Sparse Representations in Unions of Bases
英文论文写作中一些可能用到的词汇
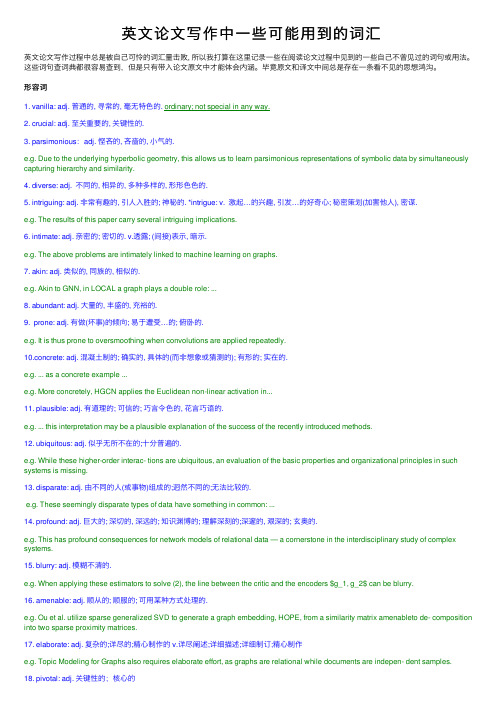
英⽂论⽂写作中⼀些可能⽤到的词汇英⽂论⽂写作过程中总是被⾃⼰可怜的词汇量击败, 所以我打算在这⾥记录⼀些在阅读论⽂过程中见到的⼀些⾃⼰不曾见过的词句或⽤法。
这些词句查词典都很容易查到,但是只有带⼊论⽂原⽂中才能体会内涵。
毕竟原⽂和译⽂中间总是存在⼀条看不见的思想鸿沟。
形容词1. vanilla: adj. 普通的, 寻常的, 毫⽆特⾊的. ordinary; not special in any way.2. crucial: adj. ⾄关重要的, 关键性的.3. parsimonious:adj. 悭吝的, 吝啬的, ⼩⽓的.e.g. Due to the underlying hyperbolic geometry, this allows us to learn parsimonious representations of symbolic data by simultaneously capturing hierarchy and similarity.4. diverse: adj. 不同的, 相异的, 多种多样的, 形形⾊⾊的.5. intriguing: adj. ⾮常有趣的, 引⼈⼊胜的; 神秘的. *intrigue: v. 激起…的兴趣, 引发…的好奇⼼; 秘密策划(加害他⼈), 密谋.e.g. The results of this paper carry several intriguing implications.6. intimate: adj. 亲密的; 密切的. v.透露; (间接)表⽰, 暗⽰.e.g. The above problems are intimately linked to machine learning on graphs.7. akin: adj. 类似的, 同族的, 相似的.e.g. Akin to GNN, in LOCAL a graph plays a double role: ...8. abundant: adj. ⼤量的, 丰盛的, 充裕的.9. prone: adj. 有做(坏事)的倾向; 易于遭受…的; 俯卧的.e.g. It is thus prone to oversmoothing when convolutions are applied repeatedly.10.concrete: adj. 混凝⼟制的; 确实的, 具体的(⽽⾮想象或猜测的); 有形的; 实在的.e.g. ... as a concrete example ...e.g. More concretely, HGCN applies the Euclidean non-linear activation in...11. plausible: adj. 有道理的; 可信的; 巧⾔令⾊的, 花⾔巧语的.e.g. ... this interpretation may be a plausible explanation of the success of the recently introduced methods.12. ubiquitous: adj. 似乎⽆所不在的;⼗分普遍的.e.g. While these higher-order interac- tions are ubiquitous, an evaluation of the basic properties and organizational principles in such systems is missing.13. disparate: adj. 由不同的⼈(或事物)组成的;迥然不同的;⽆法⽐较的.e.g. These seemingly disparate types of data have something in common: ...14. profound: adj. 巨⼤的; 深切的, 深远的; 知识渊博的; 理解深刻的;深邃的, 艰深的; ⽞奥的.e.g. This has profound consequences for network models of relational data — a cornerstone in the interdisciplinary study of complex systems.15. blurry: adj. 模糊不清的.e.g. When applying these estimators to solve (2), the line between the critic and the encoders $g_1, g_2$ can be blurry.16. amenable: adj. 顺从的; 顺服的; 可⽤某种⽅式处理的.e.g. Ou et al. utilize sparse generalized SVD to generate a graph embedding, HOPE, from a similarity matrix amenableto de- composition into two sparse proximity matrices.17. elaborate: adj. 复杂的;详尽的;精⼼制作的 v.详尽阐述;详细描述;详细制订;精⼼制作e.g. Topic Modeling for Graphs also requires elaborate effort, as graphs are relational while documents are indepen- dent samples.18. pivotal: adj. 关键性的;核⼼的e.g. To ensure the stabilities of complex systems is of pivotal significance toward reliable and better service providing.19. eminent: adj. 卓越的,著名的,显赫的;⾮凡的;杰出的e.g. To circumvent those defects, theoretical studies eminently represented by percolation theories appeared.20. indispensable: adj. 不可或缺的;必不可少的 n. 不可缺少的⼈或物e.g. However, little attention is paid to multipartite networks, which are an indispensable part of complex networks.21. post-hoc: adj. 事后的e.g. Post-hoc explainability typically considers the question “Why the GNN predictor made certain prediction?”.22. prevalent: adj. 流⾏的;盛⾏的;普遍存在的e.g. A prevalent solution is building an explainer model to conduct feature attribution23. salient: adj. 最重要的;显著的;突出的. n. 凸⾓;[建]突出部;<军>进攻或防卫阵地的突出部分e.g. It decomposes the prediction into the contributions of the input features, which redistributes the probability of features according to their importance and sample the salient features as an explanatory subgraph.24. rigorous: adj. 严格缜密的;严格的;谨慎的;细致的;彻底的;严厉的e.g. To inspect the OOD effect rigorously, we take a causal look at the evaluation process with a Structural Causal Model.25. substantial: adj. ⼤量的;价值巨⼤的;重⼤的;⼤⽽坚固的;结实的;牢固的. substantially: adv. ⾮常;⼤⼤地;基本上;⼤体上;总的来说26. cogent: adj. 有说服⼒的;令⼈信服的e.g. The explanatory subgraph $G_s$ emphasizes tokens like “weak” and relations like “n’t→funny”, which is cogent according to human knowledge.27. succinct: adj. 简练的;简洁的 succinctly: adv. 简⽽⾔之,简明扼要地28. concrete: adj. 混凝⼟制的;确实的,具体的(⽽⾮想象或猜测的);有形的;实在的 concretely: adv. 具体地;具体;具体的;有形地29. predominant:adj. 主要的;主导的;显著的;明显的;盛⾏的;占优势的动词1. mitigate: v. 减轻, 缓和. (反 enforce)e.g. In this work, we focus on mitigating this problem for a certain class of symbolic data.2. corroborate: v. [VN] [often passive] (formal) 证实, 确证.e.g. This is corroborated by our experiments on real-world graph.3. endeavor: n./v. 努⼒, 尽⼒, 企图, 试图.e.g. It encourages us to continue the endeavor in applying principles mathematics and theory in successful deployment of deep learning.4. augment: v. 增加, 提⾼, 扩⼤. n. 增加, 补充物.e.g. We also augment the graph with geographic information (longitude, latitude and altitude), and GDP of the country where the airport belongs to.5. constitute: v. (被认为或看做)是, 被算作; 组成, 构成; (合法或正式地)成⽴, 设⽴.6. abide: v. 接受, 遵照(规则, 决定, 劝告); 逗留, 停留.e.g. Training a graph classifier entails identifying what constitutes a class, i.e., finding properties shared by graphs in one class but not the other, and then deciding whether new graphs abide to said learned properties.7. entail: v. 牵涉; 需要; 使必要. to involve sth that cannot be avoided.e.g. Due to the recursive definition of the Chebyshev polynomials, the computation of the filter $g_α(\Delta)f$ entails applying the Laplacian $r$ times, resulting cal operator affecting only 1-hop neighbors of a vertex and in $O(rn)$ operations.8. encompass: v. 包含, 包括, 涉及(⼤量事物); 包围, 围绕, 围住.e.g. This model is chosen as it is sufficiently general to encompass several state-of-the-art networks.e.g. The k-cycle detection problem entails determining if G contains a k-cycle.9. reveal: v. 揭⽰, 显⽰, 透露, 显出, 露出, 展⽰.10. bestow: v. 将(…)给予, 授予, 献给.e.g. Aiming to bestow GCNs with theoretical guarantees, one promising research direction is to study graph scattering transforms (GSTs).11. alleviate: v. 减轻, 缓和, 缓解.12. investigate: v. 侦查(某事), 调查(某⼈), 研究, 调查.e.g. The sensitivity of pGST to random and localized noise is also investigated.13. fuse: v. (使)融合, 熔接, 结合; (使)熔化, (使保险丝熔断⽽)停⽌⼯作.e.g. We then fuse the topological embeddings with the initial node features into the initial query representations using a query network$f_q$ implemented as a two-layer feed-forward neural network.14. magnify: v. 放⼤, 扩⼤; 增强; 夸⼤(重要性或严重性); 夸张.e.g. ..., adding more layers also leads to more parameters which magnify the potential of overfitting.15. circumvent: v. 设法回避, 规避; 绕过, 绕⾏.e.g. To circumvent the issue and fulfill both goals simultaneously, we can add a negative term...16. excel: v. 擅长, 善于; 突出; 胜过平时.e.g. Nevertheless, these methods have been repeatedly shown to excel in practice.17. exploit: v. 利⽤(…为⾃⼰谋利); 剥削, 压榨; 运⽤, 利⽤; 发挥.e.g. In time series and high-dimensional modeling, approaches that use next step prediction exploit the local smoothness of the signal.18. regulate: v. (⽤规则条例)约束, 控制, 管理; 调节, 控制(速度、压⼒、温度等).e.g. ... where $b >0$ is a parameter regulating the probability of this event.19. necessitate: v. 使成为必要.e.g. Combinatorial models reproduce many-body interactions, which appear in many systems and necessitate higher-order models that capture information beyond pairwise interactions.20. portray:描绘, 描画, 描写; 将…描写成; 给⼈以某种印象; 表现; 扮演(某⾓⾊).e.g. Considering pairwise interactions, a standard network model would portray the link topology of the underlying system as shown in Fig. 2b.21. warrant: v. 使有必要; 使正当; 使恰当. n. 执⾏令; 授权令; (接受款项、服务等的)凭单, 许可证; (做某事的)正当理由, 依据.e.g. Besides statistical methods that can be used to detect correlations that warrant higher-order models, ... (除了可以⽤来检测⽀持⾼阶模型的相关性的统计⽅法外, ...)22. justify: v. 证明…正确(或正当、有理); 对…作出解释; 为…辩解(或辩护); 调整使全⾏排满; 使每⾏排齐.e.g. ..., they also come with the assumption of transitive, Markovian paths, which is not justified in many real systems.23. hinder:v. 阻碍; 妨碍; 阻挡. (反 foster: v. 促进; 助长; 培养; ⿎励; 代养, 抚育, 照料(他⼈⼦⼥⼀段时间))e.g. The eigenvalues and eigenvectors of these matrix operators capture how the topology of a system influences the efficiency of diffusion and propagation processes, whether it enforces or mitigates the stability of dynamical systems, or if it hinders or fosters collective dynamics.24. instantiate:v. 例⽰;⽤具体例⼦说明.e.g. To learn the representation we instantiate (2) and split each input MNIST image into two parts ...25. favor:v. 赞同;喜爱, 偏爱; 有利于, 便于. n. 喜爱, 宠爱, 好感, 赞同; 偏袒, 偏爱; 善⾏, 恩惠.26. attenuate: v. 使减弱; 使降低效⼒.e.g. It therefore seems that the bounds we consider favor hard-to-invert encoders, which heavily attenuate part of the noise, over well conditioned encoders.27. elucidate:v. 阐明; 解释; 说明.e.g. Secondly, it elucidates the importance of appropriately choosing the negative samples, which is indeed a critical component in deep metric learning based on triplet losses.28. violate: v. 违反, 违犯, 违背(法律、协议等); 侵犯(隐私等); 使⼈不得安宁; 搅扰; 亵渎, 污损(神圣之地).e.g. Negative samples are obtained by patches from different images as well as patches from the same image, violating the independence assumption.29. compel:v. 强迫, 迫使; 使必须; 引起(反应).30. gauge: v. 判定, 判断(尤指⼈的感情或态度); (⽤仪器)测量, 估计, 估算. n. 测量仪器(或仪表);计量器;宽度;厚度;(枪管的)⼝径e.g. Yet this hyperparameter-tuned approach raises a cubic worst-case space complexity and compels the user to traverse several feature sets and gauge the one that attains the best performance in the downstream task.31. depict: v. 描绘, 描画; 描写, 描述; 刻画.e.g. As they depict different aspects of a node, it would take elaborate designs of graph convolutions such that each set of features would act as a complement to the other.32. sketch: n. 素描;速写;草图;幽默短剧;⼩品;简报;概述 v. 画素描;画速写;概述;简述e.g. Next we sketch how to apply these insights to learning topic models.33. underscore:v. 在…下⾯划线;强调;着重说明 n.下划线e.g. Moreover, the walk-topic distributions generated by Graph Anchor LDA are indeed sharper than those by ordinary LDA, underscoring the need for selecting anchors.34. disclose: v. 揭露;透露;泄露;使显露;使暴露e.g. Another drawback lies in their unexplainable nature, i.e., they cannot disclose the sciences beneath network dynamics.35. coincide: v. 同时发⽣;相同;相符;极为类似;相接;相交;同位;位置重合;重叠e.g. The simulation results coincide quite well with the theoretical results.36. inspect: v. 检查;查看;审视;视察 to look closely at sth/sb, especially to check that everything is as it should be名词1. capacity: n. 容量, 容积, 容纳能⼒; 领悟(或理解、办事)能⼒; 职位, 职责.e.g. This paper studies theoretically the computational capacity limits of graph neural networks (GNN) falling within the message-passing framework of Gilmer et al. (2017).2. implication: n. 可能的影响(或作⽤、结果); 含意, 暗指; (被)牵连, 牵涉.e.g. Section 4 analyses the implications of restricting the depth $d$ and width $w$ of GNN that do not use a readout function.3. trade-off:(在需要⽽⼜相互对⽴的两者间的)权衡, 协调.e.g. This reveals a direct trade-off between the depth and width of a graph neural network.4. cornerstone:n. 基⽯; 最重要部分; 基础; 柱⽯.5. umbrella: n. 伞; 综合体; 总体, 整体; 保护, 庇护(体系).e.g. Community detection is an umbrella term for a large number of algorithms that group nodes into distinct modules to simplify and highlight essential structures in the network topology.6. folklore:n. 民间传统, 民俗; 民间传说.e.g. It is folklore knowledge that maximizing MI does not necessarily lead to useful representations.7. impediment:n. 妨碍,阻碍,障碍; ⼝吃.e.g. While a recent approach overcomes this impediment, it results in poor quality in prediction tasks due to its linear nature.8. obstacle:n. 障碍;阻碍; 绊脚⽯; 障碍物; 障碍栅栏.e.g. However, several major obstacles stand in our path towards leveraging topic modeling of structural patterns to enhance GCNs.9. vicinity:n. 周围地区; 邻近地区; 附近.e.g. The traits with which they engage are those that are performed in their vicinity.10. demerit: n. 