1 Improving recognition performance
单词变形专项练习题
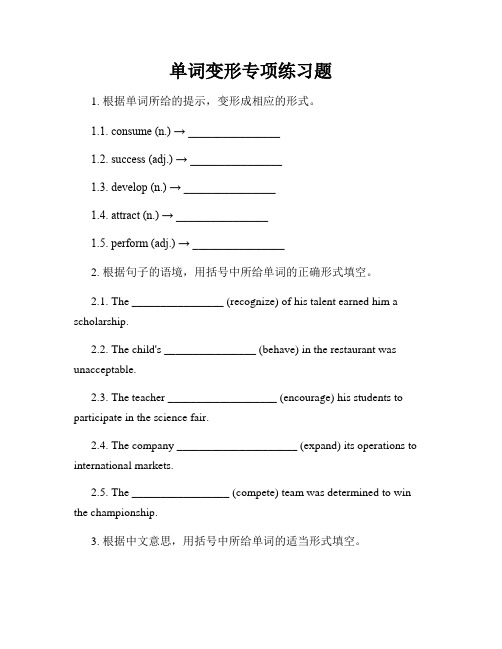
单词变形专项练习题1. 根据单词所给的提示,变形成相应的形式。
1.1. consume (n.) → ________________1.2. success (adj.) → ________________1.3. develop (n.) → ________________1.4. attract (n.) → ________________1.5. perform (adj.) → ________________2. 根据句子的语境,用括号中所给单词的正确形式填空。
2.1. The ________________ (recognize) of his talent earned him a scholarship.2.2. The child's ________________ (behave) in the restaurant was unacceptable.2.3. The teacher ___________________ (encourage) his students to participate in the science fair.2.4. The company _____________________ (expand) its operations to international markets.2.5. The _________________ (compete) team was determined to win the championship.3. 根据中文意思,用括号中所给单词的适当形式填空。
3.1. 这个课程是免费的,不需要支付任何 _________________ (额外的)费用。
3.2. 我妹妹的成绩每个学期都在 ___________________ (进步)。
3.3. 阅读是培养孩子们_________________ (创造性)思维的重要途径。
卓越绩效模式11项核心价值观
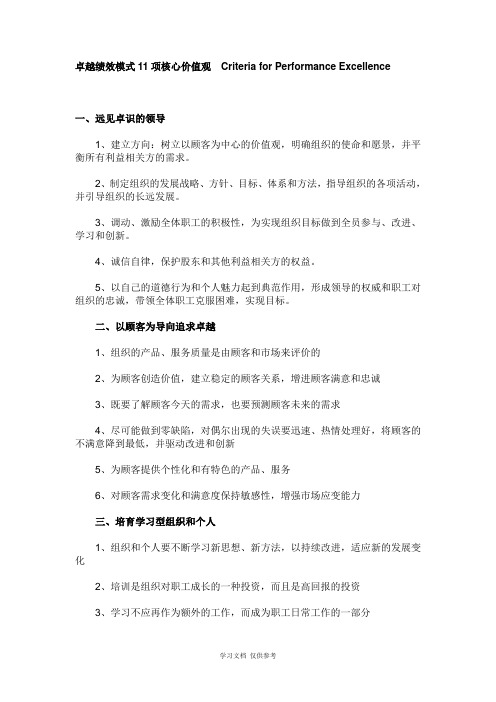
卓越绩效模式11项核心价值观Criteria for Performance Excellence一、远见卓识的领导1、建立方向:树立以顾客为中心的价值观,明确组织的使命和愿景,并平衡所有利益相关方的需求。
2、制定组织的发展战略、方针、目标、体系和方法,指导组织的各项活动,并引导组织的长远发展。
3、调动、激励全体职工的积极性,为实现组织目标做到全员参与、改进、学习和创新。
4、诚信自律,保护股东和其他利益相关方的权益。
5、以自己的道德行为和个人魅力起到典范作用,形成领导的权威和职工对组织的忠诚,带领全体职工克服困难,实现目标。
二、以顾客为导向追求卓越1、组织的产品、服务质量是由顾客和市场来评价的2、为顾客创造价值,建立稳定的顾客关系,增进顾客满意和忠诚3、既要了解顾客今天的需求,也要预测顾客未来的需求4、尽可能做到零缺陷,对偶尔出现的失误要迅速、热情处理好,将顾客的不满意降到最低,并驱动改进和创新5、为顾客提供个性化和有特色的产品、服务6、对顾客需求变化和满意度保持敏感性,增强市场应变能力三、培育学习型组织和个人1、组织和个人要不断学习新思想、新方法,以持续改进,适应新的发展变化2、培训是组织对职工成长的一种投资,而且是高回报的投资3、学习不应再作为额外的工作,而成为职工日常工作的一部分4、学习内容不仅限于技能和岗位培训,还应包括意识教育、研究开发、顾客需求研究、最正确工作方法和标杆学习5、开展互相学习和经验交流,在组织内部做到知识共享〔KMS〕6、强调学习的有效性四、尊重职工和合作伙伴1、在内部,要提高职工满意度:对职工的承诺、保障及与工会的合作;创造公平竞争环境;对优秀职工的认可;为职工提供发展时机;在企业内做到知识共享,帮助职工实现目标;营造一个鼓励职工迎接挑战的环境2、在外部,与顾客、供应商、银行、社会团体等建立战略联盟与合作伙伴关系3、建立战略合作伙伴关系的原则是:实现互利和优势互补,增强双方实力和获利能力4、成功的内部和外部合作伙伴关系应建立长远的战略目标,从制度和渠道上保证做到互相沟通,共同认识取得成功的关键要求五、灵活性和快速反应1、电子商务的出现缩短了贸易距离和时间2、为了实现快速反应,要缩短产品更新周期和产品、服务的生产周期,精简机构和简化工作程序,实施同步工程和业务流程再造〔BPR〕3、为了满足全球市场、顾客多样化需求,不能满足于简单的“按规定办事”、“按标准生产”,还要有更多的灵活性4、培养掌握多种能力的职工更为重要,以便胜任工作岗位和任务变化的需要5、时间将成为非常重要的指标,时间的改进会推动组织质量、成本和效率方面的改进六、关注未来1、持续增长和市场领先地位能给利益相关方以长期信心2、要制定组织的发展战略,分析和预测影响组织发展的各种因素3、根据组织确定的战略目标,制定中长期、短期计划,并配置所需的资源,保证战略目标的实现4、为了追求组织持续、稳定的发展,要重视与职工和供应商的同步发展〔联盟与合作〕七、创新的管理1、创新是对产品、服务和过程的富有意义的变革,为组织带来新的绩效,为利益相关方创造新的价值2、创新不仅仅局限于研究开发部门的技术和产品创新,管理创新也很重要。
perceived organizational performance
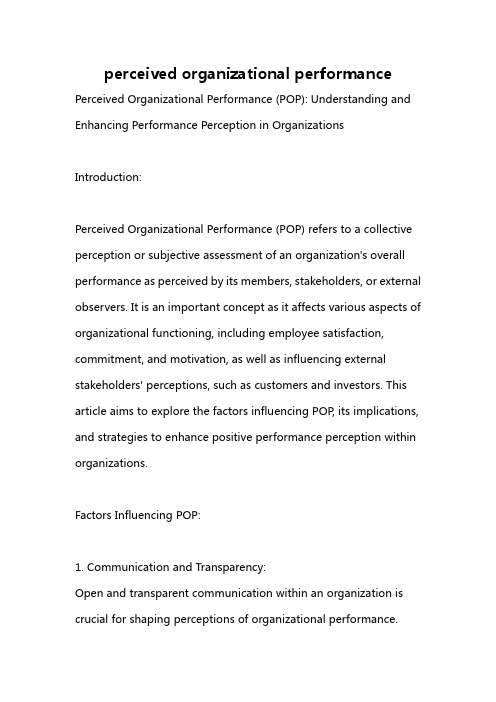
perceived organizational performance Perceived Organizational Performance (POP): Understanding and Enhancing Performance Perception in OrganizationsIntroduction:Perceived Organizational Performance (POP) refers to a collective perception or subjective assessment of an organization's overall performance as perceived by its members, stakeholders, or external observers. It is an important concept as it affects various aspects of organizational functioning, including employee satisfaction, commitment, and motivation, as well as influencing external stakeholders' perceptions, such as customers and investors. This article aims to explore the factors influencing POP, its implications, and strategies to enhance positive performance perception within organizations.Factors Influencing POP:1. Communication and Transparency:Open and transparent communication within an organization is crucial for shaping perceptions of organizational performance.Employees and stakeholders need to be informed about the organization's objectives, strategies, and progress towards goals. Regular updates, town hall meetings, or employee forums can provide opportunities for communication, clarification, and addressing concerns.2. Leadership and Management Practices:Leadership plays a critical role in shaping perceptions of organizational performance. Effective leaders demonstrate qualities such as integrity, competence, and vision, which can contribute to positive POP. Additionally, management practices such as performance feedback, recognition, and fair reward systems can influence employees' perceptions of performance.3. Employee Engagement and Empowerment:Engaged and empowered employees tend to have a more positive perception of organizational performance. Organizations should provide opportunities for employees to participate indecision-making processes, contribute ideas, and feel a sense of ownership. Empowering employees through training and development programs can also enhance their capabilities and motivation.4. Organizational Culture:The organizational culture, including its values, norms, and practices, shapes perceptions of performance. A culture that values excellence, innovation, and continuous improvement can foster positive POP. Conversely, a culture that tolerates mediocrity, lacks accountability, or stifles creativity may result in negative perceptions of performance.Implications of