Feature-based non-manifold modeling system to integrate design and
人工智能英文参考文献(最新120个)
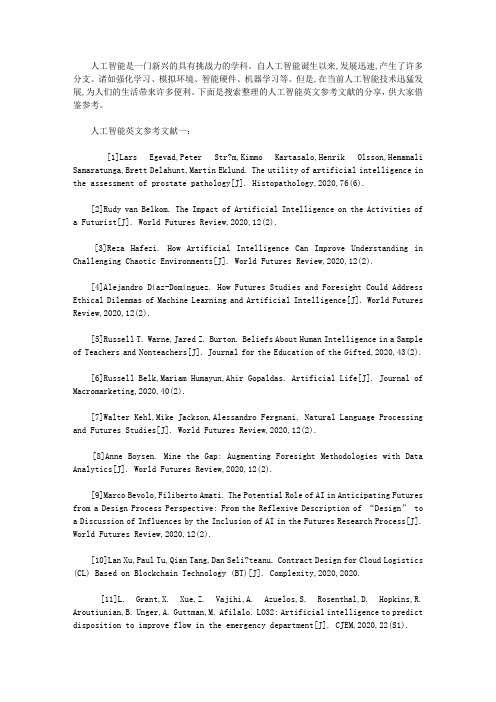
人工智能是一门新兴的具有挑战力的学科。
自人工智能诞生以来,发展迅速,产生了许多分支。
诸如强化学习、模拟环境、智能硬件、机器学习等。
但是,在当前人工智能技术迅猛发展,为人们的生活带来许多便利。
下面是搜索整理的人工智能英文参考文献的分享,供大家借鉴参考。
人工智能英文参考文献一:[1]Lars Egevad,Peter Str?m,Kimmo Kartasalo,Henrik Olsson,Hemamali Samaratunga,Brett Delahunt,Martin Eklund. The utility of artificial intelligence in the assessment of prostate pathology[J]. Histopathology,2020,76(6).[2]Rudy van Belkom. The Impact of Artificial Intelligence on the Activities ofa Futurist[J]. World Futures Review,2020,12(2).[3]Reza Hafezi. How Artificial Intelligence Can Improve Understanding in Challenging Chaotic Environments[J]. World Futures Review,2020,12(2).[4]Alejandro Díaz-Domínguez. How Futures Studies and Foresight Could Address Ethical Dilemmas of Machine Learning and Artificial Intelligence[J]. World Futures Review,2020,12(2).[5]Russell T. Warne,Jared Z. Burton. Beliefs About Human Intelligence in a Sample of Teachers and Nonteachers[J]. Journal for the Education of the Gifted,2020,43(2).[6]Russell Belk,Mariam Humayun,Ahir Gopaldas. Artificial Life[J]. Journal of Macromarketing,2020,40(2).[7]Walter Kehl,Mike Jackson,Alessandro Fergnani. Natural Language Processing and Futures Studies[J]. World Futures Review,2020,12(2).[8]Anne Boysen. Mine the Gap: Augmenting Foresight Methodologies with Data Analytics[J]. World Futures Review,2020,12(2).[9]Marco Bevolo,Filiberto Amati. The Potential Role of AI in Anticipating Futures from a Design Process Perspective: From the Reflexive Description of “Design” to a Discussion of Influences by the Inclusion of AI in the Futures Research Process[J]. World Futures Review,2020,12(2).[10]Lan Xu,Paul Tu,Qian Tang,Dan Seli?teanu. Contract Design for Cloud Logistics (CL) Based on Blockchain Technology (BT)[J]. Complexity,2020,2020.[11]L. Grant,X. Xue,Z. Vajihi,A. Azuelos,S. Rosenthal,D. Hopkins,R. Aroutiunian,B. Unger,A. Guttman,M. Afilalo. LO32: Artificial intelligence to predict disposition to improve flow in the emergency department[J]. CJEM,2020,22(S1).[12]A. Kirubarajan,A. Taher,S. Khan,S. Masood. P071: Artificial intelligence in emergency medicine: A scoping review[J]. CJEM,2020,22(S1).[13]L. Grant,P. Joo,B. Eng,A. Carrington,M. Nemnom,V. Thiruganasambandamoorthy. LO22: Risk-stratification of emergency department syncope by artificial intelligence using machine learning: human, statistics or machine[J]. CJEM,2020,22(S1).[14]Riva Giuseppe,Riva Eleonora. OS for Ind Robots: Manufacturing Robots Get Smarter Thanks to Artificial Intelligence.[J]. Cyberpsychology, behavior and social networking,2020,23(5).[15]Markus M. Obmann,Aurelio Cosentino,Joshy Cyriac,Verena Hofmann,Bram Stieltjes,Daniel T. Boll,Benjamin M. Yeh,Matthias R. Benz. Quantitative enhancement thresholds and machine learning algorithms for the evaluation of renal lesions using single-phase split-filter dual-energy CT[J]. Abdominal Radiology,2020,45(1).[16]Haytham H. Elmousalami,Mahmoud Elaskary. Drilling stuck pipe classification and mitigation in the Gulf of Suez oil fields using artificial intelligence[J]. Journal of Petroleum Exploration and Production Technology,2020,10(10).[17]Rüdiger Schulz-Wendtland,Karin Bock. Bildgebung in der Mammadiagnostik –Ein Ausblick <trans-title xml:lang="en">Imaging in breast diagnostics—an outlook [J]. Der Gyn?kologe,2020,53(6).</trans-title>[18]Nowakowski Piotr,Szwarc Krzysztof,Boryczka Urszula. Combining an artificial intelligence algorithm and a novel vehicle for sustainable e-waste collection[J]. Science of the Total Environment,2020,730.[19]Wang Huaizhi,Liu Yangyang,Zhou Bin,Li Canbing,Cao Guangzhong,Voropai Nikolai,Barakhtenko Evgeny. Taxonomy research of artificial intelligence for deterministic solar power forecasting[J]. Energy Conversion and Management,2020,214.[20]Kagemoto Hiroshi. Forecasting a water-surface wave train with artificial intelligence- A case study[J]. Ocean Engineering,2020,207.[21]Tomonori Aoki,Atsuo Yamada,Kazuharu Aoyama,Hiroaki Saito,Gota Fujisawa,Nariaki Odawara,Ryo Kondo,Akiyoshi Tsuboi,Rei Ishibashi,Ayako Nakada,Ryota Niikura,Mitsuhiro Fujishiro,Shiro Oka,Soichiro Ishihara,Tomoki Matsuda,Masato Nakahori,Shinji Tanaka,Kazuhiko Koike,Tomohiro Tada. Clinical usefulness of a deep learning‐based system as the first screening on small‐bowel capsule endoscopy reading[J]. Digestive Endoscopy,2020,32(4).[22]Masashi Fujii,Hajime Isomoto. Next generation of endoscopy: Harmony with artificial intelligence and robotic‐assisted devices[J]. Digestive Endoscopy,2020,32(4).[23]Roberto Verganti,Luca Vendraminelli,Marco Iansiti. Innovation and Design in the Age of Artificial Intelligence[J]. Journal of Product Innovation Management,2020,37(3).[24]Yuval Elbaz,David Furman,Maytal Caspary Toroker. Modeling Diffusion in Functional Materials: From Density Functional Theory to Artificial Intelligence[J]. Advanced Functional Materials,2020,30(18).[25]Dinesh Visva Gunasekeran,Tien Yin Wong. Artificial Intelligence in Ophthalmology in 2020: A Technology on the Cusp for Translation and Implementation[J]. Asia-Pacific Journal of Ophthalmology,2020,9(2).[26]Fu-Neng Jiang,Li-Jun Dai,Yong-Ding Wu,Sheng-Bang Yang,Yu-Xiang Liang,Xin Zhang,Cui-Yun Zou,Ren-Qiang He,Xiao-Ming Xu,Wei-De Zhong. The study of multiple diagnosis models of human prostate cancer based on Taylor database by artificial neural networks[J]. Journal of the Chinese Medical Association,2020,83(5).[27]Matheus Calil Faleiros,Marcello Henrique Nogueira-Barbosa,Vitor Faeda Dalto,JoséRaniery Ferreira Júnior,Ariane Priscilla Magalh?es Tenório,Rodrigo Luppino-Assad,Paulo Louzada-Junior,Rangaraj Mandayam Rangayyan,Paulo Mazzoncini de Azevedo-Marques. Machine learning techniques for computer-aided classification of active inflammatory sacroiliitis in magnetic resonance imaging[J]. Advances in Rheumatology,2020,60(1078).[28]Balamurugan Balakreshnan,Grant Richards,Gaurav Nanda,Huachao Mao,Ragu Athinarayanan,Joseph Zaccaria. PPE Compliance Detection using Artificial Intelligence in Learning Factories[J]. Procedia Manufacturing,2020,45.[29]M. Stévenin,V. Avisse,N. Ducarme,A. de Broca. Qui est responsable si un robot autonome vient à entra?ner un dommage ?[J]. Ethique et Santé,2020.[30]Fatemeh Barzegari Banadkooki,Mohammad Ehteram,Fatemeh Panahi,Saad Sh. Sammen,Faridah Binti Othman,Ahmed EL-Shafie. Estimation of Total Dissolved Solids (TDS) using New Hybrid Machine Learning Models[J]. Journal of Hydrology,2020.[31]Adam J. Schwartz,Henry D. Clarke,Mark J. Spangehl,Joshua S. Bingham,DavidA. Etzioni,Matthew R. Neville. Can a Convolutional Neural Network Classify Knee Osteoarthritis on Plain Radiographs as Accurately as Fellowship-Trained Knee Arthroplasty Surgeons?[J]. The Journal of Arthroplasty,2020.[32]Ivana Nizetic Kosovic,Toni Mastelic,Damir Ivankovic. Using Artificial Intelligence on environmental data from Internet of Things for estimating solar radiation: Comprehensive analysis[J]. Journal of Cleaner Production,2020.[33]Lauren Fried,Andrea Tan,Shirin Bajaj,Tracey N. Liebman,David Polsky,Jennifer A. Stein. Technological advances for the detection of melanoma: Part I. Advances in diagnostic techniques[J]. Journal of the American Academy of Dermatology,2020.[34]Mohammed Amoon,Torki Altameem,Ayman Altameem. Internet of things Sensor Assisted Security and Quality Analysis for Health Care Data Sets Using Artificial Intelligent Based Heuristic Health Management System[J]. Measurement,2020.[35]E. Lotan,C. Tschider,D.K. Sodickson,A. Caplan,M. Bruno,B. Zhang,Yvonne W. Lui. Medical Imaging and Privacy in the Era of Artificial Intelligence: Myth, Fallacy, and the Future[J]. Journal of the American College of Radiology,2020.[36]Fabien Lareyre,Cédric Adam,Marion Carrier,Juliette Raffort. Artificial Intelligence in Vascular Surgery: moving from Big Data to Smart Data[J]. Annals of Vascular Surgery,2020.[37]Ilesanmi Daniyan,Khumbulani Mpofu,Moses Oyesola,Boitumelo Ramatsetse,Adefemi Adeodu. Artificial intelligence for predictive maintenance in the railcar learning factories[J]. Procedia Manufacturing,2020,45.[38]Janet L. McCauley,Anthony E. Swartz. Reframing Telehealth[J]. Obstetrics and Gynecology Clinics of North America,2020.[39]Jean-Emmanuel Bibault,Lei Xing. Screening for chronic obstructive pulmonary disease with artificial intelligence[J]. The Lancet Digital Health,2020,2(5).