Data Mining Geoscientific Data Sets Using Self Organizing Maps
数据挖掘易出现单词

1、data mining数据挖掘Both data excavation technology and computer intelligence are the important technique supporting this study数据挖掘技术及计算机智能的发展是研究的重要技术支持。
2、data warehouse 数据仓库During this period, data warehousing vendors were recognizing the need to provide tools that were more tightly integrated, had full GUI invocation and were priced more attractively在这一时期,数据仓库供应商认识到了提供更紧密集成,拥有完整图形用户接口并且价格更吸引人的工具之需求。
3、OLAP 联机分析技术(On-Line Analytical Processing)The component's distribution of bio-oil, which was produced from Fraximus mandshurica by flashpyrolysis in a fluidized bed reactor, was determined by GC-MS analysis结合色质联机技术分析了由流化床热裂解水曲柳获得的生物油主要组分的分布。
4、OLTP 联机事务处理OLTP is mainly used for the application service of an organization.操作型处理主要是为一个组织某些方面的应用服务的5、Integrated 综合的;完整的;互相协调的how will they integrate...are they ? integrated into the large Indian society?他们是否融入了,印度社会呢?6、Summarized 总结The issue of vagueness is summarized in a more technical way by the philosopher Karl Popper who described--who introduced the term of falsifiability.关于概念模糊的问题,哲学家卡尔·波普用一个更加专业的术语,进行了总结,他提出了“可证伪性“这个术语。
Data Mining是什么意思
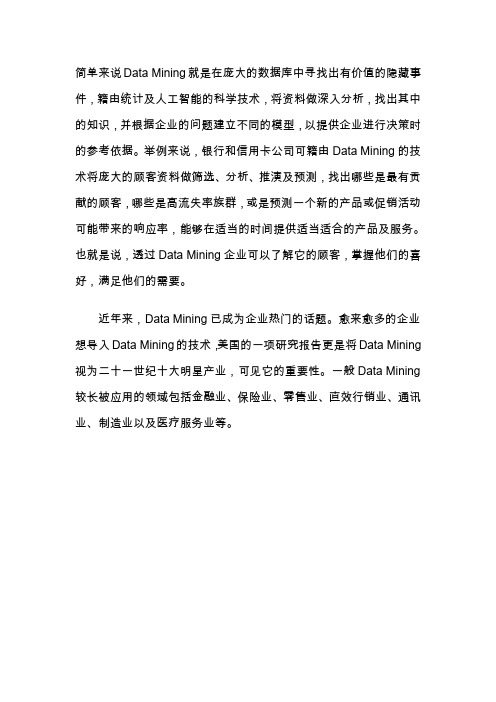
简单来说Data Mining就是在庞大的数据库中寻找出有价值的隐藏事件,籍由统计及人工智能的科学技术,将资料做深入分析,找出其中的知识,并根据企业的问题建立不同的模型,以提供企业进行决策时的参考依据。
