A personal view of statistical packages for linear regression. In
Stata统计软件概述说明书

2A brief description of StataStata is a statistical package for managing,analyzing,and graphing data.Stata is available for a variety of platforms.Stata may be used either as a point-and-click application or as a command-driven package.Stata’s GUI provides an easy interface for those new to Stata and for experienced Stata users who wish to execute a command that they seldom use.The command language provides a fast way to communicate with Stata and to communicate more complex ideas.Here is an extract of a Stata session using the GUI:(Throughout the Stata manuals,we will refer to various datasets.These datasets are all avail-able from https:///data/r18/.For easy access to them within Stata,type webuse dataset name,or select File>Example datasets...and click on Stata18manual datasets.) .webuse lbw(Hosmer&Lemeshow data)We select Data>Describe data>Summary statistics and choose to summarize variables low, age,and smoke,whose names we obtained from the Variables window.We click on OK.12[U]2A brief description of Stata.summarize low age smokeVariable Obs Mean Std.dev.Min Maxlow189.3121693.464609301age18923.2381 5.2986781445smoke189.3915344.489389801Stata shows us the command that we could have typed in command mode—summarize low age smoke—before displaying the results of our request.Next wefit a logistic regression model of low on age and smoke.We select Statistics>Binary outcomes>Logistic regression,fill in thefields,and click on OK..logistic low age smokeLogistic regression Number of obs=189LR chi2(2)=7.40Prob>chi2=0.0248 Log likelihood=-113.63815Pseudo R2=0.0315low Odds ratio Std.err.z P>|z|[95%conf.interval]age.9514394.0304194-1.560.119.8936482 1.012968smoke 1.997405.642777 2.150.032 1.063027 3.753081_cons 1.062798.80487810.080.936.2408901 4.689025 Note:_cons estimates baseline odds.Here is an extract of a Stata session using the command language:[U]2A brief description of Stata3 .use https:///data/r18/auto(1978automobile data).summarize mpg weightVariable Obs Mean Std.dev.Min Maxmpg7421.2973 5.7855031241weight743019.459777.193617604840The user typed summarize mpg weight and Stata responded with a table of summary statistics. Other commands would produce different results:.generate gp100m=100/mpg.label var gp100m"Gallons per100miles".format gp100m%5.2f.correlate gp100m weight(obs=74)gp100m weightgp100m 1.0000weight0.8544 1.0000.regress gp100m weight gear_ratioSource SS df MS Number of obs=74F(2,71)=96.65 Model87.4543721243.7271861Prob>F=0.0000 Residual32.121888671.452420967R-squared=0.7314Adj R-squared=0.7238 Total119.57626173 1.63803097Root MSE=.67262gp100m Coefficient Std.err.t P>|t|[95%conf.interval]weight.0014769.00015569.490.000.0011665.0017872 gear_ratio.1566091.26511310.590.557-.3720115.6852297 _cons.0878243 1.1984340.070.942-2.301786 2.477435 .scatter gp100m weight,by(foreign)4[U]2A brief description of StataStata’s model for a dataset is that of a table—the rows are the observations and the columns are the variables:.list mpg weight gp100m in1/10mpg weight gp100m1.222,930 4.552.173,350 5.883.222,6404.554.203,2505.005.154,0806.676.183,670 5.567.262,230 3.858.203,280 5.009.163,880 6.2510.193,400 5.26Observations are numbered;variables are named.Stata is fast.That speed is due partly to careful programming,and partly because Stata keeps the data in memory.Stata’sfile model is that of a word processor:a dataset may exist on disk,but the dataset in memory is a copy.Datasets are loaded into memory,where they are worked on,analyzed, changed,and then perhaps stored back on disk.Working on a copy of the data in memory makes Stata safe for interactive use.The only way to harm the permanent copy of your data on disk is if you explicitly save over it.Having the data in memory means that the dataset size is limited by the amount of computer memory.Stata stores the data in memory in an efficient format—you will be surprised how much data canfit.Nevertheless,if you work with extremely large datasets,you may run into memory constraints.You will want to learn how to store your data as efficiently as possible;see[D]compress. Stata,Stata Press,and Mata are registered trademarks of StataCorp LLC.Stata andStata Press are registered trademarks with the World Intellectual Property Organization®of the United Nations.Other brand and product names are registered trademarks ortrademarks of their respective companies.Copyright c 1985–2023StataCorp LLC,College Station,TX,USA.All rights reserved.。
spss计算cv值
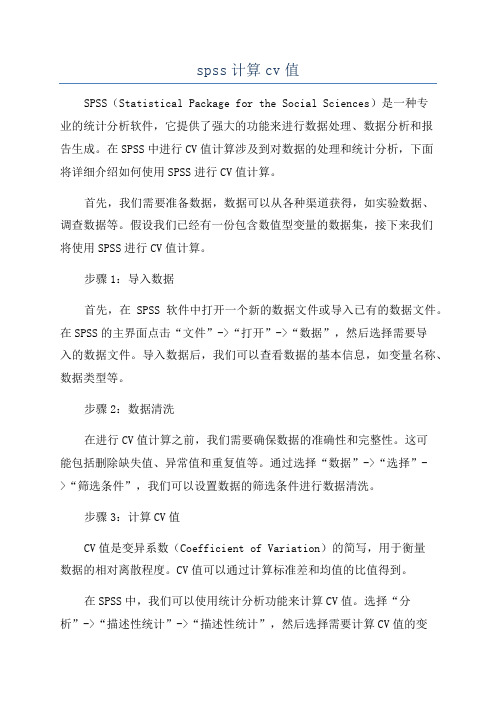
spss计算cv值SPSS(Statistical Package for the Social Sciences)是一种专业的统计分析软件,它提供了强大的功能来进行数据处理、数据分析和报告生成。
在SPSS中进行CV值计算涉及到对数据的处理和统计分析,下面将详细介绍如何使用SPSS进行CV值计算。
首先,我们需要准备数据,数据可以从各种渠道获得,如实验数据、调查数据等。
假设我们已经有一份包含数值型变量的数据集,接下来我们将使用SPSS进行CV值计算。
步骤1:导入数据首先,在SPSS软件中打开一个新的数据文件或导入已有的数据文件。
在SPSS的主界面点击“文件”->“打开”->“数据”,然后选择需要导入的数据文件。
导入数据后,我们可以查看数据的基本信息,如变量名称、数据类型等。
步骤2:数据清洗在进行CV值计算之前,我们需要确保数据的准确性和完整性。
这可能包括删除缺失值、异常值和重复值等。
通过选择“数据”->“选择”->“筛选条件”,我们可以设置数据的筛选条件进行数据清洗。
步骤3:计算CV值CV值是变异系数(Coefficient of Variation)的简写,用于衡量数据的相对离散程度。
CV值可以通过计算标准差和均值的比值得到。
在SPSS中,我们可以使用统计分析功能来计算CV值。
选择“分析”->“描述性统计”->“描述性统计”,然后选择需要计算CV值的变量,并将其添加到“变量”框中。
点击“统计”按钮,在弹出的对话框中选择“均值”和“标准差”,然后点击“确定”。
SPSS将输出一个包含均值、标准差和CV值的统计分析结果表。
在该结果表中,CV值将显示在“标准差”列的最后一列。
步骤4:结果解读和分析获取CV值后,我们可以进行结果的解读和分析。
CV值越大,表示数据的离散程度越大,数据之间的差异越大;CV值越小,表示数据的离散程度越小,数据之间的差异越小。
根据研究的具体内容和背景,我们可以对CV值进行分析和讨论。
简历上怎么写数学建模模板

简历上怎么写数学建模模板I am a highly motivated and diligent individual with a strong background in mathematics and data analysis. I have a Bachelor's degree in Mathematics and a Master's degree in Applied Mathematics, both of which have provided me with a solid foundation in mathematical principles and problem-solving techniques.In my academic and professional experiences, I have honed my skills in mathematical modeling and data analysis. I have completed several projects that require the use of mathematical models to analyze complex data sets and make informed decisions.I am proficient in a variety of programming languages, such as Python and R, which I use to build and implement mathematical models.One of my proudest achievements is a project I completed during my Master's degree program, where I developed a mathematical model to predict customer churn in a telecommunications company. By analyzing customer data and applying statistical techniques, I was able to accurately predict customer behavior and recommend strategies to reduce churn rates.I am passionate about using mathematics to solve real-world problems and make a positive impact on society. I am eager to apply my skills and knowledge in a challenging and dynamic work environment, where I can continue to develop my expertise in mathematical modeling and data analysis.。
