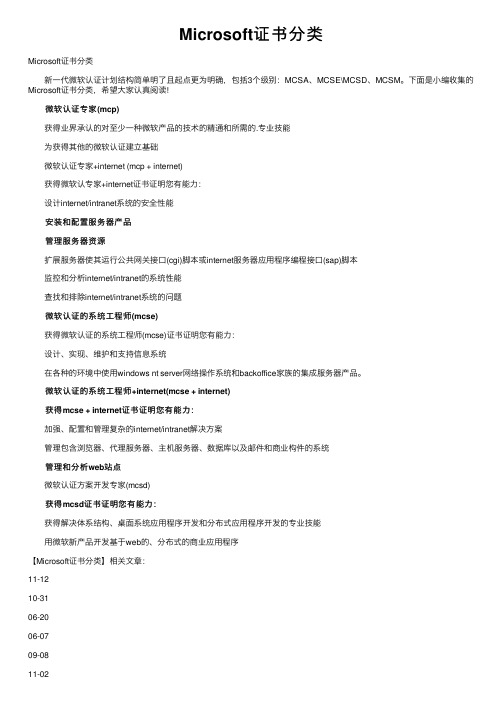
Microsoft证书分类Microsoft证书分类 新⼀代微软认证计划结构简单明了且起点更为明确,包括3个级别:MCSA、MCSE\MCSD、MCSM。
下⾯是⼩编收集的Microsoft证书分类,希望⼤家认真阅读! 微软认证专家(mcp) 获得业界承认的对⾄少⼀种微软产品的技术的精通和所需的.专业技能 为获得其他的微软认证建⽴基础 微软认证专家+internet (mcp + internet) 获得微软认专家+internet证书证明您有能⼒: 设计internet/intranet系统的安全性能 安装和配置服务器产品 管理服务器资源 扩展服务器使其运⾏公共⽹关接⼝(cgi)脚本或internet服务器应⽤程序编程接⼝(sap)脚本 监控和分析internet/intranet的系统性能 查找和排除internet/intranet系统的问题 微软认证的系统⼯程师(mcse) 获得微软认证的系统⼯程师(mcse)证书证明您有能⼒: 设计、实现、维护和⽀持信息系统 在各种的环境中使⽤windows nt server⽹络操作系统和backoffice家族的集成服务器产品。
微软认证的系统⼯程师+internet(mcse + internet) 获得mcse + internet证书证明您有能⼒: 加强、配置和管理复杂的internet/intranet解决⽅案 管理包含浏览器、代理服务器、主机服务器、数据库以及邮件和商业构件的系统 管理和分析web站点 微软认证⽅案开发专家(mcsd) 获得mcsd证书证明您有能⼒: 获得解决体系结构、桌⾯系统应⽤程序开发和分布式应⽤程序开发的专业技能 ⽤微软新产品开发基于web的、分布式的商业应⽤程序【Microsoft证书分类】相关⽂章:11-1210-3106-2006-0709-0811-0208-2308-2211-02向你推荐的相关⽂章相关⽂章列表11-1210-3106-2006-0709-0811-0208-2308-2211-02m 0 L 8 g 4 h 5 h G w z p 9 y N a E c T v k 4 + 3 s A o O 7 v o a 6 7 j 7 L 2 a I z y U E S J U v K l W C n 7 U P I 6 e 0 x H R 7 a S P y d + s 3 2 c / j 0 A Z S N U 1 M o a d I x S O C s F d M U u i m I + i 4 8 B 1 N G M A d S x G k b G A A w g G q e I A d T B j A H U s Y o B G L D q m C I G U E c 5 B l D H K g Z g w K p j i h h A H e U Y Q B 2 r G I A B q 4 4 p Y g B 1 l F s M Q H m 4 o 2 M 6 7 u E 0 p x s N S l 5 1 + r R I 5 R G Q P l o q + 1 b y 2 k K A 5 j r j S c l J G U O Z p H u U + H B 9 D O g s H C e 4 L h A 7 T h w q E O W V v O M z e n d e M Y C a M m I A G z j F A B 5 B i Q G 8 i k Q 5 c J Q x t T L + G + X S 7 t S Q 0 w H U C i Q d w K u M p B P n 4 2 N T j 3 Q u W h y 5 A m z b g O 2 L Q L k C U H 8 / N 1 4 5 o W h R 0 a K 9 7 V j J K 1 e A m l Y k P t I B p A P 4 Q i A G U N O C 0 8 i U 9 5 L R m B h A 3 g D u C C g n b Q z g B x o A / Y 1 A t N V X H p 0 U J x u 5 4 k p R 1 x q 3 Y 1 E U K + U t Q z G T Y 7 s 6 N n o l 5 5 S P Y z u t j Z 5 + D y A G U H d 9 l 8 n U a K t F U c H F A K 7 1 m F l j + V h U D A D g R w t q d h 8 E y 8 q h N N 8 Y Q A z g X w R u n 8 m g 3 w q c K 8 C r g G j L K V f 7 x s A Y w H E + K I Z d j 4 A u n a Q D A E g 6 x Q C W l U N p v u k A 0 g G k A 5 i U G y 2 o X A F k 7 z p t I O 3 I n J w r c 5 0 G x P 8 T S x 2 A a y O U j B k Y r p y U F m 7 1 y e n E c Y Q x x V f 5 d E A Z Q w u k Y 4 1 R T k 6 e X H z M 8 B u + A d D F r w j I d O P w K 6 k x g P p d + 6 o f / b o 0 r e i K m t g t n u a r m E 8 M 4 I k Z + s g 5 I 8 p 5 E i n k U t G 5 B B c D o M h v x 7 v 4 S A e w g Q A t z n Q A 6 Q A q Z e 0 0 6 h h A 8 X S u E P M c E w M 4 / h p O M V T e X j q 4 V d a 4 4 p V X M Z 9 c A Y o m k w 4 g H U D F K J Q i P P t R d n o F o D 8 N q N y R X R u s E H B W T I c B K O K h b a K C D 8 1 L e Q O g e T n 3 T f f n f P f p W D s G Q N U F 3 g y u K g b D l u 9 T U J H G A O r X K 4 q t o r c Y g K E a 0 g H U Q Y w B x A B K a n G 2 c a U F D w T F A O r g x Q B i A C W 1 x A A e Y X K 2 g y U C i k E 0 r x h A D K A k r R h A D K A k l I 0 g p 3 a o w S n 3 8 I 7 H c 2 U f + G N A Z R G V 5 O d x H S C O c u 3 Y 9 1 V F T T F R 9 q F 8 p 8 C l q y u u P d u b g u + Q w 9 H v A 3 A W m 4 s o Z 0 4 U x B j A K 4 v O w n H O R f V 2 x b V j A K D t U 4 o z B l C / p 6 Y D o J Z S j + / Q 7 i y b X A G K XC l E F a e + h 1 F T m h I 7 + G l H 5 8 n i PD m d c 1 H c r 7 i 2 k 6 c Y A O g m 6 G l H x d Z G b A y g TE 0 M A B R I x 0 k4 Y i5 v A G V N D / 8 C j 9 O A n I X j n K u O 0 p / I K67 t 5 C k d A D C 4 d A D 18 n E W j n O u + g 5 i A P h v A9 J T + N 3 u t V Q 8 s x O E d j L O t S l P t 7 V d X C l d o p K v g t f W G G X f 1 L B o v P N 9 Z / r F r L N / G l A B 1 3 k K O 9 d 3 5 e X M i Q p L + U o z L b Q Y w C t i l K c Y w A Y C T m F R U X c R Q v O i w o o B v C K s G L I L d + f a S s e Z K w C t O I