举例来说,银行和信用卡公司可籍由Data Mining的技术将庞大的顾客资料做筛选、分析、推演及预测,找出哪些是最有贡献的顾客,哪些是高流失率族群,或是预测一个新的产品或促销活动可能带来的响应率,能够在适当的时间提供适当适合的产品及服务。
也就是说,透过Data Mining企业可以了解它的顾客,掌握他们的喜好,满足他们的需要。
近年来,Data Mining已成为企业热门的话题。
愈来愈多的企业想导入Data Mining的技术,美国的一项研究报告更是将Data Mining 视为二十一世纪十大明星产业,可见它的重要性。
一般Data Mining 较长被应用的领域包括金融业、保险业、零售业、直效行销业、通讯业、制造业以及医疗服务业等。
关于大数据你应该知道的50个专业术语
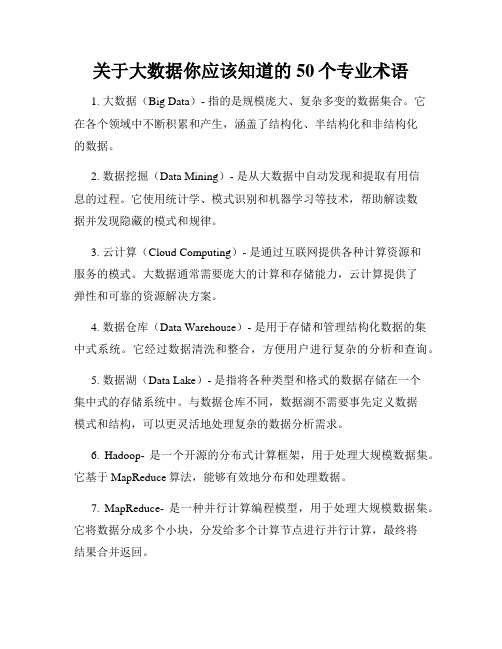
关于大数据你应该知道的50个专业术语1. 大数据(Big Data)- 指的是规模庞大、复杂多变的数据集合。
它在各个领域中不断积累和产生,涵盖了结构化、半结构化和非结构化的数据。
2. 数据挖掘(Data Mining)- 是从大数据中自动发现和提取有用信息的过程。
它使用统计学、模式识别和机器学习等技术,帮助解读数据并发现隐藏的模式和规律。
3. 云计算(Cloud Computing)- 是通过互联网提供各种计算资源和服务的模式。
大数据通常需要庞大的计算和存储能力,云计算提供了弹性和可靠的资源解决方案。
4. 数据仓库(Data Warehouse)- 是用于存储和管理结构化数据的集中式系统。
它经过数据清洗和整合,方便用户进行复杂的分析和查询。
5. 数据湖(Data Lake)- 是指将各种类型和格式的数据存储在一个集中式的存储系统中。
与数据仓库不同,数据湖不需要事先定义数据模式和结构,可以更灵活地处理复杂的数据分析需求。
6. Hadoop- 是一个开源的分布式计算框架,用于处理大规模数据集。
它基于MapReduce算法,能够有效地分布和处理数据。
7. MapReduce- 是一种并行计算编程模型,用于处理大规模数据集。
它将数据分成多个小块,分发给多个计算节点进行并行计算,最终将结果合并返回。
8. Spark- 是一个快速、通用、高级的大数据处理引擎。
它支持内存计算,能够在大数据集上进行复杂的数据处理和分析。
9. 数据可视化(Data Visualization)- 是将数据以图表、图形和其他可视化形式展示的过程。
它能够帮助用户更好地理解和分析数据,发现潜在的信息和见解。
10. 数据清洗(Data Cleaning)- 是处理和修正数据中的错误、缺失和不一致之前的过程。
清洗后的数据更加准确可靠,有助于后续的分析和应用。
11. 数据集成(Data Integration)- 是将来自不同数据源的数据合并成一个统一的数据集的过程。
数据挖掘名词解释
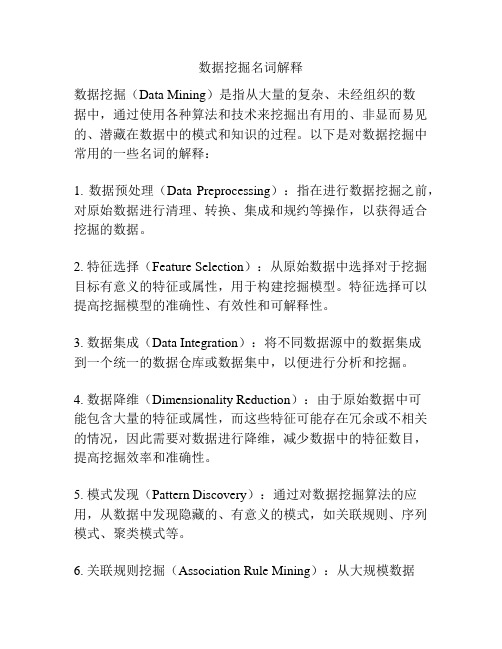
数据挖掘名词解释数据挖掘(Data Mining)是指从大量的复杂、未经组织的数据中,通过使用各种算法和技术来挖掘出有用的、非显而易见的、潜藏在数据中的模式和知识的过程。
以下是对数据挖掘中常用的一些名词的解释:1. 数据预处理(Data Preprocessing):指在进行数据挖掘之前,对原始数据进行清理、转换、集成和规约等操作,以获得适合挖掘的数据。
