基于线要素得三维测配色系统校准算法(IJIGSP-V1-N1-3)
一种基于边缘线的三目立体匹配方法
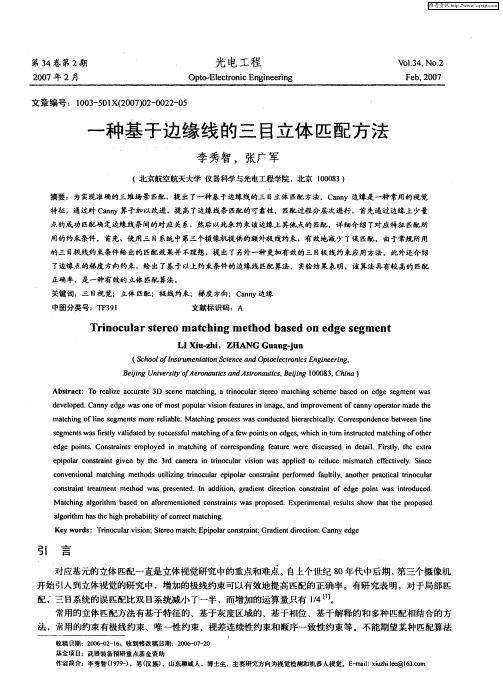
维普资讯
第3 4卷第 2 期
20 0 7年 2月
光 电工程
Opo- e to c En n e i g t Elc r ni gie rn
Vo . 4 No. 13 , 2
Fe 2 0 b, 0 7
文 章编号 :10 — 0 2 0 )2 0 2 — 5 3 5 0 70 — 0 2 0 0 1 X(
一
种 基 于边 缘 线 的三 目立体 匹配 方 法
李 秀智 ,张广军
(北京航空航天大学 仪器科学与光 电工程学院 ,北京 10 8 0 0 3)
摘要 :为 实现 准确 的三 维场景 匹配,提 出 了一种基 于边缘线的三 目立体 匹配方法。C n y边缘 是一种 常用的视 觉 an
特征 ,通过对 C ny算子加 以改进 ,提 高了边缘线条 匹配的可 靠性。 匹配过程分层次进行,首先通 过边缘 上少量 an 点的成功 匹配确定边缘 线条间的对应 关 系,然后以此 来约束该边缘上其他点的 匹配。详细介绍 了对应特征 匹配所 用的约束条件。首先,使用三 目系统 中第三个摄像机提 供 的额外极线约束 ,有效地减 少了误 匹配 。由于常规 所用 的三 目极 线约束条件给 出的 匹配效 果并 不理想 ,提 出了另外一种 更加有效的三 目极线约 束应用 方法 。此外还介绍 了边缘点的梯度方 向约束,给 出 了基 于以上 约束条件的边缘线 匹配算法.实验结 果表 明,该 算法具有较 高的匹配
d v l p d Ca n d e wa n fmo t o u a ii n f au e ma e a d i r v me to a n p r t rma e t e e eo e . n y e g s o e o s p p l r so e t r si i g , n v n mp o e n fc n y o e a o d h ma c i g o n e me t r e ib e Ma c ig p o e s wa o d c e ir r h c l . re p n e c ewe n l e th n fl e s g n s mo e r l l . i a th n r c s s c n u t d h e a c ia l Co r s o d n e b t e i y n s g n swa r t a ia e y s c e s u t hn f w o n so d e , ih i u n i sr ce t h n f te e me t s f sl v l t d b u c s f l i y d mac i g o f p i t ne g s wh c nt r n t td mac i g o h r ae u o e g ons d e p it.Co sr it mp o e n mac i g o o r s o d n e t r r ic s e n d t i n t n s e l y d i th n f c re p n ig f au e we e d s u s d i e a l i t ,t e e ta a .F r l h x r s y e i l o sr i tg v n b h r a r n t n c lr v s n wa p l d t e u e mima c fe t e y i c p p a c n tan i e y t e 3 d c me a i r o ua ii s a p i o r d c s t h e c i l .S n e o r i o e v
基于人类色彩感知的图像自动分割(IJIGSP-V1-N1-4)
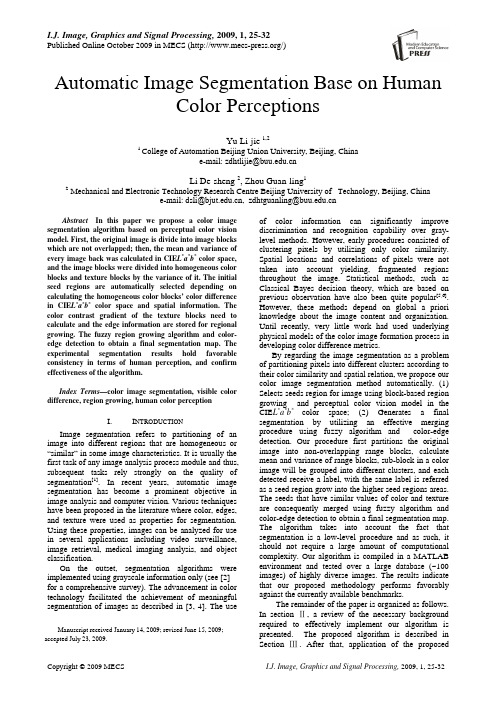
I.J. Image, Graphics and Signal Processing, 2009, 1, 25-32Published Online October 2009 in MECS (/)Automatic Image Segmentation Base on HumanColor PerceptionsYu Li-jie 1,21 College of Automation Beijing Union University, Beijing, Chinae-mail: zdhtlijie@Li De-sheng 2, Zhou Guan-ling12 Mechanical and Electronic Technology Research Centre Beijing University of Technology, Beijing, Chinae-mail: dsli@, zdhtguanling@Abstract—In this paper we propose a color image segmentation algorithm based on perceptual color vision model. First, the original image is divide into image blocks which are not overlapped; then, the mean and variance of every image back was calculated in CIE L*a*b* color space, and the image blocks were divided into homogeneous color blocks and texture blocks by the variance of it. The initial seed regions are automatically selected depending on calculating the homogeneous color blocks’ color difference in CIE L*a*b* color space and spatial information. The color contrast gradient of the texture blocks need to calculate and the edge information are stored for regional growing. The fuzzy region growing a lgorithm and color-edge detection to obtain a final segmentation map. The experimental segmentation results hold favorable consistency in terms of human perception, and confirm effectiveness of the algorithm.Index Terms—color image segmentation, visible color difference, region growing, human color perceptionI.I NTRODUCTIONImage segmentation refers to partitioning of an image into different regions that are homogeneous or “similar” in some image characteristics. It is usually the first task of any image analysis process module and thus, subsequent tasks rely strongly on the quality of segmentation[1]. In recent years, automatic image segmentation has become a prominent objective in image analysis and computer vision. Various techniques have been proposed in the literature where color, edges, and texture were used as properties for segmentation. Using these properties, images can be analyzed for use in several applications including video surveillance, image retrieval, medical imaging analysis, and object classification.On the outset, segmentation algorithms were implemented using grayscale information only (see [2] for a comprehensive survey). The advancement in color technology facilitated the achievement of meaningful segmentation of images as described in [3, 4]. The use of color information can significantly improve discrimination and recognition capability over gray-level methods. However, early procedures consisted of clustering pixels by utilizing only color similarity. Spatial locations and correlations of pixels were not taken into account yielding, fragmented regions throughout the image. Statistical methods, such as Classical Bayes decision theory, which are based on previous observation have also been quite popular[5,6]. However, these methods depend on global a priori knowledge about the image content and organization. Until recently, very little work had used underlying physical models of the color image formation process in developing color difference metrics.By regarding the image segmentation as a problem of partitioning pixels into different clusters according to their color similarity and spatial relation, we propose our color image segmentation method automatically. (1) Selects seeds region for image using block-based region growing and perceptual color vision model in the CIE L*a*b* color space; (2) Generates a final segmentation by utilizing an effective merging procedure using fuzzy algorithm and color-edge detection. Our procedure first partitions the original image into non-overlapping range blocks, calculate mean and variance of range blocks, sub-block in a color image will be grouped into different clusters, and each detected receive a label, with the same label is referred as a seed region grow into the higher seed regions areas. The seeds that have similar values of color and texture are consequently merged using fuzzy algorithm and color-edge detection to obtain a final segmentation map. The algorithm takes into account the fact that segmentation is a low-level procedure and as such, it should not require a large amount of computational complexity. Our algorithm is compiled in a MATLAB environment and tested over a large database (~100 images) of highly diverse images. The results indicate that our proposed methodology performs favorably against the currently available benchmarks.The remainder of the paper is organized as follows. In section Ⅱ, a review of the necessary background required to effectively implement our algorithm is presented. The proposed algorithm is described in Section Ⅲ. After that, application of the proposed26 Automatic Image Segmentation Base on Human Color Perceptionsalgorithm is discussed in section Ⅳ, and we draw our conclusion in the last section.II.BACKGROUNDA. color space conversionThe choice of the color space can be a very important decision which can dramatically influence the results of the segmentation. Many images are stored with RGB format, so it is easier for the subsequent