A vhdl-based approach for power estimation of embedded systems
极大似然估计法在高压断路器可靠性分析中的应用_马世骁

收稿日期:2006-09-26.基金项目:辽宁省博士后基金资助项目(BSH -2004921038);辽宁省博士启动基金资助项目(20041020).作者简介:马世骁(1963-),男,辽宁沈阳人,教授,博士,主要从事并行工程方面的研究.文章编号:1000-1646(2007)01-0040-03极大似然估计法在高压断路器可靠性分析中的应用马世骁1,2a ,牟 瑞3,卢建伟2b ,林 莘2a(1.沈阳建筑大学管理学院,沈阳110168;2.沈阳工业大学a.电气工程学院,b.理学院,沈阳110023;3.辽宁省科学技术基金服务中心,沈阳110004)摘 要:为了提出一种在非完全寿命试验条件下判别高压断路器可靠性的方法,为进一步开展相关研究提供理论依据,促进高压电器行业的发展,结合高压断路器寿命分布实例,对目前有关高压断路器可靠性研究的现状进行分析,提出了建立高压断路器寿命可靠性判别模型的基本方法,并应用数学方法对其进行分析和判别.通过对断路器寿命的均值和方差进行估计,并应用拟牛顿算法对其求解,判断出高压断路器首次故障时间.通过实例分析、模型的建立和数学计算,提出的适用于寿命周期较长的高压断路器寿命可靠性的判定方法及计算结果,对评价高压断路器可靠性等技术参数具有指导意义,对于研究如何判断高压断路器可靠性有一定的理论价值和实际意义.关 键 词:高压断路器;可靠性;极大似然函数;点估计;拟牛顿算法中图分类号:TP 202.1 文献标识码:AApplication of maxi 2likelihood estimation method in analysis of high voltage circuit breakerMA Shi 2xiao 1,2a ,MU Rui 3,L U Jian 2wei 2b ,L IN Xin 2a(1.School of Management Engineering ,Shenyang Jianzhu University ,Shenyang 110168,China ;2a.School of Electrical Engineering ,2b.School of Science ,Shenyang University of Technology ,Shenyang 110023,China ;3.Liaoning Scientific Technical Fund Service Center ,Shenyang 110004,China )Abstract :In order to put forward a method to judge the reliability of high voltage circuit breaker (HVCB )under the non 2complete life span trial conditions ,and to provide some theoretical evidence for further relevant research and development of high voltage electric appliance ,the present situations of the research on the reliability of HVCB has been analyzed.And the basic method to judge the reliability of the models of the life span for HVCB has been proposed ,analyzed and judged using the mathematical approach.Through estimating the mean value and variance of the life span ,and applying the quasi 2Newton algorithm ,the first breakdown time of HVCB can be judged.By analyzing the demonstrated example ,establishing the model and calculating ,the judgement method for the reliability of HVCB with long life span is presented ,which is instructive to assess the technical parameter such as the reliability of HVCB and which has theoretical and practical meaning to research how to judge the reliability of HVCB.K ey w ords :HVCB ;reliability ;maxi 2likelihood function ;point 2estimation ;quasi 2Newton algorithm 近年来,随着高压电器制造商和电力部门对高压电器可靠性认识的提高,高压电器的可靠性研究逐步得到人们的重视.但是由于高压电器可靠性研究要涉及很多领域(如高压电器试验测试、机械、数学、力学等),而且要解决很多不同领域的交叉问题,所以人们至今对它研究很少.可以说,目前高压电器的可靠性研究在高压电器研究领域几乎是一个空白,能否填补这一空白,直接关系着第29卷第1期2007年2月沈 阳 工 业 大 学 学 报Journal of Shenyang University of TechnologyVol 129No 11Feb.2007高压电器乃至整个电力系统的未来[1-2].虽然许多高压电器制造厂家越来越重视提高高压电器的可靠性,可是由于没有理论做指导,他们也很难在生产实际中真正提高产品的可靠性.如果能够建立一套高压电器可靠性理论体系,也必将会给我国高压电器制造商提供一套在节省成本的基础上提高高压电器可靠性的方法,促进我国高压电器行业的发展[3-4].1 高压断路器寿命分布案例分析本文的研究对象是目前一种被国内广泛应用的户内真空断路器,本文将对整机系统及一些重要零部件的可靠性数据进行分析.这种型号的真空断路器从研制开发、投入运行到目前为止已经有7年时间,总体可靠性较高.由于多年来对这种断路器可靠性的极大关注[5],本文从最初投入运行的这种断路器中随机选择了10000台,对其运行可靠性进行了统计.统计的结果是这10000台断路器从投入运行开始到现在为止,仅有12台出现故障,如表1所示.表1给出这12台故障断路器的首次故障时间及其原因,将这12台故障断路器进行编号,依次为1~12.从表1中可以看到,这12台的故障都出在弹簧机构上,灭弧室故障率为0,其中3台储能电机的换向器接触不良,2台二次线路板接触不良,1台计数器拉杆断裂,两台微动开关烧损,1台油缓冲器漏油,2台合闸线圈烧损,1台绝缘拉杆的轴销断裂.2 可靠性模型的建立评价产品可靠性水平高低的最具说服力的一个可靠性数量指标就是产品的寿命,因此,对产品寿命的研究一直是可靠性工程中一项极为重要的基础性研究工作.从概率论与数理统计的角度来说,对寿命这一可靠性指标的描述是应该建立在对其均值和方差的准确描述基础上的,应该通过对寿命均值和方差的研究来分析产品的寿命情况.表1给出的是该种断路器从最早投入运行到现在大约7年时间里,10000台断路器中仅发生故障12台(其余各台仍然可靠运行)的可靠性数据,而且发生故障的这12台断路器经过维修后,又立即投入运行,始终保持被统计的断路器总台数为10000台,所以,从可靠性试验的角度来说,这相当于可靠性寿命试验中的有替换定时截尾寿命试验,即当一个产品失效后立即进行维修后继续受试,或用同型号产品接替受试,试验中保持被试产品总数不变.当进行到事先规定的时间时停止试验,观察试验中失效的产品个数,同时,判定该种断路器的寿命是服从正态分布.因此,本文将以正态分布下的有替换定时截尾寿命试验模型作为该种断路器寿命的可靠性模型.正态分布下的有替换定时截尾寿命试验模型是指在产品寿命服从正态分布以及试验中采取有替换的条件下,若共有n个试品进行寿命试验,当试验到预先确定的试验时间t s时终止,此时观察共有λ个失效,其失效时间为t1≤t2≤…≤tλ≤t s,而另外(n-λ)个试品将在(ts,∞)内失效,用这些截尾子样数据估计失效分布中的未知特征参数[6].表1 断路器首次故障时间及故障原因T ab.1 First breakdow n time and failure reason ofcircuit breaker断路器编号首次故障时间/年故障原因1 4.7储能电机2 3.8储能电机3 5.4储能电机4 3.8二次线路5 5.0二次线路6 4.8计数器拉杆7 3.6微动开关8 5.1微动开关9 6.0油缓冲器10 4.5合闸线圈11 4.5合闸线圈12 6.3绝缘拉杆轴销断裂3 极大似然估计法的应用根据上述建立的模型,本文将概率论中的极大似然估计法应用于高压断路器寿命可靠性的判定中并采用拟牛顿算法求解.该方法是利用总体X的分布函数F(X;θ)(其中X为随机变量,θ为待估参数)及子样x i(i=1,2,…,n)的统计结果建立极大似然函数,然后运用数值分析方法求解极大似然函数取得极大值时所对应参数的值,这个(些)参数的值就是所要得到的可靠性参数值[7-8].14第1期马世骁,等:极大似然估计法在高压断路器可靠性分析中的应用 311 极大似然函数对于正态分布下的有替换定时截尾寿命试验模型,其对数极大似然函数为ln L(λ)=ln n!(n-λ)!-λln2πσ2-12∑λi=1(t i-μ)2σ2+(n-λ)ln1-∫t s012πσ2ex p-(t-μ)22σ2d t(1)式中:L(λ)———极大似然函数;n———被试断路器总台数,这里n=10000台;λ———失效断路器台数,这里λ=12台;t i———第i台断路器的失效时间,如表1所示;t s———事先确定的试验截止时间,本文t s=7年;μ,σ2———断路器寿命的均值和方差,它们就是所要计算的可靠性参数.由概率论可知,能够使得对数极大似然函数(1)取得极大值的μ,σ2,就是所要求得的可靠性参数μ和σ2的点估计值.本文应用拟牛顿算法求解式(1)的极大值,优化函数为min f(μ,σ)=ln L(λ)(2) 3.2 μ,σ2的计算μ,σ2由下述算法给出:步骤1 给定初始点x(1)∈R2,矩阵H(1)∈R2×2对称正定,计算精度ε>0,k=1;步骤2 计算 f(x(1)),令p(k)=-H(k) f(x(k));步骤3 计算步长αk,使f(x(1)+αk p(k))=minα≥0f(x(k)+αp(k));步骤4 令x(k+1)=x(k)+αk p(k),计算 f(x(k+1));步骤5 若‖ f(x(k+1))‖≤ε,则x3=x(k+1)为所求的解,终止计算,否则转步骤6;步骤6 令s(k)=x(k+1)-x(k),q(k)= f(x(k+1))-f(x(k)),H(k+1)=H(k)+I-q(k)(s(k))T(q(k))T s(k)H(k)I-s(k)(q(k))T(q(k))T s(k)+q(k)(q(k))T(q(k))T s(k),k=k+1,转到步骤2.其中,x=μσ2,H(1)为二阶单位矩阵.利用该算法求得最优化问题(2)的最优解为μ=7187,σ2=2155.对于断路器这种可维修设备而言,其寿命就是指其首次故障时间,因此本文的计算结果又表明该种真空断路器首次故障时间的均值约为7187年,方差为2155年.这就给人们提示了一个很重要的问题:该种真空断路器在投入运行后的第8年左右可能会出现首次故障,因此,应该在第8年对该断路器安排一次预防性维修,以提高其运行可靠性.4 结 论1)给出了一种在非完全寿命试验下对断路器寿命可靠性的判别方法,这种方法对寿命周期较长的产品是尤为适用的.2)研究的对象是一种目前在我国用量很大的户内真空断路器,通过对其寿命的可靠性分析,得出了具有实际应用价值的结论:该种型号真空断路器的首次故障时间大约在8年左右,因此,应该在第8年左右对该种型号的真空断路器安排一次预防性检修,以消除故障隐患,提高其运行可靠性.3)为进一步提高产品的可靠性,提示该产品生产厂家应对断路器在第8年左右发生的故障给以极大的关注,并尽可能在设计过程中消除这些故障.4)应用极大似然估计法对断路器可靠性进行判别,结果准确可靠,对评价断路器运行可靠性具有一定的理论价值.参考文献:[1]林莘.现代高压电器技术[M].北京:机械工业出版社,2002.(L IN Xin.Modern high voltage apparatus technology[J].Beijing:China Machine Press,2002.)[2]李光辉,张培铭.智能化电器可靠性技术[J].低压电器,2000(1):12-15.(L I Guang2hui,ZHAN G Pei2ming.Intelligent electricalapparatus reliability technique[J].Low Voltage Electri2cal Apparatus,2000(1):12-15.)[3]苑舜.高压开关设备状态监测与诊断技术[M].北京:机械工业出版社,2001.(Y UAN Shun.C ondition m onitoring and diagnosis technol2ogy of high v oltage apparatus[M].Beijing:Mechanical In2dustry Publishing C ompany,2001.)[4]卢昆祥.电子产品可靠性管理[M].天津:天津科学技术出版社,1987.(LU Kun2xiang.Reliability administration of electronicproduct[M].Tianjin:Tianjin Science and TechnologyPress,1987.)[5]石飞,林莘,徐建源,等.真空断路器中关键零件机械可靠性计算与分析[J].高压电器,2005,41(1):32-35.(SHI Fei,L IN Xin,XU Jian2yuan,et al.Mechanical re2liability calculation and analysis for key mechanical com2ponents in vacuum circuit breaker[J].High VoltageApparatus,2005,41(1):32-35.)(下转第47页) 24 沈 阳 工 业 大 学 学 报第29卷Trans,IA,1992,28(1):72-80.[7]黄俊,王兆安.电力电子变流技术:3版[M].北京:机械工业出版社,1997.(HUAN G J un,WAN G Zhao2an.Power electronics con2 verting technique[M].Beijing:Mechanic Industry Press,1997.)[8]王振红.数字电路设计与应用实践教程:下篇[M].北京:机械工业出版社,2003.(WAN G Zhen2hong.Design and appliance of digital cir2 cuit[M].Beijing:Mechanic Industry Press,2003.)(责任编辑:邓美艳 英文审校:刘晓明)(上接第34页)参考文献:[1]申永良,李维芬.纯铜及其铜合金[J].冶金丛刊,1998(6):21-23.(SHEN Y ong2liang,L I Wei2fen.Copper and its alloy [J].Metallurgical Collections,1998(6):21-23.) [2]徐增华.金属耐蚀材料[J].腐蚀与防护,2001,22(10):458-460.(XU Zeng2hua.Metallic corrosion2resistant materials [J].Corrosion&Protection,2001,22(10):458-460.)[3]王建国,曹福辛.BFe102121白铜和双相不锈钢在海水管路中应用的综合性能分析[J].材料开发与应用, 2001,16(3):1-5.(WAN G Jian2guo,CAO Fu2xin.The analysis of the comprehensive properties of BFe102121Cu2Ni alloy and dual phase stainless steel used in sea water pipeline sys2 tem[J].Development and Application of Materials, 2001,16(3):1-5.)[4]中国机械工程学会焊接学会.焊接手册(2)[M].北京:机械工业出版社,1996.(China Welding Institute.Welding handbook(2)[M].Beijing:China Machine Press,1996.)[5]王宗杰,臧汝恒,李德元.工程材料焊接技术问答[M].北京:机械工业出版社,2002.(WAN G Z ong2jie,ZAN G Ru2heng,L I De2yuan.Basic problem about the welding technology of engineering materials[M].Beijing:China Machine Press,2002.) [6]季杰,井绪贵,张文钺.铜渗透裂纹机理[J].焊接学报,2004,25(3):124-128.(J I Jie,J IN G Xu2gui,ZHAN G Wen2yue.Mechanism of infiltrated cracking[J].Transactions of China Welding Institute,2004,25(3):124-128.)[7]刘殿宝,高峰,刘放.厚壁大口径BFe102121铁白铜管焊接试验[J].焊接,2002(3):30-32.(L IU Dian2bao,G AO Feng,L IU Fang.Welding proce2 dure of BFe102121copper2Nickel alloy thick2wall major diameter pipe[J].Welding Journal,2002(3):30232.)[8]周振丰.焊接冶金学[M].北京:机械工业出版社,1996.(ZHOU Zhen2feng.Welding metallurgy[M].Beijing: China Machine Press,1996.)(责任编辑:王艳香 英文审校:陈立佳)(上接第42页)[6]翟国富,王淑娟,姜守旭,等.电器可靠性失效分析中极大似然估计方法的研究[J].中国电机工程学报, 2001,21(3):90-92.(ZHAI Guo2fu,WAN G Shu2juan,J IAN G Shou2xu,et al.The research on method of maximum likelihood esti2 mation for apparatus reliability failure analysis[J].Pro2 ceedings of the CSEE,2001,21(3):90-92.)[7]龙永红.概率论与数理统计[M].北京:高等教育出版社,2000.(LON G Y ong2hong.Probability and numerical statistics [M].Beijing:Advanced Education Press,2000.)[8]聂高辉.参数θ的函数f(θ)的极大似然估计[J].大学数学,2005,21(5):87-90.(N IE G ao2hui.Maximum likelihood estimation of func2 tions of parameters[J].College Math,2005,21(5): 87-90.)(责任编辑:邓美艳 英文审校:刘晓明)74第1期厉 伟,等:便携式工频绝缘试验系统研究开发 。
Synopsys RTL Architect
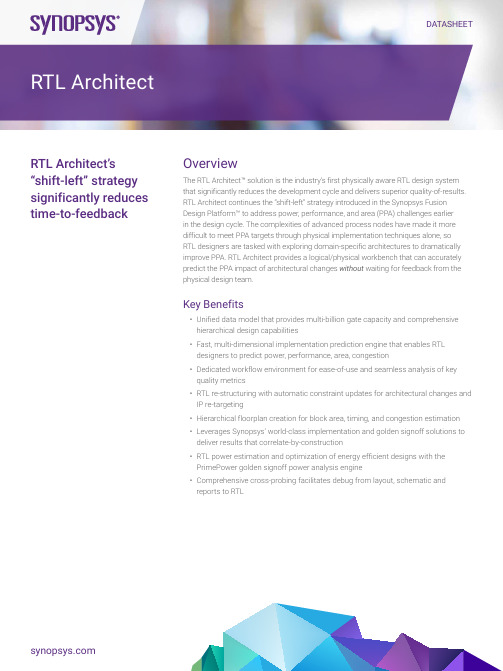
DATASHEET OverviewThe RTL Architect™ solution is the industry’s first physically aware RTL design system that significantly reduces the development cycle and delivers superior quality-of-results. RTL Architect continues the “shift-left” strategy introduced in the Synopsys Fusion Design Platform™ to address power, performance, and area (PPA) challenges earlierin the design cycle. The complexities of advanced process nodes have made it more difficult to meet PPA targets through physical implementation techniques alone, so RTL designers are tasked with exploring domain-specific architectures to dramatically improve PPA. RTL Architect provides a logical/physical workbench that can accurately predict the PPA impact of architectural changes without waiting for feedback from the physical design team.Key Benefits• Unified data model that provides multi-billion gate capacity and comprehensive hierarchical design capabilities• Fast, multi-dimensional implementation prediction engine that enables RTLdesigners to predict power, performance, area, congestion• Dedicated workflow environment for ease-of-use and seamless analysis of key quality metrics• RTL re-structuring with automatic constraint updates for architectural changes and IP re-targeting• Hierarchical floorplan creation for block area, timing, and congestion estimation • Leverages Synopsys’ world-class implementation and golden signoff solutions to deliver results that correlate-by-construction• RTL power estimation and optimization of energy efficient designs with thePrimePower golden signoff power analysis engine• Comprehensive cross-probing facilitates debug from layout, schematic andreports to RTLRTL Architect’s “shift-left” strategy significantly reduces time-to-feedback RTL Architect2Predictive ModelingRTL Architect’s new Predictive Engine (PE) is derived from Synopsys’ implementation environment and enables rapid multi-dimensional analysis and optimization of RTL to predict PPA of downstream implementation accurately. This Predictive Engine utilizes new correct-by-construction modeling, leveraging the proven and widely used core implementation algorithms and architectures of the Synopsys Fusion Design Platform. This ensures tight correlation to the best implementation.This also allows the RTL designers to experiment and tune their HDL