2. 特征选择(Feature Selection):从原始数据中选择对于挖掘目标有意义的特征或属性,用于构建挖掘模型。
特征选择可以提高挖掘模型的准确性、有效性和可解释性。
3. 数据集成(Data Integration):将不同数据源中的数据集成到一个统一的数据仓库或数据集中,以便进行分析和挖掘。
4. 数据降维(Dimensionality Reduction):由于原始数据中可能包含大量的特征或属性,而这些特征可能存在冗余或不相关的情况,因此需要对数据进行降维,减少数据中的特征数目,提高挖掘效率和准确性。
5. 模式发现(Pattern Discovery):通过对数据挖掘算法的应用,从数据中发现隐藏的、有意义的模式,如关联规则、序列模式、聚类模式等。
6. 关联规则挖掘(Association Rule Mining):从大规模数据集中挖掘出频繁出现的项集和项集之间的关联规则。
关联规则挖掘常用于市场篮子分析、购物推荐、交叉销售等领域。
7. 分类(Classification):根据已知的样本和样本的标签,训练分类模型,然后用于对未标注样本的分类预测。
分类是数据挖掘中的一项重要任务,常用于客户分类、欺诈检测、垃圾邮件过滤等场景。
8. 聚类(Clustering):根据数据中的相似性或距离度量,将样本划分为若干个组或簇,使得同组内的样本更加相似,不同组之间的样本差异更大。
聚类可用于市场细分、用户群体划分、图像分析等领域。
9. 时间序列分析(Time Series Analysis):针对按时间顺序排列的数据,通过挖掘数据中的趋势、周期性、季节性等模式,预测未来的走势和变化。
国立高雄应用科技大学电机工程系资料探勘实验室解读

班級:博電一甲 學號:1097404103 姓名:鍾翔航
實驗室首頁:.tw/
利用文件探勘技術,針對萃取癌症知識之生物資訊研究
跨語言與單一語言文件抄襲偵測之研究方法
Data Mining 是什麼?
• Data Mining是從資料中找出有用珍貴知識的一個過程
1.計畫應用 應用導入計畫 2.專案監督維護 監督與維護計畫 3.結案報告 結案報告或簡報 4.檢視專案 經驗傳承及技術文件
18
Evaluate Results
Plan Deployment Plan Monitering & Maintenance Produce Final Report Review Project
Assess Situation
Determine Data Mining Goals Produce Project Plan
交叉通路銷售 顧客終身價值及利潤價值 維持顧客模型 詐欺理賠分析
目標客戶分析
客戶區隔 需求預測分析
Copyright 2003-12, SPSS Taiwan Corp.
DM處理流程 (1997年於阿姆斯特丹正式誕生CRISP-DM SIG)
1.
定義企業問題
2.
資料準備
整合性服務
6.
分析服務 報表服務
良率分析 製程改善 需求預測
醫療業
病人流失管理 潛在新病人分析 病人屬性區隔
Copyright 2003-12, SPSS Taiwan Corp.