M x K a T T 7 k N p q V 1 5 O Y 1 a m Y t S q u w 7 B v C E M g W E k q T c t Z 3 3 2 n Q A d c a U o l U M q 5 7 R P D I G A E 4 1 S p Q C L j 3 t Z v Q 6 1 3 f l 5 c y J G m + u A L k C / I u A 7 Q q g u D E 9 K a j 5 K D l 1 F K d y V 6 N 7 o c Z A 5 3 f H U y 0 o 6 1 N u F b O k u D v 3 T f f 3 2 V W 7 P g V Q C K G b j w H U U a Z C r M 9 8 T i T V g p I F L Z A Y w A b K F M S O u 7 N T P F f c n y L 2 G M D x V j 8 G E A N Q a u 8 + h h p T h / k 4 1 z g E z t N g i p W y N t 1 7 D C A G o O g s B i C g F g M Q Q H s a Q g 0 u b w B v Y n C K N H I F y B W g o p u l j 4 C V B I / G d J w s o x z p o + X 0 O 9 v G v 2 Z M M a X 7 m M 2 v n F I 0 X x p / R Y 1 8 n s 7 w R 7 o V n m I A V C 0 g n h I S A w D g G k N p o R m X n v 4 A F s 2 L 6 m 3 5 F c A J 5 G g u C q I z J 0 p I D M C J f n 2 u K 2 o k H U C d v 2 n k F c l V 7 u d 0 j H P f 1 M h y B a i L V z F 9 1 5 U z H U C d J y m S F o4 i B r q G s h H n G n k D e G R A 4 T w G A F T s P A n B s p + h t H A U M d A 1 6 B 6 U f a Q D q K O s c L 7 U A O p b 2 4 / s E O 9 + F n V H p n N R 8 1 F O R 7 r G 6 u L s u M q 4 d N V R n F R T s 7 c B Z d / 4 U w A l Y a d j O d f f m k s p w l F O t D i V t e k a M Y C 6 g m I A d a y k S M W x p I X A I K U I Y w B 1 g N M B 1 L G i u l L q K R 3 A E 8 o x g O M C n c 0 Q A z i O r x P D G E A M 4 I 6 A 0 / w 6 T q + z r5 a 5 A h w 3 q + k M S s t y c k q 2 X 4 0 9 e6 y h w l V O V A W n G M A j a j E A R U V g T A y g L j h 6 o g I a 0 g E M w P r R B k A F p L x G 0 x O H 3 n 1 m p7 B r 7 R l O T o N z z U X 3 r W C o a I F 2 R c 6 f 1 a d a d8 Y 7 T U b J a / g G Q C d T S K d i j A G 8 s k J x p 5 j H A G g l s P g Y A M A r B h A D + E I g H Q A o n E l o O o A i j v S k v U 3 r a t u d c 6 U D K B L e F J Y O A A C d D i A d Q D o A U D C F 0 H Q A B Z B m9 + A 8 A h 4 3 J T e G z s 6 r K A 8 5 7 L I d g N L y b q H g 3 K B C L G 1 5 X f t 2 i 1 p W m G G g g j t d 1 s k T z Z e u v f p h 1 I n t 6 d 8 E j A G 8 0 k U F S g l 3 x 3 f k S 4 v Q + Q h I 1 4 4 B A I X F A G I A F b n Q I o w B V F D 9 E z O t Q f q n w e r L F h Z v + K 2 n T m H R v Y / i O 0 5 U V 6 4 z A T m v S 0 6 e K L 5 0 7 X Q A Q F 3 p A N I B V O R C i z A d Q A X V w i G c D u A R S O e p l g 7 A I 9 K t W W I A H m w / b l e E + l T 8 y y 3 U 2 U k u l V h X Q S u d T E c r O s J A + c 4 E n Y s a X I W v M 2 M o J k6 T U f Z F a 4 f u7 / M q E w O o U R M D q O G 0 9 + h U n8 U f S Q s k B r D B w c p T T Z F E O o B H 1 O i p M n v w S gf w i o B L b 4 q R U o N L B w A c J R 1 A H S z F Z O q z 6 5 G 0 Q N I B p A O 4 I x A D q B d e D K C O 1 S y S 4 kg N L h0 A 4 C k G U A e L C r c + 8 7 F I W i A / u g O g d 3 2 F G t c a T s E p d z i 6 D y V f m p e y h s L h 1 h h n 4 V B s l R O V G s P s X a Q D d 5 r v j I / h p w B O 4 O l j 0 U q x 0 7 V n j z W U q J l 4 a V 4 d Q h z l G w N w W e n 2 P F R X M Q D A B y 2 0 G A B 7 C a c H C 4 1 P B 8 D 4 S A f w h F c M A L j l I D Q d w H E M l W 5 Q M c s Y Q A z A r t Y Y g B 3 S h w l z B X j C 1 3 n f T Q d w X L w x g O M Y p g M A G M Y A X s F y Y g K o + A y N A V D E W L y 1 A x j 9 N C B L a U 4 6 / R R A e V 2 m + S r 3 J b o P J 1 E 0 X 8 U A a P d D c 5 o 9 m H Z w T j X S 9 a C 4 c u / f 4 p e C K s Q q 4 o 0 B P C K g Y E i N i Z q S o g V l D N 0 7 3 f d e J 6 X k v D U m B v C E i i I 4 l x g 6 W m f l b u k y v n Q A r G w V L b I V f v 2 K A c Q A 7 g h Q w V H j i w G w 8 q R 8 s N n / R M c A Y g A x A K F y q P n l C r A B M g X F 6 Y i U w I 4 H o V w B X l F 2 c i 7 U + X A I 1 Q / V + v I 3 A P q C 7 Q S X r q 2 I h B K i F O c I E 7 o / R Q x 0 f 8 o a 9 G 1 g t g b N V + F D 0 Q n V N T U G O v / s G q X s D / 9 h E E q U s k F a I M r G 6 T 4 U w c U A 6 i d 6 B x + K T q h + Y w A