Active Sampling for Feature Selection
人工智能领域中英文专有名词汇总
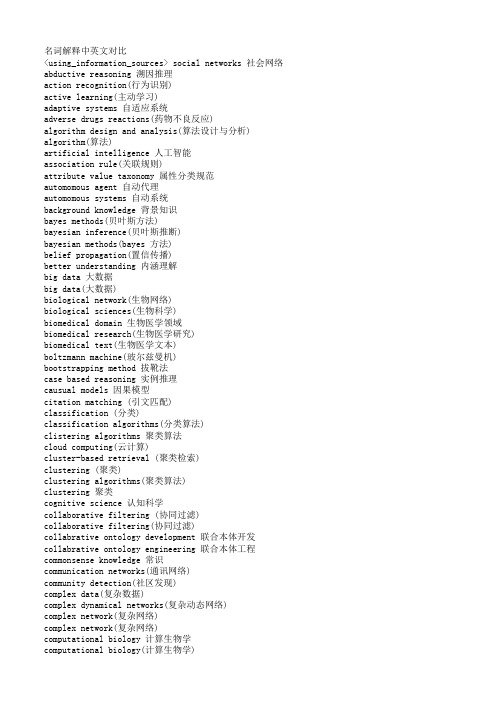
名词解释中英文对比<using_information_sources> social networks 社会网络abductive reasoning 溯因推理action recognition(行为识别)active learning(主动学习)adaptive systems 自适应系统adverse drugs reactions(药物不良反应)algorithm design and analysis(算法设计与分析) algorithm(算法)artificial intelligence 人工智能association rule(关联规则)attribute value taxonomy 属性分类规范automomous agent 自动代理automomous systems 自动系统background knowledge 背景知识bayes methods(贝叶斯方法)bayesian inference(贝叶斯推断)bayesian methods(bayes 方法)belief propagation(置信传播)better understanding 内涵理解big data 大数据big data(大数据)biological network(生物网络)biological sciences(生物科学)biomedical domain 生物医学领域biomedical research(生物医学研究)biomedical text(生物医学文本)boltzmann machine(玻尔兹曼机)bootstrapping method 拔靴法case based reasoning 实例推理causual models 因果模型citation matching (引文匹配)classification (分类)classification algorithms(分类算法)clistering algorithms 聚类算法cloud computing(云计算)cluster-based retrieval (聚类检索)clustering (聚类)clustering algorithms(聚类算法)clustering 聚类cognitive science 认知科学collaborative filtering (协同过滤)collaborative filtering(协同过滤)collabrative ontology development 联合本体开发collabrative ontology engineering 联合本体工程commonsense knowledge 常识communication networks(通讯网络)community detection(社区发现)complex data(复杂数据)complex dynamical networks(复杂动态网络)complex network(复杂网络)complex network(复杂网络)computational biology 计算生物学computational biology(计算生物学)computational complexity(计算复杂性) computational intelligence 智能计算computational modeling(计算模型)computer animation(计算机动画)computer networks(计算机网络)computer science 计算机科学concept clustering 概念聚类concept formation 概念形成concept learning 概念学习concept map 概念图concept model 概念模型concept modelling 概念模型conceptual model 概念模型conditional random field(条件随机场模型) conjunctive quries 合取查询constrained least squares (约束最小二乘) convex programming(凸规划)convolutional neural networks(卷积神经网络) customer relationship management(客户关系管理) data analysis(数据分析)data analysis(数据分析)data center(数据中心)data clustering (数据聚类)data compression(数据压缩)data envelopment analysis (数据包络分析)data fusion 数据融合data generation(数据生成)data handling(数据处理)data hierarchy (数据层次)data integration(数据整合)data integrity 数据完整性data intensive computing(数据密集型计算)data management 数据管理data management(数据管理)data management(数据管理)data miningdata mining 数据挖掘data model 数据模型data models(数据模型)data partitioning 数据划分data point(数据点)data privacy(数据隐私)data security(数据安全)data stream(数据流)data streams(数据流)data structure( 数据结构)data structure(数据结构)data visualisation(数据可视化)data visualization 数据可视化data visualization(数据可视化)data warehouse(数据仓库)data warehouses(数据仓库)data warehousing(数据仓库)database management systems(数据库管理系统)database management(数据库管理)date interlinking 日期互联date linking 日期链接Decision analysis(决策分析)decision maker 决策者decision making (决策)decision models 决策模型decision models 决策模型decision rule 决策规则decision support system 决策支持系统decision support systems (决策支持系统) decision tree(决策树)decission tree 决策树deep belief network(深度信念网络)deep learning(深度学习)defult reasoning 默认推理density estimation(密度估计)design methodology 设计方法论dimension reduction(降维) dimensionality reduction(降维)directed graph(有向图)disaster management 灾害管理disastrous event(灾难性事件)discovery(知识发现)dissimilarity (相异性)distributed databases 分布式数据库distributed databases(分布式数据库) distributed query 分布式查询document clustering (文档聚类)domain experts 领域专家domain knowledge 领域知识domain specific language 领域专用语言dynamic databases(动态数据库)dynamic logic 动态逻辑dynamic network(动态网络)dynamic system(动态系统)earth mover's distance(EMD 距离) education 教育efficient algorithm(有效算法)electric commerce 电子商务electronic health records(电子健康档案) entity disambiguation 实体消歧entity recognition 实体识别entity recognition(实体识别)entity resolution 实体解析event detection 事件检测event detection(事件检测)event extraction 事件抽取event identificaton 事件识别exhaustive indexing 完整索引expert system 专家系统expert systems(专家系统)explanation based learning 解释学习factor graph(因子图)feature extraction 特征提取feature extraction(特征提取)feature extraction(特征提取)feature selection (特征选择)feature selection 特征选择feature selection(特征选择)feature space 特征空间first order logic 一阶逻辑formal logic 形式逻辑formal meaning prepresentation 形式意义表示formal semantics 形式语义formal specification 形式描述frame based system 框为本的系统frequent itemsets(频繁项目集)frequent pattern(频繁模式)fuzzy clustering (模糊聚类)fuzzy clustering (模糊聚类)fuzzy clustering (模糊聚类)fuzzy data mining(模糊数据挖掘)fuzzy logic 模糊逻辑fuzzy set theory(模糊集合论)fuzzy set(模糊集)fuzzy sets 模糊集合fuzzy systems 模糊系统gaussian processes(高斯过程)gene expression data 基因表达数据gene expression(基因表达)generative model(生成模型)generative model(生成模型)genetic algorithm 遗传算法genome wide association study(全基因组关联分析) graph classification(图分类)graph classification(图分类)graph clustering(图聚类)graph data(图数据)graph data(图形数据)graph database 图数据库graph database(图数据库)graph mining(图挖掘)graph mining(图挖掘)graph partitioning 图划分graph query 图查询graph structure(图结构)graph theory(图论)graph theory(图论)graph theory(图论)graph theroy 图论graph visualization(图形可视化)graphical user interface 图形用户界面graphical user interfaces(图形用户界面)health care 卫生保健health care(卫生保健)heterogeneous data source 异构数据源heterogeneous data(异构数据)heterogeneous database 异构数据库heterogeneous information network(异构信息网络) heterogeneous network(异构网络)heterogenous ontology 异构本体heuristic rule 启发式规则hidden markov model(隐马尔可夫模型)hidden markov model(隐马尔可夫模型)hidden markov models(隐马尔可夫模型) hierarchical clustering (层次聚类) homogeneous network(同构网络)human centered computing 人机交互技术human computer interaction 人机交互human interaction 人机交互human robot interaction 人机交互image classification(图像分类)image clustering (图像聚类)image mining( 图像挖掘)image reconstruction(图像重建)image retrieval (图像检索)image segmentation(图像分割)inconsistent ontology 本体不一致incremental learning(增量学习)inductive learning (归纳学习)inference mechanisms 推理机制inference mechanisms(推理机制)inference rule 推理规则information cascades(信息追随)information diffusion(信息扩散)information extraction 信息提取information filtering(信息过滤)information filtering(信息过滤)information integration(信息集成)information network analysis(信息网络分析) information network mining(信息网络挖掘) information network(信息网络)information processing 信息处理information processing 信息处理information resource management (信息资源管理) information retrieval models(信息检索模型) information retrieval 信息检索information retrieval(信息检索)information retrieval(信息检索)information science 情报科学information sources 信息源information system( 信息系统)information system(信息系统)information technology(信息技术)information visualization(信息可视化)instance matching 实例匹配intelligent assistant 智能辅助intelligent systems 智能系统interaction network(交互网络)interactive visualization(交互式可视化)kernel function(核函数)kernel operator (核算子)keyword search(关键字检索)knowledege reuse 知识再利用knowledgeknowledgeknowledge acquisitionknowledge base 知识库knowledge based system 知识系统knowledge building 知识建构knowledge capture 知识获取knowledge construction 知识建构knowledge discovery(知识发现)knowledge extraction 知识提取knowledge fusion 知识融合knowledge integrationknowledge management systems 知识管理系统knowledge management 知识管理knowledge management(知识管理)knowledge model 知识模型knowledge reasoningknowledge representationknowledge representation(知识表达) knowledge sharing 知识共享knowledge storageknowledge technology 知识技术knowledge verification 知识验证language model(语言模型)language modeling approach(语言模型方法) large graph(大图)large graph(大图)learning(无监督学习)life science 生命科学linear programming(线性规划)link analysis (链接分析)link prediction(链接预测)link prediction(链接预测)link prediction(链接预测)linked data(关联数据)location based service(基于位置的服务) loclation based services(基于位置的服务) logic programming 逻辑编程logical implication 