[40]Andrea Laghi. Cautions about radiologic diagnosis of COVID-19 infection driven by artificial intelligence[J]. The Lancet Digital Health,2020,2(5).人工智能英文参考文献二:[41]K. Orhan,I. S. Bayrakdar,M. Ezhov,A. Kravtsov,T. ?zyürek. Evaluation of artificial intelligence for detecting periapical pathosis on cone‐beam computed tomography scans[J]. International Endodontic Journal,2020,53(5).[42]Avila A M,Mezi? I. Data-driven analysis and forecasting of highway traffic dynamics.[J]. Nature communications,2020,11(1).[43]Neri Emanuele,Miele Vittorio,Coppola Francesca,Grassi Roberto. Use of CT andartificial intelligence in suspected or COVID-19 positive patients: statement of the Italian Society of Medical and Interventional Radiology.[J]. La Radiologia medica,2020.[44]Tau Noam,Stundzia Audrius,Yasufuku Kazuhiro,Hussey Douglas,Metser Ur. Convolutional Neural Networks in Predicting Nodal and Distant Metastatic Potential of Newly Diagnosed Non-Small Cell Lung Cancer on FDG PET Images.[J]. AJR. American journal of roentgenology,2020.[45]Coppola Francesca,Faggioni Lorenzo,Regge Daniele,Giovagnoni Andrea,Golfieri Rita,Bibbolino Corrado,Miele Vittorio,Neri Emanuele,Grassi Roberto. Artificial intelligence: radiologists' expectations and opinions gleaned from a nationwide online survey.[J]. La Radiologia medica,2020.[46]?. ? ? ? ? [J]. ,2020,25(4).[47]Savage Rock H,van Assen Marly,Martin Simon S,Sahbaee Pooyan,Griffith Lewis P,Giovagnoli Dante,Sperl Jonathan I,Hopfgartner Christian,K?rgel Rainer,Schoepf U Joseph. Utilizing Artificial Intelligence to Determine Bone Mineral Density Via Chest Computed Tomography.[J]. Journal of thoracic imaging,2020,35 Suppl 1.[48]Brzezicki Maksymilian A,Bridger Nicholas E,Kobeti? Matthew D,Ostrowski Maciej,Grabowski Waldemar,Gill Simran S,Neumann Sandra. Artificial intelligence outperforms human students in conducting neurosurgical audits.[J]. Clinical neurology and neurosurgery,2020,192.[49]Lockhart Mark E,Smith Andrew D. Fatty Liver Disease: Artificial Intelligence Takes on the Challenge.[J]. Radiology,2020,295(2).[50]Wood Edward H,Korot Edward,Storey Philip P,Muscat Stephanie,Williams George A,Drenser Kimberly A. The retina revolution: signaling pathway therapies, genetic therapies, mitochondrial therapies, artificial intelligence.[J]. Current opinion in ophthalmology,2020,31(3).[51]Ho Dean,Quake Stephen R,McCabe Edward R B,Chng Wee Joo,Chow Edward K,Ding Xianting,Gelb Bruce D,Ginsburg Geoffrey S,Hassenstab Jason,Ho Chih-Ming,Mobley William C,Nolan Garry P,Rosen Steven T,Tan Patrick,Yen Yun,Zarrinpar Ali. Enabling Technologies for Personalized and Precision Medicine.[J]. Trends in biotechnology,2020,38(5).[52]Fischer Andreas M,Varga-Szemes Akos,van Assen Marly,Griffith L Parkwood,Sahbaee Pooyan,Sperl Jonathan I,Nance John W,Schoepf U Joseph. Comparison of Artificial Intelligence-Based Fully Automatic Chest CT Emphysema Quantification to Pulmonary Function Testing.[J]. AJR. American journal ofroentgenology,2020,214(5).[53]Moore William,Ko Jane,Gozansky Elliott. Artificial Intelligence Pertaining to Cardiothoracic Imaging and Patient Care: Beyond Image Interpretation.[J]. Journal of thoracic imaging,2020,35(3).[54]Hwang Eui Jin,Park Chang Min. Clinical Implementation of Deep Learning in Thoracic Radiology: Potential Applications and Challenges.[J]. Korean journal of radiology,2020,21(5).[55]Mateen Bilal A,David Anna L,Denaxas Spiros. Electronic Health Records to Predict Gestational Diabetes Risk.[J]. Trends in pharmacological sciences,2020,41(5).[56]Yao Xiang,Mao Ling,Lv Shunli,Ren Zhenghong,Li Wentao,Ren Ke. CT radiomics features as a diagnostic tool for classifying basal ganglia infarction onset time.[J]. Journal of the neurological sciences,2020,412.[57]van Assen Marly,Banerjee Imon,De Cecco Carlo N. Beyond the Artificial Intelligence Hype: What Lies Behind the Algorithms and What We Can Achieve.[J]. Journal of thoracic imaging,2020,35 Suppl 1.[58]Guzik Tomasz J,Fuster Valentin. Leaders in Cardiovascular Research: Valentin Fuster.[J]. Cardiovascular research,2020,116(6).[59]Fischer Andreas M,Eid Marwen,De Cecco Carlo N,Gulsun Mehmet A,van Assen Marly,Nance John W,Sahbaee Pooyan,De Santis Domenico,Bauer Maximilian J,Jacobs Brian E,Varga-Szemes Akos,Kabakus Ismail M,Sharma Puneet,Jackson Logan J,Schoepf U Joseph. Accuracy of an Artificial Intelligence Deep Learning Algorithm Implementing a Recurrent Neural Network With Long Short-term Memory for the Automated Detection of Calcified Plaques From Coronary Computed Tomography Angiography.[J]. Journal of thoracic imaging,2020,35 Suppl 1.[60]Ghosh Adarsh,Kandasamy Devasenathipathy. Interpretable Artificial Intelligence: Why and When.[J]. AJR. American journal of roentgenology,2020,214(5).[61]M.Rosario González-Rodríguez,M.Carmen Díaz-Fernández,Carmen Pacheco Gómez. Facial-expression recognition: An emergent approach to the measurement of tourist satisfaction through emotions[J]. Telematics and Informatics,2020,51.[62]Ru-Xi Ding,Iván Palomares,Xueqing Wang,Guo-Rui Yang,Bingsheng Liu,Yucheng Dong,Enrique Herrera-Viedma,Francisco Herrera. Large-Scale decision-making: Characterization, taxonomy, challenges and future directions from an Artificial Intelligence and applications perspective[J]. Information Fusion,2020,59.[63]Abdulrhman H. Al-Jebrni,Brendan Chwyl,Xiao Yu Wang,Alexander Wong,Bechara J. Saab. AI-enabled remote and objective quantification of stress at scale[J]. Biomedical Signal Processing and Control,2020,59.[64]Gillian Thomas,Elizabeth Eisenhauer,Robert G. Bristow,Cai Grau,Coen Hurkmans,Piet Ost,Matthias Guckenberger,Eric Deutsch,Denis Lacombe,Damien C. Weber. The European Organisation for Research and Treatment of Cancer, State of Science in radiation oncology and priorities for clinical trials meeting report[J]. European Journal of Cancer,2020,131.[65]Muhammad Asif. Are QM models aligned with Industry 4.0? A perspective on current practices[J]. Journal of Cleaner Production,2020,258.[66]Siva Teja Kakileti,Himanshu J. Madhu,Geetha Manjunath,Leonard Wee,Andre Dekker,Sudhakar Sampangi. Personalized risk prediction for breast cancer pre-screening using artificial intelligence and thermal radiomics[J]. Artificial Intelligence In Medicine,2020,105.[67]. Evaluation of Payer Budget Impact Associated with the Use of Artificial Intelligence in Vitro Diagnostic, Kidneyintelx, to Modify DKD Progression:[J]. American Journal of Kidney Diseases,2020,75(5).[68]Rohit Nishant,Mike Kennedy,Jacqueline Corbett. Artificial intelligence for sustainability: Challenges, opportunities, and a research agenda[J]. International Journal of Information Management,2020,53.[69]Hoang Nguyen,Xuan-Nam Bui. Soft computing models for predicting blast-induced air over-pressure: A novel artificial intelligence approach[J]. Applied Soft Computing Journal,2020,92.[70]Benjamin S. Hopkins,Aditya Mazmudar,Conor Driscoll,Mark Svet,Jack Goergen,Max Kelsten,Nathan A. Shlobin,Kartik Kesavabhotla,Zachary A Smith,Nader S Dahdaleh. Using artificial intelligence (AI) to predict postoperative surgical site infection: A retrospective cohort of 4046 posterior spinal fusions[J]. Clinical Neurology and Neurosurgery,2020,192.[71]Mei Yang,Runze Zhou,Xiangjun Qiu,Xiangfei Feng,Jian Sun,Qunshan Wang,Qiufen Lu,Pengpai Zhang,Bo Liu,Wei Li,Mu Chen,Yan Zhao,Binfeng Mo,Xin Zhou,Xi Zhang,Yingxue Hua,Jin Guo,Fangfang Bi,Yajun Cao,Feng Ling,Shengming Shi,Yi-Gang Li. Artificial intelligence-assisted analysis on the association between exposure to ambient fine particulate matter and incidence of arrhythmias in outpatients of Shanghai community hospitals[J]. Environment International,2020,139.[72]Fatemehalsadat Madaeni,Rachid Lhissou,Karem Chokmani,Sebastien Raymond,Yves Gauthier. Ice jam formation, breakup and prediction methods based on hydroclimatic data using artificial intelligence: A review[J]. Cold Regions Science and Technology,2020,174.[73]Steve Chukwuebuka Arum,David Grace,Paul Daniel Mitchell. A review of wireless communication using high-altitude platforms for extended coverage and capacity[J]. Computer Communications,2020,157.[74]Yong-Hong Kuo,Nicholas B. Chan,Janny M.Y. Leung,Helen Meng,Anthony Man-Cho So,Kelvin K.F. Tsoi,Colin A. Graham. An Integrated Approach of Machine Learning and Systems Thinking for Waiting Time Prediction in an Emergency Department[J]. International Journal of Medical Informatics,2020,139.