U s Y 3 4 G E C 9 c J R T m B o T p X R W z J R b J d e O I q S 4 O 8 3 H u b 9 0 A E V 1 p w M o A r X 3 l 2 g G f w w m H c B x f B W T i Q E U c Y 8 B F I G K A W w C p R R n R 5 c R A y j q O g Z Q B C o G 8 F 4 G M P q 7 A P S e Q e N n r 5 l 1 q f 2 J V N x V y Z f m R d v a 2 f z K H r f m U + 7 n H S e R c w 2 K u w t b q o + 9 G q D v J T T + s 3 Z i A I + 0 O c V A h R g D e E V A M W q K u 5 N z a g K K I V N M p m v E A G I A X w j Q Q q B C p M U x O y E 7 r m R K v n R M D I A i t h F P h a s K i 6 Z K T 6 J 0 A O k A / k W A t v Q 0 P l c A k 5 n Q + y s 1 E v W d I 2 8 A N a S V A 6 Q 2 8 3 5 U O o B 9 j H Y j F A J X t q + 7 G z r R m B T B U Y N T + H C u Q T s v Z 7 6 U W 4 U P q t 3 p G q P f C E R B o a A r p 1 r H G p T A W T z N V 7 n X r u S J 7 k / h n B q D w p / U O g + + 0 K S s T 8 d Q 3 G c a w d 8 D c B K y U r w U d C X e S R R 1 / Q 6 e 6 P 5 i A I q K 6 u 8 i o 9 l j A B 7 c 8 S y 0 Q N I B 1 C G m 2 M 5 m T g d g u H M q h K Q D e A Q + B h A D q C B A a y 0 d Q A X V E 2 K c R O U K 8 E g Q x T Y d w D Y C e Q M 4 o f C / p q Q i T Q d Q J 4 N i G w O I A d T V Z Y q k I o 0 B 1 I G n 2 M Y A T A Z A W 1 H n 5 5 x 1 e f y N d L 0 z 0 H m U X K + K 1 b t x T r F X H g G V F / e t M U 7 O l X 3 g K 8 C 7 i Y E W L t 0 f F d s s 3 i k G J S 8 q I B p / y 0 k Z o + y F j H H m 5 N K b g p W y j x j A k 1 J i A K + l Q z F Z b W S k + J V C U 6 4 T o z F O r G I A G y i 7 H J n O Q 0 V 4 i 3 e K Q V m f C o j G u 4 t N 2 e P W G G U f u Q I 8 I a C A q I y h p N P C p a c d z S d X g N u v o K h 3 G U 5 8 a d E q D 4 0 u v S l m q d R T r g C 5 A t w R o A K i 8 Y q o Y w B 1 s 5 T 4 c P 1 C k A 6 i O t Z Q O g B l j G s v d G 3 n N U M R 3 M p T m J 7 o 9 D R X u z u K i f K e M F w j B v A I D S 2 o 2 d 3 d K S B K u l K c d A y N n x X I F e d y 8 k f N Z 9 Y t U S 1 M c Y 8 B x A C + E K B F S O N j A K z v o w Y k m U w M I A Y Q A 9 g u T F q A u Q I w g 7 t k d K 4 A 5 z 4 6 0 f u u d K o 1 / P U h K l 7 n P n I F o O i D + B h A D G C v I w J y u o d e 1 g B G v x L s u z i 1 U t B b e 1 d e z 6 l T z 1 r O j n 1 Q z u n + l D c A p d j o G A V b O o b G 3 / a g j K F 7 H 3 4 P g I r B 6 X B 0 r t W F Q + + K C r H K G G p k l P M Y w C s C 9 G G 0 Q 7 t T 4 0 0 H U P P M d A A 1 n G Y n V z q A u m G k A 6 j r b f q 3 A T t O z n Q A j 2 T R D u 4 2 m m I I 5 L E b q m i E j q H x M Y B d 2 v 4 G d L R R 6 Q D q h M Q A X r G K A W z o h 9 6 X l D u q A j y 9 O 9 P T S 8 l J G U P 3 o e C 7 a o 2 6 H e 1 H K t j S M T R + e Q f g K s 5 9 + O v 3 o o 6 T 5 a p E K T i u G q P w R M 1 H 6 c g U b l d h q K y r 7 M / 2 0 4 B K w k 7 S 6 V y j e A V E Z Y w T r 6 v N F Q N Y w 4 i i w x j A E 1 c K i M q Y N R L p W T U G 0 I P z 8 y q K D m M A M Q C 7 W m M A d k h L E 8 Y A w E N j r g A l T U l B M Q A J t s O D Y g A x g M M i c k w Q A 3 C g y O e I A c Q A u G p O G B E D O A H U w p R W A y i s d 5 k Q + j m 8 O 3 E q + I 5 8 F T H Q f Y x w d O 7 P l d O M c y V f V 1 7 K l 9 i c u O M f B n I X j 2 M + h U D H u l 9 z U D F 0 5 B s D q D O s 8 E E 5 V 4 q W r q H s I w Z Q 1 8 k w s o M o m m Y M o I 6 Y U j i U 8 x h A n Q 8 c q R C I F 5 k M o G L o y D c G U G d Y 4 Y N y H g O o 8 4 E j F Q L x I j G A M m R O P l y F l j e A b Q R y B S j L e h x I R e o s k F F W 6 Q D q x C p 8 U M 4 v 2 w G M f i t w H b 6 + S E X U N D s X s b d 1 q b B m a 9 O 5 6 L 5 n 8 R Q T Z 6 5 O z l f O d c W 1 P z U a A 3 i U P h W 7 s 7 W M A b y i u b J w F F O k P 0 W r m C X F Z K q r G E A M Y K 9 b o K a o i N p 5 l b n i X L R o O 8 w n H c A G y l T s 6 Q B e E Y g B X K u T S Q e w d 8 T 9 8 / 8 x g L p 4 l Y c t Q M V n 6 M q T s + M U V s y S Y h I D A K q L A c Q A K n J x F e F l D c B Z C B V A / 4 2 h D