Tracking
ADTracking功能指标说明
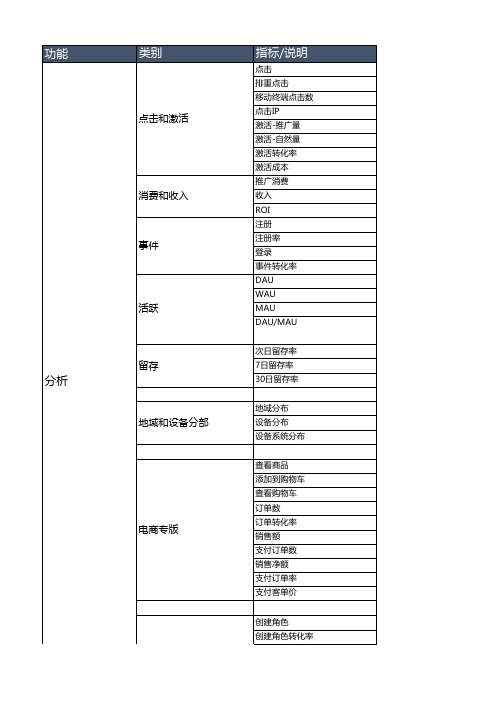
事件数求和/设备排重求和 事件数求和/设备排重求和 事件数求和/设备排重求和 事件数求和/设备排重求和 订单设备数/活跃设备数 订单金额统计 事件数求和/设备排重求和 事件数求和/设备排重求和 支付订单数/订单数 销售净额/支付订单数 创建角色设备数求和 创建角色设备数/活活跃设备数 支付成功的货币金额统计 游戏收入/活跃设备数
计算方式
点击数求和 排重点击数求和(依次按照广告平台业务ID,设备ID,cookieid) 移动点击判断条件,设备ID,UA中的devicetype,osversion ; 点击IP数求和 激活数求和 自然激活数求和 激活数/排重点击数; 成本花费/激活数(不包括推广期以外的激活以及自然激活) 用户输入的成本或通过设定的推广时间和结算方式方式来动态计算 应用内的付费求和 收入/成本花费。(收入数据不包括自然激活收入) 达成设备数求和 达成设备数求和 达成设备数求和 事件设备数/活跃数 活跃设备求和,TDID排重 过去7天活跃设备求和,TDID排重 过去30天活跃设备求和,TDID排重 当日活跃设备TDID和过去30日活跃TDID重复数/MAU 某日新增TDID/次日活跃TDID 某日新增TDID/7日活跃TDID 某日新增TDID/30日活跃TDID
游戏内付费成功的设备数 活跃设备中,付费的设备数占比; 游戏内付费金额的统计 活跃玩家的平均付费金额
录,付费,以及用户自定义的事件导出
录,付费,以及用户自定义的事件的详单数据的S2S传输
设定异常IP段阀值上限,达到上限值后对应的点击或者激活将会被标记为异常
的时间差,满足设定的时间差后,对应的激活将会被标记为异常
点击到激活的异常时差 Deeplink投放
通过设定点击和激活的时间差,满足设定的时间差后,对应的激活
各船司货物跟踪查询网址
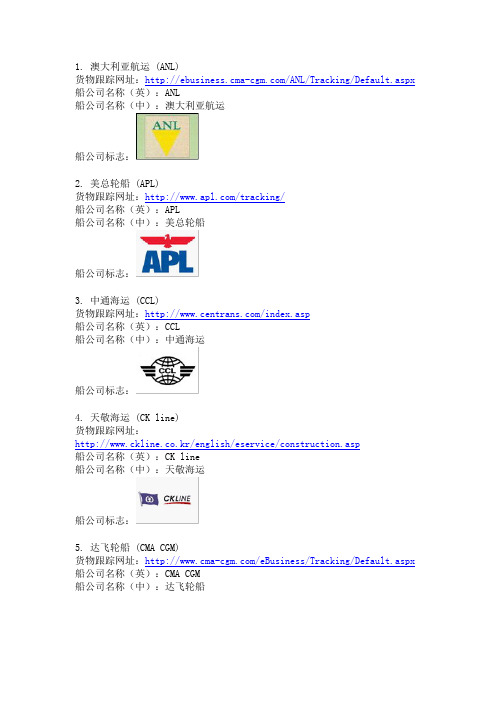
1. 澳大利亚航运 (ANL)货物跟踪网址:/ANL/Tracking/Default.aspx 船公司名称(英):ANL船公司名称(中):澳大利亚航运船公司标志:2. 美总轮船 (APL)货物跟踪网址:/tracking/船公司名称(英):APL船公司名称(中):美总轮船船公司标志:3. 中通海运 (CCL)货物跟踪网址:/index.asp船公司名称(英):CCL船公司名称(中):中通海运船公司标志:4. 天敬海运 (CK line)货物跟踪网址:http://www.ckline.co.kr/english/eservice/construction.asp船公司名称(英):CK line船公司名称(中):天敬海运船公司标志:5. 达飞轮船 (CMA CGM)货物跟踪网址:/eBusiness/Tracking/Default.aspx 船公司名称(英):CMA CGM船公司名称(中):达飞轮船船公司标志:6. 正利航业 (CNC)货物跟踪网址:船公司名称(英):CNC船公司名称(中):正利航业船公司标志:7. 中远集运 (COSCON)货物跟踪网址:/ebusiness/service/cargoTracking.do?action=init 船公司名称(英):COSCON船公司名称(中):中远集运船公司标志:8. 北欧亚 (CSAV NORASIA)货物跟踪网址:/index_en.htm船公司名称(英):CSAV NORASIA船公司名称(中):北欧亚船公司标志:9. 中海集运 (CSCL)货物跟踪网址:http://222.66.158.204/cargo_track/cargo_track.jsp船公司名称(英):CSCL船公司名称(中):中海集运船公司标志:10. 达贸航运 (Delmas)货物跟踪网址:船公司名称(英):Delmas船公司名称(中):达贸航运船公司标志:11. 长荣海运 (EMC,Evergreen)货物跟踪网址:/船公司名称(英):EMC,Evergreen船公司名称(中):长荣海运船公司标志:12. 阿联酋航运 (ESL,Emirates)货物跟踪网址:/船公司名称(英):ESL,Emirates船公司名称(中):阿联酋航运船公司标志:13. 远东船务 (FESCO)货物跟踪网址:http://www.fesco.ru/en/tracking/船公司名称(英):FESCO船公司名称(中):远东船务船公司标志:14. 金星轮船 (Gold Star)货物跟踪网址:/cargo_tracing.html 船公司名称(英):Gold Star船公司名称(中):金星轮船船公司标志:15. 大新华 (Grand)货物跟踪网址:/船公司名称(英):Grand船公司名称(中):大新华船公司标志:16. 海文船务 (HaiWin)货物跟踪网址:/cn/sailing.aspx船公司名称(英):HaiWin船公司名称(中):海文船务船公司标志:17. 汉堡南美 (Hamburg Sud)货物跟踪网址:/WWW/EN/E-Business/Track_and_Trace/index.jsp 船公司名称(英):Hamburg Sud船公司名称(中):汉堡南美船公司标志:18. 海华轮船 (HASCO)货物跟踪网址:/船公司名称(英):HASCO船公司名称(中):海华轮船船公司标志:19. 兴亚海运 (Heung-A)货物跟踪网址:http://www.heung-a.co.kr/chinese/cargo_web/cargotrace.cfm船公司名称(英):Heung-A船公司名称(中):兴亚海运船公司标志:20. 韩进海运 (HJ,HanJin)货物跟踪网址:/eservice/alps/cn/cargo/Cargo.do 船公司名称(英):HJ,HanJin船公司名称(中):韩进海运船公司标志:21. 赫伯罗特 (H-L,Hapag-Lloyd)货物跟踪网址:/en/home.html船公司名称(英):H-L,Hapag-Lloyd船公司名称(中):赫伯罗特船公司标志:22. 现代商船 (HMM,Hyundai)货物跟踪网址:/cms/business/china/trackTrace/trackTrace/index.j sp船公司名称(英):HMM,Hyundai船公司名称(中):现代商船船公司标志:23. 伊朗国航 (IRISL,IRIS Lines)货物跟踪网址:/CLS/CargoTracking/CargoTracking/FindContain er.aspx船公司名称(英):IRISL,IRIS Lines船公司名称(中):伊朗国航船公司标志:24. 神原汽船 (Kambara Kisen)货物跟踪网址:http://www.kambara-kisen.co.jp/mychart/cargotrack/search.php 船公司名称(英):Kambara Kisen船公司名称(中):神原汽船船公司标志:25. 川崎汽船 (K-L,KLine)货物跟踪网址:http://206.103.2.35/GctApp/search船公司名称(英):K-L,KLine船公司名称(中):川崎汽船船公司标志:26. 高丽海运 (KMTC)货物跟踪网址:http://www.kmtc.co.kr/船公司名称(英):KMTC船公司名称(中):高丽海运船公司标志:27. 协和海运 (KYOWA)货物跟踪网址:http://www.kyowa-line.co.jp/en/index.html船公司名称(英):KYOWA船公司名称(中):协和海运船公司标志:28. 马士基航运 (Maersk)货物跟踪网址:/appmanager/船公司名称(英):Maersk船公司名称(中):马士基航运船公司标志:29. 玛丽亚娜 (MARIANA)货物跟踪网址:船公司名称(英):MARIANA船公司名称(中):玛丽亚娜船公司标志:30. 南美邮船 (MARUBA)货物跟踪网址:.ar/main.asp?region 船公司名称(英):MARUBA船公司名称(中):南美邮船船公司标志:31. 美森轮船 (Matson)货物跟踪网址:船公司名称(英):Matson船公司名称(中):美森轮船船公司标志:32. 马士基所属近洋航线 (MCC)货物跟踪网址:.sg/船公司名称(英):MCC船公司名称(中):马士基所属近洋航线船公司标志:33. 民生轮船 (Minsheng)货物跟踪网址:/船公司名称(英):Minsheng船公司名称(中):民生轮船船公司标志:34. 马来西亚航运 (MISC)货物跟踪网址:.my/船公司名称(英):MISC船公司名称(中):马来西亚航运船公司标志:35. 商船三井 (MOL)货物跟踪网址:http://www.mol.co.jp/船公司名称(英):MOL船公司名称(中):商船三井船公司标志:36. 地中海航运 (MSC)货物跟踪网址:http://www.mscgva.ch/tracking/index.html船公司名称(英):MSC船公司名称(中):地中海航运船公司标志:37. 南星海运 (NAMSUNG)货物跟踪网址:http://www.namsung.co.kr/eng/eservice/sw_search.ns.tsp 船公司名称(英):NAMSUNG船公司名称(中):南星海运船公司标志:38. 尼罗河航运 (NDS,NileDutch)货物跟踪网址:/schedules.php船公司名称(英):NDS,NileDutch船公司名称(中):尼罗河航运船公司标志:39. 日本邮船 (NYK)货物跟踪网址:船公司名称(英):NYK船公司名称(中):日本邮船船公司标志:40. 新安通 (ONTO)货物跟踪网址:/newEbiz1/EbizPortalFG/portal/html/index.html 船公司名称(英):ONTO船公司名称(中):新安通船公司标志:41. 东方海外 (OOCL)货物跟踪网址:/schi/ourservices/eservices/trackandtrace/船公司名称(英):OOCL船公司名称(中):东方海外船公司标志:42. 奥林汽船 (Orient)货物跟踪网址:http://www.orientferry.co.jp/cn.html船公司名称(英):Orient船公司名称(中):奥林汽船船公司标志:43. 太平船务 (PIL)货物跟踪网址:船公司名称(英):PIL船公司名称(中):太平船务船公司标志:44. 宏海箱运 (RCL)货物跟踪网址:/船公司名称(英):RCL船公司名称(中):宏海箱运船公司标志:45. 南非海运 (SAF,Safmarine)货物跟踪网址:/wps/portal/Safmarine/Home?WCM_GLOBAL_CONT EXT=/wps/wcm/connect/Safmarine-Chinese/Safmarine/Home船公司名称(英):SAF,Safmarine船公司名称(中):南非海运船公司标志:46. 萨姆达拉 (Samudera)货物跟踪网址:/船公司名称(英):Samudera船公司名称(中):萨姆达拉船公司标志:47. 印度国航 (SCI)货物跟踪网址:/newsite/default.asp 船公司名称(英):SCI船公司名称(中):印度国航船公司标志:48. 德胜航运 (Senator)货物跟踪网址:/Senator.html船公司名称(英):Senator船公司名称(中):德胜航运船公司标志:49. 长锦商船 (Sinokor)货物跟踪网址:http://www.sinokor.co.kr/船公司名称(英):Sinokor船公司名称(中):长锦商船船公司标志:50. 中外运 (Sinotrans)货物跟踪网址:船公司名称(英):Sinotrans船公司名称(中):中外运船公司标志:51. 新海丰 (SITC)货物跟踪网址:/hangyun/searchcargo.asp 船公司名称(英):SITC船公司名称(中):新海丰船公司标志:52. 速达海运 (Speeda)货物跟踪网址:.sg/船公司名称(英):Speeda船公司名称(中):速达海运船公司标志:53. 泛洋轮船 (STX,STX-Pan Ocean)货物跟踪网址:/HP2401/track.do船公司名称(英):STX,STX-Pan Ocean船公司名称(中):泛洋轮船船公司标志:54. 德翔航运 (TSL,TS Lines)货物跟踪网址:/船公司名称(英):TSL,TS Lines船公司名称(中):德翔航运船公司标志:55. 阿拉伯航运 (UASC)货物跟踪网址:/aspx/OnlineShipping.aspx船公司名称(英):UASC船公司名称(中):阿拉伯航运船公司标志:56. 澳门永发 (WFL)货物跟踪网址:/company/Company_Browse.aspx?id=1船公司名称(英):WFL船公司名称(中):澳门永发船公司标志:57. 万海航运 (WHL,WanHai)货物跟踪网址:/index_whl.jsp?file_num=15040&web=whlwww&i_url=w hlwww/cargoTrack.jsp船公司名称(英):WHL,WanHai船公司名称(中):万海航运船公司标志:58. 威兰德船务 (Winland)货物跟踪网址:船公司名称(英):Winland船公司名称(中):威兰德船务船公司标志:59. 阳明海运 (YML,Yangming)货物跟踪网址:/simplified_version/track_trace/track_trace_carg o_tracking.asp船公司名称(英):YML,Yangming船公司名称(中):阳明海运船公司标志:60. 以星航运 (ZIM)货物跟踪网址:http://www.zim.co.il/Tracing.aspx?id=166&l=4船公司名称(英):ZIM船公司名称(中):以星航运船公司标志:61. CSAV (南美轮船)货物跟踪网址:/index_en.htm船公司名称(英):南美轮船船公司名称(中):CSAV。
tracking
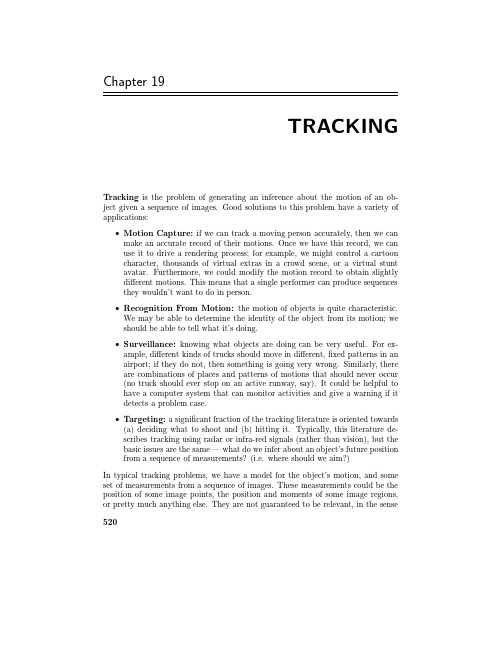