过失,缺点,短处; (学校给学⽣记的)过失分e.g. However, their principal demerit is that their implementations are time-consuming when the studied network is large in size. Another介/副/连词1. notwithstanding:prep. 虽然;尽管 adv. 尽管如此.e.g. Notwithstanding this fundamental problem, the negative sampling strategy is often treated as a design choice.2. albeit: conj. 尽管;虽然e.g. Such methods rely on an implicit, albeit rigid, notion of node neighborhood; yet this one-size-fits-all approach cannot grapple with the diversity of real-world networks and applications.3. Hitherto:adv. 迄今;直到某时e.g. Hitherto, tremendous endeavors have been made by researchers to gauge the robustness of complex networks in face of perturbations.短语1.in a nutshell: 概括地说, 简⾔之, ⼀⾔以蔽之.e.g. In a nutshell, GNN are shown to be universal if four strong conditions are met: ...2. counter-intuitively: 反直觉地.3. on-the-fly:动态的(地), 运⾏中的(地).4. shed light on/into:揭⽰, 揭露; 阐明; 解释; 将…弄明⽩; 照亮.e.g. These contemporary works shed light into the stability and generalization capabilities of GCNs.e.g. Discovering roles and communities in networks can shed light on numerous graph mining tasks such as ...5. boil down to: 重点是; 将…归结为.e.g. These aforementioned works usually boil down to a general classification task, where the model is learnt on a training set and selected by checking a validation set.6. for the sake of:为了.e.g. The local structures anchored around each node as well as the attributes of nodes therein are jointly encoded with graph convolution for the sake of high-level feature extraction.7. dates back to:追溯到.e.g. The usual problem setup dates back at least to Becker and Hinton (1992).8. carry out:实施, 执⾏, 实⾏.e.g. We carry out extensive ablation studies and sensi- tivity analysis to show the effectiveness of the proposed functional time encoding and TGAT-layer.9. lay beyond the reach of:...能⼒达不到e.g. They provide us with information on higher-order dependencies between the components of a system, which lay beyond the reach of models that exclusively capture pairwise links.10. account for: ( 数量或⽐例上)占; 导致, 解释(某种事实或情况); 解释, 说明(某事); (某⼈)对(⾏动、政策等)负有责任; 将(钱款)列⼊(预算).e.g. Multilayer models account for the fact that many real complex systems exhibit multiple types of interactions.11. along with: 除某物以外; 随同…⼀起, 跟…⼀起.e.g. Along with giving us the ability to reason about topological features including community structures or node centralities, network science enables us to understand how the topology of a system influences dynamical processes, and thus its function.12. dates back to:可追溯到.e.g. The usual problem setup dates back at least to Becker and Hinton (1992) and can conceptually be described as follows: ...13. to this end:为此⽬的;为此计;为了达到这个⽬标.e.g. To this end, we consider a simple setup of learning a representation of the top half of MNIST handwritten digit images.14. Unless stated otherwise:除⾮另有说明.e.g. Unless stated otherwise, we use a bilinear critic $f(x, y) = x^TWy$, set the batch size to $128$ and the learning rate to $10^{−4}$.15. As a reference point:作为参照.e.g. As a reference point, the linear classification accuracy from pixels drops to about 84% due to the added noise.16. through the lens of:透过镜头. (以...视⾓)e.g. There are (at least) two immediate benefits of viewing recent representation learning methods based on MI estimators through the lens of metric learning.17. in accordance with:符合;依照;和…⼀致.e.g. The metric learning view seems hence in better accordance with the observations from Section 3.2 than the MI view.It can be shown that the anchors selected by our Graph Anchor LDA are not only indicative of “topics” but are also in accordance with the actual graph structures.18. be akin to:近似, 类似, 类似于.e.g. Thus, our learning model is akin to complex contagion dynamics.19. to name a few:仅举⼏例;举⼏个来说.e.g. Multitasking, multidisciplinary work and multi-authored works, to name a few, are ingrained in the fabric of science culture and certainly multi-multi is expected in order to succeed and move up the scientific ranks.20. a handful of:⼀把;⼀⼩撮;少数e.g. A handful of empirical work has investigated the robustness of complex networks at the community level.21. wreak havoc: 破坏;肆虐;严重破坏;造成破坏;浩劫e.g. Failures on one network could elicit failures on its coupled networks, i.e., networks with which the focal network interacts, and eventually those failures would wreak havoc on the entire network.22. apart from: 除了e.g. We further posit that apart from node $a$ node $b$ has $k$ neighboring nodes.。
On Recovery of Sparse Signals Via Minimization
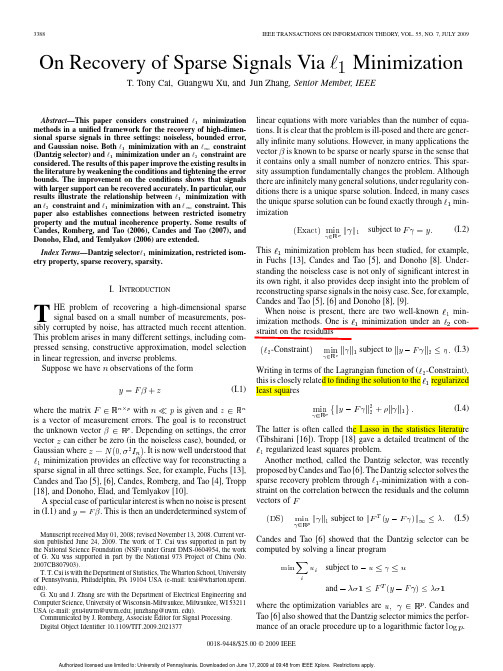
3388IEEE TRANSACTIONS ON INFORMATION THEORY, VOL. 55, NO. 7, JULY 2009On Recovery of Sparse Signals Via `1 MinimizationT. Tony Cai, Guangwu Xu, and Jun Zhang, Senior Member, IEEEAbstract—This paper considers constrained `1 minimization methods in a unified framework for the recovery of high-dimensional sparse signals in three settings: noiseless, bounded error, and Gaussian noise. Both `1 minimization with an ` constraint (Dantzig selector) and `1 minimization under an `2 constraint are considered. The results of this paper improve the existing results in the literature by weakening the conditions and tightening the error bounds. The improvement on the conditions shows that signals with larger support can be recovered accurately. In particular, our results illustrate the relationship between `1 minimization with an `2 constraint and `1 minimization with an ` constraint. This paper also establishes connections between restricted isometry property and the mutual incoherence property. Some results of Candes, Romberg, and Tao (2006), Candes and Tao (2007), and Donoho, Elad, and Temlyakov (2006) are extended.11linear equations with more variables than the number of equations. It is clear that the problem is ill-posed and there are generally infinite many solutions. However, in many applications the vector is known to be sparse or nearly sparse in the sense that it contains only a small number of nonzero entries. This sparsity assumption fundamentally changes the problem. Although there are infinitely many general solutions, under regularity conditions there is a unique sparse solution. Indeed, in many cases the unique sparse solution can be found exactly through minimization subject to (I.2)Index Terms—Dantzig selector`1 minimization, restricted isometry property, sparse recovery, sparsity.I. INTRODUCTION HE problem of recovering a high-dimensional sparse signal based on a small number of measurements, possibly corrupted by noise, has attracted much recent attention. This problem arises in many different settings, including compressed sensing, constructive approximation, model selection in linear regression, and inverse problems. Suppose we have observations of the formTThis minimization problem has been studied, for example, in Fuchs [13], Candes and Tao [5], and Donoho [8]. Understanding the noiseless case is not only of significant interest in its own right, it also provides deep insight into the problem of reconstructing sparse signals in the noisy case. See, for example, Candes and Tao [5], [6] and Donoho [8], [9]. minWhen noise is present, there are two well-known imization methods. One is minimization under an constraint on the residuals -Constraint subject to (I.3)(I.1) with is given and where the matrix is a vector of measurement errors. The goal is to reconstruct the unknown vector . Depending on settings, the error vector can either be zero (in the noiseless case), bounded, or . It is now well understood that Gaussian where minimization provides an effective way for reconstructing a sparse signal in all three settings. See, for example, Fuchs [13], Candes and Tao [5], [6], Candes, Romberg, and Tao [4], Tropp [18], and Donoho, Elad, and Temlyakov [10]. A special case of particular interest is when no noise is present . This is then an underdetermined system of in (I.1) andManuscript received May 01, 2008; revised November 13, 2008. Current version published June 24, 2009. The work of T. Cai was supported in part by the National Science Foundation (NSF) under Grant DMS-0604954, the work of G. Xu was supported in part by the National 973 Project of China (No. 2007CB807903). T. T. Cai is with the Department of Statistics, The Wharton School, University of Pennsylvania, Philadelphia, PA 19104 USA (e-mail: tcai@wharton.upenn. edu). G. Xu and J. Zhang are with the Department of Electrical Engineering and Computer Science, University of Wisconsin-Milwaukee, Milwaukee, WI 53211 USA (e-mail: gxu4uwm@; junzhang@uwm. edu). Communicated by J. Romberg, Associate Editor for Signal Processing. Digital Object Identifier 10.1109/TIT.2009.2021377Writing in terms of the Lagrangian function of ( -Constraint), this is closely related to finding the solution to the regularized least squares (I.4) The latter is often called the Lasso in the statistics literature (Tibshirani [16]). Tropp [18] gave a detailed treatment of the regularized least squares problem. Another method, called the Dantzig selector, was recently proposed by Candes and Tao [6]. The Dantzig selector solves the sparse recovery problem through -minimization with a constraint on the correlation between the residuals and the column vectors of subject to (I.5)Candes and Tao [6] showed that the Dantzig selector can be computed by solving a linear program subject to and where the optimization variables are . Candes and Tao [6] also showed that the Dantzig selector mimics the perfor. mance of an oracle procedure up to a logarithmic factor0018-9448/$25.00 © 2009 IEEEAuthorized licensed use limited to: University of Pennsylvania. Downloaded on June 17, 2009 at 09:48 from IEEE Xplore. Restrictions apply.CAI et al.: ON RECOVERY OF SPARSE SIGNALS VIAMINIMIZATION3389It is clear that some regularity conditions are needed in order for these problems to be well behaved. Over the last few years, many interesting results for recovering sparse signals have been obtained in the framework of the Restricted Isometry Property (RIP). In their seminal work [5], [6], Candes and Tao considered sparse recovery problems in the RIP framework. They provided beautiful solutions to the problem under some conditions on the so-called restricted isometry constant and restricted orthogonality constant (defined in Section II). These conditions essentially require that every set of columns of with certain cardinality approximately behaves like an orthonormal system. Several different conditions have been imposed in various settings. For example, the condition was used in Candes and in Candes, Romberg, and Tao [5], Tao [4], in Candes and Tao [6], and in Candes [3], where is the sparsity index. A natural question is: Can these conditions be weakened in a unified way? Another widely used condition for sparse recovery is the Mutual Incoherence Property (MIP) which requires the to be pairwise correlations among the column vectors of small. See [10], [11], [13], [14], [18]. minimization methods in a In this paper, we consider single unified framework for sparse recovery in three cases, minnoiseless, bounded error, and Gaussian noise. Both constraint (DS) and minimization imization with an constraint ( -Constraint) are considered. Our under the results improve on the existing results in [3]–[6] by weakening the conditions and tightening the error bounds. In particular, miniour results