[75]Matteo Terzi,Gian Antonio Susto,Pratik Chaudhari. Directional adversarial training for cost sensitive deep learning classification applications[J]. Engineering Applications of Artificial Intelligence,2020,91.[76]Arman Kilic. Artificial Intelligence and Machine Learning in Cardiovascular Health Care[J]. The Annals of Thoracic Surgery,2020,109(5).[77]Hossein Azarmdel,Ahmad Jahanbakhshi,Seyed Saeid Mohtasebi,Alfredo Rosado Mu?oz. Evaluation of image processing technique as an expert system in mulberry fruit grading based on ripeness level using artificial neural networks (ANNs) and support vector machine (SVM)[J]. Postharvest Biology and Technology,2020,166.[78]Wafaa Wardah,Abdollah Dehzangi,Ghazaleh Taherzadeh,Mahmood A. Rashid,M.G.M. Khan,Tatsuhiko Tsunoda,Alok Sharma. Predicting protein-peptide binding sites with a deep convolutional neural network[J]. Journal of Theoretical Biology,2020,496.[79]Francisco F.X. Vasconcelos,Róger M. Sarmento,Pedro P. Rebou?as Filho,Victor Hugo C. de Albuquerque. Artificial intelligence techniques empowered edge-cloud architecture for brain CT image analysis[J]. Engineering Applications of Artificial Intelligence,2020,91.[80]Masaaki Konishi. Bioethanol production estimated from volatile compositions in hydrolysates of lignocellulosic biomass by deep learning[J]. Journal of Bioscience and Bioengineering,2020,129(6).人工智能英文参考文献三:[81]J. Kwon,K. Kim. Artificial Intelligence for Early Prediction of Pulmonary Hypertension Using Electrocardiography[J]. Journal of Heart and Lung Transplantation,2020,39(4).[82]C. Maathuis,W. Pieters,J. van den Berg. Decision support model for effects estimation and proportionality assessment for targeting in cyber operations[J]. Defence Technology,2020.[83]Samer Ellahham. Artificial Intelligence in Diabetes Care[J]. The American Journal of Medicine,2020.[84]Yi-Ting Hsieh,Lee-Ming Chuang,Yi-Der Jiang,Tien-Jyun Chang,Chung-May Yang,Chang-Hao Yang,Li-Wei Chan,Tzu-Yun Kao,Ta-Ching Chen,Hsuan-Chieh Lin,Chin-Han Tsai,Mingke Chen. Application of deep learning image assessment software VeriSee? for diabetic retinopathy screening[J]. Journal of the Formosan Medical Association,2020.[85]Emre ARTUN,Burak KULGA. Selection of candidate wells for re-fracturing in tight gas sand reservoirs using fuzzy inference[J]. Petroleum Exploration and Development Online,2020,47(2).[86]Alberto Arenal,Cristina Armu?a,Claudio Feijoo,Sergio Ramos,Zimu Xu,Ana Moreno. Innovation ecosystems theory revisited: The case of artificial intelligence in China[J]. Telecommunications Policy,2020.[87]T. Som,M. Dwivedi,C. Dubey,A. Sharma. Parametric Studies on Artificial Intelligence Techniques for Battery SOC Management and Optimization of Renewable Power[J]. Procedia Computer Science,2020,167.[88]Bushra Kidwai,Nadesh RK. Design and Development of Diagnostic Chabot for supporting Primary Health Care Systems[J]. Procedia Computer Science,2020,167.[89]Asl? Bozda?,Ye?im Dokuz,?znur Begüm G?k?ek. Spatial prediction of PM 10 concentration using machine learning algorithms in Ankara, Turkey[J]. Environmental Pollution,2020.[90]K.P. Smith,J.E. Kirby. Image analysis and artificial intelligence in infectious disease diagnostics[J]. Clinical Microbiology and Infection,2020.[91]Alklih Mohamad YOUSEF,Ghahfarokhi Payam KAVOUSI,Marwan ALNUAIMI,Yara ALATRACH. Predictive data analytics application for enhanced oil recovery in a mature field in the Middle East[J]. Petroleum Exploration and Development Online,2020,47(2).[92]Omer F. Ahmad,Danail Stoyanov,Laurence B. Lovat. Barriers and pitfalls for artificial intelligence in gastroenterology: Ethical and regulatory issues[J]. Techniques and Innovations in Gastrointestinal Endoscopy,2020,22(2).[93]Sanne A. Hoogenboom,Ulas Bagci,Michael B. Wallace. Artificial intelligence in gastroenterology. The current state of play and the potential. How will it affect our practice and when?[J]. Techniques and Innovations in Gastrointestinal Endoscopy,2020,22(2).[94]Douglas K. Rex. Can we do resect and discard with artificial intelligence-assisted colon polyp “optical biopsy?”[J]. Techniques and Innovations in Gastrointestinal Endoscopy,2020,22(2).[95]Neal Shahidi,Michael J. Bourke. Can artificial intelligence accurately diagnose endoscopically curable gastrointestinal cancers?[J]. Techniques and Innovations in Gastrointestinal Endoscopy,2020,22(2).[96]Michael Byrne. Artificial intelligence in gastroenterology[J]. Techniques and Innovations in Gastrointestinal Endoscopy,2020,22(2).[97]Piet C. de Groen. Using artificial intelligence to improve adequacy of inspection in gastrointestinal endoscopy[J]. Techniques and Innovations in Gastrointestinal Endoscopy,2020,22(2).[98]Robin Zachariah,Andrew Ninh,William Karnes. Artificial intelligence for colon polyp detection: Why should we embrace this?[J]. Techniques and Innovations in Gastrointestinal Endoscopy,2020,22(2).[99]Alexandra T. Greenhill,Bethany R. Edmunds. A primer of artificial intelligence in medicine[J]. Techniques and Innovations in Gastrointestinal Endoscopy,2020,22(2).[100]Tomohiro Tada,Toshiaki Hirasawa,Toshiyuki Yoshio. The role for artificial intelligence in evaluation of upper GI cancer[J]. Techniques and Innovations in Gastrointestinal Endoscopy,2020,22(2).[101]Yahui Jiang,Meng Yang,Shuhao Wang,Xiangchun Li,Yan Sun. Emerging role of deep learning‐based artificial intelligence in tumor pathology[J]. Cancer Communications,2020,40(4).[102]Kristopher D. Knott,Andreas Seraphim,Joao B. Augusto,Hui Xue,Liza Chacko,Nay Aung,Steffen E. Petersen,Jackie A. Cooper,Charlotte Manisty,Anish N. Bhuva,Tushar Kotecha,Christos V. Bourantas,Rhodri H. Davies,Louise A.E. Brown,Sven Plein,Marianna Fontana,Peter Kellman,James C. Moon. The Prognostic Significance of Quantitative Myocardial Perfusion: An Artificial Intelligence–Based Approach Using Perfusion Mapping[J]. Circulation,2020,141(16).[103]Muhammad Asad,Ahmed Moustafa,Takayuki Ito. FedOpt: Towards Communication Efficiency and Privacy Preservation in Federated Learning[J]. Applied Sciences,2020,10(8).[104]Wu Wenzhi,Zhang Yan,Wang Pu,Zhang Li,Wang Guixiang,Lei Guanghui,Xiao Qiang,Cao Xiaochen,Bian Yueran,Xie Simiao,Huang Fei,Luo Na,Zhang Jingyuan,Luo Mingyan. Psychological stress of medical staffs during outbreak of COVID-19 and adjustment strategy.[J]. Journal of medical virology,2020.[105]. Eyenuk Fulfills Contract for Artificial Intelligence Grading of Retinal Images[J]. Telecomworldwire,2020.[106]Kim Tae Woo,Duhachek Adam. Artificial Intelligence and Persuasion: A Construal-Level Account.[J]. Psychological science,2020,31(4).[107]McCall Becky. COVID-19 and artificial intelligence: protecting health-care workers and curbing the spread.[J]. The Lancet. Digital health,2020,2(4).[108]Alca?iz Mariano,Chicchi Giglioli Irene A,Sirera Marian,Minissi Eleonora,Abad Luis. [Autism spectrum disorder biomarkers based on biosignals, virtual reality and artificial intelligence].[J]. Medicina,2020,80 Suppl 2.[109]Cong Lei,Feng Wanbing,Yao Zhigang,Zhou Xiaoming,Xiao Wei. Deep Learning Model as a New Trend in Computer-aided Diagnosis of Tumor Pathology for Lung Cancer.[J]. Journal of Cancer,2020,11(12).[110]Wang Fengdan,Gu Xiao,Chen Shi,Liu Yongliang,Shen Qing,Pan Hui,Shi Lei,Jin Zhengyu. Artificial intelligence system can achieve comparable results to experts for bone age assessment of Chinese children with abnormal growth and development.[J]. PeerJ,2020,8.[111]Hu Wenmo,Yang Huayu,Xu Haifeng,Mao Yilei. Radiomics based on artificial intelligence in liver diseases: where we are?[J]. Gastroenterology report,2020,8(2).[112]Batayneh Wafa,Abdulhay Enas,Alothman Mohammad. Prediction of the performance of artificial neural networks in mapping sEMG to finger joint angles via signal pre-investigation techniques.[J]. Heliyon,2020,6(4).[113]Aydin Emrah,Türkmen ?nan Utku,Namli G?zde,?ztürk ?i?dem,Esen Ay?e B,Eray Y Nur,Ero?lu Egemen,Akova Fatih. A novel and simple machine learning algorithm for preoperative diagnosis of acute appendicitis in children.[J]. Pediatric surgery international,2020.[114]Ellahham Samer. Artificial Intelligence in Diabetes Care.[J]. The Americanjournal of medicine,2020.[115]David J. Winkel,Thomas J. Weikert,Hanns-Christian Breit,Guillaume Chabin,Eli Gibson,Tobias J. Heye,Dorin Comaniciu,Daniel T. Boll. Validation of a fully automated liver segmentation algorithm using multi-scale deep reinforcement learning and comparison versus manual segmentation[J]. European Journal of Radiology,2020,126.[116]Binjie Fu,Guoshu Wang,Mingyue Wu,Wangjia Li,Yineng Zheng,Zhigang Chu,Fajin Lv. Influence of CT effective dose and convolution kernel on the detection of pulmonary nodules in different artificial intelligence software systems: A phantom study[J]. European Journal of Radiology,2020,126.[117]Georgios N. Kouziokas. A new W-SVM kernel combining PSO-neural network transformed vector and Bayesian optimized SVM in GDP forecasting[J]. Engineering Applications of Artificial Intelligence,2020,92.[118]Qingsong Ruan,Zilin Wang,Yaping Zhou,Dayong Lv. A new investor sentiment indicator ( ISI ) based on artificial intelligence: A powerful return predictor in China[J]. Economic Modelling,2020,88.[119]Mohamed Abdel-Basset,Weiping Ding,Laila Abdel-Fatah. The fusion of Internet of Intelligent Things (IoIT) in remote diagnosis of obstructive Sleep Apnea: A survey and a new model[J]. Information Fusion,2020,61.[120]Federico Caobelli. Artificial intelligence in medical imaging: Game over for radiologists?[J]. European Journal of Radiology,2020,126.以上就是关于人工智能参考文献的分享,希望对你有所帮助。