of the RGB color space is used. The main disadvantage of the RGB color space in applications with natural images is a high correlation between its components: about 0.78 for r BR (cross correlation between the B and R channel), 0.98 for r RG and 0.94 for r GB [7]. It makes the choice of the RGB threshold very difficult. Another problem is the perceptual non-uniformity, such as the low correlation between the perceived difference of two colors and the Euclidian distance in the RGB space. In this paper, we choose CIE L *a *b * color space to work on due to its three major properties:(1) Separation of achromatic information from chromatic information; (2) uniform color space, and (3) similar to human visual perception [8].The definition of CIE L *a *b * is based on nonlinearly-compressed CIE XYZ color space coordinates, which is derived from RGB as follows equation (1):⎥⎥⎥⎦⎤⎢⎢⎢⎣⎡⎥⎥⎥⎦⎤⎢⎢⎢⎣⎡=⎥⎥⎥⎦⎤⎢⎢⎢⎣⎡B G R Z Y X 5943.50565.00000.00601.05907.40000.11302.17517.17689.2 (1)Based on this definition, L *a *b * is defined as follows:⎪⎪⎪⎪⎪⎪⎭⎪⎪⎪⎪⎪⎪⎬⎫⎪⎪⎪⎪⎪⎪⎩⎪⎪⎪⎪⎪⎪⎨⎧⎥⎥⎥⎦⎤⎢⎢⎢⎣⎡⎟⎟⎠⎞⎜⎜⎝⎛−⎟⎟⎠⎞⎜⎜⎝⎛=⎥⎥⎥⎦⎤⎢⎢⎢⎣⎡⎟⎟⎠⎞⎜⎜⎝⎛−⎟⎟⎠⎞⎜⎜⎝⎛=−⎟⎟⎠⎞⎜⎜⎝⎛=3131*3131*31*20050016116n n n n n Z Z Y Y b Y Y X X a Y Y L (2) Where⎪⎩⎪⎨⎧+>=otherwise q q if q q f ,11616787.7008856.0,)(31(3)()nn nZY X,, represents a reference white asdefined by a CIE standard illuminant, in this case, andare obtained by setting 100===B G R in equation(1) ⎟⎟⎠⎞⎜⎜⎝⎛⎭⎬⎫⎩⎨⎧∈n n n Z Z Y Y X X q ,,.B. Visible color differenceRecent researches indicate that most of color image segmentation algorithms are very sensitive to color difference calculation or color similarity measure [9]. It is safe to say that the accuracy of color difference calculation determines the performance of various color image segmentation approaches.The perceptual difference between any two colors can be ideally represented as the Euclidean distance between their coordinates in the CIE L *a *b * color space, and are considered perceptually distinguishable [10].In CIE L *a *b * color space, the L * is brightness degree in meter system, and a *, b * is color degree in meter system. Thus, the brightness degree difference between (1*1*1*,,b a L )and (2*2*2*,,b a L )is2*1**L L L −=∇, The difference of chroma is22*22*21*21**b a b a C +−+=∇ , and thedifference of hue is⎟⎟⎠⎞⎜⎜⎝⎛−⎟⎟⎠⎞⎜⎜⎝⎛=∇−−2*2*11*1*1*tan tan a b a b H . Overall Colour difference can be expressed by thegeometry distance of space:2*2*2**)H ()C ()L (HH C C L L S K S K S K E Δ+Δ+Δ=∇ (4) The parametric factors K L , K C and K H are set forcorrecting the variations contributed from experimental or background conditions. To compute the CIE94 color difference 94*E∇, the following parameter valves areset for *E ∇ in (4):*H *C L H C L 015.01S ,045.01S 1S 1,K K K C C +=+===== (5)0*>∇L , it shows the sample color is paler andhigher in brightness compared the standard color. Contrarily it is low.0*>∇C , it shows the sample color is partial redcompared the standard color. Contrarily it is partialgreen.0*>∇H , it shows the sample color is partialyellow compared the standard color. Contrarily it is partial blue.The unit of chromatism is NBS (the abbreviation of National Bureau of Standards, then it has a NBS chromatism unit when 1*=∇E ). A 24-bit color image contains up to 16 million colors. Most of the colors can not be differentiated by human beings, because humanAutomatic Image Segmentation Base on Human Color Perceptions 27eyes are relatively less sensitive to colors. Y.H.Gong’s [11]research show there is close relation between the human color perception and the NBS color distance, The NBS color distance is devised through a number of subjective color evaluation experiments to better approximate human color perception, which is shown in table.1. Moreover, the values of E*ab can be roughly classified into four different levels to reflect the degrees of color difference perceived by human. The color difference is hardly perceptible when E*ab is smaller than 3.0; is perceptible but still tolerable when E*ab between 3.0 and 6.0; and is usually not acceptable when E*ab is larger than 6.0. Hence, in this paper, we define a color difference to be “visible” if its E*ab value is larger than 6.0.T ABLE .1 THE CORRESPONDENCE BETWEEN THE HUMANCOLOR PERCEPTION AND THE NBS UNITSNBS unitHuman perception <3.0 Slightly different 3.0~6.0 Remarkably different But acceptable 6.0~12.0 very different 12.0~Different colorC. Color Gradient:boundary edge The color gradient is used for a gradual blend of color which can be considered as an even gradation from low to high values. Mathematically, the gradient of a two-variable function (here the image intensity function) is at each image point a 2D vector with the components given by the derivatives in the horizontal and vertical directions. At each image point, the gradient vector points in the direction of largest possible intensity increase, and the length of the gradient vector corresponds to the rate of change in that direction.Wesolkowski compared several edge detectors in multiple color space, and he draw the conclusion that the performance of Sobel operator is superior to others [12]. So Sobel operator is used in this paper, Figure.1 shows the proposed boundary Sobel operator masks: (a) horizontal mask and (b) vertical mask. To construct a color contrast gradient, the eight neighbors of each pixel are searched to calculate the difference of lightness and the color-opponent dimensions with each neighbor pixel. Here the color difference replaces the color contrast gradient, and E ∇ denotes the color difference between two pixels, which is determined by equation (4). Let V x , V y denotes the gradient along x and y direction respectively. And the 3*3 mask is showed in Figure.2.-1 0 1 -2 0 2 -1 0 2-1 -2 -10 0 0 1 2 1(a)(b)Figure.1 Boundary Sobel operator masks:(a) horizontal mask, (b) vertical maska 1 a 2 a 3 a 4 (x ,y )a 5 a 6a 7a 8Figure.2 3×3 neighborhood region()()()()()382716321876,,2,22a a E a a E a a E a a a a a a v x ∇+∇+∇=++−++=(6)()()()()()684513641853,,2,22a a E a a E a a E a a a a a a v y ∇+∇+∇=++−++=(7)Then the magnitude of the Sobel operator can becalculated as follows:22y x v v G += (8)As Based upon this definition of color contrast gradient, it is possible to build up the following color edge detection application.The conventional region-edge integrating algorithm stores the edge information on the pixel itself. When there are different regions on both sides of a certain edge, however, it is necessary to decide which region the edge pixel itself belongs to, and this makes the algorithm cumbersome. In this paper, we propose to store the edge information not on a pixel itself but on a boundary between pixels [13,14]. We define the boundary pixel as the pixel that virtually exists between two real pixels