y n O N p z e H z / v U Y M / R U X 3 r W B O 1 + 5 Y g + 7 b e d K 6 u w Z F D 2 T / z p 8 F I O t + x Q 4 / B V C E o i S w N S Y G U E c y B l D v W C h W i j H V m f s T G Q P Y Q C w G U J c R F b V i 7 H S N e v b 7 k b T V T g e w j + l D t 0 3 / P D i b X o u O A d R x o 8 U Z A 6 h j m w 7 A g x W e J Q Z Q h y w G k C t A X S 2 v k X k D K K L X 8 S 2 9 j t O 5 Y 4 0 i p K W w X A F K M M l B 2 A D o i a O 0 U S s 7 A K V A K P o K h j S v D g w 7 i p P u e 8 Y F x U T h i W r B W R / K 2 j G A J 9 S c g h s R o g i L 5 k X F 3 l U 4 1 D T o v r v 2 o R Q b H U O x o v P f 4 m M A M Y B d 3 V A z U T 7 a o m v s J r 0 R Q N d Q j F r J a z Q m B m A o T k q i 8 8 R J B 3 D 8 g c 7 J R w z A 8 A h I C 8 p 5 x 1 H E Q P N V 1 q C u T 3 O 6 z U / z o m L v a p 3 p q U b 3 3 b U P y r k S T 7 F S 1 s g V w N B l U O B j A B s n 0 e D r 1 D G A O l Z U h 3 k D 2 E D M K b h c A X I F U I r y a 0 w 6 g O L p 7 D x R l b m O k F w d 6 x J D x / c Z l G u f 0 y y r m O 7 F f f f D 4 N t 0 A E r R u g p q T 0 S u / 3 f l G w O o M x I D 2 M B K K b Y R 5 F T U N F 4 5 i Z z 7 q 0 t t P 9 K 1 9 x j A P t Z 7 L X h 9 h v 1 I R W / U m G Z r f I t H Q C e I y l z 7 N B + P i A E c x 5 D O Q A u N z v / Z g g u / S 4 L m F Q M A j 3 0 K I Q r x d E w M g C J 2 P J 4 W m r K i o j e a V w w g B n B H I F e A e p n S Q q v P / D c y B g D e G Z R T 0 E W i 8 + u t i l C + y z 7 o 3 p V 9 0 6 L q 0 J X y x S x l z B a + P 7 o D U A R E Q V Q E 1 F E I V 9 x H x 7 5 j A I 8 o x w C o 6 k B X c g u N A R g A H k y h GH g M I A Z Q u v M S 2 e Y K Q N D y x c Y A X r G k m K Q D M O g x B m A A U Z i C i v 2 2 R D q A d A D p A A b F t t r I q A f E A C 7 W A V A C l X j X 6 + d s b b r G V Q u H F g g 9 H R X + n B 8 1 K u 8 r F J P R H p 2 c d + y D a v q z W / r u v x a c k u s k a n W x 0 U 8 B l G L f G h M D q J / a i s m 4 N B 0 D 2 E A y B n D c B m I A M Y B D K l J a G b o g X U N x 6 n Q A r 6 x Q T J y G 7 N L I b R 6 a F 4 2 f r Z E O g D K 5 E R 8 D M I A 4 m C I d Q D q A Q + q i x a k s R t d I B 1 B H O Q b wD Q y g T n d f p O t 1 V / l 0 o G + X 2 y u t 3 L v S v o 7 w c s 2 1E o + ZF q 6 Y 1 9 S Q R 5 8 C r B Y 8 f V 1 2 5 U v v q K 51 9 + Z Z K S x X 0 S p 3 Z2 o k e z i S / 1 e 0 s J I n B a v h x 4 A E q K 7 Y K 4 L 7 E / Y e A 6 i z f E W N p g O o 8 4 e / R g q m P h S 6 U l g x g D p 1 K3 l K B 1 D n a R i p t H 2 G Z X e n W C m s G M A u P f e A l T z F A O o 8 x Q A 2 E H B + M k L F S I 3 3 i o U 2 e + M w S P M + h R O r v A E 8 M U P B d R I 7 m 2 u l4 N M B 1 F l e y R M 1 3 V v 8 f + n 0 I L V e w 7 w G A A A A A E l F T k S u Q m C C " s t y l e = " d i s p l a y : b l o c k ; " b d s f i d = " 1 7 6 " > / d i v >。
NF8480M5 产品技术白皮书(浙江 attractive 高性能服务器系列)说明书
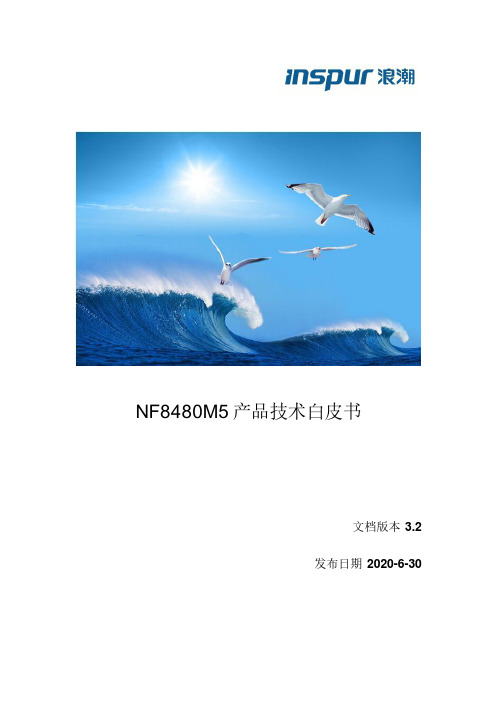
NF8480M5产品技术白皮书文档版本3.2发布日期2020-6-30版权所有© 浪潮集团有限公司2020。
保留一切权利。
未经事先书面同意,本文档的任何部分不得复制或以任何形式或任何方式修改、外传注:您购买的产品、服务或特性等应受浪潮集团商业合同和条款的约束。
本文档中描述的全部或部分产品、服务或特性可能不在您的购买或使用范围之内。
除非合同另有约定,浪潮集团对本文档内容不做任何明示或默示的声明或保证。
由于产品版本升级或其他原因,本文档内容会不定期进行更新。
除非另有约定,本文档仅作为使用指导,本文档中的所有陈述、信息和建议不构成任何明示或暗示的担保。
Inspur和“浪潮”是浪潮集团的注册商标。
Windows是微软公司的注册商标。
Intel、X eon是Intel公司的注册商标。
其他商标分别属于其相应的注册公司。
技术服务电话:4008600011地址:中国济南市浪潮路1036号浪潮电子信息产业股份有限公司邮编:250101目录目录............................................................................................................................................................... i i1 产品概述 (4)2 产品特点 (5)3 逻辑架构 (7)4 产品介绍 (8)4.1 前面板 (8)4.1.1 24×3.5"/2.5"前面板正视图 (8)4.1.2 OLED液晶诊断屏 (8)4.1.3 硬盘托架指示灯 (9)4.2 内部俯视图 (10)4.3 后面板 (11)4.4 主板布局 (12)4.5 Riser卡 (13)4.6 硬盘背板 (14)5 系统规格 (16)6 兼容性列表 (20)6.1 处理器 (20)6.2 内存 (22)6.3 存储 (24)6.3.1 SATA/SAS 硬盘型号 (24)6.3.2 SSD硬盘型号 (25)6.3.3 U.2 NVME SSD硬盘 (25)6.3.4 PCI-E NVME SSD (26)6.3.5 M.2硬盘 (26)6.4 RAID/SAS 卡 (26)6.5 网卡 (27)6.6 FC HBA 卡 (29)6.7 HCA 卡 (29)6.8 GPU (29)6.9 电源 (30)6.10 操作系统 (31)7 配置注意选项 (32)8 系统管理 (33)9 认证 (35)10 支持与服务 (36)11 新技术点描述 (37)11.1 Intel 可扩展架构 (37)11.2 Intel VROC 技术 (37)11.3 QAT 技术 (37)11.4 Memory Mirroring (37)11.5 Memory Rank Sparing (37)11.6 功耗感知技术 (38)12 相关文档 (39)13 商标 (40)1 产品概述浪潮英信服务器NF8480M5是浪潮针对政府、通信、金融、能源及各类大型企业的业务应用需求,基于全新一代英特尔®至强® 可扩展计算平台设计的一款高端四路机架服务器。