基于Ls-Dyna软件2种材料模型的碳纤维复合材料层合板面内剪切有限元仿真
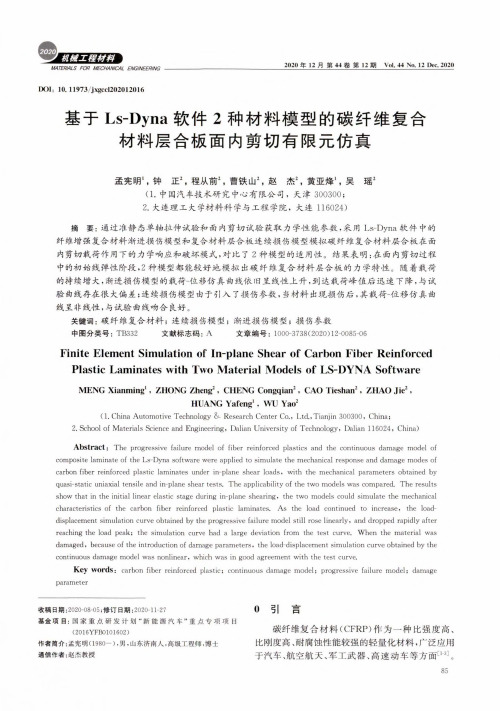
2020年12月第44卷第12期Vol.J4No.12Dec.202() MATERIALS FOR MECHANICAL ENGINEERINGDOI:10.11973/jxgccl202012016基于Ls-Dyna软件2种材料模型的碳纤维复合材料层合板面内剪切有限元仿真孟宪明',钟正S程从前2,曹铁山S赵杰2,黄亚烽-吴瑶2(1.中国汽车技术研究中心有限公司,天津300300;2.大连理工大学材料科学与工程学院,大连116024)摘要:通过准静态单轴拉伸试验和面内剪切试验获取力学性能参数,采用Ls-Dyna软件中的纤维增强复合材料渐进损伤模型和复合材料层合板连续损伤模型模拟碳纤维复合材料层合板在面内剪切载荷作用下的力学响应和破坏模式,对比了2种模型的适用性。
结果表明:在面内剪切过程中的初始线弹性阶段,2种模型都能较好地模拟出碳纤维复合材料层合板的力学特性。
随着载荷的持续增大,渐进损伤模型的载荷-位移仿真曲线依旧呈线性上升,到达载荷峰值后迅速下降,与试验曲线存在很大偏差;连续损伤模型由于引入了损伤参数,当材料出现损伤后.其载荷-位移仿真曲线呈非线性,与试验曲线吻合良好。
关键词:碳纤维复合材料;连续损伤模型;渐进损伤模型;损伤参数中图分类号:TB332文献标志码:A文章编号:1000-3738(2020)12-0085-06Finite Element Simulation of In-plane Shear of Carbon Fiber ReinforcedPlastic Laminates with Two Material Models of LS-DYNA SoftwareMENG Xianming1.ZHONG Zheng2.CHENG Congqian2,CAO Tieshan2.ZHAO Jie2,HUANG Yafeng*,WU Yao2(1.China Automotive Technology&Research Center Co.,Ltd.,Tianjin300300,China;2.School of Materials Science and Engineering,Dalian University of Technology»Dalian116024,China)Abstract:The progressive failure model of fiber reinforced plastics and the continuous damage model of composite laminate of the Ls-Dyna software were applied to simulate the mechanical response and damage modes of carbon fiber reinforced plastic laminates under in-plane shear loads,with the mechanical parameters obtained by quasi-static uniaxial tensile and in-plane shear tests.The applicability of the two models was compared.The results show that in the initial linear elastic stage during in-plane shearing,the two models could simulate the mechanical characteristics of the carbon fiber r&nforced plastic laminates.As the load continued to increase,the loaddisplacement simulation curve obtained by the progressive failure model still rose linearly,and dropped rapidly after reaching the load peak;the simulation curve had a large deviation from the test curve.When the material was damaged,because of the introduction of damage parameters,the load-displacement simulation curve obtained by the continuous damage model was nonlinear,which was in good agreement with the test curve.Key words:carbon fiber reinforced plastic;continuous damage model;progressive failure model;damage parameter收稿日期:2020-08-05;修订日期:2020-11-27基金项目:国家重点研发计划“新能源汽车”重点专项项目(2O16YFBO1O16O2)作者简介:孟宪明(1980—),男,山东济南人,高级工程师•博士通信作者:赵杰教授0引言碳纤维复合材料(CFRP)作为一种比强度高、比刚度高、耐腐蚀性能较强的轻量化材料,广泛应用于汽车、航空航天、军工武器、高速动车等方面口切。
建立自助管理系统英语作文
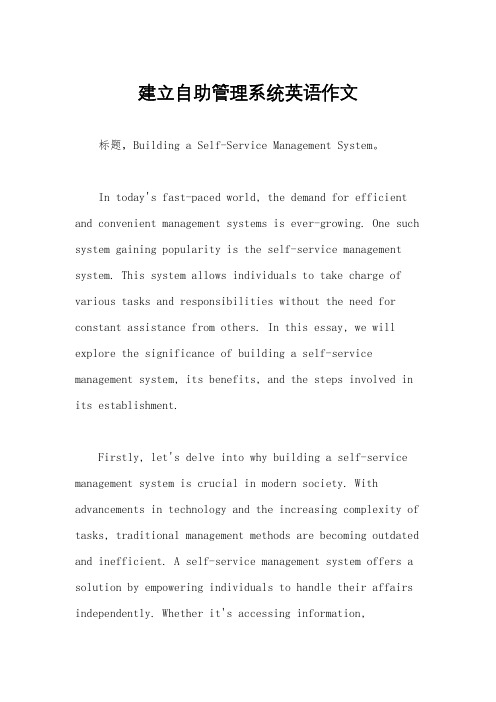
建立自助管理系统英语作文标题,Building a Self-Service Management System。
In today's fast-paced world, the demand for efficient and convenient management systems is ever-growing. One such system gaining popularity is the self-service management system. This system allows individuals to take charge of various tasks and responsibilities without the need for constant assistance from others. In this essay, we will explore the significance of building a self-service management system, its benefits, and the steps involved in its establishment.Firstly, let's delve into why building a self-service management system is crucial in modern society. With advancements in technology and the increasing complexity of tasks, traditional management methods are becoming outdated and inefficient. A self-service management system offers a solution by empowering individuals to handle their affairs independently. Whether it's accessing information,completing transactions, or resolving issues, users can accomplish tasks quickly and conveniently through a user-friendly interface.The benefits of a self-service management system are manifold. One of the most significant advantages is enhanced efficiency. By eliminating the need for manual intervention, processes become streamlined, reducing the time and resources required to perform tasks. Moreover,self-service systems operate 24/7, allowing users to access services at their convenience, irrespective of time or location. This flexibility not only improves user satisfaction but also contributes to overall productivity.Another advantage is cost-effectiveness. Implementing a self-service management system reduces the reliance on human personnel, leading to cost savings for organizations. Additionally, the scalability of these systems enables them to accommodate a large volume of users without incurring substantial expenses. This scalability is particularly beneficial in industries with fluctuating demand, such as customer service and e-commerce.Furthermore, a self-service management system promotes empowerment and autonomy among users. By providing themwith the tools and resources to manage their affairs independently, individuals feel a sense of control and ownership over their actions. This empowerment fosters confidence and self-reliance, attributes that areinvaluable in both personal and professional spheres.Now, let's outline the steps involved in establishing a self-service management system:1. Assess Needs and Objectives: Begin by conducting a thorough assessment of the organization's requirements and goals. Identify the tasks and processes that can be automated or transitioned to self-service.2. Choose the Right Technology: Select a reliable and user-friendly platform for building the self-service system. Consider factors such as accessibility, security, and scalability.3. Design User Interface: Develop an intuitive andeasy-to-navigate interface that caters to the needs of diverse users. Incorporate features such as search functionality, personalized dashboards, and clear instructions.4. Integrate Systems: Integrate the self-service management system with existing databases, applications, and communication channels to ensure seamless operation and data exchange.5. Provide Comprehensive Support: Offer comprehensive support resources, including FAQs, tutorials, and troubleshooting guides, to assist users in navigating the system effectively.6. Gather Feedback: Continuously gather feedback from users to identify areas for improvement and refine theself-service system accordingly.7. Ensure Security and Compliance: Implement robust security measures to safeguard sensitive information andensure compliance with relevant regulations, such as GDPRor HIPAA.8. Promote Adoption: Launch a targeted marketing campaign to promote the benefits of the self-service management system and encourage adoption among users.In conclusion, building a self-service managementsystem is paramount in the digital age, offering efficiency, cost-effectiveness, and empowerment. By following the outlined steps and leveraging appropriate technology, organizations can establish a system that enhances user experience and drives success in the long term. Embracing self-service management is not just a trend but a strategic imperative for organizations looking to thrive in a competitive landscape.。
微小型四旋翼无人直升机建模及控制方法研究

第1页
国防科学技术大学研究生院学位论文 ABSTRACT
Micro/mini quadrotor is all excellent,novel vertical take-offand landing Unmanned Aerial VehielefOAV)for both military and civilian usages.Based OR a summary of the research status quo,the key technologies and the future applications of the micro/mini quadrotor,this paper concentrates on its special characteristics,mainly researched the problems On mathematical modeling,nonlinear con仕oller and state estimation.Some important theoretical analysis and
s协n酊ofthe system is analyzed.Simulations show that the proposed controllers are validity.