各產業的應用層面
銀行 風險管理 行銷回應預測 維持顧客模型 顧客終身價值及利潤價值 多通路行為管理 信用卡冒用偵測 保險業 生命科學 基因抗藥反應分析 基因序列分析 藥物區隔分析 藥物市場風險評估 娛樂業 客戶流失管理
什么是数据挖掘
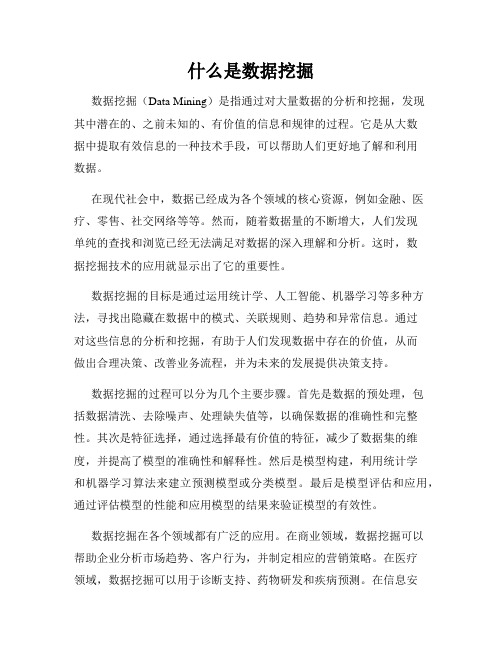
什么是数据挖掘数据挖掘(Data Mining)是指通过对大量数据的分析和挖掘,发现其中潜在的、之前未知的、有价值的信息和规律的过程。
它是从大数据中提取有效信息的一种技术手段,可以帮助人们更好地了解和利用数据。
在现代社会中,数据已经成为各个领域的核心资源,例如金融、医疗、零售、社交网络等等。
然而,随着数据量的不断增大,人们发现单纯的查找和浏览已经无法满足对数据的深入理解和分析。
这时,数据挖掘技术的应用就显示出了它的重要性。
数据挖掘的目标是通过运用统计学、人工智能、机器学习等多种方法,寻找出隐藏在数据中的模式、关联规则、趋势和异常信息。
通过对这些信息的分析和挖掘,有助于人们发现数据中存在的价值,从而做出合理决策、改善业务流程,并为未来的发展提供决策支持。
数据挖掘的过程可以分为几个主要步骤。
首先是数据的预处理,包括数据清洗、去除噪声、处理缺失值等,以确保数据的准确性和完整性。
其次是特征选择,通过选择最有价值的特征,减少了数据集的维度,并提高了模型的准确性和解释性。
然后是模型构建,利用统计学和机器学习算法来建立预测模型或分类模型。
最后是模型评估和应用,通过评估模型的性能和应用模型的结果来验证模型的有效性。
数据挖掘在各个领域都有广泛的应用。
在商业领域,数据挖掘可以帮助企业分析市场趋势、客户行为,并制定相应的营销策略。
在医疗领域,数据挖掘可以用于诊断支持、药物研发和疾病预测。
在信息安全领域,数据挖掘可以帮助识别网络攻击和异常行为。
在社交网络领域,数据挖掘可以用于用户画像分析、推荐系统等。
然而,数据挖掘也存在一些挑战和风险。
首先是数据质量问题,不完整、不准确的数据可能导致挖掘结果的不准确性。
其次是隐私保护问题,许多数据挖掘任务需要使用个人隐私数据,因此在数据挖掘过程中要注意保护个人隐私。
另外,过度依赖数据挖掘结果也可能导致误导和错误决策的风险,需要在决策过程中综合考虑多个因素。
总的来说,数据挖掘是一种重要的技术手段,可以帮助人们从海量数据中发现有用的信息,提高决策的准确性和效率。
26全球在地化、在地全球化

26:全球在地化(Glocalization)、在地全球化請舉例說明「全球在地化」與「在地全球化」之意義,及對教育改革的影響一、全球在地化(Glocalization)定義:羅伯森(Roland Robertson)(1992)提出「全球在地化」的概念。
.所謂「全球在地化」指跨國企業(組織)考量世界各地的特殊情況,將公司或旗下的商品或服務做適當調整,以符應當地文化和社會特性及需求,並藉以增加銷售成果。
二、全地化浪潮的衝擊-英語學習國中→國小、華語的「在地全球化」現象擺脫被全球化的「主流」意識形態宰制,找出「主體性」的具體作法、彰顯適性教學的教育政策、掙脫工具牢籠,預測未來教育。
除了生活外,教育也受到全地化浪潮的衝擊,故也有全球在地化和在地化的現象。
例如:台灣的教育政策將英語學習,從原來的國中過程,改為從國小即開始,這就是因應全球化的一種改變。
全球各地也有愈來愈多得外國人學習華語,只是其腔調仍各有不同,這種現象對華語而言,也是一種華語的「在地全球化」現象。
總之,全球在地化和在地全球化都是受到全球化趨勢的影響後產生的現象,也都是為了因應全球化的衝擊。
總之,無論全球在地化或在地全球化,都為了能在全球化得衝擊下,擺脫被全球化的「主流」意識形態宰制,並企圖找出「主體性」的具體作法。
此等精神也彰顯在強調適性教學的教育政策上。
析言之,真正的教學是教人不只是教書,教學的旨趣在於激發學生自主性自律能力,並發揮學生的創意。
教育若欲提升個人或社群的競爭力,就不能不培養學生展現具自我特色的創意。
三、適性學習的理想-孩子自己活出自己,開發個人的潛能。
有鑑於全球在地化和再地全球化之時空的急遽變化。
則亦是因國本世紀教育改革所以將重點置於落實適性教學的重要原因。
適性學習的理想在於「不只別讓任何孩子落後而已」,且應該讓孩子自己活出自己,開發個人的潛能。
四、適性化學習定義:適性化學習係英國教育科技能部,配合每位學生的才能與需要,實施適性化學習。
我所知道的一点DataMining-电子邮件系统
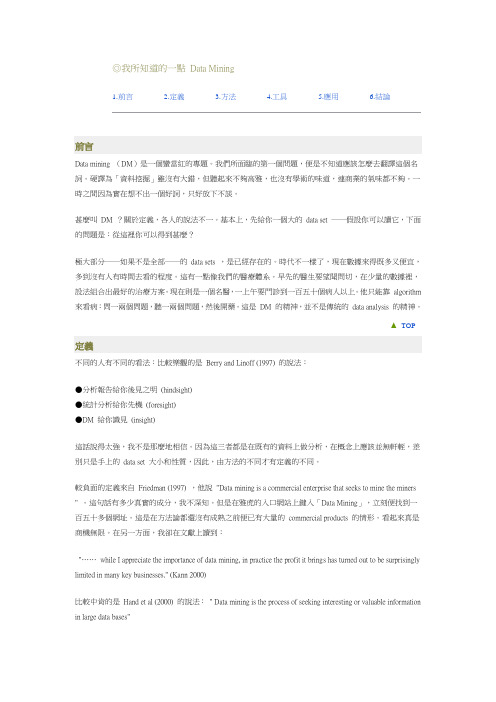
◎我所知道的一點Data Mining1.前言2.定義3.方法4.工具5.應用6.結論◎以上內容提供者:趙民德中央研究院統計科學研究所◎◎資料採礦(Data Mining)連載之一‧何謂DATA MINING‧DATA MINING和統計分析的不同‧為什麼需要DATA MINING何謂DATA MINING?資料採礦的工作(Data Mining)是近年來資料庫應用領域中,相當熱門的議題。
它是個神奇又時髦的技術,但卻也不是什麼新東西,因為Data Mining使用的分析方法,如預測模型(迴歸、時間數列)、資料庫分割(Database Segmentation)、連接分析(Link Analysis)、偏差偵測(Deviation Detection)等;美國政府從第二次世界大戰前,就在人口普查以及軍事方面使用這些技術,但是資訊科技的進展超乎想像,新工具的出現,例如關連式資料庫、物件導向資料庫、柔性計算理論(包括Neural network、Fuzzy theory、Genetic Algorithms、Rough Set等)、人工智慧的應用(如知識工程、專家系統),以及網路通訊技術的發展,使從資料堆中挖掘寶藏,常常能超越歸納範圍的關係;使Data Mining成為企業智慧的一部份。
Data Mining是一個浮現中的新領域。
在範圍和定義上、推理和期望上有一些不同。
挖掘的資訊和知識從巨大的資料庫而來,它被許多研究者在資料庫系統和機器學習(Machine learning)當作關鍵研究議題,而且也被企業體當作主要利基的重要所在。
有許多不同領域的專家,對Data Mining展現出極大興趣,例如在資訊服務業中,浮現一些應用,如在Internet之資料倉儲和線上服務,並且增加企業的許多生機。
隨著資訊科技的進步以及電子化時代的來臨,現今企業所面對的是一個與以往截然不同的競爭環境。
在資訊科技的推波助瀾下,不僅企業競爭的強度與速度倍數於以往,激增的市場交易也使得各企業所需儲存與處理的資料量越來越龐大。
- 1、下载文档前请自行甄别文档内容的完整性,平台不提供额外的编辑、内容补充、找答案等附加服务。
- 2、"仅部分预览"的文档,不可在线预览部分如存在完整性等问题,可反馈申请退款(可完整预览的文档不适用该条件!)。
- 3、如文档侵犯您的权益,请联系客服反馈,我们会尽快为您处理(人工客服工作时间:9:00-18:30)。