clearly illustrate the relationship between mization with an constraint and minimization with an constraint (the Dantzig selector). In addition, we also establish connections between the concepts of RIP and MIP. As an application, we present an improvement to a recent result of Donoho, Elad, and Temlyakov [10]. In all cases, we solve the problems under the weaker condition (I.6) The improvement on the condition shows that for fixed and , signals with larger support can be recovered. Although our main interest is on recovering sparse signals, we state the results in the general setting of reconstructing an arbitrary signal. It is sometimes convenient to impose conditions that involve only the restricted isometry constant . Efforts have been made in this direction in the literature. In [7], the recovery result was . In [3], the weaker established under the condition was used. Similar conditions have condition also been used in the construction of (random) compressed and sensing matrices. For example, conditions were used in [15] and [1], respectively. We shall remark that, our results implies that the weaker conditionsparse recovery problem. We begin the analysis of minimization methods for sparse recovery by considering the exact recovery in the noiseless case in Section III. Our result improves the main result in Candes and Tao [5] by using weaker conditions and providing tighter error bounds. The analysis of the noiseless case provides insight to the case when the observations are contaminated by noise. We then consider the case of bounded error in Section IV. The connections between the RIP and MIP are also explored. Sparse recovery with Gaussian noise is treated in Section V. Appendices A–D contain the proofs of technical results. II. PRELIMINARIES In this section, we first introduce basic notation and definitions, and then present a technical inequality which will be used in proving our main results. . Let be a vector. The Let support of is the subset of defined byFor an integer , a vector is said to be -sparse if . For a given vector we shall denote by the vector with all but the -largest entries (in absolute value) set to zero and define , the vector with the -largest entries (in absolute value) set to zero. We shall use to denote the -norm of the vector . the standard notation Let the matrix and , the -restricted is defined to be the smallest constant isometry constant such that (II.1) for every -sparse vector . If , we can define another quantity, the -restricted orthogonality constant , as the smallest number that satisfies (II.2) for all and such that and are -sparse and -sparse, respectively, and have disjoint supports. Roughly speaking, the and restricted orthogonality constant isometry constant measure how close subsets of cardinality of columns of are to an orthonormal system. and For notational simplicity we shall write for for hereafter. It is easy to see that and are monotone. That is if if Candes and Tao [5] showed that the constants related by the following inequalities and (II.3) (II.4) are (II.5)suffices in sparse signal reconstruction. The paper is organized as follows. In Section II, after basic notation and definitions are reviewed, we introduce an elementary inequality, which allow us to make finer analysis of theAs mentioned in the Introduction, different conditions on and have been used in the literature. It is not always immediately transparent which condition is stronger and which is weaker. We shall present another important property on andAuthorized licensed use limited to: University of Pennsylvania. Downloaded on June 17, 2009 at 09:48 from IEEE Xplore. Restrictions apply.3390IEEE TRANSACTIONS ON INFORMATION THEORY, VOL. 55, NO. 7, JULY 2009which can be used to compare the conditions. In addition, it is especially useful in producing simplified recovery conditions. Proposition 2.1: If , then (II.6)Theorem 3.1 (Candes and Tao [5]): Let satisfies. Suppose (III.1)Let be a -sparse vector and minimizer to the problem. Then .is the uniqueIn particular,.A proof of the proposition is provided in Appendix A. Remark: Candes and Tao [6] imposes in a more recent paper Candes [3] uses consequence of Proposition 2.1 is that a strictly stronger condition than sition 2.1 yields implies that and . A direct is in fact since Propowhich means .As mentioned in the Introduction, other conditions on and have also been used in the literature. Candes, Romberg, and . Candes and Tao Tao [4] uses the condition [6] considers the Gaussian noise case. A special case with noise of Theorem 1.1 in that paper improves Theorem 3.1 level to by weakening the condition from . Candes [3] imposes the condition . We shall show below that these conditions can be uniformly improved by a transparent argument. A direct application of Proposition 2.2 yields the following result which weakens the above conditions toWe now introduce a useful elementary inequality. This inequality allows us to perform finer estimation on , norms. It will be used in proving our main results. Proposition 2.2: Let be a positive integer. Then any descending chain of real numbersNote that it follows from (II.3) and (II.4) that , and . So the condition is weaker . It is also easy to see from (II.5) and than is also weaker than (II.6) that the condition and the other conditions mentioned above. Theorem 3.2: Let . Suppose satisfiessatisfiesand obeys The proof of Proposition 2.2 is given in Appendix B. where III. SIGNAL RECOVERY IN THE NOISELESS CASE As mentioned in the Introduction, we shall give a unified minimization with an contreatment for the methods of straint and minimization with an constraint for recovery of sparse signals in three cases: noiseless, bounded error, and Gaussian noise. We begin in this section by considering the simplest setting: exact recovery of sparse signals when no noise is present. This is an interesting problem by itself and has been considered in a number of papers. See, for example, Fuchs [13], Donoho [8], and Candes and Tao [5]. More importantly, the solutions to this “clean” problem shed light on the noisy case. Our result improves the main result given in Candes and Tao [5]. The improvement is obtained by using the technical inequalities we developed in previous section. Although the focus is on recovering sparse signals, our results are stated in the general setting of reconstructing an arbitrary signal. with and suppose we are given and Let where for some unknown vector . The goal is to recover exactly when it is sparse. Candes and Tao [5] showed that a sparse solution can be obtained by minimization which is then solved via linear programming.. Then the minimizerto the problem., i.e., the In particular, if is a -sparse vector, then minimization recovers exactly. This theorem improves the results in [5], [6]. The improvement on the condition shows that for fixed and , signals with larger support can be recovered accurately. Remark: It is sometimes more convenient to use conditions only involving the restricted isometry constant . Note that the condition (III.2) implies . This is due to the factby Proposition 2.1. Hence, Theorem 3.2 holds under the condican also be used. tion (III.2). The condition Proof of Theorem 3.2: The proof relies on Proposition 2.2 and makes use of the ideas from [4]–[6]. In this proof, we shall also identify a vector as a function by assigning .Authorized licensed use limited to: University of Pennsylvania. Downloaded on June 17, 2009 at 09:48 from IEEE Xplore. Restrictions apply.CAI et al.: ON RECOVERY OF SPARSE SIGNALS VIAMINIMIZATION3391Let Letbe a solution to the and letminimization problem (Exact). be the support of . WriteClaim:such that. Let In fact, from Proposition 2.2 and the fact that , we have(III.4)For a subset characteristic function of, we use , i.e., if if .to denote theFor each , let . Then is decomposed to . Note that ’s are pairwise disjoint, , and for . We first consider the case where is divisible by . For each , we divide into two halves in the following manner: with where is the first half of , i.e., andIt then follows from Proposition 2.2 thatProposition 2.2 also yieldsand We shall treat four equal parts. as a sum of four functions and divide withintofor any and. ThereforeWe then define that Note thatfor .by. It is clear(III.3)In fact, since, we haveSince, this yieldsIn the rest of our proof we write . Note that . So we get the equation at the top of the following page. This yieldsThe following claim follows from our Proposition 2.2.Authorized licensed use limited to: University of Pennsylvania. Downloaded on June 17, 2009 at 09:48 from IEEE Xplore. Restrictions apply.3392IEEE TRANSACTIONS ON INFORMATION THEORY, VOL. 55, NO. 7, JULY 2009It then follows from (III.4) thatIV. RECOVERY OF SPARSE SIGNALS IN BOUNDED ERROR We now turn to the case of bounded error. The results obtained in this setting have direct implication for the case of Gaussian noise which will be discussed in Section V. and let Letwhere the noise is bounded, i.e., for some bounded set . In this case the noise can either be stochastic or deterministic. The minimization approach is to estimate by the minimizer of subject to We now turn to the case that is not divisible by . Let . Note that in this case and are understood as and , respectively. So the proof for the previous case works if we set and for and We specifically consider two cases: and . The first case is closely connected to the Dantzig selector in the Gaussian noise setting which will be discussed in more detail in Section V. Our results improve the results in Candes, Romberg, and Tao [4], Candes and Tao [6], and Donoho, Elad, and Temlyakov [10]. We shall first consider where satisfies shallLet be the solution to the (DS) problem given in (I.1). The Dantzig selector has the following property. Theorem 4.1: Suppose satisfying . If and with (IV.1) then the solution In this case, we need to use the following inequality whose proof is essentially the same as Proposition 2.2: For any descending chain of real numbers , we have to (DS) obeys (IV.2) with In particular, if . and is a -sparse vector, then .andRemark: Theorem 4.1 is comparable to Theorem 1.1 of Candes and Tao [6], but the result here is a deterministic one instead of a probabilistic one as bounded errors are considered. The proof in Candes and Tao [6] can be adapted to yield aAuthorized licensed use limited to: University of Pennsylvania. Downloaded on June 17, 2009 at 09:48 from IEEE Xplore. Restrictions apply.CAI et al.: ON RECOVERY OF SPARSE SIGNALS VIAMINIMIZATION3393similar result for bounded errors under the stronger condition . Proof of Theorem 4.1: We shall use the same notation as in the proof of Theorem 3.2. Since , letting and following essentially the same steps as in the first part of the proof of Theorem 3.2, we getwhere the noise satisfies . Once again, this problem can be solved through constrained minimization subject to (IV.3)An alternative to the constrained minimization approach is the so-called Lasso given in (I.4). The Lasso recovers a sparse regularized least squares. It is closely connected signal via minimization. The Lasso is a popular to the -constrained method in statistics literature (Tibshirani [16]). See Tropp [18] regularized least squares for a detailed treatment of the problem. By using a similar argument, we have the following result on the solution of the minimization (IV.3). Theorem 4.2: Let vector and with . Suppose . If is a -sparseIf that, then and for every , and we have. The latter forces . Otherwise(IV.4) then for any To finish the proof, we observe the following. 