code without multiple, back-and-forth, hand-offs to synthesis and to pinpoint timing bottlenecks in their source code to improve RTL quality.Design PlanningRTL Architect’s hierarchical, design planning, infrastructure automatically generates a physical implementation, with clock trees, to provide the RTL designer with accurate power, timing and area estimates. Additionally, the RTL block integrator can use the design planning capabilities to integrate in-house and third-party IP (as seen in Figure 1 Arteris ® IP FlexNoC ® Interconnect Integration) including bus and pipeline register planning. This fast and deep look-ahead allows the designers to not only predict but also drivephysical implementation.Figure 1: Arteris IP FlexNoC Interconnect IntegrationRTL Design ExperienceThe RTL Architect experience is built around the RTL designer. The PE maps leaf cells back to the RTL so that the designer can see the direct impact a code change has on PPA. Figure 2 RTL Cross-Probing, shows the cross-probing capability from various design views to RTL. Color coded reports indicate severity level.Layout viewRTL Architect Unified GUI EnvironmentRTL view view viewFigure 2: RTL Cross-Probing©2020 Synopsys, Inc. All rights reserved. Synopsys is a trademark of Synopsys, Inc. in the United States and other countries. A list of Synopsys trademarks isavailable at /copyright .html . All other names mentioned herein are trademarks or registered trademarks of their respective owners.02/28/20.CS469885535_RTL Architect_DS.For example, the designer can see how the logic is physically implemented by cross-probing from a report directly to the layout. This is useful for seeing the predicted congestion hotspots caused by RTL, so they can experiment with different architectures to reduce the congestion. Figure 3 shows the high degree of correlation between the place and route engines of RTL Architect and Fusion Compiler™.Fusion CompilerRTL Architect Figure 3: RTL Architect vs. Fusion Compiler CongestionAnother key concern for RTL designers is power usage. The interactive power summary report provides an overview of key power metrics, such as, switching and glitch power, leakage, and clock gating efficiency. The results are based on the PrimePower golden signoff power analysis engine. The report data can be sorted, filtered and cross-probed to RTL.Bridging the GapRTL Architect significantly improves the quality of RTL before handing off to implementation. It addresses the limitations of the existing solutions which are hampered by inaccuracies that impact productivity as downstream implementation tools compensate. The shift-left strategy identifies and corrects physical implementation issues early in the design cycle to achieve aggressive PPA targets at advanced nodes through better RTL.。
信号奇异性计算方法在电噪声信号中的应用_赵健 (1)
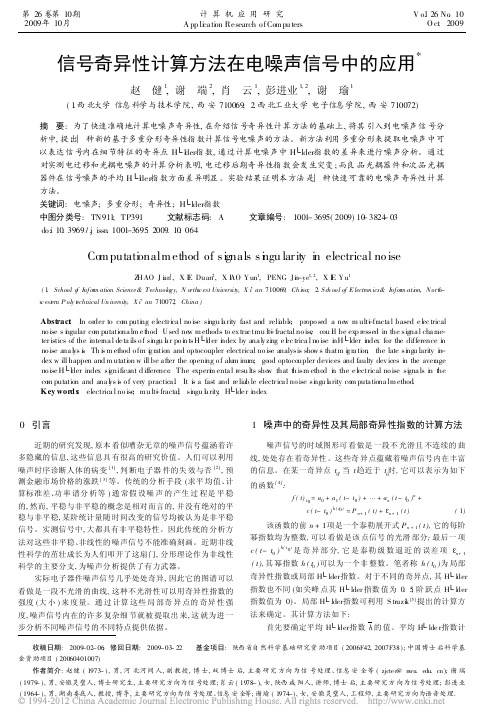
的典型仿真信号, 它们 的 HÊ lder指 数 h 的值 分别 为 - 0. 5, 0,
0. 5。其中 h 的值为 - 0. 5和 0. 5的 信号就 是通常所 说的白 噪 声和分数布朗运动信号。这 三种信 号可以 利用傅里 叶滤波 法
产生 [ 7] 。对这 三种信 号的 局部 奇异 值进 行计 算, 最后 将各 个
-
h (x0, sl ) = { log( Wf ( sl, t0 ) ) - [ h log(N ) + c] } /
[ log( sl ) - log(N ) ]
( 6)
式 ( 6) 类 似 于 两 点 间 的 斜 率 公 式, 其 斜 率 值 即 为 HÊ lder 指
数值。
为了验证算法与程序的准确性, 分别产生三种奇异值确定
指数也不同 (如尖峰 点其 HÊ lder 指数 值为 0. 5, 阶 跃点 HÊ lder
指数值为 0)。局部 HÊ lder指数可利用 S truzik[5] 提出的计算方
法来确定。其计算方法如下:
首先要确定平均 HÊ lder指数 h的 值。平均 HÊ lde r指数计
收稿日期: 2009-02-06; 修回日期: 2009-03- 22 基金项目: 陕西省自然科学基础研究资 助项目 ( 2006F42, 2007F38 ) ; 中国博士后 科学基 金资助项目 ( 20060401007)
实际电子器件噪声信号几乎处处奇异, 因此它的图谱可以 看做是一段不光滑的曲线, 这种不光滑性可以用奇异性指数的 强度 (大 小 ) 来 度量。通 过 计算 这些 局 部奇 异 点的 奇异 性 强 度, 噪声信号内在的许多 复杂细 节就被 提取出 来, 这 就为进 一 步分析不同噪声信号的不同特点提供依据。
激光二极管恒流驱动电源的仿真设计
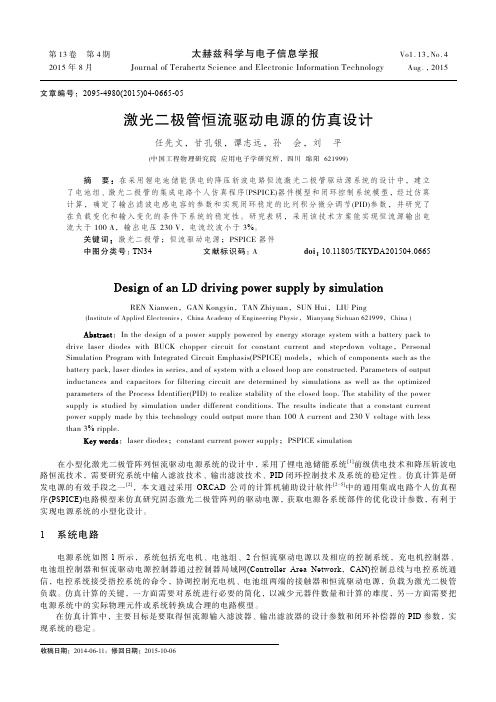
第13卷 第4期 太赫兹科学与电子信息学报Vo1.13,No.4 2015年8月 Journal of Terahertz Science and Electronic Information Technology Aug.,2015 文章编号:2095-4980(2015)04-0665-05激光二极管恒流驱动电源的仿真设计任先文,甘孔银,谭志远,孙 会,刘 平(中国工程物理研究院 应用电子学研究所,四川 绵阳 621999)摘 要:在采用锂电池储能供电的降压斩波电路恒流激光二极管驱动源系统的设计中,建立了电池组、激光二极管的集成电路个人仿真程序(PSPICE)器件模型和闭环控制系统模型,经过仿真计算,确定了输出滤波电感电容的参数和实现闭环稳定的比列积分微分调节(PID)参数,并研究了在负载变化和输入变化的条件下系统的稳定性。
研究表明,采用该技术方案能实现恒流源输出电流大于100A,输出电压230V,电流纹波小于3%。
关键词:激光二极管;恒流驱动电源;PSPICE器件中图分类号:TN34 文献标识码:A doi:10.11805/TKYDA201504.0665Design of an LD driving power supply by simulationREN Xianwen,GAN Kongyin,TAN Zhiyuan,SUN Hui,LIU Ping(Institute of Applied Electronics,China Academy of Engineering Physic,Mianyang Sichuan 621999,China )Abstract:In the design of a power supply powered by energy storage system with a battery pack to drive laser diodes with BUCK chopper circuit for constant current and step-down voltage,PersonalSimulation Program with Integrated Circuit Emphasis(PSPICE) models,which of components such as thebattery pack, laser diodes in series, and of system with a closed loop are constructed. Parameters of outputinductances and capacitors for filtering circuit are determined by simulations as well as the optimizedparameters of the Process Identifier(PID) to realize stability of the closed loop. The stability of the powersupply is studied by simulation under different conditions. The results indicate that a constant currentpower supply made by this technology could output more than 100A current and 230V voltage with lessthan 3% ripple.Key words:laser diodes;constant current power supply;PSPICE simulation在小型化激光二极管阵列恒流驱动电源系统的设计中,采用了锂电池储能系统[1]前级供电技术和降压斩波电路恒流技术,需要研究系统中输入滤波技术、输出滤波技术、PID闭环控制技术及系统的稳定性。
State of charge estimation of lithium-ion batteries using the open-circuit
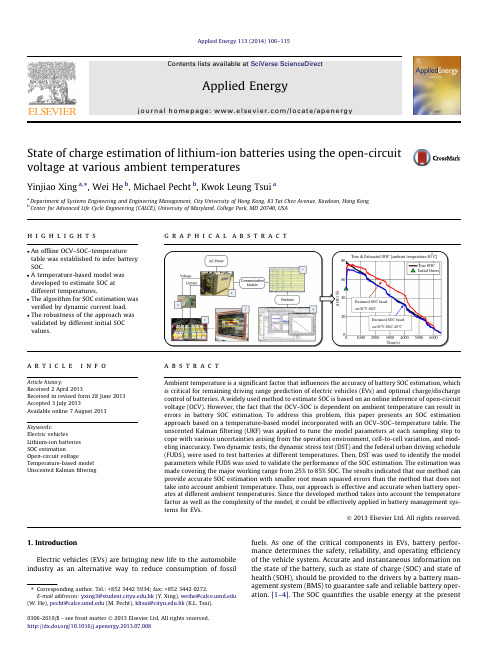
State of charge estimation of lithium-ion batteries using the open-circuit voltage at various ambienttemperaturesYinjiao Xing a ,⇑,Wei He b ,Michael Pecht b ,Kwok Leung Tsui aa Department of Systems Engineering and Engineering Management,City University of Hong Kong,83Tat Chee Avenue,Kowloon,Hong Kong bCenter for Advanced Life Cycle Engineering (CALCE),University of Maryland,College Park,MD 20740,USAh i g h l i g h t sg r a p h i c a l a b s t r a c t100020003000400050006000020406080Time(s)S O C (%)True & Estimated SOC [ambient temperature:40oC]True SOC Initial GuessEstimated SOC based on OCV-SOCEstimated SOC based on OCV-SOC-40°Ca r t i c l e i n f o Article history:Received 2April 2013Received in revised form 28June 2013Accepted 3July 2013Available online 7August 2013Keywords:Electric vehiclesLithium-ion batteries SOC estimationOpen-circuit voltageTemperature-based model Unscented Kalman filteringa b s t r a c tAmbient temperature is a significant factor that influences the accuracy of battery SOC estimation,which is critical for remaining driving range prediction of electric vehicles (EVs)and optimal charge/discharge control of batteries.A widely used method to estimate SOC is based on an online inference of open-circuit voltage (OCV).However,the fact that the OCV–SOC is dependent on ambient temperature can result in errors in battery SOC estimation.To address this problem,this paper presents an SOC estimation approach based on a temperature-based model incorporated with an OCV–SOC–temperature table.The unscented Kalman filtering (UKF)was applied to tune the model parameters at each sampling step to cope with various uncertainties arising from the operation environment,cell-to-cell variation,and mod-eling inaccuracy.Two dynamic tests,the dynamic stress test (DST)and the federal urban driving schedule (FUDS),were used to test batteries at different temperatures.Then,DST was used to identify the model parameters while FUDS was used to validate the performance of the SOC estimation.The estimation was made covering the major working range from 25%to 85%SOC.The results indicated that our method can provide accurate SOC estimation with smaller root mean squared errors than the method that does not take into account ambient temperature.Thus,our approach is effective and accurate when battery oper-ates at different ambient temperatures.Since the developed method takes into account the temperature factor as well as the complexity of the model,it could be effectively applied in battery management sys-tems for EVs.Ó2013Elsevier Ltd.All rights reserved.1.IntroductionElectric vehicles (EVs)are bringing new life to the automobile industry as an alternative way to reduce consumption of fossil fuels.As one of the critical components in EVs,battery perfor-mance determines the safety,reliability,and operating efficiency of the vehicle system.Accurate and instantaneous information on the state of the battery,such as state of charge (SOC)and state of health (SOH),should be provided to the drivers by a battery man-agement system (BMS)toguarantee safe and reliable battery oper-ation.[1–4].The SOC quantifies the usable energy at the present0306-2619/$-see front matter Ó2013Elsevier Ltd.All rights reserved./10.1016/j.apenergy.2013.07.008⇑Corresponding author.Tel.:+852********;fax:+852********.E-mail addresses:yxing3@.hk (Y.Xing),weihe@ (W.He),pecht@ (M.Pecht),kltsui@.hk (K.L.Tsui).cycle,while the SOH denotes the remaining performance of the battery over its whole life cycle[5].Battery SOC is a direct and immediate look at the remaining charge of the battery,which re-flects residual range of an EV.This has gained more attention due to drivers’range anxiety i.e.running out of power on the road. Additionally,an accurate SOC is an indicator of how to improve a battery’s operational reliability,extend its lifespan,and optimize the power management of the vehicle[1,2,6].However,SOC can-not be measured directly but must be estimated according to mea-surable parameters such as current and voltage.Moreover, ambient temperature is a critical factor that affects the accuracy of SOC estimation[7–12].There are three main types of methods for SOC estimation:cou-lomb counting,machine learning methods,and their combination using a model-based estimation approach.These three types of methods are described below.Coulomb counting is a straightforward method for estimating SOC that accumulates the net charge at the last time period in units of ampere-hours(Ah).Its performance is highly reliant on the pre-cision of current sensors and the accurate estimation of the initial SOC[3,13].However,coulomb counting is an open-loop estimator that does not eliminate the accumulation of measurement errors and uncertain disturbances.In addition,it is not able to determine the initial SOC,and address the variation of the initial SOC caused by self-discharging.Without the knowledge of the initial SOC,this method will cause accumulating errors on SOC estimation.Taking into account these factors,regular recalibration is recommended and widely used by methods such as fully discharging the battery, or referring to other measurements such as open-circuit voltage (OCV),as suggested in[3,6,7,14].Machine learning approaches,including artificial neural net-works,fuzzy logic–based models,and support vector machines, have been used to estimate SOC online.Li et al.[15]designed a 12-input-2-level merged fuzzy neural network(FNN)that was fused with a reduced-form generic algorithm(RGA)to estimate SOC.Bo et al.