ch5 数据库系统概念(第6版)第五章高级SQL
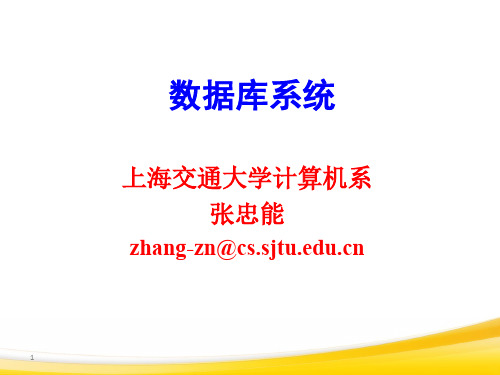
SQL:1999 还支持大量的命令式结构,例如
存储过程
存储过程的优点:
使用存储过程可以减少网络流量 增强代码的重用性和共享性 使用存储过程可以加快系统运行速度 使用存储过程保证安全性
存储过程的创建
写SQL语句 测试SQL语句 如得到所需结果,则创建结果 执行过程
触发器
触发器
触发器 是一条语句,当对数据库做修改时,它自动被系 统执行. 要设置触发器机制,必须满足: 指明什么条件下触发器被执行. 指明触发器执行的动作是什么. SQL-92 标准并不包括触发器,但是许多DB系统支持触发 器。 触发器于SQL:1999被引进到SQL标准 , 但是更早就通过非 标准语法被大部分数据库所支持.
SQL允许用if-then-else语句,for和while循环,等等 ,来定义过程.
存储过程
可以在数据库中存储过程 然后通过call语句来执行 允许外部应用程序对数据库进行操作,而无需了解内 部细节
面向对象方面将在22章介绍 (基于对象的数据库)*
函数和过程
SQL:1999 支持函数和过程
过程结构*
注意: 大部分数据库系统对下列标准语法实现了自 己的变种 复合语句: begin … end, While 和 repeat 语句:
end while
repeat
set n = n + 1
- 1、下载文档前请自行甄别文档内容的完整性,平台不提供额外的编辑、内容补充、找答案等附加服务。
- 2、"仅部分预览"的文档,不可在线预览部分如存在完整性等问题,可反馈申请退款(可完整预览的文档不适用该条件!)。
- 3、如文档侵犯您的权益,请联系客服反馈,我们会尽快为您处理(人工客服工作时间:9:00-18:30)。
Introduction to IR Systems: Supporting Boolean Text SearchChapter 27Information Retrievalv A research field traditionally separate from Databases•Goes back to IBM, Rand and Lockheed in the 50’s•G. Salton at Cornell in the 60’s•Lots of research since thenv Products traditionally separate•Originally, document management systems for libraries,government, law, etc.•Gained prominence in recent years due to web searchIR vs. DBMSv Seem like very different beasts:v Both support queries over large datasets, use indexing.•In practice, you currently have to choose between the two.IR’s“Bag of Words”Modelv Typical IR data model:•Each document is just a bag (multiset) of words (“terms”) v Detail 1: “Stop Words”•Certain words are considered irrelevant and not placed inthe bag•e.g., “the”•e.g., HTML tags like <H1>v Detail 2: “Stemming”and other content analysis •Using English-specific rules, convert words to their basicform•e.g., “surfing”, “surfed”--> “surf”Boolean Text Searchv Find all documents that match a Boolean containment expression:“Windows”AND (“Glass”OR “Door”)AND NOT “Microsoft”v Note:Query terms are also filtered via stemming and stop words.v When web search engines say “10,000 documents found”, that’s the Boolean search result size (subject to a common “max # returned”cutoff).Text “Indexes”v When IR people say “text index”…•Usually mean more than what DB people mean v In our (DB) terms, both “tables”and indexes •Really a logical schema (i.e., tables)•With a physical schema (i.e., indexes)•Usually not stored in a DBMS•Tables implemented as files in a file system•We’ll talk more about this decision soonA Simple Relational Text Indexv Create and populate a tableInvertedFile(term string,docURL string)v Build a B+-tree or Hash index on InvertedFile.term •Alternative 3 (<Key, list of URLs> as entries in index) critical here for efficient storage!!•Fancy list compression possible, too•Note: URL instead of RID, the web is your “heap file”!•Can also cache pages and use RIDsv This is often called an “inverted file”or “inverted index”•Maps from words -> docsv Can now do single-word text search queries!An Inverted File•“databases”•“microsoft”Handling Boolean Logicv How to do “term1”OR “term2”?•Union of two DocURL sets!v How to do “term1”AND “term2”?•Intersection of two DocURL sets!•Can be done by sorting both lists alphabetically and merging thelistsv How to do “term1”AND NOT “term2”?•Set subtraction, also done via sortingv How to do “term1”OR NOT “term2”•Union of “term1”and “NOT term2”.