Chapter19TRACKING Tracking is the problem of generating an inference about the motion of an ob-ject given a sequence of images.Good solutions to this problem have a variety ofapplications:•Motion Capture:if we can track a moving person accurately,then we can make an accurate record of their motions.Once we have this record,we can use it to drive a rendering process;for example,we might control a cartoon character,thousands of virtual extras in a crowd scene,or a virtual stunt avatar.Furthermore,we could modify the motion record to obtain slightly different motions.This means that a single performer can produce sequences they wouldn’t want to do in person.•Recognition From Motion:the motion of objects is quite characteristic.We may be able to determine the identity of the object from its motion;we should be able to tell what it’s doing.•Surveillance:knowing what objects are doing can be very useful.For ex-ample,different kinds of trucks should move in different,fixed patterns in an airport;if they do not,then something is going very wrong.Similarly,there are combinations of places and patterns of motions that should never occur (no truck should ever stop on an active runway,say).It could be helpful to have a computer system that can monitor activities and give a warning if it detects a problem case.•Targeting:a significant fraction of the tracking literature is oriented towards(a)deciding what to shoot and(b)hitting it.Typically,this literature de-scribes tracking using radar or infra-red signals(rather than vision),but the basic issues are the same—what do we infer about an object’s future position from a sequence of measurements?(i.e.where should we aim?)In typical tracking problems,we have a model for the object’s motion,and some set of measurements from a sequence of images.These measurements could be the position of some image points,the position and moments of some image regions, or pretty much anything else.They are not guaranteed to be relevant,in the sense 520Section19.1.Tracking as an Abstract Inference Problem521 that some could come from the object of interest and some might come from other objects,or from noise.19.1Tracking as an Abstract Inference ProblemMuch of this chapter will deal with the algorithmics of tracking.In particular,we will see tracking as a probabilistic inference problem.The key technical difficultyis maintaining an accurate representation of the posterior on object position given measurements,and doing so efficiently.We model the object as having some internal state;the state of the object at the i’th frame is typically written as X i.The capital letters indicate that this is a random variable—when we want to talk about a particular value that this variable takes,we will use small letters.The measurements obtained in the i’th frame are values of a random variable Y i;we shall write y i for the value of a measurement, and,on occasion,we shall write Y i=y i for emphasis.There are three main problems:•Prediction:we have seen y0,...,y i−1—what state does this set of mea-surements predict for the i’th frame?to solve this problem,we need to obtaina representation of P(X i|Y0=y0,...,Y i−1=y i−1).•Data association:Some of the measurements obtained from the i-th framemay tell us about the object’s state.Typically,we use P(X i|Y0=y0,...,Y i−1= y i−1)to identify these measurements.•Correction:now that we have y i—the relevant measurements—we needto compute a representation of P(X i|Y0=y0,...,Y i=y i).19.1.1Independence AssumptionsTracking is very difficult without the following assumptions:•Only the immediate past matters:formally,we requireP(X i|X1,...,X i−1)=P(X i|X i−1)This assumption hugely simplifies the design of algorithms,as we shall see;furthermore,it isn’t terribly restrictive if we’re clever about interpreting X ias we shall show in the next section.•Measurements depend only on the current state:we assume that Y iis conditionally independent of all other measurements given X i.This meansthatP(Y i,Y j,...Y k|X i)=P(Y i|X i)P(Y j,...,Y k|X i) Again,this isn’t a particularly restrictive or controversial assumption,but ityields important simplifications.522Tracking Chapter19 These assumptions mean that a tracking problem has the structure of inference on a hidden Markov model(where both state and measurements may be on a continuous domain).You should compare this chapter with section??,which the use of hidden Markov models in recognition.19.1.2Tracking as InferenceWe shall proceed inductively.Firstly,we assume that we have P(X0),which is our “prediction”in the absence of any evidence.Now correcting this is easy:when we obtain the value of Y0—which is y0—we have thatP(X0|Y0=y0)=P(y0|X0)P(X0)P(y0)=P(y0|X0)P(X0)P(y0|X0)P(X0)d X0∝P(y0|X0)P(X0)All this is just Bayes rule,and we either compute or ignore the constant of pro-portionality depending on what we need.Now assume we have a representation of P(X i−1|y0,...,y i−1).PredictionPrediction involves representingP(X i|y0,...,y i−1)Our independence assumptions make it possible to writeP(X i|y0,...,y i−1)=P(X i,X i−1|y0,...,y i−1)d X i−1=P(X i|X i−1,y0,...,y i−1)P(X i−1|y0,...,y i−1)d X i−1=P(X i|X i−1)P(X i−1|y0,...,y i−1)d X i−1CorrectionCorrection involves obtaining a representation ofP(X i|y0,...,y i)Our independence assumptions make it possible to writeP(X i|y0,...,y i)=P(X i,y0,...,y i) P(y0,...,y i)Section 19.2.Linear Dynamic Models and the Kalman Filter 523=P (y i |X i ,y 0,...,y i −1)P (X i |y 0,...,y i −1)P (y 0,...,y i −1)P (y 0,...,y i )=P (y i |X i )P (X i |y 0,...,y i −1)P (y 0,...,y i −1)P (y 0,...,y i )=P (y i |X i )P (X i |y 0,...,y i −1) P (y i |X i )P (X i |y 0,...,y i −1)d X i19.1.3OverviewThe key algorithmic issue involves finding a representation of the relevant prob-ability densities that (a)is sufficiently accurate for our purposes and (b)allows these two crucial sums to be done quickly and easily.The simplest case occurs when the dynamics are linear,the measurement model is linear,and the noise models are Gaussian (section 19.2).Non-linearities introduce a host of unpleasant problems (section 19.3)and we discuss some current methods for handling them (section 19.4;the appendix gives another method that is unreliable but occasion-ally useful).We discuss data association in section 19.5,and show some examples of tracking systems in action in section 21.3.19.2Linear Dynamic Models and the Kalman FilterThere are good relations between linear transformations and Gaussian probability densities.The practical consequence is that,if we restrict attention to linear dy-namic models and linear measurement models,both with additive Gaussian noise,all the densities we are interested in will be Gaussians.Furthermore,the question of solving the various integrals we encounter can usually be avoided by tricks that allow us to determine directly which Gaussian we are dealing with.19.2.1Linear Dynamic ModelsIn the simplest possible dynamic model,the state is advanced by multiplying it by some known matrix (which may depend on the frame),and then adding a normal random variable of zero mean,and known covariance.Similarly,the measurement is obtained by multiplying the state by some matrix (which may depend on the frame),and then adding a normal random variable of zero mean and known covariance.We use the notationx ∼N (µ,Σ)to mean that x is the value of a random variable with