Data Mining Geoscientific Data Sets Using SelfOrganizing MapsS.J. Fraser(1), B.L. Dickson(2)(1) CSIRO Exploration & Mining , QCAT PO Box 883 Kenmore 4069, Australia, Stephen.Fraser@csiro.au(2) Dickson Research Pty Ltd, 47 Amiens St, Gladesville, 2111, Bruce.Dickson@.auAbstractGeoscientists are increasingly challenged by the joint interpretation of ever-expandingamounts of new and historic, spatially-located exploration data (e.g., geochemistry,geophysics, geology, mineralogy, elevation data, etc.). And because, we can gather datafaster than it can be interpreted, the availability of geographic information systems (GIS)has, to some extent, compounded, rather than reduced this problem. Research into theanalysis and interpretation methods for data held in a GIS is in its infancy. A limited numberof “advanced” interpretation methods have been developed; however, these often rely on apriori knowledge, training, or assumptions about mineralisation models. Objective,unsupervised methods for the spatial analysis of disparate data sets are needed.We have investigated and developed a new computational “tool” to assist in theinterpretation of spatially located mineral exploration data sets. Our procedures are basedon the data-ordering and visualization capabilities of the Self Organizing Map (SOM),combined with interactive software to investigate and display the spatial context of thederived SOM “clusters”. These computational procedures have the capacity to improve theefficiency and effectiveness of geoscientists as they attempt to discover and understand theoften subtle signals associated with specific geological processes (e.g., mineralization), andseparate them from the effects of overprinting noise caused by other processes such asmetamorphism or weathering.Based on the principles of “ordered vector quantization”, the SOM approach has theadvantage that all input data samples are represented as vectors in a data-space defined bythe number of observations (variables) for each sample. The SOM procedure is anexploratory data analysis technique whereby patterns and relationships within a databaseare internally derived (unsupervised) based on measures of vector similarity (e.g., Euclideandistance and the dot product). The outputs of a SOM analysis are highly visual, whichassists the analyst in understanding the data’s internal relationships.Keywords: Self Organizing Maps, Data Mining, GeosciencesIntroductionGeoscientists in general and explorationists in particular, commonly suffer data overload. Volumes of open-file reports, digital geological maps, geophysical data sets, and remotely sensed data are typically available. When these data are combined with the results of current exploration activities, serious data-overload problems can occur.Geographic Information Systems (GIS) and their capacity to store spatially located (digital) data have not necessarily assisted in the interpretation of data. More often than not, the incorporation of data into a GIS is seen as the ‘goal’, whereas in reality, it is only the first step in the data analysis and interpretation procedure. GIS are important in that they allow spatially located exploration data to be stored in a database (ideally with checks as to the data’s validity and integrity). However, the mechanisms for data interpretation in such systems, have not kept pace with the enthusiasm with which data can be collected and stored.Traditional multivariate statistical approaches are often confused by data sets with variable relationships that are non-linear, by data distributions that are non-normal (typically with multiple populations), and by the data sets themselves that may be disparate, sparsely-filled, (contain “nulls”), with both continuous and discontinuous numeric