[16]developed parallel chaos immune evolutionary programming(PICEP)to train a neural network model in which five input variables were selected.This approach was used to esti-mate the SOC of nickel–metal hydride(Ni/MH)batteries.The per-formance of the kind of black-box models is reliant on the reliability of the training data,i.e.whether it is sufficient to cover the entire loading conditions.Once the battery operated at the un-known loading conditions,the robustness of these models was subject to challenge.Wang[17]employed a support vector ma-chine to model the dynamic behavior of a Ni/MH battery under dy-namic current loading.However,model training is time consuming and requires a large amount of data.Also,the estimation based on this model causes a large prediction error due to the uncertainty of the new data set.A model-basedfiltering estimation approach is being widely ap-plied due to its close-loop nature and concerning various uncer-tainties.Both electrochemical models and equivalent circuit models aim to capture the dynamic behavior of the battery.The former are usually presented in the form of partial differential equations with many unknown parameters.They are accurate but not desirable in practice because of a high requirement for memory and computation.To guarantee the accuracy of the model and the feasibility,equivalent circuit models have been imple-mented in BMSs such as the enhanced self-correcting(ESC)model and the hysteresis model,as found in[10,18,19],and one or two-order resistance–capacitance(RC)network models[1,2,10,11,20]. OCV is a vital element in the above-mentioned battery equivalent models and is a function of SOC in nature.The premise of utilizing OCV–SOC is that the battery needs to rest a long time and terminal voltage approaches the OCV.However,in real life,a long resting time may not be possible.To make up for theflaws of OCV meth-ods,nonlinearfiltering techniques based on state-space models have been developed to enhance SOC estimation through combin-ing coulomb counting and OCV[7].Plett applied extended Kalman filters(EKF)into BMS to implement SOC estimation of a lithium polymer battery(LiPB)using different battery models in [10,21,22].Plett later proposed the use of two sigma-point Kalman filtering(SPKF)estimators,including the unscented Kalmanfilter (UKF)and central difference Kalmanfilter(CDKF),in[18,23].Sub-sequently,adaptive EKF[7,20],dual EKF[11],and adaptive UKF[3] were developed to improve the accuracy of the SOC estimation based on their own sample sets and some common equivalent cir-cuit models.Charkhgard and Farrokhi[13]also proposed the com-bination of NN and EKF to estimate SOC.NN was employed to train a lithium-ion battery model using some charging data from the battery.The effectiveness of this method was not verified under the dynamic discharging data,which would lead to a larger uncer-tainty on estimating SOC.However,several existing issues are seldom addressed in the literature.Firstly,the temperature dependence of the OCV–SOC lookup table is seldom discussed with regard to battery SOC esti-mation.Instead,a single OCV–SOC table constructed at a certain temperature(e.g.,room temperature)is widely employed.It will cause a large error in inferring SOC when the battery is operating at other ambient temperatures(not room temperature) [1,8,10,11].Secondly,lithium-ion batteries have a relativelyflat OCV curve over the SOC,especially for lithium iron phosphate (LiFePO4)batteries,which are widely used in the electric vehicle market[24].That means a small error on the inferred OCV will produce a larger deviation in SOC.Thirdly,different models were adopted by individuals based on their own experimental data. Although a sophisticated model with more parameters might be able to provide a smaller modeling error,it would run the risk of adding more uncertainties,such as over-fitting problems and the introduction of unnecessary noises,especially concerning temperature.Therefore,it makes more sense to investigate a gen-eric but accurate temperature-based model with fewer parame-ters for real-time applications.Kim et al.[25]considered temperature as an input variable into afirst-order RC circuit model.However,the effect of temperature on the OCV–SOC was ignored due to a slight difference between OCV curves from 30%SOC to80%at different temperatures.Moreover,their samples have an obvious linear slope of OCV–SOC that is prone to infer SOC accurately.Nevertheless,for a relativelyflat OCV curve dependent on ambient temperature,it is significant not only to develop an accurate and generic model considering ambient temperature,but also to enhance the capability of online estimation due to the uncertainty,including unit-to-unit varia-tion,measurement noise,operational uncertainties,and model inaccuracy[26].In this paper,a temperature-based internal resistance(R int)bat-tery model combined with a nonlinearfiltering method was put forward to improve the SOC estimation of lithium-ion batteries un-der dynamic loading conditions at different ambient temperatures. The research proceeds as follows.Three tests at different tempera-tures are introduced in Section2.The dynamic stress test(DST)and federal urban driving schedule(FUDS)are two kinds of dynamic loading conditions tested at different temperatures to identify the model parameters and verify the estimated performance, respectively.The purpose of the OCV–SOC–temperature(OCV–SOC–T)test is to extend the OCV–SOC behavior to temperature field.Due to various uncertainties of the system,UKF-based SOC estimator is proposed due to its superiority of reaching to the 3rd order of any nonlinearity over the EKF.The implemented procedure for our battery study is followed by Section3.The experimental results are presented in Section4to compare our developed method based on OCV–SOC–T with the originalY.Xing et al./Applied Energy113(2014)106–115107estimation using a single OCV–SOC table.The robustness is vali-dated and compared under the different initial true values and dif-ferent initial guesses of SOC.2.ExperimentsThe experiment setup is shown in Fig.1.It consisted of (1)lith-ium-ion cells (LiFePO4)of the 18650cylindrical type (the key spec-ifications are shown in Table 1);(2)Vötsch temperature test chamber (The cells were placed in cell holders in the chamber);(3)a current and voltage sampling cable for loading and sampling;(4)a battery test system (Arbin BT2000tester);(5)a PC with Ar-bins’Mits Pro Software (v4.27)for battery charging/discharging control;(6)Matlab R2009b for data analysis.During battery oper-ation,the sampling time of current,voltage was 1s.Three separate test schedules were conducted on the battery test bench for model identification,OCV–SOC–T table construction,and method valida-tion,respectively.2.1.Model identification testFor model identification,the dynamic stress test (DST)was run from 0°C to 50°C at an interval of 10°C.DST is employed to investigate the dynamic electric behavior of the battery.It is designed by US Advanced Battery Consortium (USABC)to simu-late a variable-power discharge regime that represents the expected demands of an EV battery [27].A completed DST cycle is 360s long and can be scaled down to any desired maximum demand regarding the specifications of the test samples.There-fore,in our study,DST was run continuously from 100%SOC at 3.6V to empty at 2V over several cycles in a discharge process.The positive current responds to discharging while the negative denotes charging.The measured current and voltage profile at 20°C is shown in Fig.2.2.2.The OCV–SOC–T testOCV is a function of SOC for the cells.If the cell is able to rest for a long period until the terminal voltage approached the true OCV,OCV can be used to infer SOC accurately.However,this method is not practical for dynamic SOC estimation.To address this issue,the SOC can be estimated by combining the online identification of the OCV with the predetermined offline OCV–SOC lookup table.Taking into account the temperature dependence of the OCV–SOC table,the OCV–SOC test was conducted from 0°C to 50°C at an interval of 10°C.The test procedure at each temperature is the same as fol-lows.Firstly,the cell was fully charged using a constant current of 1C-rate (1C-rate means that a full discharge of the battery takes approximately 1h)until the voltage reached to the cut-off voltage of 3.6V and the current was 0.01C.Secondly,the cell was fully dis-charged at a constant rate of C/20until the voltage reached 2.0V,which corresponds to 0%SOC.Finally,the cell was fully charged at a constant rate of C/20to 3.6V,which corresponds to 100%SOC.The terminal voltage of the cell is considered as a close approximation to the real equilibrium potential [6,10].AsshownFig.1.Schematic of the battery test bench.Table 1The key specifications of the test samples.Type Nominal voltage Nominal capacity Upper/lower cut-off voltage Maximum continuous discharge current LiFePO 43.3V1.1Ah3.6V/2.0V30A010002000300040005000-2024Time (s)C u r r e n t (A )in Fig.3,the equilibrium potential during the charging process is higher than that during discharging process.It accounts for a hys-teresis phenomenon of the OCV during the charging/discharging.In our paper,the OCV curve was defined as the average value of the charge and discharge equilibrium potentials.The effect of the hys-teresis was ignored.In addition,referring to[28],when SOC is normalized relative to the specific cell capacity,the OCV–SOC curve can be referred to as being unique for the same type at the same testing condition.Fig.3shows the average OCV at20°C.A flat OCV slope between25%and80%SOC is emphasized in another small plot in Fig.3.Its effect will be discussed in Section3.1.2.3.Method validation testA validation test with a more sophisticated dynamic current profile,the federal urban driving schedule(FUDS),was conducted to verify the estimation algorithm based on the developed model. FUDS is a dynamic electric vehicle performance test based on a time–velocity profile from an automobile industry standard vehi-cle[27].In the laboratory test,a dynamic current sequence was transferred from the time–velocity profile,programmed to charge or discharge the battery and applied to battery performance test [3,17,20,29].Similar to the DST test,the current sequence is scaled tofit the specification of the test battery and the limitation of the testing system of Fig.1.The current profile of FUDS causes varia-tion of the SOC from fully charged at3.6V to empty at2V.The FUDS test was also run form0°C to50°C at an interval of10°C. The measured current,voltage profile,and the cumulative SOC at 20°C are shown in Fig.4.A completed FUDS current profile over 1372s is emphasized in another graph in Fig.4(a).3.Battery modelingFor lithium-ion batteries,the internal resistance(R int)model is generic and straightforward to characterize a battery’s dynamics with one estimated parameter.Although a sophisticated model with more parameters would possibly show a well-fitting result, such as an equivalent circuit model with several amounts of paral-lel resistance–capacitance(RC)networks,it would also pose a risk of over-fitting and introducing more uncertainties for online esti-mation at the same time.Especially taking into account tempera-ture factor,more complexity should be imposed on battery modeling.Therefore,we would prefer a simple model to a sophis-ticated model if the former had generalization ability and provided sufficiently good results.In this paper,model modification based on the original R int model is proposed to balance the model com-plexity and the accuracy of battery SOC estimation.The schematic of the original R int model is shown in Fig.5.U term;k¼U OCVÀI kÂRð1ÞU OCV/fðSOC kÞð2ÞIn Eqs.