逻辑蕴涵logistic regression(logistic 回归)machine learning 机器学习machine translation(机器翻译)management system(管理系统)management( 知识管理)manifold learning(流形学习)markov chains 马尔可夫链markov processes(马尔可夫过程)matching function 匹配函数matrix decomposition(矩阵分解)matrix decomposition(矩阵分解)maximum likelihood estimation(最大似然估计)medical research(医学研究)mixture of gaussians(混合高斯模型)mobile computing(移动计算)multi agnet systems 多智能体系统multiagent systems 多智能体系统multimedia 多媒体natural language processing 自然语言处理natural language processing(自然语言处理) nearest neighbor (近邻)network analysis( 网络分析)network analysis(网络分析)network analysis(网络分析)network formation(组网)network structure(网络结构)network theory(网络理论)network topology(网络拓扑)network visualization(网络可视化)neural network(神经网络)neural networks (神经网络)neural networks(神经网络)nonlinear dynamics(非线性动力学)nonmonotonic reasoning 非单调推理nonnegative matrix factorization (非负矩阵分解) nonnegative matrix factorization(非负矩阵分解) object detection(目标检测)object oriented 面向对象object recognition(目标识别)object recognition(目标识别)online community(网络社区)online social network(在线社交网络)online social networks(在线社交网络)ontology alignment 本体映射ontology development 本体开发ontology engineering 本体工程ontology evolution 本体演化ontology extraction 本体抽取ontology interoperablity 互用性本体ontology language 本体语言ontology mapping 本体映射ontology matching 本体匹配ontology versioning 本体版本ontology 本体论open government data 政府公开数据opinion analysis(舆情分析)opinion mining(意见挖掘)opinion mining(意见挖掘)outlier detection(孤立点检测)parallel processing(并行处理)patient care(病人医疗护理)pattern classification(模式分类)pattern matching(模式匹配)pattern mining(模式挖掘)pattern recognition 模式识别pattern recognition(模式识别)pattern recognition(模式识别)personal data(个人数据)prediction algorithms(预测算法)predictive model 预测模型predictive models(预测模型)privacy preservation(隐私保护)probabilistic logic(概率逻辑)probabilistic logic(概率逻辑)probabilistic model(概率模型)probabilistic model(概率模型)probability distribution(概率分布)probability distribution(概率分布)project management(项目管理)pruning technique(修剪技术)quality management 质量管理query expansion(查询扩展)query language 查询语言query language(查询语言)query processing(查询处理)query rewrite 查询重写question answering system 问答系统random forest(随机森林)random graph(随机图)random processes(随机过程)random walk(随机游走)range query(范围查询)RDF database 资源描述框架数据库RDF query 资源描述框架查询RDF repository 资源描述框架存储库RDF storge 资源描述框架存储real time(实时)recommender system(推荐系统)recommender system(推荐系统)recommender systems 推荐系统recommender systems(推荐系统)record linkage 记录链接recurrent neural network(递归神经网络) regression(回归)reinforcement learning 强化学习reinforcement learning(强化学习)relation extraction 关系抽取relational database 关系数据库relational learning 关系学习relevance feedback (相关反馈)resource description framework 资源描述框架restricted boltzmann machines(受限玻尔兹曼机) retrieval models(检索模型)rough set theroy 粗糙集理论rough set 粗糙集rule based system 基于规则系统rule based 基于规则rule induction (规则归纳)rule learning (规则学习)rule learning 规则学习schema mapping 模式映射schema matching 模式匹配scientific domain 科学域search problems(搜索问题)semantic (web) technology 语义技术semantic analysis 语义分析semantic annotation 语义标注semantic computing 语义计算semantic integration 语义集成semantic interpretation 语义解释semantic model 语义模型semantic network 语义网络semantic relatedness 语义相关性semantic relation learning 语义关系学习semantic search 语义检索semantic similarity 语义相似度semantic similarity(语义相似度)semantic web rule language 语义网规则语言semantic web 语义网semantic web(语义网)semantic workflow 语义工作流semi supervised learning(半监督学习)sensor data(传感器数据)sensor networks(传感器网络)sentiment analysis(情感分析)sentiment analysis(情感分析)sequential pattern(序列模式)service oriented architecture 面向服务的体系结构shortest path(最短路径)similar kernel function(相似核函数)similarity measure(相似性度量)similarity relationship (相似关系)similarity search(相似搜索)similarity(相似性)situation aware 情境感知social behavior(社交行为)social influence(社会影响)social interaction(社交互动)social interaction(社交互动)social learning(社会学习)social life networks(社交生活网络)social machine 社交机器social media(社交媒体)social media(社交媒体)social media(社交媒体)social network analysis 社会网络分析social network analysis(社交网络分析)social network(社交网络)social network(社交网络)social science(社会科学)social tagging system(社交标签系统)social tagging(社交标签)social web(社交网页)sparse coding(稀疏编码)sparse matrices(稀疏矩阵)sparse representation(稀疏表示)spatial database(空间数据库)spatial reasoning 空间推理statistical analysis(统计分析)statistical model 统计模型string matching(串匹配)structural risk minimization (结构风险最小化) structured data 结构化数据subgraph matching 子图匹配subspace clustering(子空间聚类)supervised learning( 有support vector machine 支持向量机support vector machines(支持向量机)system dynamics(系统动力学)tag recommendation(标签推荐)taxonmy induction 感应规范temporal logic 时态逻辑temporal reasoning 时序推理text analysis(文本分析)text anaylsis 文本分析text classification (文本分类)text data(文本数据)text mining technique(文本挖掘技术)text mining 文本挖掘text mining(文本挖掘)text summarization(文本摘要)thesaurus alignment 同义对齐time frequency analysis(时频分析)time series analysis( 时time series data(时间序列数据)time series data(时间序列数据)time series(时间序列)topic model(主题模型)topic modeling(主题模型)transfer learning 迁移学习triple store 三元组存储uncertainty reasoning 不精确推理undirected graph(无向图)unified modeling language 统一建模语言unsupervisedupper bound(上界)user behavior(用户行为)user generated content(用户生成内容)utility mining(效用挖掘)visual analytics(可视化分析)visual content(视觉内容)visual representation(视觉表征)visualisation(可视化)visualization technique(可视化技术) visualization tool(可视化工具)web 2.0(网络2.0)web forum(web 论坛)web mining(网络挖掘)web of data 数据网web ontology lanuage 网络本体语言web pages(web 页面)web resource 网络资源web science 万维科学web search (网络检索)web usage mining(web 使用挖掘)wireless networks 无线网络world knowledge 世界知识world wide web 万维网world wide web(万维网)xml database 可扩展标志语言数据库附录 2 Data Mining 知识图谱(共包含二级节点15 个,三级节点93 个)间序列分析)监督学习)领域 二级分类 三级分类。
Feature Selection for Machine Learning Comparing a Correlation-based Filter Approach to the

Algorithms that perform feature selection as a preprocessing step prior to learning can generally be placed into one of two broad categories. One approach, referred to as the wrapper (John, Kohavi, and P eger, 1994) employs|as a subroutine|a statistical re-sampling technique (such as cross validation) using the actual target learning algorithm to estimate the accuracy of feature subsets. This approach has proved useful but is very slow to execute because the learning algorithm is called repeatedly. For this reason, wrappers do not scale well to large datasets containing many features. Another approach, called the lter (John, Kohavi, and P eger, 1994), operates independently of any learning algorithm|undesirable features are ltered out of the data before induction commences. Filters typically make use of all the available training data when selecting a subset of features. Some look for consistency in the data|that is, they note when every combination of values for a feature subset is associated with a single class label (Almuallim and Dietterich, 1992). Another method (Koller and Sahami, 1996) eliminates features whose information content is subsumed by some number of the remaining features. Still other methods attempt to rank features according to a relevancy score (Kira and Rendell, 1992 Holmes and Nevill-Manning, 1995). Filters have proven to be much faster than wrappers and hence can be applied to large data sets containing many features. Their general nature allow them to be used with any learner, unlike the wrapper, which must be re-run when switching from one learning algorithmarch for good features. The results presented in this paper show that CFS compares favourably with the wrapper but requires far less computation.
