Xu ECCV2014 - Exploiting Low-Rank Structure from Latent Domains for Domain Generalization
国际留学本科生线上“机器学习”课程教学改革探索与实践
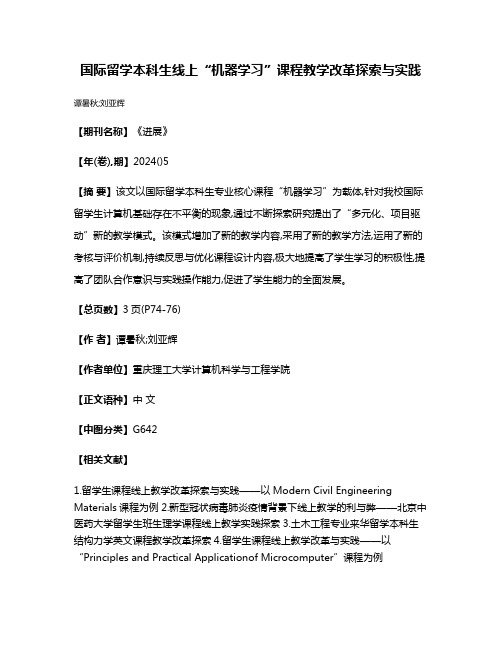
国际留学本科生线上“机器学习”课程教学改革探索与实践谭暑秋;刘亚辉
【期刊名称】《进展》
【年(卷),期】2024()5
【摘要】该文以国际留学本科生专业核心课程“机器学习”为载体,针对我校国际留学生计算机基础存在不平衡的现象,通过不断探索研究提出了“多元化、项目驱动”新的教学模式。
该模式增加了新的教学内容,采用了新的教学方法,运用了新的考核与评价机制,持续反思与优化课程设计内容,极大地提高了学生学习的积极性,提高了团队合作意识与实践操作能力,促进了学生能力的全面发展。
【总页数】3页(P74-76)
【作者】谭暑秋;刘亚辉
【作者单位】重庆理工大学计算机科学与工程学院
【正文语种】中文
【中图分类】G642
【相关文献】
1.留学生课程线上教学改革探索与实践——以Modern Civil Engineering Materials课程为例
2.新型冠状病毒肺炎疫情背景下线上教学的利与弊——北京中医药大学留学生班生理学课程线上教学实践探索
3.土木工程专业来华留学本科生结构力学英文课程教学改革探索
4.留学生课程线上教学改革与实践——以“Principles and Practical Applicationof Microcomputer”课程为例
因版权原因,仅展示原文概要,查看原文内容请购买。
基于隐语义模型(LFM)的协同过滤推荐算法(ALS)

基于隐语义模型(LFM)的协同过滤推荐算法(ALS)隐语义模型(Latent Factor Model,LFM)是一种常用于协同过滤推荐算法的模型。
它的基本思想是假设用户和物品的评分是由用户和物品的隐含特征决定的,通过学习用户和物品的隐含特征来进行推荐。
在基于隐语义模型的协同过滤推荐算法中,一般会使用交替最小二乘法(Alternating Least Squares,ALS)来优化模型参数。
ALS算法的基本思想是通过迭代的方式,交替固定用户的隐含特征或物品的隐含特征,更新另一方的隐含特征,直到收敛为止。
具体来说,ALS算法的流程如下:1.初始化用户和物品的隐含特征矩阵。
可以随机初始化,也可以使用其他方法初始化。
2.交替更新用户和物品的隐含特征矩阵。
a.固定用户的隐含特征矩阵,更新物品的隐含特征矩阵。
具体的更新公式如下:其中,P是用户的隐含特征矩阵,Q是物品的隐含特征矩阵,R是用户对物品的评分矩阵,I是单位矩阵,λ是正则化参数。
b.固定物品的隐含特征矩阵,更新用户的隐含特征矩阵。
具体的更新公式如下:通过迭代地进行a和b步骤,直到达到收敛条件为止。
3.使用学习到的用户和物品的隐含特征矩阵,计算用户对未评分物品的预测评分。
通过上述的步骤,就可以学习到用户和物品的隐含特征,进而进行推荐。
LFM+ALS算法在实际应用中具有较好的性能。
它不仅可以处理用户和物品的冷启动问题(通过学习用户和物品的隐含特征),还可以提高推荐的准确性和召回率。
总结起来,基于隐语义模型的协同过滤推荐算法(ALS)是一种通过学习用户和物品的隐含特征来进行推荐的方法。
它通过交替最小二乘法来优化模型参数,具有较好的性能和推荐效果。
西北工业大学网络空间安全学院贡献首个国内自主挖掘的RISC-V处理器设计中危漏洞

西北工业大学网络空间安全学院贡献首个国内自主挖掘的
RISC-V处理器设计中危漏洞
佚名
【期刊名称】《信息网络安全》
【年(卷),期】2024()5
【摘要】近日,国家计算机网络与应急技术处理协调中心(CNCERT)专题报道西北工业大学网络空间安全学院胡伟教授团队在RISC-V SonicBOOM处理器设计中挖掘出中危漏洞。
这是国内首个自主挖掘的RISC-V处理器设计上可远程利用的中危漏洞,也是国内首个处理器硬件安全领域国家重点研发计划项目——纳米级芯片硬件综合安全评估关键技术研究的重要进展。
【总页数】1页(P811-811)
【正文语种】中文
【中图分类】TP3
【相关文献】
1.潘光:青春的答卷——记西北工业大学航海学院“自主水下航行器”创新团队骨干潘光教授
2.西北工业大学成立西部地区首个国家保密学院
3.逐科学之梦筑安全之芯——记西北工业大学网络空间安全学院副教授胡伟
4.西北工业大学网络空间安全学院参与承担首个处理器芯片硬件安全国家重点研发计划项目
5.国内发现首个充电桩行业安全漏洞
因版权原因,仅展示原文概要,查看原文内容请购买。
目标检测综述

如上图所示,传统目标检测的方法一般分为三个阶段:首先在给定的图像上选择一些候选的区域,然后对这些区域提取特征,最后使用训练的分类器进行分类。
下面我们对这三个阶段分别进行介绍。
(1) 区域选择这一步是为了对目标的位置进行定位。
由于目标可能出现在图像的任何位置,而且目标的大小、长宽比例也不确定,所以最初采用滑动窗口的策略对整幅图像进行遍历,而且需要设置不同的尺度,不同的长宽比。
这种穷举的策略虽然包含了目标所有可能出现的位置,但是缺点也是显而易见的:时间复杂度太高,产生冗余窗口太多,这也严重影响后续特征提取和分类的速度和性能。
(实际上由于受到时间复杂度的问题,滑动窗口的长宽比一般都是固定的设置几个,所以对于长宽比浮动较大的多类别目标检测,即便是滑动窗口遍历也不能得到很好的区域)(2) 特征提取由于目标的形态多样性,光照变化多样性,背景多样性等因素使得设计一个鲁棒的特征并不是那么容易。
然而提取特征的好坏直接影响到分类的准确性。
(这个阶段常用的特征有 SIFT、 HOG 等)(3) 分类器主要有 SVM, Adaboost 等。
总结:传统目标检测存在的两个主要问题:一是基于滑动窗口的区域选择策略没有针对性,时间复杂度高,窗口冗余;二是手工设计的特征对于多样性的变化并没有很好的鲁棒性。
对于传统目标检测任务存在的两个主要问题,我们该如何解决呢?对于滑动窗口存在的问题, region proposal 提供了很好的解决方案。
region proposal (候选区域) 是预先找出图中目标可能出现的位置。
但由于 regionproposal 利用了图像中的纹理、边缘、颜色等信息,可以保证在选取较少窗口(几千个甚至几百个) 的情况下保持较高的召回率。
这大大降低了后续操作的时间复杂度,并且获取的候选窗口要比滑动窗口的质量更高(滑动窗口固定长宽比) 。
比较常用的 region proposal 算法有selective Search 和 edge Boxes ,如果想具体了解 region proposal 可以看一下PAMI2015 的“What makes for effective detection proposals?”有了候选区域,剩下的工作实际就是对候选区域进行图像分类的工作 (特征提取 +分类)。
一种保护用户数据隐私的抗拜占庭攻击的联邦学习方法[发明专利]
![一种保护用户数据隐私的抗拜占庭攻击的联邦学习方法[发明专利]](https://img.taocdn.com/s3/m/e7bc42aa85868762caaedd3383c4bb4cf7ecb7b9.png)
专利名称:一种保护用户数据隐私的抗拜占庭攻击的联邦学习方法
专利类型:发明专利
发明人:李洪伟,郝猛,邢鹏志,曾加,翟一晓,徐婕妤,陈涵霄,汤殿华,张希琳,张源,刘鹏飞
申请号:CN202111589802.7
申请日:20211223
公开号:CN114239862A
公开日:
20220325
专利内容由知识产权出版社提供
摘要:本发明提供一种保护用户数据隐私的抗拜占庭攻击的联邦学习方法,将服务器端分为两部分,计算服务器和服务提供服务器,由这两个服务器共同完成安全计算,各自得到有效性检测结果、反映服务器梯度方向与本地梯度方向相似性的余弦相似度结果,此过程中两中服务器无法通过各自的数据推算参与方的数据集内容,保护了用户数据隐私。
同时,服务器端得到的通过有效性检测结果以及余弦相似度结果得到的聚合可信分数能有效完成接收数据筛选,剔除无效数据后更新全局模型。
发明在进行联邦学习时技能保护用户数据隐私有能够抵御强拜占庭攻击,具有较高的扩展性和性能表现,能够满足不同规模的数据场景。
申请人:电子科技大学
地址:611731 四川省成都市高新区(西区)西源大道2006号
国籍:CN
代理机构:电子科技大学专利中心
代理人:邹裕蓉
更多信息请下载全文后查看。
基于深度学习的局部实例搜索
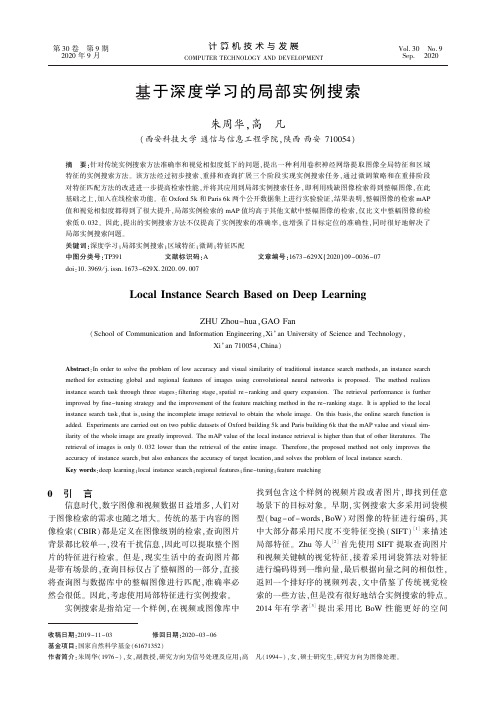
收稿日期:2019-11-03 修回日期:2020-03-06基金项目:国家自然科学基金(61671352)作者简介:朱周华(1976-),女,副教授,研究方向为信号处理及应用;高 凡(1994-),女,硕士研究生,研究方向为图像处理㊂基于深度学习的局部实例搜索朱周华,高 凡(西安科技大学通信与信息工程学院,陕西西安710054)摘 要:针对传统实例搜索方法准确率和视觉相似度低下的问题,提出一种利用卷积神经网络提取图像全局特征和区域特征的实例搜索方法㊂该方法经过初步搜索㊁重排和查询扩展三个阶段实现实例搜索任务,通过微调策略和在重排阶段对特征匹配方法的改进进一步提高检索性能,并将其应用到局部实例搜索任务,即利用残缺图像检索得到整幅图像,在此基础之上,加入在线检索功能㊂在Oxford 5k 和Paris 6k 两个公开数据集上进行实验验证,结果表明,整幅图像的检索mAP 值和视觉相似度都得到了很大提升,局部实例检索的mAP 值均高于其他文献中整幅图像的检索,仅比文中整幅图像的检索低0.032㊂因此,提出的实例搜索方法不仅提高了实例搜索的准确率,也增强了目标定位的准确性,同时很好地解决了局部实例搜索问题㊂关键词:深度学习;局部实例搜索;区域特征;微调;特征匹配中图分类号:TP 391 文献标识码:A 文章编号:1673-629X (2020)09-0036-07doi :10.3969/j.issn.1673-629X.2020.09.007Local Instance Search Based on Deep LearningZHU Zhou -hua ,GAO Fan(School of Communication and Information Engineering ,Xi ’an University of Science and Technology ,Xi ’an 710054,China )Abstract :In order to solve the problem of low accuracy and visual similarity of traditional instance search methods ,an instance search method for extracting global and regional features of images using convolutional neural networks is proposed.The method realizes instance search task through three stages :filtering stage ,spatial re -ranking and query expansion.The retrieval performance is further improved by fine -tuning strategy and the improvement of the feature matching method in the re -ranking stage.It is applied to the local instance search task ,that is ,using the incomplete image retrieval to obtain the whole image.On this basis ,the online search function is added.Experiments are carried out on two public datasets of Oxford building 5k and Paris building 6k that the mAP value and visual sim⁃ilarity of the whole image are greatly improved.The mAP value of the local instance retrieval is higher than that of other literatures.The retrieval of images is only 0.032lower than the retrieval of the entire image.Therefore ,the proposed method not only improves the accuracy of instance search ,but also enhances the accuracy of target location ,and solves the problem of local instance search.Key words :deep learning ;local instance search ;regional features ;fine -tuning ;feature matching0 引 言信息时代,数字图像和视频数据日益增多,人们对于图像检索的需求也随之增大㊂传统的基于内容的图像检索(CBIR )都是定义在图像级别的检索,查询图片背景都比较单一,没有干扰信息,因此可以提取整个图片的特征进行检索㊂但是,现实生活中的查询图片都是带有场景的,查询目标仅占了整幅图的一部分,直接将查询图与数据库中的整幅图像进行匹配,准确率必然会很低㊂因此,考虑使用局部特征进行实例搜索㊂实例搜索是指给定一个样例,在视频或图像库中找到包含这个样例的视频片段或者图片,即找到任意场景下的目标对象㊂早期,实例搜索大多采用词袋模型(bag -of -words ,BoW )对图像的特征进行编码,其中大部分都采用尺度不变特征变换(SIFT )[1]来描述局部特征㊂Zhu 等人[2]首先使用SIFT 提取查询图片和视频关键帧的视觉特征,接着采用词袋算法对特征进行编码得到一维向量,最后根据向量之间的相似性,返回一个排好序的视频列表,文中借鉴了传统视觉检索的一些方法,但是没有很好地结合实例搜索的特点㊂2014年有学者[3]提出采用比BoW 性能更好的空间第30卷 第9期2020年9月 计算机技术与发展COMPUTER TECHNOLOGY AND DEVELOPMENT Vol.30 No.9Sep. 2020Fisher向量[4]和局部特征聚合描述符(VLAD)[5]来描述SIFT特征的空间关系,从而进行实例检索㊂随着深度学习的发展,深度卷积神经网络特征被广泛应用于计算机视觉的各个领域,如图像分类[6-7]㊁语音识别[8]等,均取得了不错的效果,因此有学者也将其引入到图像检索领域㊂起初,研究者们利用神经网络的全连接层特征进行图像检索[9],后来很多研究者开始转向卷积层特征的研究[10],并且证明卷积层特征的性能更好㊂Eva等人[11]采用词袋模型对卷积神经网络(CNNs)提取的特征进行编码,然后分别进行初次搜索,局部重排,扩展查询,从而实现实例检索㊂实例检索需采用局部特征实现,因此许多生成区域信息的方法相继出现,最简单的是滑动窗口,之后有学者提出使用Selective Search生成物体候选框[12-13],但是这些方法将生成候选区域和特征提取分开进行㊂Faster R-CNN[14]是一个端到端的网络,它可以同时提取卷积层特征和生成候选区域㊂文献[15]提出将微调之后的目标检测网络Faster R-CNN应用到实例检索中,使用区域提议网络(region proposal network, RPN)生成候选区域,从而得到查询图区域特征与数据库图像区域特征,特征匹配之后排序得到检索结果,在两个建筑物数据集上取得了不错的效果㊂何涛在其论文中[16]针对Faster R-CNN网络效率较低的问题提出了端到端的深度区域哈希网络(DRH),使用VGG16作为特征提取器,滑动窗口和RPN网络得到候选区域,并将两种方法进行对比,整个网络最后阶段对特征进行哈希编码并计算汉明距离进行排序,从而得到检索结果,文中为了排除不同场景㊁不同光照和拍照角度产生的干扰,使用局部信息进行检索㊂以上两篇文献尽管均使用局部信息进行检索,但都是为了排除干扰信息将查询图中的目标标记出来,查询图依然是整幅图像㊂实际搜索图像时某些图片会有残缺,此时就无法通过标记目标进行检索,因此实例检索除了常见的利用局部特征进行整幅图像的检索之外,局部图像的检索亦有着非常重要的现实意义㊂现有的检索方法的检索效果不是很理想,因此文中针对以上两个问题首先改进整幅图像的检索并提高其检索性能,之后利用图像的部分信息(例如建筑物的顶部㊁嫌疑人的部分特征等)检索得到整幅图像,实现局部图像的检索㊂同时,考虑到实际检索时,输入均为一幅图像,输出为一组图像,因此,文中在局部实例检索的基础之上加入在线检索功能,可以实现局部图像的实时搜索㊂因此,主要有以下两大方面的贡献和创新:(1)基于深度学习的实例搜索㊂一方面,通过微调策略提高了实例搜索的精确度;另一方面,针对候选框得分(scores)和余弦距(cosine)两种相似性度量方法存在的不足,提出将两种方法相结合,以获得更好的检索效果㊂(2)基于深度学习的局部实例搜索㊂由于局部图像的检索具有重大的现实意义,将全局实例搜索算法应用在局部实例检索任务中,即利用残缺图片信息搜索得到整幅图像,并加入在线检索功能,输入局部查询图,便可以得到查询结果和所属建筑物的名字㊂1 相关理论1.1 基于Faster R-CNN的区域特征提取如图1所示,Faster R-CNN由卷积层(Conv layers),RPN网络,RoI pooling,分类和回归四部分构成㊂图1 Faster R-CNN结构 卷积层用于提取图像特征,可以选择不同结构的网络,输入为整张图片,输出为提取的特征称为feature maps㊂文中采用VGG16[7]的中间卷积层作为特征提取器㊂RPN网络用于推荐候选区域,这个网络是用来代替之前的selective search[13]的,输入为图片,输出为多个矩形区域以及对应每个矩形区域含有物体的概率㊂首先将一个3*3的滑窗在feature maps上滑动,每个窗口中心点映射到原图并生成9种矩形框(9anchor boxes),之后进入两个同级的1*1卷积,一个分支通过softmax进行二分类,判断anchor boxes属于foreground还是background㊂另一分支计算anchor㊃73㊃ 第9期 朱周华等:基于深度学习的局部实例搜索boxes的bounding box regression的偏移量㊂Proposal 层结合两个分支的输出信息去冗余后保留N个候选框,称为proposals㊂传统的CNN网络,输入图像尺寸必须是固定大小的,但是Faster R-CNN的输入是任意大小的,RoI pooling作用是根据候选区域坐标在特征图上映射得到区域特征并将其pooling成固定大小的输出,即对每个proposal提取固定尺寸的特征图㊂分类和回归模块,一方面通过全连接层和softmax 层确定每个proposal的类别,另一方面回归更加精确的目标检测框,输出候选区域在图像中的精确坐标㊂1.2 微调Faster R-CNN微调,即用预训练模型重新训练自己的数据,现有的VGG16FasterR-CNN预训练模型主要是基于VOC2007数据集中20类常见的物体预训练得到的㊂文中的数据集是建筑物,与VOC2007图片相似度和特征都相差较大,如果依然采用预训练模型,效果必然不好,再加上从头开始训练,需要大量的数据㊁时间和计算资源㊂而文中所选用的数据集较小,因此需要进行微调,这样不仅可以节省大量时间和计算资源,同时还可以得到一个较好的模型㊂微调主要分为以下三个步骤:(1)数据预处理㊂首先需要对数据集进行数据清洗,主要去除无效值和纠正错误数据,提高数据质量㊂其次,由于文中的数据集较小且每类样本分布不均衡,因此选择性地对每类图片作图像增强处理,其中图像增强方法包括水平翻转,增加高斯噪声,模糊处理,改变图像对比度㊂最后将每幅图片中的目标样例标记出来㊂(2)建立训练集和测试集㊂一般是按一定的比例进行分配,但是文中选用的数据集存在样本不均衡的问题,有些类别特别多,有些类别特别少㊂由于是将所有图片全部放入同一个文件夹,然后依次读取样本分配训练集和测试集,如果按比例分配,小类样本参与训练的机会就会比大类少,训练出来的模型将会偏向于大类,使得大类性能好,小类性能差㊂平衡采样策略就是把样本按类别分组,每个类别生成一个样本列表,制作训练集时从各个类别所对应的样本列表中随机选择样本,这样可以保证每个类别参与训练的机会比较均衡㊂(3)修改相关网络参数,进行训练㊂主要修改网络文件中的类别数和类名,然后不断调节超参数,使性能达到最好㊂1.3 实例搜索实例检索一般经过初次搜索,局部重排,扩展查询三部分完成㊂初次搜索首先提取查询图和数据库所有图像的全局特征,然后计算特征之间的相似度,最后经过排序得到初步的检索结果㊂文中提取VGG16网络的最后一个卷积层(Conv5_3)特征㊂局部重排是将初次搜索得到的前K幅图片作为新的数据库进行重排,基本思路是提取查询图和数据库的区域特征并进行匹配,根据匹配结果进行排序从而得到查询结果㊂这里查询图和数据库的区域特征提取方法不同,其中,查询图的区域特征是用groundtruth 给定的边界框对整幅图像特征进行裁剪得到的,而数据库的区域特征是经过RPN网络和RoI pooling池化得到的特征(pool5)㊂扩展查询(query expansion,QE)是取出局部重排返回的前K个结果,对其特征求和取平均作为新的查询图,再做一次检索,属于重排的一种㊂2 实 验2.1 数据集和评价指标(1)实验环境㊂文中所有实验均在NVIDIA GeForce RTX2080上进行,所用系统为Ubuntu18.04,使用的深度学习框架为Caffe,编程语言为Python㊂(2)数据集介绍㊂在两个公开的建筑物数据集Oxford[17]和Paris[18]上进行实验㊂其中Oxford包含5063张图片,Paris包含6412张图片,但是有20张被损坏,因此有6392张图片可用㊂两个数据集都来自Flickr,共有11类建筑物,同一种建筑物有5张查询图,因此每个数据集总共有55张查询图,除此之外,两个数据集相同类别建筑物的场景㊁拍照角度和光照都有所不同,而且有很多本来不是同一种建筑物但是从表面看上去却非常相似的图片㊂(3)评价指标㊂平均精度均值(mean average precision,mAP)是一个反映了图像检索整体性能的指标,如式(1)和(2)所示㊂MAP=∑N k=1AP查询图的个数(1) AP=∑N k=1(P(k)㊃rel(k))相关图片个数(2)其中,N表示返回结果总个数,P(k)表示返回结果中第k个位置的查准率,rel(k)表示返回结果中第k个位置的图片是否与查询图相关,相关为1,不相关为0㊂MAP是多次查询后,对每次检索的平均精度AP值求㊃83㊃ 计算机技术与发展 第30卷和取平均㊂这里对是否相关做进一步解释:两个数据集的groundtruth有三类,分别是good,ok和junk,如果检索结果在good和ok中,则判为与查询图相关,如果在junk中,则判为不相关㊂2.2 基于深度学习的实例检索2.2.1 方 法先尝试使用VGG16Faster R-CNN预训练模型进行检索,在两个数据集上MAP值仅0.5左右,接着文中使用微调策略,只冻结了前两个卷积层,更新了之后所有的网络层权值,通过不断调参,训练一个精度尽可能高的模型㊂其中,微调过程中分别采用数据增强和平衡采样技术对数据进行处理㊂具体地,对于Oxford 数据集,建筑物radcliffe_camera的数量高达221张,而建筑物pitt_rivers仅有6张,其他9类样本数量在7至78之间不等,数量差距相当大,因此,选择性地对数量小的6类样本通过上面提到的方法进行数据增强,使小类样本数量增大㊂对其进行数据增强之后,虽然样本数量差距缩小,但是依然存在不均衡的问题,如果将所有样本放入一个文件夹中,按比例分配训练集和测试集,则依然会导致训练出来的模型小类性能差的问题㊂因此,将每类样本生成一个列表,再从每个列表中随机选取一定数量的样本作为该类的训练样本㊂除此之外,文献[15]在局部重排部分使用了两种特征匹配方法,一种是直接利用候选框对应得分(scores)进行排序㊂数据库中每幅图片经过Proposal layer会得到300个区域提议(proposal)的坐标和对应得分,找到查询图对应类的最高得分作为查询图和数据库每幅图片的相似度,再从高到低进行排序就可以得到检索结果㊂另一种是利用余弦距(cosine)进行排序㊂数据库中的每幅图片经过RoI pooling可以得到300个特征向量,计算查询图与数据库中每幅图片的300个区域特征的余弦距,最小距离对应的候选框即就是和查询图最相似的区域提议,然后把所有的最小距离再从小到大进行排序,就可以得到相似度排序㊂第一种方法虽然得到的边界框(bounding box regression)定位较准,mAP值也很高,但是视觉相似度并不是很高㊂而且根据候选框得分进行排序,每类建筑物的得分和排序都是一定的,因此每次相同类别的不同查询返回结果都是相同的,不会根据查询图片的不同而返回不同的排序㊂第二种方法得到的检索结果,图像相似度很高,但是边界框定位不是很准确㊂文中将两种方法结合(scores+cosine),利用余弦距计算相似度并排序,选择得分最高的候选框进行目标定位,这样既解决了视觉相似度不够的问题,也解决了相同类别的不同查询返回结果相同的问题,同时又解决了目标定位不准的问题㊂2.2.2 参数设置本节主要讨论扩展查询中的参数k对实验结果的影响,并选取一个最优值作为本实验的默认值㊂在特征匹配方法选用余弦距的情况下,在两个数据集上分别测试了k等于3㊁4㊁5㊁6㊁7时的mAP值,实验结果如表1所示㊂表1 不同k值对mAP值的影响k Oxford Paris30.9090.88940.9100.88850.9100.88560.9120.88970.9120.888 从表1综合来看,文中选用6作为k的默认值㊂2.2.3 结果与分析表2是文中与其他文献mAP值的对比,最后两项是文中三种方法的实验结果㊂可以看出,相比于其他文献,文中方法的检索性能得到很大的提升,比目前最好的方法分别高出6.1%和4%,说明文中方法优于其他检索方法㊂文中方法与文献[15]所采用方法类似,但结果却得到了很大的改善,通过分析认为,虽然所用数据集都相同,但是生成的训练集和测试集完全不同,而且文中采用数据增强方法,使得样本的数量增加了3~4倍,使用平衡采样的方法保证小类样本可以得到和大类样本同样的训练机会㊂除此之外,网络超参数对于模型的影响非常大,因此文中对参数进行调优,使得训练出来的模型更好㊂表2 文中方法与其他方法的mAP值对比方法Oxford ParisR.Tao等人[3]0.765-Razavian等人[10]0.5560.697Eva等人[11]0.7390.819CA-SR[15]0.7720.824CS-SR[15]0.7860.842DRH[16]0.8510.849Ours(scores)0.9030.890 Ours(cosine/cosine+scores)0.9120.890 图2是两组对比图,图(a)和图(b)是对建筑物all_ souls和louvre分别用候选框得分和余弦距进行排序的结果,其中,左边1列是查询图,右边5列是查询返回结果,表示与查询相关(下同)㊂图3是all_souls的两个不同查询用得分进行排序得到的检索结果㊂图4是将两种匹配方法结合得到的检索结果㊂从图2可以看出利用候选框得分排序得到的结果目标定位较准确,但是返回结果的背景㊁光照㊁拍照角㊃93㊃ 第9期 