Thirdly,the Active Disturbances Rejection Con廿oilem(ADRC)are designed for the direct driven states ofthe quadrotor to stabilize the vehicle and control the flight height;the PD-ADRC double dosed loops are in仃oduced to diminish the zero d)m珊ni晦then the Lyapunov stability of the doublo closed loops is analyzcd’SO that the quadrotr call hover.11圮validity of these
基于MIGA算法的完全对称翼型优化设计方法研究

Chinese Journal of Turbomachinery Vol.65,2023,No.5Research on Optimization Design Methodology for FullySymmetric Airfoil Profile Based on MIGA Algorithm *Sen Zhang 1,*Yi-ming Gao 1Si-yu Tian 2Hua-xing Li 3De-ke Xi 3(1.School of Mechanical and Power Engineering,Henan Polytechnic University;2.Department of Mechanical Engineering,North China Electric Power University;3.Institute of Aeronautics,Northwestern Polytechnical University)Abstract:A parametric design method was proposed to express the fully symmetric airfoil profile by using the fiveorder Bezier polynomials with six control points.The airfoil profile is controlled by six dimensionless abscissa and ordinate control coefficients and the maximum relative thickness of the airfoil.Furthermore,the Fortran language was used to compile the parametric design program code of the fully symmetric airfoil,which was integrated with ICEM grid generation program and Fluent flow field simulation program into the Isight automatic optimization platform.The multiisland genetic algorithm was used to solve the corresponding objective function.And,the optimization design of fully symmetric airfoil was realized.Taking the elliptical airfoil with a relative thickness of 16%as a reference,and taking 4,8and 12degrees as the design angles of attack,the airfoil A was designed for Re =2.5×106.The comparison results between A and reference airfoil profile show that the profile of the optimized airfoil A is close to the diamond shape,and its loading decreases in the area of x /cϵ(0.04,0.3)while increases in the area of x /cϵ(0.3,0.96).The loading distribution along the chord line is more balanced.In addition,under the design angles of attack,the lift drag ratio of optimized airfoil A has increased by 28.56%and 17.64%,respectively.Under the nondesign angles of attack,its aerodynamic performance has also been significantly improved,which confirms the reliability of the optimization design method constructed in this work and lays a good theoretical foundation for the optimization design of other complex reversible airfoils.Keywords:Reversible Airfoil Profile;Bezier Curves;Isight Platform;Multi-island Genetic Algorithm;Optimization Design摘要:提出了一种利用6控制点5阶Bezier 多项式对完全对称翼型进行表达的参数化设计方法,并通过6个无量纲的横纵坐标控制系数和翼型最大相对厚度控制翼型型线。
“101_计划”背景下基于OBE-CDIO_理念的材料专业实验教学探索
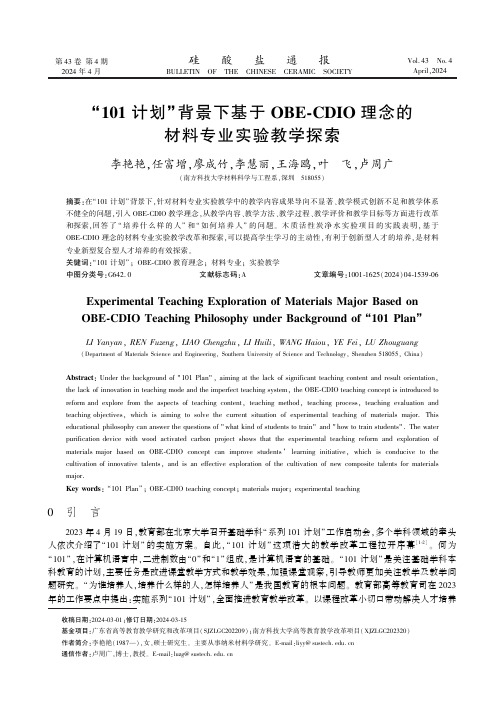
第43卷第4期2024年4月硅㊀酸㊀盐㊀通㊀报BULLETIN OF THE CHINESE CERAMIC SOCIETY Vol.43㊀No.4April,2024101计划 背景下基于OBE-CDIO 理念的材料专业实验教学探索李艳艳,任富增,廖成竹,李慧丽,王海鸥,叶㊀飞,卢周广(南方科技大学材料科学与工程系,深圳㊀518055)摘要:在 101计划 背景下,针对材料专业实验教学中的教学内容成果导向不显著㊁教学模式创新不足和教学体系不健全的问题,引入OBE-CDIO 教学理念,从教学内容㊁教学方法㊁教学过程㊁教学评价和教学目标等方面进行改革和探索,回答了 培养什么样的人 和 如何培养人 的问题㊂木质活性炭净水实验项目的实践表明,基于OBE-CDIO 理念的材料专业实验教学改革和探索,可以提高学生学习的主动性,有利于创新型人才的培养,是材料专业新型复合型人才培养的有效探索㊂关键词: 101计划 ;OBE-CDIO 教育理念;材料专业;实验教学中图分类号:G642.0㊀㊀文献标志码:A ㊀㊀文章编号:1001-1625(2024)04-1539-06Experimental Teaching Exploration of Materials Major Based on OBE-CDIO Teaching Philosophy under Background of 101PlanLI Yanyan ,REN Fuzeng ,LIAO Chengzhu ,LI Huili ,WANG Haiou ,YE Fei ,LU Zhouguang(Department of Materials Science and Engineering,Southern University of Science and Technology,Shenzhen 518055,China)Abstract :Under the background of "101Plan",aiming at the lack of significant teaching content and result orientation,the lack of innovation in teaching mode and the imperfect teaching system,the OBE-CDIO teaching concept is introduced to reform and explore from the aspects of teaching content,teaching method,teaching process,teaching evaluation and teaching objectives,which is aiming to solve the current situation of experimental teaching of materials major.This educational philosophy can answer the questions of "what kind of students to train"and "how to train students".The water purification device with wood activated carbon project shows that the experimental teaching reform and exploration of materials major based on OBE-CDIO concept can improve students learning initiative,which is conducive to the cultivation of innovative talents,and is an effective exploration of the cultivation of new composite talents for materials major.Key words : 101Plan ;OBE-CDIO teaching concept;materials major;experimental teaching收稿日期:2024-03-01;修订日期:2024-03-15基金项目:广东省高等教育教学研究和改革项目(SJZLGC202209);南方科技大学高等教育教学改革项目(XJZLGC202320)作者简介:李艳艳(1987 ),女,硕士研究生㊂主要从事纳米材料学研究㊂E-mail:liyy@通信作者:卢周广,博士,教授㊂E-mail:luzg@ 0㊀引㊀言2023年4月19日,教育部在北京大学召开基础学科 系列101计划 工作启动会,多个学科领域的牵头人依次介绍了 101计划 的实施方案㊂自此, 101计划 这项浩大的教学改革工程拉开序幕[1-2]㊂何为 101 ,在计算机语言中,二进制数由 0 和 1 组成,是计算机语言的基础㊂ 101计划 是关注基础学科本科教育的计划,主要任务是改进课堂教学方式和教学效果,加强课堂观察,引导教师更加关注教学及教学问题研究㊂ 为谁培养人,培养什么样的人,怎样培养人 是我国教育的根本问题㊂教育部高等教育司在2023年的工作要点中提出:实施系列 101计划 ,全面推进教育教学改革㊂以课程改革小切口带动解决人才培养1540㊀评述广角硅酸盐通报㊀㊀㊀㊀㊀㊀第43卷模式大问题,实现高等教育改革创新发展新突破[3]㊂南方科技大学自建立以来,一直致力于探索创新型人才培养模式,争创中国特色社会主义大学范例,学校的办学特色为 创知㊁创新㊁创业 ㊂通过不断探索与实践,学校入选第二轮 双一流 建设高校名单㊂实验教学作为本科阶段最重要的实践教学环节,能够充分展现学校多元化㊁全方位的实践教育水平,是 双一流 建设中学科建设和人才培养中极为重要的实践组成部分㊂因此, 双一流 建设高校也需要有一流的实验教学作为支撑[4]㊂目前,高校专业实验教学中普遍存在验证性实验内容占比偏大,开放性和设计性的综合性实验项目占比偏少,传统灌输式课堂较多,翻转课堂较少等现状,不利于提升学生的学习主动性和积极性,也不利于学生创新能力的培养㊂因此,在 101计划 和 双一流 建设背景下,材料专业实验教学如何以学生为中心,以成果为导向,不断探索先进的教育理念,并将其融入人才培养的全过程,探索创新型人才的培养,是急需解决的首要任务,也是回答 怎样培养人 的根本问题的有效途径㊂近年来,以麻省理工学院为核心的高校提出了CDIO工程教育理念,成为工程教育的最新研究成果㊂因此,为了提升实验教学效果和学生的创新能力以及专业综合竞争力,培养适合时代发展需要的高层次复合型材料专业人才,教学团队开展了基于OBE-CDIO理念的材料专业实验教学改革与探索,旨在通过引导学生独立开展实验㊁设计产品并深入探究,实现 知识㊁能力和价值 的全面培养目标,回答 怎样培养人 的问题 [5-6]㊂1㊀OBE-CDIO教学理念内涵OBE(outcome based education)是一种基于成果的教育模式,也被称为成果导向教育㊂这里所说的成果是学生最终取得的学习结果,是学生通过某一阶段后所能达到的最大能力㊂教师需要向学生阐明学习成果,并结合多元化㊁个性化的学习要求,引导学生通过自我挑战和学习过程发挥个人潜能,再根据学生的成果反馈来改进原有的课程设计与课程教学㊂这种以最终目标为起点㊁反向进行课程设计的方式,有助于开展教学活动的探索㊂CDIO:是C构思(conceive)㊁D设计(design)㊁I实现(implenment)㊁O运作(operate),它以产品研发到运行的生命周期为载体,通过项目推行过程引导学生沉浸式融入,充分调动学生的主观能动性和积极性㊂CDIO教学理念是近年来国际工程教育改革的最新成果㊂截至目前,我国有一百余所高校加入了 CDIO工程教育联盟 ㊂OBE是成果导向教育,回答了 培养什么样的人 的问题,旨在将本科生培养成符合发展需要的高层次复合型人才㊂CDIO教育模式回答了 如何培养人 的问题,旨在实现 知识㊁能力和价值 的全面培养目标㊂OBE-CDIO人才培养理念是两个理念的有效结合与互补,能够有效实现创新型复合型人才的发展㊂图1㊀基于OBE-CDIO理念的材料学综合性实验项目框架㊀第4期李艳艳等: 101计划 背景下基于OBE-CDIO理念的材料专业实验教学探索1541日常生活所见皆材料,材料专业与我们的生活紧密相关,是应用性和实践性都很强的专业,而专业的实践教学在培养学生创新能力和综合素养方面发挥着重要的作用[7]㊂因此,如何增强学生的创新能力和综合素养,一直是我校关注的问题㊂我校材料系教学实验室自成立以来,不断进行改革与探索㊂在实验教学中,基于OBE-CDIO理念开展综合性实验项目,教学框架如图1所示㊂以产品为成果目标,以 材料合成-材料表征-性能测试-产品应用 为主线,让学生在实践中充分学习材料的制备方法㊁表征技术㊁性能测试和产品制作㊂2㊀基于CDIO理念的实验教学改革与探索通过一系列的教学改革与探索,我校材料科学与工程专业的实验课程构建了 理化基础-专业基础-专业方向-综合设计 的四级实验平台,设置了 实验课程-实验项目-实验知识和技能 三个层次的模块化实验课程和项目体系,深入探索了满足新工科和 双一流 建设背景下创新型人才培养需求的项目引导式教学模式㊂因此,基于OBE-CDIO理念在材料学实验教学的项目引导式教学中,开展材料学综合实验的OBE-CDIO 教学,其与传统教学的对比如图2所示㊂图2㊀基于OBE-CDIO理念的教学与传统教学的对比2.1㊀优化实验内容,建设设计性㊁综合性实验项目在教学内容上,整合现有实验内容,基于理化基础㊁专业基础和专业方向的相关知识进行梳理,特别选取与基础知识关联并具有开放性和设计性的项目,并将其设置为综合性实验项目,供学生选择㊂例如,高灵敏度酒精测试仪的制备与应用,学生在‘材料合成与表征实验“课程中已经学习了半导体氧化物气敏传感器的制备及应用,这为项目的设计与开展奠定了基础㊂在此项目设计中,学生首先需要拆解商业的酒精测试仪,分析每部分的组成,特别是气敏传感器器件的结构与组成㊂通过这一过程,学生可以了解材料在器件中的具体应用以及材料加工工艺㊂基于OBE反向设计实验内容,在设计产品中结合CDIO理念开展实验设计与实施,为实现OBE-CDIO教育理念奠定基础㊂2.2㊀改变教学方法,引入多样化教学方法验证性实验的教学方法多为注入式教学和演示实验,教学方法单一,缺乏灵活性和多样性㊂基于CDIO 的教学理念,需要在教学过程中不断与学生互动交流,需采用多样化的教学方法,如:案例式教学,引入工程案例,为学生设计产品提供参考;互动式教学,在开展实验和设计产品过程中,需不断地进行探讨;探究式教学,在实验过程中,难免遇到实验失败或者产品设计不合理的情况,需要反复尝试进行探究,加强探究式学习㊂以光敏传感器的制作与应用实验项目为例,我们结合商业化的光敏电阻,指导将每个涂层刮开,分析材料组成,引导学生思考材料的加工工艺,从而自主设计光敏传感器的制作流程㊂因此,基于OBE-CDIO理念,可以引入多样化的教学方法,增强课堂互动㊂1542㊀评述广角硅酸盐通报㊀㊀㊀㊀㊀㊀第43卷2.3㊀开展翻转课堂传统课堂以教师为中心,学生被动学习㊂而基于OBE-CDIO理念的实验教学改革,是以学生为中心,以成果为导向,开展翻转课堂㊂教师给定目标产品以及具体要求,学生在课前调研产品相关资料及查阅大量文献,据此设计实验方案㊂课堂上,学生以小组为单位开展实验,若有疑问,及时与小组成员和老师进行交流讨论㊂学生成为课堂的主人,而老师扮演助教的角色㊂翻转课堂的开展,有利于激发学生的学习兴趣,增强学习的主动性及探究性,提升学习效果㊂2.4㊀加强过程性评价基于OBE-CDIO理念的设计性实验项目的开展,注重构思㊁设计㊁实现和运行的过程,可以体现自主学习㊁团队协作㊁沟通表达㊁探究学习等能力㊂通过调研文献㊁设计实验㊁开展实验㊁分析结果㊁解决问题㊁口头汇报及撰写报告等方面形成过程性评价,重点评价学生自主学习能力㊁分析问题和解决问题的创新能力以及团队合作能力㊂2.5㊀融入课程思政,加强育人目标以实验项目为载体,深入挖掘实验项目的思政元素,在推行项目引导式教学的各个环节中,充分融入思政元素㊂基于OBE-CDIO理念的教学,育人目标不只是由知识目标组成,而是由 知识目标㊁能力目标和价值目标 组成的三重目标㊂以在 材料微纳加工的实验 为例,我们结合我国光刻技术的 卡脖子 