1) . be the submatrix obIn fact, let tained by extracting the columns of according to the in, as in [6]. Then dices in , the minimizer to the problem (IV.3) obeys (IV.5) with .imProof of Theorem 4.2: Notice that the condition , so we can use the first part of the proof plies that of Theorem 3.2. The notation used here is the same as that in the proof of Theorem 3.2. First, we haveand2) In factNote thatSoWe get the result by combining 1) and 2). This completes the proof. We now turn to the second case where the noise is bounded with . The problem is to in -norm. Let from recover the sparse signalRemark: Candes, Romberg, and Tao [4] showed that, if , then(The was set to be This impliesin [4].) Now suppose which yields. ,Authorized licensed use limited to: University of Pennsylvania. Downloaded on June 17, 2009 at 09:48 from IEEE Xplore. Restrictions apply.3394IEEE TRANSACTIONS ON INFORMATION THEORY, VOL. 55, NO. 7, JULY 2009since and Theorem 4.2 that, with. It then follows fromProof of Theorem 4.3: It follows from Proposition 4.1 thatfor all -sparse vector where . Therefore, Theorem 4.2 improves the above result in Candes, Romberg, and Tao [4] by enlarging the support of by 60%. Remark: Similar to Theorems 3.2 and 4.1, we can have the estimation without assuming that is -sparse. In the general case, we haveSince Theorem 4.2, the conditionholds. ByA. Connections Between RIP and MIP In addition to the restricted isometry property (RIP), another commonly used condition in the sparse recovery literature is the mutual incoherence property (MIP). The mutual incoherence property of requires that the coherence bound (IV.6) be small, where are the columns of ( ’s are also assumed to be of length in -norm). Many interesting results on sparse recovery have been obtained by imposing conand the sparsity , see [10], ditions on the coherence bound [11], [13], [14], [18]. For example, a recent paper, Donoho, is a -sparse Elad, and Temlyakov [10] proved that if with , then for any , the vector and minimizer to the problem ( -Constraint) satisfiesRemarks: In this theorem, the result of Donoho, Elad, and Temlyakov [10] is improved in the following ways. to 1) The sparsity is relaxed from . So roughly speaking, Theorem 4.3 improves the result in Donoho, Elad, and Temlyakov [10] by enlarging the support of by 47%. is usually very 2) It is clear that larger is preferred. Since small, the bound is tightened from to , as is close to .V. RECOVERY OF SPARSE SIGNALS IN GAUSSIAN NOISE We now turn to the case where the noise is Gaussian. Suppose we observe (V.1) and wish to recover from and . We assume that is known and that the columns of are standardized to have unit norm. This is a case of significant interest, in particular in statistics. Many methods, including the Lasso (Tibshirani [16]), LARS (Efron, Hastie, Johnstone, and Tibshirani [12]) and Dantzig selector (Candes and Tao [6]), have been introduced and studied. The following results show that, with large probability, the Gaussian noise belongs to bounded sets. Lemma 5.1: The Gaussian error satisfies (V.2) and (V.3) Inequality (V.2) follows from standard probability calculations and inequality (V.3) is proved in Appendix D. Lemma 5.1 suggests that one can apply the results obtained in the previous section for the bounded error case to solve the Gaussian noise problem. Candes and Tao [6] introduced the Dantzig selector for sparse recovery in the Gaussian noise setting. Given the observations in (V.1), the Dantzig selector is the minimizer of subject to where . (V.4)with, provided.We shall now establish some connections between the RIP and MIP and show that the result of Donoho, Elad, and Temlyakov [10] can be improved under the RIP framework, by using Theorem 4.2. The following is a simple result that gives RIP constants from MIP. The proof can be found in Appendix C. It is remarked that the first inequality in the next proposition can be found in [17]. Proposition 4.1: Let be the coherence bound for and Now we are able to show the following result. Theorem 4.3: Suppose with satisfying (or, equivalently, the minimizer is a -sparse vector and . Let . If ), then for any . Then (IV.7),to the problem ( -Constraint) obeys (IV.8)with.Authorized licensed use limited to: University of Pennsylvania. Downloaded on June 17, 2009 at 09:48 from IEEE Xplore. Restrictions apply.CAI et al.: ON RECOVERY OF SPARSE SIGNALS VIAMINIMIZATION3395In the classical linear regression problem when , the least squares estimator is the solution to the normal equation (V.5) in the convex program The constraint (DS) can thus be viewed as a relaxation of the normal (V.3). And similar to the noiseless case, minimization leads to the “sparsest” solution over the space of all feasible solutions. Candes and Tao [6] showed the following result. Theorem 5.1 (Candes and Tao [6]): Suppose -sparse vector. Let be such that Choose the Dantzig selector is asince . The improvement on the error bound is minor. The improvement on the condition is more significant as it shows signals with larger support can be recovered accurately for fixed and . Remark: Similar to the results obtained in the previous sections, if is not necessarily -sparse, in general we have, with probabilitywhere probabilityand, and within (I.1). Then with large probability, obeys (V.6) where and .with.1As mentioned earlier, the Lasso is another commonly used method in statistics. The Lasso solves the regularized least squares problem (I.4) and is closely related to the -constrained minimization problem ( -Constraint). In the Gaussian error be the mincase, we shall consider a particular setting. Let imizer of subject to (V.7)Remark: Candes and Tao [6] also proved an Oracle Inequality in the Gaussian noise setting under for the Dantzig selector . With some additional work, our the condition approach can be used to improve [6, Theorems 1.2 and 1.3] by . weakening the condition to APPENDIX A PROOF OF PROPOSITION 2.1 Let be -sparse and be supports are disjoint. Decompose such that is -sparse. Suppose their aswhere . Combining our results from the last section together with Lemma 5.1, we have the following results on the Dantzig seand the estimator obtained from minimizalector tion under the constraint. Again, these results improve the previous results in the literature by weakening the conditions and providing more precise bounds. Theorem 5.2: Suppose matrix satisfies Then with probability obeys (V.8) with obeys (V.9) with . , and with probability at least , is a -sparse vector and the-sparse for and for . Using the Cauchy–Schwartz inequality, we have, the Dantzig selectorThis yields we also have. Since . APPENDIX B PROOF OF PROPOSITION 2.2,Remark: In comparison to Theorem 5.1, our result in Theto orem 5.2 weakens the condition from and improves the constant in the bound from to . Note thatCandes and Tao [6], the constant C was stated as C appears that there was a typo and the constant C should be C .1InLet)= . It = 4=(1 0 0Authorized licensed use limited to: University of Pennsylvania. Downloaded on June 17, 2009 at 09:48 from IEEE Xplore. Restrictions apply.3396IEEE TRANSACTIONS ON INFORMATION THEORY, VOL. 55, NO. 7, JULY 2009where eachis given (and bounded) byThereforeand the inequality is proved. Without loss of generality, we assume that is even. Write APPENDIX C PROOF OF PROPOSITION 4.1 Let be a -sparse vector. Without loss of generality, we as. A direct calculation shows sume that thatwhereandNow let us bound the second term. Note thatNowThese give usand henceFor the second inequality, we notice that follows from Proposition 2.1 that. It thenand APPENDIX D PROOF OF LEMMA 5.1 The first inequality is standard. For completeness, we give . Then each a short proof here. Let . Hence marginally has Gaussian distributionAuthorized licensed use limited to: University of Pennsylvania. Downloaded on June 17, 2009 at 09:48 from IEEE Xplore. Restrictions apply.CAI et al.: ON RECOVERY OF SPARSE SIGNALS VIAMINIMIZATION3397where the last step follows from the Gaussian tail probability bound that for a standard Gaussian variable and any constant , . is We now prove inequality (V.3). Note that a random variable. It follows from Lemma 4 in Cai [2] that for anyHencewhere. It now follows from the fact that[6] E. J. Candes and T. Tao, “The Dantzig selector: Statistical estimation when p is much larger than n (with discussion),” Ann. Statist., vol. 35, pp. 2313–2351, 2007. [7] A. Cohen, W. Dahmen, and R. Devore, “Compressed Sensing and Best k -Term Approximation” 2006, preprint. [8] D. L. Donoho, “For most large underdetermined systems of linear equations the minimal ` -norm solution is also the sparsest solution,” Commun. Pure Appl. Math., vol. 59, pp. 797–829, 2006. [9] D. L. Donoho, “For most large underdetermined systems of equations, the minimal ` -norm near-solution approximates the sparsest near-solution,” Commun. Pure Appl. Math., vol. 59, pp. 907–934, 2006. [10] D. L. Donoho, M. Elad, and V. N. Temlyakov, “Stable recovery of sparse overcomplete representations in the presence of noise,” IEEE Trans. Inf. Theory, vol. 52, no. 1, pp. 6–18, Jan. 2006. [11] D. L. Donoho and X. Huo, “Uncertainty principles and ideal atomic decomposition,” IEEE Trans. Inf. Theory, vol. 47, no. 7, pp. 2845–2862, Nov. 2001. [12] B. Efron, T. Hastie, I. Johnstone, and R. Tibshirani, “Least angle regression (with discussion,” Ann. Statist., vol. 32, pp. 407–451, 2004. [13] J.-J. Fuchs, “On sparse representations in arbitrary redundant bases,” IEEE Trans. Inf. Theory, vol. 50, no. 6, pp. 1341–1344, Jun. 2004. [14] J.