POP:1. Employee Satisfaction and Commitment:Positive POP can lead to higher levels of employee satisfaction and commitment. When employees perceive their organization as performing well, they are more likely to feel proud to be part of it, enjoy their work, and exhibit higher levels of commitment.2. Customer and Stakeholder Perception:Customers and external stakeholders often base their decisions on their perception of an organization's performance. Positive POP can attract customers, investors, and partners, leading to increased customer loyalty, trust, and financial support.3. Employee Motivation and Productivity:Perceptions of a high-performing organization can enhance employee motivation and productivity levels. Employees who perceive their organization as successful are likely to be more motivated to contribute their best efforts, leading to higher productivity levels and better business outcomes.Strategies to Enhance POP:1. Regular Performance Communication:Organizations should establish clear channels of communication to regularly update employees and stakeholders about organizational performance. This can include the use of dashboards, reports, or presentations to share progress towards goals, providing transparency and fostering a shared understanding of performance.2. Employee Involvement and Recognition Programs:Involving employees in decision-making processes and recognizing their contributions can enhance their perception of organizational performance. Empowerment, autonomy, andrecognition programs can create a sense of ownership and pride.3. Training and Development:Investing in employee training and development programs can enhance their skills, knowledge, and abilities, leading to improved performance perception. Providing opportunities for growth and learning can demonstrate an organization's commitment to employee development.4. Continual Improvement Initiatives:Adopting a culture of continual improvement can positively impact POP. Encouraging innovation, experimentation, and learning from failures can demonstrate a commitment to excellence and continuous growth.Conclusion:Perceived Organizational Performance influences various aspects of an organization, such as employee satisfaction, commitment, customer perception, and overall productivity. By focusing on factors such as communication, leadership, employee engagement,and fostering a positive organizational culture, organizations can enhance POP. Regular performance communication, employee involvement, recognition programs, training and development initiatives, and prioritizing continual improvement can contribute to improving organizational performance perception and creating a positive work environment.。
年终总结英文开头语
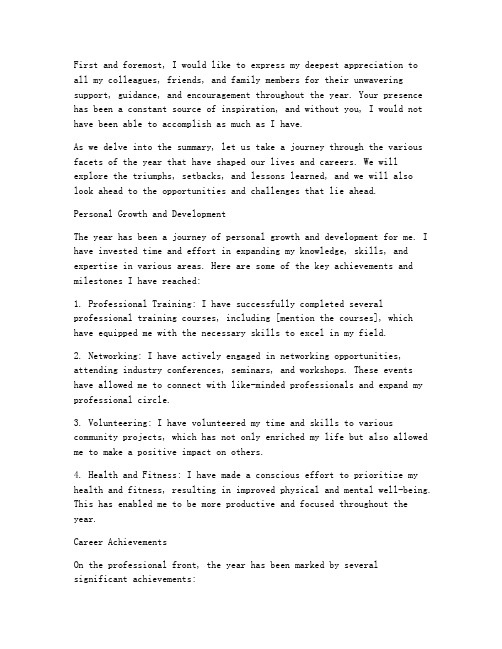
First and foremost, I would like to express my deepest appreciation toall my colleagues, friends, and family members for their unwavering support, guidance, and encouragement throughout the year. Your presence has been a constant source of inspiration, and without you, I would not have been able to accomplish as much as I have.As we delve into the summary, let us take a journey through the various facets of the year that have shaped our lives and careers. We will explore the triumphs, setbacks, and lessons learned, and we will also look ahead to the opportunities and challenges that lie ahead.Personal Growth and DevelopmentThe year has been a journey of personal growth and development for me. I have invested time and effort in expanding my knowledge, skills, and expertise in various areas. Here are some of the key achievements and milestones I have reached:1. Professional Training: I have successfully completed several professional training courses, including [mention the courses], which have equipped me with the necessary skills to excel in my field.2. Networking: I have actively engaged in networking opportunities, attending industry conferences, seminars, and workshops. These events have allowed me to connect with like-minded professionals and expand my professional circle.3. Volunteering: I have volunteered my time and skills to various community projects, which has not only enriched my life but also allowed me to make a positive impact on others.4. Health and Fitness: I have made a conscious effort to prioritize my health and fitness, resulting in improved physical and mental well-being. This has enabled me to be more productive and focused throughout the year.Career AchievementsOn the professional front, the year has been marked by severalsignificant achievements:1. Project Success: I successfully led a team of [mention the team size] members in completing [mention the project name], which was delivered on time and within budget. The project received positive feedback from