Unsupervised signal restoration using hidden Markov chains with copulas
生态农业发展下的生态农场建设
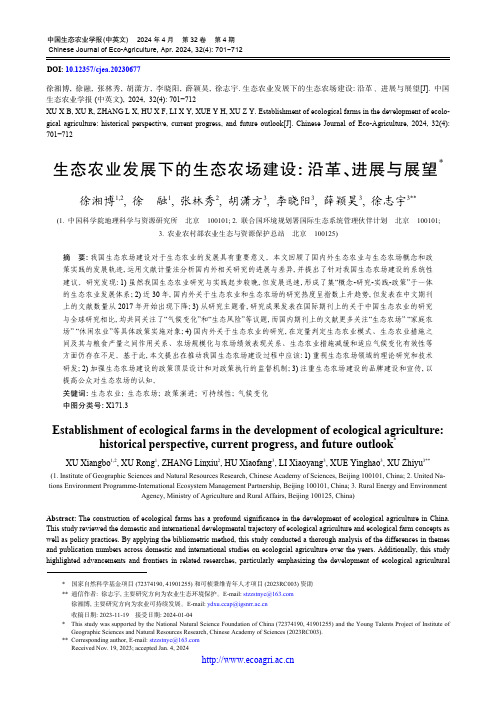
中国生态农业学报(中英文) 2024年4月 第 32 卷 第 4 期Chinese Journal of Eco-Agriculture, Apr. 2024, 32(4): 701−712DOI: 10.12357/cjea.20230677徐湘博, 徐融, 张林秀, 胡潇方, 李晓阳, 薛颖昊, 徐志宇. 生态农业发展下的生态农场建设: 沿革、进展与展望[J]. 中国生态农业学报 (中英文), 2024, 32(4): 701−712XU X B, XU R, ZHANG L X, HU X F, LI X Y, XUE Y H, XU Z Y. Establishment of ecological farms in the development of ecolo-gical agriculture: historical perspective, current progress, and future outlook[J]. Chinese Journal of Eco-Agriculture, 2024, 32(4): 701−712生态农业发展下的生态农场建设: 沿革、进展与展望*徐湘博1,2, 徐 融1, 张林秀2, 胡潇方3, 李晓阳3, 薛颖昊3, 徐志宇3**(1. 中国科学院地理科学与资源研究所 北京 100101; 2. 联合国环境规划署国际生态系统管理伙伴计划 北京 100101;3. 农业农村部农业生态与资源保护总站 北京 100125)摘 要: 我国生态农场建设对于生态农业的发展具有重要意义。
本文回顾了国内外生态农业与生态农场概念和政策实践的发展轨迹, 运用文献计量法分析国内外相关研究的进展与差异, 并提出了针对我国生态农场建设的系统性建议。
研究发现: 1)虽然我国生态农业研究与实践起步较晚, 但发展迅速, 形成了集“概念-研究-实践-政策”于一体的生态农业发展体系; 2)近30年, 国内外关于生态农业和生态农场的研究热度呈指数上升趋势, 但发表在中文期刊上的文献数量从2017年开始出现下降; 3)从研究主题看, 研究成果发表在国际期刊上的关于中国生态农业的研究与全球研究相比, 均共同关注了“气候变化”和“生态风险”等议题, 而国内期刊上的文献更多关注“生态农场” “家庭农场” “休闲农业”等具体政策实施对象; 4)国内外关于生态农业的研究, 在定量判定生态农业模式、生态农业措施之间及其与粮食产量之间作用关系、农场规模化与农场绩效表现关系、生态农业措施减缓和适应气候变化有效性等方面仍存在不足。
无监督学习的使用中常见错误(Ⅲ)

无监督学习(Unsupervised Learning)是机器学习领域的一个重要分支,它通过对数据的自动分析和模式识别来发现数据中的规律和结构。
与监督学习不同,无监督学习不需要事先标记好的训练数据,因此在很多实际应用中具有很大的吸引力。
然而,正是因为其自由度较高,无监督学习在使用中也容易出现一些常见的错误。
本文将从几个常见的角度来探讨无监督学习的使用中常见的错误。
数据预处理是无监督学习中一个很关键的环节。
不正确的数据预处理会直接影响到后续模型的性能。