•“Not term2”= all docs not containing term2. Large set!!•Usually not allowed!v Refinement: What order to handle terms if you have many ANDs/NOTs?Boolean Search in SQLv (SELECT docURL FROM InvertedFileWHERE word = “windows ”INTERSECT SELECT docURLFROM InvertedFileWHERE word = “glass ”OR word = “door ”)EXCEPT SELECT docURLFROM InvertedFileWHERE word=“Microsoft ”ORDER BY relevance()“Windows ”AND (“Glass ”OR “Door ”)AND NOT “Microsoft ”Boolean Search in SQLv Really only one SQL query in Boolean Search IR:•Single-table selects, UNION, INTERSECT, EXCEPT v relevance()is the “secret sauce”in the search engines:•Combos of statistics, linguistics, and graph theorytricks!•Unfortunately, not easy to compute this efficientlyusing typical DBMS implementation.Computing Relevancev Relevance calculation involves how often search terms appear in doc, and how often they appear in collection:•More search terms found in doc àdoc is more relevant•Greater importance attached to finding rare termsv Doing this efficiently in current SQL engines is not easy:•“Relevance of a doc wrt a search term”is a function that is calledonce per doc the term appears in (docs found via inv. index):•For efficient fn computation, for each term, we can store the #times it appears in each doc, as well as the # docs it appears in.•Must also sort retrieved docs by their relevance value.•Also, think about Boolean operators (if the search has multipleterms) and how they affect the relevance computation!•An object-relational or object-oriented DBMS with good supportfor function calls is better, but you still have long execution path-lengths compared to optimized search engines.Fancier: Phrases and “Near”v Suppose you want a phrase•E.g., “Happy Days”v Different schema:•InvertedFile(term string, count int, position int, DocURLstring)•Alternative 3 index on termv Post-process the results•Find “Happy”AND “Days”•Keep results where positions are 1 off•Doing this well is like join processingv Can do a similar thing for “term1”NEAR “term2”•Position < k offUpdates and Text Searchv Text search engines are designed to be query-mostly:•Deletes and modifications are rare•Can postpone updates(nobody notices, no transactions!)•Updates done in batch (rebuild the index)•Can’t afford to go off-line for an update?•Create a 2nd index on a separate machine•Replace the 1st index with the 2nd!•So no concurrency control problems•Can compress to search-friendly, update-unfriendly format v Main reason why text search engines and DBMSs are usually separate products.•Also, text-search engines tune that one SQL query to death!DBMS vs. Search Engine ArchitectureSimple DBMS DBMSSearch EngineIR vs. DBMS Revisitedv Semantic Guarantees•DBMS guarantees transactional semantics•If inserting Xact commits, a later query will see the update•Handles multiple concurrent updates correctly ••Postpone insertions until convenient•No model of correct concurrencyv Data Modeling & Query Complexity•DBMS supports any schema & queries•Requires you to define schema•Complex query language hard to learn•IR supports only one schema & query•No schema design required (unstructured text)•Trivial to learn query languageIR vs. DBMS, Contd.v Performance goals•DBMS supports general SELECT•Plus mix of INSERT, UPDATE, DELETE•General purpose engine must always perform “well”•IR systems expect only one stylized SELECT •Plus delayed INSERT, unusual DELETE, no UPDATE.•Special purpose, must run super-fast on “The Query”•Users rarely look at the full answer in Boolean SearchLots More in IR …v How to “rank”the output? I.e., how to compute relevance of each result item w.r.t. the query?•Doing this well / efficiently is hard!v Other ways to help users “paw through”the output?