随机森林构建方法英语作文
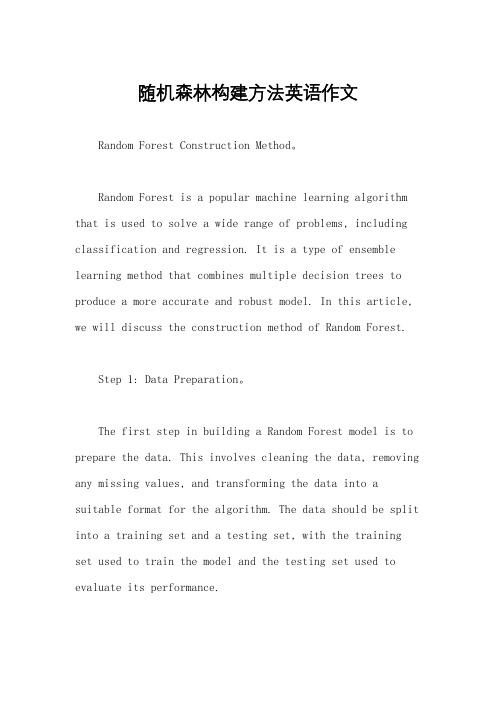
随机森林构建方法英语作文Random Forest Construction Method。
Random Forest is a popular machine learning algorithm that is used to solve a wide range of problems, including classification and regression. It is a type of ensemble learning method that combines multiple decision trees to produce a more accurate and robust model. In this article, we will discuss the construction method of Random Forest.Step 1: Data Preparation。
The first step in building a Random Forest model is to prepare the data. This involves cleaning the data, removing any missing values, and transforming the data into a suitable format for the algorithm. The data should be split into a training set and a testing set, with the training set used to train the model and the testing set used to evaluate its performance.Step 2: Random Sampling。
Random Forest uses a technique called bagging, which involves randomly sampling the data with replacement to create multiple subsets of the data. Each subset is used to train a decision tree, and the results are combined to produce the final model. The number of subsets is determined by the user and is typically set to a value between 100 and 1000.Step 3: Decision Tree Construction。
随机森林算法改进综述
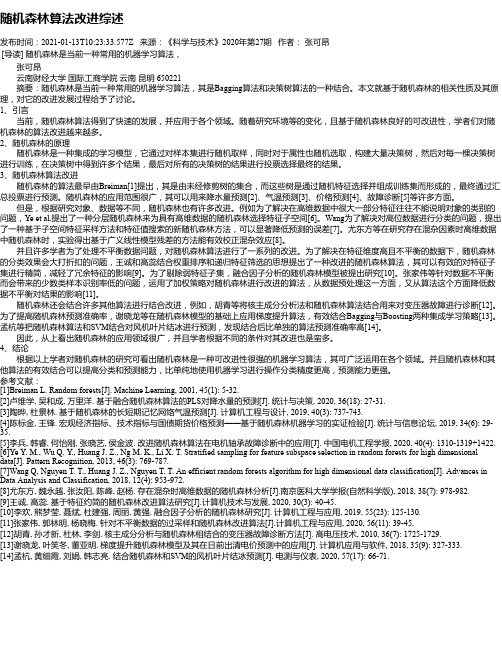
随机森林算法改进综述发布时间:2021-01-13T10:23:33.577Z 来源:《科学与技术》2020年第27期作者:张可昂[导读] 随机森林是当前一种常用的机器学习算法,张可昂云南财经大学国际工商学院云南昆明 650221摘要:随机森林是当前一种常用的机器学习算法,其是Bagging算法和决策树算法的一种结合。
本文就基于随机森林的相关性质及其原理,对它的改进发展过程给予了讨论。
1、引言当前,随机森林算法得到了快速的发展,并应用于各个领域。
随着研究环境等的变化,且基于随机森林良好的可改进性,学者们对随机森林的算法改进越来越多。
2、随机森林的原理随机森林是一种集成的学习模型,它通过对样本集进行随机取样,同时对于属性也随机选取,构建大量决策树,然后对每一棵决策树进行训练,在决策树中得到许多个结果,最后对所有的决策树的结果进行投票选择最终的结果。
3、随机森林算法改进随机森林的算法最早由Breiman[1]提出,其是由未经修剪树的集合,而这些树是通过随机特征选择并组成训练集而形成的,最终通过汇总投票进行预测。
随机森林的应用范围很广,其可以用来降水量预测[2]、气温预测[3]、价格预测[4]、故障诊断[5]等许多方面。
但是,根据研究对象、数据等不同,随机森林也有许多改进。
例如为了解决在高维数据中很大一部分特征往往不能说明对象的类别的问题,Ye et al.提出了一种分层随机森林来为具有高维数据的随机森林选择特征子空间[6]。
Wang为了解决对高位数据进行分类的问题,提出了一种基于子空间特征采样方法和特征值搜索的新随机森林方法,可以显著降低预测的误差[7]。
尤东方等在研究存在混杂因素时高维数据中随机森林时,实验得出基于广义线性模型残差的方法能有效校正混杂效应[8]。
并且许多学者为了处理不平衡数据问题,对随机森林算法进行了一系列的改进。
为了解决在特征维度高且不平衡的数据下,随机森林的分类效果会大打折扣的问题,王诚和高蕊结合权重排序和递归特征筛选的思想提出了一种改进的随机森林算法,其可以有效的对特征子集进行精简,减轻了冗余特征的影响[9]。
CAD英文翻译
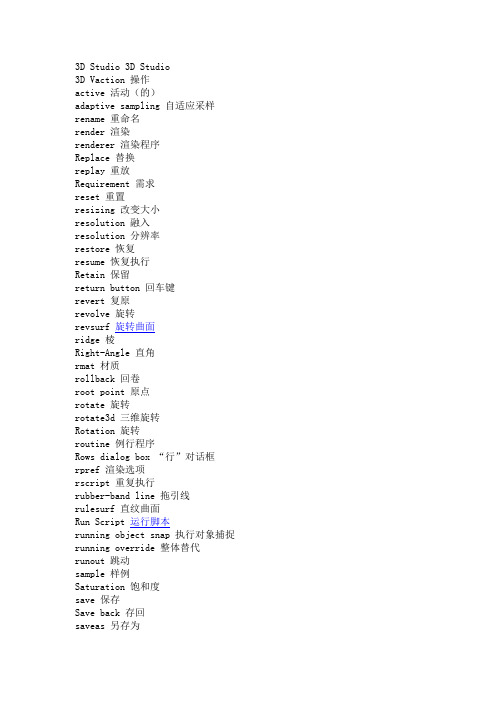
3D Studio 3D Studio3D Vaction 操作active 活动(的)adaptive sampling 自适应采样rename 重命名render 渲染renderer 渲染程序Replace 替换replay 重放Requirement 需求reset 重置resizing 改变大小resolution 融入resolution 分辨率restore 恢复resume 恢复执行Retain 保留return button 回车键revert 复原revolve 旋转revsurf 旋转曲面ridge 棱Right-Angle 直角rmat 材质rollback 回卷root point 原点rotate 旋转rotate3d 三维旋转Rotation 旋转routine 例行程序Rows dialog box “行”对话框rpref 渲染选项rscript 重复执行rubber-band line 拖引线rulesurf 直纹曲面Run Script 运行脚本running object snap 执行对象捕捉running override 整体替代runout 跳动sample 样例Saturation 饱和度save 保存Save back 存回saveas 另存为saveimg 保存图像scale 比例缩放,(缩放)比例 if used scale factor 比例因子Scaled to Fit 按图纸空间缩放scan 扫描Scatter 散布图scene 场景schema 模式schema 模式screen 屏幕script 脚本script files 脚本文件scroll bar 滚动条SDI(MDI) 单文档界面(多文档界面)SE Isometric 东南等轴测SE Isometric 东南等轴测Search for Help on 搜索帮助section 区域,部分,节(相对于章节)section 切割section 截面see 参见segment 段,线段select 选择select all 全部选择Select object 对象选择selectable 可选择的selection 选择集,选择selection sets 选择集Separate 分割Separate 分割Serial number 序列号session 任务set 设置(v),集合(n)setting 设置shade 着色,灰度(用于单色 Gray ) shader 着色程序shadow map 阴影贴图shape 形Sharpness 尖锐度sheet 表,板(for ACIS only)Shell 抽壳shell SHELLShell 抽壳shortcut 快捷键show 显示Silhouette 轮廓Single Face 单一表面single-pen plotter 单笔式绘图仪size 数目sizing 调整大小sketch 徒手画slice 剖切面(as n.)slice 剖切slide 幻灯片slide libraries 幻灯库smooth shading 平滑着色smoothing angle 平滑角度snap 捕捉snap angle 捕捉角度snap grid 捕捉栅格Snap mode 捕捉模式snap resolution 捕捉分辨率SnapTips 捕捉提示solid 填充solid (二维)填充/(三维)实体Solid Fill 实体填充solid modeler 实体建模solids 实体sorting 排序source applications 源文件source point 源点space 空间spacing 间距Special Edit 特定编辑specific 指定(的)specific 特有的specify 指定specular reflection 镜面反射(高光)Spelling 拼写检查sphere 球面spherical projection 球面投影spline 样条曲线spline frame 样条曲线框架splinedit 样条编辑Split 拆分spooler 缓冲(文件)spotlight anglesstack 堆栈Stack 堆叠stacked text 叠式文本stamp 戳记Standard 标准Start 起点start angle 起点角度start tangent 起点切向Start Up dialog box “启动”对话框starting 起始statements 状态说明statistics 统计信息stats 统计status 状态stlout STL 输出Stochastic 随机Stone Color 石质颜色straighten 拉直stretch 拉伸Strikeout Maybe 删去style 样式substitute 替换subtract 差集Suffix 后缀support directory 支持目录support files 支持文件suppress 禁止suppress 不输出Suppress 收缩surface 曲面SW Isometric 西南等轴测SW Isometric 西南等轴测swap file 交换文件swatch 样本sweep 延伸sweep 抹去。
主动选择 的英语表达
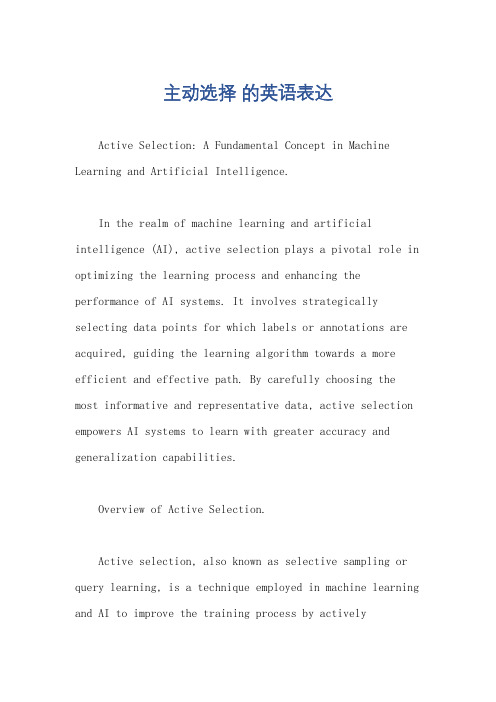
主动选择的英语表达Active Selection: A Fundamental Concept in Machine Learning and Artificial Intelligence.In the realm of machine learning and artificial intelligence (AI), active selection plays a pivotal role in optimizing the learning process and enhancing the performance of AI systems. It involves strategically selecting data points for which labels or annotations are acquired, guiding the learning algorithm towards a more efficient and effective path. By carefully choosing the most informative and representative data, active selection empowers AI systems to learn with greater accuracy and generalization capabilities.Overview of Active Selection.Active selection, also known as selective sampling or query learning, is a technique employed in machine learning and AI to improve the training process by activelyselecting the most valuable data points for labeling or annotation. Rather than passively accepting all available data, active selection algorithms prioritize data points that are most likely to reduce the uncertainty of the model and enhance its learning outcomes.The core principle of active selection is to identify data points that:Are highly informative: These data points carry a significant amount of information that can help the learning algorithm discern patterns and make accurate predictions.Are representative of the underlying distribution: Active selection aims to select data points that are representative of the entire dataset, ensuring that the model learns from a diverse set of examples.Minimize redundancy: Active selection algorithms avoid choosing data points that are similar to those already labeled or annotated, preventing the model from overfittingto specific examples.Benefits of Active Selection.Incorporating active selection into machine learning and AI systems offers a multitude of benefits, including:Improved model accuracy: By focusing on the most informative data points, active selection helps the learning algorithm build models with higher predictive accuracy.Reduced labeling or annotation costs: Active selection algorithms efficiently identify the most valuable data points, minimizing the need for manual labeling or annotation efforts, thereby saving time and resources.Faster training time: By selecting the most informative data, active selection enables the learning algorithm to converge to an optimal solution more quickly, reducing training time and improving efficiency.Enhanced generalization capabilities: Active selection helps the model learn from a diverse set of data, leadingto better generalization capabilities and improved performance on unseen data.Types of Active Selection Algorithms.A variety of active selection algorithms have been developed to suit different machine learning models anddata types. Some common algorithms include:Uncertainty sampling: This algorithm selects datapoints that the model is most uncertain about, encouraging the model to explore areas where it has limited knowledge.Query by committee: This algorithm leverages multiple models to identify data points on which the models disagree, indicating the need for additional information.Expected model change: This algorithm selects data points that are expected to have the greatest impact on the model's predictions, leading to more targeted learning.Representative sampling: This algorithm aims to select data points that are representative of the entire dataset, ensuring that the model is exposed to a diverse range of examples.Applications of Active Selection.Active selection finds applications in a wide range of machine learning and AI tasks, including:Natural language processing (NLP): Identifying the most informative sentences or documents for annotation to improve text understanding and classification.Computer vision: Selecting the most representative images or video frames for annotation to enhance object detection, image classification, and scene understanding.Medical imaging: Prioritizing the most critical medical images for analysis to improve disease diagnosis and prognosis.Speech recognition: Identifying the most challenging speech segments for annotation to enhance speech recognition accuracy.Recommender systems: Selecting the most relevant items or users for feedback to improve personalized recommendations.Conclusion.Active selection is a powerful technique in machine learning and AI that enables systems to learn more effectively and efficiently. By strategically selecting the most valuable data points for labeling or annotation, active selection algorithms reduce labeling or annotation costs, improve model accuracy, enhance generalization capabilities, and accelerate training time. As the field of AI continues to evolve, active selection will play an increasingly important role in optimizing the performance and efficiency of AI systems across a wide range of applications.。
Navigator 600磷酸盐分析器说明书
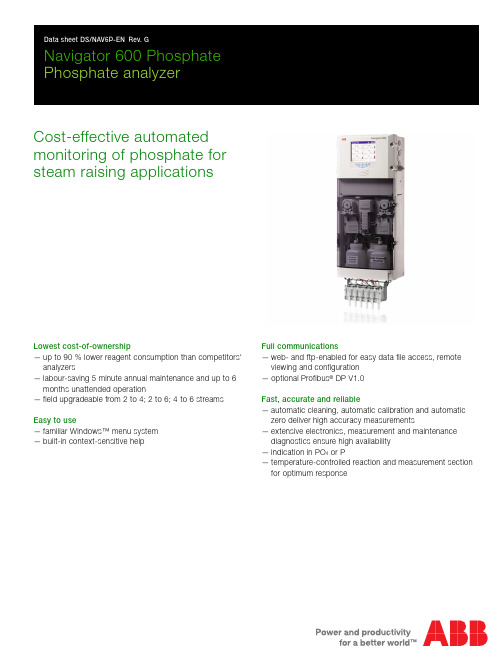
Navigator 600 Phosphate Phosphate analyzerCost-effective automated monitoring of phosphate forsteam raising applicationsLowest cost-of-ownership—up to 90 % lower reagent consumption than competitors' analyzers—labour-saving 5 minute annual maintenance and up to 6 months unattended operation—field upgradeable from 2 to 4; 2 to 6; 4 to 6 streams Easy to use—familiar Windows™ menu system —built-in context-sensitive helpFull communications—web- and ftp-enabled for easy data file access, remote viewing and configuration —optional Profibus ® DP V1.0Fast, accurate and reliable—automatic cleaning, automatic calibration and automatic zero deliver high accuracy measurements—extensive electronics, measurement and maintenance diagnostics ensure high availability —indication in PO 4 or P—temperature-controlled reaction and measurement section for optimum responseNavigator 600 Phosphate Phosphate analyzer2DS/NAV6P–EN Rev. GIntroductionMany years of experience and innovation in the design and successful application of continuous chemical analyzers has been combined with the latest electronics and production technologies to produce the Navigator 600 Series of analyzers from ABB.Developed as fully continuous analyzers offering wide dynamic ranging, the Navigator 600 Series incorporates greater simplicity and functionality than ever before. Based on colorimetric techniques, they feature a liquid-handling section carefully designed to reduce routine maintenance. Utilizing powerful electronics, advanced features such as automatic calibration,continuous sample analysis and programmable multi-stream switching ensure accurate and simple measurement of phosphate.Process data, as well as the content of alarm and audit logs,can be saved to a removable SD card in binary and comma-delimited formats for record keeping and analysis using ABB’s DataManager data analysis software package.A very low cost of ownership has been achieved by reducing the reagent consumption and simplifying the maintenance requirements.The size of the analayzer has been reduced to a compact,ergonomically-designed, wall-mounted case containing all the components.OperationGeneralThe Navigator 600 Phosphate is an on-line analyzer, designed to provide continuous monitoring of phosphate concentration,utilizing a standard colorimetric analysis principle.Liquid HandlingThe chemistry employed for phosphate measurement is the industry standard Molybdenum Blue reaction. Sample and reagents are drawn into the analyzer by two multichannel peristaltic pumps. These are designed and constructed to ensure that only simple yearly maintenance is required. The reagents are added to the sample in a temperature-controlled reaction block and the fully reacted sample is then passed through an in-line measuring cuvette.The optical measuring system enables accurate detection of phosphate concentrations from 0 to 15 ppm phosphate (PO 4)(0to 5ppm phosphorous [P]).The analyzer also includes a manual sampling facility that enables the analysis of grab samples.Solution ReplacementLiquid Handling Section ContinuousReagents3 months Calibration Standard 3 months Cleaning Solution3 monthsNavigator 600 Phosphate Phosphate analyzerDS/NAV6P–EN Rev. G 3ElectronicsThe electronics are mounted on the analyzer's back plate with the display and key pad accessible from the front of the unit.Indication of all parameters is provided by a large backlit LCD display that is easy to read in all light conditions. Under normal operating conditions, measured values are displayed;programming data is displayed during set-up and also on demand. Units and range of measurement, alarm values and standard solution values are examples of the many programmable functions.Keeping simplicity of operation at the forefront of design, six fingertip-operated tactile membrane switches control local operation of the analyzer and provide easy access to all parameters.The Navigator 600 Phosphate is provided with 4 dedicated relays, 6user-programmable relays and 6 current outputs as standard. Profibus DP V1.0 is available as an option.Ethernet CommunicationsThe Navigator 600 Phosphate can provide 10BaseT Ethernet communications via a standard RJ45 connector and uses industry-standard protocols TCP/IP , FTP and HTTP . The use of standard protocols enables easy connection into existing PC networks.Data File Access via FTP (File Transfer Protocol)The Navigator 600 Phosphate features FTP server functionality.The FTP server in the analyzer is used to access its file system from a remote station on a network. This requires an FTP client on the host PC. Both MS-DOS® and Microsoft® Internet Explorer version 5.5 or later can be used as an FTP client.⏹Using a standard web-browser or other F TP client, datafiles contained within the analyzer's memory or memory card can be accessed remotely and transferred to a PC or network drive.⏹Four individual FTP users' names and passwords can beprogrammed into the Navigator 600 Phosphate. An access level can be configured for each user.⏹All FTP log-on activity is recorded in the audit log of theinstrument.⏹Using ABB’s data file transfer scheduler program, datafiles from multiple instruments can be backed-up automatically to a PC or network drive for long-term storage, ensuring the security of valuable process data and minimizing the operator intervention required.Display and KeypadChart View DisplayNavigator 600 Phosphate(FTP Server)Navigator 600 Phosphate(FTP Server)FTP ClientEthernetNavigator 600 Phosphate Phosphate analyzer4DS/NAV6P–EN Rev. GEmbedded Web ServerThe Navigator 600 Phosphate has an embedded web-server that provides access to web pages created within the instrument. The use of HTTP (Hyper Text Transfer Protocol)enables standard web browsers to view these pages.⏹Accessible through the web pages are the current displayof the analyzer, detailed information on stream values,reagent and solution levels, measurement status and other key information.⏹The audit and alarm logs stored in the Navigator 600Phosphate's internal buffer memory and memory card can be viewed on the web pages.⏹Operator messages can be entered via the web server,enabling comments to be logged to the instrument.⏹The web pages and the information they contain arerefreshed regularly, enabling them to be used as a supervision tool.⏹The analyzer's configuration can be selected from anexisting configuration in the internal memory or a new configuration file transferred to the instrument via FTP .