stata 离散值

stata 离散值Stata, as a powerful statistical software package, is widely used in various fields for data analysis. When dealing with discrete variables in Stata, it offers a range of tools and commands to facilitate efficient and accurate analysis. Discrete variables, often represented by categorical or integer data, play a crucial role in understanding the structure and patterns within a dataset.在Stata中处理离散值时,我们可以利用一系列命令和工具来简化并优化分析过程。
离散变量,通常以分类数据或整数数据的形式出现,对于理解数据集的结构和模式至关重要。
One common task when analyzing discrete variables in Stata is to generate frequency tables. This involves counting the occurrences of each unique value in a variable and summarizing the results. The `tabulate` command is particularly useful for this purpose, as it provides a quick and straightforward way to visualize the distribution of discrete values.在Stata中分析离散值时,一个常见的任务是生成频数表。
2025届湖北省武汉市硚口区高三起点考试七月质量检测英语试卷
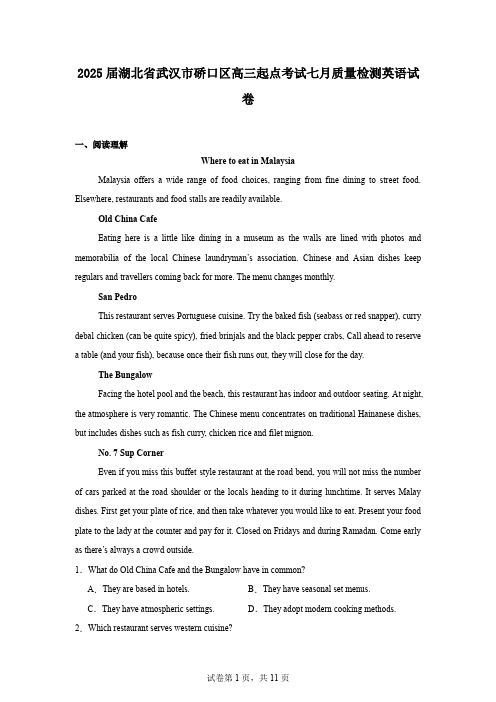
2025届湖北省武汉市硚口区高三起点考试七月质量检测英语试卷一、阅读理解Where to eat in MalaysiaMalaysia offers a wide range of food choices, ranging from fine dining to street food. Elsewhere, restaurants and food stalls are readily available.Old China CafeEating here is a little like dining in a museum as the walls are lined with photos and memorabilia of the local Chinese laundryman’s association. Chinese and Asian dishes keep regulars and travellers coming back for more. The menu changes monthly.San PedroThis restaurant serves Portuguese cuisine. Try the baked fish (seabass or red snapper), curry debal chicken (can be quite spicy), fried brinjals and the black pepper crabs, Call ahead to reserve a table (and your fish), because once their fish runs out, they will close for the day.The BungalowFacing the hotel pool and the beach, this restaurant has indoor and outdoor seating. At night, the atmosphere is very romantic. The Chinese menu concentrates on traditional Hainanese dishes, but includes dishes such as fish curry, chicken rice and filet mignon.No. 7 Sup CornerEven if you miss this buffet-style restaurant at the road bend, you will not miss the number of cars parked at the road shoulder or the locals heading to it during lunchtime. It serves Malay dishes. First get your plate of rice, and then take whatever you would like to eat. Present your food plate to the lady at the counter and pay for it. Closed on Fridays and during Ramadan. Come early as there’s always a crowd outside.1.What do Old China Cafe and the Bungalow have in common?A.They are based in hotels.B.They have seasonal set menus.C.They have atmospheric settings.D.They adopt modern cooking methods. 2.Which restaurant serves western cuisine?A.Old China Cafe.B.No.7 Sup Corner.C.The Bungalow.D.San Pedro.3.What do we know about No. 7 Sup Corner?A.It is inconveniently located.B.It is a self-service restaurant.C.It requires a restaurant booking.D.It opens every day except the weekends.Norwegian playwright and author Jon Fosse has just been awarded the 2023 Nobel Prize in Literature for his “innovative plays and prose which give voice to the unsayable”.The Swedish Academy credits Fosse as “one of the most widely performed playwrights in the world”, although the 64-year-old originally made his name as a novelist, beginning with Red, Black in 1983. He has since written many works of prose and poetry. “His literary works, spanning a variety of genres(体裁), comprises about 40 plays and a wealth of novels, poetry collections, essays, children’s books and translations,” said Anders Olsson, chairman of the Nobel Committee for Literature.Jon Fosse draws inspiration from his rural living environment and personal struggles, which have deeply influenced his distinctive writing style. Growing up in a small coastal village, Fosse was immersed in the beauty of nature and the vastness of the sea, which filled him with a sense of solitude and introspection(内省) that is reflected in his works. Fosse’s writing discussed themes of desolateness(孤独,苍凉), longing, and the search for meaning in life. Additionally, his battles with depression have enabled him to explore the depths of human emotions and existential des-pair.“He touches you so deeply when you read his works,” said Anders Olsson. “What is special about him is the closeness in his writing. It touches your deepest feelings-anxieties, insecurities, questions of life and death-which are things that every human being actually faces from the very beginning. In that sense I think he reaches very far and there is a sort of a universal impact of every-thing that he writes-it has appeal to this basic humanity.”Fosse is the first-ever laureate writing in Nynorsk, one of the two official languages of Norwegian, but only used by just 10% of the population. As The Guardian writes, “Many Nynorsk speakers see Fosse ‘as a kind of national hero’ for his championing of the language.” Fosse’s recognition on a global stage promotes the visibility and importance of Nynorsk as a writtenstandard. It will attract more attention to Nynorsk literature, inspire and encourage Nynorsk speakers to continue preserving and promoting their linguistic(语言的) heritage.4.What do we know about Jon Fosse from paragraph 2?A.His most popular work is the play Red, Black.B.He accomplished various genres of literature works.C.Many famous plays are the inspiration for his writing.D.His language skills earned him the Nobel Prize in Literature.5.What does paragraph 3 mainly talk about?A.Jon Fosse’s tough experiences.B.Jon Fosse’s motivation for writing.C.The major themes of Jon Fosse’s works.D.The effect of Jon Fosse’s life on his writing style.6.What is special about Jon Fosse’s works according to Anders Olsson?A.They explore the meaning of life.B.They touch human’s deepest emotions.C.They show the bond between humans and nature.D.They appeal to readers to focus on personal feelings.7.What contribution of Jon Fosse is mentioned in the last paragraph?A.He explains the importance of linguistic diversity.B.He has improved the international status of Norway.C.He promotes the development and preservation of Nynorsk.D.He shapes the basic writing standard of Norwegian literature.It’s one of the most common questions adults ask children: what do you want to be when you grow up? Although childhood is supposed to be fun, kids also discuss important topics regarding their futures. Now, a new study finds that children who set big goals regarding their future status and education often set themselves up for success as they age.The findings, published in the Journal of Personality and Social Psychology, are the first to reveal a connection between