and that has an infinitesimal width. The advantages of using the boundary pixels to keep the edge information, which we call the boundary edge, are as follows: (1) the control of region growth becomes easier and (2) the whole algorithm becomes simpler. In this paper set the threshold T tofilter the low gradient and only store the boundary pixel with high gradient.III.PROPOSED ALGORITHMFigure.3 has shown the architecture of the proposed algorithm. Firstly, an input color image is conversed from RGB color space into CIE L *a *b * color space, and choose a block size n n ×. The block size should be just small enough that the eye will average color over the block. Divide the input image into small and non-overlapping rectangular block, calculate mean and variance of range blocks, we need to construct a suitable method to display the data in order to investigate relationships of the regions. The data can be modeled using an (N, P) matrix, where N is the total number of blocks in the image, and P is the total number of variables that contain information about each block. The range blocks can classify two different blocks, we apply region growing and visible color difference to obtain initial seed regions. Thirdly, the color sobel detector is28 Automatic Image Segmentation Base on Human Color Perceptionsused to detect the texture blocks, using the acquired L*a*b* data, the magnitude of the gradient ()j i G,of the color image field is calculated. Fourthly, the initial seed regions are hierarchically merged based on the color spatial and adjacent information; the segmented color image is outputted at last. The rest parts of this section will discuss the details.A.C LASSIFICATION WITH V ARIANCE AND M EANIn this paper, the block variance and mean are the methods to classify. Blocks variance is usually used to classify the simplicity or complexity for each block. The average color value () of block is defined as:∑∑===ninjijixnnx11*1(8) The variance variance value()vbvavl,,of block is defined as follows:()∑∑==−=ninjiijxxxnn11*1δ (9)Where n is the size of the block and ix(()***,,b a Lxi∈) is the pixel value of the range blocks.The image blocks are classified into two different groups according to variance difference: one is monotone color block; the other is texture and edge block there is a variety of color. The variance value of monotone color block is very small, same time texture block is very big because of the edge, the variance value can set the threshold T to distinguish between two different types of image block, the value 0.05 is selected as the threshold based on our experiments, if a higher value is used, a smaller number of pixels will be classified as homogenous color blocks and some objects may be missed; oppositely, a higher number of pixels will be classified as texture block and different regions may be connected. Figure.4 has show the image block classification sketch map.○○○×○×○×○Figure. 4 image block classification sketch mapB.I NITIAL S EED R EGIONS G ENERATATIONAfter the segmentation of block partitioning, there will be a problem of over-segmentation. Those small over segmented regions should be merged into a large region to get the initial seed regions, to satisfy the need of image analysis and other follow-up treatment. The initial seed regions are generated by detecting all monotone color block in the image. In order to prevent multiple seed generation within monotone and connected image blocks, the first requirement is to enforce that seeds region be larger than 0.25% of the image. The reason of this rule is that the tiny region often is blotted region. The second requirement is toenforce the color different distanceijE∇>6.0 any of seed regions. It is briefly described as follows:1) Select monotone color block within the image;2) Detect adjacent monotone color block, calculate the color different distance between candidate and itsnearest neighbor seed region, ifijE∇<6.0, merge regions to existent seed region, else add a new seed region.3)Detect non-adjacent monotone color blocks, calculate the color different distance between candidateand its nearest neighbor seed region, ifijE∇<6.0, mergeAutomatic Image Segmentation Base on Human Color Perceptions 29regions to existent seed region, else add a new seed region.4) Scan the runs, and assign preliminary labels and recording label equivalences in a local equivalence table. After previously processed, there are totally m different color seed regions {}m S S S S L 321,,, they corresponding n homogeneous color regions, a color set may remark one or more color blocks that aren’t adjacent in spatial location, label the homogeneous color blocks with its own sequence number to declare the initial seed regions, record as {}n B B B L ,2,1.C. F UZZY REGION -G ROWING A LGORITHMThe number and the interior of the regions are defined after marker extraction. However a lot of undecided pixels are not assigned to any region. Most of them are located around the contours of the regions. Assigning these pixels to a given region can be considered as decision process that precisely defines the partition. The segmentation procedure in the present investigation is a fuzzy region-growing algorithm that is based on a fuzzy rule. Our final objective is to split an original image I into a number of homogeneous but disjoint regions R j by one-pixel width contours:k j R R R I k j nj j ≠===I U φ,1(10)The region growing is essentially a grouping procedure that groups pixels or sub regions into larger regions in which the homogeneity criterion holds. Sarting from a single seed region, a segmented region is created by merging the neighboring pixels or the adjacent regions around a current pixel. The operations are repeatedly performed until there is no pixel that does not belong to a certain region.Since our strategy in segmenting natural color images is an effective combination of an accurate segmentation by the color difference and the color gradient, and a rough segmentation by the local fractal dimension feature, it is inevitable to employ a technique for information integration. We adopt fuzzy rules to integrate the different features. We use the following criteria where each fuzzy rule has a corresponding membership function.[Rule 1] the first image feature is the color difference between the average value g ave (R k ) of a region and the value of a pixel g(i,j) under investigation:()()j i g R g E k ave ,−=∇ (11)The corresponding fuzzy rule for fuzzy set SMALL is R1: If color difference is small then probably merge else probably not merge.