•Document “clustering”, document visualizationv How to take advantage of hyperlinks?•Really cute tricks here!v How to use compression for better I/O performance?•E.g., making RID lists smaller•Try to make things fit in RAM!v How to deal with synonyms, misspelling, abbreviations?v How to write a good web crawler?Computing Relevance, Similarity: The Vector Space ModelDocument Vectorsv Documents are represented as “bags of words”v Represented as vectors when used computationally•A vector is like an array of floating point•Has direction and magnitude•Each vector holds a place for every term in thecollection•Therefore, most vectors are sparseDocument Vectors:One location for each word.Document Vectors nova galaxy heat h ’wood film role diet fur 1053510108791051010910579610 287513A B C D E F G H I Document idsWe Can Plot the VectorsStarDiet Assumption:Documents that are “close”in space are similar.Vector Space Modelv Documents are represented as vectors in term space•Terms are usually axis•Documents represented by binary vectors of terms v Queries represented the same as documents v A vector distance measure between the query and documents is used to rank retrieved documents•Query and Document similarity is based on length and direction of their vectors•Vector operations to capture boolean queryconditions•Terms in a vector can be “weighted”in many waysVector Space Documents and QueriesD 1D 2D 3D 4D 5D 6D 7D 8D 9D 10D 11t 2t 3t 1Boolean term combinations Q is a query –also representedas a vectorAssigning Weights to TermsŒBinary Weights•Raw term frequencyŽtf x idf•Recall the Zipf distribution•Want to weight terms highly if they are •frequent in relevant documents …BUT•infrequent in the collection as a wholeBinary Weightsv Only the presence (1) or absence (0) of a term is included in the vectorRaw Term Weightsv The frequency of occurrence for the term in each document is included in the vectorTF x IDF Weightsv tf x idf measure:•Term Frequency (tf)•Inverse Document Frequency (idf) --a way to dealwith the problems of the Zipf distributionv Goal:Assign a tf* idf weight to each term in each documentZipf DistributionL i K Ranking of frequencyTF x IDF Calculation)/log(*k ik ik n N tf w =log T contain that in documents of number the collection in the documents of number total in T term of frequency document inverse document in T term of frequency document in term ÷øöçèæ======n N idf C n C N C idf D tf D k T k k k k k k ik ik ikInverse Document Frequencyv IDF provides high values for rare words and low values for common words4110000log 698.22010000log 301.0500010000log 010********log =÷øöçèæ=÷øöçèæ=÷øöçèæ=÷øöçèæFor a collection of 10000 documentså==tk k ik k ik ik n N tf n N tf w 122)]/[log()()/log(TF x IDF Normalizationv Normalize the term weights (so longer documents are not unfairly given more weight)•The longer the document, the more likely it is for a given term to appear in it, and the more often a given term is likely to appear in it. So, we want to reduce the importance attached to a termappearing in a document based on the length of the document.Pair-wise Document Similaritynova galaxy heath ’woodfilmroledietfur1315221541A BC DHow to compute document similarity?Pair-wise Document Similaritynova galaxy heat h ’wood film role diet fur1315221541A B C Då=*===ti ii t t w w D D sim w w w D w w w D 121212,222121,12111),(...,,...,,9)11()42(),(0),(0),(0),(0),(11)32()51(),(=*+*======*+*=D C sim D B sim C B sim D A sim C A sim B A simPair-wise Document Similarity(cosine normalization)normalizedcosine )()(),(edunnormaliz ),(...,,...,,12212112121121212,222121,12111åååå====**=*===ti i t i i ti iiti i i tt w w w wD D sim w w D D sim w w w D w w w DVector Space “Relevance ”Measure)()(),( :comparison similarity in the normalize otherwise),( :normalized weights term if absent is term a if 0 ...,,,...,,121211,2121åååå====**=*====tj dtj qjtj d qji tj d qj i qt q q d d d i ijijij iti i www wD Q sim w w D Q sim w w w w Q w w w DComputing Relevance Scores98.0 42.064.0 ])7.0()2.0[(*])8.0()4.0[()7.0*8.0()2.0*4.0(),(yield?comparison similarity their does What )7.0,2.0(document Also,)8.0,4.0(or query vect have Say we 222222==+++===D Q sim D QVector Space with Term Weights and Cosine Matching1.00.80.60.40.20.80.60.40.21.0Term B Term AD i =(d i1,w di1;d i2, w di2;…;d it , w dit )Q =(q i1,w qi1;q i2, w qi2;…;q it , w qit )ååå====tj tj d q tj d q i ijj ij j w w w w D Q sim 11221)()(),(.042.064.0])7.0()2.0[(])8.0()4.0[()7.08.0()2.04.0()2,(2222==+×+×+×=D Q sim .058.056.),(1==D Q simText Clusteringv Finds overall similarities among groups of documentsv Finds overall similarities among groups of tokensv Picks out some themes, ignores othersText ClusteringTerm 1Term 2Clustering is“The art of finding groups in data.”•--Kaufmann LAR and Rousseeu PJ. (1990). FindingGroups in Data: An Introduction to Cluster Analysis. Wiley, Inc.Problems with Vector Spacev There is no real theoretical basis for the assumption of a term space•It is more for visualization than having any realbasis•Most similarity measures work about the same v Terms are not really orthogonal dimensions •Terms are not independent of all other terms;remember our discussion of correlated terms intextProbabilistic Modelsv Rigorous formal model attempts to predict the probability that a given document will be relevant to a given queryv Ranks retrieved documents according to this probability of relevance (Probability Ranking Principle)v Relies on accurate estimates of probabilitiesProbability Ranking Principlev If a reference retrieval system’s response to each request is a ranking of the documents in the collections in the order of decreasing probability of usefulness to the user who submitted the request, where the probabilities are estimated as accurately as possible on the basis of whatever data has been made available to the system for this purpose, then the overall effectiveness of the system to its users will be the best that is obtainable on the basis of that data.Stephen E. Robertson, J. Documentation1977Iterative Query RefinementQuery Modificationv Problem:How can we reformulate the query to help a user who is trying several searches to get at the same information?•Thesaurus expansion:•Suggest terms similar to query terms•Relevance feedback:•Suggest terms (and documents) similar toretrieved documents that have been judged tobe relevantWhale Orangutan Cod Salmon SharkDolphin GorillaRelevance Feedbackv Main Idea:•Modify existing query based on relevancejudgements•Extract terms from relevant documents and addthem to the query•AND/OR re-weight the terms already in thequeryv There are many variations:•Usually positive weights for terms from relevantdocs•Sometimes negative weights for terms from non-relevant docsv Users, or the system, guide this process by selecting terms from an automatically-generated list.Rocchio Methodv Rocchio automatically•Re-weights terms•Adds in new terms (from relevant docs)•have to be careful when using negative terms•Rocchio is not a machine learning algorithmRocchio Method0.25)to and 0.75 to set best to studies some (in t terms nonrelevan and relevant of importance the tune and ,chosen documents relevant -non of number the chosen documents relevant of number the document relevant -non for the vector the document relevant for the vector the query initial for the vector the 21012110121g b g b a g b a =====-+=åå==n n i S i R Q whereS n R n Q Q i i i n i n i i。