life goal development and future success in school or the workplace. Rodica Damian, an associate professor of psychology at the University of Houston, and otherresearchers discovered that as children grow up, their goals naturally begin to change. However, as some childhood goals fall away, other goals related to a family stay strong. These include being close to relatives, building more friendships or finding a romantic partner, and even becoming more involved in your community or helping others.During the study, researchers compared how their goals evolved as children moved from adolescence to adulthood and how a person’s goals impacted their success in school and as an employee later on. Overall, a child’s goals focusing on their education and future status were the most consistent predictors (预测因素) of income in adulthood. Simply put, when a child dreams big about doing well in school or achieving great success as an adult, these goals accurately paint a picture of how successful these children will be. So, the message is simple: dream big and dream of success, kids! Those goals can drive you to success when you grow up!“Our work proves a strong connection between a child’s life goals, educational achievement, and future occupational outcomes. This information is valuable for parents and educators who can use it to encourage children to set ambitious goals. Additionally, it helps develop strategies to support individuals in achieving their goals and reaching their full potential,” Damian concludes. 8.What does the study mainly focus on?A.Why children change their dreams over time.B.Whether childhood goals relate to future success.C.Whether early education determines future career.D.How childhood experiences impact a person’s choice.9.Which of the following goals remains strong as children grow up?A.Connecting with others.B.Achieving academic success.C.Hunting for a high-salary jobD.Improving personal social status.10.What message does the author convey in paragraph 3?A.Dreaming big is potentially associated with success.B.Childhood achievement s greatly affect future careers.C.Educational goals may be more achievable than others.D.Adjusting goals can create new opportunities for success.11.What is the purpose of the last paragraph?A.To explain the purpose of the study.B.To offer suggestions for future studies.C.To stress the significance of the study.D.To point out the limitations of the study.Spiders are master builders, expertly turning silk into complex 3D webs that serve as their home and hunting ground. To gain a deeper understanding of their world, scientists have translated the structure of a spider’s web into music.“The spider lives in an environment of vibrating (振动的) strings,” says Markus Buehler, the project’s principal investigator. “They don’t see very well, so they perceive their surroundings by detecting vibrations, which have different frequencies.” Such vibrations occur, for example, when the spider stretches a strand of silk during construction or when the wind or a trapped fly moves the web.The researchers scanned a natural spider web to capture 2D cross-sections and reconstructed its 3D network using a mathematical model. They assigned different frequencies of sound to strands of the web, creating musical “notes” that they combined in patterns based on the web’s 3D structure to generate music. Then they made a harp-like (像竖琴的) virtual instrument and played the spider web music in several live performances around the world, creating an inspiring harmony of art and science.To gain insights into how spiders build webs, the researchers also scanned a web during construction, transforming each stage into music with different sounds. “The spider’s way of ‘printing’ the web is remarkable because no support material is used, as is often needed in current 3D printing methods,” Buehler says. This knowledge could help develop new 3D printers that work like spiders, enabling them to construct complex structures without using additional materials for support.The team is also interested in learning how to communicate with spiders in their own language. They recorded web vibrations produced when spiders performed different activities, such as building a web, communicating with other spiders or sending signals to admirers. “Now we’re trying to generate signals to basically speak the language of the spider,” Buehler says. “If we expose them to certain patterns of vibrations, can we affect what they do or can we begin to communicate with them? Those are really thrilling ideas, and I believe they could be achieved inthe near future.”12.What do we know about spiders?A.They have good eyesight.B.They adapt to surroundings quickly.C.They spin a web similar to a musical structure.D.They sense the environment through vibrations.13.What is paragraph 3 mainly about?A.Steps to create spider web music.B.Ways to recreate a web’s 3D network.C.Challenges of combining music with science.D.The potential applications of 3D printing methods.14.What do the underlined words “This knowledge” in paragraph 4 refer to?A.3D printers can construct complex structures.B.Spiders’ vibration patterns vary with activities.C.Spiders build webs without using additional materials.D.The web-building process can be translated into music.15.What is Buehler’ attitude towards communicating with spiders?A.Hopeful.B.Doubtful.C.Uncertain.D.Disapproving.The storms in our lives often leave behind gifts if we open our eyes to see them. As the skies clear and you start to emerge from the loss, look for the silver linings. 16 Going through difficulties requires courage and determination. You have to dig deep and find the strength you didn’t know you possessed to overcome the challenges. 17 That self-knowledge and confidence in your abilities will serve you well going forward.Coming out the other side of trouble sometimes gives you a fresh outlook. Priorities shift.18 You have a deeper appreciation for life’s simple moments and clarity about what matters. Use that insight to guide your choices and cherish each day. 19 The old way of doing things no longer works, so you must rebuild and reimagine your life. Though change is hard, it opens up possibilities for new growth and exciting adventures. Look at this as a chance for a fresh start. You can pursue new dreams or make along-wished-for switch in your situation. The future isunwritten.Your struggles and pain have given you understanding for what others maybe going through in their own lives. You now recognize the suffering of people around you and desire to help ease their burdens. Use your experience to be there for friends and family members facing difficulties.20 Your sympathy can make a real difference.A.Dramatic changes often lead to transformation.B.They’re there if you are bent on searching for them.C.Offer a listening ear and share your story with them.D.Storms shape us, but the silver linings make us suffer.E.What seemed important before now pales in comparison.F.Now you know you have the inner power to weather storms.G.This new perspective removed previous doubt about yourself.