(1)and(2),U term,k is the measured terminal voltage of the battery under a normal dynamic current load at time k,and I k is the dynamic current at the same time.The positive current re-sponds to discharging while the negative value means charging.R is the simplified total internal resistance of the battery.U OCV is a function of SOC of the battery that should be tested following the procedure as presented in Section2.2.The battery model Eq.(1) can be used to infer OCV directly according to the measured termi-Schematic of the internal resistance(R int)modelY.Xing et al./Applied Energy113(2014)106–1151093.1.Model parameter identificationThe DST was run on the LiFePO4batteries to identify the model parameter R in Eq.(1).Taking the current and voltage profile of DST at20°C as an example,the voltage and current are measured and recorded from fully charged to empty with a sampling period of1s based on our battery test bench.The accumulative charge(exper-imental SOC)is calculated synchronously from100%SOC.Thus, the parameter R can befitted using a sequence of the current,volt-age,and the offline OCV–SOC by the least square algorithm.In terms of thefitted R value,the model performance can be evalu-ated based on the measured terminal voltage(U term,k)and the esti-mated voltage(U term;k).Fig.6shows the measured and the estimated voltage response on the DST profile at20°C based on the original model.In statistics,the mean absolute error(MAE)and the root mean squared(RMS)error can be used together to evaluate the good-ness-of-fit of the model.These two indicators are given by the fol-lowing equations respectively.MAE¼1X nk¼1j e k jð3ÞRMS error¼ffiffiffiffiffiffiffiffiffiffiffiffiffiffiffiffiffiffiffiffiffiffiffiffiffiffiffi1nX nk¼1ðe kÞ2rð4ÞHere,e k is the modeling error(U term,kÀU term;k)at time k.The MAE measures how close forecasts are to the corresponding out-comes without considering the direction.The RMS error is more sensitive to large errors than the MAE.It is able to characterize the variation in errors.The statistics list of the model is shown in Table2.According to the small graph in Fig.3,the OCV slope,namely, dOCV/dSOC is approximately equal to0.0014between25%and 80%SOC.This means that the deviation on the OCV inference will cause an estimated deviation of SOC up to21%when there is a MAE of0.0288V based on the current model.Additionally,a large mean error was plotted over time in Fig.6.Thus,the residuals should be reduced to improve the model adequacy with smaller MAE and RMS error values.3.2.Model improvement and validation3.2.1.The OCV–SOC–T table for model improvementAccording to the test in Section2.2,six OCV curves were ob-tained from0°C to50°C at an interval of10°C.Fig.7(a)empha-sizes the differences of OCV–SOC curves between30%and80% SOC at different temperatures.It can be seen that SOC0°C is much larger than other SOC values at higher temperatures when the OCV inference is the same,i.e.,3.3V.It makes sense that the releas-able capability of the charge is reduced at low temperatures. Fig.7(b)shows the SOC values if the OCV inference was equal to the specific values from3.28V to3.32V at intervals of0.01V at three temperatures:0°C,20°C,40°C.One issue of interest can be seen in Fig.7(b).That is,the same OCV inference at different temperatures corresponds to different SOC values.For example,the SOC difference between0°C and 40°C reaches approximately22%at an OCV of3.30V.Therefore, we propose adding the OCV–SOC–T to the battery model to im-prove the model accuracy.The improved battery model is as follows:U term;k¼U OCVðSOC k;TÞÀI kÂRðTÞþCðTÞð5Þwhere U OCV is a function of SOC and ambient temperature(T).C(T)is a function of temperature that facilitates the reduction of the offset due to model inaccuracy and environmental conditions.Fig.8 shows the measured and the estimated voltage response on the DST profile at20°C based on the proposed model.It can be found that the mean error of the new model is reduced with small varia-tions as compared to the original model in Fig.6.Another issue of interest in Fig.7(b)is that a small deviation of 0.01V in OCV inference will lead to a large difference in SOC at the same temperature condition.It is the same issue as shown in Fig.3. Therefore,if the SOC estimation were directly inferred from a bat-tery model,it would have a high requirement on the model and measurement accuracy.To address this issue and improve the accuracy of the SOC estimation,the model-based unscented Kal-manfiltering approach was employed and introduced in Section4.3.2.2.The validation of the proposed modelBased on the developed model in Eq.(5),it is noted that the spe-cific OCV–SOC look-up table should be selected in terms of the ambient temperature(here it is viewed as an average value).Least squarefitting was also used to identify model parameters,R and C. Thefitted model parameter list and the statistics list of the pro-posed model are shown in Table3.In comparison to thefitted results at20°C of Table2,here the MAE is one order of magnitude smaller than that of the original model and the RMS modeling error is also reduced.In addition, the correlation coefficient(Corrcoef)was calculated for residual analysis.The Corrcoef(e k,I k)values close to zero indicate that the residuals and the input variable hardly have linear relationship. Thus,the corrected model can be betterfitted on the dynamic cur-rent load.Onefinding of interest is C values that can befitted over the ambient temperature(T)using a regression curve,as Fig.9 shows.Referring to the paper[30],the exponential function can be selected tofit C values over T because the internal elements of the battery,i.e.,battery resistance follow the Arrhenius equation, which has exponential dependency on the temperature.In our study,five C values at0°C,10°C,20°C,25°C,30°C,40°C wereTable2Model parameter and statistics list of thefitted error of the model.R(X)Mean absolute error RMS modeling error0.24450.0288V0.0301110Y.Xing et al./Applied Energy113(2014)106–115used for curvefitting while C(50°C)was used to test thefitted per-formance of this exponential function.The95%prediction bounds are shown in Fig.9based on C values andfitted curve.Apparently, C(50°C)drops within the95%prediction bounds.It can be seen that the function of C(T)in Fig.9can be used to estimate C when the corresponding temperature test has not been run.4.Algorithm implementation for online estimationThe online SOC estimation has strong nonlinearity.This point can be seen from any battery model in which OCV has a nonlinear relationship with SOC.Additionally,the uncertainties due to the model inaccuracy,measurement noise,and operating conditions will cause a large variation in the estimation.The model-based nonlinearfiltering approach has been developed to implement dy-namic SOC estimation.The objective is to estimate the hidden sys-tem state,estimate the model parameters for system identification, or both.Thus,an error-feedback-based unscented Kalmanfiltering approach is proposed by shifting the system noise to improve the accuracy of the estimation.4.1.Unscented KalmanfilteringThe extended Kalmanfiltering(EKF)technique has become a popular technique for addressing the issue of state or parameter estimation for nonlinear systems.The rationale behind EKF is still the KF approach based on state space modeling.It aims to utilize the error between the current measurement and the model output to adjust the model state by virtue of a Kalman gain.Its principle and implementation can be found in[31].Since KF is only available for linear systems,extended Kalmanfiltering(EKF)used a lineari-zation process at each time step to approximate a nonlinear system through thefirst-order Taylor series expansion[31,32].However, thefirst order approximation will probably lead to large errors inTable3Fitted model parameter list and statistics list of modelfitting.T(°C)R(X)C Mean absoluteerrors(V)RMSmodelingerrorsCorrcoef(e k,I k)00.2780À0.05520.01530.0188 1.36eÀ13100.2396À0.04360.01120.01348.45eÀ14200.2249À0.03600.00870.0105 1.09eÀ13250.2020À0.03260.00800.0095 1.02eÀ13300.1838À0.02890.00730.0085À7.62eÀ13400.1565À0.02370.00600.0071 2.85eÀ13500.1816À0.02010.00990.0131 3.15eÀ14Y.Xing et al./Applied Energy113(2014)106–115111the true posterior mean and covariance of the noise and could even result in divergence of thefilter.Under this situation,unscented Kalmanfiltering(UKF)based on unscented transformation was suggested to avoid the weakness that comes from using Taylor ser-ies expansion.The core idea of UKF is easier to approximate the state distribu-tion that is represented by a minimal set of chosen sample points called sigma points,which can capture the mean and covariance of Gaussian random variable when propagated through a nonlinear system.The state-space model of a nonlinear system is represented as follows:x k¼fðx kÀ1;u kÀ1Þþw kÀ1yk¼hðx k;u kÞþm kð6Þwhere x k is the system state vector and y k is the measurement vec-tor at time k.Correspondingly,f(Á)and h(Á)are the state function and the measurement function,respectively;u k is the known input vector;w k$Nð0;PwÞis the Gaussian process noise;and v k$Nð0;PVÞis the Gaussian measurement noise.Assume the state x has mean x and covariance P ing the unscented transformation (UT),the state will be transformed as a matrix of2n+1sigma vec-tors v i with corresponding weights w i.These sigma points are shown in the following equation:vkÀ1¼½ xð0ÞkÀ1; xð1:nÞkÀ1; xðnþ1:2nÞkÀ1¼½ x kÀ1; x kÀ1þffiffiffiffiffiffiffiffiffiffiffiffiffiffiffiffiffiffiffiffiffiffiffiffiffiðnþkÞP kÀ1p; x kÀ1ÀffiffiffiffiffiffiffiffiffiffiffiffiffiffiffiffiffiffiffiffiffiffiffiffiffiðnþkÞP kÀ1pð7Þwhere n is the dimension of the state and k is a scaling parameter. These sigma points are propagated through a nonlinear function,re-estimated,and then they are used to capture the posterior mean and covariance to the3rd Taylor expansion,as shown in Eqs.(9) and(10).yi¼hðv iÞ;i¼0;...;2nð8Þ y%X2ni¼0wðiÞmyið9ÞP y%X2ni¼0wðiÞcf y iÀ y gf y iÀ yg Tð10Þwhere wðiÞm and wðiÞc are the weights of the corresponding sigma points that can be calculated in[33–35]for details.4.2.SOC estimation based on proposed methodIn our battery study,the state vector is x=[SOC,R]T.Thefirst state equation in Eq.