现状,让学生意识到材料专业科学研究的重要性,培养学生的家国情怀㊂项目以小组为单位开展,在实验项目的设计与探究中,培养学生的团队合作意识以及集体主义精神,提升学生的沟通与交流水平㊂在产品设计与探究过程中,失败是难以避免的,培养学生抗挫折能力以及不服输的勇气㊂同时,在实验探究中,培养学生的严谨务实的科研精神和精益求精的工匠精神,提升学生的创新能力[8]㊂通过在教学内容㊁教学方法㊁教学过程㊁教学评价及教学目标的改革与探索,极大地提升了学生学习的主动性,增强了课堂互动效果,激发了学生的学习兴趣,加强了教学反思与总结,有利于培养创新型复合型人才㊂3㊀基于OBE-CDIO理念的木质活性炭净水实验项目探究目前,在材料学综合实验课程中,基于OBE-CDIO理念已开展了多项实验项目,接下来将以 木质活性炭净水实验项目 为例,从教师的教学㊁学生的学习和教学的成效进行阐述[9]㊂3.1㊀教师的多样化教学教师主要提供指导和建议,同时扮演助教的角色㊂首先教师需要介绍商业净水装置,并引导学生通过拆解净水装置,了解其组成及结构㊂学生需要制备其中的核心净水材料活性炭,然后再设计制作一套净水装置㊂因此,教师需要讲解木质活性炭净水实验项目的基本介绍及要求,在学生设计活性炭合成实验方案及净水装置时提供指导建议,在学生合成木质活性炭和表征时进行辅助支持㊂实验课堂为翻转课堂,教师为学生开展实验保驾护航,在学生制备和表征活性炭㊁进行性能测试及设计净水装置时,及时进行指导并答疑解惑;在学生项目汇报时进行指导和点评,对学生提交的实验报告进行批阅㊂3.2㊀学生的探究式学习学生需要查阅大量木质活性炭相关文献,并选择合适的活性炭原料,如松塔㊁花生壳㊁核桃壳㊁椰壳等,设计活性炭合成和表征的实验方案,并以小组为单位开展实验,此外,需要结合3D打印知识设计并打印净水装置㊂整个实验项目过程中,学生为教学活动的主导,自主开展实验,并进行项目探究㊂实验项目结束,需要进行项目汇报,并完成实验报告㊂以选择松塔为原材料的小组为例,学生每人设计一份实验方案,课堂上与老师和同组同学交流讨论后,形成一份小组的实验方案㊂将松塔洗净㊁烘干并粉碎,过筛进行活化处理后烘干并放入马弗炉中进行煅烧,即可制得活性炭材料㊂在此过程中,可探究松塔的尺寸㊁活化剂的选择及活化时间㊁煅烧温度及煅烧时间等不同条件对活性炭性能的影响㊂通过‘木质活性炭试验方法亚甲基蓝吸附值的测定“(GB/T12496.10 1999)和‘木质活性炭试验方法碘吸附值的测定“(GB/T12496.8 2015)这两个国家标准,分别测定合成的活性炭材料对亚甲基蓝的吸附性能和碘吸附值,从而确定不同条件下合成的木质活性炭的净水性能,进而筛选合㊀第4期李艳艳等: 101计划 背景下基于OBE-CDIO理念的材料专业实验教学探索1543适的条件㊂此外,对于木质活性炭进行一系列的形貌和结构表征,如扫描电子显微镜㊁X射线衍射仪及比表面积测试等㊂在材料合成和表征的过程中,设计制作净水装置,并用3D打印进行产品打印,学生绘制的净水装置组件如图3所示,在此过程中,学生深入了解产品的设计㊁制作与修改等,最终完成净水装置的设计㊂图3㊀学生设计的净水装置组件3.3㊀教学的成效基于OBE-CDIO理念的木质活性炭净水实验项目探究如图4所示㊂以净水装置为导向,通过实验项目的构思㊁设计㊁实践和运作,可以培养学生的调研精神㊁团队精神和探究精神㊂此外,有一组同学基于此实验项目,参加第十一届广东大学生材料创新大赛,获得无机非金属材料分赛区三等奖㊂以赛促教,以赛促学㊂通过参加竞赛,进一步提升了学生的综合素养㊂图4㊀基于OBE-CDIO理念的木质活性炭净水实验项目探究1544㊀评述广角硅酸盐通报㊀㊀㊀㊀㊀㊀第43卷4㊀结㊀语基于OBE-CDIO理念,在材料专业的综合实验教学中,以产品设计为导向,开展项目引导式教学,在教学内容㊁教学方法㊁教学过程㊁教学评价和教学目标等方面进行改革与探索,有效提升了学生学习的积极性和主动性,增强了学生分析问题㊁发现问题和解决问题的综合素养,为 101计划 背景下拔尖创新人才的培养奠定基础,对于实验教学的改革具有重要的指导意义㊂如何将项目引导式教学的内容开展得更丰富,产品设计得更全面,将是我们进一步探索的目标㊂参考文献[1]㊀孙㊀滔. 101计划 ,低调实施中[N].中国科学报,2023-08-17(3).[2]㊀张永刚.推开 课堂 一扇窗:参与101计划课堂提升工作的感悟[J].计算机教育,2022(9):5-6.[3]㊀陈㊀曦.以课改小切口解决人才培养大问题[N].科技日报,2023-09-13(5).[4]㊀张海峰. 双一流 背景下的一流实验室建设研究[J].实验技术与管理,2017,34(12):6-10.[5]㊀李福平,白力静,汤玉斐,等.基于CDIO理念的 材料科学基础 课程思政设计与实施[J].科教导刊,2021(23):148-151.[6]㊀郭㊀勇,陈玉霞,涂道伍,等.一流本科专业背景下基于CDIO工程教育模式的木材科学与工程专业实践教学体系改革研究[J].中国多媒体与网络教学学报(上旬刊),2023(3):36-39.[7]㊀秦来顺,唐㊀高,赵春鱼.材料计量与标准化特色新工科人才培养的探索与实践:以中国计量大学材料科学与工程专业为例[J].硅酸盐通报,2023,42(4):1513-1518.[8]㊀程㊀化,卢周广,王海鸥,等.课程思政在 材料学综合实验 项目引导式教学法中的实践与探索[J].云南民族大学学报(自然科学版),2023,32(2):271-276.[9]㊀李艳艳,刘昱晟,王洪轩,等.木质活性炭净水实验探究[J].山东化工,2022,51(5):211-212.。
Atlas Copco 产品说明书.pdf_1718558211.4035933

DZS mono-claw &DZM multi-claw vacuum & low pressure solutionsThere is always abetter wayFor more than a century this guiding principle has drivenAtlas Copco’s engineers and designers to develop new technologies and revolutionize productivity in the compressed air and vacuum world. It is the driving force in everything we do. Many of the Group’s products have become global success stories. T he innovation of a rotary screw vacuum pump with Variable Speed Drive delivering on average 50% energy savings, is one of them.Successful companies bring innovative products that contribute to the productivity of their customers. Premium products are technologically more advanced. On the other hand a brilliant design that offers simplicity is also innovation. Y ou should be offered a solution with the lowest cost of ownership. T o score better than competition, innovation is key. What we want to achieve for you are lower lifecycle costs, higher productivity, less energy consumption, simple operation and control and also easy maintenance.That is also what we aimed for when designing our new dry claw vacuum solutions: they stand for sustainable productivity.Connect and empowerThousands of applications – central systems in hospitals, CNC routing, pneumatic conveying, milking parlors – share a common need: vacuum. And that is what Atlas Copco provides – safely, reliably, cost-effectively and continuously. Connect with Atlas Copco to get the utility you need to empower your processes and operations, and drive your productivity.Reliability you can trustFrom our manufacturing base in Antwerp, we have delivered tens of thousands of claw pumps. T hey are working quietly and efficiently in all corners of the world. T hey are characterized by a low failure rate over a long lifetime, even in the most challenging conditions.Masters of innovationThe durability of our claw pumps is rooted in our expertise. We acquired Edwards, the company that first introduced claw technology to vacuum applications in the high tech electronics industry. In additionAtlas Copco was the first to innovate multi-claw technology for compressors. T he combined knowledge and experience of these two companies leads to low cost, efficient and reliable products.Fully certifiedOur dry claw machines meet the demanding requirements of ISO 9001, ISO 14001 and OHSAS 18001, as verified by Lloyds of London. T hey are backed up a market-leading warranty and a service support network across 180 countries. T he result? 24/7 assurance that your products and your processes are constantly keptin the best possible working order.Efficient pumps with low lifecycle costsThe new range based on Atlas Copco’s dry claw technology features an innovative design which createsa new benchmark in the claw pump market. T he simplicity, robustness, efficiency and contaminant handling capability of these pumps make them the smart choice for your application. Built to the exacting standards and quality demanded by our customers, the new dry claw pump provides you with a trouble-free and cost-effective solution to meet your needs.Built to lastManufactured on the fundamentals of Atlas Copco compressor design,these pumps feature some innovative construction materials and coatingsfor a lifetime of reliable operation even in harsh conditions.Easy maintenanceLong life bearings and seals, a separate and isolated pumping element,and the simplest of designs suited for quick access to the pumpingchamber make for easy cleaning in the event of product carry-over.Unrivalled performance• Extensive product range.• Unrivalled ultimate vacuum level.• Market leading performance.• Best in class power consumption.• Minimal machine lifecycle costs.• Low noise levels.Designed for a long life of reliable, efficient operationThe dry claw machine boasts some fantastic design features including stainless steel corrosion resistant claws. T he renowned Atlas Copco surface coating applied to the pumps process chambers has proven to be unrivalled in our compressors.Upgraded bearings and sealsBased on proven compressor and screw vacuum pump design for long trouble free life even in the harshest applications.4Compact machine with a small footprintIdeally suited for replacement market, compressor house installations and OEM machinery.5Cleverly designed element• Easily accessible pumping chamber for cleaning without accessing the gearbox and disturbing the timing. • Modular design allows flexibility and efficiency in terms of maintenance and life cycle costs.3Set-point control option• Allows you to optimize the energy you use to maintain your process duty point.• The lowest possible flow will be delivered to match your required duty point or speed – nothing is wasted.7Variable Speed Drive compatible (VSD)• Significant energy savings: up to 50% compared to fixed speed machines.• Improved response to varying demand.• Opportunity to replace multiple machines and centralize pumps in one location to optimize system performance.6Innovative features• Dry (oil-free) pumping chamber.• Efficient motor with variable speed option for complete controllability.• Simple maintenance with contact-free internals eliminating wear.• Cool running and simple air cooling.• Compact design with a small footprint.1Built-in corrosion resistance (standard)• Corrosion resistant stainless steel claws.• Durable coated pumping chamber.