a normal probability dis-tribution with mean µand covariance Σ;notice that this means that,if x is one-dimensional —we’d write x ∼N (µ,v )—that its standard deviation is √v .We can write our dynamic model asx i ∼N (D i x i −1;Σd i )524Tracking Chapter19y i∼N(M i x i;Σm i)Notice that the covariances could be different from frame to frame,as could the matrices.While this model appears very limited,it is in fact extremely powerful; we show how to model some common situations below.Drifting PointsLet us assume that x encodes the position of a point.If D i=Id,then the point is moving under random walk—its new position is its old position,plus some Gaussian noise term.This form of dynamics isn’t obviously useful,because it appears that we are tracking stationary objects.It is quite commonly used for objects for which no better dynamic model is known—we assume that the random component is quite large,and hope we can get away with it.This model also illustrates aspects of the measurement matrix M.The most important thing to keep in mind is that we don’t need to measure every aspect of the state of the point at every step.For example,assume that the point is in3D: now if M3k=(0,0,1),M3k+1=(0,1,0)and M3k+2=(1,0,0),then at every third frame we measure,respectively,the z,y,or x position of the point.Notice that we can still expect to be able to track the point,even though we measure only one component of its position at a given frame.If we have sufficient measurements,we can reconstruct the state—the state is observable.We explore observability in the exercises.Constant VelocityAssume that the vector p gives the position and v the velocity of a point moving with constant velocity.In this case,p i=p i−1+(∆t)v i−1and v i=v i−1.This means that we can stack the position and velocity into a single state vector,and our model applies.In particular,x=pvandD i=Id(∆t)Id0IdNotice that,again,we don’t have to observe the whole state vector to make a useful measurement.For example,in many cases we would expect thatM i=Id0i.e.that we see only the position of the point.Because we know that it’s moving with constant velocity—that’s the model—we expect that we could use these measurements to estimate the whole state vector rather well.Section19.2.Linear Dynamic Models and the Kalman Filter525the state space is two dimensional—one coordinate for position,one for velocity.The figure on the top left shows a plot of the state;each asterisk is a different state.Notice that the vertical axis(velocity)shows some small change,compared with the horizontal axis.This small change is generated only by the random component of the model,so that the velocity is constant up to a random change.Thefigure on the top right shows thefirst component of state(which is position)plotted against the time axis.Notice we have something that is moving with roughly constant velocity.Thefigure on the bottom overlays the measurements(the circles)on this plot.We are assuming that the measurements are of position only,and are quite poor;as we shall see,this doesn’t significantly affect our ability to track.Constant AccelerationAssume that the vector p gives the position,vector v the velocity and vector a the acceleration of a point moving with constant acceleration.In this case,p i= p i−1+(∆t)v i−1,v i=v i−1+(∆t)a i−1and a i=a i−1.Again,we can stack the position,velocity and acceleration into a single state vector,and our model applies.526Tracking Chapter19In particular,x=pvaandD i=Id(∆t)Id00Id(∆t)Id00IdNotice that,again,we don’t have to observe the whole state vector to make a useful measurement.For example,in many cases we would expect thatM i=Id00i.e.that we see only the position of the point.Because we know that it’s moving with constant acceleration—that’s the model—we expect that we could use thesethe line.On the left,we show a plot of thefirst two components of state—the position on the x-axis and the velocity on the y-axis.In this case,we expect the plot to look like (t2,t),which it does.On the right,we show a plot of the position against time—note that the point is moving away from its start position increasingly quickly.Periodic MotionAssume we have a point,moving on a line with a periodic movement.Typically,its position p satisfies a differential equation liked2pdt2=−pSection 19.2.Linear Dynamic Models and the Kalman Filter 527This can be turned into a first order linear differential equation by writing the velocity as v ,and stacking position and velocity into a vector u =(p,v );we then have d u dt= 01−10 u =S u Now assume we are integrating this equation with a forward Euler method,where the steplength is ∆t ;we haveu i =u i −1+∆t d udt=u i −1+∆t S u i −1= 1∆t −∆t 1u i −1We can either use this as a state equation,or we can use a different integrator.If we used a different integrator,we might have some expression in u i −1,...,u i −n —we would need to stack u i −1,...,u i −n into a state vector and arrange the matrix appropriately (see the exercises).This method works for points on the plane,in 3D,etc.as well (again,see the exercises).Highe r Orde r Mode lsAnother way to look at a constant velocity model is that we have augmented the state vector to get around the requirement that P (x i |x 1,...,x i −1)=P (x i |x i −1).We could write a constant velocity model in terms of point position alone,as long as we were willing to use the position of the i −2’th point as well as that of the i −1’th point.In particular,writing position as p ,we would haveP (p i |p 1,...,p i −1)=N (p i −1+(p i −1−p i −2),Σd i )This model assumes that the difference between p i and p i −1is the same as the difference between p i −1and p i −2—i.e.that the velocity is constant,up to the random element.A similar remark applies to the constant acceleration model,which is now in terms of p i −1,p i −2and p i −3.We augmented the position vector with the velocity vector (which represents p i −1−p i −2)to get the state vector for a constant velocity model;similarly,we augmented the position vector with the velocity vector and the acceleration vector (which represents (p i −1−p i −2)−(p i −2−p i −3))to get a constant acceleration model.We might reasonably want the new position of the point to depend on p i −4or other points even further back in the history of the point’s track;to represent dynamics like this,all we need to do is augment the state vector to a suitable size.Notice that it can be somewhat difficult to visualize how the model will behave.There are two approaches to determining what D i needs to be;in the first,we know something about the dynamics and can write it down,as we have done here;in the second,we need to learn it from data —we put discussion of this topic off.528Tracking