stakeholders, and it has had a positive impact on the company's bottom line.2. Performance Recognition: My performance has been recognized by my superiors, and I have received [mention any awards or accolades] for my contributions to the organization.3. Promotion: I have been promoted to [mention the new position], which comes with increased responsibilities and opportunities for growth.Challenges and Lessons LearnedWhile the year has been filled with achievements, it has also presented several challenges and lessons:1. Time Management: I have learned the importance of effective time management, especially when juggling multiple projects and responsibilities. By prioritizing tasks and setting realistic deadlines, I have been able to maintain productivity and meet my goals.2. Adaptability: The year has required me to adapt to changing circumstances and unexpected challenges. This has taught me to be flexible and resilient, enabling me to navigate through difficult situations with ease.3. Communication: Effective communication has been crucial in ensuring that my team and I are aligned and working towards common goals. I have focused on improving my communication skills, which has led to better collaboration and outcomes.Aspirations for the Upcoming YearAs we look ahead to the upcoming year, I am filled with excitement and anticipation. Here are some of my aspirations for the future:1. Continuous Learning: I plan to continue my education and professional development, exploring new areas of expertise and expanding my skill set.2. Leadership: I aim to become an effective leader, inspiring and guiding my team to achieve their full potential and contribute to the organization's success.3. Balancing Personal and Professional Life: I want to maintain a healthy work-life balance, ensuring that I have time to spend with family and friends, as well as pursuing my personal interests.4. Making a Difference: I am committed to making a positive impact on the community and the world, through my work and personal initiatives.In conclusion, as we reflect on the year that has passed, we can seethat it has been a year of growth, challenges, and achievements. We have learned valuable lessons, developed our skills, and made a positive impact on our lives and the lives of others. As we move forward, let us carry these experiences and lessons with us, and let them guide us towards a brighter and more fulfilling future. Here's to the new year, and the endless possibilities it holds!。
distinguish from作句子开头
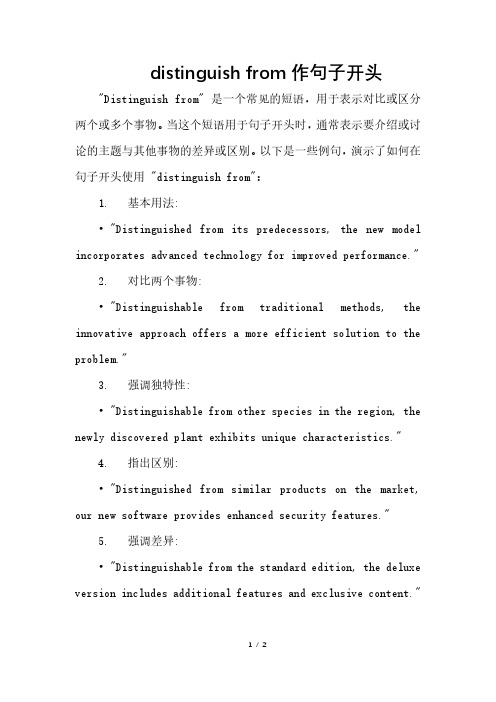
distinguish from作句子开头"Distinguish from" 是一个常见的短语,用于表示对比或区分两个或多个事物。
当这个短语用于句子开头时,通常表示要介绍或讨论的主题与其他事物的差异或区别。
以下是一些例句,演示了如何在句子开头使用 "distinguish from":1. 基本用法:• "Distinguished from its predecessors, the new model incorporates advanced technology for improved performance."2. 对比两个事物:• "Distinguishable from traditional methods, the innovative approach offers a more efficient solution to the problem."3. 强调独特性:• "Distinguishable from other species in the region, the newly discovered plant exhibits unique characteristics."4. 指出区别:• "Distinguished from similar products on the market, our new software provides enhanced security features."5. 强调差异:• "Distinguishable from the standard edition, the deluxe version includes additional features and exclusive content."在这些例句中,"Distinguish from" 被用来引导读者关注句子中提到的事物与其他事物的差异或独特之处。
绩效沟通英语作文
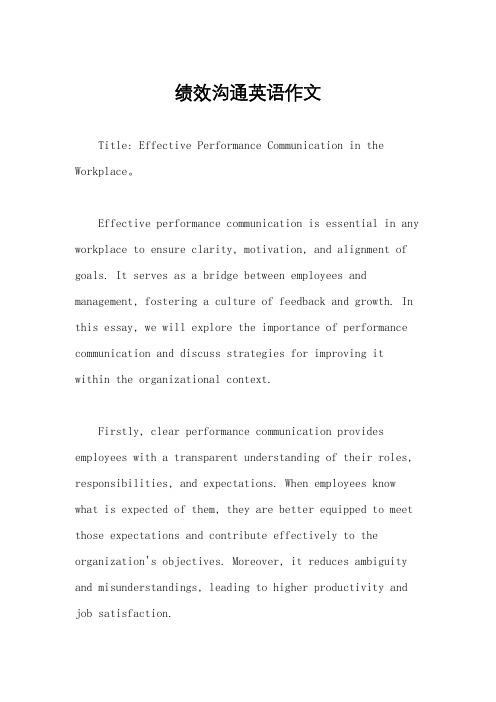
绩效沟通英语作文Title: Effective Performance Communication in the Workplace。
Effective performance communication is essential in any workplace to ensure clarity, motivation, and alignment of goals. It serves as a bridge between employees and management, fostering a culture of feedback and growth. In this essay, we will explore the importance of performance communication and discuss strategies for improving itwithin the organizational context.Firstly, clear performance communication provides employees with a transparent understanding of their roles, responsibilities, and expectations. When employees know what is expected of them, they are better equipped to meet those expectations and contribute effectively to the organization's objectives. Moreover, it reduces ambiguity and misunderstandings, leading to higher productivity and job satisfaction.Secondly, performance communication plays a crucial role in employee development and growth. Through regular feedback and performance evaluations, employees can identify their strengths and areas for improvement. Constructive feedback enables employees to enhance their skills and competencies, ultimately benefiting both the individual and the organization. Additionally, performance discussions serve as opportunities to set meaningful goals and track progress over time, fostering a culture of continuous