-J. Fuchs, “Recovery of exact sparse representations in the presence of bounded noise,” IEEE Trans. Inf. Theory, vol. 51, no. 10, pp. 3601–3608, Oct. 2005. [15] M. Rudelson and R. Vershynin, “Sparse reconstruction by convex relaxation: Fourier and Gaussian measurements,” in Proc. 40th Annu. Conf. Information Sciences and Systems, Princeton, NJ, Mar. 2006, pp. 207–212. [16] R. Tibshirani, “Regression shrinkage and selection via the lasso,” J. Roy. Statist. Soc. Ser. B, vol. 58, pp. 267–288, 1996. [17] J. Tropp, “Greed is good: Algorithmic results for sparse approximation,” IEEE Trans. Inf. Theory, vol. 50, no. 10, pp. 2231–2242, Oct. 2004. [18] J. Tropp, “Just relax: Convex programming methods for identifying sparse signals in noise,” IEEE Trans. Inf. Theory, vol. 52, no. 3, pp. 1030–1051, Mar. 2006. T. Tony Cai received the Ph.D. degree from Cornell University, Ithaca, NY, in 1996. He is currently the Dorothy Silberberg Professor of Statistics at the Wharton School of the University of Pennsylvania, Philadelphia. His research interests include high-dimensional inference, large-scale multiple testing, nonparametric function estimation, functional data analysis, and statistical decision theory. Prof. Cai is the recipient of the 2008 COPSS Presidents’ Award and a fellow of the Institute of Mathematical Statistics.Inequality (V.3) now follows by verifying directly that for all . ACKNOWLEDGMENT The authors wish to thank the referees for thorough and useful comments which have helped to improve the presentation of the paper. REFERENCES[1] W. Bajwa, J. Haupt, J. Raz, S. Wright, and R. Nowak, “Toeplitz-structured compressed sensing matrices,” in Proc. IEEE SSP Workshop, Madison, WI, Aug. 2007, pp. 294–298. [2] T. Cai, “On block thresholding in wavelet regression: Adaptivity, block size and threshold level,” Statist. Sinica, vol. 12, pp. 1241–1273, 2002. [3] E. J. Candes, “The restricted isometry property and its implications for compressed sensing,” Compte Rendus de l’ Academie des Sciences Paris, ser. I, vol. 346, pp. 589–592, 2008. [4] E. J. Candes, J. Romberg, and T. Tao, “Stable signal recovery from incomplete and inaccurate measurements,” Commun. Pure Appl. Math., vol. 59, pp. 1207–1223, 2006. [5] E. J. Candes and T. Tao, “Decoding by linear programming,” IEEE Trans. Inf. Theory, vol. 51, no. 12, pp. 4203–4215, Dec. 2005.Guangwu Xu received the Ph.D. degree in mathematics from the State University of New York (SUNY), Buffalo. He is now with the Department of Electrical engineering and Computer Science, University of Wisconsin-Milwaukee. His research interests include cryptography and information security, computational number theory, algorithms, and functional analysis.Jun Zhang (S’85–M’88–SM’01) received the B.S. degree in electrical and computer engineering from Harbin Shipbuilding Engineering Institute, Harbin, China, in 1982 and was admitted to the graduate program of the Radio Electronic Department of Tsinghua University. After a brief stay at Tsinghua, he came to the U.S. for graduate study on a scholarship from the Li Foundation, Glen Cover, New York. He received the M.S. and Ph.D. degrees, both in electrical engineering, from Rensselaer Polytechnic Institute, Troy, NY, in 1985 and 1988, respectively. He joined the faculty of the Department of Electrical Engineering and Computer Science, University of Wisconsin-Milwaukee, and currently is a Professor. His research interests include image processing and computer vision, signal processing and digital communications. Prof. Zhang has been an Associate Editor of IEEE TRANSACTIONS ON IMAGE PROCESSING.Authorized licensed use limited to: University of Pennsylvania. Downloaded on June 17, 2009 at 09:48 from IEEE Xplore. Restrictions apply.。
2014_ICASSP_EFFICIENT CONVOLUTIONAL SPARSE CODING

EFFICIENT CONVOLUTIONAL SPARSE CODINGBrendt WohlbergTheoretical DivisionLos Alamos National LaboratoryLos Alamos,NM87545,USAABSTRACTWhen applying sparse representation techniques to images,the standard approach is to independently compute the rep-resentations for a set of image patches.Thismethod performs very well in a variety of applications,butthe independent sparse coding of each patch results in a rep-resentation that is not optimal for the image as a whole.Arecent development is convolutional sparse coding,in whicha sparse representation for an entire image is computed by re-placing the linear combination of a set of dictionary vectorsby the sum of a set of convolutions with dictionaryfilters.Adisadvantage of this formulation is its computational expense,but the development of efficient algorithms has received someattention in the literature,with the current leading method ex-ploiting a Fourier domain approach.The present paper intro-duces a new way of solving the problem in the Fourier do-main,leading to substantially reduced computational cost.Index Terms—Sparse Representation,Sparse Coding,Convolutional Sparse Coding,ADMM1.INTRODUCTIONOver the past15year or so,sparse representations[1]havebecome a very widely used technique for a variety of prob-lems in image processing.There are numerous approaches tosparse coding,the inverse problem of computing a sparse rep-resentation of a signal or image vector s,one of themost widely used being Basis Pursuit DeNoising(BPDN)[2]arg minx 12D x−s 22+λ x 1,(1)where D is a dictionary matrix,x is the sparse representation, andλis a regularization parameter.When applied to images, decomposition is usually applied independently to a set of overlapping image patches covering the image;this approach is convenient,but often necessitates somewhat ad hoc subse-quent handling of the overlap between patches,and results in a representation over the whole image that is suboptimal.This research was supported by the U.S.Department of Energy through the LANL/LDRD Program.More recently,these techniques have also begun to be ap-plied,with considerable success,to computer vision problems such as face recognition[3]and image classification[4,5,6]. It is in this application context that convolutional sparse rep-resentations were introduced[7],replacing(1)with arg min{x m}12md m∗x m−s22+λmx m 1,(2)where{d m}is a set of M dictionaryfilters,∗denotes convo-lution,and{x m}is a set of coefficient maps,each of which is the same size as s.Here s is a full image,and the{d m} are usually much smaller.For notational simplicity s and x m are considered to be N dimensional vectors,where N is the the number of pixels in an image,and the notation{x m}is adopted to denote all M of the x m stacked as a single column vector.The derivations presented here are for a single image with a single color band,but the extension to multiple color bands(for both image andfilters)and simultaneous sparse coding of multiple images is mathematically straightforward.The original algorithm proposed for convolutional sparse coding[7]adopted a splitting technique with alternating minimization of two subproblems,thefirst consisting of the solution of a large linear system via an iterative method, and the other a simple shrinkage.The resulting alternating minimization algorithm is similar to one that would be ob-tained within an Alternating Direction Method of Multipliers (ADMM)[8,9]framework,but requires continuation on the auxiliary parameter to enforce the constraint inherent in the splitting.All computation is performed in the spatial domain, the authors expecting that computation in the Discrete Fourier Transform(DFT)domain would result in undesirable bound-ary artifacts[7].Other algorithms that have been proposed for this problem include coordinate descent[10],and a proximal gradient method[11],both operating in the spatial domain.Very recently,an ADMM algorithm operating in the DFT domain has been proposed for dictionary learning for con-volutional sparse representations[12].The use of the Fast Fourier Transform(FFT)in solving the relevant linear sys-tems is shown to give substantially better asymptotic perfor-mance than the original spatial domain method,and evidence is presented to support the claim that the resulting boundary2014 IEEE International Conference on Acoustic, Speech and Signal Processing (ICASSP)effects are not significant.The present paper describes a convolutional sparse coding algorithm that is derived within the ADMM framework and exploits the FFT for computational advantage.It is very sim-ilar to the sparse coding component of the dictionary learning algorithm of[12],but introduces a method for solving the linear systems that dominate the computational cost of the al-gorithm in time that is linear in the number offilters,instead of cubic as in the method of[12].2.ADMM ALGORITHMRewriting(2)in a form suitable for ADMM by introducing auxiliary variables{y m},we havearg min {x m},{y m}12md m∗x m−s22+λmy m 1 such that x m−y m=0∀m,(3)for which the corresponding iterations(see[8,Sec.3]),with dual variables{u m},are{x m}(k+1)=arg min{x m}12md m∗x m−s22+ρ2mx m−y(k)m+u(k)m22(4){y m}(k+1)=arg min{y m}λmy m 1+ρ2mx(k+1)m−y m+u(k)m22(5)u(k+1) m =u(k)m+x(k+1)m−y(k+1)m.(6)Subproblem(5)is solved via shrinkage/soft thresholding asy(k+1) m =Sλ/ρx(k+1)m+u(k)m,(7)whereSγ(u)=sign(u) max(0,|u|−γ),(8) with sign(·)and|·|of a vector considered to be applied element-wise.The computational cost is O(MN).The only computationally expensive step is solving(4), which is of the formarg min {x m}12md m∗x m−s22+ρ2mx m−z m 22.(9)2.1.DFT Domain FormulationAn obvious approach is to attempt to exploit the FFT for ef-ficient implementation of the convolution via the DFT convo-lution theorem.(This does involve some increase in memory requirement since the d m are zero-padded to the size of the x m before application of the FFT.)Define linear operators D m such that D m x m=d m∗x m,and denote the variables D m,x m,s,and z m in the DFT domain byˆD m,ˆx m,ˆs,andˆz m respectively.It is easy to show via the DFT convolution theorem that(9)is equivalent toarg min{ˆx m}12mˆDmˆx m−ˆs22+ρ2mˆx m−ˆz m 22(10)with the{x m}minimizing(9)being given by the inverse DFT of the{ˆx m}minimizing(10).DefiningˆD= ˆDˆD1...,ˆx=⎛⎜⎝ˆx0ˆx1...⎞⎟⎠,ˆz=⎛⎜⎝ˆz0ˆz1...⎞⎟⎠,(11) this problem can be expressed asarg minˆx12ˆDˆx−ˆs22+ρ2ˆx−ˆz 22,(12) the solution being given by(ˆD HˆD+ρI)ˆx=ˆD Hˆs+ρˆz.(13) 2.2.Independent Linear SystemsMatrixˆD has a block structure consisting of M concatenated N×N diagonal matrices,where M is the number offilters and N is the number of samples in s.ˆD HˆD is an MN×MN matrix,but due to the diagonal block(not block diagonal) structure ofˆD,a row ofˆD H with its non-zero element at col-umn n will only have a non-zero product with a column ofˆD with its non-zero element at row n.As a result,there is no interaction between elements ofˆD corresponding to differ-ent frequencies,so that(as pointed out in[12])one need only solve N independent M×M linear systems to solve(13). Bristow et al.[12]do not specify how they solve these linear systems(and their software implementation was not available for inspection),but since they rate the computational cost of solving them as O(M3),it is reasonable to conclude that they apply a direct method such as Gaussian elimination.This can be very effective[8,Sec. 4.2.3]when it is possible to pre-compute and store a Cholesky or similar decomposition of the linear system(s),but in this case it is not practical unless M is very small,having an O(M2N)memory requirement for storage of these decomposition.Nevertheless,this remains a reasonable approach,the only obvious alternative being an iterative method such as conjugate gradient(CG).A more careful analysis of the unique structure of this problem,however,reveals that there is an alternative,and vastly more effective,solution.First,define the m th block of the right hand side of(13)asˆr m=ˆD H mˆs+ρˆz m,(14)so that⎛⎜⎝ˆr 0ˆr 1...⎞⎟⎠=ˆDH ˆs +ρˆz .(15)Now,denoting the n th element of a vector x by x (n )to avoid confusion between indexing of the vectors themselves and se-lection of elements of these vectors,definev n =⎛⎜⎝ˆx 0(n )ˆx 1(n )...⎞⎟⎠b n =⎛⎜⎝ˆr 0(n )ˆr 1(n )...⎞⎟⎠,(16)and define a n as the column vector containing all of the non-zero entries from column n of ˆDH ,i.e.writing ˆD =⎛⎜⎜⎜⎝ˆd 0,00...ˆd 1,00...0ˆd 0,10...0ˆd 1,10...00ˆd 0,2...00ˆd 1,2...........................⎞⎟⎟⎟⎠(17)thena n =⎛⎜⎝ˆd ∗0,nˆd ∗1,n ...⎞⎟⎠,(18)where ∗denotes complex conjugation.The linear system to solve corresponding to element n of the {x m }is (a n a H n +ρI )v n =b n .(19)The critical observation is that the matrix on the left handside of this system consists of a rank-one matrix plus a scaled identity.Applying the Sherman-Morrison formula(A +uv H )−1=A −1−A −1uv H A −11+u H A −1v (20)gives(ρI +aa H )−1=ρ−1 I −aaHρ+a H a,(21)so that the solution to (19)isv n =ρ−1b n −a H n b nρ+a H n a na n.