二、完形填空In my early thirties, I decided to climb Mount Kilimanjaro, the highest mountain in Africa at 5, 895meters above sea level.After months of preparation, I started my great 21 . At the base of the mountain, I met Mik, a local porter, who warmly 22 me. Mik’s job was to carry my equipment up the mountain, set up the tent, and carry everything back down after reaching the 23 .The first day of the climb was 24 the rain forest, across a slippery , muddy ground covered with tree roots and vines (藤蔓). Mik was carrying 30 kilos on his head! By that evening, we made it to 3,000meters. The air was 25 and it was colder. When I arrived at the campsite for the night, my tent was already set up and waiting for me.Day two was much steeper and rockier. I really had to 26 what I was doing. And I felt guilty for the tough 27 Mik had to work in. Yet when I turned to Mik, he said with the biggest smile, “pole, pole,” which means “ 28 , take it easy” in his native language. I 29 back, my burden lighter somehow.Over the next five days, the climb got 30 difficult. The temperatures could 31 from 21℃to below freezing in a few hours. At 5, 700 meters, there’s only half the oxygen 32in each breath compared to at sea level. That leaves many people with severe headache. Yet Mik always had a smile and a positive attitude. It had an enormous impact on me, giving me the 33 to keep going.Even today, when I find myself 34 with anything in life, I just think back to Mik and his smile. A great attitude can bring joy to those around you, or even 35 strangers to the top of a mountain.21.A.performance B.business C.presentation D.adventure 22.A.questioned B.congratulated C.greeted D.invited 23.A.base B.peak C.forest D.border 24.A.outside B.beside C.through D.over 25.A.thinner B.softer C.drier D.sweeter 26.A.focus on B.turn on C.bring up D.break up 27.A.locations B.conditions C.atmosphere D.competition 28.A.stop B.hurry C.relax D.move 29.A.ran B.talked C.smiled D.rode 30.A.slightly B.increasingly C.automatically D.equally 31.A.swing B.circle C.climb D.last 32.A.predictable B.available C.noticeable D.affordable 33.A.impression B.platform C.opportunity D.strength 34.A.cooperating B.struggling C.associating D.communicating 35.A.persuade B.introduce C.motivate D.recommend三、语法填空阅读下面短文,在空白处填入1个适当的单词或括号内单词的正确形式。
封装专用英语词汇

常见封装形式简介DIP = Dual Inline Package = 双列直插封装HDIP = Dual Inline Package with Heat Sink = 带散热片的双列直插封装SDIP = Shrink Dual Inline Package = 紧缩型双列直插封装SIP = Single Inline Package = 单列直插封装HSIP = Single Inline Package with Heat Sink = 带散热片的单列直插封装SOP = Small Outline Package = 小外形封装HSOP = Small Outline Package with Heat Sink = 带散热片的小外形封装eSOP = Small Outline Package with exposed thermal pad = 载体外露于塑封体的小外形封装SSOPTSSOPTQPFPQFPLQPFeLQPFDFN =QFN =TO =SOT =BGA =BQFP =CAD =CBGA =CCGA =CSP =DFP =DSO =3D =2D =FCB =IC =I/O =LSI =MBGA = Metal BGA = 金属基板BGAMCM = Multichip Module = 多芯片组件MCP = Multichip Package =多芯片封装MEMS = Microelectro Mechanical System = 微电子机械系统MFP = Mini Flat Package = 微型扁平封装MSI = Medium Scale Integration = 中规模集成电路OLB = Outer Lead Bonding = 外引脚焊接PBGA = Plastic BGA = 塑封BGAPC = Personal Computer = 个人计算机PGA = Pin Grid Array = 针栅阵列SIP = System In a Package = 系统级封装SOIC = Small Outline Integrated Circuit = 小外形封装集成电路SOJ = Small Outline J-Lead Package = 小外形J形引脚封装SOP = Small Outline Package = 小外形封装SOP = System On a Package = 系统级封装WB = Wire Bonding = 引线健合WLP = Wafer Level Package = 晶圆片级封装常用文件、表单、报表中英文名称清除通知单Purge notice工程变更申请 ECR(Engineering Change Request)戴尔专案收据数据表核对表文件清单设备清单报名表日报表周报表月报表年报表年度报表财务报表品质报表生产报表符合性报告(材质一致性证明) COC(Certificate of Compliance) 稽核报告 Audit report品质稽核报告 Quality audit report制程稽核报告Process audit report5S 稽核报告 5S audit report客户稽核报告Customer audit report供应商稽核报告Supplier audit report年度稽核报告 Annual audit report内部稽核报告 Internal audit report外部稽核报告External audit reportSPC 报表(统计制程管制) Statistical process control工序能力指数(Cpk) Process capability index(规格)上限Upper limit(规格)下限 Lower limit规格上限Upper Specification Limit(USL) 规格下限 Lower Specification Limit(LSL)上控制限(或管制上限) Upper Control Limit(UCL)下控制限(或管制下限)Lower Control Limit(LCL)最大值 Maximum value平均值Average value最小值Minimum value临界值MRB 单(TECN压焊图订购单送货单/询价单返工单减薄:Grind??[ɡraind ]?vt. & vi. 磨碎;嚼碎n .磨,碾Crack???[kr?k]?? vt. & vi.? (使…)开裂, 破裂n.?裂缝, 缝隙Ink?[i?k] ?n. ?墨水, 油墨Die [dai]? vt. & vi. ?死亡(芯片)Dot?[d?t] ?n .?点, 小圆点Mounting?[‘maunti?] ?n.? 装备,衬托纸Tape?[teip] ?n.? 带子;录音磁带; 录像带Size?[saiz] ?n. ?大小, 尺寸,尺码Thick?[θik]? adj. 厚的,厚重的Thickness?[‘θiknis]?? n.? 厚(度), 深(度)宽 (度) Position?[p?‘zi??n]? n. ?方位,位置Rough?[r?f] ?adj .?粗糙的; 不平的Fine?[fain]? adj. ?美好的, 优秀的, 优良的, 杰出的Speed [spi:d]? n.? 速度, 速率Spark[spɑ:k]?n.? 火花; 火星Out?[aut]? adv. 离开某地, 不在里面;(火或灯)熄灭Grindstone???[‘ɡraindst?un]? n. ?磨石、砂轮MounterCard?[k划片:Street? n. ?大街, 街道Blade?[bleid] ?n. ?刀口, 刀刃,刀片Cut?[k?t]?vt. & vi. ?切, 剪, 割, 削Speed[spi:d]n.? 速度, 速率Spindle?[‘spindl]? n.? 主轴, (机器的)轴Size?[saiz]? n. ?大小, 尺寸?,尺码Cooling?['ku:li?]adj.? 冷却(的)Kerf?[k?:f] ?n.? 锯痕,截口,切口Width?[widθ] ?n . ?宽度, 阔度, 广度Chip?[t?ip] ?n. ?碎片、缺口Chipping[‘t?ipi?]n. ?碎屑,破片Crack[kr?k]vt . (使…)开裂,破裂n . 裂缝, 缝隙Missing?[‘misi?] adj. 失掉的,失踪的,找不到的Die [dai]? vt. & vi.? 死亡(芯片)Saw?[s?:]? n. ?锯vt. & vi. ?锯,往复运动Street ?[stri:t]n.? 大街, 街道Film?[film]? ?n.? 影片, 电影(薄膜,蓝膜)mount---刀片tape------上料---空气pressure---校准ink------限制cover---切割water---wheel---检查feed---new---新清洗? center上芯:Bonder?[‘b?nd?]? n. 联接器,接合器,粘合器Die attach material epoxy粘片胶Epoxy??[e‘p?ksi]?? n. 环氧树脂(导电胶)Material??[m?‘ti?ri?l]?? n.? 材料, 原料Non-conductive epoxy绝缘胶Conductive??[k?n‘d?ktiv]?? adj. 传导的Dispenser??[dis‘pens?] ?n. ?配药师, 药剂师Nozzle?[‘n?zl] ?n. ?管嘴, 喷嘴Rubber??[‘r?b?]? n. ?(合成)橡胶,橡皮Tip?[tip]?n. ?尖端, 末端Die pick-up tool 吸嘴Tool?[tu:l]? ?n.? 工具, 用具Collect?[k?‘lekt]? vt. ?收集, 采集(吸嘴)Ejector?[i‘d?ekt?]? n. ?驱逐者,放出器,排出器Pin?[pin] ?n. 针,大头针, 别针Lead Frame引线框架Lead??[li:d]? vt. & vi. ?带路, 领路, 指引Pick Level 捡拾芯片高度Head pick delay 粘接头拾取延迟Head bond delay 粘接头粘接延时Pick delay捡拾芯片延时Bond delay 粘接芯片延时Index?[‘indeks] ?n. ?索引;标志, 象征; 量度Clamp?[kl?mp]? vt. & vi. ?夹紧; 夹住n.?夹具Index clamp delay 步进夹转换延时Index delay 框架步进延时Shear??[?i?]?? vt.? 剪羊毛, 剪n.?大剪刀Test?[test]? n. ?测验,化验,试验, 检验Die shear test 推晶试验Thickness?['θiknis] ?n.? 厚(度), 粗Coverage?[‘k?v?rid?] ?n. ?覆盖范围Epoxy thickness & coverage 导电胶厚度和覆盖率Orientation[,?:rien‘tei??n]? n.? 方向, 目标Die Orientation 芯片方向Epoxy curing银浆烘烤Edge?[ed?]?n.?? 边, 棱, 边缘Partial?[‘pɑ:??l] ?adj.? 