learning and development.Furthermore, effective performance communication enhances employee engagement and morale. When employees feel valued and recognized for their contributions, they are more likely to be motivated and engaged in their work. Regular feedback and recognition reinforce positive behaviors and achievements, creating a positive work environment conducive to high performance.To improve performance communication in the workplace, organizations can implement several strategies:1. Establish clear performance expectations: Clearly define roles, responsibilities, and performance metrics to ensure alignment with organizational goals.2. Provide regular feedback: Schedule periodic performance reviews and one-on-one meetings to provide constructive feedback and discuss progress towards goals.3. Foster open communication: Encourage an open-door policy where employees feel comfortable sharing their concerns, ideas, and feedback with management.4. Offer training and development opportunities: Invest in employee training and development programs to enhance skills and competencies and support career growth.5. Recognize and reward achievements: Acknowledge and reward employees for their contributions and achievements to reinforce positive behaviors and promote a culture of appreciation.6. Utilize technology: Leverage performance management software and tools to streamline performance evaluations, track progress, and facilitate communication between managers and employees.In conclusion, effective performance communication is crucial for organizational success. By providing clear expectations, regular feedback, and opportunities for development, organizations can empower employees to reach their full potential and drive performance excellence. By implementing the strategies outlined above, organizations can create a culture of transparency, accountability, and continuous improvement, ultimately leading to greater employee satisfaction and business success.。
提高识别度,保持存在感作文800
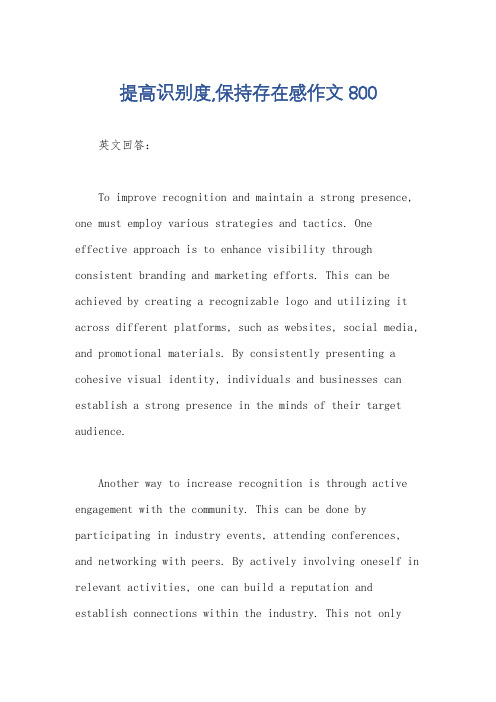
提高识别度,保持存在感作文800英文回答:To improve recognition and maintain a strong presence, one must employ various strategies and tactics. One effective approach is to enhance visibility through consistent branding and marketing efforts. This can be achieved by creating a recognizable logo and utilizing it across different platforms, such as websites, social media, and promotional materials. By consistently presenting a cohesive visual identity, individuals and businesses can establish a strong presence in the minds of their target audience.Another way to increase recognition is through active engagement with the community. This can be done by participating in industry events, attending conferences, and networking with peers. By actively involving oneself in relevant activities, one can build a reputation and establish connections within the industry. This not onlyincreases recognition but also opens up opportunities for collaboration and growth.Furthermore, maintaining a strong online presence is essential in today's digital age. This can be achieved through search engine optimization (SEO) techniques, creating valuable and engaging content, and leveraging social media platforms. By consistently delivering high-quality content and actively engaging with followers, individuals and businesses can attract a larger audience and increase their recognition.In addition to these strategies, it is important to constantly innovate and adapt to changing trends. Staying ahead of the curve and offering unique products or services can help differentiate oneself from competitors and increase recognition. By keeping a close eye on market trends and consumer demands, individuals and businesses can identify opportunities for innovation and stand out in the crowd.In summary, improving recognition and maintaining astrong presence requires consistent branding, active community engagement, a strong online presence, and a focus on innovation. By implementing these strategies andadapting to changing trends, individuals and businesses can increase their visibility and establish a strong presencein their respective industries.中文回答:为了提高识别度和保持存在感,我们需要采取各种策略和方法。
[2015](face++)Naive-Deep Face Recognition Touching the Limit of LFW Benchmark or Not
Naive-Deep Face Recognition Touching the Limit of LFW Benchmark or Not](https://img.taocdn.com/s3/m/49198117227916888486d7ca.png)
DeepID2+
DeepFace
92 90 88 86
#Dataset ~ 10K #Dataset < 100K #Dataset > 100K
Multiple LE + comp
84 2009
2010
2011
2012 Year
2013
2014
2015
Figure 1. A data perspective to the LFW history. Large amounts of web-collected data is coming up with the recent deep learning waves. Extreme performance improvement is gained then. How does big data impact face recognition?