Chapter1919.2.2Kalman FilteringAn important feature of the class of models we have described is that all the conditional probability models we need to deal with are normal.In particular,P(X i|y1,...,y i−1)is normal;as is P(X i|y1,...,y i).This means that they are relatively easy to represent—all we need to do is maintain representations of the mean and the covariance for the prediction and correction phase.In particular,our model will admit a relatively simple process where the representation of the mean and covariance for the prediction and estimation phase are updated.19.2.3The Kalman Filter for a1D State VectorThe dynamic model is nowx i∼N(d i x i−1,σ2di)y i∼N(m i x i,σ2m i)We need to maintain a representation of P(X i|y0,...,y i−1)and of P(X i|y0,...,y i).In each case,we need only represent the mean and the standard deviation,becausethe distributions are normal.NotationWe will represent the mean of P(X i|y0,...,y i−1)as X−i and the mean of P(X i|y0,...,y i) as X+i—the superscripts suggest that they represent our belief about X i immedi-ately before and immediately after the i’th measurement arrives.Similarly,we will represent the standard deviation of P(X i|y0,...,y i−1)asσ−i and of P(X i|y0,...,y i)asσ+i.In each case,we will assume that we know P(X i−1|y0,...,y i−1),meaningthat we know X+i−1andσ+i−1.Tricks with IntegralsThe main reason that we work with normal distributions is that their integrals are quite well behaved.We are going to obtain values for various parameters as integrals,usually by change of variable.Our current notation can make appropriate changes a bit difficult to spot,so we writeg(x;µ,v)=exp−(x−µ)22vWe have dropped the constant,and for convenience are representing the variance (as v),rather than the standard deviation.This expression allows some convenient transformations;in particular,we haveg(x;µ,v)=g(x−µ;0,v)Section19.2.Linear Dynamic Models and the Kalman Filter529g(m;n,v)=g(n;m,v)g(ax;µ,v)=g(x;µ/a,v/a2)We will also need the following fact:∞−∞g(x−u;µ,v a)g(u;0,v b)du∝g(x;µ,v2a+v2b)(there are several ways to confirm that this is true:the easiest is to look it up in tables;more subtle is to think about convolution directly;more subtle still is to think about the sum of two independent random variables).We need a further identity.We haveg(x;a,b)g(x;c,d)=g(x;ad+cbb+d,bdb+d)f(a,b,c,d)here the form of f is not significant,but the fact that it is not a function of x is. The exercises show you how to prove this identity.PredictionWe haveP(X i|y0,...,y i−1)=P(X i|X i−1)P(X i−1|y0,...,y i−1)dX i−1NowP(X i|y0,...,y i−1)=P(X i|X i−1)P(X i−1|y0,...,y i−1)dX i−1)∝ ∞−∞g(X i;d i X i−1,σ2di)g(X i−1;X+i−1,(σ+i−1)2)dX i−1∝ ∞−∞g((X i−d i X i−1);0,σ2d i)g((X i−1−X+i−1);0,(σ+i−1)2)dX i−1∝ ∞−∞g((X i−d i(u+X+i−1));0,(σd i)2)g(u;0,(σ+i−1)2)du∝ ∞−∞g((X i−d i u);d i X+i−1,σ2d i)g(u;0,(σ+i−1)2)du∝ ∞−∞g((X i−v);d i X+i−1,σ2d i)g(v;0,(d iσ+i−1)2)dv∝g(X i;d i X+0,σ2di +(d iσ+i−1)2)530Tracking Chapter19 where we have applied the transformations above,and changed variable twice.All this means thatX−i=d i X+i−1(σ−i)2=σ2di+(d iσ+i−1)2CorrectionWe haveP(X i|y0,...,y i)=P(y i|X i)P(X i|y0,...,y i−1)P(y i|X i)P(X i|y0,...,y i−1)dX i∝P(y i|X i)P(X i|y0,...,y i−1)We know X−i andσ−i ,which represent P(X i|y0,...,y i−1).Using the notation above,we haveP(X i|y0,...,y i)∝g(y i;m i X i,σ2m i)g(X i;X−i,(σ−i)2)=g(m i X i;y i,σ2mi )g(X i;X−i,(σ−i)2)=g(X i;y im i,σ2mim2i)g(X i;X−i,(σ−i)2)and by pattern matching to the identity above,we haveX+i=X−iσ2mi+m i y i(σ−i)2σ2mi+m2i(σ−i)2σ+i=σ2mi(σ−i)2(σ2mi+m2i(σ−i)2)19.2.4The Kalman Update Equations for a General State Vector We obtained a1D tracker without having to do any integration using special prop-erties of normal distributions.This approach works for a state vector of arbitrary dimension,but the process of guessing integrals,etc.,is a good deal more elabo-rate than that shown in section19.2.3.We omit the necessary orgy of notation —it’s a tough but straightforward exercise for those who really care(you should figure out the identitiesfirst and the rest follows)—and simply give the result in algorithm??.Section19.2.Linear Dynamic Models and the Kalman Filter531 Dynamic Model:x i∼N(d i x i−1,σd i)y i∼N(m i x i,σm i)Start Assumptions:x−0andσ−0are knownUpdate Equations:Predictionx−i =d i x+i−1σ−i=σ2d i+(d iσ+i−1)2Update Equations:Correctionx+ i =x−iσ2mi+m i y i(σ−i)2σ2mi+m2i(σ−i)2σ+i=σ2mi(σ−i)2(σ2mi+m2i(σ−i)2)Algorithm19.1:The1D Kalmanfilter updates estimates of the mean and co-variance of the various distributions encountered while tracking a one-dimensional state variable using the given dynamic model.19.2.5Forward-Backward SmoothingIt is important to notice that P(X i|y0,...,y i)is not the best available represen-tation of X i;this is because it doesn’t take into account the future behaviour of the point.In particular,all the measurements after y i could affect our represen-tation of X i.This is because these future measurements might contradict the estimates obtained to date—perhaps the future movements of the point are more in agreement with a slightly different estimate of the position of the point.However, P(X i|y0,...,y i)is the best estimate available at step i.What we do with this observation depends on the circumstances.If our appli-532Tracking Chapter19 Dynamic Model:x i∼N(D i x i−1,Σd i)y i∼N(M i x i,Σm i)Start Assumptions:x−0andΣ−0are knownUpdate Equations:Predictionx−i =D i x+i−1Σ−i =Σdi+D iσ+i−1D iUpdate Equations:CorrectionK i=Σ−i M T iM iΣ−i M T i+Σm i−1x+i=x−i+K iy i−M i x−iΣ+i=[Id−K i M i]Σ−iAlgorithm19.2:The Kalmanfilter updates estimates of the mean and covariance of the various distributions encountered while tracking a state variable of some fixed dimension using the given dynamic model.cation requires an immediate estimate of position—perhaps we are tracking a car in the opposite lane—there isn’t much we can do.If we are tracking off-line—perhaps,for forensic purposes,we need the best estimate of what an object was doing given a videotape—then we can use all data points,and so we want to rep-resent P(X i|y0,...,y N).A common alternative is that we need a rough estimate immediately,and can use an improved estimate that has been time-delayed by a number of steps.This means we want to represent P(X i|y0,...,y i+k)—we have to wait till time i+k for this representation,but it should be an improvement on P(X i|y0,...,y i).Section19.2.Linear Dynamic Models and the Kalman Filterconstant velocity(compare withfigure19.1).The state is plotted with open circles,asa function of the step i.The*-s give x−i ,which is plotted slightly to the left of thestate to indicate that the estimate is made before the measurement.The x-s give themeasurements,and the+-s give x+i ,which is plotted slightly to the right of the state.Thevertical bars around the*-s and the+-s are3standard deviation bars,using the estimate of variance obtained before and after the measurement,respectively.When the measurement is noisy,the bars don’t contract all that much when a measurement is obtained(compare withfigure19.4).Introducing a Backward FilterNow we haveP(X i|y0,...,y N)=P(X i,y i+1,...,y N|y0,...,y i)P(y0,...,y i)P(y0,...,y N)=P(y i+1,...,y N|X i,y0,...,y i)P(X i|y0,...,y i)P(y0,...,y i)P(y0,...,y N)=P(y i+1,...,y N|X i)P(X i|y0,...,y i)P(y0,...,y i)P(y0,...,y N)=P(X i|y i+1,...,y N)P(X i|y0,...,y i)P(y i+1,...,y N)P(y0,...,y i) P(X i)P(y0,...,y N)Tracking Chapter19 constant acceleration(compare withfigure19.2).The state is plotted with open circles,as a function of the step i.The*-s give x−i ,which is plotted slightly to the left of thestate to indicate that the estimate is made before the measurement.The x-s give themeasurements,and the+-s give x+i ,which is plotted slightly to the right of the state.Thevertical bars around the*-s and the+-s are3standard deviation bars,using the estimate of variance obtained before and after the measurement,respectively.When the measurement is noisy,the bars don’t contract all that much when a measurement is obtained.The fraction in brackets should look like a potential source of problems to you;in fact,we will be able to avoid tangling with it by a clever trick.What is impor-tant about this form is that we are combining P(X i|y0,...,y i)—which we know how to obtain—with P(X i|y i+1,...,y N).We actually know how to obtain arepresentation of P(X i|y i+1,...,y N),too.We could simply run the Kalmanfilter backwards in time,using backward dynamics,and take the predicted representation of X i(we leave the details of relabelling the sequence,etc.to the exercises).Combining RepresentationsNow we have two representations of X i:one obtained by running a forwardfilter, and incorporating all measurements up to y i;and one obtained by running a back-wardfilter,and incorporating all measurements after y i.We need to combine these representations.Instead of explicitly determining the missing terms in equation??, we can get the answer by noting that this is like having another measurement.InSection19.2.Linear Dynamic Models and the Kalman Filter535 particular,we have a new measurement generated by X i—that is,the result of the backwardfilter—to combine with our estimate from the forwardfilter.We know how to combine estimates with measurements,because that’s what the Kalman filter equations are for.All we need is a little notation.We will attach the superscript f to the estimate from the forwardfilter,and the superscript b to the estimate from the backward filter.We will write the mean of P(X i|y0,...,y N)as X∗i and the covariance of P(X i|y0,...,y N)asΣ∗i.We regard the representation of X b i as a measurementof X i with mean X b,−i and covarianceΣb,−i—the minus sign is because the i’thmeasurement cannot be used twice,meaning the backwardfilter predicts X i using y N...y i+1.This measurement needs to be combined with P(X i|y0,...,y i),whichhas mean X f,+i and covarianceΣf,+i(when we substitute into the Kalman equations,these will take the role of the representation before a measurement,because the valueof the measurement is now X b,−i ).Substituting into the Kalman equations,wefindK∗i=Σf,+iΣf,+i+Σb,−i−1Σ∗i=[I−K i]Σ+,fiX∗i=X f,+i +K∗iX b,−i−X f,+iIt turns out that a little manipulation(exercises!)yields a simpler form,which we give in algorithm3.Forward-backward estimates can make a substantial difference, asfigure19.5illustrates.PriorsIn typical vision applications,we are tracking forward in time.This leads to aninconvenient asymmetry:we may have a good idea of where the object started, but only a poor one of where it stopped,i.e.we are likely to have a fair prior for P(x0),but may have difficulty supplying a prior for P(x N)for the forward-backwardfilter.One option is to use P(x N|y0,...,y N)as a prior.This is a dubious act,as this probability distribution does not in fact reflect our prior beliefabout P(x N)—we’ve used all the measurements to obtain it.The consequences can be that this distribution understates our uncertainty in x N,and so leads to a forward-backward estimate that significantly underestimates the covariance for the later states.An alternative is to use a the mean supplied by the forwardfilter, but enlarge the covariance substantially;the consequences are a forward-backward estimate that overestimates the covariance for the later states(comparefigure19.5 withfigure19.6).Not all applications have this asymmetry;for example,if we are engaged in a forensic study of a videotape,we might be able to start both the forward tracker536Tracking Chapter19 Forwardfilter:Obtain the mean and variance of P(X i|y0,...,y i)using the Kalmanfilter.These are X f,+i andΣf,+i.Backwardfilter:Obtain the mean and variance of P(X i|y i+1,...,y N)usingthe Kalmanfilter running backwards in time.These are X b,−i andΣb,−i.Combining forward and backward estimates:Regard the backward esti-mate as a new measurement for X i,and insert into the Kalmanfilter equations to obtainΣ∗i=(Σf,+i)−1+(Σb,−i)−1−1X∗i=Σ∗i(Σf,+i)−1X f,+i+(Σb,−i)−1X b,−iAlgorithm19.3:The forward backward algorithm combines forward and backward estimates of state to come up with an improved estimate.and the backward tracker by hand,and provide a good estimate of the prior in each case.If this is possible,then we have a good deal more information which may be able to help choose correspondences,etc.—the forward tracker shouldfinish rather close to where the backward tracker starts.Smoothing over an IntervalWhile our formulation of forward-backward smoothing assumed that the backward filter started at the last data point,it is easy to start thisfilter afixed number of steps ahead of the forwardfilter.If we do this,we obtain an estimate of state in real time(essentially immediately after the measurement),and an improved estimate somefixed numbers of measurements later.This is sometimes useful.Furthermore, it is an efficient way to obtain most of the improvement available from a backward filter,if we can assume that the effect of the distant future on our estimate is relatively small compared with the effect of the immediate future.Notice that we need to be careful about priors for the backwardfilter here;we might take the forward estimate and enlarge its covariance somewhat.19.3Non-Linear Dynamic ModelsIf we can assume that noise is normally distributed,linear dynamic models are rea-sonably easy to deal with,because a linear map takes a random variable with a normal distribution to another random variable with a(different,but easily deter-。