朱周华等:基于深度学习的局部实例搜索度㊁颜色㊁对比度和样例大小与查询图相差很大,而利用余弦距排序得到的结果候选框定位不是很准,但从背景㊁样例大小等视觉角度来看相似度较高㊂图3中all _souls 的两个不同查询图返回结果中不仅图片一样,而且顺序也相同㊂因此可以看出两种方法各有缺陷㊂图2 不同建筑物的同一个查询分别利用得分和余弦距得到的排序图3 建筑物all _souls 的两个不同查询根据得分得到的排序图4是将两种方法结合得到的返回结果,与图2相比视觉相似度提高了,目标定位也更准确了,与图3相比,同一个建筑物的两个不同查询返回结果也会根据查询图片的不同而改变,且从表2最后一行可以看出该方法比使用候选框得分在Oxford 上得到的mAP 值高0.009,与使用余弦距得到的mAP 值相同㊂因此认为,以上提出的特征匹配方法得到的返回结果在不降低mAP 值的基础上提高了检索的准确率㊂图4 综合得分和余弦距两种方法得到的检索结果2.3 基于深度学习的局部实例检索2.3.1 方 法本节局部图像的检索是建立在2.2节基础之上的,正是由于整幅图像检索采用候选区域特征实现,局部图像的检索才得以实现㊂较之于整幅图像的检索,局部图像的检索具有同样重大的现实意义㊂生活中常会因为某个原因使图片变得残缺,且难以识别,那么此时就需要使用局部图像检索得到原始图像的完整信息㊂比如,通过某建筑物的顶部搜索得到整幅图像从而识别该建筑物㊂或者可以应用在刑侦工作中,当摄像机捕获到的是某犯罪嫌疑人的部分特征时,可以通过已有的部分特征在图像库或者其他摄像头下搜索得到该嫌疑人的完整信息㊂由于目前没有一个现成的残缺图像库,因此本节利用截图工具对整幅图像作裁剪处理以模拟残缺图像,即从图像库选取不同实例通过裁剪得到不同大小,不同背景,不同角度,不同颜色的局部查询图㊂由于图像库图像都是整幅图像,在尺寸和包含的信息方面与局部查询图相差很大,因此局部检索最大的难点在于如何处理局部图像㊂2.2节会对输入图片统一进行缩放,那么局部查询图片输入后,先进行放大,则会导致原始输入图像失真,提取特征后再对其进行裁剪又会进一步丢失大部分图像信息,因此根本无法得到正确的检索结果㊂文中对其进行以下处理:即输入查询图后,先将局部查询填充至与数据库图像相同大小(图像库的图像基本都是1024*768或者768*1024大小的),这样对图像进行统一缩放,提取特征,按比例㊃04㊃ 计算机技术与发展 第30卷裁剪之后,得到的正是局部图像的特征,再与图像库匹配,则会输出正确的排序结果㊂本节输入为建筑物的局部图像,输出为局部查询所属的建筑物图像,并且会标记出局部查询在所属建筑物中的位置㊂目前,很多文献(如[15])都比较注重算法的研究,基本都采用离线的形式实现图像检索,不仅离线建立特征库,查询图也是成批输入到网络中进行离线检索,得到的结果也是成批保存起来,可是实际应用中,一般都是将查询图逐幅输入进行实时检索,因此文中在前文基础之上,加入了在线检索功能,最后实现在线局部实例检索㊂2.3.2 结果与分析如图5是通过裁剪建筑物radcliffe _camera 和triomphe 的原图,得到的5个不同查询图,分别选取一幅进行检索,得到了完整的建筑物,且标记出了局部查询图像在整个建筑物中的位置,如图6所示㊂最终mAP 值分别为0.880和0.857㊂从检索结果可以看出返回结果的视觉相似度极高,目标定位准确,且mAP 值高于其他文献中整幅图像的检索准确率㊂因此,可以证明文中提出的全局搜索算法在局部图像检索任务中亦能取得很好的效果㊂图5 两种建筑物的五个局部查询图图6 两组局部查询的检索结果在此之前,只有文献[19]为了证明行人重识别系统的普适性,使用CaffeNet 和VGG 16两个网络模型在Oxford 数据集上对局部建筑物图像进行了测试,得到的mAP 值分别为0.662和0.764,远低于文中的准确率㊂因此提出的局部实例搜索的性能良好㊂在线检索功能按照输入图片,可得到查询结果和查询建筑物的名字,且文中在没有使用任何编码算法的情况下,在两个数据集上检索一幅图的平均耗时分别为5.7s 和7s ,经检测,90%的时间都耗费在利用特征向量计算相似度部分㊂如图7所示,分别是bodleian 和eiffel 的检索结果和总耗时㊂图7 在线检索结果3摇结束语为了进一步提高实例检索性能,针对以往的利用候选框得分和余弦距进行特征匹配的不足,提出将两种方法结合,即利用余弦距计算相似度并排序,选择得分最高的候选框进行目标定位㊂并使用微调策略重新训练预训练模型从而使其适用于文中的实例检索㊂相比于其他方法,文中采用的方法在性能方面有明显的提升㊂在此基础之上,利用残缺图像搜索得到整幅图像,性能高于其他文献整幅图像的检索,且仅比文中整幅图像检索低0.032,实验结果证明提出的全局搜索算法同样适用于局部图像检索任务㊂之后加入在线检索功能,在没有任何编码的情况下检索一幅图像平均耗时仅需5.7s ~7s ㊂在未来的工作中,可以进一步加入编码模块,以提高检索速度,并且可以在更大的数据集上进行测试㊂参考文献:[1] LOWE D G.Object recognition from local scale -invariant fea⁃tures [C ]//International conference on computer vision.㊃14㊃ 第9期 朱周华等:基于深度学习的局部实例搜索Kerkyra,Greece:IEEE,1999:1150-1157.[2] ZHU C,SATOH rge vocabulary quantization for searchinginstances from videos[C]//International conference on multime⁃dia retrieval.New York,NY,United States:ACM,2012:1-8.[3] TAO R,GAVVES E,SNOEK C G M,et al.Locality in genericinstance search from one example[C]//Conference on computer vision and pattern recognition.Columbus,OH,USA:IEEE, 2014:2099-2106.[4] PERRONNIN F,LIU Y,SÁNCHEZ J,et rge-scale imageretrieval with compressed Fisher vectors[C]//Conference on computer vision and pattern recognition.San-Francisco,CA, USA:IEEE,2010:3384-3391.[5] GONG Y,WANG L,GUO R,et al.Multi-scale orderless poolingof deep convolutional activation features[C]//European confer⁃ence on computer vision.Zürich,Switzerland:Springer,2014: 392-407.[6] KRIZHEVSKY A,SUTSKEVER I,HINTON G E.Imagenetclassification with deep convolutional neural networks[C]//Neural information processing systems.New York,NY,United States:ACM,2012:1097-1105.[7] SIMONYAN K,ZISSERMAN A.Very deep convolutional net⁃works for large-scale image recognition[C]//Computer vision and pattern recognition.Boston,MA,USA:IEEE,2015:1-14.[8] HINTON G,DENG L,YU D,et al.Deep neural networks for a⁃coustic modeling in speech recognition[J].IEEE Signal Process⁃ing Magazine,2012,29(6):82-97.[9] WAN J,WANG D,HOI S C H,et al.Deep learning for content-based image retrieval:a comprehensive study[C]//International conference on multimedia.New York,NY,United States:ACM, 2014:157-166.[10]RAZAVIAN A S,SULLIVAN J,CARLSSON S,et al.Visual in⁃stance retrieval with deep convolutional networks[J].ITE Trans⁃actions on Media Technology and Applications,2016,4(3):251-258.[11]MOHEDANO E,MCGUINNESS K,O’CONNOR N E,et al.Bags of local convolutional features for scalable instance search[C]//International conference on multimedia retrieval.NewYork,NY,United States:ACM,2016:327-331. [12]UIJLINGS J R R,VAN DE SANDE K E A,GEVERS T,et al.Selective search for object recognition[J].International Journal of Computer Vision,2013,104(2):154-171.[13]GIRSHICK R,DONAHUE J,DARRELL T,et al.Rich feature hi⁃erarchies for accurate object detection and semantic segmentation[C]//Computer vision and pattern recognition.Columbus,OH,USA:IEEE,2014:580-587.[14]REN S,HE K,GIRSHICK R,et al.Faster R-CNN:towards real-time object detection with region proposal networks[C]//Neural information processing systems.New York,NY,United States: ACM,2015:91-99.[15]SALVADOR A,GIRÓ-I-NIETO X,MARQUÉS F,et al.FasterR-CNN features for instance search[C]//Conference on com⁃puter vision and pattern recognition s Vegas,NV, USA:IEEE,2016:9-16.[16]何 涛.基于深度学习和哈希的图像检索的方法研究[D].成都:电子科技大学,2018.[17]PHILBIN J,CHUM O,ISARD M,et al.Object retrieval withlarge vocabularies and fast spatial matching[C]//Conference on computer vision and pattern recognition.Minneapolis,MN,USA: IEEE,2007:1-8.[18]PHILBIN J,CHUM O,ISARD M,et al.Lost in quantization:im⁃proving particular object retrieval in large scale image databases[C]//Conference on computer vision and pattern recognition.Anchorage,AK,USA:IEEE,2008:1-8.[19]ZHENG Z,ZHENG L,YANG Y.A discriminatively learnedCNN embedding for person re-identification[J].Multimedia Computing,Communications,and Applications,2017,14(1):1-20.㊃24㊃ 计算机技术与发展 第30卷。
基于主干增强和特征重排的反无人机目标跟踪

基于主干增强和特征重排的反无人机目标跟踪
郑滨汐;杨志钢;丁钰峰
【期刊名称】《液晶与显示》
【年(卷),期】2024(39)4
【摘要】视频图像中面向无人机的目标跟踪是反无人机任务中的重要一环。
无人机低空飞行背景复杂,同时在视频图像中目标像素占比较小,都给目标跟踪增加了难度。
针对以上问题,以SiamRPN++为基础,提出了一种引入改进的主干网络和特征重排的孪生神经网络目标跟踪算法(SiamAU)。
首先,在主干网络中加入ECA-Net 注意力机制网络,同时对激活函数进行改进,以提升复杂背景下的特征表征能力;然后,对主干网络输出的浅层特征进行浅层降维并与后三层深层特征进行融合,得到更适合无人机等小目标跟踪的改进深度融合特征。
在DUT Anti-UAV数据集
上,SiamAU算法的成功率和精确率达到了60.5%和88.1%,相比基准算法提升了5.6%和8.1%。
在两个公开数据集上的测试结果表明,在反无人机场景中SiamAU 算法的跟踪表现优于目前主流的算法。
【总页数】11页(P532-542)
【作者】郑滨汐;杨志钢;丁钰峰
【作者单位】哈尔滨工程大学信息与通信工程学院
【正文语种】中文
【中图分类】TP391
【相关文献】