[Rule 2] The second intensity feature is the color contrast gradient, or the value of the boundary edges between the pixel and its adjacent region. A new pixel may be merged into a region if the gradient is low. If the gradient is high, the pixel will not be merged. Weemploy the boundary Sobel operator to calculate the color gradient and to achieve an accurate segmentation at the strong-edge regions. The boundary edges effectively protect the unnecessary growth of regions around the edges. The fuzzy rule for fuzzy set LOW becomes:R2: If gradient is low then probably merge elseFigure.4 shows the two membership functions corresponding to each fuzzy rule. After the fuzzification by the above two rules, min-max inference takes place using the fuzzy sets shown in Figure.5. Then the conventional centroid defuzzification method is applied. A pixel is applied. A pixel is really merged when the homogeneity criterion is satisfied to an extent of 50% after defuzzification.The fuzzy region growing processing is briefly described as follows:1) For one of the initial seed regions {}m B B B L ,2,1, first from the upper left corner of the adjacent texture blocks, seeked the pixels that has thesame color and features, and merged them into the seek region. When the adjacent sub-block has been accepted, the seed region grows as a new seed region, updated the new seed region’s features. Repeated the process until the near of sub-blocks have not acceptable, the suspension of the process of growth.2) Repeat (1) until all seed regions growing are accomplished.D. M ERGE S MALL R EGIONSThough over-segmentation problem is diminished through above procedures, in most case a further process of eliminating the small regions is still required to produce the more clear objects or homogenous regions. In our algorithm, those small regions occur often along the contour of objects or inside a complex region due to compound texture. Thus, the merger of the region aimed at the effective removal of noise and image detail will be merged into the connected area.The merged processing is given as follows.1) The tiny region is regarded as bloted region andto be filtered;30 Automatic Image Segmentation Base on Human Color Perceptions2)The other fragmented region is merged base onthe spatial location relation with the adjacentregion.The relation of spatial location between objects isgiven as follows. If an object were entirely surroundedby another object, or at least three directions, as shownin figure.6 and figure.7, that belongs to an interior object,we merged it into peripheral region. If the target is notsurrounded by the other object, as shown in Figure.8, itwas regarded as a border and merged with theconditions of edge.1 1 1 1 1 1 12 1 1 1 1 2 2 1 1 2 2 1 1 1 1 1 1 1 Figure.6 An object was entirelysurrounded byanother object1 1 1 1 21 12 1 21 12 2 21 2 2 1 21 1 1 1 2Figure. 7 An objectwas surrounded byanother object fromthree directions1 1 3331 1 2331122312 2331 1 133Figure.8 A smallobject located in twoobjectsFinally, if the number of pixels in a region is lowerthan a given smallest-region threshold that is determinedby the size of image, we will merge this region into itsneighborhood with the smallest color difference.This rule is the last step in our segmentationalgorithm. Based on our experiments, we select 1/150 ofthe total number of pixels of the given color image asthe threshold. This procedure repeats until no region hassize less than the threshold. Finally, a segmented imageis produced.IV.E XPERIMENT SIMULATION AND RESULT ANALYSISTo verify the performance of the proposedsegmentation algorithm, we experiment with colorremote sensing images and natural images. We showresults of some of these experiments. Figure.9 andFigure.10 show some typical results of our color imagesegmentation algorithm.In fact, we can compare our algorithm andtraditional SRG algorithm here. Our method use regionrather than pixel as initial seeds. In this sense, high-levelknowledge of the image partitions can be exploitedthrough the choice of the seeds much better becauseregion has more information compared to pixels.(a) (b)(c) (d)(e)Figure 9. fabric image segmentation experimental results(a) Original image (b) color contrast gradient(c) Green object (d) Purple object(a) (b)(c) (d)Figure 10. capsicum image segmentation experimental results(a) Original image (b) color contrast gradient(c) Red capsicum (d) Green capsicum(a) (b)Automatic Image Segmentation Base on Human Color Perceptions 31(c) (d)(e)Figure 11. capsicum image segmentation experimental results(a) Original image (b) color contrast gradient(c) Red capsicum (d) yellow capsicum (e)green capsicumV.C ONCLUSIONThis work presents a computationally efficientmethod designed for automatic segmentation of colorimages with varied complexities. Firstly, the originalimage is divide into rectangular image blocks which arenot overlapped; then, the mean and variance of eachimage black was calculated in CIE L*a*b*color space,and the image blocks were divided into homogeneouscolor blocks and texture blocks by the variance of it.The Initial seed regions are automatically selecteddepending on calculating the homogeneous colorblocks’ color difference in CIE L*a*b*color space andspatial and adjacent information. The color contrastgradient of the texture blocks need to calculate and theinformation of boundary pixels are storage. Finally, theregion growing and merging algorithm was to use andachieve the segmentation result.The meaningful experiment results of color imagesegmentation hold favorable consistency in terms ofhuman perception and satisfy the content-based imageretrieval and recognition process. There are mainly twodisadvantages in our algorithm. First, although using thefixed threshold values can produce reasonably goodresults, it may not generate the best results for all theimages. Second, when an image is highly color textured(i.e. there are numerous tiny objects with mixed colors),our algorithm may fail to obtain satisfied results becausethe mean value and variance could not represent theproperty of the region well. How to combine otherproperties such as texture into the algorithm to improvesegmentation performance is the point of our furtherresearch.A CKNOWLEDGMENTThis paper is fully supported by theNational Science and Technology InfrastructureProgram of China (No.13001790200701).R EFERENCES[1] ZHANG YU-JIN. Image project(media), image analysis.Beijing. Tsinghua University Press, 2005[2]H. Cheng, X. Jiang, Y. Sun and J. Wang, Color imagesegmentation: Advances & prospects, Pat. Rec., Vol. 34, No.12, pp. 2259-2281, Dec. 2001.[3] J. Wu, H. Yan, and A. Chalmers, “Color imagesegmentation using fuzzy clustering and supervised learning”,Journal of Elec. Imag., Vol. 3, No. 4, pp. 397–403, Oct. 1994.[4] P. Schmid, Segmentation of digitized dermatoscopicimages by two-dimensional color clustering,IEEE Trans. onMed. Image., Vol. 18, No.2, pp. 164–171, Feb. 1999.