(22)The only vector operations here are inner products,element-wise addition,and scalar multiplication,so that this method is O (M )instead of O (M 3)as in [12].The cost of solving N of these systems is O (MN ),and the cost of the FFTs is O (MN log N ).Here it is the cost of the FFTs that dominates,whereas in [12]the cost of solving the DFT domain linear systems dominates the cost of the FFTs.This approach can be implemented in an interpreted lan-guage such as Matlab in a form that avoids explicit iteration over the N frequency indices by passing data for all N in-dices as a single array to the relevant linear-algebraic routines (commonly referred to as vectorization in Matlab terminol-ogy).Some additional computation time improvement is pos-sible,at the cost of additional memory requirements,by pre-computing a H n /(ρ+a Hn a n )in (22).2.3.Algorithm SummaryThe proposed algorithm is summarized in Alg.1.stop-ping criteria are those discussed in [8,Sec.3.3],together withan upper bound on the number of iterations.The options for the ρupdate are (i)fixed ρ(i.e.no update),(ii)the adaptive update strategy described in [8,Sec. 3.4.1],and the multi-plicative increase scheme advocated in [12].Input :image s ,filter dictionary {d m },parameters λ,ρPrecompute:FFTs of {d m }→{ˆDm },FFT of s →ˆs Initialize:{y m }={u m }=0while stopping criteria not met doCompute FFTs of {y m }→{ˆym },{u m }→{ˆu m }Compute {ˆxm }using the method in Sec.2.2Compute inverse FFTs of {ˆxm }→{x m }{y m }=S λ/ρ({x m }+{u m }){u m }={u m }+{x m }−{y m }Update ρif appropriate endOutput :Coefficient maps {x m }Algorithm 1:Summary of proposed ADMM algorithm The computational cost of the algorithm components is O (MN log N )for the FFTs,order O (MN )for the proposed linear solver,and O (MN )for both the shrinkage and dual variable update,so that the cost of the entire algorithm is O (MN log N ),dominated by the cost of FFTs.In contrast,the cost of the algorithm proposed in [12]is O (M 3N )(there is also an O (MN log N )cost for FFTs,but it is dominated by the O (M 3N )cost of the linear solver),and the cost of the original spatial-domain algorithm [7]is O (M 2N 2L ),where L is the dimensionality of the filters.3.DICTIONARY LEARNINGThe extension of (2)to learning a dictionary from training data involves replacing the minimization with respect to x m with minimization with respect to both x m and d m .The op-timization is invariably performed via alternating minimiza-tion between the two variables,the most common approach consisting of a sparse coding step followed by a dictionary update [13].The commutativity of convolution suggests that the DFT domain solution of Sec.2.1can be directly applied in minimizing with respect to d m instead of x m ,but this is not possible since the d m are of constrained size,and must be zero-padded to the size of the x m prior to a DFT domain im-plementation of the convolution.If the size constraint is im-plemented in an ADMM framework [14],however,the prob-lem is decomposed into a computationally cheap subproblem corresponding to a projection onto to constraint set,and an-other subproblem that can be efficiently solved by extending the method in Sec.2.1.This iterative algorithm for the dictio-nary update can alternate with a sparse coding stage to form amore traditional dictionary learning method [15],or the sub-problems of the sparse coding and dictionary update algo-rithms can be combined into a single ADMM algorithm [12].4.RESULTScomparison of execution times for the algorithm (λ=0.05)with different methods of solving the linear system,for a set of overcomplete 8×8DCT dictionaries and the 512×512greyscale Lena image,is presented in Fig.1.It is worth em-phasizing that this is a large image by the standards of prior publications on convolutional sparse coding;the test images in [12],for example,are 50×50and 128×128pixels in size.The Gaussian elimination solution is computed using a Cholesky decomposition (since it is,in general,impossible to cache this decomposition,it is necessary to recompute it at every solution),as implemented by the Matlab mldivide function,and is applied by iterating over all frequencies in the apparent absence of any practical alternative.The conjugate gradient solution is computed using two different relative error tolerances.A significant part of the computational advantage here of CG over the direct method is that it is applied simultaneously over all frequencies.The two curves for the proposed solver based on the Sherman-Morrison formula illustrate the significant gain from an implementation that simultaneously solves over all frequencies and that the relative advantage of doing so de-creases with increasing M .Dictionary size (M )E x e c u t i o n t i m e (s )512256128641e+051e+041e+031e+021e+01Fig.1.A comparison of execution times for 10steps of the ADMM algorithm for different methods of solving the lin-ear system:Gaussian elimination (GE),Conjugate Gradient with relative error tolerance 10−5(CG 10−5)and 10−3(CG 10−3),and Sherman-Morrison implemented with a loop over frequencies (SM-L)or jointly over all frequencies (SM-V).The performance of the three ρupdate strategies dis-cussed in the previous section was compared by sparse cod-ing a 256×256Lena image using a 9×9×512dictionary (from [16],by the authors of [17])with a fixed value of λ=0.02and a range of initial ρvalues ρ0.The resulting values of the functional in (2)after 100,500,and 1000itera-tions of the proposed algorithm are displayed in Table 1.The adaptive update strategy uses the default parameters of [8,Sec. 3.4.1],and the increasing strategy uses a multiplica-tive update by a factor of 1.1with a maximum of 105,as advocated by [12].In summary,a fixed ρcan perform well,but is sensitive to a good choice of parameter.When initialized with a small ρ0,the increasing ρstrategy provides the most rapid decrease in functional value,but thereafter converges very slowly.Over-all,unless rapid computation of an approximate solution is desired,the adaptive ρstrategy appears to provide the best performance,with the least sensitivity to choice of ρ0.This is-sue is complex,however,and further experimentation is nec-essary before drawing any general conclusions that could be considered valid over a broad range of problems.Iter.ρ010−210−1100101102103Fixed ρ10028.2727.8018.1010.099.7611.6050028.0522.2511.118.899.1110.13100027.8017.009.648.828.969.71Adaptive ρ10021.6216.9714.5610.7111.1411.4150010.8110.239.819.019.189.0910009.449.219.068.838.878.84Increasing ρ10014.789.829.509.9011.5115.155009.559.459.469.8911.4714.5110009.539.449.459.8811.4113.97Table parison of functional value convergence for thesame problem with three different ρupdate strategies.5.CONCLUSIONA computationally efficient algorithm is proposed for solving the convolutional sparse coding problem in the Fourier do-main.This algorithm has the same general structure as a pre-viously proposed approach [12],but enables a very significantreduction in computational cost by careful design of a linear solver for the most critical component of the iterative algo-rithm.The theoretical computational cost of the algorithm is reduced from O (M 3)to O (MN log N )(where N is the di-mensionality of the data and M is the number of elementsin the dictionary),and is also shown empirically to result in greatly reduced computation time.The significant improve-ment in efficiency of the proposed approach is expected togreatly increase the range of problems that can practically be addressed via convolutional sparse representations.6.REFERENCES[1]A.M.Bruckstein,D.L.Donoho,and M.Elad,“Fromsparse solutions of systems of equations to sparse mod-eling of signals and images,”SIAM Review,vol.51, no.1,pp.34–81,2009.doi:10.1137/060657704[2]S.S.Chen,D.L.Donoho,and M.A.Saunders,“Atomicdecomposition by basis pursuit,”SIAM Journal on Sci-entific Computing,vol.20,no.1,pp.33–61,1998.doi:10.1137/S1064827596304010[3]J.Wright,A.Y.Yang,A.Ganesh,S.S.Sastry,andY.Ma,“Robust face recognition via sparse representa-tion,”IEEE Transactions on Pattern Analysis and Ma-chine Intelligence,vol.31,no.2,pp.210–227,February 2009.doi:10.1109/tpami.2008.79[4]Y.Boureau,F.Bach,Y.A.LeCun,and J.Ponce,“Learn-ing mid-level features for recognition,”in Proceedings of the IEEE Conference on Computer Vision and Pat-tern Recognition(CVPR),June2010,pp.2559–2566.doi:10.1109/cvpr.2010.5539963[5]J.Yang,K.Yu,and T.S.Huang,“Supervisedtranslation-invariant sparse coding,”Proceedings of the IEEE Conference on Computer Vision and Pat-tern Recognition(CVPR),pp.3517–3524,2010.doi:10.1109/cvpr.2010.5539958[6]J.Mairal,F.Bach,and J.Ponce,“Task-driven dictionarylearning,”IEEE Transactions on Pattern Analysis and Machine Intelligence,vol.34,no.4,pp.791–804,April 2012.doi:10.1109/tpami.2011.156[7]M.D.Zeiler,D.Krishnan,G.W.Taylor,and R.Fer-gus,“Deconvolutional networks,”in Proceedings of the IEEE Conference on Computer Vision and Pat-tern Recognition(CVPR),June2010,pp.2528–2535.doi:10.1109/cvpr.2010.5539957[8]S.Boyd,N.Parikh,E.Chu,B.Peleato,and J.Eckstein,“Distributed optimization and statistical learning via the alternating direction method of multipliers,”Founda-tions and Trends in Machine Learning,vol.3,no.1,pp.1–122,2010.doi:10.1561/2200000016[9]J.Eckstein,“Augmented Lagrangian and alternatingdirection methods for convex optimization:A tutorial and some illustrative computational results,”Rutgers Center for Operations Research,Rutgers University, Rutcor Research Report RRR32-2012,December 2012.[Online].Available:/pub/ rrr/reports2012/322012.pdf[10]K.Kavukcuoglu,P.Sermanet,Y.Boureau,K.Gregor,M.Mathieu,and Y.A.LeCun,“Learning convolutionalfeature hierachies for visual recognition,”in Advances in Neural Information Processing Systems(NIPS2010), 2010.[11]R.Chalasani,J.C.Principe,and N.Ramakrishnan,“A fast proximal method for convolutional sparse cod-ing,”in Proceedings of the International Joint Confer-ence on Neural Networks(IJCNN),Aug.2013,pp.1–5.doi:10.1109/IJCNN.2013.6706854[12]H.Bristow, A.Eriksson,and S.Lucey,“Fast con-volutional sparse coding,”in Proceedings of the IEEE Conference on Computer Vision and Pat-tern Recognition(CVPR),Jun.2013,pp.391–398.doi:10.1109/CVPR.2013.57[13]B.Mailh´e and M.D.Plumbley,“Dictionary learningwith large step gradient descent for sparse representa-tions,”in Latent Variable Analysis and Signal Sepa-ration,ser.Lecture Notes in Computer Science,F.J.Theis,A.Cichocki,A.Yeredor,and M.Zibulevsky,Eds.Springer Berlin Heidelberg,2012,vol.7191,pp.231–238.doi:10.1007/978-3-642-28551-629[14]M.V.Afonso,J.M.Bioucas-Dias,and M. A.T.Figueiredo,“An Augmented Lagrangian approach to the constrained optimization formulation of imaging inverse problems,”IEEE Transactions on Image Pro-cessing,vol.20,no.3,pp.681–695,March2011.doi:10.1109/tip.2010.2076294[15]K.Engan,S.O.Aase,and J.H.Husøy,“Method ofoptimal directions for frame design,”in Proceedings of the IEEE International Conference on Acoustics, Speech,and Signal Processing(ICASSP),vol.5,1999, pp.2443–2446.doi:10.1109/icassp.1999.760624 [16]J.Mairal,Software available from http://lear.inrialpes.fr/people/mairal/denoise ICCV09.tar.gz.[17]J.Mairal,F.Bach,J.Ponce,G.Sapiro,and A.Zis-serman,“Non-local sparse models for image restora-tion,”in Proceedings of the IEEE International Con-ference on Computer Vision(CVPR),2009,pp.2272–2279.doi:10.1109/iccv.2009.5459452。
Unnatural L0 Sparse Representation for Natural Image Deblurring

(e) our x ˜
Hale Waihona Puke (f) final restored image
Figure 1. Intermediate unnatural image representation exists in many state-of-the-art approaches.