1.基于无人机航拍技术的城市主干路直线段车辆运行特征研究
2.增强特征信息的孪生网络无人机目标跟踪方法
3.基于特征融合与通道感知的无人机红外目标跟踪算法
4.基于可分辨特征的无人机视觉多目标跟踪
5.基于注意力特征融合的无人机多目标跟踪算法
因版权原因,仅展示原文概要,查看原文内容请购买。
TAFSSL: TASK ADAPTIVE FEATURE SUB-SPACE LEARNING F
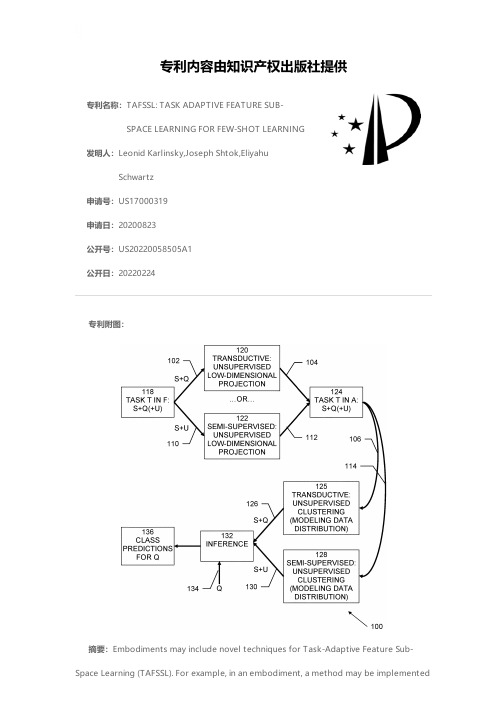
专利名称:TAFSSL: TASK ADAPTIVE FEATURE SUB-SPACE LEARNING FOR FEW-SHOT LEARNING发明人:Leonid Karlinsky,Joseph Shtok,EliyahuSchwartz申请号:US17000319申请日:20200823公开号:US20220058505A1公开日:20220224专利内容由知识产权出版社提供专利附图:摘要:Embodiments may include novel techniques for Task-Adaptive Feature Sub-Space Learning (TAFSSL). For example, in an embodiment, a method may be implementedin a computer system comprising a processor, memory accessible by the processor, and computer program instructions stored in the memory and executable by the processor, the method comprising: training a machine learning system to classify features in images by: generating a sample set comprising one or a few labeled training samples and one or a few additional samples containing instances of target classes, performing dimensionality reduction computed on the samples in the sample set to form a dimension reduced sub-space, generating class representatives in the dimension reduced sub-space using clustering, and classifying features in images using the trained machine learning system.申请人:International Business Machines Corporation地址:Armonk NY US国籍:US更多信息请下载全文后查看。
- 1、下载文档前请自行甄别文档内容的完整性,平台不提供额外的编辑、内容补充、找答案等附加服务。
- 2、"仅部分预览"的文档,不可在线预览部分如存在完整性等问题,可反馈申请退款(可完整预览的文档不适用该条件!)。
- 3、如文档侵犯您的权益,请联系客服反馈,我们会尽快为您处理(人工客服工作时间:9:00-18:30)。
Exploiting Low-Rank Structure from Latent Domains for Domain GeneralizationZheng Xu,Wen Li,Li Niu,and Dong XuSchool of Computer Engineering,Nanyang Technological University,Singapore Abstract.In this paper,we propose a new approach for domain gener-alization by exploiting the low-rank structure from multiple latent sourcedomains.Motivated by the recent work on exemplar-SVMs,we aim totrain a set of exemplar classifiers with each classifier learnt by using onlyone positive training sample and all negative training samples.Whilepositive samples may come from multiple latent domains,for the posi-tive samples within the same latent domain,their likelihoods from eachexemplar classifier are expected to be similar to each other.Based onthis assumption,we formulate a new optimization problem by introduc-ing the nuclear-norm based regularizer on the likelihood matrix to theobjective function of exemplar-SVMs.We further extend Domain Adap-tation Machine(DAM)to learn an optimal target classifier for domainadaptation.The comprehensive experiments for object recognition andaction recognition demonstrate the effectiveness of our approach for do-main generalization and domain adaptation.Keywords:Latent domains,domain generalization,domain adapta-tion,exemplar-SVMs.1IntroductionDomain adaptation techniques,which aim to reduce the domain distribution mismatch when the training and testing samples come from different domains, have been successfully used for a broad range of vision applications such as object recognition and video event recognition[23,17,16,10,11,12,6,7,24].As a related research problem,domain generalization differs from domain adapta-tion because it assumes the target domain samples are not available during the training process.Without focusing on the generalization ability on the specific target domain,domain generalization techniques aim to better classify testing data from any unseen target domain[26,22].Please refer to Section2for a brief review of existing domain adaptation and domain generalization techniques.For visual recognition,most existing domain adaptation methods treat each dataset as one domain[23,17,16,10,11,12,6,7,24].However,the recent works show the images or videos in one dataset may come from multiple hidden domains[20,15].In[20],Hofffman et al.proposed a constrained clustering method to discover the latent domains and also extended[23]for multi-domain adapta-tion by learning multiple transformation matrices.In[15],Gong et al.partitioned D.Fleet et al.(Eds.):ECCV2014,Part III,LNCS8691,pp.628–643,2014.c Springer International Publishing Switzerland2014Exploiting Low-Rank Structure from Latent Domains629 the training samples from one domain into multiple domains by simultaneously maximizing distinctiveness and learnability.However,it is a non-trivial task to discover the characteristic latent domains by explicitly partitioning the training samples into multiple clusters because many factors(e.g.,pose and illumination) overlap and interact in images and videos in complex ways[15].In this work,we propose a new approach for domain generalization by explic-itly exploiting the intrinsic structure of positive samples from multiple latent do-mains without partitioning the training samples into multiple clusters/domains. Our work builds up the recent ensemble learning method exemplar-SVMs,in which we aim to train a set of exemplar classifiers with each classifier learnt by using one positive training sample and all negative training samples.While pos-itive samples may come from multiple latent domains characterized by different factors,for the positive samples captured under similar conditions(e.g.,frontal-view poses),their likelihoods from each exemplar classifier are expected to be similar to each ing the likelihoods from all the exemplar classifiers as the feature of each positive sample,we assume the likelihood matrix consisting of the features of all positive samples should be low-rank in the ideal case.Based on this assumption,we formulate a new objective function by introducing a nu-clear norm based regularizer on the likelihood matrix into the objective function of exemplar-SVMs in order to learn a set of more robust exemplar classifiers for domain generalization and domain adaptation.To solve the new optimization problem,we further introduce an intermediate variable F modelling the ideal likelihood matrix,and arrive at a relaxed objective function.Specifically,we minimize the objective function of exemplar-SVMs