• Suitable for many harsh applications.2Multi-claw vacuum systemA complete multi-claw vacuum pumping system in a box. A solution perfectly designed to meet your larger process demands with a central vacuum system based on the renowned Atlas Copco compressor philosophy. T his vacuum pumping package offers a completely controllable system ranging from 44 to 1,230 m3/h integrated into one cabinet with a simple ‘plug & play’ concept.Low noiseAtlas Copco prides itself on offering environmentally friendlysolutions and noise plays a big part. Our reputation for designingand building noise reducing canopies is renowned in the industryand comes from many years of experience in the worldof compressors.Plug & playThis concept of design greatly reduces complexity and installation costscompared to other central systems designs. T he package not only consistsof the pumps but also comprises the common inlet manifold and anexhaust manifold resulting in two simple pipe connections.The control system including the Variable Speed Drive and all necessaryprocess transmitters and safety devices are incorporated in a ‘coolzone’ adding to its simplicity and reliability.Complete controllabilityAtlas Copco’s DZM-VSD multi-vacuum pump controllers allows the DZM package to start/stop and control multiple vacuum pumps simultaneously according to set working pressures. T he controller comes with a built-in frequency inverter for the lead pump and the lag pumps are started with soft starters to lighten the load and lengthen the life of the pump.Fixed speed (these base load machines could also be your old vacuum pumps)Vacuum demandVSDSave energy & cut costsThanks to smart algorithms, thecontroller delivers an optimal blend of product performance. It does this by measuring the working pressure and ensuring the collective pumps work together and deliver proportionately.Elektronikon ® monitoring systemElektronikon ® is a state-of-the-art monitoring system for your vacuum pumps. It is simple yet comprehensive and leads to energy savings. It can also integrate your plant management system thanks to a remote monitoring option.T echnical specifications - DZS mono-clawVacuumLow PressurePumping speed based on air at 20°C. T olerance +/-10%.60Hz Versions supplied with NPT adaptorsDimensional tolerance +/-10mm/0.4"Weight tolerance (oil included) +/- 2kg/4.4lbsDimensions & weightPumping speed based on air at 20°C. T olerance +/-10%.60Hz Versions supplied with NPT adaptorsLHWDZS 065 V – DZS 150 VDZS 150 PDZ S 300 VDZS 300 PDZ S 065 VDZS 150 P DZS 300 PDZ S 150 VDZPerformance80160180120140801001803001002001003504006060801006016025040204040501402002020120150Pumpingspeed(m³/h)Pumpingspeed(m³/h)Pumpingspeed(m³/h)Pumpingspeed(cfm)Pumpingspeed(m³/h)Pumpingspeed(cfm) Pressure bar(g)Pressure bar(g)Pressure hPa (mbar)Pressure hPa (mbar)100100100200200200300300300400400400500500500600600600700700700800800800900900900100010001000Pumpingspeed(m³/h)Pumpingspeed(cfm)50 Hz operation30 Hz operation20 Hz operation60 Hz operation40 Hz operationVacuumLow PressureT echnical specifications DZM multi-clawFigures based on 50Hz operation, 400 V.Figures based on lead pump with 20 to 60Hz operation, subsequence pumps operating at 50Hz, 400 V.Pumping speed based on air at 20°C. T olerance +/-10%.Shaft power based on one pump at minimum speed 1200rpm and maximum power achieved when lead pump is at 60Hz operation and lag pumps at 50Hz.*US 60Hz Versions supplied with ANSI adaptor flangesDimensions & weightDimensional tolerances +/- 10mm / 0.4"Weight tolerances (oil included) +/- 20kg/44lbsWLHCOMMITTED TO SUSTAINABLE PRODUCTIVITY6996 0061 01 © 2017, A t l a s C o p c o , B e l g i u m . A l l r i g h t s r e s e r v e d . D e s i g n s a n d s p e c i fi c a t i We stand by our responsibilities towards our customers, towards the environment and the people around us. We make performance stand the test of time. T his is what we call – Sustainable Productivity./vacuum。
华硕 ROG Strix GL503VS 游戏记录器说明手册说明书

& Trace Gas Analyzer SystemsValco Instruments Co. Inc.Turnkey AnalyzerStand-Alone OperationTools forScienceMedicineVICI Trace Gas Analyzer Systems (TGAS) are fully configured and tested gas chromatographs designed for use in high purity and ultra high purity analysis. Each instrument is fully configured and tested per user requirements. A full documentation package delivered with each instrument includes a method validation report, capability data, bill of materials, and method parameters.Configurations for most bulk, specialty, and electronics gases are available. Standard configurations include He,H 2, N 2, Ar, O 2, CO, CO 2, CH 4, C 2H 4, C 3H 6, CF 4, C 2F 6, C 3F 8, NF 3,HBr, AsH 3, PH 3, SiF 4, and SiH 4.The TGAS can be set up to run automatically, or user-configured for single run analysis. This makes the TGAS an ideal option for benchtop applications in the lab or for continuous duty in a process. With the optional sampling system, the instrument can do batch or individual analysis from a fill manifold or trailer fill stanchion.Secure TelemetryThe VICI TGAS is a completestand-alone solution for autonomous chromatographic analysis, from sample prep to final report. Everything is included in the TGAS housing,from the computer with all the necessary software and hardware to the touch-enabled wide screen display.A wireless mouse and keyboard are optional.The TGAS can be configured to select the sample and associated method, introduce the sample, run the analysis,store the data, integrate the chromatogram, and calculate the results.Turnkey analyzer for lab or process Fully stand-alone operation Secure telemetry for remote view/controlHighly configurable modular design MDQs for most analytes < 1 ppb Results data can be printed via a network printer or to a local user-provided printer. The same results can be output to an analog signal for DCS and other control schemes, or to the OPC server for database or spreadsheet updates.Functionality for copper-based LAN connection and secured WIFI make the instrument available and data accessible.While the TGAS is a fully functional standalone GC, there are those times when a brief check is all that is needed.Why put on your PPE and walk out into the plant or waltz across the lab to see if the analysis of that batch of samples has finished? Just point your PC browser to the TGAS secured web service, provide the proper user name and password, and access a fully configurable interface to the TGAS. There s not even any software to install!The system can be configured for various levels of access: View Only User has access to integration and reports. Select/Start Analysis User can load a sample and initiate a run, a batch of runs, or a sequence.Calibration Update User can update or modify calibrations.Method Change User can manipulate the method,valve timing, flows, integration parameters, and temp-erature programs.With appropriate IT approval/assistance, the TGAS can be accessed through a secure connection from the internet,allowing a technician to provide needed assistance without a road trip for a service call. A real time and money saver!tel:800 367-8424fax:713 688-8106**************Valco Instruments Co. Inc.VICI AG InternationalNorth America, South America, and Australia/Oceania call:Europe, Asia, and Africa call:tel:Int + 41 41 925-6200fax:Int + 41 41 925-6201************10/15VICI ® is a registered trademark of Valco Instruments Co. Instruments and VICI AGTrace impurities in oxygenArgon 3 ppmNitrogen 2 ppmMethane 4 ppmBulk argonTrace impurities in argonBulk argonN i t r o g e nMethaneCarbon monoxideTrace impurities in nitrogenArgonHydrogenBulk nitrogenBulk nitrogen M e t h a n eCarbon monoxideModular DesignMDQs < 1 ppbUnparalleled VICI ExpertiseThe design of the TGAS allows a very wide range of applications to be run on a single instrument. The TGAS can be configured with a single detector or as many as four,depending on the application. The PDHID can be run in three modes Helium Ionization, Photo Ionization, or Electon Capture. In 2016 a Micro TCD will be added,extending the range capability for applications from < 1 ppb to > 99%.In addition to the wide dynamic range and low level sensitivity, the TGAS can be configured for redundancy so that there is always a hot backup for any two channel method. Or, two channels can be configured for one or more gas method(s), with the other two channels available for an entirely different method or gas type. There are lots of possibilities with