部分的, 不完全的Mirror?[‘mir?]?? ?n. ? 镜子Missing?[‘misi?] adj.? 失掉的,失踪的,找不到的Edge die / partial die边缘片 / 边沿芯片Mirror die光片 / 镜子芯片Missing die 掉芯 / 漏芯 / 掉片Splash [spl??]vt. 使(液体)溅起vi.(液体)溅落Splatter ?[‘spl?t?]vt. & vi.? (使某物)溅泼Diagram [‘dai?ɡr?m] ?n. ?图解, 简图, 图表Ink splash / ink splatter墨溅Die bonding diagram 上芯图Die shesr test 推片实验/推晶试验Die shear tester 推片试验机Die shesr tool 推片头Metal corrosion晶粒腐蚀/芯片腐蚀wafer------银胶---参数manual---错误input------压力vacuum---针---改机---调整contact---崩边pause------校准cassette---铁圈nozzle---点胶头 writer---划胶头压焊:Wire?[‘wai?]?n.?金属丝, 金属线;电线, 导线Bond?[b?nd]? n.?接合, 结合vt.?使粘结, 使结合Wire bond / Wiring bonding 压焊/焊丝/球焊Gold wire金丝Pad? [p?d]? vt.?给…装衬垫, 加垫子n.垫,护垫Bond pad 焊点、铝垫1st bond第一焊点Pad size焊点尺寸 / 铝垫尺寸Capillary?[k?‘pil?ri]?n.毛细管;毛细血管(劈刀)Pitch??[pit?]?? 程度; 强度; 高度Pad pitch铝垫间距 / 焊点间距EFO打火烧球loop?[lu:p]? n.? 圈, 环, 环状物Loop height 孤高Wire pull test 拉力试验Ball shear test 金球推力试验PIN 1 第一脚Ball height 球高Ball diameter球径Cratering??[‘kreit?ri?]?n. ?缩孔;陷穴(弹坑)KOH etching test KOH腐蚀试验Bond Cratering test 压焊腐蚀试验(弹坑试验) Thermal?[‘θ?:m?l]?adj. 热的,热量的Compression?[k?m‘pre??n]?n.?挤压, 压缩TCB( Thermal Compression Bond)热压焊N2 BOXRTPCFBIMetal?[‘metl]?n.?金属Discolor?[dis‘k?l?] ?v.使脱色;(使)变色,(使)褪色Oxide?[‘?ksaid]n.?氧化物Metal Discolor铝条变色Bond Pad Discolor 铝垫变色Bond Pad Oxide铝垫氧化Stick?[stik] ?vt. & vi.?粘贴, 张贴Peeling?[‘pi:li?] n. 剥皮,剥下的皮Crateri ng??[‘kreit?ri?]n. ?缩孔;陷穴(弹坑)Nonstick bond on pad 铝垫不粘Bond pad peeling 铝垫脱落Bond pad cratering铝垫弹坑Limit?[‘limit] ?vt.?限制; 限定Scratch?[skr?t?]?vt. & vi.?抓, 搔,?刮伤High loop弧度太高Loop short 弧度短路Overhang?[,?uv?‘h??]vt. 伸出; 悬挂于…之上Residue?[‘rezidju:]?n. 剩余, 余渣Distortion?[dis‘t?:??n] ?n. 歪曲,曲解Wire overhang on LD 跨越引线框架Wire residue 残丝LF distortion 引线框架变形Quantity?[‘kw?ntiti] ?n.?数目, 数量Mismatch?[‘mis’m?t?] ?vt. 使配错,使配合不当Scrap?[skr?p] ?n.?废料vt.?废弃, 丢弃Scratch?[skr?t?] vt. 刮伤Quantity Mismatch 数量不符Empty M. not scrap空粘未报废Teach---BQM---Bond offweld off---管脚脱焊 crater---裂缝 gold wire---金线 missing ball---球未烧好 weak bond---虚焊塑封:Mold?[m?uld]? n.?模子,铸型vt.?浇铸,塑造Molding?[‘m?uldi?]?n.?成型(塑封)Compound?[‘k?mpaund]?n.?复合物, 化合物Moiding M/C;Mold Press塑封机Press?[pres]? n.?印刷机Heater?[‘hi:t?]?n.?加热器; 炉子Pre-heater 预热机Chase?[t?eis]? n.?追捕, 追猎Mold die / Mold chase 塑封模具MGP mold MGP多缸模具temperTransfer speed注塑速度Transfer time注塑时间PMC time (Post Mold Cure Time)后固化时间Load / unload上料/下料Sweep ?[swi:p]?vt. & vi.?扫, 打扫, 拂去Wire Sweep 冲丝Open 开路Short 短路Fill ?[fil] vt. & vi. ?(使)充满, (使)装满, 填满Underfill?['?nd?fil]?n.?(孔型)未充满Body underfilled胶体未灌满Incomplete [,?nk?m‘pli:t] adj. 不完全的, 未完成的Incomplete mold 未封满Chip ?[t?ip]? n.?碎片,缺口BTM width / length 背面宽 / 长Top width / length 正面宽 / 长PKG thick 塑封体厚度Mismatch [‘mis’m?t?] vt.?使配错,使配合不当Mold mismatch / PKG mismatch 包封偏差(胶体错位)Offset [‘?fset] vt.?抵消, 补偿Misalignment [‘mis?lainm?nt]?n.?未对准Mold offset / PKG misalignment偏心PMC(post mold cure)后固化Dummy [‘d?mi]?n.??人体模型Strip [strip]? vt.?剥去,?剥夺, 夺走Dummy molded strip空封Mold flash 废胶Gate [ɡeit]? n.?门, 栅栏门AgingGun [ɡ?n]?? n.??枪, 炮Coating [‘k?uti?]?n.??涂层, 覆盖层Material ?[m?‘ti?ri?l]? n.?材料, 原料,素材, 资料Air Gun气枪Die Coating芯片涂胶Auto die coating M/C 芯片涂胶机Die Coating Material覆晶胶Cart [kɑ:t] ?n.?手推车ASS’YB Cart 后站推车Tablet [‘t?blit]? n. ?药片、胶囊Loader [‘l?ud?] ? n.? 装货的人,装货设备,装弹机Preheater [‘pri:’hi:t?] ? n. 预热器Fixture [‘fikst??] n. (房屋等的)固定装置Auto Tablet Loader 自动排胶粒机OffloadTransferMotor---CylinderPickerPin---针Cable–导线 Profile---温度曲线 Alarm---报警Error---错误 Driver---驱动 Sensor–感应器Inspection---检查Parameter---参数 Manual---手动,手册Reset---复位 Initialing---初始化 Guide–导轨Substrate---基板 Device---产品种类 Lot Traveller---随工单Magazine---盒子 Cylinder –汽缸 Bearing–轴承Stop---停止Emergency Stop---紧急停止 Gripper --夹子Heat–加热器Pipe–管子Temperature---温度Hopper–漏斗Compress air–压缩空气Over flow—反面漏胶Semiconductor---半导体 Molding –模封Operation–操作Flange–法兰盘 Pump–泵Chamber–腔体Vent–气孔Value–值Alarm---报警Error---错误 Inspection---检查 Parameter---参数 Manual---手动,手册Reset---复位Initialing---初始化Incomplete fill模封不全inchingmismatch---毛刺切筋1 切筋2 切筋模3 成形模4 分离模5 冲废6 检测7 再成型机模具Reform Die8 再成型机 Reform system9 料盘 Plastic tray10 连筋 Uncut dambar11 毛刺burr14 溢料Junk15 裂纹Crack16 离层(分层) Delaminating17 管脚反翘 Lead tip bend18 筋未切 Dam-bar uncut19 筋凸出 Dam-bar protrusion20 筋切入Dam-bar cut in打印 Marking1 打印Marking2 印章345 正印6 背印7 镜头89 漏打10 断字11 缺字121314 重印151619 电流21 字体(字形) Font22 定位针 Location pin23 胶皮打印机 Pad printer24 激光打印机 Laser Marking M/C25 后固化 PMC(Post Mold Cure)26 后固化烤箱 PMC Oven27 打印污斑 Marking stain28 印记移位Marking shift电镀 Plating1 电镀 Plating2 来料Incoming3 冲废 Dejunk4 热煮软化槽 Socking Tank7 检验 Inspection 外观检测8 烘烤9 出料101112 搭锡131415 发黄16 发黑17 变色1819 粘污202122 外观2324 无铅化 Pb-free / lead free25 结合力 Adhesive force26 可靠性Reliability27 电解Electrolytic deflash28 清洗(自来水)City water29 高压清洗 High pressure rinse30 脱脂 Descale31 清洗(纯水) DI water32 活化(合金) Activation33 预镀、预浸 Pre-dip34 电镀Plating35 吹风 Air blow36 中和Neutralization37 褪镀Stripper38 拖出394041 纯锡42 纯水(43 水压44454647 C5152 预浸53545556 中和59 烘干60 锡球 Solder ball61 锡厚度和成分 Sn thickness & composition62 冲废De-junk去胶渣63 去溢料 Degate 冲塑,冲胶64 去飞边 Deflash 去胶(塑封工序)65 锡铅电镀 Tin lead plating66 无铅电镀 Lead free plating; Pure tin plating67 镀层起泡Solder bump68 镀层剥落 Solder peel off69 镀层偏厚或偏薄 Thick or Thin Plating70 退锡 Solder remove71 电镀报废Plating scrap72 锡渣 Solder peeling73 电镀锡块Solder bump74757677测试1 测试2 打印34 编带568 划伤9 打错101112 模糊 Fade mark13 脚长Lead length14 脚宽 Lead width15 站立度 Stand up16 脚间距 Lead pitch17 共面性 Coplanarity18 跨度 Row space19 电性能测试 Electrical test20 塑料管 Plastic tube21 编带 Reel / Tape22 托盘,盘装 Tray23 扫描测脚 Leads Scan/Inspection24 扫描测脚机Leads scanner25 投影仪 Profile Projector测试LaserLampTrackBeamSensor 传感器Motor 马达Driver 驱动器Index 步进Tool 模具Press 模具Punch 刀具Jam 卡料Forming 成型Cylinder气缸Laser head 光头Magazine 盒子Tube 管子Tray 板子ArmLampAlarmErrorRetestSampleResample 重新抽样Black box盛放未测试产品的黑盒子Testing area 测试区域Test chuck 测试平台Device Interface Board(DIB) 芯片测试接口板DUT 正在测试芯片A/D (analog-to-digital) converter 模/数转换模块EOT 测试结束信号SOT测试开始信号BIN signal 分BIN 信号Socket 测试座JIG/Test Head 测试盒/测试头Interface Card 接口通讯卡Interface Cable 接口通讯线CheckerFingerCapacitor box 电容盒。
spss效度分析
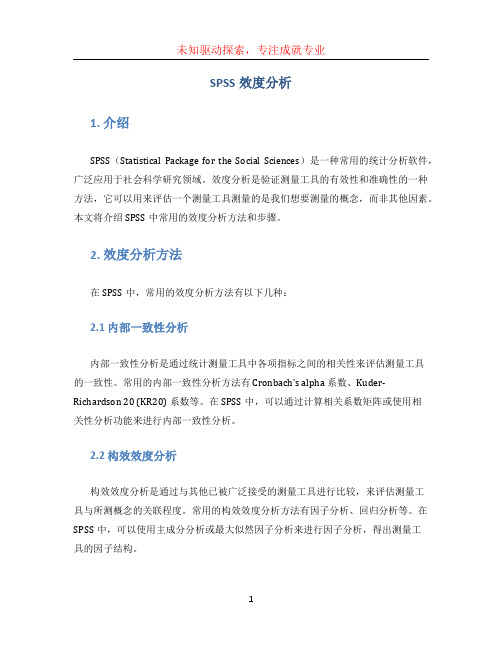
SPSS效度分析1. 介绍SPSS(Statistical Package for the Social Sciences)是一种常用的统计分析软件,广泛应用于社会科学研究领域。
效度分析是验证测量工具的有效性和准确性的一种方法,它可以用来评估一个测量工具测量的是我们想要测量的概念,而非其他因素。
本文将介绍SPSS中常用的效度分析方法和步骤。
2. 效度分析方法在SPSS中,常用的效度分析方法有以下几种:2.1 内部一致性分析内部一致性分析是通过统计测量工具中各项指标之间的相关性来评估测量工具的一致性。
常用的内部一致性分析方法有Cronbach’s alpha 系数、Kuder-Richardson 20 (KR20) 系数等。
在SPSS中,可以通过计算相关系数矩阵或使用相关性分析功能来进行内部一致性分析。
2.2 构效效度分析构效效度分析是通过与其他已被广泛接受的测量工具进行比较,来评估测量工具与所测概念的关联程度。
常用的构效效度分析方法有因子分析、回归分析等。
在SPSS中,可以使用主成分分析或最大似然因子分析来进行因子分析,得出测量工具的因子结构。
2.3 与外部变量关系效度分析与外部变量关系效度分析是通过测量工具与其他已知变量之间的关联程度来评估测量工具的效度。
常用的与外部变量关系效度分析方法有相关性分析、卡方检验、t检验等。
在SPSS中,可以使用相关性分析功能来计算测量工具与其他变量之间的相关系数,或使用t检验功能来比较测量工具在不同组别间的差异。
3. SPSS效度分析步骤在进行SPSS效度分析时,通常需要以下步骤:3.1 收集数据首先,需要收集一组用于效度分析的数据。
数据可以通过问卷调查、实验设计等方式获取。
3.2 数据预处理在进行效度分析之前,需要对收集到的数据进行预处理,包括数据清洗、缺失数据处理等。
SPSS提供了丰富的数据处理功能,可以帮助我们对数据进行加工和清洗。