(11)follows the coulomb counting method mentioned in Section1.Peukert effect and capacity aging could be partially compensated when introducing the process noise x1,kÀ1.A random walk is applied to the model parameter R regard-ing the cell-to-cell variation and operation uncertainties.Tuning the R will also be able to compensate for the variation of the C in our proposed model.The terminal voltage of the battery is the measured vector y=U term,that is,the proposed battery model as shown in Eq.(5).State function:SOC k¼SOC kÀ1ÀI kÀ1ÂD t=C nþx1;kÀ1R k¼R kÀ1þx2;kÀ1ð11ÞMeasurement function:U term;k¼U OCVðSOC k;TÞÀI kÂR kðTÞþCðTÞþm kð12Þwhere I k is the current as the input(u k in Eq.(6))at time k;D t is the sampling interval,which is1s according to the sampling rate;and C n is the rated capacity.The rated capacity of the test samples is1.1 Ah.And x1,k,x2,k and m k are zero-mean white stochastic processes with covariancesPx1,Px2andPm,respectively.According to the proposed state-space model in Eqs.(11)and(12),the procedure of SOC estimation based on UKF is summarized in Table4.Table4Summary of the UKF approach for SOC estimation.Initialize:–Measure ambient temperature,prepare U OCV(SOC,T)and R0,C0–Initial guess:S0,–Covariance matrix:P o–Process and measurement noise covariance:Pw0;PVGenerate sigma points at time kÀ1,(k2½l;...;1 ):vkÀ1¼S kÀ1R kÀ1¼½ v kÀ1; v kÀ1þffiffiffiffiffiffiffiffiffiffiffiffiffiffiffiffiffiffiffiffiffiffiffiffiffiðnþkÞP kÀ1p; v kÀ1ÀffiffiffiffiffiffiffiffiffiffiffiffiffiffiffiffiffiffiffiffiffiffiffiffiffiðnþkÞP kÀ1pPredict the prior state mean and covariance–Calculate sigma points through state function:v ik j kÀ1¼S i k j kÀ1R ik j kÀ1"#¼S i kÀ1ÀI kÀ1ÂD tC nR i kÀ1"#;i¼1; (2)–Calculate the prior mean and covariance:^xÀk ¼P2ni¼0w imv ik j kÀ1;PÀk¼P2ni¼0w ichv ik j kÀ1À^xÀkihv ik j kÀ1À^xÀki TþPwUpdate using the measurement function–Calculate sigma points y k j kÀ1¼U OCVðS k j kÀ1;TÞÀI kÂRðTÞk j kÀ1þCðTÞ–Calculate the propagated mean:^yÀk ¼P2ni¼0w i m y ik j kÀ1–Calculate the covariance of the measurement:P yÀk ;yÀk¼P2ni¼0w j c½y ik j kÀ1À^yÀkTþPm–Calculate the cross-covariance and the state and measurement:of battery SOC estimation by UKF112Y.Xing et al./Applied Energy113(2014)106–115。
Silicon Labs Wireless Gecko设备功耗测量说明书
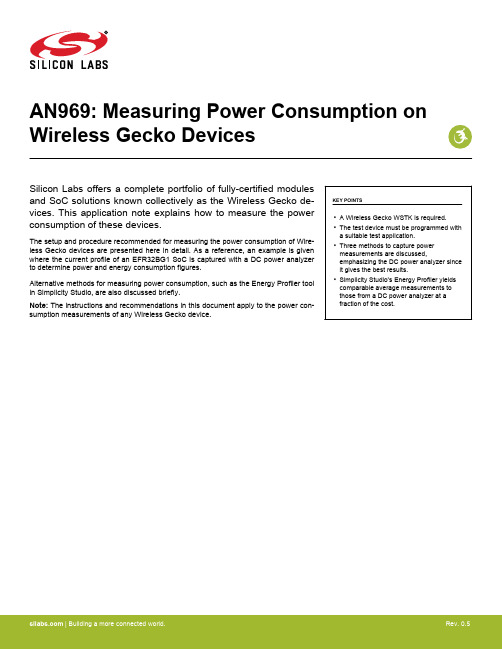
AN969: Measuring Power Consumption on Wireless Gecko DevicesSilicon Labs offers a complete portfolio of fully-certified modulesand SoC solutions known collectively as the Wireless Gecko de-vices. This application note explains how to measure the power consumption of these devices.The setup and procedure recommended for measuring the power consumption of Wire-less Gecko devices are presented here in detail. As a reference, an example is given where the current profile of an EFR32BG1 SoC is captured with a DC power analyzer to determine power and energy consumption figures.Alternative methods for measuring power consumption, such as the Energy Profiler tool in Simplicity Studio, are also discussed briefly.Note: The instructions and recommendations in this document apply to the power con-sumption measurements of any Wireless Gecko device.KEY POINTS•A Wireless Gecko WSTK is required.•The test device must be programmed with a suitable test application.•Three methods to capture power measurements are discussed, emphasizing the DC power analyzer since it gives the best results.•Simplicity Studio’s Energy Profiler yields comparable average measurements to those from a DC power analyzer at a fraction of the cost.Documentation 1. DocumentationThe documents listed below (sorted by topic) may be helpful during the evaluation. You may download them directly from after registering and creating a user account, or you may navigate through Simplicity Studio's documentation interface to find them and then download them.Hardware•The applicable Wirelesss Starter Kit User's Guide•The applicable radio board reference manualSoftware and Tools•AN0822: Simplicity Studio User Guide (for Simplicity Studio 4)•Simplicity Studio 5 User’s Guide, available at https// and through the Simplicity Studio 5 help menu•UG343: Multi-Node Energy Profiler User’s Guide•AN0948: Power Configurations and DC-DC•AN1246: EFR32BG SoC Bluetooth® Smart Device Power Consumption Measurements2. SetupTo evaluate power consumption you will need the following:1.Obtain a Wireless Starter Kit and radio board.2.Build and install a suitable test application.3.Configure the WSTK as described in section 2.3 Configuring the WSTK Main Board.2.1 Wireless Starter Kit OverviewAs seen in the figure below, the Wireless Gecko Wireless Starter Kit consists of three components:•The Wireless Starter Kit (WSTK) main board•The Radio Board•The Expansion Board (included in WSTKs for modules only, not SoCs)Figure 2.1. Wireless Gecko Wireless Starter KitThe actual Wireless Gecko device is contained in the radio board. Therefore, the radio board will vary depending on the Wireless Gecko device you want to work with (module or SoC). The figures below show the radio boards for a BGM111 module and then for an EFR32BG1 SoC.Figure 2.2. Module Radio BoardFigure 2.3. SoC Radio BoardFor power measurements, only the WSTK and the radio board are necessary. See the applicable reference manual for details about the WSTK.2.2 Building and Loading a Test ApplicationIf you have not already done so, install Simplicity Studio and the relevant SDK. Each SDK includes several software examples to create application projects. Follow the directions in the applicable SDK Quick Start Guide to install Simplicity Studio, and build and flash the example project to the device.2.3 Configuring the WSTK Main BoardThe WSTK main board supports three options for delivering power to the radio board: (1) through USB, (2) from a coin-cell battery, and (3) from an external power supply.For cleaner power measurements, an external supply is the better choice because powering through USB enables other circuitry on the WSTK main board that may, in some cases, lead to slightly higher or noisier current readings. When powering from a coin-cell battery, the battery’s series resistance or its limited current-sourcing capability after aging may lead to current consumption profiles with a pro-nounced exponential rise or decay response that could mask the real power consumption of the device under test (DUT).To power the Wireless Gecko device from an external source, change the power switch of the WSTK main board to BAT and apply 3.3 V (or your expected main supply voltage) at the nodes highlighted in the figure below (soldering a two-pin header there may be useful). The silkscreen on the WSTK board shows the polarity of the nodes.Figure 2.4. WSTK Main Board Powering Options3. ProcedureSince the current profile of Wireless Gecko devices varies rapidly and dynamically over several orders of magnitude while switching between different active and sleep modes, measuring the power consumption of these devices accurately is non-trivial.This section introduces one of the best instruments available for observing the power consumption of Wireless Gecko devices: the Key-sight N6705B DC power analyzer. Moreover, it will show you how to configure it both to power a Wireless Gecko device and to meas-ure its current consumption.For cases where a DC power analyzer is not accessible, see 4. Alternative Methods.3.1 N6705B DC Power Analyzer OverviewThe N6705B DC Power Analyzer integrates some of the capabilities offered by a power supply, a multi-meter, a scope, and a data logger all in one box. While its chassis supports up to four DC power modules, only one is needed for basic measurements, such as those discussed here. To learn more about the N6705B, consult the manufacturer’s website. The figure below shows its front panel.Figure 3.1. N6705B DC Power Analyzer3.2 Configuring the DC Power AnalyzerTo configure the N6705B as an external supply to power a Wireless Gecko device, perform the following steps:1.Power the instrument and turn it on.2.Under “Select Output” in the front panel, choose the DC module to be used as source (default is “1”).3.Under “Source” go to Settings, and enter the configuration in the following figure.Note: Note that the following figure shows 4-wire sense, as is also shown in the figure in section 3.3 Running a Test. 4-wire sense can be used in situations where high current is expected in order to maximize accuracy. Before enabling 4-wire sensing, connect the +Sense and -Sense terminals to the same measurement points as the +Output and -Output terminal connections, as shown in section 3.3 Running a Test. For most situations where high current is not expected, 2-wire sense can be used. Compare between 4-wire and 2-wire measurement set-ups to determine which configuration is appropriate for your use case.4.Close the window.Figure 3.2. DC Power Analyzer Source SettingsThe primary advantage of the DC power analyzer is that it can measure the current drawn by the load connected to it, like a regular multi-meter, by sampling it in time like a scope with very good resolution (up to 256k samples per capture). To configure the analyzer as both a meter and a scope, perform the following steps:1.Under “Select Output” in the front panel, choose the DC module you are using as source.2.Go to “Properties”, and enter the configuration seen in the following figure as the meter properties.Figure 3.3. DC Power Analyzer Meter Settings3.Close the window.4.Under “Select Output” in the front panel, choose the DC module being used as the source.5.Under “Measure”, select "Scope View".6.Go to “Properties”, and enter the configuration in the following figure as the Scope Properties.Figure 3.4. DC Power Analyzer Scope Settings7.Close the window.3.3 Running a TestAt this point, your testbench should resemble the figure below. The radio board with the Wireless Gecko device running a test applica-tion should be mounted on the WSTK main board. The WSTK main board should be powered through its external supply nodes. The power switch on the main board should be set to BAT. Finally, the DC analyzer should both supply 3.3 V (or your expected main supply voltage) to the WSTK main board and measure its current consumption.Note: The coin cell battery should be removed from the WSTK main board battery connector when using the BAT + and – pins from external supply.To start a test and view the current consumption of the DUT, perform the following steps:1.Under “Select Output” in the N6705B front panel, choose the DC module you are using as source.2.Under “Measure”, select Scope View.3.Under “Power Supply Outputs” press the "On" button to enable the DC module you are using as source. At this point, the devicewill reset, power up, and then start functioning.4.Press the "Run/Stop" button on the N6705B front panel to start monitoring the current consumed by the device as it functions.e the knobs under “Waveform Display” to adjust the x and y axis scaling of the signal measured for a better view of the currentprofiles captured.Figure 3.5. Testbench for 4-Wire Sensing Power Consumption MeasurementsThe following figure shows the current profile captured for a Series 1 EFR32xG radio board running an SoC-iBeacon example applica-tion, as described in detail in AN1246: EFR32BG SoC Bluetooth® Smart Device Power