100 98 96 94
Accuracy
DeepID2 GaussianFace DeepID TL Joint Bayesian High-dim LBP Tom-vs-Pete Bayesian Face Revisited Associate-Predict Hybrid Deep Learning FR+FCN
Naive-Deep Face Recognition: Touching the Limit of LFW Benchmark or Not?
Erjin Zhou Face++, Megvii Inc.
zej@
Zhimin Cao Face++, Megvii Inc.
czm@
and requires very low false positive rate. Unfortunately, empirical results show that a generic method trained with webcollected data and high LFW performance doesn’t imply an acceptable result on such an application-driven benchmark. When we keep the false positive rate in 10−5 , the true positive rateis 66%, which does not meet our application’s requirement. By summarizing these experiments, we report three main challenges in face recognition: data bias, very low false positive criteria, and cross factors. Despite we achieve very high accuracy on the LFW benchmark, these problems still exist and will be amplified in many specific real-world applications. Hence, from an industrial perspective, we discuss several ways to direct the future research. Our central concern is around data: how to collect data and how to use data. We hope these discussions will contribute to further study in face recognition.
- 1、下载文档前请自行甄别文档内容的完整性,平台不提供额外的编辑、内容补充、找答案等附加服务。
- 2、"仅部分预览"的文档,不可在线预览部分如存在完整性等问题,可反馈申请退款(可完整预览的文档不适用该条件!)。
- 3、如文档侵犯您的权益,请联系客服反馈,我们会尽快为您处理(人工客服工作时间:9:00-18:30)。
Improving recognition performance by modelling pronunciation variationJudith Kessens and Mirjam WesterDepartment of Language & SpeechUniversity of NijmegenE-mail: {kessens|wester}@let.kun.nlAbstractThis paper describes a method for improving the performance of a continuous speech recognizer by modelling pronunciation variation. Although the improvements obtained with this method are small, they are in line with those reported by other authors. A series of experiments was carried out to model pronunciation variation. In the first set of experiments word internal pronunciation variation was modelled by applying a set of four phonological rules to the words in the lexicon. In the second set of experiments, variation across word boundaries was also modelled. The results obtained with both methods are presented in detail. Furthermore, statistics are given on the application of the four phonological rules on the training database. We will explain why the improvements obtained with this method are small and how we intend to increase the improvements in our future research.1Figure 1: Architecture of Spoken Dialogue System 2IntroductionAt the Department of Language and Speech at the University of Nijmegen, we are working on a Spoken Dialogue System (SDS) that will be employed to automate part of a public transport information service. This system was adapted from a German prototype (Steinbiss et al., 1995) developed by Philips Research Labs (Aachen, Germany), and was further improved by means of a bootstrapping method (Strik et al. 1996 and 1997).The architecture of the SDS is shown in Figure 1. The SDS consists of a telephone interface, a continuous speech recognizer (CSR), a natural language processor (NLP), a dialogue management (DM) module which is connected to a database, and a text-to-speech (TTS) synthesizer. The telephone interface is responsible for the interaction between the telephone network and the SDS. The incoming speech signal is converted into sequences of words by the CSR. The NLP searches for information in the sequences of recognized words, for example desired departure time or time of arrival. The DM module stores the information found by the NLP and checks whether or not it is complete. If information is missing, the system continues to ask questions until all necessary information is obtained. The DM looks up the answer in a database, formulates a reply in text form, and sends it on to the TTS synthesizer. The TTS synthesizer converts the text into a speech signal and passes this signal to the telephone interface, which in turn sends the message through the telephone line to the user.In the present research, we are only concerned with the CSR component of the SDS. This CSR was gradually improved through a bootstrapping method, by adding more data. However, since a point was reached at which no further increase in performance could be obtained by adding more data, new methods of improving the system were sought. Given that the SDS is an interactive system the kind of speech callers use may be extremely varied. The manner in which people speak to a machine can vary from using very sloppy articulation to hyper articulation. Therefore, it is obvious to expect that the system’s performance might be improved by modelling pronunciation variation.Pronunciation variation can be divided into two kinds of variation. The first kind of variation is variation in the realized sequence of phones a word consists of, and the second kind is variation in the acoustic realization of sounds, the so called allophonic variation. Up till now, we have only studied3the first kind of pronunciation variation, because we expect the allophonic variation to be implicitly modelled in the HMMs.In the next section, some of the difficulties caused by pronunciation variation are discussed for both the training and the recognition procedure. First, we explain how recognition works and how modelling pronunciation variation may improve it. Next, we explain the training procedure and how pronunciation variation can be modelled during training. In the following section, the method for modelling pronunciation variation is explained in detail. Subsequently, the results obtained are analysed in detail. In the last section, we discuss why the improvements obtained so far with this method are small, and how we intend to adapt the method to obtain considerably higher recognition performance.How does a continuous speech recognizer work? RecognitionThe CSR component of the spoken dialogue system converts incoming speech signals into corresponding sequences of words. In the online SDS, the CSR passes a number of sequences of words on to the NLP, in the form of a wordgraph. From this wordgraph, it is possible to compute that sequence of words which best fits to the incoming speech signal, the so called Best Sentence (BS).Nowadays, almost all CSRs are probabilistic machines. This means that the CSR calculates the probability that the incoming speech signal is the result of the production of a specific sequence of words. This probability is calculated for a lot of potential sequences of words. The sequence with the highest probability is “recognized”. Before a CSR can be used, it has to be trained. During training the recognizer “learns” the probability of observing a specific speech signal when a certain sequence of words is uttered.The CSR can only recognize words which are in the lexicon. The words are listed in the lexicon in two forms: an orthographic form and a transcription in basic sound units. These basic sounds are all Dutch phonemes and a few non-speech sounds. In this article, the basic recognition units are called “phones”. During recognition, the phone transcriptions for the words are looked up in the lexicon and the words are replaced by the corresponding 4sequences of phones. For each phone there is a corresponding phone model, the so called hidden Markov model (HMM). The statistics of the corresponding phone are stored in this model.During the