1.1. Analysis
Prior MAP-based approaches can be roughly categorized into two groups, i.e., methods with explicit edge prediction 1
taining salient image edges. These maps are vital to make motion deblurring accomplishable in different MAP-variant frameworks. Implicit Regularization Shan et al. [20] adopted a sparse image prior. This method, in the first a few iterations, uses a large regularization weight to suppress insignificant structures and preserve strong ones, creating crisp-edge image results, as exemplified in Fig. 1(b). This scheme is useful to remove harmful subtle image structures, making kernel estimation generally follow correct directions in iterations. Krishnan et al. [16] used an L1 /L2 regularization scheme. The main feature is to adapt L1 -norm regularization by treating the L2 -norm of image gradients as a weight in iterations. One intermediate result from this method is shown in Fig. 1(c). The main difference between this form and that of [20] is on the way to adapt regularization strength in iterations. Note both of them suppress details in the early stage during optimization. Explicit Filter and Selection In [19, 3], shock filter is introduced to create a sharpened reference map for kernel estimation. Cho and Lee [3] performed bilateral filter and edge thresholding in each iteration to remove small- and medium-amplitude structures (illustrated in Fig. 1(d)), also avoiding the trivial solution. Xu and Jia [25] proposed a texture-removal strategy, explained and extended in [28], to guide edge selection and detect large-scale structures. The resulting edge map in each step is also a small-edge-subdued version from the natural input. These two schemes have been extensively validated in motion deblurring. Unnatural Representation The above techniques enable several successful MAP frameworks in motion deblurring. All of them have their intermediate image results or edge maps different from a natural one, as shown in Fig. 1, due to only containing high-contrast and step-like structures while suppressing others. We generally call them unnatural representation, which is the key to robust kernel estimation in motion deblurring.
Sparse Subspace Clustering_ Algorithm, Theory, and Applications
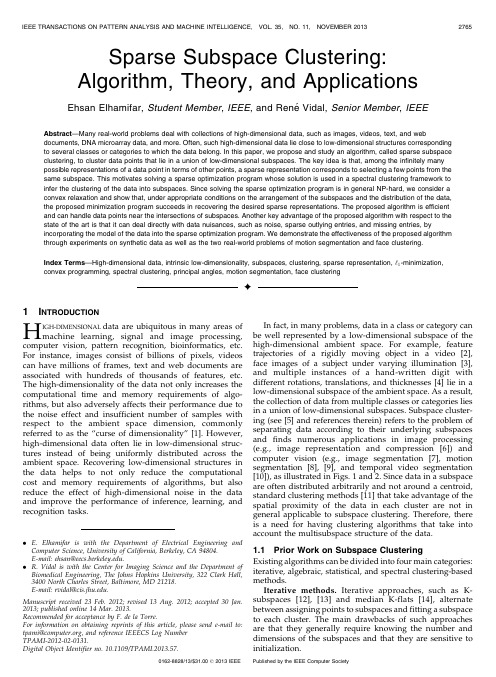
. E. Elhamifar is with the Department of Electrical Engineering and Computer Science, University of California, Berkeley, CA 94804. E-mail: ehsan@.
IEEE TRANSACTIONS ON PATTERN ANALYSIS AND MACHINE INTELLIGENCE, VOL. 35, NO. 11, NOVEMBER 2013
2765
Sparse Subspace Clustering: Algorithm, Theory, and Applications
Index Terms—High-dimensional data, intrinsic low-dimensionality, subspaces, clustering, sparse representation, ‘1-minimization, convex programming, spectral clustering, principal angles, motion segmentation, face clustering
On Single Image Scale-Up using Sparse-Representations(Michael Elad 的文章)
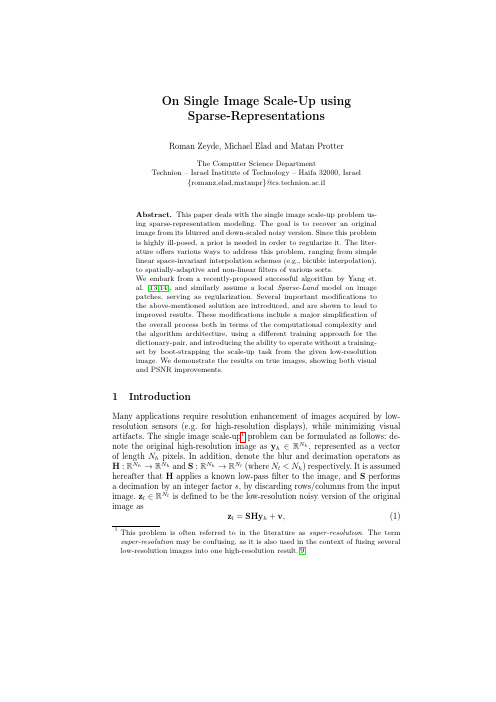
2
Roman Zeyde, Michael Elad and Matan Protter
for an additive i.i.d. white Gaussian noise, denoted by v ∼ N 0, σ2I . Given zl, the problem is to find yˆ ∈ RNh such that yˆ ≈ yh. Due to the
hereafter that H applies a known low-pass filter to the image, and S performs
a decimation by an integer factor s, by discarding rows/columns from the input
{romanz,elad,matanpr}@cs.technion.ac.il
Abstract. This paper deals with the single image scale-up problem using sparse-representation modeling. The goal is to recover an original image from its blurred and down-scaled noisy version. Since this problem is highly ill-posed, a prior is needed in order to regularize it. The literature offers various ways to address this problem, ranging from simple linear space-invariant interpolation schemes (e.g., bicubic interpolation), to spatially-adaptive and non-linear filters of various sorts. We embark from a recently-proposed successful algorithm by Yang et. al. [13,14], and similarly assume a local Sparse-Land model on image patches, serving as regularization. Several important modifications to the above-mentioned solution are introduced, and are shown to lead to improved results. These modifications include a major simplification of the overall process both in terms of the computational complexity and the algorithm architecture, using a different training approach for the dictionary-pair, and introducing the ability to operate without a trainingset by boot-strapping the scale-up task from the given low-resolution image. We demonstrate the results on true images, showing both visual and PSNR improvements.
Sparse Representations

Sparse RepresentationsTara N. SainathSLTC Newsletter, November 2010Sparse representations (SRs), including compressive sensing (CS), have gained popularity in the last few years as a technique used to reconstruct a signal from few training examples, a problem which arises in many machine learning applications. This reconstruction can be defined as adaptively finding a dictionary which best represents the signal on a per sample basis. This dictionary could include random projections, as is typically done for signal reconstruction, or actual training samples from the data, which is explored in many machine learning applications. SRs is a rapidly growing field with contributions in a variety of signal processing and machine learning conferences such as ICASSP, ICML and NIPS, and more recently in speech recognition. Recently, a special session on Sparse Representations took place at Interspeech 2010 in Makuhari, Japan from March 26-30, 2010. Below work from this special session is summarized in more detail.FACE RECOGNITION VIA COMPRESSIVE SENSINGYang et al. present a method for image-based robust face recognition using sparse representations [1]. Most state-of-the art face recognition systems suffer from limited abilities to handle image nuisances such as illumination, facial disguise, and pose misalignment. Motivated by work in compressive sensing, the described method finds the sparsest linear combination of a query image using all prior training images, where the dominant sparse coefficients reveal the identity of the query image. In addition, extensions of applying sparse representations for face recognition also address a wide range of problems in the field of face recognition, such as dimensionality reduction, image corruption, and face alignment. The paper also provides useful guidelines to practitioners working in similar fields, such as speech recognition.EXEMPLAR-BASED SPARSE REPRESENTATION FEATURESIn Sainath et al. [2], the authors explore the use of exemplar-based sparse representations (SRs) to map test features into the linear span of training examples. Specifically, given a test vector y and a set of exemplars from the training set, which are put into a dictionary H, y is represented as a linear combination of training examples by solving y = H&beta subject to a spareness constraint on &beta . The feature H&beta can be thought of as mapping test sample y back into the linear span of training examples in H.The authors show that the frame classification accuracy using SRs is higher than using a Gaussian Mixture Model (GMM), showing that not only do SRs move test features closer totraining, but also move the features closer to the correct class. A Hidden Markov Model (HMM) is trained on these new SR features and evaluated in a speech recognition task. On the TIMIT corpus, applying the SR features on top of our best discriminatively trained system allows for a 0.7% absolute reduction in phonetic error rate (PER). Furthermore, on a large vocabulary 50 hour broadcast news task, a reduction in word error rate (WER) of 0.3% absolute, demonstrating the benefit of these SR features for large vocabulary.OBSERVATION UNCERTAINTY MEASURES FOR SPARSE IMPUTATIONMissing data techniques are used to estimate clean speech features from noisy environments by finding reliable information in the noisy speech signal. Decoding is then performed based on either the reliable information alone or using both reliable and unreliable information where unreliable parts of the signal are reconstructed using missing data imputation prior to decoding. Sparse imputation (SI) is an exemplar-based reconstruction method which is based on representing segments of the noisy speech signal as linear combinations of as few as possible clean speech example segments.Decoding accuracy depends on several factors including the uncertainty in the speech segment. Gemmeke et al. propose various uncertainty measures to characterize the expected accuracy of a sparse imputation based missing data method [3]. In experiments on noisy large vocabulary speech data, using observation uncertainties derived from the proposed measures improved the speech recognition performance on features estimated with SI. Relative error reductions up to 15% compared to the baseline system using SI without uncertainties were achieved with the best measures.SPARSE AUTO-ASSOCIATIVE NEURAL NETWORKS: THEORY AND APPLICATION TO SPEECH RECOGNITIONGarimella et al. introduce a sparse auto-associative neural network (SAANN) in which the internal hidden layer output is forced to be sparse [4]. This is done by adding a sparse regularization term to the original reconstruction error cost function, and updating the parameters of the network to minimize the overall cost. The authors show the benefit of SAANN on the TIMIT phonetic recognition task. Specifically, a set of perceptual linear prediction (PLP) features are provided as input into the SAANN structure, and a set of sparse hidden layer outputs are produced and used as features. Experiments with the SAANN features on the TIMIT phoneme recognition system show a relative improvement in phoneme error rate of 5.1% over the baseline PLP features.DATA SELECTION FOR LANGUAGE MODELING USING SPARSE REPRESENTATIONSThe ability to adapt language models to specific domains from large generic text corpora is of considerable interest to the language modeling community. One of the key challenges is toidentify the text material relevant to a domain in the generic text collection. The text selection problem can be cast in a semi-supervised learning framework where the initial hypothesis from a speech recognition system is used to identify relevant training material. Sethy et al [5] present a novel sparse representation formulation which selects a sparse set of relevant sentences from the training data which match the test set distribution. In this formulation, the training sentences are treated as the columns of the sparse representation matrix and then-gram counts as the rows. The target vector is the n-gram probability distribution for the test data. A sparse solution to this problem formulation identifies a few columns which can best represent the target test vector, thus identifying the relevant set of sentences from the training data. Rescoring results with the language model built from the data selected using the proposed method yields modest gains on the English broadcast news RT-04 task, reducing the word error rate from 14.6% to 14.4%.SPARSE REPRESENTATIONS FOR TEXT CATEGORIZATIONGiven the superior performance of SRs compared to other classifiers for both image classification and phonetic classification, Sainath et al. extends the use of SRs for text classification [6], a method which has thus far not been explored for this domain. Specifically, Sainath et al. show how SRs can be used for text classification and how their performance varies with the vocabulary size of the documents. The research finds that the SR method offers promising results over the Naive Bayes (NB) classifier, a standard baseline classifier used for text categorization, thus introducing an alternative class of methods for text categorization.CONCLUSIONSThis article presented an overview about sparse representation research in the areas of face recognition, speech recognition, language modeling and text classification. For more information, please see:[1] A. Yang, Z. Zhou, Y. Ma and S. Shankar Sastry, "Towards a robust face recognition system using compressive sensing", in Proc. Interspeech, September 2010.