[5] Daily, M.J., J.G. Harris, K.E. Olin, K. Reiser, D.Y. Tseng,and F.M. Vilnrotter, Knowledge-based Vision TechniquesAnnual Technical Report. U.S. Army ETL, Fort Belvoir, VA,October, 1987.[6]Healey, G. and T. Binford, The Role and Use of Color in aGeneral Vision System. Proc. of the DARPA IU Workshop,Los Angeles, CA, pp. 599-613, February, 1987.[7]GONG Sheng-rong, Digital image processing and analysis.Beijing. Tsinghua Unversity Press, 2005[8]G.Wyszecki and W.Stiles, Color Science: Concepts andMetheds, Quantitative Data and Formulae, 2nd ed. NewYork: Wiley, 1982.[9]H.D. Cheng, X.H. Jiang, Y. Sun, et al. “Color imagesegmentation: advances and prospects”. Pattern Recognition,2001, pp. 2259- 2281[10] Qi Yonghong and Zhou Shshenqi, “Review on Uniformcolor space and Color Difference Formula”, Print World,2003.9:16-19.[11] Gong Y.H, Proietti G. Image indexing and retrievalbased on human perceptual color clustering. The internationalconference on computer vision, Munbai, 1998[12]S. Wesolkowski, M.E. Jernigan, R.D. Dony, “Comparisonof color image edge detectors in multiple color space”. ICIP-2000, pp. 796 – 799[13]J. Maeda, V.V.Anh,T.Ishizaka and y. suzuki, “ Integrationof local fractal dimension and boundary edge in segmentingnatural images” , Proc. IEEE Int. Conf. on Image Processing,vol.I, pp.845-848, 1996.[14]J. Maeda, T. Lizawa, T. Ishizaka, C. Ishikawa and Y.Suzuki, “Segmentation diffusion and linking of boundaryedges”, Pattern Recognition, vol.31(12), pp.1993-1999, 1998.[15] Ye Qixiang, Gao Wen, Wang Weiqiang, Hang Tiejun.“A Color Image Segmentation Algorithm by Using Color andSpatial Information”. Journal of Software. 2004, 15(4):522-530.[16] Hsin-Chia Chen, Sheng-Jyh Wang. “The use of visiblecolor difference in the quantitative evaluation of color imagesegmentation”. Vision, image and signal processing, IEEEproceedings. 2006, Vol.153, pp.598 - 609.YU Li-jie, female, is a Lecturer at the College ofAutomation Beijing Union University. She is currentlyworking toward the Ph.D. degree in the Mechanical &Electronic Technology Research Center majoring in Test &Control Technology in the Beijing University of Technology.Her research interests include computer vision, digital imageprocessing and Software Development. Her teaching interestsinclude digital image processing, Object-Orientedprogramming, and web database technology.。
基于RGB三分量亮度法线相似度的立体匹配算法
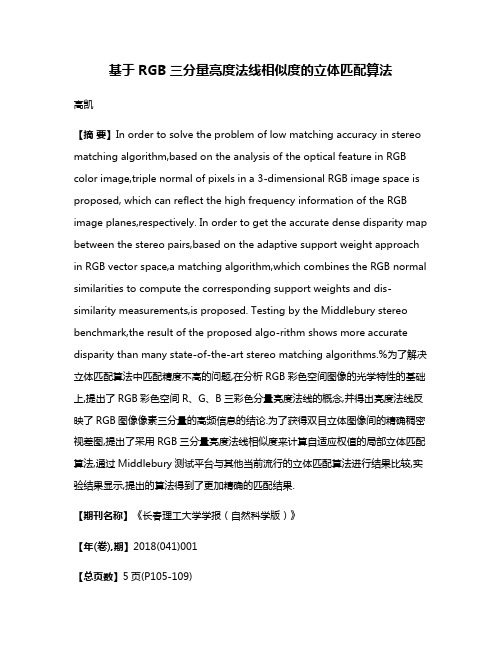
基于RGB三分量亮度法线相似度的立体匹配算法高凯【摘要】In order to solve the problem of low matching accuracy in stereo matching algorithm,based on the analysis of the optical feature in RGB color image,triple normal of pixels in a 3-dimensional RGB image space is proposed, which can reflect the high frequency information of the RGB image planes,respectively. In order to get the accurate dense disparity map between the stereo pairs,based on the adaptive support weight approach in RGB vector space,a matching algorithm,which combines the RGB normal similarities to compute the corresponding support weights and dis-similarity measurements,is proposed. Testing by the Middlebury stereo benchmark,the result of the proposed algo-rithm shows more accurate disparity than many state-of-the-art stereo matching algorithms.%为了解决立体匹配算法中匹配精度不高的问题,在分析RGB彩色空间图像的光学特性的基础上,提出了RGB彩色空间R、G、B三彩色分量亮度法线的概念,并得出亮度法线反映了RGB图像像素三分量的高频信息的结论.为了获得双目立体图像间的精确稠密视差图,提出了采用RGB三分量亮度法线相似度来计算自适应权值的局部立体匹配算法,通过Middlebury测试平台与其他当前流行的立体匹配算法进行结果比较,实验结果显示,提出的算法得到了更加精确的匹配结果.【期刊名称】《长春理工大学学报(自然科学版)》【年(卷),期】2018(041)001【总页数】5页(P105-109)【关键词】立体匹配;自适应权值;RGB亮度法线;RGB彩色空间【作者】高凯【作者单位】长春理工大学电子信息工程学院,长春130022【正文语种】中文【中图分类】TP391.41稠密双目立体匹配是计算机视觉领域中广泛研究的问题之一。
基于加权视觉色差的三维色域匹配算法

Ab ta t A h e — i n in l src : t r ed me so a GM A ( a u p ig ag rt m )i i h ih e iu l oo g m tma pn lo i h n whc t eweg td vs a lr h c
t o d m e i na m utm a pi g. w i nso lga p n
Ke w r s h e — i n i n l a t ma p n y o d :t r e d me so a g mu p i g; w eg t d i u l c l r i e e c i h e v s a o o d f r n e; b r c n r f a y e ti c
基于加权 视觉色差 的三维色域 匹配算法
穆 宝 忠 , 玉 玲 , 飞鸿 刘 余
( 浙江人学 光电系现 代光学仪器目家 点实验 室 , 浙 杭州 3 0 2 ) 1 0 7
摘要 : 出 了以加 权 视 觉 色差 为评 价标 准 的三 维 色域 匹配算 法 。加权 视 觉 色差 公 式 由 提
MU o z o g, U — i g , Ba h n II Yu ln YU ih n Fe o g ( tt K y I b r tr f d r p i l n tu n a i ,Z ei gUn v ri , t n z o 1 0 7 Chn ) S ae e a o a o yo Mo e n O t a l sr me tt n h j n ie s y ta g h u 3 0 2 , ia c o a t
C E1 9 色差 公 式 引 申 而 出 , 据 权 重 系 数 在 色差 边 界 的 GB 矩 阵 内确 定 了动 态 的 空 间 I 94 根 D
基于注意力机制的立体匹配算法
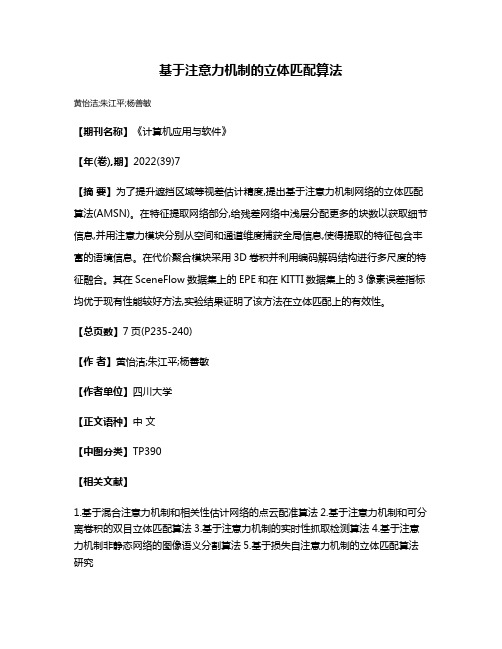
基于注意力机制的立体匹配算法
黄怡洁;朱江平;杨善敏
【期刊名称】《计算机应用与软件》
【年(卷),期】2022(39)7
【摘要】为了提升遮挡区域等视差估计精度,提出基于注意力机制网络的立体匹配算法(AMSN)。
在特征提取网络部分,给残差网络中浅层分配更多的块数以获取细节信息,并用注意力模块分别从空间和通道维度捕获全局信息,使得提取的特征包含丰富的语境信息。
在代价聚合模块采用3D卷积并利用编码解码结构进行多尺度的特征融合。
其在SceneFlow数据集上的EPE和在KITTI数据集上的3像素误差指标均优于现有性能较好方法,实验结果证明了该方法在立体匹配上的有效性。
【总页数】7页(P235-240)
【作者】黄怡洁;朱江平;杨善敏
【作者单位】四川大学
【正文语种】中文
【中图分类】TP390
【相关文献】
1.基于混合注意力机制和相关性估计网络的点云配准算法
2.基于注意力机制和可分离卷积的双目立体匹配算法
3.基于注意力机制的实时性抓取检测算法
4.基于注意力机制非静态网络的图像语义分割算法
5.基于损失自注意力机制的立体匹配算法研究
因版权原因,仅展示原文概要,查看原文内容请购买。
一个基于着色和动态规划的3-维匹配问题算法

计算机科学 2 0 V 13 №. 0 7 o. 4 7
一
个 基 于着 色和 动态 规 划 的 3维 匹配 问题 算 法 ) 一
宁 丹 王建 新
( 中南大学信 息科 学 与工程 学 院 长 沙 40 8 ) 1 03
摘 要 3维 匹配 问题是六个经典 的 N 一 P完全 问题之 一 , 调度 、 配 、 在 分 交通和 网络流 等 问题 方面有很 强的应 用。参 数 计算理论是近 年来发展起 来的研 究和解决 N - 问题 的新方 法。针 对 3维 匹配 问题 , P难 一 目前确 定式参数 算法的 最好 结果是 O (6 ) 1强 。本文结合着 色和动 态规划技 术 , 出了一个算法运行 时间为 0 (.2 ) 提 34强 的确 定式参数 算法 , 大大
3维匹配问题 (一 i e s n l thn rbe 简称 3 一 3D m n i a Macigpo l o m, 一 D Macigpo l 是 图的 匹配 问题 中的一 个很 典 型 的问 t n rbe h m)
一
组并且这 k个三元组 中没有两个符号在任意一个坐标上 是相
c n l a ty i r v s t e p e i u e td t r i it l o i m. mp o e h r vo s b s e e m n s i ag rt c h Ke wo d 3 Di n i n 1 t h n , o o o i g y rs 一 me so a ma c i g C l rc d n ,Dy a cp o r mmi g n mi r g a n
网络流等方面有很强 的应用 。下面首先给 出问题 的定义 ¨ : 1 ] 定义 1 图 的 匹 配 问题 ) 给 定 一 个 图 G一 ( ( , ( G) E ( ) ( 表示 图 G 中点 的集 合 , G) 示 图 G 中边 的集 G) , G) E( 表 合 。图 G 的一个匹配 P( 是 E( 的一个 子集 , P( 中 G) G) 且 G) 任何两条边都 不存 在公 共点 。如果 匹配 P( 中的边可 以覆 G) 盖 图 G的每一个点 , 则该 匹配 是完美 匹配。
基于立体图对的颜色校正算法设计

基于立体图对的颜色校正算法设计
栾亚群;李淑英
【期刊名称】《科学技术与工程》
【年(卷),期】2014(014)013
【摘要】基于多摄像机的视觉应用中通常假设统一的颜色响应.但是当摄像机之间存在较大的成像特性差异或光照变化时,所获得的立体图对间就会出现严重的色彩差别.这种差别会导致后续颜色匹配的不准确,并进一步影响立体视觉算法的性能.为了解决这个问题,提出了一个鲁棒的基于图像分割和特征点匹配的颜色校正算法.和传统的全局校正或者有参考物体的校正算法不同,提出了一种基于区域的校正算法.该方法不仅避免了全局校正算法无法满足局部需求的矛盾,同时也摆脱了设置参考物体的复杂和低效.大量实验证明了所提出算法的有效性和鲁棒性.
【总页数】10页(P71-79,95)
【作者】栾亚群;李淑英
【作者单位】长安大学电子与控制工程学院,西安710064;航天科技集团第16研究所,西安710100
【正文语种】中文
【中图分类】TP391.41
【相关文献】
1.基于ArcEngine的灾害地质立体图图切剖面算法设计与实现 [J], 裴丽娜;孔春芳;刘刚;吴勇;乔立锦;张军强
2.基于颜色校正和去模糊的水下图像增强方法 [J], 魏冬;刘浩;陈根龙;宫晓蕙
3.一种基于图像RGB通道混合的颜色校正算法 [J], 罗天;王毅;袁霞
4.立体图像视差匹配算法设计与实现 [J], 蒋里;季晓勇
5.基于ICC阶调调整的陶瓷喷墨印刷颜色校正 [J], 付文亭;梁立霖
因版权原因,仅展示原文概要,查看原文内容请购买。
一种基于直方图匹配的立体视频颜色校正算法
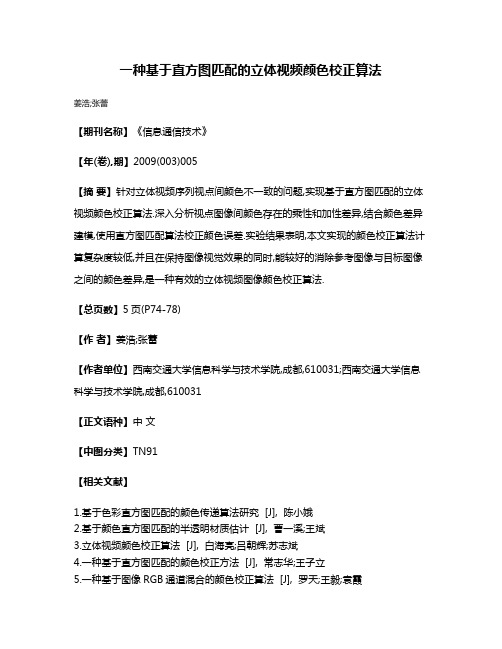
一种基于直方图匹配的立体视频颜色校正算法
姜浩;张蕾
【期刊名称】《信息通信技术》
【年(卷),期】2009(003)005
【摘要】针对立体视频序列视点间颜色不一致的问题,实现基于直方图匹配的立体视频颜色校正算法.深入分析视点图像间颜色存在的乘性和加性差异,结合颜色差异建模,使用直方图匹配算法校正颜色误差.实验结果表明,本文实现的颜色校正算法计算复杂度较低,并且在保持图像视觉效果的同时,能较好的消除参考图像与目标图像之间的颜色差异,是一种有效的立体视频图像颜色校正算法.