recognition phase, the CSR attempts to recognize an unknown sequence of words. If all possible sequences of words had to be generated, the number of hypotheses would be vast. Fortunately, from the start all hypotheses are scored according to their probability. The probability of a word is calculated on the basis of the HMMs which correspond to the phones a word consists of. The majority of the hypotheses appear to be much less probable than the best hypotheses so that they can be removed from the list of possible solutions without consequences. For each possible sequence of words which remains, the optimal alignment and the corresponding probability are calculated. Finally, the sequence of words with the highest probability is recognized.For each word in the lexicon there is only one phone transcription: this is the canonical phone transcription which represents the most probable pronunciation of the word, based on introspective linguistic knowledge. Using a lexicon with only one canonical phone transcription leads to the problem that a word which is pronounced differently from the pronunciation in the lexicon may be incorrectly recognized. An example is the pronunciation of Delft (Dutch city).Suppose the canonical pronunciation in the lexicon is: /dElft/Suppose the realized pronunciation is: /dEl«f/In the realized pronunciation /«/ is inserted and /t/ is deleted with respect to the canonical pronunciation in the lexicon. In this example, the calculated probability of the realized pronunciation of the word is lower than it would have been if the spoken phone sequence had been exactly equal to the phone transcription in the lexicon. The probabilities for /l/ and /f/ are lower, because /«/ is inserted. For the phone /t/ the probability is also lower, because /t/ is not realized at all. In this situation, it is possible that an incorrect word has a higher probability than the uttered word, and consequently the incorrect word is recognized.A possible solution for this problem is to allow for multiple pronunciations in the lexicon instead of a single pronunciation. During recognition, these added pronunciation variants function as additional hypotheses. It is then to be expected that the actual realized pronunciation will deviate less from the most probable variant than when a canonical lexicon is used.5TrainingBefore we are able to do any kind of recognition, the phone models need to be trained. To train the phone models, it is necessary to have a large amount of recorded speech material (corpus) with corresponding transcriptions.The training procedure consists of the following steps:+The phone transcriptions of the words are looked up in the lexicon.Each utterance is replaced by its phone transcription; in this way, the phone transcription for the whole utterance is obtained.+The Viterbi algorithm is used to find the optimal alignment between the speech signal and the phone transcription. In fact, this alignment isa segmentation because the boundaries are determined for each phoneunit in the transcription. For each alignment, the Viterbi algorithm calculates a probability. This probability can be interpreted as the chance that the phone transcription and the speech signal belong together. The optimal alignment is the alignment with the highest probability.+After segmentation, all parts of the speech material which correspond to the same phone are statistically processed. This results in a stochastic model (HMM-model) for each basic recognition unit (phone). To obtain reliable estimates of the model’s parameters, it is necessary to use a large number of realizations of each phone to train the models.All steps are repeated a number of times in an iterative process. There is mathematical proof that the average probability of the transcribed words is improved each iteration.In addition to the phone models, a language model is also trained. A language model predicts the probability of occurrence of a word (unigram), or of a sequence of words (bigram, trigram etc.). These language models play an important role in the recognition task. However, in this research, the language models remain unchanged, so we will pay no further attention to this topic here.If a canonical lexicon is used during training, a similar difficulty arises as in the recognition procedure. The pronunciation of a word can differ from the pronunciation in the lexicon represented by the canonical phone transcription. If the phone models are trained on the basis of this wrong phone sequence, parts of the speech signal train the wrong model, and consequently the phone models become contaminated. If we look at the same6example of the Dutch city Delft, we see that in the spoken word /«/ is inserted and /t/ is deleted with respect to the canonical pronunciation. So, in this case, the models for /l/ and /f/ are contaminated, because parts of the speech signal where /«/ is spoken train the models for /l/ and /f/. The model for /t/ is also contaminated, because parts of the speech signal train the model, while /t/ is not realized at all.A possible solution to this problem can be to annotate manually what has been said in the speech material and to train new, less contaminated phone models on the basis of this more accurately annotated training corpus. The main disadvantage of manually annotating is that it is time-consuming and therefore costly. For this reason, we propose a method in which the CSR is used to annotate the speech material automatically. In order to do so, a lexicon is needed with multiple pronunciation variants for each word. The new phone models are expected to be less contaminated than the original ones. Therefore, they allow for less pronunciation variation than the original phone models. In order to optimally use the new phone models during recognition, it is necessary to use a lexicon in which the pronunciation variation is explicitly modelled, i.e. a lexicon with multiple pronunciations for a word.Method and MaterialMethodThe starting-point of the current research was a CSR in which a lexicon was used with only one, canonical pronunciation for each word. In order to model pronunciation both in training and in recognition, it is necessary to generate a lexicon with multiple pronunciations for each word. The method we used resembles those used previously with success by Cohen (1989) and Lamel & Adda (1996). In this approach, phonological rules are used to generate pronunciation variants automatically, i.e. to expand the lexicon. The expanded lexicon can then be used during training, recognition, or both. During recognition, the old recognition lexicon is simply replaced by the new one in order to make it possible to recognize pronunciation variants. During training, the pronunciation variants are used to obtain new phone models as follows:1.Start off with a single pronunciation lexicon, containing canonical7pronunciation forms. Use the original corpus and this single pronunciation lexicon to calculate the first version of the phone models.2.Choose a set of phonological rules.3.Generate a multiple pronunciation lexicon by expanding the singlepronunciation lexicon with pronunciation variants obtained with the set of phonological rules.4.Do a forced recognition in order to determine which variants havebeen realized in the corpus. During this recognition, the CSR is forced to choose the pronunciation variant which is the best