aaa cooper tracking - aftership -回复
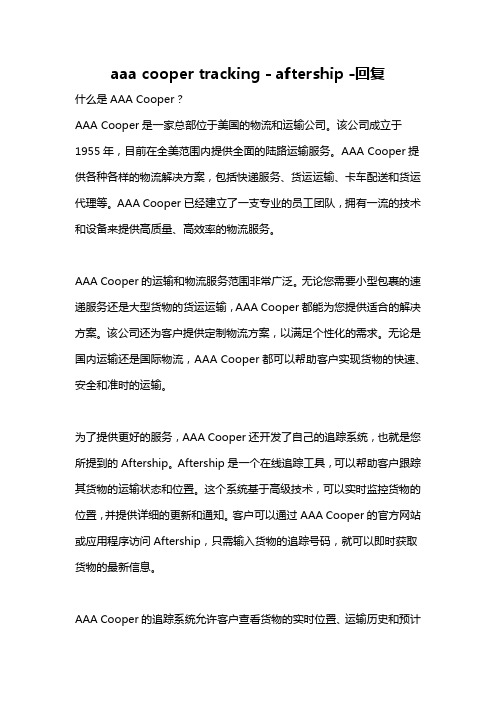
aaa cooper tracking - aftership -回复什么是AAA Cooper?AAA Cooper是一家总部位于美国的物流和运输公司。
该公司成立于1955年,目前在全美范围内提供全面的陆路运输服务。
AAA Cooper提供各种各样的物流解决方案,包括快递服务、货运运输、卡车配送和货运代理等。
AAA Cooper已经建立了一支专业的员工团队,拥有一流的技术和设备来提供高质量、高效率的物流服务。
AAA Cooper的运输和物流服务范围非常广泛。
无论您需要小型包裹的速递服务还是大型货物的货运运输,AAA Cooper都能为您提供适合的解决方案。
该公司还为客户提供定制物流方案,以满足个性化的需求。
无论是国内运输还是国际物流,AAA Cooper都可以帮助客户实现货物的快速、安全和准时的运输。
为了提供更好的服务,AAA Cooper还开发了自己的追踪系统,也就是您所提到的Aftership。
Aftership是一个在线追踪工具,可以帮助客户跟踪其货物的运输状态和位置。
这个系统基于高级技术,可以实时监控货物的位置,并提供详细的更新和通知。
客户可以通过AAA Cooper的官方网站或应用程序访问Aftership,只需输入货物的追踪号码,就可以即时获取货物的最新信息。
AAA Cooper的追踪系统允许客户查看货物的实时位置、运输历史和预计到达时间。
同时,系统还提供了自动通知功能,当货物发生任何重要变化时,客户会立即收到通知。
这种实时追踪和通知功能使AAA Cooper成为一家受欢迎的物流提供商,让客户能够全程跟踪他们的货物,并及时做出相应的计划和决策。
要使用AAA Cooper的追踪系统,客户只需访问AAA Cooper官方网站或下载他们的应用程序,并注册一个账户。
一旦账户注册完成,客户可以登录并输入货物的追踪号码。
随后,系统将显示货物的实时位置和其他详细信息。
客户还可以设置偏好,选择是否接收自动更新和通知。
track的用法总结大全
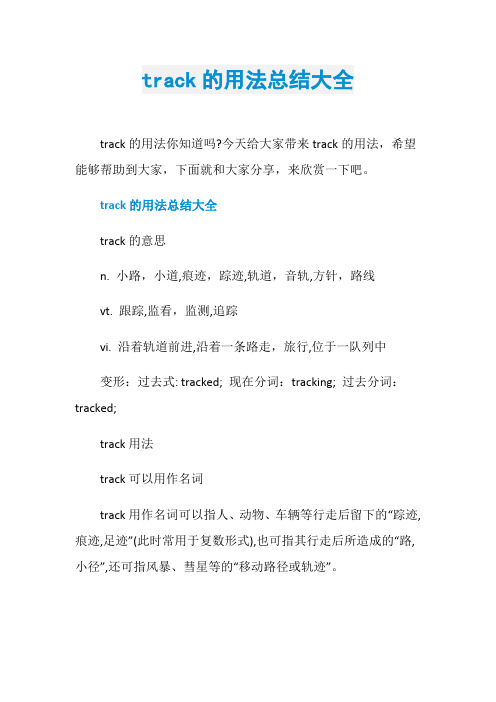
track的用法总结大全track的用法你知道吗?今天给大家带来track的用法,希望能够帮助到大家,下面就和大家分享,来欣赏一下吧。
track的用法总结大全track的意思n. 小路,小道,痕迹,踪迹,轨道,音轨,方针,路线vt. 跟踪,监看,监测,追踪vi. 沿着轨道前进,沿着一条路走,旅行,位于一队列中变形:过去式: tracked; 现在分词:tracking; 过去分词:tracked;track用法track可以用作名词track用作名词可以指人、动物、车辆等行走后留下的“踪迹,痕迹,足迹”(此时常用于复数形式),也可指其行走后所造成的“路,小径”,还可指风暴、彗星等的“移动路径或轨迹”。
track还可作“(火车的)轨道”“站台”“跑道”“轮带,履带”“唱片的一段录音”“(声)道”“滑道〔轨〕”等解,有时还可引申指“行为的方式”或“人生的常道”。
track作“跑道”解时,在句中常用作定语。
(hard) on sbs track〔on the track of〕的意思是“追踪〔寻找〕某人,寻找某物”,指“追踪〔寻找〕某人”时,这两种表达方式均可; 指“寻找某物”时,则一般只用on the track of sth 结构。
track用作名词的用法例句The tram is being switched on to another track .电车正被移至另外一条轨道上。
The cyclist went at full sail along the track.自行车手沿着跑道全速前进。
I quickly lose her track in the crowd.我在人群中失去了她的踪迹。
track可以用作动词track用作名词时意思是“足迹,痕迹”,转化成动词意思是“追踪,尾随”,指偷偷地跟在某人或某物的后边而不被发现,从而寻找某人或某物的所在地,强调跟踪这一过程而不强调跟踪的结果,常含有“搜索”的意味。
跟踪Tracking
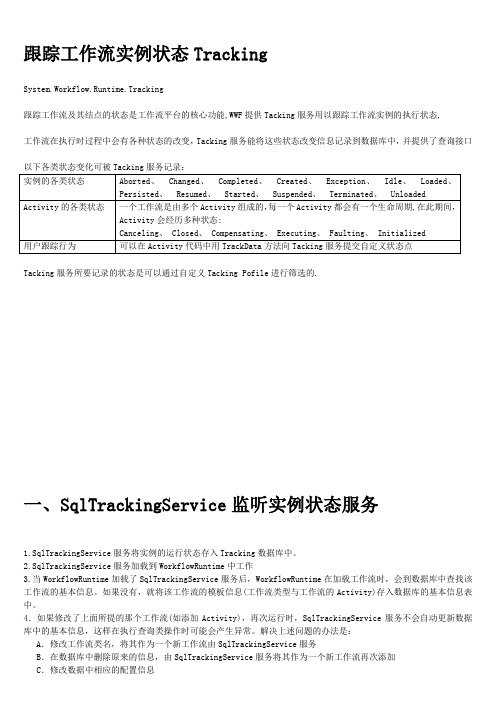
跟踪工作流实例状态TrackingSystem.Workflow.Runtime.Tracking跟踪工作流及其结点的状态是工作流平台的核心功能,WWF提供Tacking服务用以跟踪工作流实例的执行状态,工作流在执行时过程中会有各种状态的改变,Tacking服务能将这些状态改变信息记录到数据库中,并提供了查询接口Tacking服务所要记录的状态是可以通过自定义Tacking Pofile进行筛选的.一、SqlTrackingService监听实例状态服务1.SqlTrackingService服务将实例的运行状态存入Tracking数据库中。
2.SqlTrackingService服务加载到WorkflowRuntime中工作3.当WorkflowRuntime加载了SqlTrackingService服务后,WorkflowRuntime在加载工作流时,会到数据库中查找该工作流的基本信息。
如果没有,就将该工作流的模板信息(工作流类型与工作流的Activity)存入数据库的基本信息表中。
4.如果修改了上面所提的那个工作流(如添加Activity),再次运行时,SqlTrackingService服务不会自动更新数据库中的基本信息,这样在执行查询类操作时可能会产生异常。
解决上述问题的办法是:A.修改工作流类名,将其作为一个新工作流由SqlTrackingService服务B.在数据库中删除原来的信息,由SqlTrackingService服务将其作为一个新工作流再次添加C.修改数据中相应的配置信息1.添加服务到引擎进行跟踪在WorkflowRuntime宿主程序中2.SqlTrackingQuery状态查询类SqlTrackingWorkflowInstance被查询对象类1.通过SqlTrackingQuery的TryGetWorkflow方法得到SqlTrackingWorkflowInstance对象2.通过SqlTrackingWorkflowInstance对象的属性与集合访问各类具体信息无须在WorkflowRuntime宿主程序中属性集合方法WorkflowTrackingRecord 实例状态类WorkFlow状态对象WorkflowTrackingRecord以集合成员的方式存于SqlTrackingWorkflowInstance的WorkflowEvents 集合中无须在WorkflowRuntime宿主程序中属性EventArgs实例[挂起、终止、异常]的具体信息由WorkflowTrackingRecord.EventArgs返回TrackingWorkflowSuspendedEventArgs :挂起TrackingWorkflowTerminatedEventArgs:终止(异常就是引起终止的原因之一)TrackingWorkflowExceptionEventArgs:异常等TrackingWorkflow______Args对象,通过数据类型转换后可得到挂起 / 终止 / 异常的具体信息ActivityTrackingRecord结点状态类Activity状态对象ActivityTrackingRecord以集合成员的方式存于SqlTrackingWorkflowInstance的ActivityEvents 集合中WorkflowRuntime属性UserTrackingRecord业务状态类业务状态对象:UserTrackingRecord以集合成员的方式存于SqlTrackingWorkflowInstance的UserEvents集合中业务状态如何添加见[在Activty中向Tracking添加业务状态]WorkflowRuntime属性RuleActionTrackingEvent策略集中的策略状态(Policy节点)Workflow.Activities.Rules.RuleActionTrackingEvent具体见自定义Tracking的该部分二、自定义Tracking服务WWF提供一个tracking基本结构,可以使用他跟踪去实例改变的数据与状态,在加载项里,他提供了可伸缩性去建立更多跟踪服务应用,为一些用户的商业应用自写义tracking服务,需要实现TrackingChannel 与TrackingServic这两个类,TrackingChannel接收引擎发送的各种tracking记录,TrackingServic服务为引擎提供了接口The tracking service provides the runtime with tracking profiles based on specific parameters and conditions. It is also responsible for providing a tracking channel that receives the data sent by the runtime.引擎调用tracking服务是同步的,工作流实例执行一个阻塞直到从tracking服务有方法返回TrackingChannel实现TrackingService实现高级说明TrackingParameters类引擎将实例以[TrackingParameters类]形式传给[自定义跟踪服务TrackingService],[自定义跟踪服务]再传给[自定义Tracking通道]以[通道]在向外抛数据时可以使用传进来的该对象得到一些实例信息属性三、自定义筛选Tacking Pofile1.Tacking服务所要记录的状态是可以通过自定义Tacking Pofile进行筛选的.2.默认Tacking服务对 [实例的各类状态]、[Activity的各类状态]、[用户跟踪行为]的所有状态进行记录,可以自定义自定义Tacking Pofile让Tacking服务只记录实际需要的状态.以下各类状态变化可被Tacking服务记录,也可用Tacking Pofile进行筛选:3.自定义Tacking Pofile将生成一个XML串,存入TrackingProfile表的TrackingProfileXml字段中4.默认的Tacking Pofile以一个XML串的形式存于DefaultTrackingProfile表的TrackingProfileXml字段中5.自定义Tacking Pofile只对指定的工作流有效,默认的Tacking Pofile对所有没有自定义Tacking Pofile的工作流有效。
5g trackingareacode的值域
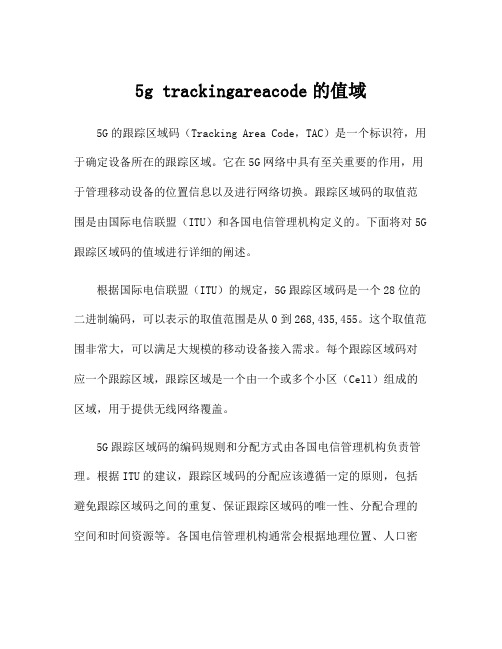
5g trackingareacode的值域5G的跟踪区域码(Tracking Area Code,TAC)是一个标识符,用于确定设备所在的跟踪区域。
它在5G网络中具有至关重要的作用,用于管理移动设备的位置信息以及进行网络切换。
跟踪区域码的取值范围是由国际电信联盟(ITU)和各国电信管理机构定义的。
下面将对5G 跟踪区域码的值域进行详细的阐述。
根据国际电信联盟(ITU)的规定,5G跟踪区域码是一个28位的二进制编码,可以表示的取值范围是从0到268,435,455。
这个取值范围非常大,可以满足大规模的移动设备接入需求。
每个跟踪区域码对应一个跟踪区域,跟踪区域是一个由一个或多个小区(Cell)组成的区域,用于提供无线网络覆盖。
5G跟踪区域码的编码规则和分配方式由各国电信管理机构负责管理。
根据ITU的建议,跟踪区域码的分配应该遵循一定的原则,包括避免跟踪区域码之间的重复、保证跟踪区域码的唯一性、分配合理的空间和时间资源等。
各国电信管理机构通常会根据地理位置、人口密度等因素来划分跟踪区域,并为每个跟踪区域分配一个唯一的跟踪区域码。
在5G网络中,跟踪区域码的取值决定了设备所属的跟踪区域。
移动设备在连接到网络后,会被分配一个跟踪区域码,并根据该码值进行网络切换和位置更新。
当设备进入一个新的跟踪区域时,它会向网络发送位置更新请求,网络会根据设备所在的跟踪区域码来确定其位置,并作出相应的调度决策。
跟踪区域码的值域在5G网络中扮演着重要的角色。
它不仅仅是标识设备所在跟踪区域的一个数字,还可以用于设备的位置管理、网络资源调度、信令传输等方面。
跟踪区域码的取值范围足够大,可以满足未来大规模设备接入的需求,同时也为网络规划和优化提供了灵活性。
总之,5G跟踪区域码的取值范围是从0到268,435,455,根据各国电信管理机构的规定进行分配和管理。
这个范围的大小可以满足大规模设备接入的需求,并为网络提供可靠的位置信息和调度能力。
TrackingService 、TrackingChannel 自定义跟踪服务
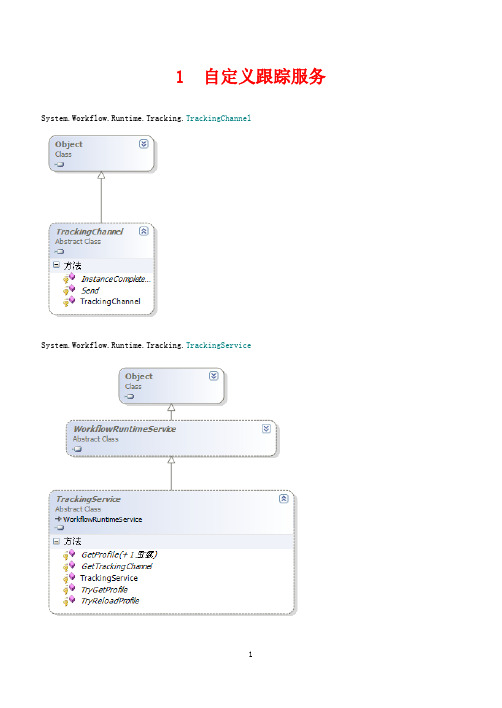
//筛选业务状态点(用户状态点)中的规则
if(erDataisSystem.Workflow.Activities.Rules.RuleActionTrackingEvent)
3.TrackingChannel接收引擎发送的各种tracking记录,
4.TrackingServic为引擎提供了接口
5.引擎调用tracking服务是同步的,工作流实例执行一个阻塞直到从tracking服务有方法返回
6.WF的工作流引擎是个黑箱子,所有有关工作流实例运行的情况或事件只有WF引擎知道,Hosting如果想知道,那么需要一个查询的界面。Tracking就是这个查询界面
{RuleActionTrackingEventobj3_rule = (RuleActionTrackingEvent)erData;
Console.WriteLine("规则名: "+ obj3_rule.RuleName.ToString());
//Policy绑定的规则集中的每个规则都会发送一组状态,Policy有点像职责链,具体以后讲
//Policy规则是自动将信息抛出的,但在类型上算上用户状态
}
}
}
}
}
//筛选结点
if(recordisActivityTrackingRecord)
{
ActivityTrackingRecordobj2 = (ActivityTrackingRecord)record;
Console.WriteLine("时间: "+ obj2.EventDateTime.ToString());
- 1、下载文档前请自行甄别文档内容的完整性,平台不提供额外的编辑、内容补充、找答案等附加服务。
- 2、"仅部分预览"的文档,不可在线预览部分如存在完整性等问题,可反馈申请退款(可完整预览的文档不适用该条件!)。
- 3、如文档侵犯您的权益,请联系客服反馈,我们会尽快为您处理(人工客服工作时间:9:00-18:30)。