Consumption Measurements. On the left, the large periodic bursts correspond to the current consumed by the SoC while broadcasting a beacon every 100 ms. The flat parts be-tween the bursts correspond to the current consumed while in sleep mode. The spikes observed while in sleep mode correspond to the periodic dumping of charge on the load capacitor of the DC-DC converter to refresh its output voltage. See AN0948.1/AN0948.2: Power Configurations and DC-DC for more details about DC-DC converter operation and power configurations for Series 1 and Series 2 devi-ces, respectively.On the right, a zoomed-in view of one of the large bursts shows in more detail the timing and magnitude of the TX and RX events taking place while broadcasting the beacon (and listening for a response) over the three channel frequencies assigned for advertising in the Bluetooth LE protocol.Figure 3.6. EFR32BG Radio Board Current Consumption Profile Example4. Alternative MethodsMeasuring the power consumption of Wireless Gecko devices with a DC Power Analyzer is ideal but not always feasible. For this rea-son, this section provides a brief overview of two alternative methods for making power measurements. For cost-constrained develop-ment environments, a combination of the two methods described in this section (Energy Profiler and Voltage Sampling with a scope) can be a good alternative to a high-end instrument, such as the N6705B.4.1 Energy ProfilerThe Energy Profiler in Simplicity Studio is a software tool that works together with the Advanced Energy Monitoring (AEM) circuitry built into the WSTK main board to allow measuring, plotting, and optimizing in real time the current and energy consumption of your test device. To learn more about AEM, see the WSTK's Reference Manual. For more details on the Energy Profiler, refer to to UG343: Mul-ti-Node Energy Profiler User’s Guide.Assuming that the Wireless Gecko device is already programmed with the desired application, you can measure its power consumption with the Energy Profiler by performing the following steps:1.Connect the WSTK main board to your computer via USB.2.Set the power switch on the WSTK main board to AEM.3.Open Simplicity Studio.4.Select the WSTK "J-Link Silicon Labs" in the Debug Adapters view.5.Click the Tools icon ('wrench'), select Energy Profiler, and click [OK].6.Click [Quick Access] under Current Device, and select Start Energy Capture.7.Select the WSTK "J-Link Silicon Labs" and click [OK].8.Play with the Y and X axis scaling and scrolling options to adjust the display or9.Click the green "Running" button to stop capture (label changes to "Paused").The following three figures show Energy Profiler screenshots of the curves and measurements obtained for a Series 2 EFR32xG22 SoC radio board running the SoC-iBeacon application mentioned in section 3.3 Running a Test. Specifically, the first figure shows plots of the current profile captured (two beacon broadcast events) and the DUT’s average current on a logarithmic scale. The second figure highlights the average current, average power and total energy consumption measured for the DUT while in sleep mode (cursors can be enabled in the Energy Profiler by left-clicking and dragging on the plot), also on a logarithmic scale. Finally, the third figure shows a zoomed-in view of one of the beacon broadcasts and its corresponding current, power, and energy measured in linear scale.Figure 4.1. Current Profile and Supply Voltage for a Series 2 EFR32BG22 SoC Running the SoC-iBeacon ApplicationFigure 4.2. Measured Current/Power/Energy in Sleep ModeFigure 4.3. Measured Current/Power/Energy in Active ModeNote: To display the plot of the measured supply, click the [Voltage] control on the top-right area of the Energy Profiler window. Also, for a better view of low-magnitude current measurements, change the Y-axis scaling from linear to logarithmic by clicking the control with a curve on the bottom-left area of the plot.The Energy Profiler offers certain advantages over a DC power analyzer including lower cost, portability, and real-time energy debug-ging and optimization capabilities. However, its “scope” capabilities and measurement accuracy (1 μA) are limited by the 10 kHz maxi-mum sampling rate of the AEM circuitry on the WSTK. Because of this, the resolution of the plots displayed is insufficient to see every transition in the current profile of a test device in detail. This may be inconvenient in some cases but, most importantly, the overall aver-age measurements provided by the Energy Profiler closely approximate those for a DC power analyzer.4.2 Voltage Sampling Across ResistorAnother method for measuring power consumption is illustrated in the diagram below. By placing a low-value resistor in series with the supply node to the DUT and using an oscilloscope to sample the voltage difference across the resistor while the DUT is in operation, the current and energy consumption of the DUT can be estimated.Figure 4.4. Testbench Setup for Power Measuring MethodWith a typical two channel scope, the current profile can be plotted as a math function defined by (CH2-CH1)/R while triggering with the falling edge of CH2.The main advantage of this approach is that measurements can be collected quickly with relatively easy-to-access lab instruments. Al-so, unlike the Energy Profiler, this approach allows you to observe every transition in the current profile of the test device easily since the sampling resolution of most modern scopes is relatively high. Some important drawbacks, however, include that measurement ac-curacy for very low signal levels (for example, during sleep) can be poor due to the thermal noise added by the series resistor at the supply node and to any offsets in your setup while taking a differential measurement.Document Revision History 5. Document Revision HistoryRevision 0.5•Remove reference to AN713: Measuring EM35x Power Consumption for Sleepy End Devices using EmberZNet.•Update Simplicity Studio instructions and references so they apply to both v4 and v5.Revision 0.4•Move Bluetooth i-Beacon testing on Series 1 EFR32BG devices to the new AN1246: EFR32BG SoC Bluetooth® Smart Device Pow-er Consumption Measurements.•Generalize the content in this document so that it applies to all EFR32xG devices.Smart. Connected. Energy-Friendly.Products /productsQuality/qualitySupport and CommunitySilicon Laboratories Inc.400 West Cesar ChavezAustin, TX 78701USADisclaimerSilicon Labs intends to provide customers with the latest, accurate, and in-depth documentation of all peripherals and modules available for system and software implementers using or intending to use the Silicon Labs products. Characterization data, available modules and peripherals, memory sizes and memory addresses refer to each specific device, and "Typical" parameters provided can and do vary in different applications. Application examples described herein are for illustrative purposes only. Silicon Labs reserves the right to make changes without further notice to the product information, specifications, and descriptions herein, and does not give warranties as to the accuracy or completeness of the included information. Without prior notification, Silicon Labs may update product firmware during the manufacturing process for security or reliability reasons. Such changes will not alter the specifications or the performance of the product. Silicon Labs shall have no liability for the consequences of use of the information supplied in this document. This document does not imply or expressly grant any license to design or fabricate any integrated circuits. The products are not designed or authorized to be used within any FDA Class III devices, applications for which FDA premarket approval is required, or Life Support Systems without the specific written consent of Silicon Labs. A "Life Support System" is any product or system intended to support or sustain life and/or health, which, if it fails, can be reasonably expected to result in significant personal injury or death. Silicon Labs products are not designed or authorized for military applications. Silicon Labs products shall under no circumstances be used in weapons of mass destruction including (but not limited to) nuclear, biological or chemical weapons, or missiles capable of delivering such weapons. Silicon Labs disclaims all express and implied warranties and shall not be responsible or liable for any injuries or damages related to use of a Silicon Labs product in such unauthorized applications.Trademark InformationSilicon Laboratories Inc.®, Silicon Laboratories®, Silicon Labs®, SiLabs® and the Silicon Labs logo®, Bluegiga®, Bluegiga Logo®, Clock B uilder®, CMEMS®, DSPLL®, EFM®, EFM32®, EFR, Ember®, Energy Micro, Energy Micro logo and combinations thereof, "the world’s most energy friendly microcontrollers", Ember®, EZLink®, EZRadio®, EZRadioPRO®, Gecko®, Gecko OS, Gecko OS Studio, ISOmodem®, Precision32®, ProSLIC®, Simplicity Studio®, SiPHY®, Telegesis, the Telegesis Logo®, USBXpress® , Zentri, the Zentri logo and Zentri DMS, Z-Wave®, and others are trademarks or registered trademarks of Silicon Labs. ARM, CORTEX, Cortex-M3 and THUMB are trademarks or registered trademarks of ARM Holdings. Keil is a registered trademark of ARM Limited. Wi-Fi is a registered trademark of the Wi-Fi Alliance. All other products or brand names mentioned herein are trademarks of their respective holders.。
电池储能系统SOC神经网络融合估计方法