data and text. The SOM, ordered vector-quantization approach can overcome many of these problematic issues.A number of “advanced” interpretation methods have been developed for the GIS environment, such as “Weights of Evidence” (see, Bonham–Carter and Agterberg, 1999), “Neural Networks” (see Brown et. al, 2000) and other “Expert Systems”. These “advanced” methods often rely on a priori knowledge, training, or a subjective approach (assumptions about mineralization models, and the probabilities as to the significance of particular occurrences or features), which may or may not exist, be relevant, or available. There are very few techniques that enable a user to explore and analyze the relationships between the various data-layers stored in a GIS in an objective quantitative fashion.The authors have an ongoing interest in the development of tools and techniques to assist in the integrated analysis, interpretation and visualization of various exploration and mining related data sets, especially those with spatial or geographic attributes. For some time, they have been promoting the use of Self Organizing Map (SOM - Kohonen, 2001) as a knowledge discovery or exploratory, data analysis tool.The Self Organizing Map procedures are described in detail elsewhere (Kohonen, 2001). Briefly, however, if one represents all sample points as vectors in a data-space defined by the number of observations, the SOM procedure provides a non-parametric mapping (regression) that transforms an n-dimensional representation of these high dimensional, nonlinearly-related data items to a typically two-dimensional representation, in a fashion that provides both an un-supervised clustering and a highly visual representation of the data’s relationships. SOM procedures are used in a range of applications, but they are having a major impact in the fields of data exploration (Kaski, 1997) and data mining (Vesanto, 2000).The SOM procedures being developed by the authors are aimed at providing geoscientists with access to new methods for determining the intricate relationships, within and between multiple, spatially-located and complex data sets. The analysis and visualization provided via SOM has the potential to be significant to both spatial and non-spatial investigations involving both resource discovery and utilization.Except for some specific applications, SOM procedures have not been widely accepted in the geosciences nor used by the exploration and mining industries. Because of the relatively recent development of the technique, there is much to learn about the potential and application of SOM for analyzing resource related data sets. SOM has been widely used for data analysis in the fields of finance, speech analysis, astronomy (see Kaski et. al, 1998; Garcia-Berro et al., 2003) and more recently in petroleum well log and seismic interpretation (Strecker & Uden, 2002; Briqueu et al., 2002) and geochemisrtry and hyperspectral data (Penn, 2005).The SOM technique has characteristics and capabilities that make it ideal for geoscience applications, including: •An ability to identify and define subtle relationships within and between diverse data, such as continuous (e.g., geophysical logs) and categorical (e.g., rock-type) variables;•No required prior knowledge about the nature or number of clusters within the data (unsupervised);•No assumptions