description of the speech signal. It is only possible to choose between different phone transcriptions of the same word, but not between different words. By substituting the initial transcriptions with those selected during forced recognition, new phone transcriptions for the training corpus are obtained automatically.5.The new transcriptions are used to calculate updated phone models.Steps 4 and 5 can be repeated a number of times. We expect the updated phone models to be less contaminated than the original ones. When these updated phone models are used during forced recognition the correct variant will be chosen more often than when the original phone models are used. Therefore, each iteration the newly updated phone models will be less contaminated. Steps 2 through 5 can be repeated for different sets of rules.Our ultimate goal is to find the rules that are optimal in the sense that application of these rules gives the greatest increase in recognition performance. The goal of the current research was to test whether the method proposed above is suitable for our purposes. In order to do so, the method was first tested by using four phonological rules which were applied word internally, as will be explained below. Next, cross-word variation was modelled for a set of frequently occurring word sequences.Phonological rulesIn order to select the initial set of phonological rules, a number of criteria were followed. Variation occurs both within words and across words. Given the use of a lexicon in our CSR, it was obvious to begin with word internal variation. Therefore, the first criterion was to choose rules of word phonology. Second, we decided to start with rules concerning those phenomena that are known to be most detrimental to automatic speech recognition. Of the three possible recognition errors, i.e. insertions, deletions, and substitutions, we expect the first two to have the greatest consequences 8for speech recognition, because they affect the number of segments present in different realizations of the same word. Therefore, starting with rules concerning insertions and deletions was the second criterion we adopted. The third criterion was to choose rules that are frequently applied. Frequently applied can be interpreted in two ways. First, a rule can be frequent because it is frequently applied whenever the context for its application is met. Second, the context in which a rule is applicable can be very frequent, even though the rule is not applied in most of the cases. Obviously, it is this latter case of “frequent occurrence” which is most interesting for automatic speech recognition, since it is difficult to predict in this case which variant should be selected as the canonical form, while in the former case the most frequent form would probably suffice as sole transcription. The fourth criterion (related to the previous one) was that the rules should regard phones that are relatively frequent in Dutch, since rules that concern frequent phones will influence the recognizer's performance to a larger extent. Finally, we decided to start with rules that have been extensively described in the literature, so as to avoid possible effects of overgeneration and undergeneration due to incorrect specification of the rules.On the basis of the above-mentioned criteria, the following four rules were selected. The description of the four rules is after Booij (1995):1./«/-deletion:When a Dutch word has two consecutive syllables headed by /«/, the first /«/ may be deleted, provided that the resulting onset consonant cluster is an obstruent + liquid cluster. Example:latere/la t«r«/ /la tr«/‘later’2./t/-deletion:The rule of /t/-deletion is one of the processes that typically occurs in fast speech, but to a lesser extent in careful speech. If a /t/ in a coda is preceded by an obstruent, and followed by another consonant, the /t/ may be deleted. Example:snelstmogelijk/snElstmox«l«k/ /snElsmox«l«k/‘fastest’If the preceding consonant is a sonorant, /t/-deletion is possible, but then the following consonant must be an obstruent. If the obstruent following the9sonorant + /t/ cluster is a /k/, deletion does not apply. If a /t/ is preceded by a sonorant, and also followed by a sonorant, deletion is impossible.Example:‘s avonds/sav nts/ /sav ns/‘in the evening’Booij does not mention that in some Dutch dialects /t/-deletion also occur in word-final position. We decided to apply the /t/-deletion rule in word-final position following an obstruent (unless the obstruent is an /s/).Example:Utrecht/ytrExt/ /ytrEx/‘Dutch city’3./n/-deletion:In standard Dutch, syllable-final /n/ can be dropped after /«/, except in the indefinite article een /«n/ ‘a’. For many speakers, in particular in the western part of the Netherlands, the deletion of /n/ is obligatory. An /n/ is deleted if it is the final /n/ of a syllable after /«/ and if that syllable is not a verbal stem. Example:reizen/rEiz«n/ /rEiz«/‘to travel’4./«/-epenthesis:In nonhomorganic consonant clusters in coda position /«/ may be inserted. If the second of the two consonants involved is an /s/ or a /t/, or if the cluster is a nasal followed by a homorganic consonant, /«/-insertion is not possible. Example:Delft/dElft/ /dEl«ft/‘Dutch city’MaterialThe speech material was collected with an online version of the SDS connected to an ISDN line. The training and test material consisted of 25,104 utterances (83,890 words) and 6,276 utterances (21,108 words), respectively. 10The most important characteristics of the CSR are the following. The input signals consist of 8 kHz, 8-bit A-law coded samples. Feature extraction is done every 10 ms for frames with a width of 16 ms. The first step in feature analysis is an FFT analysis to calculate the spectrum. Next, the energy is calculated in 14 mel-scaled filter bands between 350 and 3400 Hz. The final processing stage is the application of a discrete cosine transformation on the log filterbank coefficients. Besides these 14 cepstral coefficients, the 14 delta coefficients are also used. This makes a total of 28 feature coefficients. The CSR uses acoustic models (HMMs), language models (unigram and bigram), and a lexicon. The HMMs consist of three segments of two identical states, of which one state per segment can be skipped.The canonical training lexicon contains 1,412 entries, which are all the words in the training corpus, while the recognition lexicon contains 1,154 entries. There were no out-of-vocabulary (OOV) words in the test corpus, which is a slightly artificial condition. The reason for this is that we wanted to measure the effect of modelling pronunciation variation and to avoid a situation in which a lot of errors would be caused by OOV words.The four phonological rules selected for investigation affect 38% of the words in each lexicon. In a number of cases, more than one rule could be applied to one word. On average, 1.3 variants were generated for each word. ResultsForced recognitionAs forced recognition is an essential part of our method, a small-scale experiment was conducted to check whether the forced recognition procedure worked correctly. Listeners were asked to perform the same task as the forced recognition, which was to assess which pronunciation variant had been spoken. Their results were then compared to the results of the forced recognition. From this experiment we could conclude that the correct