第50 卷第 10 期2023年10 月Vol.50,No.10Oct. 2023湖南大学学报(自然科学版)Journal of Hunan University(Natural Sciences)电池储能系统SOC神经网络融合估计方法孙玉树1,2,李宏川3,王波3,贾东强3,裴玮1,2,唐西胜1,2†(1.中国科学院电工研究所,北京 100190;2.中国科学院大学,北京 100049;3.国网北京市电力公司,北京 100031)摘要:为了更好地获取电池储能系统当前的运行状态,提出了基于神经网络融合的电池储能系统SOC估计方法.首先,对比分析了前馈(BP)、门控循环单元(GRU)和长短时记忆(LSTM)神经网络算法的优劣,BP计算时间较短,LSTM对时序数据估计精度较高;然后,利用KL散度、皮尔逊相关系数和灰色关联度分析了不同输入参量和SOC的相关程度,并和LSTM估计结果相比对,筛选出对电池储能系统SOC影响较大的特征参量;最后,应用经验模态分解算法将SOC数据分解为多个分量,利用样本熵将分量聚合为高低两个频段,进而应用BP、LSTM神经网络算法分频段估计,和单一策略相比,该方法在提高SOC估计精度的同时,减少了计算时间.关键词:电池储能系统;SOC融合估计;相关性分析;经验模态分解;样本熵中图分类号:TM 614 文献标志码:ASOC Neural Network Fusion Estimation Method for BatteryEnergy Storage SystemSUN Yushu1,2,LI Hongchuan3,WANG Bo3,JIA Dongqiang3,PEI Wei1,2,TANG Xisheng1,2†(1.Institute of Electrical Engineering, Chinese Academy of Sciences, Beijing 100190, China;2.University of Chinese Academy of Sciences, Beijing 100049, China;3.State Grid Beijing Electric Power Company, Beijing 100031, China)Abstract:To better obtain the current operating state of the battery energy storage system,a state of charge (SOC) evaluation method of the battery energy storage system based on neural network fusion is proposed. First, the advantages and disadvantages of back-propagation (BP),gated recurrent unit (GRU),and long and short-term memory (LSTM)neural network algorithms are compared. The calculation time of BP is usually short,while the estimation accuracy of LSTM for temporal data is high. Then the correlation degree between different input parameters and SOC is analyzed by KL divergence,Pearson correlation coefficient,and grey correlation degree. Compared with the LSTM estimation results, the characteristic parameters that have a greater impact on the SOC of the battery energy storage system are selected. Finally,the empirical mode decomposition algorithm is applied to∗收稿日期:2023-03-15基金项目:国家重点研发计划项目(2021YFB2402002),National Key Research and Development Program of China (2021YFB2402002);中国科学院青年创新促进会项目(2023000018),Youth Innovation Promotion Association of Chinese Academy of Sciences (2023000018)作者简介:孙玉树(1987—),男,北京人,中国科学院电工研究所副研究员,博士† 通信联系人,E-mail:*************文章编号:1674-2974(2023)10-0031-10DOI:10.16339/ki.hdxbzkb.2023235湖南大学学报(自然科学版)2023 年decompose the SOC data into multiple components, and the sample entropy is used to aggregate the components into high and low-frequency bands. BP and LSTM neural network algorithms are used to estimate SOC in different frequency bands. Compared with a single strategy, the proposed method not only improves the estimation accuracy of SOC, but also reduces the calculation time.Key words:battery energy storage system;SOC fusion estimation;correlation analysis;empirical mode decom⁃position;sample entropy截至2022年底,中国已投运的电力储能累计装机达59.4 GW,同比增长37%.其中,新型储能累计装机规模首次突破 10 GW,超过2021年同期的2倍,达到12.7 GW.电池储能作为重要的灵活性调节资源,占据新型储能的主导地位,在提高新能源消纳、提升可靠供电等方面具有积极作用,是构建新型电力系统不可或缺的组成部分[1].在电池市场规模日益扩大的背景下,荷电状态(State of Charge,SOC)估计等相关研究越来越得到人们的重视.如果未对电池储能系统SOC进行精准估计,可能会导致起火、爆炸等事故的发生,危及储能电站甚至电力系统的安全稳定运行.因此,高精度、快速实时的SOC估计是保障电池运行安全、延长使用寿命的核心技术,对电池更大规模的应用,特别是电站级电池储能系统安全经济运维,具有重要的实用价值[2-3].在电池实际应用中,其表现出非线性、时变性、影响因素复杂性和不确定性等特征,造成了SOC估计难度大、精度不高和适应能力不足,由此产生了较多SOC估计算法及其改进策略.1)基于经验的估计算法:罗勇等[4]提出的带容量修正的安时积分法,可用于SOC的实时估计或作为评价其他SOC估计策略的基准. Xing等[5]将锂电池进行长时间静置,测量其开路电压和SOC,拟合出两者之间的函数关系,实现对锂电池SOC估计.上述开环估计算法原理简单、容易实现且计算复杂度低,但常需要满足许多前提条件,且因为缺少反馈环节,算法鲁棒性较差.2)基于模型的估计算法:Liu等[6]基于电化学单粒子模型,设计了终端电压反馈注入非线性观测器来监测锂离子电池的SOC.张宵洋等[7]提出了基于分数阶模型自适应扩展卡尔曼粒子滤波的SOC估计方法. Fu等[8]建立了Thevenin模型,采用线性卡尔曼滤波器进行参数在线辨识.杜常清等[9]基于戴维南电池模型,提出一种卡尔曼滤波与扩展卡尔曼滤波相结合的算法估算SOC. Bai等[10]考虑到温度变化对锂离子电池荷电状态估计的影响,提出了一种基于自适应双扩展卡尔曼滤波的荷电状态估计方法.上述电化学模型虽能较好地表征电池内外部特性,但其辨识较为复杂;等效电路模型结构清晰,参数易于辨识,但模型精度与复杂度难以兼顾,无法反映电化学微观过程.3)基于数据的估计算法:王语园等[11]提出了基于最小二乘支持向量机机器学习的锂离子电池SOC 估计模型. Fan等[12]提出了一种基于U-Net架构卷积神经网络的SOC估计方法,该方法可以处理变长输入数据和输出等长SOC估计结果. Gong等[13]提出了一种基于深度学习的新型深度神经网络模型,该模型以10 s采样率的电池电压、电流和温度组成的数据单元为输入,SOC估算值为输出. Chen等[14]提出了一种基于扩展输入和约束输出的长短期记忆和循环神经网络用于电池SOC估计.上述基于数据驱动的SOC估计方法仅依靠系统输入与输出间的映射关系即可建立SOC估计模型,极大地简化了电池建模过程,但需要大量数据集进行训练来建立各变量之间的关系,精度依赖训练数据集质量,且计算量大. 4)模型数据混合估计算法:单独使用上述3类方法可能会影响SOC估计精度或估计速率,因此,多类方法融合估计方法也得到了快速发展,通过优势互补取得更为准确的SOC估算结果.杨帆等[15]基于二阶Thevenin等效模型,提出一种将无迹卡尔曼滤波与BP神经网络相结合的SOC估计方法. Cui等[16]提出了一种基于改进的双向门控循环单元网络和无迹卡尔曼滤波的混合方法来实现不同温度下电池SOC的实时稳定估计.上述研究主要侧重电池单体的SOC研究,对系统级的分析较少,电池储能系统较电池单体数据量大,运行影响因素众多,数据在线获取难度大,SOC32第 10 期孙玉树等:电池储能系统SOC 神经网络融合估计方法估计的映射关系更为复杂等,因此本文应用数据驱动的方式进行电池储能系统的SOC 估计.首先,对比分析了不同神经网络对电池储能系统SOC 估计的结果;进而利用不同的相关性评价指标筛选对电池储能系统SOC 估计影响较大的特征因素;最后,利用经验模态分解和样本熵对电池储能系统进行分频处理,并利用不同的神经网络对不同频段数据进行估计,从而提高电池储能系统SOC 估计的精度和速度.1 长短时记忆神经网络由于递归神经网络在训练过程中容易发生梯度爆炸或消失,长短时记忆(Long Short-Term Memory ,LSTM )神经网络[17]应运而生,其优势是在网络中引入遗忘门,和传统递归神经网络相比,其更擅长处理长时间尺度历史信息.所添加的遗忘门,会选择性地遗忘和记忆某些历史信息,当其输出值接近0,则说明某些历史信息被选择忘记;当输出值接近1,则倾向于保留记忆更多的历史信息.故,LSTM 避免了部分信息的简单重复覆盖,能够有效解决递归神经网络容易梯度爆炸或消失的问题.LSTM 网络结构如 图1所示.f t =σ(w f ⋅[h t -1, x t ]+b f )(1)ìíîi t =σ(w i ⋅[h t -1,x t ]+b i )c t =tanh(w c ⋅[h t -1,x t ]+b c )(2)c t =f t ⋅c t -1+i t ⋅c t σ(3)ìíîo t =σ(w o ⋅[h t -1,x t ]+b o )h t =o t ⋅tanh(c t )(4)式中:式(1)为遗忘门的更新;式(2)为输入门的更新;式(3)为状态门的更新;式(4)为输出门的更新;x t 为输入数据,如温度、电压、电流等;f t 为遗忘门输出;w 、b 为各层神经元的权系数,表示输入层输出;c t 为卷积层输出;o t 为输出层输出;h t 为最终输出数据. 2 电池储能系统SOC 估计为了分析不同因素对电池储能系统SOC 的影响,选取国内某储能电站系统的总电压、电流、绝缘电阻、平均电压、平均温度和累计充电电量6个参量进行SOC 状态估计(如图2~图7所示),采样间隔为1 min ,时长为5 760 min.为了更好地利用神经网络算法对SOC 进行估计,需对样本数据进行预处理,以防止较大的梯度更新.本文采用离差标准化,将输入和输出数据进行线性变化,使其取值在[0,1]之间.d =s -min (s )max (s )-min (s )(5)式中:s 为某一输入样本;max (s )为输入样本的最大值;min (s )为输入样本的最小值;d 为归一化后数据值.为了评价电池储能系统SOC 的估计精度,采用均方根误差(Root-Mean-Square Error ,RMSE )进行表述,计算公式如下所示.图1 LSTM 神经网络结构Fig. 1 LSTM neural network structure图2 总电压Fig. 2 Total voltage图3 电流Fig. 3 Current33湖南大学学报(自然科学版)2023 年R MSE=(6)2.1 不同神经网络算法对比为了分析不同神经网络算法对估计结果的影响,利用BP 神经网络、GRU 神经网络和LSTM 神经网络对电池储能系统SOC 进行估计,采用前80%作为训练数据,后20%作为测试数据.电池储能系统的SOC 曲线如图8所示,利用6个输入参数估计的BP结果如图9所示,GRU 结果如图10所示,LSTM 结果如图11所示.表1给出了3种算法的RMSE 和计算时间,可以看出,LSTM 的RMSE 最小,为0.040 2;而BP 计算时间最短,仅需要2.12 s ;GRU 的RMSE 和计算时间均处于中间值.因此,基于BP 和LSTM 各自的优势,本文以两者的融合方法进行电池储能系统SOC 的估计.2.2 SOC 估计影响因素为了分析不同因素对电池储能系统SOC 估计的影响程度,本节分析总电压、电流、绝缘电阻、平均电压、平均温度和累计充电电量6个指标与SOC的相图4 绝缘电阻Fig. 4 Insulation resistance图5 平均电压Fig. 5 Average voltage图6 平均温度Fig. 6 Average temperature图8 电池储能系统SOCFig. 8 Battery energy storage system SOC图9 BP 神经网络估计结果Fig. 9 BP neural network estimation results图7 累计充电电量Fig. 7 Cumulative charge quantity34第 10 期孙玉树等:电池储能系统SOC 神经网络融合估计方法关性.为了增强对比性,采用KL 散度、皮尔逊相关系数和灰色关联度进行输入指标与SOC 的相关性分析.2.2.1 KL 散度KL (Kullback-Leibler )散度[18],也称相对熵,能够度量2个过程概率分布的差别.设p (x )、q (x )表示2个过程的概率,则KL 距离为:δ(p ,q )=∑x ∈Np ()x logp ()xq ()x (7)进而p (x )、q (x )之间的KL 散度为:D (p ,q )=δ(p ,q )+δ(q ,p)(8)KL 散度是对2个过程概率分布夹角的度量,数值越大,说明差别越大;反之,越小;当2类概率分布完全相同时,数值为0.当求X ={x 1,x 2,⋯,x n }和Y ={y 1,y 2,⋯,y n }间的KL 散度时,假定概率分布分别为p (x )、q (x ).首先,计算信号X 的概率分布,本文采用非参数估计法求解概率分布:p (x )=1nh ∑i =1n K éëêêùûúúx i -x h,x ∈R(9)式中:p (x )为核密度估计后的概率密度函数;h 为给定的正数,称为窗宽或平滑参数;K (·)为核函数,常用高斯核函数.K (u )=12πe -u 2/2(10)同理,可以得到Y 的概率分布q (x ).将p (x )、q (x )代入式(7)求解X 和Y 的KL 距离(p ,q )和(q ,p ),进一步利用式(8)计算出KL 散度值D (p ,q ).利用KL 散度分析不同输入(总电压、电流、绝缘电阻、平均电压、平均温度、累计充电电量)和输出SOC 的关联程度,如表2所示.总电压的KL 散度值最小,平均电压的KL 散度值最大,即总电压和SOC 的关联程度最高,平均电压和SOC 的关联程度最低,关联度重要性从高到低排序依次为总电压->电流->累计充电电量->绝缘电阻->平均温度->平均电压.2.2.2 皮尔逊相关系数皮尔逊相关系数[19]广泛用于度量两个变量之间的线性相关程度γ=∑(X -Xˉ)(Y -Y ˉ)∑(X -Xˉ)2∑(Y -Y ˉ)2(11)式中:X ˉ、Y ˉ分别为变量X 、Y 的期望值.其中-1≤γ≤1.其性质如下:当γ>0时,表示两变量正相关;γ<0时,两变量为负相关;当γ=0时,表示两变量无线性相关关系.当|γ|=1时,表示两变量为完全线性相关,即为函数关系;当0<|γ|<1时,表示两变量存在一定程度的线性相关.且|γ|越接近1,两变量线性关系越密切;|γ|越接近0,表示两变量的线性相关越弱.图10 GRU 神经网络估计结果Fig. 10 GRU neural network estimation results图11 LSTM 神经网络估计结果Fig. 11 LSTM neural network estimation results表1 不同算法的估计结果Tab. 1 Estimation results of different algorithms算法BPGRU LSTMRMSE0.168 50.047 00.040 2计算时间/s 2.12184.66373.75表2 不同输入参数和SOC 的KL 散度Tab. 2 KL divergence for different inputparameters and SOC参数KL散度总电压0.444 5电流0.894 5绝缘电阻4.183 4平均电压1.047 6e+03平均温度18.362 6累计充电电量1.956 535湖南大学学报(自然科学版)2023 年利用皮尔逊相关系数分析6个输入和输出SOC 的相关性,分析结果如表3所示,其中平均电压的皮尔逊相关系数绝对值最大,电流的最小,关联度重要性从高到低依次为平均电压->总电压->平均温度->累计充电电量->绝缘电阻->电流.2.2.3灰色关联度灰色关联度[20]通过分析不同曲线的几何接近性评估它们之间的关系,接近性越高,说明相关性越紧密.假定参考序列X 0和比较序列X k 分别表示为:X 0={x 0(1),x 0(2),⋯,x 0(n )}(12)X k ={x k (1),x k (2),⋯,x k (n )}(13)式中:n 为样本数量;x 0(n )和x k (n )均为样本数据.初始化X 0和X k ,以减少参数在维度上的差异:Y 0=X 0x 0(1)={1,x 0(2)x 0(1),⋯,x 0(n )x 0(1)}={y 0(1),y 0(2),⋯,y 0(n )}(14)Y k =X k x k (1)={1,x k (2)x k (1),⋯,x k (n )x k (1)}={y k (1),y k (2),⋯,y k (n )}(15)假定ξi (h )为Y 0和Y k 在h 时刻的灰色关联系数,则:ξi (h )=min kmin h||Y 0(h )-Y k (h )||Y 0(h )-Y k (h )+ρmaxk max h||Y 0(h )-Y k (h )+ρmax kmax h||Y 0(h )-Y k (h )||Y 0(h )-Y k (h )+ρmax k max h||Y 0(h )-Y k (h )(16)式中:ρ为分辨系数,本文取0.5.故,Y 0和Y k 的灰色关联度为:ζi =1Q ∑i =1nξi (h )(17)式中:i =1,2,⋯,n ;h =1,2,⋯,Q .利用灰色关联度分析6个输入和输出SOC 的相关性,分析结果如表4所示,其中总电压和平均电压的数值相同,且最大,电流的最小,关联度重要性从高到低依次为平均电压=总电压->平均温度->累计充电电量->绝缘电阻->电流.由于LSTM 对时序数据的估计精度较高,利用LSTM 进行电池储能系统SOC 估计,在6个输入中,每次删除一个输入因素,分析不同因素缺失对电池储能系统SOC 估计精度的影响,分析结果如表5所示.在某一因素缺失的情况下,得到的RMSE 越大,说明该因素对SOC 估计精度影响越大,反之较小.由此可以获得,不同因素的重要性从高到低排序依次为电流->总电压->平均电压->平均温度->累计充电电量->绝缘电阻.综上所述,在进行影响因素重要性分析时,虽然KL 散度、皮尔逊相关系数和灰色关联度均能够一定程度上反映某个因素的重要程度,但还应以LSTM 等方法实际分析结果为主.本文数据中电流和总电压删除时,RMSE 相近且最大,而在电池储能系统实际运行当中,电流和总电压的变化也是系统电量变化的主要因素,所以两者是电池储能系统SOC 估计中的最关键因素.3 多神经网络融合策略目前,大多数神经网络估计方法主要采用单一模型,在对不同研究主体进行分析时,很难一直保持良好的性能,为此,本文采用多种神经网络融合方法进行电池储能系统SOC 估计.首先,利用经验模态分解算法将需要估计的数据进行多时间尺度分解,然后利用样本熵进行复杂性分析,将复杂性相似的分量进行分类聚合,进而利用不同的神经网络算法进行估计.表3 不同输入参数和SOC 的皮尔逊相关系数Tab. 3 Pearson correlation coefficients for different inputparameters and SOC参数γ总电压0.461 2电流0.001 8绝缘电阻-0.006 0平均电压0.490 3平均温度0.271 4累计充电电量-0.010 0表4 不同输入参数和SOC 的灰色关联度Tab. 4 Grey correlation for different inputparameters and SOC参数ζi总电压0.996 0电流0.665 0绝缘电阻0.981 9平均电压0.996 0平均温度0.995 9累计充电电量0.995 8表5 LSTM 估计结果Tab. 5 LSTM estimation results参数RMSE 总电压0.091 1电流0.094 8绝缘电阻0.049 4平均电压0.080 3平均温度0.062 9累计充电电量0.061 136第 10 期孙玉树等:电池储能系统SOC 神经网络融合估计方法3.1 经验模态分解算法经验模态分解(Empirical Mode Decomposition ,EMD )算法[21]不需要预先定义基函数,只需根据信号自身的时间尺度特征进行分解,即可获取局部化特征,非常适用于处理非线性非平稳随机信号.EMD 将信号序列分解为一系列关于时间轴对称的固有模态函数(Intrinsic Mode Function ,IMF )c i (i =1,2,⋯,n )与剩余趋势分量r n . IMF 须满足以下条件:1)在整个数据序列内,极值点与过零点个数相等或相差不超过1;2)在任一时间点上,局部均值为零.满足以下要求之一即终止分解:1)c i 或r n 小于预定值;2)r n 变为单调函数,不再能获取固有模态函数.综上,信号x (t )经EMD 分解后的表达式为:x (t )=∑i =1n c i (t )+r n(18)利用EMD 算法对图8中的SOC 曲线进行分解,分解后曲线如图12所示,共12个IMF 分量和1个残余分量.从IMF1到IMF12,频率依次减小,残余分量单调递减.3.2 样本熵近似熵只需较少的数据就可以度量序列的复杂性,但由于存在固有的对自身数据段的比较,所以计算时会产生偏差,且其取值与数据长度有关,一致性较差.由此,精度更高的样本熵[22]被提出,数据序列越复杂,样本熵值就越大,反之,越小,原理如下:假设时间序列{x i }为x (1),x (2),⋯,x (N )(N 为数据量):1)将序列{x i }按顺序组成m 维矢量,即X (i )=[x (i ),x (i +1),⋯,x (i +m -1)],其中i =1,2,⋯,N -m +1.2)定义X (i )与X (j )之间的距离d m (X (i ),X (j ))为两者对应元素差值最大值:d m (X (i ),X (j ))=max 0~m -1|x (i +k )-x (j +k)|(19)对于每一个i 值计算X (i )与其余矢量X (j )(j =1,2,⋯,N -m +1&j ≠i )之间的d m (X (i ),X (j )).3)给定相似容限r (r >0),统计每一个i 值d m (X (i ),X (j ))<r 的数量,然后计算其与距离总数N -m 的比值,记为B m i (r ):B m i(r )=1N -mnum {d m(X (i ),X (j ))<r }(20)式中:j =1,2,⋯,N -m +1&j ≠i ;num 为d m (X (i ),)X (j )<r 数量.该过程定义为X (i )模板匹配过程;B m i (r )表示任一个X (j )与模板的匹配概率.4)进而获取B m i (r )的平均值为:B m(r )=1N -m +1∑i =1N -m +1B mi()r (21)5)增加维数为m +1,重复步骤1)~步骤3),则B m +1(r )的平均值为:Bm +1(r )=1N -m∑i =1N -m B m +1i()r (22)由此可获取样本熵定义:图12 IMF 和残余分量Fig. 12 IMF and residual component37湖南大学学报(自然科学版)2023 年S E (m ,r )=lim N →∞ìíîï-ln(B m +1()r B m ()r )üýþï(23)当N 取有限值时,样本熵估计值为:S E (N ,m ,r )=-ln éëêêB m +1()r B m ()r ùûúú(24)样本熵的取值与m 、r 有关,但其一致性较好,熵值的变化趋势不受m 和r 的影响,本文取m = 2,r = 0.2 S D (r 一般为0.1~0.25 S D ,其中S D 为时间序列的标准差).利用样本熵计算每一个IMF 分量的值,如图13所示.随着IMF 分量频段频率的降低,样本熵数值大致呈逐渐降低趋势,即随着频率的降低,数据的波动变小,复杂度降低,自相似度较好. IMF1~IMF5具有较大的样本熵值,在IMF5与IMF6之间样本熵大幅度降低,IMF6~IMF12具有较小的样本熵值,且大致线性降低,数值均小于0.1;另外,求取样本熵平均值为0.302 8,IMF1~IMF5的样本熵值在平均值之上,而IMF6~IMF12数值均在平均值之下.因此将SOC 曲线分为两个频段,高频段由IMF1~IMF5这5个分量组成,低频段由IMF6~IMF12这7个分量加残余分量组成.高频段如图14所示,低频段如图15所示.3.3 多时间尺度神经网络SOC 估计基于Thinkpad T14的MatlabR2022a 软件,利用BP 和LSTM 两种策略分别对高、低频段进行估计,估计结果如表6所示.其中BP 的神经元数为5;LSTM 最大轮数为1 500,最小批次为800,初始学习率为0.01,学习率折扣为0.8. BP 对高频分量进行估计时,其精度大幅度提高,RMSE 仅为0.046 3;对低频分量进行估计时,RMSE 为0.152 9;估计后的高频分量和低频分量进行重构,由于数据之间的互补性,重构后的RMSE 为0.144 2.由此可以看出分段估计的方式比直接估计的精度要高.基于LSTM 时,高低频段的估计误差和直接估计类似,但重构后,整体的估计精度有一定程度的提高.由此可见,SOC 分频段后,每个频段的规律性增强,采用相同神经网络算法进行估计时,其精度会有一定程度的改善;再加上估计频段重构后,其数据存在一定程度上的互补性,重构后的数据精度也会提高.为了进一步验证分频段估计的优势,采用BP 对高频分量进行估计,如图16所示;利用LSTM 对低频分量进行估计,如图17所示;两者组合估计后进行图15 低频段Fig. 15 Low-frequency section表6 不同算法的高低频段估计结果Tab. 6 High and low frequency band estimation results ofdifferent algorithms算法BPLSTM RMSE0.168 50.040 2高频RMSE 0.046 30.040 3低频RMSE 0.152 90.040 5重构RMSE 0.144 20.037 9图13 各IMF 分量的样本熵Fig. 13 Sample entropy of each IMF component图14 高频段Fig. 14 High-frequency section38第 10 期孙玉树等:电池储能系统SOC 神经网络融合估计方法数据重构,如图18所示.从表7可以看出,不同神经网络相结合,也能够提高SOC 估计的精度,因此,采用分频段估计相比直接估计能够提高SOC 的估计精确度;再者,从计算时间来看,BP 进行高频估计耗时0.167 2 s ,LSTM 进行低频估计耗时235.18 s ,两者共耗时约为235.35 s ,相比LSTM 不分频估计时的373.75 s ,节约37.03%的时间.4 结 论针对电池储能系统状态精准估计问题,提出了基于深度学习融合的SOC 估计方法.首先,对比分析了BP 、GRU 和LSTM 对电池储能系统时序数据的估计效果;然后,利用KL 散度、皮尔逊相关系数和灰色关联度分析不同输入参数与SOC 的关系,三种相关性分析方法虽然各自能够在一定程度上反映某个输入参量的重要性,但在实际应用中还应以LSTM 估计的结果为准;再者,利用经验模态分解算法将SOC 分解为多个IMF 分量,利用样本熵将其分成高低两个频段,并应用BP 和LSTM 神经网络算法对两个频段分别估计,融合算法比单一算法估计精度至少提升5%,比单一LSIM 算法计算时间节约37.03%,从而为电池储能系统的规模化应用提供参考.