about statistical distributions of variables or linear correlations between variables;•Robust handling of missing and noisy data;•Additional analysis tools, such as component analysis, spatial analysis and the ability to use a pre-computed SOM as a classification framework for a new dataset.ResultsThree examples using our SOM approach for the analysis of geoscience data sets shall be presented.The first study used SOM to perform an analysis on some 40,000 located geochemical samples from drill-holes around a known copper-gold deposit. Each sample was assayed for up to 13 elements; however 60% of the variable cells were nulls. A consequence of the data being collected over a 10 year period, with different element suites being used as the paragenetic model for the deposit evolved. Three main gold populations were highlighted using the SOM procedure within the data set. The first we propose relates to transported particulate gold within overlying Mesozoic sediments, the second to hydromorphically transported gold that is being moved into the overlying sediments; and the third relates to gold at the interface/ unconformity between the overlying sediments and the basement lithologies. The SOM procedure was also able to highlight three spatial groupings of anomalous gold values. One was considered to be extensions to the known mine mineralization; the second related to a known prospect some four kilometers away from the mine; while the third occurs some 25km away in a scout drill hole that was part of a regional grid, drilled during regional evaluation. The geochemical samples from this third region, were not assayed for the same element suite for the holes around the known mine, but were based on an earlier, superseded model for mineralization in the area. The SOM procedure however, was able to assign those samples to similar groupings of samples around the mine, despite the fact that key elements were not assayed for in those samples.In the second study over another Au-prospect, geochemical assay measurements were supplemented by a geologist’s logged alteration descriptions. In this case the alteration descriptions were used as labels and not actually included in the SOM analysis. Two distinct high Au associations were delimited by the SOM analysis of some ten elements for each sample. One Au-association was related to high Ag values; the other Au association was related to only moderate Ag values. These two Au populations when plotted spatially form coherent spatial patterns. On a scatter plot of Au and Ag, values coloured by their SOM-assigned groupings, a distinct trend could be observed that we believe indicates the “process of mineralization”. This information can be used on spatial plots as a “vector-to-ore”. When the alterationlabels were overlain onto points on the scatter plot, there is a general trend evident from poorly mineralized propylitic samples through to highly mineralized samples exhibiting silica flooding; though not all samples logged as “silica-flooding” were highly mineralized.Our third study involves the use of SOM to assist in the analysis of hyperspectral reflectance data acquired by the HyLogger core–logging system on coal cores. The SOM procedure was applied to approximately 40,000 spectra, each with 522 channels of spectral values, to find natural “groupings” within these data that could