3.3 内部一致性分析使用SPSS中的相关性分析功能,计算测量工具中各项指标之间的相关系数。
工厂常用术语100
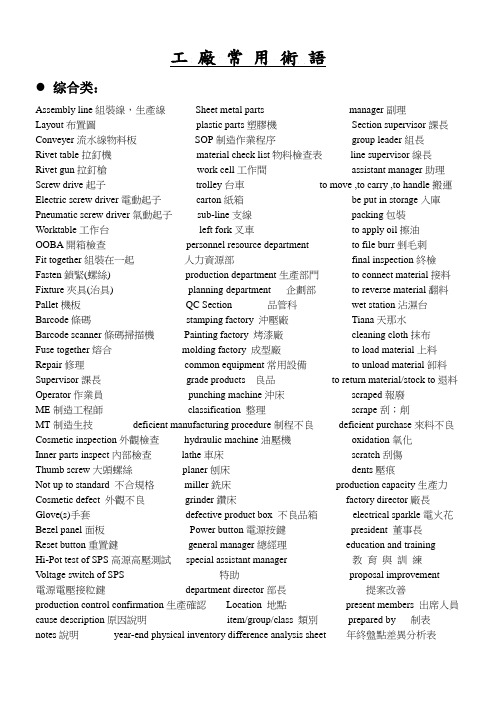
工廠常用術語综合类:Assembly line組裝線,生產線Sheet metal parts manager副理Layout布置圖plastic parts塑膠機Section supervisor課長Conveyer流水線物料板SOP制造作業程序group leader組長Rivet table拉釘機material check list物料檢查表line supervisor線長Rivet gun拉釘槍work cell工作間assistant manager助理Screw drive起子trolley台車to move ,to carry ,to handle搬運Electric screw driver電動起子carton紙箱be put in storage入庫Pneumatic screw driver氣動起子sub-line支線packing包裝Worktable工作台left fork叉車to apply oil擦油OOBA開箱檢查personnel resource department to file burr剉毛刺Fit together組裝在一起人力資源部final inspection終檢Fasten鎖緊(螺絲) production department生產部門to connect material接料Fixture夾具(治具) planning department 企劃部to reverse material翻料Pallet機板QC Section 品管科wet station沾濕台Barcode條碼stamping factory 沖壓廠Tiana天那水Barcode scanner條碼掃描機Painting factory 烤漆廠cleaning cloth抹布Fuse together熔合molding factory 成型廠to load material上料Repair修理common equipment常用設備to unload material卸料Supervisor課長grade products 良品to return material/stock to退料Operator作業員punching machine沖床scraped報廢ME制造工程師classification 整理scrape刮;削MT制造生技deficient manufacturing procedure制程不良deficient purchase來料不良Cosmetic inspection外觀檢查hydraulic machine油壓機oxidation氧化Inner parts inspect內部檢查lathe車床scratch刮傷Thumb screw大頭螺絲planer刨床dents壓痕Not up to standard 不合規格miller銑床production capacity生產力Cosmetic defect 外觀不良grinder鑽床factory director廠長Glove(s)手套defective product box 不良品箱electrical sparkle電火花Bezel panel面板Power button電源按鍵president 董事長Reset button重置鍵general manager總經理education and trainingHi-Pot test of SPS高源高壓測試special assistant manager 教育與訓練V oltage switch of SPS 特助proposal improvement電源電壓接粒鍵department director部長提案改善production control confirmation生產確認Location 地點present members 出席人員cause description原因說明item/group/class 類別prepared by 制表notes說明year-end physical inventory difference analysis sheet 年終盤點差異分析表●系統文件類:QS Quality system 品質系統ES Engineering standardization 工程標准H Huston (美國)休斯敦C Compaq (美國)康柏公司C China 中國大陸A Assembly 組裝(廠)S Stamping 沖壓(廠)P Painting 烤漆(廠)I Intel 英特爾公司T TAIWAN 台灣IWS International workman standard 工藝標准ISO International standard organization國際標准化組織GS General specification 一般規格BOM Bill of material 物料清單ECN Engineering Change Notes 工程更改通知ECO Engineering Change Order 工程更改要求(客戶)PCN Process Change Notice 工序改動通知PMP Product Management Plan 生產管制計劃SIP Specification in process 制程檢驗規范PS Package specification 包裝規范SPEC Specification 規格DWG Drawing 圖面IS Inspection specification 成品檢驗規范SOP Standard operation procedure 制造作業規范●部門名稱類PMC Production & material control 生產和物料控制PPC Production plan control 生產計劃控制MC Manufacture control物料控制ME Manufacture engineering制造工程部PE Project engineering產品工程部A/C Accountant dept會計師P/A Personal & administration人事行政部DC Document center 資料中心QE Quality engineering品質工程(部)QA Quality assurance品質保證(處)QC Quality control品質管制(處)PD Product department生產部LAB Laboratory實驗室IE Industrial engineering工業工程R&D Research & design設計開發部制造統計品管專類SPC Statistical Process Control 統計制程管制SQC Statistical Quality Control 統計品質管制R Range 全距AR Average Range 全距帄均值UCL Upper Control Limit 管制上限LCL Lower Control Limit 管制下限MAX Maximum 最大值MIN Minimum 最小值GRR Gauge Reproducibility & Repeatability 量具之再制性及重測性判斷量可靠與否DIM Dimension 尺寸FREQ Frequency 頻率QCC Quality Control Circle 品質圖QIT Quality Improvement Team品質改善小組PDCA Plan Do Check Action計劃執行檢查總結ZD Zero Defect零缺點QP Quality policy品質方針TQM Total quality Management全面品質管理MRB Material Reject Bill退貨單LQL Limiting Quality Management最低品質水帄RMA Return Material Audit退料認可QAN Quality Ameliorate Notice品質改善活動ADM Absolute Dimension Measurement全尺測量QT Quality Target品質目標7QCTools 7Quality Control Tools 品管七大手法其它OEM Original Equipment Manufacture 原設備制造PC Personal Computer 個人電腦CPU Central Computer 中央處理器NHK North of Hongkong 中國大陸I/O Input/Output 輸入、輸出C=0 Critical=0 極嚴重,不允許5S希臘語整理,整頓,清掃,清潔,素養ANIN Attention 知會ESD Electricity-static Discharge 靜電排放A.S.A.P. As Soon As Possible 盡可能快的D/C Date Code 生產日期碼APP Approve 核准認可承認CHK Check 確認AM Ante Meridian 上午PM Post Meridian 下午CD Compact Dist 光碟CD-ROM Compact Dist Read-Only Memory只讀光碟FDD Floppy Disk Drive 軟碟機HDD Hard Disk Drive 硬碟機REF Reference 僅供參考CONN Connector 連接器ASS’Y Assembly 裝配組裝MAT’Y Material 材料IC Integrated Circuit 集成電路TYP Type 類型WDR Weekly Delivery Requirement 周出貨需求CT Cycle Time 制程周期L/T Lead Time 前置時間(生產前准備時間)S/T Standard Time 標准時間P/M Product Market 產品市場3c Computer, Commumcation ,cunsumer electronic’s 消費性電子5W1H When, Where, Who, What, Why, How to5M Man, machine, Material, Method, Measurement4M1H Man, Material, Money, Method, Time 人力,物力,財務,技術,時間(資源)SQA Strategy Quality Assurance 策略品質保證SSQA Sales and service Quality Assurance 銷售及服務品質保證LRR Lot Reject Rate 批退率BS Brian storming 腦力激蕩EMI Electronic Magnetion Inspect 高磁測試FMIF Frequency Modulatim Inspect 高頻測試USD 美元(美国货币名称)HKD 港币(中国香港地区货币名称)NTD 台币(中国台湾地区货币名称)RMB Renminbi 人民币(中国货币名称)。
- 1、下载文档前请自行甄别文档内容的完整性,平台不提供额外的编辑、内容补充、找答案等附加服务。
- 2、"仅部分预览"的文档,不可在线预览部分如存在完整性等问题,可反馈申请退款(可完整预览的文档不适用该条件!)。
- 3、如文档侵犯您的权益,请联系客服反馈,我们会尽快为您处理(人工客服工作时间:9:00-18:30)。
65 7. A Personal View of Statistical Packages for LinearRegressionJerome VanclayThis module presents some personal observations and views on statistical packages for forestry research, with particular attention on suitability for regression analysis, and important activity in development of systems models. There are many packages able to assist statistical analyses of data, designed for different purposes and audiences. This module illustrates some of the features that are particularly important when choosing a package for linear regression analyses. Among these is the graphical abilities of a package, especially the ease with which data (and residuals) can be plotted, because a model may be judged by the residuals rather than by the R-squared statistic. However, preferences for particular features and approaches are personal, so provided that candidates have the functionality one needs, a package may be chosen on the basis of personal preference. If you don’t like the one you have, or find that it cannot handle your data or your analysis, look for another package. There are many well designed and tested statistical packages available, so it should always be possible to find one that suits your needs and budget.1. INTRODUCTIONTwo-variable and multivariate analysis are important steps in fitting relationships for use in systems models. Many statistical packages for use on personal computers are available with regression capabilities, and there is great variation in range of capabilities, ease of use and cost. This is a personal overview of statistical packages used by the author, emphasizing the utility of the package for fitting curves to data using linear regression. It is not a comprehensive review, and does not consider