⏹The analyzer's real-time clock can be set via the webserver. Alternatively, the clocks of multiple analyzers can be synchronized using ABB's F ile Transfer Scheduler software.Email NotificationVia the Navigator 600 Phosphate's built-in SMTP client, the analyzer is able to email notification of important events. Emails triggered from alarms or other critical events can be sent to multiple recipients. The analyzer can also be programmed to email reports of the current measurement status or other parameters at specific times during the day.ProfibusThe Navigator 600 Phosphate can be equipped (option) with Profibus DP V1.0 to enable full communications and controlintegration with distributed control systems.Navigator 600 Phosphate Phosphate analyzerDS/NAV6P–EN Rev. G 5MaintenanceThe analyzer has been designed to maximize on-line availability by reducing routine maintenance to a minimum.Yearly maintenance consists of simply replacing pump capstans and pump tube assemblies, an operation that can take as little as five minutes.F ully automatic calibration, zeroing and cleaning functions enable the analyzer to keep itself operational with minimal manual intervention. A predictive alarm alerts the user before cleaning, calibration or reagent solution replacement is required.OptionsMulti-stream FacilityA fully programmable multi-stream option is available on the Navigator 600 Phosphate on-line analyzer, providing up to six-stream capability including individual current output and visual indication as well as user-programmable stream sequencing.The analyzers are designed to be easily upgradeable in the field from 2 to 4 or 6 streams and from 4 to 6 streams.Simple to Replace Pump Tube AssembliesSix Streams DisplayNavigator 600 Phosphate Phosphate analyzer6DS/NAV6P–EN Rev. GSpecificationPhosphate MeasurementRangeFully user programmable 0 ... 15 ppm PO 4 (0 ... 5 ppm P), minimum range 0 ... 1 ppm PO 4 ( 0 ... 0.33 ppm P)Measurement ModesContinuousContinuous measurement operationSample stream selectionAvailable in 1, 2, 4 or 6 streams (4 and 6 stream configurations can accommodate 3 and 5 streams respectively, if required)Measurement PerformanceResponse time<15 mins. (90 % step change)Typical accuracy<±3 % of reading or ±0.1 ppm (whichever is the greater) over the range 0 ... 10 ppm PO 4<±5 % of reading over the range 10 ... 15 ppm PO 4Repeatability<±1.5 % of reading or ±0.05 ppm (whichever is the greater) over the range 0 ... 10 ppm PO 4<±2.5 % of reading over the range 10 ... 15 ppm PO 4RangeAuto Ranging 0.00 ... 2.99 /3.0 ... 15.0Solution RequirementsNumber 2 reagents 2 standard solution 1 cleaning solution Volume2.5 l max. per reagent 500 ml per standard solution 500 ml cleaning solutionReagent ConsumptionContinuous operation mode 2.5 l max. per 90 daysDisplayColor, passive matrix, liquid crystal display (LCD) with built-in backlight and contrast adjustment 76800 pixel display**A small percentage of the display pixels may be either constantly active or inactive. Max. percentage of inoperative pixels <0.01%.Dedicated operator keys⏹Group Select/Left cursor ⏹View Select/Right cursor ⏹Menu key ⏹Up/Increment key ⏹Down/Decrement key ⏹Enter keyMechanical DataIngress protectionIP31** – Wet section (critical components IP66)IP66 – Transmitter Dimensions Materials of construction Environmental DataAmbient Operating Temperature 5 ... 45 ºC (41 ... 113 ºF)Sample Temperature 5 ... 55 ºC (41 ... 131 ºF)Sample Particulate <60 microns <10 mgl –1Sample Flow Rate>20 mls/min / <500 ml/min Storage Temperature–20 ... 75 ºC (–4 ... 167 ºF)Ambient Operating Humidity Up to 95 % RH non-condensing **Not evaluated for UL or CBDiagonal display area 144 mm (5.7 in.)Height 638 mm (25.1 in.) plus constant headbracket 186 mm (7.3 in.)Width 271 mm (10.7 in.)Depth182 mm (7.2 in.)Weight15 kg (33 lbs)Electronics enclosure 20 % glass loaded polypropylene Main enclosure NorylLower tray 10 % glass loaded polypropylene DoorAcrylicNavigator 600 Phosphate Phosphate analyzerDS/NAV6P–EN Rev. G 7ElectricalSupply ranges110 to 240 V max. AC 50/60 Hz ± 10 % (90 to 264 V AC, 45/65 Hz)18 to 36 V DC (optional)Power consumption 60 W max. – AC 100 W max. – DCAnalog OutputsSingle and multi-stream analyzers6 isolated current outputs, fully assignable and programmable over a 0 ... 20 mA range (up to 22 mA if required)Alarms/Relay OutputsSingle and multi-stream instruments One per unit:⏹Out of service alarm relay ⏹Calibration in progress alarm relay ⏹Calibration failed alarm relay ⏹Maintenance/Hold alarm relaySix per unit:⏹fully user-assignable and alarm relaysRating Wetted MaterialsPMMA (acrylic)PP (polypropylene)PTFEPP (20% glass filled)PEEK NBR (nitrile)EPDM SantoprenePTFE (15% polysulphane)NORYLBorosilicate glass Acrylic adhesiveConnectivity/CommunicationsEthernet connection Bus communicationsProfibus DP V1 (optional)Data Handling, Storage and DisplaySecurityStorageRemovable Secure Digital (SD) card Trend analysis Local and remote Data transfer SD card or FTPApprovals, Certification and SafetySafety Approval cULus – pendingCE MarkCovers EMC & LV Directives (including latest version EN 61010)General Safety EN 61010–1Overvoltage Class 11 on inputs and outputs Pollution category 2EMCEmissions & immunityMeets requirements of IEC61326 for an Industrial EnvironmentVoltage 250 V AC 30 V DC Current5 A AC 5 A DC Loading (non-inductive)1250 VA150 WWeb server with ftp:for real time monitoring, configuration, data file access and email capabilityMulti level security:user, configuration, calibration and maintenance pagesNavigator 600 Phosphate Phosphate analyzer Overall DimensionsNavigator 600 Phosphate8DS/NAV6P–EN Rev. GNavigator 600 PhosphatePhosphate analyzerReagent Bottles Mounted on Optional BracketDS/NAV6P–EN Rev. G9Navigator 600 Phosphate Phosphate analyzer10DS/NAV6P–EN Rev. GOrdering InformationSupplied with analyzer:Reagent and calibration solution containersPhosphate Analyzer AW642/XXXXXXXXRange0 ... 15 ppm 5Number of Streams1 – Measuring 1 stream2 – Measuring 2 streams 4 – Measuring3 or4 streams 6 – Measuring5 or6 streams 1246CommunicationsNoneProfibus DP . V .101EnclosureStandardStandard + reagent shelf 01Power Supply110 ... 240 V AC 50/60 Hz 18 ... 36 V DC 01ReservedBuild 9ManualEnglish French Italian German Spanish 12345CertificationNoneCertificate of calibration cULus – pending012Navigator 600 Phosphate Phosphate analyzerDS/NAV6P–EN Rev. G 11Benefits Summary⏹Lowest cost-of-ownership–up to 90 % lower reagent consumption than competitors' analyzers–labour-saving 5 minute annual maintenance and up to 6 months unattended operation⏹Easy to use–familiar Windows menu system –built-in context-sensitive help⏹Full communications–web- and ftp-enabled for easy data file access,remote viewing and configuration –optional Profibus DP V1.0⏹Fast, Accurate and Reliable–temperature-controlled reaction and measurement section for optimum response–automatic cleaning, calibration and zero deliver high accuracy measurements–extensive electronics, measurement and maintenance diagnostics ensure high availability⏹Field upgradeable–2 to 4 or 6 streams; also from 4 to 6 streams, each user-programmable from 0 to 15 ppm⏹Compact size–638 mm (25.1 in.) H x 271 mm (10.7 in.) W x 182 mm (7.2 in.) D⏹Email facility–automatically email up to 6 recipients when user-selected events occur⏹Grab sample facility–for manual sampling⏹Multiple outputs and relays–6 current outputs, 4 device state and 6 user-programmable relays as standard⏹Archiving facility–SD data card for easy backup and programming⏹Instrument logs–alarm and audit logs for complete, secure recordsContact usD S /N A V 6P –E N R e v . G 10.2011ABB LimitedProcess Automation Oldends Lane StonehouseGloucestershire GL10 3TA UK Tel:+44 1453 826 661Fax:+44 1453 829 671ABB Inc.Process Automation 125 E. County Line Road Warminster PA 18974USA Tel:+1 215 674 6000Fax:+1 215 674 NoteWe reserve the right to make technical changes or modify the contents of this document without prior notice. With regard to purchase orders, the agreed particulars shall prevail. ABB does not accept any responsibility whatsoever for potential errors or possible lack of information in this document.We reserve all rights in this document and in thesubject matter and illustrations contained therein. Any reproduction, disclosure to third parties or utilization of its contents – in whole or in parts – is forbidden without prior written consent of ABB.Copyright© 2011 ABB All rights reserved 3KXA842602R1001Windows™, Microsoft™, MS-DOS™ and Internet Explorer™ are registered trademarks of Microsoft Corporation in the United States and / or other countries.PROFIBUS™ is a registered trademark of PROFIBUS corporation.。