参考文献[1]中关村储能产业技术联盟.储能产业研究白皮书[M ].北京:高科技与产业化,2023.China Energy Storage Alliance. Energy storage industry research white paper [M ].Beijing :High Technology and Industrialization ,2023.(in Chinese )[2]明彤彤,赵晶,王晓磊,等.基于改进LSTM 的脉冲大倍率工况下锂电池SOC 估计[J ].电力系统保护与控制,2021,49(8):144-150.MING T T ,ZHAO J ,WANG X L ,et al .SOC estimation of a lithium battery under high pulse rate condition based on improved LSTM [J ].Power System Protection and Control ,2021,49(8):144-150.(in Chinese )[3]王义军,左雪.锂离子电池荷电状态估算方法及其应用场景综述[J ].电力系统自动化,2022,46(14):193-207.WANG Y J ,ZUO X .Review on estimation methods for state of charge of lithium-ion battery and their application scenarios [J ].Automation of Electric Power Systems ,2022,46(14):193-207.(in Chinese )[4]罗勇,祁朋伟,黄欢,等.基于容量修正的安时积分SOC 估算方法研究[J ].汽车工程,2020,42(5):681-687.LUO Y ,QI P W ,HUANG H ,et al .Study on battery SOC estimation by ampere-hour integral method withcapacity图16 基于BP 的高频分量估计结果Fig. 16 High frequency component estimation resultsbased on BP图17 基于LSTM 的低频分量估计结果Fig. 17 Low frequency component estimation resultsbased on LSTM图18 BP 与LSTM 组合估计结果Fig. 18 Combined estimation results of BP and LSTM表7 组合算法估计结果Tab. 7 Prediction results of combinatorial algorithm算法BPLSTM高频RMSE 0.046 3―低频RMSE―0.040 5分频计算时间/s 0.167 2235.18重构RMSE 0.037 60.037 639湖南大学学报(自然科学版)2023 年correction[J].Automotive Engineering,2020,42(5):681-687.(in Chinese)[5]XING Y J,HE W,PECHT M,et al.State of charge estimation of lithium-ion batteries using the open-circuit voltage at variousambient temperatures[J].Applied Energy,2014,113:106-115.[6]LIU Y T,MA R,PANG S Z,et al.A nonlinear observer SOC estimation method based on electrochemical model for lithium-ionbattery[J].IEEE Transactions on Industry Applications,2021,57(1):1094-1104.[7]张宵洋,陈康义,吴新波.简化分数阶AEPF的锂电池SOC估计算法[J].电源技术,2022,46(10):1156-1160.ZHANG X Y,CHEN K Y,WU X B.SOC estimation algorithm oflithium battery based on simplified fractional order AEPF[J].Chinese Journal of Power Sources,2022,46(10):1156-1160.(inChinese)[8]FU S Y,LIU W,LUO W L,et al.State of charge estimation of lithium-ion phosphate battery based on weighted multi-innovationcubature Kalman filter[J].Journal of Energy Storage,2022,50:104175.[9]杜常清,吴中意,武冬梅,等.基于KF-EKF算法的动力电池SOC估计[J].武汉理工大学学报,2022,44(4):84-92.DU C Q,WU Z Y,WU D M,et al.State of charge estimation ofpower battery based on KF-EKF algorithmglish[J].Journal ofWuhan University of Technology,2022,44(4):84-92.(inChinese)[10]BAI W Y,ZHANG X H,GAO Z,et al.State of charge estimation for lithium-ion batteries under varying temperature conditionsbased on adaptive dual extended Kalman filter[J].ElectricPower Systems Research,2022,213:108751.[11]王语园,李嘉波,张福.基于粒子群算法的最小二乘支持向量机电池状态估计[J].储能科学与技术,2020,9(4):1153-1158.WANG Y Y,LI J B,ZHANG F.Battery state estimation of leastsquares support vector machine based on particle swarmoptimization[J].Energy Storage Science and Technology,2020,9(4):1153-1158.(in Chinese)[12]FAN X Y,ZHANG W G,ZHANG C P,et al.SOC estimation of Li-ion battery using convolutional neural network with U-Netarchitecture[J].Energy,2022,256:124612.[13]GONG Q R,WANG P,CHENG Z.A novel deep neural network model for estimating the state of charge of lithium-ion battery[J].Journal of Energy Storage,2022,54:105308.[14]CHEN J X,ZHANG Y,WU J,et al.SOC estimation for lithium-ion battery using the LSTM-RNN with extended input andconstrained output[J].Energy,2023,262:125375.[15]杨帆,和嘉睿,陆鸣,等.基于BP-UKF算法的锂离子电池SOC 估计[J].储能科学与技术,2023,12(2):552-559.YANG F,HE J R,LU M,et al.SOC estimation of lithium-ionbatteries based on BP-UKF algorithm[J].Energy StorageScience and Technology,2023,12(2):552-559.(in Chinese)[16]CUI Z H,KANG L,LI L W,et al.A combined state-of-charge estimation method for lithium-ion battery using an improvedBGRU network and UKF[J].Energy,2022,259:124933.[17]彭大健,裴玮,肖浩,等.数据驱动的用户需求响应行为建模与应用[J].电网技术,2021,45(7):2577-2586.PENG D J,PEI W,XIAO H,et al.Data-driven consumerdemand response behavior modelization and application[J].Power System Technology,2021,45(7):2577-2586.(in Chinese)[18]LI Z H,WU W C,ZHANG B M,et al.Kullback–Leibler divergence-based distributionally robust optimisation model forheat pump day-ahead operational schedule to improve PVintegration[J]. IET Generation,Transmission & Distribution,2018,12(13):3136-3144.[19]陈泽西,孙玉树,张妍,等. 考虑风光互补的储能优化配置研究[J].电工技术学报,2021,36(增刊1):145-153.CHEN Z X,SUN Y S,ZHANG Y,et al.Research on energystorage optimal allocation considering complementarity of windpower and PV[J]. Transactions of China Electrotechnical Society,2021,36(Sup.1): 145-153.(in Chinese)[20]陈海燕,杨振鹏,李新建,等.影响动力电池输出功率因素的灰色关联分析[J].电源技术,2019,43(6):1032-1033.CHEN H Y,YANG Z P,LI X J,et al.Grey incidence analysis forfactors influencing SOP of power battery[J].Chinese Journal ofPower Sources,2019,43(6):1032-1033.(in Chinese)[21]唐西胜,孙玉树,齐智平.基于HHT的风电功率波动及其对电力系统低频振荡的影响分析[J].电网技术,2015,39(8):2115-2121.TANG X S,SUN Y S,QI Z P.Analysis of wind power fluctuationcharacteristics and its impact on power system low frequencyoscillation based on HHT[J].Power System Technology,2015,39(8):2115-2121.(in Chinese)[22]张家安,刘东,刘辉,等.基于风速波动特征提取的超短期风速预测[J].太阳能学报,2022,43(9):308-313.ZHANG J A,LIU D,LIU H,et al.Ultra short term wind speedprediction based on wind speed fluctuation feature extraction[J].Acta Energiae Solaris Sinica,2022,43(9):308-313.(in Chinese)40。
METHOD AND EQUIPMENT FOR POWER MEASUREMENT IN AN A
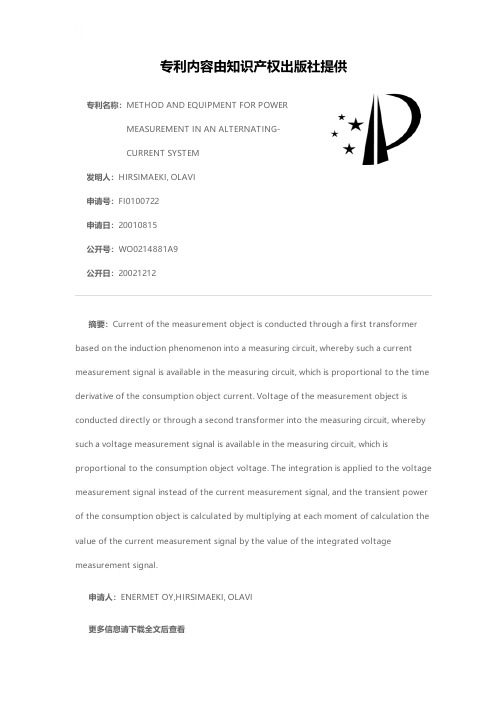
专利名称:METHOD AND EQUIPMENT FOR POWER MEASUREMENT IN AN ALTERNATING-CURRENT SYSTEM发明人:HIRSIMAEKI, OLAVI申请号:FI0100722申请日:20010815公开号:WO0214881A9公开日:20021212专利内容由知识产权出版社提供摘要:Current of the measurement object is conducted through a first transformer based on the induction phenomenon into a measuring circuit, whereby such a current measurement signal is available in the measuring circuit, which is proportional to the time derivative of the consumption object current. Voltage of the measurement object is conducted directly or through a second transformer into the measuring circuit, whereby such a voltage measurement signal is available in the measuring circuit, which is proportional to the consumption object voltage. The integration is applied to the voltage measurement signal instead of the current measurement signal, and the transient power of the consumption object is calculated by multiplying at each moment of calculation the value of the current measurement signal by the value of the integrated voltage measurement signal.申请人:ENERMET OY,HIRSIMAEKI, OLAVI更多信息请下载全文后查看。
- 1、下载文档前请自行甄别文档内容的完整性,平台不提供额外的编辑、内容补充、找答案等附加服务。
- 2、"仅部分预览"的文档,不可在线预览部分如存在完整性等问题,可反馈申请退款(可完整预览的文档不适用该条件!)。
- 3、如文档侵犯您的权益,请联系客服反馈,我们会尽快为您处理(人工客服工作时间:9:00-18:30)。
1. Introduction
An increasing number of applications in several fields like automotive, telecommunication, consumer electronics, etc. is recently being implemented by using embedded systems. These systems have become broadly used in the most recent years, due to the wide diffusion on the market of standard processors characterized by high performances and reasonable prices. We refer to embedded systems as those dedicated computing and control systems designed for specific target applications [28, 6], where dedicated software routines are provided with the system to respond to specific requireቤተ መጻሕፍቲ ባይዱents. In general, the functionality of an embedded system is constituted by a fixed number of operating ways and it is determined by the interaction between the system and the environment. According to the particular application class for which the system is dedicated, the embedded systems can be classified as data or control dominated systems [28]. In both cases, the target system architecture is composed of an hardware and a software part. The software part is typically constituted by a set of application specific software routines running on a dedicated processor or ASIP (Application Specific Instruction Processor), while the hardware part consists usually of one or more ASICs (Application Specific ICs). Due to the heterogeneous nature of the hardware and software parts of the embedded system, innovative co-design techniques have been proposed in the recent past, the goal being to meet the system-level requirements by using a concurrent design and validation approach, thus exploiting the synergism of the hardware and the software parts [6]. Several design aspects are involved in the co-design process at the system-level, including the system modeling, the capture of the functional specifications in a high-level language (co-specification), the analysis and validation of the specifications, the exploration and evaluation of the different 1
A VHDL-Based Approach for Power Estimation of Embedded Systems
William Fornaciari ^, Paolo Gubian *, Donatella Sciuto ^, Cristina Silvano *
(^) (*) Politecnico di Milano, Dipartimento di Elettronica e Informazione, P.zza L.Da Vinci, 32 - 20133 Milano, Italy, e-mail: {fornacia, sciuto}@elet.polimi.it, Fax:+39-2-2399 3411 Univ. degli Studi di Brescia, Dip. di Elettronica per l’Automazione, Via Branze, 38 - 25123 Brescia, Italy, e-mail: {gubian, silvano}@bsing.ing.unibs.it, Fax: +39-30-380014
Keywords: Embedded Systems, Low Power Design, Power Estimation, VHDL, VLSI circuits. Contact author: William Fornaciari
Abstract
Power dissipation has become one of the main constraints during the design of embedded systems and VLSI circuits in the recent years, due to the continuous increase of the integration level and the operating frequency. The aim of this paper is to present an innovative conceptual framework suitable for achieving accurate and efficient estimation of power dissipation for embedded systems described in VHDL at the behavioral and Register Transfer levels. The goal is to provide the designer with the capability of analyzing and comparing different solutions in the architectural design space, before the synthesis. The analytical power model is hierarchical, considering the different parts of the target system architecture, mainly the data-path, the memory, the control logic and the embedded core processor. Experimental results have been obtained by applying the proposed power model to benchmark circuits.
architectures with respect to some design metrics, the system-level partitioning, the cosynthesis and co-simulation. The availability of an appropriate co-design methodology, covering all these design phases, is mandatory during the design of embedded systems in order to meet the system-level requirements. These requirements are typically defined in terms of some design constraints like performance, area, power dissipation, cost, reliability, testability and development time. In particular, the technological trends toward smaller geometry and the increasing performance levels lead to high-level integration, high clock frequencies and high power dissipation. Such aspects, combined with the growing demand of battery-powered portable systems, contributed to increase the importance of the power issues during the design of embedded systems. Theref