expensive packages such as SPSS, SAS, and S-plus. Instead, it looks mainly at the free or cheap packages that do not require an annual license fee. Some basic concepts in regression analysis are first introduced, and then a number of packages with regression capabilities are reviewed – specifically Excel, CurveExpert, GLIM, ARC and ViSta.2. SOME BASIC CONCEPTS INSTATISTICSLet’s begin by re-examining the principles underlying curve fitting with regression analysis. Figure 1 is a graph with six data points. I’m going to ask you to draw the single straight line that best describes the trend evident in these points. Your line should be the free-hand equivalent of the least-squares approach used in regression analysis. Understanding the position of this line is fundamental to an understanding of regression analysis. Go ahead, and draw your line.Examining the data graphically, and developing a mental image of the best fit are important first steps in model-building. As Forest Young (2001) puts it in poetry, first, you see your datafor what they seem to bethen, you ask them for the truth- are you what you seem to me?you see with broad expanseyet ask with narrowed poweryou see and ask and seeand ask and see … and ask …with brush you paint the possibilitieswith pen you scribe the probabilitiesfor in pictures we find insightwhile in numbers we find strengthForrest W. Young, 2001Socio-economic Research Methods in Forestry66Now compare your line with those illustrated in Figure 2. Many people draw the first principal component (dotted line)rather than the least squares fit (solid line).They are minimizing the overall distance between the points and their line, but that’s not what linear regression does. Linear regression assumes that the X-values (the numbers on the horizontal axis) are known with certainty, and that any errors are associated only with the Y-values. Thus linear regression minimizes the vertical distances between the points and the lines (Figure 3). In fact, it minimizes the square of those distances – that’s why it’s called the least squares fit, and why one of the measures of goodness-of-fit is the residual mean square (the average of the vertical lines squared). How did your line compare with those in Figure 2?The ver t ic a l li n es in Fi g ur e 3 are known as res i dual s . Ex a mining the resi d uals is a usef u l way to judge the quali t y of a fi t . And because the regr e s s i o n technique is bas e d on the squar e of these res i dual s , you shoul d tak e speci a l note of lar g e res i duals ,whi c h may hav e a str o ng inf l uenc e on the shape and pos i t i on of the fit t ed li n e. Consult any standar d tex t on regress i on anal y s i s for a ful l er explanat i on. The tex t by Cook and Wei s ber g ( 1994) is a hel p ful pl a ce t o begin. Many peopl e judge the quali t y of a fi t us i ng a stati s t i c known as R-s q uared. An R-s q uared of zero indi c at e s that the fi t t e d li n e is no bet t er than a simpl e aver a ge, and an R- squar e d of one reveals a perf e c t fit .When R-squar e d is cl o s e to one, it may indi c at e agood enough.Ans c ombe (1973) created four dat a set s that rev e al some limit a t i ons of R- s quar e d and emphasi s e the need to ex a mi n e data graphic a ll y (Fi g ure 4) . The four dat a set s t h at he formulated hav e exact l y the same linear regress i on es t i m ates (Y = 3.0 + 0. 5 X), exact l y the same res i dual mean squar e (13.75) and exact l y the same R- s quar e d (0. 667) .However, despit e these si m i l ar val u es, the quali t y of the fi t var i es great l yA Personal View of Statistical Packages for Linear Regression67 betrev e al ‘pure er r or’ (t o p left ), an outl i er (bott o m left ), us e of the wrong model (a quadr a t i c ter m may be needed; top ri g ht ), and a case wher e the est i mated trend reli e s ent i r e l y on a singl e poi n t wi t h hi g h leverage (bott o m ri g ht ). Wit h out fur t her infor m ati o n, it is impos s i b l e to judge whic h of thes e model s is suit a bl e, but al l exc e pt the fi r s t cas e war r ant caref u l inv e st i gati o n.A ful l er dis c us s i o n of this ill u st r at i on was giv e n by Ans c ombe (1973) and Wei s ber g (1985), but the import a nc e of pl o t t i n g both the dat a and the model remains obv i ous. Bec a use it is so impor t ant to pl o t the data and examine the poi n ts vi s ual l y ,I am goi n g to cons i der onl y those st a t i s t i c al pack a ges that hav e st r ong gr a phic s capabili t i e s. As For r est Young (2001) say s in hi s poem, it is ‘… in pi c tures we fi n d ins i ght ,whi l e in numbers we f i nd s t r e ngth’.fit a si m ple li n ear regr e ss i on (Y = a + b X) to a small set of data. The data ar e 10 pair s of numbers ,wit h X = 1, 2, 3, …, 10 and Y = X2. Fit t i n g a st r ai g ht line isn’t part i c u larl y appropr i at e, but the R-s q uared is cl o se to one (0. 95) .We cont r as t the ins i ghts we gai n int o thi s analy s i s as we ex a mine the fi v e pac k ages – Ex c el, Cur v eEx p er t, GLIM, ARC and ViSta.3. MICROSOFT EXCELMicroSoft Excel is part of the MicroSoft Office suite of programs, and is available on many computers. It’s not my favourite package, but it is handy and sometimes may be the only statistical software available.To perform regression analysis with Excel, click on ‘Tools’ and on ‘Data Analysis’. Use the slider to find ‘Regression’, select andSocio-economic Research Methods in Forestry68click ‘OK’, and the computer screen should look like Figure 5. If the Tools menu doesn’t include ‘Data Analysis’, it may be necessary to select the add-in: Click on ‘Tools’ and on ‘Add-Ins’, and make sure that the ‘Analysis TookPak’ is selected. If you cannot find the ‘Add-Ins’, ask your computer support people to install the full version of Excel.When performing a regression analysis with Excel, be sure to plot the raw data with the fitted model, and examine the residuals (Choose both the ‘Residual Plots’ and ‘Line Fit Plots’ in the regression dialogue box,Figure 5), and do not rely only on the R-squared and the F-test to judge the adequacy of a model.Statistical procedures in Excel are not always reliable. McCullough and Wilson (1999) reported that the Statistical Reference Dataset of the American National Institute of Standards and Technology reveals problems with Excel’s univariate summary statistics, analysis of variance, linear regression, non-linear regression and random number generation.The problems appear to be present in Excel 4, Excel 95, Excel 97 and Excel 2000. Thusit may be advisable not to use Excel for complex or critical analyses.4. CURVE