and the nuclear norm of the ideal likelihood matrix F as well as the approximation error between F and the likelihood matrix.Then,we develop an alternating optimization algorithm to iteratively solve the ideal likelihood matrix F and learn the exemplar classifiers.During the testing process,we can directly use the whole or a selected set of learnt exemplar classifiers for the domain generalization task when the tar-get domain samples are not available during the training process.For domain adaptation,we propose an effective method to re-weight the selected set of ex-emplar classifiers based on the Maximum Mean Discrepancy(MMD)criterion, and we further extend the Domain Adaptation Machine(DAM)method to learn an optimal target classifier.We conduct comprehensive experiments for object recognition and human activity recognition using two datasets and the results clearly demonstrate the effectiveness of our approach for domain generalization and domain adaptation.2Related WorkDomain adaptation methods can be roughly categorized into feature based ap-proaches and classifier based approaches.The feature based approaches aim to learn domain invariant features for domain adaptation.Kulis et al.[23]proposed a distance metric learning method to reduce domain distribution mismatch by630Z.Xu et al.learning asymmetric nonlinear transformation.Gopalan et al.[17]and Gong et al.[16]proposed two domain adaptation methods by interpolating intermediate domains.To reduce the distribution mismatch,some recent approaches learnt a domain invariant subspace[2]or aligned two subspaces from both domains[14].Classifier based approaches directly learn the classifiers for domain adapta-tion,among which SVM based approaches are the most popular ones.Huang et al.[21]proposed a domain adaptation approach by re-weighting the source domain samples and then learning a weighted SVM classifier with the learnt weights.Duan et al.[10]proposed a new method called Adaptive MKL based on multiple kernel learning(MKL)[32],and a multi-domain adaptation method by selecting the most relevant source domains[13].The work in[3]developed an approach to iteratively learn the SVM classifier by labeling the unlabeled target samples and simultaneously removing some labeled samples in the source domain.There are a few works specifically designed for domain generalization. To enhance the domain generalization ability,Muandet et al.proposed to learn new domain invariant feature representations[26].Given multiple source datasets/domains,Khosla et al.[22]proposed an SVM based approach,in which the learnt weight vectors that are common to all datasets can be used for domain generalization.Our work is more related to the recent works for discovering latent domains [20,15].In[20],a clustering based approach is proposed to divide the source do-main into different latent domains.In[15],the MMD criterion is used to partition the source domain into distinctive latent domains.However,their methods need to decide the number of latent domains beforehand.In contrast,our method ex-ploits the low-rank structure from latent domains without requiring the number of latent domains.Moreover,we directly learn the exemplar classifiers without partitioning the data into clusters/domains.Our work builds up the recent work on exemplar-SVMs[25].In contrast to[25],we introduce a nuclear norm based regularizer on the likelihood ma-trix in order to exploit the low-rank structure from latent domains for domain generalization.In multi-task learning,the nuclear norm based regularizer is also introduced to enforce the related tasks share similar weight vectors when learning the classifiers for multiple tasks[1,5].However,their works assume the training and testing samples come from the same distribution without considering the domain generalization or domain adaptation tasks.Moreover,our regularizer is on the likelihood matrix such that we can better exploit the structure of positive samples from multiple latent domains.3Low-Rank Exemplar-SVMsIn this section,we introduce the formulation of our low rank exemplar-SVMs as well as the optimization algorithm.For ease of representation,in the remain-der of this paper,we use a lowercase/uppercase letter in boldface to represent a vector/matrix.The transpose of a vector/matrix is denoted by using the su-perscript .A=[a ij]∈R m×n defines a matrix A with a ij being its(i,j)-thExploiting Low-Rank Structure from Latent Domains 631element for i =1,...,m and j =1,...,n .The element-wise product between two matrices A =[a ij ]∈R m ×n and B =[b ij ]∈R m ×n is defined as C =A ◦B ,where C =[c ij ]∈R m ×n and c ij =a ij b ij .3.1FormulationIn the exemplar-SVMs model,each exemplar classifier is learnt by using one positive training sample and all the negative training samples.Let S =S +∪S −denote the set of training samples,in which S +={s +1,...,s +n }is the set of positive training samples,and S −={s −1,...,s −m }is the set of negative training samples.Each training sample s +or s −is a d -dimensional column vector,i.e.,s +,s −∈R d .In this work,we use the logistic regression function for prediction.Given any sample t ∈R d ,the prediction function can be written as:p (t |w i )=11+exp(−w i t ),(1)where w i ∈R d is the weight vector in the i -th exemplar classifier trained byusing the positive training sample s +i and all negative training samples 1.Bydefining a weight matrix W =[w 1,...,w n ]∈R d ×n ,we formulate the learning problem as follows,min W J (W )=min W W 2F +C 1ni =1l (w i ,s +i )+C 2n i =1m j =1l (w i ,s −j ),(2)where · F is the Frobenius norm of a matrix,C 1and C 2are the tradeoffparameters analogous to C in SVM,and l (w ,s )is the logistic loss,which is defined as:l (w i ,s +i )=log(1+exp(−w i s +i )),(3)l (w i ,s −j )=log(1+exp(w i s −j )).