[2] T. N. Sainath, B. Ramabhadran, D. Nahamoo, D. Kanevsky and A. Sethy,“ Exemplar-Based Sparse Representation Features for Speech Recognition ," in Proc. Interspeech, September 2010.[3] J. F. Gemmeke, U. Remes and K. J. Palomäki, "Observation Uncertainty Measures for Sparse Imputation", in Proc. Interspeech, September 2010.[4] G.S.V.S. Sivaram, S. Ganapathy and H. Hermansky, "Sparse Auto-associative Neural Networks: Theory and Application to Speech Recognition", in Proc. Interspeech, September 2010.[5] A. Sethy, T. N. Sainath, B. Ramabhadran and D. Kanevsky, “ Data Selection for Language Modeling Using Sparse Representations," in Proc. Interspeech, September 2010.[6] T. N. Sainath, S. Maskey, D. Kanevsky, B. Ramabhadran, D. Nahamoo and J. Hirschberg, “ Sparse Representations for Text Categorization," in Proc. Inte rspeech, September 2010.。
Representational Learning
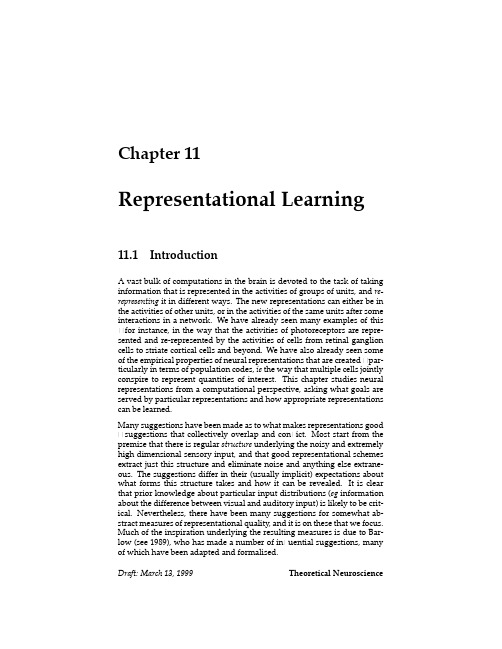
2
Representational Learning
One rather abstract and general notion comes from considering casual models. The idea is that an input, such as the image of a scene, has distal causes, such as objects at given locations illuminated by some particular lighting scheme observed from a particular viewing direction. These causes make for the structure in the input, and, since inferences and decisions are normally based on underlying causes, make for appropriate representations of input. To put it another way, the images that we see live in an O (108 )dimensional space which has one dimension for each photoreceptor (plus one dimension for time). However, the set of all images we might ever naturally see is much smaller, since it is constrained by how images are actually generated. The image generation process specifies the natural coordinates for the observable images, in terms of the things that cause them, and it is these coordinates that we seek to use in order to represent new images. More concrete notions about extracting the structure in the input (and therefore also about extracting causes) include finding lower dimensional projections of the input that nevertheless convey most of the information in the input, or code the input in a way that makes it cheap to communicate, finding projections whose activities are mutually independent (also known as factorial) or sparse, or with distributions that are statistically unlikely and therefore potentially unusually informative. A major source of statistical structure in the input comes from groups of transformations – for instance translation and scale for visual images. Extracting the structure correctly requires respecting (or discovering) these transformations. We consider a number of learning methods that are classed as being unsupervised or self-supervised, in the sense that no information other than the patterns themselves is explicitly provided to guide the representational choice. This is by contrast with supervised methods described in chapter 8, which learn appropriate representations in the service of particular tasks. A main reason for studying unsupervised methods is that they are likely to be much more common than supervised ones. For instance, there is only a derisory amount of supervisory information available to learn how to perform a task such as object recognition that is based on the activities in the O (108 )-dimensional space of activities of the photoreceptors. Unsupervised learning is also often used to try to capture what is happening during activity-dependent development. We saw in chapters 8 and 9 mathematical models that capture the nature and plasticity of the development of cells in visual cortex on the basis of the activity of input neurons. We seek to provide quantifiable goals for this unsupervised process. As will become evident, in neural terms, unsupervised learning methods are still in their infancy – they are only barely capable of generating the forms of sophisticated population code representations that we have discussed and dissected, and even central concepts, such as the notion of causes in causal models and the groups of relevant transformations that particular inputs undergo are only relatively weakly formulated. The two things that unsupervised learning methods have to work with are Peter Dayan and L.F. Abbott Draft: March 13, 1999
- 1、下载文档前请自行甄别文档内容的完整性,平台不提供额外的编辑、内容补充、找答案等附加服务。
- 2、"仅部分预览"的文档,不可在线预览部分如存在完整性等问题,可反馈申请退款(可完整预览的文档不适用该条件!)。
- 3、如文档侵犯您的权益,请联系客服反馈,我们会尽快为您处理(人工客服工作时间:9:00-18:30)。
( k )ຫໍສະໝຸດ By a representation of s in D we mean a (column) vector = 2 K (resp., in K ) such that s = D . We notice that when K > N , the vectors of D are no longer linearly independent and the representation of s is not unique. The hope is that among all possible representations of s there is a very sparse representation, i.e., a representation with few nonzero coefficients. The tradeoff is that we have to search all possible representations of s to find the sparse representations, and then determine whether there is a unique sparsest representation. Following [1] and [2], we will measure the sparsity of a representation s = D by two quantities: the `0 and the `1 norm of , respectively (the `0 -norm simply counts the number of nonzero entries of a vector). This leads to the following two minimization problems to determine the sparsest representation of s: minimize and minimize
It turns out that the optimization problem (2) is much easier to handle than (1) through the use of linear programming (LP), so it is important to know the relationship between the solution(s) of (1) and (2), and to determine sufficient conditions for the two problems to have the same unique solution. This problem has been studied in detail in [1] and later has been refined in [2] in the special case where the dictionary D is the union of two orthonormal bases. In what follows, we generalize the results of [1] and [2] to arbitrary dictionaries.1 The case where D is the union of L 2 orthonormal bases for H is studied in detail. This leads to a natural generalization of the recent results from [2] valid for L = 2. In Section II, we provide conditions for a solution of the problem
k k0 k k1
subject to s = D subject to s = D :
(1) (2)
Sparse Representations in Unions of Bases
Rémi Gribonval, Member, IEEE, and Morten Nielsen
Abstract—The purpose of this correspondence is to generalize a result by Donoho and Huo and Elad and Bruckstein on sparse representations of signals in a union of two orthonormal bases for . We consider general (redundant) dictionaries for , and derive sufficient conditions for having unique sparse representations of signals in such dictionaries. The special case 2 orthonormal bases for where the dictionary is given by the union of isstudiedinmoredetail.Inparticular,itisprovedthattheresultofDonoho and Huo, concerning the replacement of the optimization problem with a linear programming problem when searching for sparse representations, has an analog for dictionaries that may be highly redundant. Index Terms—Dictionaries, Grassmannian frames, linear programming, mutually incoherent bases, nonlinear approximation, sparse representations.
3320
IEEE TRANSACTIONS ON INFORMATION THEORY, VOL. 49, NO. 12, DECEMBER 2003
rates taken so high that further increasing them produced no visible changes in the figure. As can be seen, the a;b obtained in that way turns from a well-behaved function for the values b = 1:5, 2:5 into a quite irregularly behaved one when b approaches 2 or 3. REFERENCES
[1] I. Daubechies, Ten Lectures on Wavelets. Philadelphia, PA: SIAM, 1992. [2] K. Gröchenig, Foundations of Time-Frequency Analysis. Boston, MA: Birkhäuser, 2001. [3] A. Ron and Z. Shen, “Weyl-Heisenberg frames and Riesz bases in L (R ),” Duke Math. J., vol. 89, no. 2, pp. 237–282, 1997. [4] A. J. E. M. Janssen, “Zak transforms with few zeros and the tie,” in Advances in Gabor Analysis, H. G. Feichtinger and T. Strohmer, Eds. Boston, MA: Birkhäuser, 2003, pp. 31–70. [5] Y. I. Lyubarski˘ i, “Frames in the Bargmann space of entire functions,” in Entire and Subharmonic Functions. Providence, RI: Amer. Math. Soc., 1992, pp. 167–180. [6] K. Seip, K. Seip, and R. Wallstén, “Density theorems for sampling and interpolation in the Bargmann-Fock space I; II,” J. Reine Angew. Math., vol. 429, pp. 91–106, 1992. [7] A. J. E. M. Janssen and T. Strohmer, “Hyperbolic secants yield Gabor frames,” Appl. Comput. Harmon. Anal., vol. 12, pp. 259–267, 2002. [8] A. J. E. M. Janssen, “On generating tight Gabor frames at critical density,” J. Fourier Anal. Appl., vol. 9, no. 2, pp. 175–214, 2003. [9] H. G. Feichtinger and N. Kaiblinger, “Varying the time-frequency lattice of Gabor frames,” Trans. Amer. Math. Soc., to be published. [10] A. Cavaretta, W. Dahmen, and C. A. Micchelli, “Stationary Subdivision,” Mem. Amer. Math. Soc., vol. 53, no. 453, pp. 1–186, 1991. [11] A. J. E. M. Janssen, “The duality condition for Weyl-Heisenberg frames,” in Gabor Analysis and Algorithms, H. G. Feichtinger and T. Strohmer, Eds. Boston, MA: Birkhäuser, 1998, pp. 33–84. , “From continuous to discrete Weyl-Heisenberg frames through [12] sampling,” J. Fourier Anal. Appl., vol. 3, pp. 583–597, 1997. [13] N. Kaiblinger, “Approximation of the Fourier transform and the Gabor dual function from samples,” preprint, 2003.