【总页数】5页(P74-78)
【作者】姜浩;张蕾
【作者单位】西南交通大学信息科学与技术学院,成都,610031;西南交通大学信息科学与技术学院,成都,610031
【正文语种】中文
【中图分类】TN91
【相关文献】
1.基于色彩直方图匹配的颜色传递算法研究 [J], 陈小娥
2.基于颜色直方图匹配的半透明材质估计 [J], 曹一溪;王斌
3.立体视频颜色校正算法 [J], 白海亮;吕朝辉;苏志斌
4.一种基于直方图匹配的颜色校正方法 [J], 常志华;王子立
5.一种基于图像RGB通道混合的颜色校正算法 [J], 罗天;王毅;袁霞
因版权原因,仅展示原文概要,查看原文内容请购买。
- 1、下载文档前请自行甄别文档内容的完整性,平台不提供额外的编辑、内容补充、找答案等附加服务。
- 2、"仅部分预览"的文档,不可在线预览部分如存在完整性等问题,可反馈申请退款(可完整预览的文档不适用该条件!)。
- 3、如文档侵犯您的权益,请联系客服反馈,我们会尽快为您处理(人工客服工作时间:9:00-18:30)。
I.J. Image, Graphics and Signal Processing, 2009, 1, 17-24Published Online October 2009 in MECS (/)The Calibration Algorithm of a 3D Color Measurement System based on the Line FeatureGanhua LiXi’an Satellite Control Center, Xi’an, ChinaEmail: liganhua@Li Dong, Ligong Pan and Fan HenghaiXi’an Satellite Control Center, Xi’an, ChinaEmail: donglixscc@Abstract—This paper describes a novel 3 dimensional color measurement system. After 3 kinds of geometrical features are analyzed, the line features were selected. A calibration board with right-angled triangle outline was designed to improve the calibration precision. For this system, two algorithms are presented. One is the calibration algorithm between 2 dimensional laser range finder (2D LRF), while the other is for 2D LRF and the color camera. The result parameters were obtained through solving the constrain equations by the correspond data between the 2D LRF and other two sensors. The 3D color reconstruction experiments of real data prove the effectiveness and the efficient of the system and the algorithms.Index Terms—3D reconstruction, extrinsic calibration, laser range finderI.I NTRODUCTIONThe laser range finder (LRF) has been widely used together with a camera placed on a platform in 3D measurement [1] [2], robot motion planning [3], navigation [4] and collision avoidance [5], however, for these systems, there are still some difficult problems needing to be solved like:1.It’s difficult to measure the object far from thesystem;2.It’s difficult to measure the object outdoor;3.It’s difficult to measure a complicated scene;4.It’s difficult to measure an object with big sizeshape;5.It’s difficult to establish a colorful 3D model.For an existed 3D measurement system to solve all the problems above; the price is very high and the arts and crafts are very complicated, which means it is not fit for the common applications. For common applications, a 3D color measurement system was established based on color camera, the 2D laser range finder with invisible stripe and platform in this paper to be used in SLAM (Simultaneous Localization and Mapping) problem study.The sensors and corresponding data processing board are shown as Fig 1. As shown as Fig.1 (a), the camera is UC-800 (color 1024*768 pixels) made by UNIQ (America), and the corresponding data processing board is Meteor-II/Digital made by Matrox (America); as shown as Fig.1 (b), the LRF is LMS211 (360 spots, line scan, distance range [0,80]m, angle range[0,180]°) made by SICK (Germany), and the corresponding data board is CP132 made by Moxa (Taiwan); as shown as Fig1. (c), the platform is YA3050 made by Ya An(China), the corresponding switch is common switch form 485 protocol to 232 protocol.For the system, the critical problem is how to calibrate the 3 sensors precisely, which means to establish the homogenous transformation relationship of the datareference frames of the 3 kinds sensors. Because the LRF(a)(b)(c)Fig.1. The sensors and the corresponding data processing board.18 The Calibration Algorithm of a 3D Color Measurement System based on the Line Featureis more precise than the other sensors, we calibrate the system by calibrating the LRF with a platform and LRF with a camera. There is little reference about the calibration between LRF with invisible stripe and platform, because most 3D measurement applications [6] [7] uses the visible light spots, strip or pattern. But there are a few papers about the calibration between LRF and camera. Zhang and Pless presented a method for the extrinsic parameters calibration [8] based on the constraints between “views” of planar calibration patterns from a camera and LRF in 2004. But since the angle between the LRF’s slice plane and the checkerboard plane will affect the calibration errors, this method needs to put the checkerboard with a specific orientation, which is not easily established. Since the method is based on the data correspondence between the 3D range data and the 3D coordinates estimated by multi-views of the camera, the estimation errors of the 3D coordinates will affect the calibration result also. Bauermann proposed a “joint extrinsic calibration method” [9] based on the minimization of the Euclidean projection error of scene points in many frames captured at different view points. However, this method is only suitable for a device with the specific crane, and it establishes the correspondence between range and intensity data manually, which is also not easily established. Wasielewski and Strauss proposed a calibration method [10] mainly based on the constraints of projection of the line on the image and the intersection point of the line with the slice plane of the range finder in the world coordinate system. But the angle between the line and the slice plane of the LRF for different positions affects the calibration errors.In order to solve all the problems presented above, a 3D measurement system was established as shown as Fig. 2. Firstly this paper analyzed 3 common geometrical features, and chose the line feature and designed right-angled triangular checkerboard. Secondly, we presented the calibration algorithms of 2D LRF, color camera and platform. Lastly, we described the experiments of the algorithms and the 3D color model reconstruction.II. F EATURES A NALYSIS AND C HECKERBOARD D ESIGN To calibrate the extrinsic parameters, a set of featuresmust be selected on a calibrated object and a set of constraints equations are established by associating the measurement data of the features of different sensors. The key issues include feature selection, derivation and solution of the constraint equations.Three possible features: points, lines and faces, can be selected for the calibration. Consider the constraints on the extrinsic parameters when different features are used. First, if a point feature is used, the Position of the Camera or Platform (P-CP) is constrained on the surface of a sphere [2] [6] when the coordinates of the point with respect LRF coordinate frame is measured, provided that the measurement error is zero. When the measurement error is not zero, the camera is bounded to a spherical ring region. As shown in Fig. 3 (a), given the 3-D coordinates of the point measured by the laser range finder, the center O of the sphere is at the feature point and the radius d is the range measured by the LRF. For multiple feature points, P-CP is constrained to the intersection of the spherical surfaces. Second, consider the case when line features are used. As Fig. 3 (b) shows, P-CP is constrained in the inner space of the cylinder whose axis L is the line and radius is the measurement distance d of the LRF. For multiple line features, P-CP must be constrained in the intersection of the inner space of the cylinders. Finally, as shown in Fig. 3 (c), when a face feature is employed, P-CP is in the space between the measured plane A and the plane that is parallel to the face measured by the LRF and is apart from the face by the measured distance d . For multiple face features, the position of the camera is in the intersection of the spaces.Obviously, point features lead to the smallestintersection space for constraining the camera’s position,(a)(b)(c)Fig. 3. Three possible features.Fig. 2. The specific checkerboard is posed in the both views of the cameras and the laser range finder.and the constraints imposed by face features are the loosest. However, the light of the LRF is not visible, so it is impossible to use point features to establish the correspondence. Compared to face features, it is simpler and superior to establish correspondence between line features in the two different sensors. Lines can be easily detected by the camera. The intersection point of a line with the laser slice plane can also be easily detected by checking the changes in depth. Therefore, we adopted to use line features and design a checkerboard with right-angled triangular shape (Fig.2). The checkerboard is black so as to be easily detected in the image. At a given position and orientation of the checkerboard, we detect the projections of the two perpendicular edges of the camera and their intersections with the laser slice. Then, we establish the constraint equations by associating the intersection points with the projection. The use of a right-angled triangle is because of the fact that the intersection of the inner space of two cylinders is the smallest when they are perpendicular to each other. And for every different positions, the checkerboard is detected with two near perpendicular poses in a position as shown as the lower two checkerboards of Fig. 2. Therefore it is convenient to generate two pairs of perpendicular lines with great variety of rotation for every position.III. C ALIBRATION B ETWEEN LRF AND P LATFORM A. Problems DefinationIf t and Φ are the transition vector and the rotation vector and R is the corresponding rotation matrix, we could transform the coordinate L P of the point P in LRF Coordinate Frame (LRFCF) to the coordinate in Original Platform Coordinate Frame (OPCF), which means the coordinate frame before rotation. Because the platform just rotates up and down in pitching direction, so we could define the origin of the axis Y of platform cross the origin of the LRF coordinate frame. So we can establish (1), (2) and (3) to reduce the parameters.0, , 0Ty t t ⎡⎤=⎣⎦ (1) , , Tx y z ⎡⎤Φ=ΦΦΦ⎣⎦ (2)PL P R P t =×+ (3)We can also estimate (4) and (5) to transform the coordinate of OPCF of point P from the coordinate in Rotation Platform Coordinate Frame (RPCF) of point P. Here w Φ is the rotation vector, and the w R is the corresponding rotation matrix. Then, from (1) to (5) we could establish (6).