(4)Now we consider how to discover the latent domains in the training data.Intuitively,if there are multiple latent domains in the training data,the positive training samples should also come from several latent domains.For the positive samples captured under similar conditions (e.g.,frontal-view poses),their like-lihoods from each exemplar classifier are expected to be similar to each ing the likelihoods from all the exemplar classifiers as the feature of each pos-itive sample,we assume the likelihood matrix consisting of the likelihoods of all positive samples should be low-rank in the ideal case.Formally,we denote the likelihood matrix as G (W )=[g ij ]∈R n ×n ,where each g ij =p (s +i |w j )is the likelihood of the i -th positive training sample by using the j -th exemplar classifier.To exploit those latent domains,we thus enforce the prediction matrix 1Although we do not explicitly use the bias term in the prediction,in our experiments we append 1to the feature vector of each training sample.632Z.Xu et al.G(W)to be low-rank when we learn those exemplar-SVMs,namely,we arrive at the following objective function,J(W)+λ G(W) ∗,(5)minWwhere we use the nuclear norm based regularizer G(W) ∗to approximate the rank of G(W).It has been shown that the nuclear norm is the best convex approximation of the rank function over the unit ball of matrices[27].However, it is a nontrivial task to solve the problem in(5),because the last term is a nuclear norm based regularizer on the likelihood matrix G(W)and G(W)is a non-linear term w.r.t.W.To solve the optimization problem in(5),we introduce an intermediate matrix F∈R n×n to model the ideal G(W)such that we can decompose the last term in(5)into two parts:on one hand,we expect the intermediate matrix F should be low-rank as we discussed above;on the other hand,we enforce the likelihood matrix G(W)to be close to the intermediate matrix F.Therefore,we reformulate the objective function as follows,J(W)+λ1 F ∗+λ2 F−G(W) 2F,(6) minW,Fwhich can be solved by alternatingly optimizing two subproblems w.r.t.W and F.Specifically,the optimization problem w.r.t.W does not contain the nuclear norm based regularizer,which makes the optimization much easier.Also,the nuclear norm based regularizer only depends on the intermediate matrix F rather than a non-linear term w.r.t.W(i.e.,the likelihood matrix G(W))as in(5),thus the optimization problem w.r.t.F can be readily solved by using the Singular Value Threshold(SVT)method[4](see Section3.2for the details).Discussions:To better understand our proposed approach,in Figure1,we show an example of the learnt likelihood matrix G(W)from the“check watch”category in the IXMAS multi-view dataset by using Cam0and Cam1as the source domain.After using the nuclear norm based regularizer,we observe the block diagonal property of the likelihood matrix G(W)in Figure1(a).In Fig-ure1(b),we also display some frames from the videos corresponding to the two blocks with large values in G(W).We observe that the videos sharing higher values in the matrix G(W)are also visually similar to each other.For example, thefirst two rows in Figure1(b)are the videos from similar poses.More inter-estingly,we also observe our algorithm can group similar videos from different views in one block(e.g.,the last three rows in Figure1(b)are the videos from the same actor),which demonstrates it is beneficial to exploit the latent source domains by using our approach.3.2OptimizationIn this section,we discuss how to optimize the problem in(6).We optimize(6) by iteratively updating W and F.The two subproblems w.r.t.W and F are described in detail as follows.Exploiting Low-Rank Structure from Latent Domains 633Fig.1.An illustration of the likelihood matrix G (W ),where we observe the block diagonal property of G (W )in (a).The frames from the videos corresponding to the two blocks with large values in G (W )are also visually similar to each other in (b).Update W:When F is fixed,the subproblem w.r.t.W can be written as,min W J (W )+λ2 G (W )−F 2F ,(7)where the matrix F is obtained at the k -th iteration,and G (W )is defined as in Section 3.1.We optimize the above subproblem by using the gradientdescent technique.Let us respectively define S 1=[s +1,...,s +n ]∈R d ×n and S 2=[s −1,...,s −m ]∈R d ×m as the data matrices of positive and negative trainingsamples,and also denote H (W )= G (W )−F 2F .Then,the gradients of the two terms in (7)can be derived as follows,∂J (W )∂W=2W +C 1S 1(P 1−I )+C 2S 2P 2,(8)∂H (W )∂W =2S 1(G (W )◦(11 −G (W ))◦(G (W )−F )),(9)where P 1=diag p (s +i |w i ) ∈R n ×n is a diagonal matrix with each diagonal entry being the prediction on one positive sample by using its correspondingexemplar classifier,P 2=[p (s −i |w j )]∈Rm ×n is the prediction matrix on all neg-ative training samples by using all exemplar classifiers,I ∈R n ×n is an identity matrix,and 1∈R n is a vector with all entries being 1.Update F:When W is fixed,we can calculate the matrix G =G (W )at first,then the subproblem w.r.t.F becomes,min F λ1 F ∗+λ2 F −G 2F ,(10)which can be readily solved by using the singular value thresholding (SVT)method [4,31].Specifically,let us denote the singular value decomposition of634Z.Xu et al.Algorithm1.Optimization for Low-rank Exemplar-SVMs(LRE-SVMs) Input:Training data S,and the parameters C1,C2,λ1,λ2.1.Initialize W←W0,where W0is obtained by solving(2).2.repeat3.Calculate the likelihood matrix G(W)based on the current W.4.Solve for F by optimizing the problem in(10)with the SVT method.5.Update W by solving the problem in(7)with the gradient descent method.6.until The objective converges or the maximum number of iterations is reached. Output:The weight matrix W.G as G=UΣV ,where U,V∈R n×n are two orthogonal matrices,and Σ=diag(σi)∈R n×n is a diagonal matrix containing all the singular values. The singular value thresholding operator on G can be represented as U D(Σ)V ,where D(Σ)=diag((σi−λ12λ2)+),and(·)+is a thresholding operator by assign-ing the negative elements to be zeros.Algorithm:We summarize the optimization procedure in Algorithm1and name our method as Low-rank Exemplar-SVMs(LRE-SVMs).Specifically,we first initialize the weight matrix W as W0,where W0is obtained by solving the