Crop Guide Oat Sampling说明书
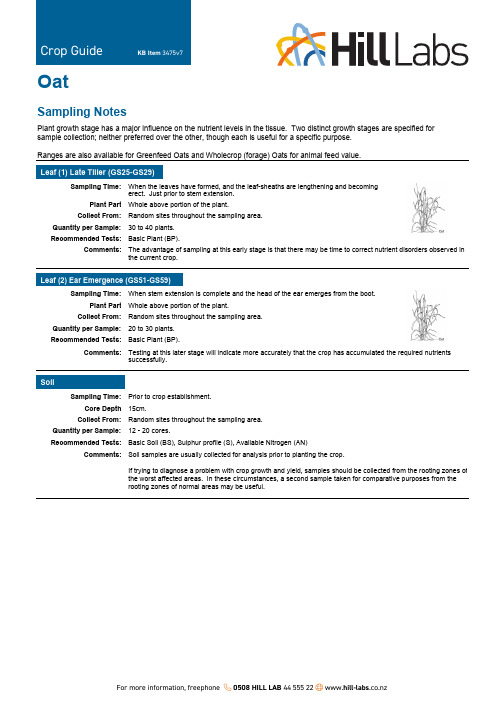
OatSampling NotesPlant growth stage has a major influence on the nutrient levels in the tissue. Two distinct growth stages are specified for sample collection; neither preferred over the other, though each is useful for a specific purpose.Ranges are also available for Greenfeed Oats and Wholecrop (forage) Oats for animal feed value.Crop Guide KB Item3475v7Leaf (1) Late Tiller (GS25-GS29)Sampling Time:Plant PartCollect From: Quantity per Sample: Recommended Tests:Comments:When the leaves have formed, and the leaf-sheaths are lengthening and becomingerect. Just prior to stem extension.Whole above portion of the plant.Random sites throughout the sampling area.30 to 40 plants.Basic Plant (BP).The advantage of sampling at this early stage is that there may be time to correct nutrient disorders observed in the current crop.Leaf (2) Ear Emergence (GS51-GS59)Sampling Time:Plant PartCollect From: Quantity per Sample: Recommended Tests:Comments:When stem extension is complete and the head of the ear emerges from the boot.Whole above portion of the plant.Random sites throughout the sampling area.20 to 30 plants.Basic Plant (BP).Testing at this later stage will indicate more accurately that the crop has accumulated the required nutrients successfully.SoilSampling Time:Core DepthCollect From: Quantity per Sample: Recommended Tests:Comments:Prior to crop establishment.15cm.Random sites throughout the sampling area.12 - 20 cores.Basic Soil (BS), Sulphur profile (S), Available Nitrogen (AN)Soil samples are usually collected for analysis prior to planting the crop.If trying to diagnose a problem with crop growth and yield, samples should be collected from the rooting zones of the worst affected areas. In these circumstances, a second sample taken for comparative purposes from the rooting zones of normal areas may be useful.OAT CROP GUIDECommentsSmall grain production and quality are greatly influenced by fertilisation.Nitrogen has been found to be the most important fertiliser element in New Zealand cereal crops. Significant responses to potassium, sulphur or magnesium have also been recorded.Different cultivars have been found to have some differences in nutrient concentrations; however, these differences arerelatively small, and one set of interpretation criteria can be used.Improper growth stage identification can result in errors in interpretation. Nutrient uptake precedes dry matter accumulation occurring between tillering and head emergence. Consequently, nutrient concentrations generally decline between these stages.Diagnosis of sulphur deficiency can be assisted by using the N:S ratio. A sulphur deficiency may exist when the N:S ratio is greater that 16:1. Severe deficiency is likely when the ratio is greater than 20:1.ReferencesJones Jr, J.B 1967. Soil testing and plant analysis. Part 2. SSSA Special Publication Series, p 49-58.Ward, R.C.; Whitney, D.A. and Westfall, D.G. 1973. Plant analysis as an aid in fertilising small grains. Soil testing and plant analysis.Lockman, R.B. 1969. Agronomy Abstracts, American Society of Agronomy, Wisconson, pg 97.Blackmore, L.C; Searle, P.L and Daly, B.K. 1987. Methods for chemical analysis of soils. NZ Soil Bureau Scientific Report 80. NZ Soil Bureau, DSIR.Reuter, D. J. and Robinson, J. B. (Eds) 1997. Plant analysis. An interpretation manual. Second edition.DisclaimerNormal Range levels shown as histograms in test reports relate specifically to the sampling procedure provided in this crop guide. The Normal Range levels in test reports and Comments provided in this Crop Guide are the most up to date available, but may be altered without notification. Such alterations are implemented immediately in the laboratory histogram reports. It is recommended that a consultant or crop specialist be involved with interpretations and recommendations.。
- 1、下载文档前请自行甄别文档内容的完整性,平台不提供额外的编辑、内容补充、找答案等附加服务。
- 2、"仅部分预览"的文档,不可在线预览部分如存在完整性等问题,可反馈申请退款(可完整预览的文档不适用该条件!)。
- 3、如文档侵犯您的权益,请联系客服反馈,我们会尽快为您处理(人工客服工作时间:9:00-18:30)。
Active Sampling for Feature SelectionSriharsha Veeramachaneni and Paolo AvesaniITC-IRST,Via Sommarive18-Loc.Pant`e,I-38050Povo,Trento,Italysriharsha,avesani@irst.itc.itAbstract.In many knowledge discovery applications the data mining step is fol-lowed by further data acquisition.New data may consist of new instances and/ornew features for the old instances.When new features are to be added an ac-quisition policy can help decide what features have to be acquired based on theirpredictive capability and the cost of acquisition.This can be posed as a feature se-lection problem where the feature values are not known in advance.We propose atechnique to actively sample the feature values with the ultimate goal of choosingbetween alternative candidate features with minimum sampling cost.Our algo-rithm is based on extracting candidate features in a“region”of the instance spacewhere the feature value is likely to alter our knowledge the most.An experimentalevaluation on a standard database shows that it is possible outperform a randomsubsampling policy in terms of the accuracy in feature selection.1IntroductionData mining is mainly concerned with data analysis and the related step of ually it is assumed that the data are given in advance and their quality and size are parameters beyond the learner’s control. The goal of the mining process is the development of an accurate predic-tive model that will aid in future decision making.Sometimes the amount and the quality of data are insufficient to per-form accurate induction.In these cases the data mining task fails.Taking the perspective of knowledge discovery,the mining process can be con-ceived not as a linear sequence of steps but as a never ending loop[15,7, 11].After an analysis step that produces a rough model a further step of data collection can be arranged to obtain a more accurate model.Viewing the mining process as an iteration of data collection and data analysis steps,the objective at each step becomes twofold:a descrip-tive/predictive model and an acquisition policy.A new decision support system has to be developed whose objective is the planning of the data acquisition campaign.The two concerns of a data acquisition plan are1)what instances to focus on and2)what features have to be taken into account.This paper aims to deal with the acquisition policy,restricted to fea-ture extraction(or measurement).This work is motivated by a research project(SMAP)in the domain of agriculture dealing with the Apple Pro-liferation disease in apple trees1.The scenario[3,9,8]is the following: biologists monitor a distributed collection of apple trees affected by the disease;the goal is to characterize the conditions for infection spreading. An archive is arranged with afinite set of records each describing a sin-gle apple tree.The monitored set contains both infected and not infected trees.All the instances are labeled with respect to this boolean classifi-cation.Each summer the archive is updated extending each record with new features.Every year the biologists start by proposing new candidate features that could be extracted(or measured)and at the end of sum-mer a new process of analysis is performed taking into account the past and new data.Since the data collection on thefield can be very expen-sive or time consuming,at the beginning of summer the biologists have to arrange a data acquisition plan by selecting a subset of the candidate features that should be really acquired.Clearly the usual approach in data mining,that regards feature selec-tion as an a posteriori task performed on a database with a large number of features that are fully extracted on the set of instances,is inappropriate in our case.For our problem the feature selection has to be performed in advance.We propose a look-ahead strategy for feature selection.Given a sam-ple of labeled instances,described with respect to a set of features,the problem is to choose between two alternative