EUROPEAN ORGANIZATION FOR NUCLEAR RESEARCHCERN-SL DIVISIONCERN-SL-2002-037 (AP) Chromaticity Measurements via RF Phase Modulation andContinuous Tune TrackingO.S. Brüning, W. Höfle, R. Jones, T. Linnecar, H. SchmicklerChromaticity diagnostics with high time resolution is of paramount importance forthe control of the dynamic events in various accelerators, in particular for the LHCcollider. This paper describes the possibility of measuring the machinechromaticity via RF phase modulation and continuous tune tracking. The RF phasemodulation can be done at much higher frequencies than a classical RF frequencyvariation and thus, allows chromaticity measurements with a time resolutionbelow the second. The paper describes the general measurement principle anddiscusses in detail open questions, which still have to be addressedexperimentally. First results from machine measurements in the CERN SPS onbeam stability during RF phase modulation are presented.8th European Particle Accelerator Conference, 3-7 June 2002Paris, FranceGeneva, Switzerland25 June, 2002SL/DIV RepsChromaticity Measurements via RF Phase Modulation and Continuous TuneTrackingO.S.Br¨u ning,W.H¨ofle,R.Jones,T.Linnecar,H.Schmickler,CERN,Geneva,SwitzerlandAbstractChromaticity diagnostics with high time resolution is of paramount importance for the control of the dynamic events in various accelerators,in particular for the LHC collider.This paper describes the possibility of measur-ing the machine chromaticity via RF phase modulation and continuous tune tracking.The RF phase modulation can be done at much higher frequencies than a classical RF fre-quency variation and thus,allows chromaticity measure-ments with a time resolution below the second.The paper describes the general measurement principle and discusses in detail open questions,which still have to be addressed experimentally.First results from machine measurements in the CERN SPS on beam stability during RF phase mod-ulation are presented.1SEXTUPOLE COMPONENTS IN THELHC DIPOLE MAGNETS Persistent current decay changes the miltipolefield com-ponents in superconducting magnets with time.These dy-namic effects impose stringent control requirements for the operation of a superconducting storage ring.Table1shows a comparison of the maximum sextupolefield error decay in existing and planned hadron colliders[1].Table1:Expected chromaticity change due to the sextupole (b3)persistent current decay in existing and planned super conducting hadron storage rings.machine total b3generated Q ∆Q due to b3decay Q x Q y Q x Q y Tevatron-140+119+8-7 HERAp-275+245+13-11RHIC-38+36+2-2LHC-450+450+150-150 The change in chromaticity occurs over a time scale of ca.20minutes during theflat injection plateau and at the beginning of the ramp over a time scale of a few tens of seconds(depending on the initial ramp speed).Stable parti-cle motion requires the control of the machine chromaticity within a window of1<Q <5.(1)For negative chromaticity values the collective bunch mo-tion becomes unstable and for chromaticity values larger than5units the single particle motion will become unsta-ble.In order to control the chromaticity of the LHC within the window given in Equation(1)one needs to dynamically correct the b3field error of the dipole magnets within0.5%. Such an accuracy is not achievable via reference magnet measurements[1]and requires additional beam based mea-surements.1.1Beam based chromaticity measurements The standard procedure for measuring the machine chro-maticity is based on frequency modulations of the radio fre-quency(RF)system.Changing the RF frequency changes the beam energy and the central orbit in the machine.The energy and orbit variations change the machine tune pro-portionally to the total machine chromaticity.The above procedure tends to be slow(measurement rate lower than 0.1Hz)and,due to the change in energy and orbit,per-turbs the normal machine operation.Due to the limited momentum acceptance of the LHC this procedure is not compatible with the nominal LHC operation[2].An alternative procedures for fast chromaticity measure-ments based on the head-tail oscillation of the bunches has been proposed in[3].However,while the method satis-fies the requirements on measurement precision and speed it turns out to blow up the beam emittance and can not be used during the nominal machine operation[4]. Controlling the machine chromaticity via beam based measurements in the LHC still requires the development of new measurement techniques which offer fast measure-ment rates with an accuracy of one unit in the chromaticity and which can be used during nominal machine operation without causing a deterioration of the beam parameters.In the following we discuss the possibility of measuring the chromaticity via RF phase modulation and continuous tune tracking.2CHROMATICITY MEASUREMENT VIA RF PHASE MODULATION Similar to an RF frequency modulation,a modulation of the RF phase also changes the particle energy and orbit in the machine.However,compared to an RF frequency modula-tion the RF phase modulation can be done at much faster frequencies which lie outside the bunch spectrum(faster measurement procedure and no emittance blow up).Fig.1 shows the resulting energy modulation versus the RF phase modulation frequency for afixed modulation amplitude of 10degrees.The solid line corresponds to a rigid bunch model and the dashed line to a soft bunch model.While the response of the rigid bunch always occurs at the excita-tion frequency,the response of the soft bunch always con-tains additional frequency components at multiples of the synchrotron frequency.For example,the two resonance re-sponses in the soft bunch model at 157Hz and 380Hz both occur at the synchrotron frequency.00.00050.0010.00150.0020.00250.0030.00350.0040.0045↑∆p/p 0→excitation frequency in Hz↑f syncFigure 1:Expected net energy modulation versus RF phase modulation frequency for a fixed modulation amplitude of 10degrees for the SPS data.The solid line corresponds to a rigid bunch model and the dashed line to a soft bunch model.Two effects limit the choice of modulation frequency and amplitude.The RF phase modulation generates a net re-duction of the RF bucket area and the maximum modula-tion amplitude will be limited by the available bucket area.A second constraint comes from the RF phase loop which will actively damp longitudinal oscillations.The modula-tion frequency must be chosen such that it does not inter-fere with the RF phase loop.In the machine experiments performed in the CERN SPS we chose a modulation amplitude of 10degrees and a modulation frequency of approximately five times the syn-chrotron frequency at which the RF phase loop is no longer active.With the above limitations the RF phase modulation can generate only a small energy modulation (∆p/p 0≈10−4for the SPS parameters)and we need to measure the re-sulting tune modulation via a high precision tune measure-ment.One possibility for measuring a tune modulation with an amplitudes