EXPERTCurveExpert has limited capabilities, fitting curves to (X, Y) pairs of data, but it is an easy way to fit an equation to data.CurveExpert automatically fits and compares 35 built-in regression models and up to 15 additional user-defined models comprising up to 19 parameters. These include both linear and non-linear regression as well as splines. There is no limit on the number of data points. Good documentation is available on the Help menu.Data entry and analysis involves simply typing pairs of data (X, Y) into the built-in spreadsheet, and pressing control-f, and CurveExpert will establish the line of best fit, graph it, and display the corresponding equation (Figure 6). Graphs can be customized and copied onto the clipboardfor use in other Windows applications.Figure 5. Image of a computer screen during a regression analysis with ExcelA Personal View of Statistical Packages for Linear Regression69The major limitation of CurveExpert is that it can use only pairs of data. This is adequate if you want to build a simple yield table based only on stand age, but means that you cannot easily include site index or other explanatory variables.CurveExpert is shareware developed by Daniel Hyams. It is available freely on the i n t e r n e t a t /~dhyams/cvxpt.htm. Users are requested to pay a once-only registration fee of US$40.5. GLIMGLIM (Generalized Linear Interactive Modelling, release 4, Aitkin et al. 1989) is an old favourite, with which I do most of my analyses. It’s compact – the whole system fits on a couple of diskettes. And it is powerful – many analyses can be completed with just a few commands (e.g. Figure 7, in which the data are simulated and analysis completed in one line). But it is not easy to use, so I rarely recommend it to others. There are no pull-down menus, and when GLIM is started, the user is greeted with a simple prompt, as it awaits a command. However, it is flexible and powerful, and is the only package that I can use to complete some analyses (e.g. Phylogenic regression, Grafen 1989). The model fitting illustrated below is achieved with the following commands:$yvariate Y$fit X$display estimates$calculate r=%yv-%fv$plot r %fvThe commands (prefixed with a ‘$’) can be abbreviated, often to one or two characters. The equation r=%yv-%fv calculates the residuals by taking the difference between the y-variables (%yv) and the fitted values (%fv). The graph is a plot of the residuals and the fitted values, and is a good way to judge the quality of a fitGLIM is marketed by the Numerical Algorithms Group in Oxford, UK (NAG Ltd, infodesk@). When I purchased my copy (several years ago), there was a once-only fee of about £100. Crawley (1993) published a helpful guide to the useof GLIM.Figure 6. Image of computer screen while fitting a curve with CurveExpertSocio-economic Research Methods in Forestry706. ARCArc (Cook and Weisberg 1999) is a revision of the program R-code (Cook and Weisberg 1994).R-code greatly influenced my approach to regression analysis, and Arc has become my favourite regression analysis package. It has many innovative tools to allow insightful graphical scrutiny of data.I quickly found Arc intuitive and easy to use.It has pull-down menus, but the user may need to consult the text (Cook and Weisberg 1999) to get the most from the package. Figure 8 shows the analysis of the data used in Figure 6 in Arc , but the real strengths of Arc become apparent only with more complex sets of data.ARC is copyrighted software, but may be distributed and used free of charge. It is a v a i l a b l e f r o m t h e w e b a t /arc.7. VISTAI’ve recently discovered ViSta, the Visual Statistics System (Young 2001), and am still learning it, so I’m not well qualified to present it. But I would like to attention to this package, because I think that it is a useful system and it has many innovative features. It encourages graphical examination of data, and has an innovative way to document what has been done, and to suggest what can be done with the data(Figure 9).Figure 7. Image of computer screen while using the statistics package GLIM (release 4)A Personal View of Statistical Packages for Linear Regression71Figure 8. Image of computer screen while using the statistical package ARCFigure 9. Image of computer screen while using VistaNote: Note symbols to show what has been done in the analysis (top left), and automatic calculation and display of residuals and influences (bottom right) for every analysis.Socio-economic Research Methods in Forestry 72ViSta is free, and can be downloaded from the web at .8. CONCLUDING COMMENTSI am not going to recommend any particular one of these packages – they all have strengths and weaknesses. I like the utility of Excel for data input, the ease of fitting equations CurveExpert, the power of GLIM, the graphics abilities of Arc, and the innovation in ViSta. But Excel has bugs, CurveExpert has limited capabilities, GLIM isn’t easy for non-statisticians, Arc doesn’t offer sufficient help (unless the text is also obtained), and ViSta takes time to learn. Preferences for particular features and approaches are personal, so provided that candidates have the functionality you need, choose a package that you like. If you don’t like the one you have, or find that it cannot handle your data or your analysis, look for another package. There are many good packages out there, and I’m sure that you’ll find one that suits your needs and budget. REFERENCESAitkin, M., Anderson, D., Francis, B. and Hinde, J. (1989), Statistical Modelling in GLIM, Oxford Statistical Science Series 4, Oxford Science Publications, Clarendon Press, Oxford.Anscombe, F.J. (1973), ‘Graphs in statistical analysis’, American Statistician 27(1): 17-21.Cook, R.D. and Weisberg, S. (1994), A n Introduction to Regression Graphic s, Wiley, New York.Cook, R.D. and Weisberg, S. (1999), Applied Regression Including Computing and Graphic s, Wiley, New York.Crawley, M.J. (1993), GLIM for Ecologists, Blackwell, Oxford.Grafen, A. (1989), ‘The phylogenetic regression’,Philosophical Transactions of the Royal Society, London, Series B, 205, 581-98.McCullough, B.D. and Wilson, B. (1999),‘On the accuracy of statistical procedures in Microsoft Excel 97’, Computational Statistics and Data Analysis, 31(1): 27-37.Vanclay, J.K. (1994), Modelli n g Fores t Gr o wth and Yiel d: Appli c at i ons to Mi x ed Tr o pical For e st s, CAB Internati o nal, Wall i ngfor d.Weisberg, S. (1985), Appli e d Li n ear Regress i on,2nd. edn., Wi l ey, New Yor k. Young, F.W. (2001), How to use ViSta: the Visual Statistics System, The Visual Statistics Project, Psychometric Laboratory, University of North Carolina.。