[], 0, 0Tw x Φ=Φ (4)w P P R P =× (5)()w L P R R P t =××+ (6)Because the w Φ could obtain from the platform’s rotation control parameters, and L P could be obtained from the laser range finder’s measurement. w R is corresponding w Φ. It will be presented how to obtain thet and Φ corresponding R .B. Constraint EquationsIf []1211,1,,1T n P P P P ′′′′=L and []1222,2,,2Tn P P P P ′′′′=L are coordinates of OPCF from the measurements of the perpendicular edges by equation (6), when the platform rotates up and down, we can establish the estimates from three property of the points sequence. • The linearity property: Because the points sequence 1P ′ and 2P ′ belong to the two line edges of the checkerboard, the errors of the line fitting for 1P ′ or 2P ′ are very small. After the two points sequences were projected to 4 groups points in coordinate frame XZ and YZ, we could fit the 4 groups points to 4 lines. (A1ZX, B1ZX), (A1YZ, B1YZ), (A2ZX, B2ZX) and (A2YZ, B2YZ) are the coefficients of the 4 lines. And the errors of the 4 line fitting are V1ZX, V1YZ, V2ZX and V2YZ, which could presents the linearity property of 1P ′ and 2P ′.X1111ZX Z YZ YZ x A z B z A y B =+⎧⎨=+⎩ (7) X2222ZX Z YZ YZx A z B z A y B =+⎧⎨=+⎩ (8) •The Perpendicular Property:From the coefficients of the 4 lines, we could alsoestablish the direction vectors 1u of 1P ′ and 2u of 2P ′. From the coefficients, we also could obtain coordinate P1 and P2 of the two fitted lines. Then we could establish V to present the Perpendicular Property. Here “·” means the scalar product of two vectors , and |…| means the absolute value.11[1 1 ]1ZX YZ u A A =, (9) 12[2 1]2ZX YZ u A A =, (10)11[1,,0]1YZ ZX YZ B p B A =− (11)22[2,,0]2YZZX YZB p B A =− (12)|12|V u u =• (13)•The Coplanarity Property:Because 1P ′ and 2P ′ belong to the same plane of the checkerboard, the distance between two fitted lines could presented the coplanarity Property of 1P ′ and 2P ′. So we could use the (9), (10), (11) and (12) to establish (14).Here “×” means the cross product of two vectors, and “||……||” means the norm of the vector.|(12)(12)|||12||u u p p D u u ו−=× (14)•The Constrain Equation:From the 3 properties, we can obtain the constrain equation (15). We solve the nonlinear optimizationproblem by using the Gauss Newton algorithm [11].222222i i i i i i i m in (V 1Z X V 1Y Z V 2Z X V 2Y Z +V +D )+++∑ (15) C. Calibration AlgorithmStep1: Set an initial value of Φ and t by a rough measurement or estimation.Step2: Set the checkerboard nearer than otherobjects in front of the laser range finder, with movements of the platform, obtain the laser range finder’s data and the range of the platform’s pitching angle for different checkerboard’s position. Step 3: Preprocess the laser range finder’s data, and establish the raw data sets for algorithm. (i) Detect the scanning points on checkerboard by the distance discontinuity.(ii) Use the median filter to delete the errorpoints.(iii) After the line fitting to obtain the endpoints of the fitted line, we could obtain the intersection point sets between checkerboard and the scanning planeand the corresponding platform rollingangle sets.Step 4: Transform the detected points coordinatesto platform reference frame by (6) to get the points sets 1P ′ and 2P ′.Step 5: Based on the 1P ′ and 2P ′, use (7), (8),(9), (10), (11), (12), (13) and (14) toestablish the property functions. The constraint function was established by (15).Step 6: By the Gauss Newton algorithm, the Φ and t could be obtained.IV. C ALIBRATION B ETWEEN LRF AND C AMERA A.. Problem DefinitionFig. 1 shows a setup with a laser range finder and a stereo vision system for acquiring the environment information. We will use this setup in the experiments. It is necessary to find the homogeneous transformation between every camera and the LRF in order to fuse the measuring of the camera and the LRF. Two coordinate frames, namely the camera coordinate frame (CCS) and the laser range finder coordinate frame (LRFCS), have been set up to represent the measurements with respect to the respective sensors. The objective here is to develop an algorithm for calibrating the orientation vector(,,)x y z θθθΦand the translation vector (,,)x y z t t t t of the camera with respect to the laser range finder. Let R be the rotation matrix corresponding to the orientation angles Φ. Denote the coordinates of a point p with respect to the CCS and LRFCS by C p and L p ,respectively. The coordinates are related as follows.C L =p Rp t + (16) The raw measurements of LRF are expressed in the polar coordinates on the slice plane as (, )ρθ, where ρ represents the distance and θ is the rotation angle. Thenthe coordinates L p is given by (17): []Tcos , sin , 0L l l ρθρθ=p (17)B. Constraint Equations: As Fig 1 shows, it denotes the two perpendicular edges of the checkerboard by lines AB and AC , respectively, and their intersections with the laser slice plane by points E and F , respectively. In the calibration, we use two kinds of measurement data obtained at different poses of the checkerboard. One kind of data is the projections of the two perpendicular lines ab and ac on the image planes, and the other is the measurements of theintersection points L E and LF in LRFCS.Assume that the camera is a pinhole camera. Given thedistortion coefficients 12345()k k k k k k ,,,, and theintrinsic parameter matrix K , a point ()C E E E x y z E ,, in the camera coordinate frame can be projected onto the point ()e ex y e , on the image plane by using (18) and (19)([12] and [13]), where (,1)d d x y , is the normalizedcoordinate including lens distortion in CCS. 24612522342234(1))2(2) (2)2d d x x k r k r k r y y k xy k r x k r y k xy ⎡⎤⎡⎤=+++⎢⎥⎢⎥⎣⎦⎣⎦⎡⎤+++⎢⎥++⎣⎦ EEE E x x z yy z r === (18)11e d e d x x y y ⎡⎤⎡⎤⎢⎥⎢⎥=⎢⎥⎢⎥⎢⎥⎢⎥⎣⎦⎣⎦K (19) As Fig. 1 shows, on the image plane, the projection ab of edge AB can be detected. L E is the point measuredby the LRF. e is the calculated projection of point L E by using (16) (17) (18) and (19). Then the distance()d e ab ,from the point e to the line ab can be calculated by using (20) [14].||||(,)||||eb ab d e ab ab ×=uu r uu ruu r (20)where “×” is the cross product of two vectors, ab uu vis thevector from point a to point b , eb uu vis the vector from point e to point b and ||…|| is the norm of the vector. Similarly, for the edge AC and intersection point F , we can also calculate the distance ()d f ac , from the calculated projection f of the point F to the projectionac of edge ACon the image plane. For different orientations and positions of the checkerboard, we obtain different distances. Introduce an index i to represent the distances at different orientations and positions. The calibration problem of the extrinsic parameters can be formulized as a problem of finding the optimal solution of the translation vector t and orientation angles Φ that minimize the sum of the distances as shown as (21).[]2min (,)(,)i i i i i i i d e a b d f a c ⎧⎫⎨⎬⎩⎭∑+ (21)Where the index i represents the i -th position and orientation of the checkerboard. We solve the nonlinear optimization problem by using the Gauss Newton algorithm [11]. The initial parameters used are values obtained by a rough measurement for the device. The initial parameters can also be obtained by the closed form solution proposed in [8]. C. Calibration Algorithm:Step1: Set an initial value of Φ and t by a roughmeasurement or estimation.Step2: For different checkerboard position, detectthe two point sets and project the points to image coordinate.(i) Detect the points in checkerboard based on the distance discontinuity of all scanning points.(ii) Use median filter to delete the errorpoints from the detected points.(iii) Use line fitting and obtain theintersection points of the scan plane and the checker board’s edge line from the fitting line’s end points.(iv) Use (16), (17) and (18) to transform thepoints from the laser range finder coordinates to camera coordinates.