candidate features and(In general,there are sev-eral candidate features to be ranked in order of relevance).The basic idea is to prescribe a policy that iteratively probes the value of the candi-date features on instances.The challenge is to minimize the cost of feature extraction.If all the candidate features have unit cost,this is equivalent to minimizing the sum of sizes of the subsampleson which the features and are respectively extracted. At the same time the policy should enable an accurate choice of the mostrelevant feature.Notice that when we conduct an exhaustive sampling, i.e.,,both and are measured on all the instances,we fall into the traditional framework of feature selection[1,6].The goal of this work is tofind a trade-off between the assessment of the feature relevance and the cost incurred in the acquisition of the feature values.The optimum solution should select the same features that a fully informed method would select,but with a small subsample of the feature values.It is supposed that after the initial data acquisition to determine fea-ture relevance a full acquisition campaign is performed only for the most promising features,while the remaining features are discarded.There-fore the total cost incurred is the sum of the cost of the acquisition driven by the active feature extraction policy and the cost of full acquisition on the chosen features.For simplicity we assume a simple cost model where all the features have equal and unit cost.After a brief overview of the related literature we sketch an acquisi-tion policy based on the notion of entropy.An empirical evaluation on a standard dataset shows that it is possible to outperform the trivial random subsampling policy.2Related WorkFeature selection is a well studied problem in machine learning[1,6]and in some respects the problem of active acquisition can be considered as a feature selection problem.Nevertheless the common premise of tradi-tional feature selection techniques is the availability of a large amount of known features whose values are available for the entire set of instances. The assessment of a feature relevance is usually performed considering all the values of the given instances.A recent work[4]proposes a feature selection method based on selec-tive sampling.The idea is to reduce the computational cost of the feature selection by reducing the number of sampled data points.Random sam-pling is replaced by selective sampling that exploits the data distribution to detect the most informative examples.The detection of closely related examples is performed using a kd-tree indexing avoiding the necessity of comparing an example against all others.Although this approach saves computational effort during the relevance assessment,to build the kd-tree all the feature values for all the examples need to be known in advance.Usually the output of a feature selection algorithm is a set of rele-vant features while a ranking may be more suitable for decision making support.A recent work that takes this perspective proposes a statistical approach that produces a rank built on a probe:the decision of keeping or discarding a given feature is based on the probability that this feature is ranked higher or lower than the probe[5].Again,even though this approach reduces computational complexity because it does not require the assessment of all the possible rankings,the single step to check the relative order between two features requires the full knowledge of their values.Our work on the active sampling for feature selection is inspired by earlier work on active learning[2,14,10].Active learning is a frame-work where the learner has the freedom to select which data points are added to its training set.The acquisition of new examples is driven by the knowledge gained by the past acquisition steps with the aim of accurately learning the concept using as few labeled examples as possible.We propose an analogous method that selects the next example with the aim of optimizing a target criterion(reduced error rate on feature ranking).In contrast to the conventional active learning paradigm,where the class labels of unlabeled samples are probed to quickly ascertain the best predictor of the class from the features,our problem is to choose from a set of class-labeled samples on which candidate features are to be extracted while still trying to obtain the best predictor for the class.Although the influence of the cost model on a learning process has been studied(see the works on cost-sensitive learning[12,13]),we cur-rently ignore this aspect for our active sampling approach.3Active Sampling of Feature ValuesConsider a set of monitored pattern instances(or subjects). Let the random variable corresponding to the class label be denoted by taking values in.We assume that the class labels for all the instances are known.are discrete valued features that can be extracted on any pattern taking on values in and re-spectively.Assume that feature is extracted for all the subjects and therefore the feature values are known.Therefore the esti-mated probability distribution on is assumed be accurate(the subscript represents the number of samples used in for estima-tion).Initially none of the instances have features extracted. The problem is to rank the candidate features according to relevance for classification of the subjects given the feature minimiz-ing the cost incurred for feature extraction.Although for the following discussion we assume is scalar valued,in general it can be a vector valued feature.Our proposed method assumes that all features are nominal valued and the probability densities in question are multinomial.The feature ex-traction policy considers each candidate feature separately.Let denote the candidate feature whose relevance we are trying to learn.Whereas a random feature extraction scheme(denoted)chooses an instance randomly from all the instances on which is not extracted,our active sampling strategy(denoted)decides on the most‘profitable’subset (or region)of instances for feature extraction.Then the candidate fea-ture is extracted on a randomly chosen instance from.Letis the result of the active policy.The active sampling scheme is an itera-tive process that proposes at each step the class label and the value taken by the previous feature of the samples on which it is most beneficial to extract the candidate feature.We define the set of all pattern instances with as region.At every iteration,the active sampling algorithm chooses the subset for the candidate feature extraction asnot already extracted onThe next region for feature extraction is chosen as follows.The intu-ition behind the algorithm is that the most informative region(informa-tion expressed in terms of entropy)at a given stage is where a sample most alters our current knowledge.At iteration of the active sampling algorithm we have an estimate (based on).The subscript for the probability estimates makes it explicit that the estimates are based onGiven the estimate for the conditional densities we can estimate the entropy in the class given both features which is given by(1)Now for every we compute,where(2)where,wherethe estimator is decoupled for each implying thatfor all where is the region whose bene-fit function is being computed.This fact can be used to show that the following benefit function is equivalent to the one in Equation3.This allows for a more efficient implementation of the active learning algorithm.As mentioned earlier our active learning algorithm considers each candidate feature separately.Therefore the candidate features and are not necessarily extracted on the same subsample of the instances.After the candidate feature is extracted on the specified number()of samples or after the cost budget is exhausted,we can construct a Bayes maximum a posteriori classifier using thefinal estimateof the joint distribution.The features are ranked based upon the error rates of these classifiers.4Experimental evaluationTo compare the active feature sampling strategy and the random feature extraction scheme for feature evaluation we use two performance mea-sures.Thefirst is