variant was chosen in 90% of the 711 cases.Within word pronunciation variationIn the first experiments, the set of four phonological rules were applied word internally to all words in the lexicon. The effects of adding pronunciation11variants were measured in Sentence Error Rates (SER = percentage of incorrectly recognized sentences). As a baseline system, we used a CSR with a canonical lexicon which contains one variant for each word. This “most probable pronunciation” can be a variant of which one of the four phonological rules has already been applied, e.g. the canonical form for reizen ‘to travel’ is /rEiz«/ (n-deletion). The SER for the baseline system is 21.48%.The multiple lexicon was used during training and/or recognition, which results a combination of four testing conditions. For training, stages 4 and 5 of our method were repeated in iteration. A gradual improvement in recognition performance was observed during the first 3 iterations. The results in SER, for all four CSRs after 3 iterations, are given in Table 1. In this table “original” means that the original corpus was used to train the phone models, “updated 3” that the updated corpus obtained after 3 iterations was used to train the new phone models, “single” means that a canonical pronunciation lexicon was used during recognition, and “multiple” means that the multiple pronunciation lexicon was used during recognition.Using the multiple lexicon during training, but not during recognition, causes a deterioration in SER of 0.57% (compare column 4 to 2). This result is in line with our expectations. The updated phone models allow for less pronunciation variation than the original phone models, so in order to benefit from the less contaminated phone models the pronunciation variation has to be modelled in the lexicon. Using the multiple pronunciation lexicon during recognition alone led to an improvement in SER of 0.42% (compare column 3 to 2). Performance improved by another 0.25% (0.67% in total) when the multiple pronunciation lexicon was used during both training and recognition (compare column 5 to 3). It thus appears that the multiple pronunciation lexicon has more effect when used during recognition than during training. However, combining the two produces the best results. Although the 12improvements in SER are not significant, the trends are in line with Lamel & Adda (1996).Detailed analysis of changes in SERThe largest improvements in performance result from the use of a multiple pronunciation lexicon. In order to get more insight into the effects of this method, the results obtained with the two lexicons (single and multiple) were analysed in further detail. For instance, we counted the number of incorrect sentences obtained with the single and the multiple pronunciation lexicon. These results are shown in Table 2. In column 2 (SER unchanged) the errors which do not affect the SER are given, while in column 4 (SER changed), the changes in SER are shown which decrease (improvements) or increase (deteriorations) SER.Table 2. Details of changes in SER due to recognition with a multipleTable 2 shows that a considerable number of incorrectly recognized sentences remain incorrect (1292) when using a multiple pronunciation lexicon for recognition. There are cases in which a better solution is chosen (56), but, since in a number of cases a worse solution is chosen (30), the two effects cancel each other out, and the nett result (26) is small. This neutralization effect explains why no significant improvements in the SERs were observed in Table 1.Improvements and deteriorations for each ruleNext, we studied the improvements and deteriorations in SER distributed over the different phonological rules. In one sentence, more than one phonological rule can cause (deterioration) or solve (improvement) errors. However, a sentence is either correct or incorrect. If an error occurs due to13two phonological rules, both rules get a count of 0.5 deterioration. Table 3 shows the improvements and deteriorations caused by each rule. In this Table, “n-del.” means n-deletion rule, “«-epe.” means «-epenthesis rule,“«-del.” means «-deletion, “t-del.” means t-deletion rule, and “unknown”means that it is unclear which rule caused or solved an error. From this Table it can be concluded that pronunciation modelling due to the n-deletion rule has the largest effect on recognition performance.About 96% of the deteriorations can be explained by confusability. This means that a word which was correctly recognized before is now incorrectly recognized, because it is confused with a pronunciation variant which has been added to the multiple pronunciation lexicon.How different selection criteria for variants in lexicon affect SERThe presence of multiple pronunciations in the training corpus makes it possible to study how the frequency of the variants included in the recognition lexicon affects SER. We performed a test in which we used a single-variant lexicon containing the least frequent pronunciation of each word, and a second test in which a single-variant lexicon containing the most frequent variant in the training corpus was used. The results are shown in Table 4.14When the single-variant lexicon containing the least frequent pronunciations is used, SER is 22.94%. However, when the variants are replaced by the most probable variants, SER drops to 20.70%. For the canonical lexicon SER is 21.48%, and for the multiple lexicon (all) SER is 20.81%. In other words, using a lexicon with the most probable variants led to a significantly better performance in SER, than using a lexicon containing the least frequent variants. Using a canonical lexicon and a multiple pronunciation lexicon leads to a recognition performance somewhere in between.These results show that selecting the right variants is crucial and that it is difficult to determine whether the method under study improves recognition performance to a sufficient extent. Since the decision is usually made by comparing performance before and after applying the method, it follows that the better the pretest performance, the smaller the improvement will be. If we had started with a lexicon containing the least probable variants, we would have concluded that modelling pronunciation variation leads to a considerable improvement. On the other hand, if we had started with a lexicon containing the most probable variants, we would have found no improvement at all. Clearly, our current results are somewhere in between. Application of the four phonological rules in spontaneous speechUsing the forced recognition procedure it is possible to investigate which pronunciation variant is spoken in the speech database we used in our experiments. The results give some insight into how the four phonological rules we used are applied in spontaneous speech. We used the training corpus, consisting of 83,890 words, for the forced recognition. Of these words 14,950 words have one or more pronunciation variants. After forced recognition it is possible to count in how many cases a specific rule has been applied. In this case, application of the rule is independent of the canonical form, thus if the canonical form for the Dutch word reizen ‘to travel’ is realized as /rEiz«/, then the count for the n-deletion rule is raised by one. Because the forced recognition works correctly in 90% of the cases, the estimated error is 10%.15。