of ∆p/p 0≈10−4is to frequency anal-yse the voltage signal of the voltage controlled oscillator (VCO)in a phase locked loop (PLL)circuit [5].While this procedure allows a precise measurement of the modulation frequency it does not provide a measurement of the modu-lation amplitude and the measured signal requires calibra-tion with an additional modulation signal of known am-plitude.One possibility for such a calibration is to excite an additional tune modulation with slightly different fre-quency via a quadrupole circuit.The quadrupole modula-tion amplitude is increased until the two modulation am-plitudes have the same amplitude in the Fourier spectrum and the tune modulation amplitude due to the RF phase modulation must be equal to the (known)amplitude of the quadrupole modulation.Since the energy modulation is known,the absolute value of the machine chromaticity can then be deduced via|∆Q |=|Q |·|∆p p 0|,(2)where Q is the machine chromaticity.Another possibil-ity for calibrating the Fourier spectrum is to choose thequadrupole modulation frequency equal to the RF phase modulation frequency and with a constant phase relation-ship of 180degrees between the two signals.If the tune modulation amplitudes of the two excitations is equal the corresponding frequency will not appear in the Fourier spectrum.While the first calibration technique generates a net tune modulation that might deteriorate the long term stability of the particle motion the second technique will not impose a net tune modulation to the particle motion.A second advantage of the second technique is that,in addi-tion to the absolute value of the machine chromaticity,it also provides the sign of the chromaticity.Fig.2shows a schematic layout of the required measure-ment setup.Figure 2:Schematic setup for a chromaticity measurement using a PLL.3MEASUREMENT SETUP IN THECERN SPSFirst measurements in the CERN SPS had the goal of demonstrating that an RF phase modulation with an am-plitude of 10degrees and a frequency of 600Hz to 800Hz does neither cause particle losses nor emittance growth.Because there was no PLL available in the CERN SPS at the time of the measurements we could not yet demonstrate the full functionality of the above measurement procedure.However,by measuring the Fourier spectrum of a trans-verse orbit pickup at a dispersion free location the mea-surements could demonstrate that the RF phase modulation indeed generates a signal in the transverse plane which is proportional to the machine chromaticity.The measured beam response to a transverse excitation depends on how close the excitation frequency is to the betatron oscillation frequency.In the presence of tune modulation (periodic change of the betatron frequency)an excitation at fixed fre-quency will thus generate a pickup signal that is modulated at the tune modulation frequency.In order to avoid spu-rious signals through dispersion at the pickup location weused an excitation in the vertical plane and a vertical pickup in the SPS experiments.4FIRST MEASUREMENT RESULTSTable 2shows the main machine parameters and Table 3the main parameters for the measurements in the CERN SPS.synch RF energy #of particles freq frequency (inj)bunches /bunch 157Hz200MHz26GeV14·1010Table 2:Main machine parameters for the measurements in the CERN SPS.510152025300.560.580.60.620.64→frequency in terms of fractional tuneFigure 3:Non-normalised spectrum of the beam position monitor reading for a vertical excitation at 17.74kHz and an RF phase modulation of 10◦at 615Hz.The vertical axis shows the frequency in terms of the fractional tune (i.q.f line /f rev and 1−f line /f rev )and the horizontal axis the amplitude of the spectral lines in arbitrary units on a lin-ear scale.The spectral line at the left corresponds to the RF phase modulation frequency and the spectral line at the right to the vertical excitation frequency.The spectrum was taken for a machine chromaticity of 10units.modulation modulation transverse betatron frequency amplitude excitation tune 615Hz 10◦17.74kHz 25.569kHz (0.5764)(0.5904)(0.5905)Table 3:Measurement parameters for the CERN SPS.The upper value for the transverse and longitudinal excitation and betatron frequencies show the frequencies in Hz and the lower values give the corresponding fractional tune val-ues.All measurements in the CERN SPS were done on a sin-gle bunch at injection energy (26GeV)with an RF phase modulation amplitude of 10degrees in the 200MHz travel-ling wave RF system.The modulation frequency was var-ied between 600Hz and 800HzFig.3shows a typical spectrum of the beam position monitor reading.The vertical axis shows the frequency in terms of the fractional tune (i.q.f line /f rev and 1−f line /f rev ).One clearly recognises the frequency line of the RF phase modulation on the left side of the spectrum at Q RF =0.576(615HZ)and the vertical excitation fre-quency at Q excitation =0.59(17.74kHz)on the right.Fig.4shows the amplitude of the spectral line correspond-ing to the RF phase modulation as a function of the ma-chine chromaticity.One clearly recognises that the mea-sured spectral line increases with the machine chromaticity.1234567891002468101214→QFigure 4:The amplitude of the spectral line corresponding to the RF phase modulation as a function of the machine chromaticity.5SUMMARY AND FUTURE PLANSThe first measurements in the CERN SPS showed that anRF phase modulation can be used to generate an energy modulation of ∆p/p 0=10−4at 600Hz to 800Hz without generating particle losses or longitudinal emittance growth.The measurements further showed that the resulting trans-verse tune modulation is proportional to the machine chro-maticity.The next steps for validating the proposed method for a fast chromaticity measurement include a continuous tune measurement with a PLL and a calibration of the PLL spectral lines via an additional tune modulation using quadrupole circuits.6REFERENCES[1]W.Fischer et al,’Beam Based Measurements of PersistentCurrent Decay in RHIC’,Phys.Rev.ST Accelerator Beams 4,2001[2]H.Schmickler,’Diagnostics and Control of the Time Evo-lution of Beam Parameters’CERN-SL-97-68presented at the 3rd European Workshop on Beam Diagnostics and In-strumentation for Particle Accelerators’,DIPAC97,Frascati,Italy,October 1997.[3] D.Cocq,O.R.Jones,H.Schmickler,’The Measurement ofChromaticity via a Head Tail Phase Shift’,8th Beam Instru-mentation Workshop BIW’98,Stanford,USA,May 1998.[4]R.Jones,H.Schmickler,’The Measurement of Q andQ in the CERN-SPS by Head Tail Phase Shift Analysis’,PAC2001,Chicago,June 2001.[5]O.Br¨u ning and F.Willeke,’Reduction of proton losses inHERA by compensating tune ripple due to power supplies’,Phys.Rev.Letters 76,3719,(1996).。