traditional exemplar-SVMs formulation in(2).Then we calculate the prediction matrix G(W)by applying the learnt classifiers on all positive samples.Next,we obtain the matrix F by solving the problem in(10)with the SVT method.After that,we use the gradient descent method to update the weight matrix W.The above steps are repeated until the objective converges.4Ensemble Exemplar ClassifiersAfter training the low-rank exemplar-SVMs as described in Section3.1,we ob-tain n exemplar classifiers.To predict the test data,we discuss how to effectively use those learnt classifiers in two situations.One is the domain generalization scenario,where the target domain samples are not available during the training process.And the other one is the domain adaptation scenario,where we have unlabeled data in the target domain during the training process.4.1Domain GeneralizationIn the domain generalization scenario,we have no prior information about the target domain.A simple way is to equally fuse those n exemplar classifiers.Given any test sample t,the prediction p(t|W)can be calculated as,p(t|W)=1nni=1p(t|w i),(11)where p(t|w i)is the prediction from the i-th exemplar classifier.Exploiting Low-Rank Structure from Latent Domains635 Recall the training samples may come from several latent domains,a better way is to only use the exemplar classifiers in the latent domain which the test data likely belongs to.As mentioned before,on one hand,an exemplar classifier tends to output relatively higher prediction scores for the positive samples from the same latent domain,and relatively lower prediction scores for the positive samples from different latent domains;on the other hand,all exemplar classifiers are expected to output low prediction scores for the negative samples.Therefore, given the test sample t during the test process,it is beneficial to fuse only the exemplar classifiers that output higher predictions,such that we output a higher prediction score if t is positive,and a low prediction score if t is negative.Let us define T(t)={i|p(t|w i)is one of the top K prediction scores for i=1,...,n} as the set of the indices of those selected exemplar classifiers,then the prediction on this test sample can be obtained as,p(t|W)=1Ki:i∈T(t)p(t|w i),(12)where K is the predefined number of exemplar classifiers that output high pre-diction scores for the test sample t.4.2Domain AdaptationWhen we have unlabeled data in the target domain during the training process, we can further assign different weights to the learnt exemplar classifiers to betterfuse the exemplar classifiers for predicting the test data from the target domain. Intuitively,when the training data of one exemplar classifier is closer to thetarget domain,we should assign a higher weight to this classifier and vice versa.Let us denote the target domain samples as{t1,...,t u},where u is the number of samples in the target domain.Based on the Maximum Mean Discrepancy(MMD)criterion[18],we define the distance between the training data of oneexemplar classifier and the target domain as follows,d i=1n+m⎛⎝nφ(s+i)+mj=1φ(s−j)⎞⎠−1uuj=1φ(t j) 2,(13)whereφ(·)is a nonlinear feature mapping function induced by the Gaussiankernel.We assign a higher weight n to the positive sample s+i when calculatingthe mean of source domain samples,since we only use one positive sample for training the exemplar classifier at each time.In other words,we duplicate thepositive sample s+i for n times and then combine the duplicated positive sampleswith all the negative samples to calculate the distance with the target domain.With the above distance,we then obtain the weight for each exemplar classifier by using the RBF function as v i=exp(−d i/σ),whereσis the bandwidth parameter,and is set to be the median value of all distances.Then,the prediction on a test sample t can be obtained as,p(t|W)=i:i∈T(t)˜v i p(t|w i),(14)636Z.Xu et al.where T(t)is defined as in Section4.1,and˜v i=v i/i:i∈T(t)v i.One potential drawback with the above ensemble method is that we need to perform the predictions for n times,and then fuse the top K prediction scores. Inspired by Domain Adaptation Machine[13],we propose to learn a single target classifier on the target domain by leveraging the predictions from the exemplar classifiers.Specifically,let us denote the target classifier as f(t)=˜w φ(t)+b. We formulate our learning problem as follows,min ˜w,b,ξi,ξ∗i,f 12˜w 2+Cui=1(ξi+ξ∗i)+λ2Ω(f),(15)s.t.˜w φ(t i)+b−f i≤ +ξi,ξi≥0,(16)f i−˜w φ(t i)−b≤ +ξ∗i,ξ∗i≥0,(17) where f=[f1,...,f u] is an intermediate variable,λand C are the tradeoffparameters,ξi andξ∗i are the slack variables in the -insensitive loss similarly as in SVR,and is a predifined small positive value in the -insensitive loss.The regularizerΩ(f)is a smoothness function defined as follows,Ω(f)=uj=1i:i∈T(t j)˜v i(f j−p(t j|w i))2,(18)where we enforce each intermediate variable f j to be similar to the predictionscores of the selected exemplar classifiers in T(t j)for the target sample t j. In the above problem,we use the -insensitive loss to enforce the predictionscore from target classifier f(t j)=˜w φ(t j)+b to be close to the intermediate variable f j.At the same time,we also use a smoothness regularizer to enforcethe intermediate variable f j to be close to the prediction scores of the selectedexemplar classifiers in T(t j)for the target sample t j.Intuitively,when˜v i is large,we enforce the intermediate variable f j to be closer to p(t j|w i),and vice versa.Recall the weight˜v i models the importance of the i-th exemplar classifierfor predicting the target sample,we expect the learnt classifier f(t)performs well for predicting the target domain samples.By introducing the dual variablesα=[α1,...,αu] andα∗=[α∗1,...,α∗u] for the constraints in(16)and(17),we arrive at its dual form as follows,min α,α∗12(α−α∗)˜K(α−α∗)+p (α−α∗)+ 1 u(α+α∗),(19)s.t.1 α=1 α∗,0≤α,α∗≤C1,(20)where˜K=K+1λI∈R u×u with K being the kernel matrix of the target do-main samples,p=[p(t1|W),...,p(t u|W)] with each entry p(t