the modular design.If higher throughput or the need to clear heavy compounds or contamination from a sample is required, there are modular Fast Temperature Programmed ovens which can drastically reduce analysis time. As an added benefit, the FTP ovens improve peak shape and height for an even greater reduction in detection limits.Depending on the analysis, the sample preparation required can be configured as an easy-to-access module, simplying service and replacement if there is ever a need. The modular design also allows a path for upgrades that are much easier to accomplish than reconfiguring an entire instrument.The standard modules are:Detectors : PHID, PDPID, PDECD, and MicroTCD (in 2016). Oven/Temperature Zones : Support for 12 programmable thermal zones and up to four Fast Temperature Pro-grammed (FTP) zones. FTP zones can be micropacked columns, metal open tubular columns, capillary columns,programmable rate injectors, vaporizers, retention gap,or absorbers/concentrators.Valve Controls : Support for up to 16 two position and four multiposition air actuated valves and 4 two position electrically actuated valves.While the TGAS embodies the latest improvements in the VICI Trace Gas Analyzer product line, we have have been a standard for analysis in the pure gas industry for more than 35 years. We continue to be the primary manufacturer of every major component in our systems, from valves and detectors to electrometers and Fast Temperature Programmers. We know that specifications and require-ments in high purity gas supply are getting tougher, and are continuously working to improve the core products which have kept the TGA in demand over the decades.For more information, please provide a detailed list of the requirements for your application. We will work diligently to prepare a quote in a timely fashion.Currently our conservative guarantee for method detection with a reasonable RSD is 10 ppb for atmospheric com-ponents, day-in and day-out. But some of customers find that once the analyzer is installed and running continuously in ultra high purity applications, TGAs are able to routinely integrate and quantify at levels of less than 1 ppb.。
- 1、下载文档前请自行甄别文档内容的完整性,平台不提供额外的编辑、内容补充、找答案等附加服务。
- 2、"仅部分预览"的文档,不可在线预览部分如存在完整性等问题,可反馈申请退款(可完整预览的文档不适用该条件!)。
- 3、如文档侵犯您的权益,请联系客服反馈,我们会尽快为您处理(人工客服工作时间:9:00-18:30)。
Journal of Mechanical Science and Technology 23 (2009) 1331~1341 www.springerlink.com/content/1738-494xDOI 10.1007/s12206-009-0407-3
Journal of Mechanical
Science and Technology
Feature-based non-manifold modeling system to integrate design and analysis of injection molding products†
Sang Hun Lee* School of Mechanical and Automotive Engineering, Kookmin University, Seoul, 136-702, Korea
(Manuscript Received December 30, 2008; Revised January 24, 2009; Accepted January 24, 2009) -------------------------------------------------------------------------------------------------------------------------------------------------------------------------------------------------------------------------------------------------------- Abstract
Current CAE systems used for both the simulation of the injection molding process and the structural analysis of plastic parts accept solid models as geometric input. However, abstract models composed of sheets and wireframes are still used by CAE systems to carry out more analyses more efficiently. Therefore, to obtain an adequate abstract model, designers often have to simplify and idealize a detailed model of a part to a specific level of detail and/or abstraction. For such a process, we developed a feature-based design system based on a non-manifold modeling kernel supporting feature-based multi-resolution and multi-abstraction modeling capabilities. In this system, the geometric models for the CAD and CAE systems are merged into a single master model in a non-manifold topological representation, and then, for a given level of detail and abstraction, a simplified solid or non-manifold model is extracted immediately for an analysis. For a design change, the design and analysis models are modified simultaneously. As a result, this feature-based design system is able to provide a more integrated environment for the design and analysis of plastic injection molding parts.
Keywords: Injection molding product; Integration of CAD and CAE; Multi-resolution; Level of detail; Level of abstraction; Feature; Solid; Non-manifold
--------------------------------------------------------------------------------------------------------------------------------------------------------------------------------------------------------------------------------------------------------
1. Introduction In the area of design and manufacturing of plastic injection molding parts, various trials have been car-ried out to develop a specialized CAD system that could be used to design of plastic parts and molds [1, 2, 3] and to develop a CAE system that could be used to simulate the injection molding process to find defects before the manufacturing stage [4-6]. The complete design process supported by the CAD and CAE sys-tems is illustrated in Fig. 1. At the initial design stage, the CAD system dedicated to the design of the plastic part can be used to enhance design productivity. The structural analysis and the molding process simulation using the CAE system can validate the initial design. If
the simulation results do not satisfy the functional requirements, the design process is repeated by feed-ing back the simulation results to the CAD system. The design-analysis/simulation cycle is iterated until the functional requirements are satisfied. Traditionally, the geometric models used in the design stage are solid models including the feature informa-tion, whereas the models used in the analysis stage are abstract non-manifold topological (NMT) models composed of medial surfaces and axes, as illustrated in Fig. 2. The abstract NMT models are created in the CAE system using its pre-processor. Therefore, the designer must create and maintain two types of a geometric model of a part at the same time. Moreover, the process to convert a solid model into an abstract NMT model is very tedious and time-consuming. †This paper was recommended for publication in revised form by
Associate Editor Tae Hee Lee *Corresponding author. Tel.: +82 2 910 4835, Fax.: +82 2 910 4839
E-mail address: shlee@kookmin.ac.kr © KSME & Springer 2009 1332 S. H. Lee / Journal of Mechanical Science and Technology 23 (2009) 1331~1341
Fig. 1. Design and manufacturing process using traditional CAD and CAE systems for injection molding parts.
To solve this problem of having to create and main-tain two types of models, two different approaches have been proposed recently. One is to develop a new simulation package that can accept solid models as geometric input, and the other is to develop a com-puter program that can automatically transform a solid model into an abstract model. In the solid-based simulation package, although the effort for transfor-mation of a solid into an abstract model is eliminated, the computing time for the simulation increases dra-matically. To shorten the time, it is still necessary to remove the insignificant features from a part model. In the automated transformation approach such as the medial axis transformation (MAT), the results ob-tained by the automated transformation programs frequently require further modifications to meet the idealization rules for simulation. To reduce the com-puting time of the automated transformation approach, the detailed features need to be removed before the transformation process is launched. Although the solid-based simulation method has recently become popular, the traditional medial-surface-based simula-tion method is still useful because this method can provide simulation results more rapidly without sig-nificant loss of accuracy. Therefore, if the idealized models for both approaches can be readily provided at various levels of detail and/or abstraction from the design model, the cycle time for the design-analysis iteration can be reduced.