based on the mean square error between the estimated er-ror rate at a particular sample size and the“true”error rate for a given feature.For us the true error rate represents the estimated error rate of the classifier trained after extracting the candidate feature on all sam-ples in the training set(denoted),i,e,the error rate of the classifier designed using(the estimate of the probability densities from all samples).For each of the schemes,a classifier is constructed after extracting the candidate feature on a given number()of sam-ples(i.e,based upon).Now the error rate of the classifier is evaluated asTherefore at every iteration of the learning scheme we can estimate the error rate of the resulting classifier to obtain a ranking.However,in real-ity we cannot extract the candidate feature for all the samples to estimate the error rates.We can circumvent this problem by obtaining a small random subsample for testing.The quantity E is a measure of the correctness in the estimated error rate for a feature after the given number of samples were extracted.We compute the error rate of the classifier for several runs of the learning scheme for the given sample size to compute the mean square error.For a particular learning scheme and for each feature the mean square error can be plotted against the sample size.The second performance measure which is based on the Spearman rank-order correlation more directly indicates the efficacy of a sampling scheme for feature ranking and therefore for feature selection.The Spear-man rank-order correlation coefficient between two vectors of scores for variables is given by2Feature16before deleting the“stalk-root”featureTo compare the performance of the active and the random learning schemes given previous features we partitioned the21features into seven sets of three.This was done instead of experimenting with all possible combinations for simplicity.Each of these sets wasfixed as the pre-vious feature set and the remaining features were evaluated as candidates.The error rates for the classifier trained on each of previous feature set(i.e,before the candidate feature is extracted on any sample) is given in Table1.Table1.Error rates in%when the classifier is trained on each set of previous features.(4,5,6)(10,11,12)(16,17,18)30.912.824.711.15Discussion of resultsFigure1shows the behaviour of the rank-order correlation coefficient(y-axis)between the vector of error rates estimated after a specific number of samples(x-axis)are extracted and the true error rates.As mentioned earlier,true error rate represents the estimated error rate of the classifier trained on all the samples in the database.The different plots correspond to different sets of previous feature vectors indicated on the top of each subplot.Our active feature extraction strategy converges more quickly to the correct ranking of the features than the random scheme.When the difference is not significant.This can be attributed to the fact that feature5individually leads to a very low error rate and therefore the candidate features have to be extracted on a large number of samples to be confidently ranked.For each set of previous features we plotted the mean square dif-ference between the true error rate and the estimated error rate(y-axis) after the candidate feature is extracted on a specific number of samples (x-axis).Figure2shows the plot for feature where the active policy prof-fered the most advantage over the random scheme.The plots indicate that the active policy can be used to lower the cost for feature evaluation.Fig.1.The plot against sample size of the Spearman rank-order correlation between estimated error rates and the true error rates.For each sample size the rank-order correlation coefficient is averaged over500runs of the experiment.For each set of previous features only the feature forwhich the active policy was most beneficial over the random scheme is shown.Fig.2.The plot of the mean square difference(computed over500runs of the experiment)be-tween estimated error rate and the true error rate.For each set of previous features only the feature for which the active policy is most advantageous(based on the area between the curves)over the random policy is shown.6Conclusions and Future WorkIn this paper we have dealt with the problem of cost-constrained feature selection in a knowledge discovery process.An active strategy that se-lects the instances for feature extraction is proposed to aid in choosing the most relevant features among a set of candidate features.The choice is based upon the absolute change between the current estimate of the entropy in the class before and the predicted entropy after the candidate feature value acquisition.A ranking is produced over the candidate fea-tures based on the estimate of the error rate of a classifier trained on a subsample of the feature values.We provided empirical evidence on a standard dataset for the domi-nance of the active sampling scheme over a random policy for subsample selection for feature evaluation.A deeper analysis should be performed to derive an active policy with a non-trivial feature acquisition cost model.The main purpose of this work was to investigate the possibility of ex-ploiting the active learning approach for feature sampling.The promising results,although restricted to a specific case study,encourage more study taking scalability factors into account.Our current method does not scale to large number of previous features because of the necessity to estimate full class-conditional distributions(we do not assume any feature inde-pendence).Moreover the current design of solution is strongly related to the specific classifier,i.e.the Bayes classifier with class-conditional multinomial feature distributions.A general solution should be indepen-dent of the specific classifier and suitable for both nominal and real val-ued features.References1. A.Blum and ngley.Selection of Relevant Features and Examples in Machine Learning.Artificial Intelligence,97(1-2):245–271,1997.2. D.A.Cohn,L.Atlas,and dner.Improving Generalization with Active Learning.Ma-chine Learning,15(2):201–221,1994.3.G.Hughes.Sampling for Decision Making in Crop Loss Assessment and Pest Management:Introduction.In Symposium on Sampling for Decision Making in Crop Loss Assessment and Pest Management,pages1080–1083,1999.4.H.Liu,H.Motoda,and L.Yu.Feature Selection with Selective Sampling.In InternationalJoint Conference on Machine Learning,pages395–402,2002.5.H.Stoppiglia,G.Dreyfus,R.Dubois,and Y.Oussar.Ranking a Random Feature for Variableand Feature Selection.Journal of Machine Learning Research,3:1399–1414,2003.6.I.Guyon and A.Elisseefi.An Introduction to Variable and Feature Selection.Journal ofMachine Learning Research,3:1157–1182,2003.7. E.Frank I.H.Witten.Data Mining.Morgan Kaufmann Publishers,1999.8.J.P.Nyrop,M.R.Binns,and W.van der Werf.Sampling for IPM Decision Making:WhereShould We Invest Time and Resources.In Symposium on Sampling for Decision Making in Crop Loss Assessment and Pest Management,pages1104–1111,1999.9.L.V.Madden and G.Hughes.Sampling for Plant Disease Incidence.In Symposium on Sam-pling for Decision Making in Crop Loss Assessment and Pest Management,pages1088–1103,1999.10.M.Saar-Tsechansky and F.Provost.Active Sampling for Class Probability Estimation andRanking.In Proc.7th International Joint Conference on Artificial Intelligence,pages911–920,2001.11. A.Susi P.Avesani,E.Olivetti.Feeding data mining.Technical report,ITC-irst,2002.12.P.Domingos.MetaCost:A General Method for Making Classifiers Cost-Sensitive.In Knowl-edge Discovery and Data Mining,pages155–164,1999.13.P.D.Turney.Cost-sensitive Classification:Empirical Evaluation of a Hybrid Genetic De-cision Tree Induction Algorithm.Journal of Artificial Intelligence Research,2:369–409, 1995.14.Nicholas Roy and Andrew McCallum.Toward optimal active learning through samplingestimation of error reduction.In Proc.18th International Conf.on Machine Learning,pages 441–448.Morgan Kaufmann,San Francisco,CA,2001.15.J.Zyt W.Klosgen,J.M.Zytkow,editor.Handbook of Data Mining and Knowledge Discovery.Oxford University Press,2002.。