(v) Use (19) to transform the points fromcamera coordinates to the image coordinates.Step3: Detect the projections of the perpendicularedges of checkerboard on the image plane and calculate their coordination on the image plane.Step4: Based on the checkerboard’s vertexescoordinates in image reference frame and the laser range finder reference frame, use (20) and (21) we could establish the constrain functions.Step5: By the Gauss Newton algorithm, theΦand t could be obtained.V. E XPERIMENTAL R ESULTSAfter we proved the effectiveness by these experiments using these two algorithms, we presented the experiment of 3D color reconstruction of a complicated scene by the calibration results to show the system’s effectiveness. We also conducted experiments on the setup shown in Fig. 1. On a pan-tilt platform, two UNIQ UC-800 cameras with 1024*768 pixels are mounted with a SICK LMS221-30206 LRF, which can provide 100 degrees and maximum 80(m) measurements with the accuracy of ±50(mm).A.. The Experimental Results of LRF and PlatformAs Fig.4 shows, the measurement of the checkerboard was reconstructed to present the precision of calibration results between LRF and camera. The real sizes of the checkerboard’s edges are 315mm, 380mm and 491mm. From the measurement of reconstruction by MATLAB software, the sizes of 3D reconstruction model’s edges are 300.3633mm, 373.4127mm and 496.2991mm. Then the mean of the errors is 8.8410mm, which is better than the accuracy of LRF. So the calibration is effective.(a) the image of checkerboard(b) projection of X-Z Frame(d) projection ofX-Y Frame(c) projection ofY-Z FrameFig.4.3D reconstruction of the checkerboard.(e) 3D color reconstruction resultB. The Experimental Results of LRF and CameraAfter detecting the point sets on the checkerboard, we found that there are lot’s of error measure points near the checkerboard’s edges, because the laser range finder have the mixture pixel effect. Fig. 5 shows the mixture pixel effect and the preprocessing results of the original data to remove the error points. Fig. 5 (a) shows the real preprocessing results of the original data. The points of Fig. 5 (a) show the detected points by discontinuity and selecting the points near from the laser range finder, and the line shows the line fitting results. The end points of the line correspond with the intersection points between the scanning plane and the checkerboard. Fig. 5 (b) shows the two main steps of the preprocessor, one is median filter as shown as the cross points, the other is line fitting, as shown as the round points, and the square points shows the original points. Form original points of the experiment we could find that it’s difficult to get the real intersection points between the scanning plane and the checkerboard plane. From the experimental results, we could find that the median filter could effectively remove the “jump points”, and the line fitting could effectively decrease the measurement error and noiseeffect.(a) the interface of data collection software(b) the preprocessor of the original dataFig.5. The original data collection and the preprocessorWe employed the proposed algorithm to calibrate the homogeneous transformation between the LRF and each of the cameras. In the experiments, the checkerboard was placed closer to the LRF than the background to easily distinguish the checkerboard from the background. 20 positions were used to calibrate the extrinsic parameters. Fig.6 shows the raw data obtained by the LRF and the cameras for a pose of the checkerboard. The upper image of Fig.5 shows the LRF’s measurements. In order to precisely detect the intersection points’ coordinate, the median filter and the least square line fitting were used to process the detected measurement data of the LRF. As the upper image of Fig. 6 shows, the bottom line of the measurements is the result of the line fitting. The edges of the checkerboard on the bottom images in Fig. 6were detected manually.To demonstrate superior performance of our method over the method in [8], we projected the measurements of the LRF onto the images of the two cameras using the extrinsic parameters calibrated by both methods. The results are shown in Fig.7. The upper solid lines represent the projections of the LRF measurement using the parameters calibrated by our method, and the lower dotted lines denote those obtained by the method in [8]. Obviously, the projected points obtained by our method more fit the scene in the two images. Therefore, it is possible to conclude that our method can calibrate theextrinsic parameters more accurately.Fig.6. The raw data obtained by the LRF and two cameras.C. The Experimental Results of 3D Color Reconstruction After the calibrations of the system, we measured acomplicated scene indoor. Because the sensors could scanthe scene and objects by scanning movement, the systemcould measure bigger objects and more complicated scenes, which could be more far from sensors than lot’s exist measurement system. Fig. 8 shows 3D color reconstruction of point clouds for the complicated scene indoors, and the Fig.8 (b) shows the 3 shot screen of the 3D reconstruction model from 3 different views. Fromthe reconstruction result, we could find that the measurement system could reconstruct the scene true tonature and in detail, such as the paper on the table. Fig. 8shows the other reconstruction results of the complex scene indoors. VI. C ONCLUSION A novel 3D color measurement system was presented in this paper for SLAM problem. After three common features were compared with each other, the calibration algorithms for LRF, camera and platform were presented based on the line feature. The real data experimental results of the algorithms and the 3D color reconstruction model show the effectiveness and the efficient of algorithms and the system.A CKNOWLEDGMENTThe work really thanks the Prof. Yunhui Liu of Hongkong Chinese University. Without his kindly help and the direction, the work and the project could not be completed.R EFERENCES[1] V. Brajovic, K. Mori, and N. Jankovic, “100 Frames/sCmos Range Image Sensor,” Digest of Technical Papers, 2001 IEEE International Solid-State Circuits Conference, pp. 256, 257 and 453, February 2001.[2] L. D. Reid, “Projective Calibration of A Laser-stripeRange Finder,” Image and Vision Computing, vol.14, no.9, pp.659-666, October 1996.[3] H. Baltzakis, A. Argyros, and P. Trahanias, “Fusion ofLaser and Visual Data for Robot Motion Planning and Collision Avoidance,” International Journal of Machine Vision and Applications, vol.15, no. 2, pp. 92-100, 2003. [4] T. Hong, R. Bostelman, and R. Madhavan, “ObstacleDetection Using A TOF Range Camera for Indoor AGV,” Navigation, PerMIS 2004, Gaithersburg, MD, June 2004. [5] I. Bauermann, E. Steinbach, “Joint Calibration of A Rangeand Visual Sensor for the Acquisition of RGBZ Concentric Mosaics”, VMV2005, November 2005.[6] J. Forest, J. Salvi, "A Review of Laser Scanning Three-dimensional Digitizers,” In IEEE/RSJ International Conference on Intelligent Robots and System. EPFL Lausanne, Switzerland, vol.1, pp. 73-78, 2002.[7] J. Davis and X. Chen, “A Laser Range Scanner Designedfor Minimum Calibration Complexity,” In 2001 IEEEThird International Conference on 3D Digital Imaging and Modeling, Canada, pp. 91-98, 2001. [8] Q. L. Zhang, R. Pless. Extrinsic calibration of a camera and laser range finder (improves camera calibration). In 2004 IEEE/RSJ International Conference on IntelligentRobots and systems, Sendai, Japan, 2004, 2301~2306.[9] I. Bauermann, E. Steinbach. Joint calibration of a range and visual sensor for the acquisition of RGBZ concentric mosaics. VMV2005, November 2005.[10] S. Wasielewski, and O. Strauss. Calibration of a multi-sensor system laser rangefinder/camera. Proceedings of the Intelligent Vehicles '95 Symposium, Sep. 25-Sep. 26, 1995, Detroit, USA Sponsored by IEEE Industrial Electronics Society, 1995, 472~477.[11] J. Stoer, and R. Burlisch. Introduction to NumericalAnalysis. Springer Verlag New York Inc, 1980. [12] G. Q. Wei, and S. D. Ma, “Implicit and Explicit CameraCalibration: Theory and Experiments,” IEEE Transaction Pattern Analysis and Machine Intelligence, 16 (5): 169-180,1994.[13] G. Q. Wei, and S. D. Ma, “Implicit and Explicit Camera Calibration: Theory and Experiments,” IEEE TransactionPattern Analysis and Machine Intelligence, 16 (5): 169-180,1994.[14] H. Wang, “The Calculation of the Distance betweenBeeline and dot,” Journal of Shangluo Teachers College of China, vol. 19, no.2, pp.16-19, 121, June 2005.Li Ganhua (Xi’an, China, June 10, 1977 ), Ph. D (2007). He get the Ph. D in National University of Defense Technology, which is in the Changsha city, Hunan province of China. His major field is information technology, image process, data fuse, target recognition.G.H. Li is the senior engineer in Xi’an satellite control center. He has been the main actor of more than 10 important project witch are supported by military foundation, 863 high technology foundation and the country predict foundation. He is interest in the 3D reconstruction technology, target recognition and the image process.Fig.7. Projections of the LRF measurements onto the image planes of the cameras using the parameters calibrated by our method andthe method in [8]. (Upper image: the left camera, lower image: theright camera)。