j|W)defined in(14)being the“virtual label”for the target sample t j as in DAM[13].In DAM[13],the virtual labels of all the target samples are obtained by fusing the same set of source classifiers.In contrast,we use the predictions from different selected exemplar classifiers to obtain the virtual labels of different target sam-ples.Therefore,DAM can be treated as a special case of our work by using the same classifiers for all test samples in(15).Exploiting Low-Rank Structure from Latent Domains637 5ExperimentsIn this section,we evaluate our low-rank exemplar-SVMs(LRE-SVMs)approach for domain generalization and domain adaptation,respectively.5.1Experimental SetupFollowing the work in[15],we use the Office-Caltech dataset[28,16]for visual object recognition and the IXMAS dataset[30]for multi-view action recognition.Office-Caltech[28,16]dataset contains the images from four domains de-noted by A,C,D,and W,in which the images are from Amazon,Caltech-256, and two more datasets captured with digital SLR camera and webcam,respec-tively.The ten common categories among the4domains are utilized in our eval-uation.We extract the DeCAF6feature[9]for the images in the Office-Caltech dataset,which has achieved promising results in visual recognition.IXMAS dataset[30]contains the videos from eleven actions captured by five cameras(Cam0,Cam1,...,Cam4)from different viewpoints.Each of the eleven actions is performed three times by twelve actors.To exclude the irregularly performed actions,we keep thefirstfive actions(check watch,cross arms,scratch head,sit down,get up)performed by six actors(Alba,Andreas, Daniel,Hedlena,Julien,Nicolas),as suggested in[15].We extract the dense trajectories features[29]from the videos,and use K-means clustering to build a codebook with1,000clusters for each of thefive descriptors(i.e.,trajectory, HOG,HOF,MBHx,MBHy).The bag-of-words features are then concatenated to a5,000dimensional feature for each video sequence.Following[15],we treat the images from different sources in the Office-Caltech dataset as different domains,and treat the videos from different viewpoints in the IXMAS dataset as different domains,respectively.In our experiments,we mix several domains as the source domain for training the classifiers and use the remaining domains as the target domain for testing.For the domain gener-alization task,the samples from the target domain are not available during the training process.For the domain adaptation task,the unlabeled samples from the target domain can be used to reduce the domain distribution mismatch in the training process.We compare our low-rank exemplar-SVMs with several state-of-the-art unsu-pervised domain adaptation methods,as well as the methods specifically pro-posed for discovering the latent domains.Note that our approach does not require domain labels for both domain generalization and domain adaptation tasks. 5.2Domain GeneralizationWefirst evaluate our low-rank exemplar-SVMs(LRE-SVMs)for domain gen-eralization.We compare our proposed method with the domain generalization method by undoing dataset bias(Undo-Bias)in[22],and the latent domain discovering methods[20,15].We additionally report the results from the dis-criminative sub-categorization(Sub-C)method[19],as it can also be applied toTable1.Recognition accuracies(%)of different methods for domain generalization. Our LRE-SVMs approach does not require domain labels or target domain data during the training process.The best results are denoted in boldface.Source A,C D,W C,D,W Cam0,1Cam2,3,4Cam0,1,2,3Target D,W A,C A Cam2,3,4Cam0,1Cam4SVM82.6876.0690.7371.7063.8356.61 Sub-C[19]82.6178.6590.7578.1176.9064.04Undo-Bias[22]80.4969.9890.9869.0360.5656.84[20](Ensemble)79.2368.0680.7571.5551.0249.70[20](Match)71.2661.4272.0363.8160.0448.91[15](Ensemble)84.0177.1191.6575.0468.9857.64[15](Match)80.6376.5290.8471.5960.7355.37E-SVMs82.7380.8591.4776.8668.0472.98LRE-SVMs84.5981.1791.8779.9680.1574.97our application.As the Undo-Bias method[22]requires the domain label infor-mation,we provide the groundtruth domain labels to train the classifier for this method.For all other methods,we mix multiple domains as the source domain for training the classifiers.For the latent domain discovering methods[20,15],after partitioning the source domain data into different domains using their methods,we train an SVM classifier on each domain,and then fuse those classifiers for predicting the test samples.We employ two strategies to fuse the learnt classifiers as suggested in[15],which are referred to as the ensemble strategy and the match strategy, respectively.The ensemble strategy is to re-weight the decision values from dif-ferent SVM classifiers by using the domain probabilities learnt with the method in[20].In the match strategy,wefirst select the most relevant domain based on the MMD criterion,and then use the SVM classifier from this domain to predict the test samples.Moreover,we also report the results from the baseline SVM method,which is trained by using all training samples in the source domain.The results from exemplar-SVMs(E-SVMs)are also reported,which is a special case of our pro-posed LRE-SVMs,and we also use the method in(12)to fuse the selected top K exemplar classifiers for the prediction.For our method,we empirically fix K=5,and C1=10C2for all our experiments.We set the parameters C2=0.01,λ1=100,andλ2=1000on the Office-Caltech dataset,and C2=1 andλ1=λ2=10on the IXMAS datast.For baseline methods,we choose the optimal parameters according to their recognition accuracies on the test dataset.The experimental results on two datasets are summarized in Table1.We observe that the Sub-C method is comparable or better than the SVM method. The results of Undo-Bias are worse than SVM in most cases even after providing the ground-truth domain labels.One possible explanation is that there are many factors(e.g.,pose and illumination)interacting in images and videos in complex ways[15],so even the ground truth domain labels may not be the optimal。