be related to facies within the sediments and layering within the coal. In this case the SOM was used to simplify a very complex data set by “clumping” the data into meaningful packages that could be related to the geology by the domain analyst. DiscussionIn each study, the SOM procedure provided fundamental new knowledge, or assisted in simplifying the complexity present within these data, to assist in their analysis and interpretation. In both the first and second examples, the SOM visualizations alerted the analyst to evidence of geological processes present in the data, which assisted in their interpretation. In the third example, the SOM was used to simplify a complex data set into patterns that could be related to the coal sequence sedimentary packages. These capabilities are valuable contributions towards the analysis of geoscientific data sets, which are further enhanced by an ability to display the SOM outputs in their spatial context. The SOM procedure is an exploratory data analysis technique that derives the patterns and relationships within a data set in an unsupervised fashion based on measures of vector similarity (e.g., Euclidean distance and the dot product). The outputs of a SOM analysis are highly visual, which assists the analyst in understanding the data’s internal relationships, and relating them to geological processes.ReferencesG.F. Bonham–Carter and F.P Agterberg: Arc-WofE: a GIS tool for statistical integration of mineral explorationdatasets. pp. 497–500, Proceedings International Statistical Institute, Helsinki, August 11–16, 1999.L. Briqueu, S. Gottlib-Zeh, M. Ramadan, and J. Brulhet:Traitement des disgraphies a l’aide d’un reseau de neurons du type <<carte auto-organisatrice>>: application a l’etude lithogique de la couche silteuse de Marcoule (Gard France). C.R. Geoscience 334 (2002) 31-337. 2002.W.M. Brown, T.D. Gedeon, D.I. Groves, and R.G. Barnes; Artificial neural networks: a new method for mineral prospectivity mapping. Australian Journal of Earth Sciences; (2000) 47, 757-770, 2000.B.L. Dickson, D.A. Clark D.A., and S.J.Fraser: New techniques for interpretation of aerial gamma-ray surveys. FinalReport Project P491. CSIRO Exploration and Mining Report 653R, 20 pages, includes a CD ROM. 1999.E. Garcia-Berro, S. Santiago-Torres, and J.Isern: Using self-organizing maps to identify potential halo white dwarfs.Neural Networks 16 (2003) 405–410. 2003S. Kaski: Data exploration using self-organizing maps; Acta Polytechnica Scandinavica, Mathematics, Computing and Management in Engineering Series No. 82, Espoo 1997, 57 pp. Published by the Finnish Academy of Technology, 1997.S. Kaski, J. Kangas, and T. Kohonen: Bibliography of Self-Organizing Map (SOM) Papers: 1981--1997, Neural Computing Surveys, 1: 102-350. Available from /~jagota/NCS/. 1998.T. Kohonen: Self-Organizing Maps. Third Extended Edition, Springer Series in Information Sciences, Vol. 30, Springer, Berlin, Heidelberg, New York, 2001.T. Kohonen: Self Organized Formation of Topological Correct Feature Maps. Biol. Cyberbetics. Vol 43, 1982, pp.59-96, 1982.B. S. Penn: Using Self-Organizing maps to visualize high-dimensional data. Computers and Geosciences 31, 531-544.2005.U. Strecker, and R. Uden: Data mining of poststack seismic attribute volumes using Kohonen self-organizing maps.The Leading Edge, October 2002, pp1032 -1037. 2002。