常见的错误之一是忽略数据的分布情况。
在进行聚类或者降维等任务时,很多人会忽视数据的分布情况,直接将原始数据输入到算法中进行处理。
然而,如果数据的分布不均匀或者存在异常值,这样的处理结果很可能会偏离真实情况。
因此,在进行无监督学习之前,我们需要对数据的分布进行分析,如果发现数据存在较大的偏斜或者异常值,需要对数据进行相应的处理,比如去除异常值、进行数据平衡等。
另一个常见的错误是选择不合适的模型。
在无监督学习中,模型的选择是非常重要的。
不同的问题需要不同的模型来进行处理。
比如,在进行聚类任务时,我们可以选择K-means、层次聚类、DBSCAN等不同的算法,但是如果我们选择的算法不适合当前数据的特点,那么得到的聚类结果很可能会失真。
因此,在选择模型之前,我们需要对数据的特点有一个较好的认识,然后根据数据的特点来选择合适的模型。
另外,很多人在使用无监督学习时容易陷入“黑盒”陷阱。
所谓“黑盒”陷阱指的是使用无监督学习模型时只关注模型的输出结果,而忽视了模型内部的运行机制。
这样的做法会导致我们对数据的理解不深入,也无法发现模型内部的问题。
因此,在使用无监督学习模型时,我们需要对模型的内部机制有一个比较清晰的认识,理解模型的输入输出关系,以及模型参数对结果的影响,这样才能更好地使用模型。
此外,很多人在使用无监督学习时也容易陷入维度灾难。
维度灾难指的是在高维空间中进行数据分析时会面临的挑战,比如数据稀疏性、计算复杂度等。
基于跨模态蒸馏的无监督行人重识别算法
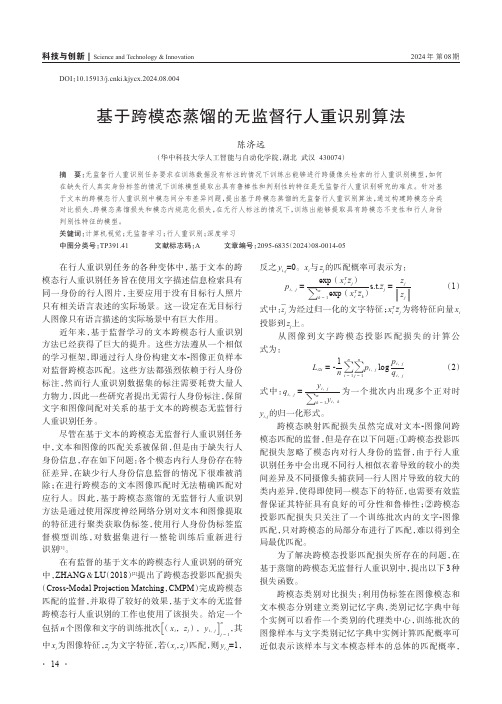
DOI :10.15913/ki.kjycx.2024.08.004基于跨模态蒸馏的无监督行人重识别算法陈济远(华中科技大学人工智能与自动化学院,湖北 武汉 430074)摘 要:无监督行人重识别任务要求在训练数据没有标注的情况下训练出能够进行跨摄像头检索的行人重识别模型,如何在缺失行人真实身份标签的情况下训练模型提取出具有鲁棒性和判别性的特征是无监督行人重识别研究的难点。
针对基于文本的跨模态行人重识别中模态间分布差异问题,提出基于跨模态蒸馏的无监督行人重识别算法,通过构建跨模态分类对比损失、跨模态蒸馏损失和模态内规范化损失,在无行人标注的情况下,训练出能够提取具有跨模态不变性和行人身份判别性特征的模型。
关键词:计算机视觉;无监督学习;行人重识别;深度学习中图分类号:TP391.41 文献标志码:A 文章编号:2095-6835(2024)08-0014-05在行人重识别任务的各种变体中,基于文本的跨模态行人重识别任务旨在使用文字描述信息检索具有同一身份的行人图片,主要应用于没有目标行人照片只有相关语言表述的实际场景。
这一设定在无目标行人图像只有语言描述的实际场景中有巨大作用。
近年来,基于监督学习的文本跨模态行人重识别方法已经获得了巨大的提升。
这些方法遵从一个相似的学习框架,即通过行人身份构建文本-图像正负样本对监督跨模态匹配。
这些方法都强烈依赖于行人身份标注,然而行人重识别数据集的标注需要耗费大量人力物力,因此一些研究者提出无需行人身份标注,保留文字和图像间配对关系的基于文本的跨模态无监督行人重识别任务。
尽管在基于文本的跨模态无监督行人重识别任务中,文本和图像的匹配关系被保留,但是由于缺失行人身份信息,存在如下问题:各个模态内行人身份存在特征差异,在缺少行人身份信息监督的情况下很难被消除;在进行跨模态的文本图像匹配时无法精确匹配对应行人。
因此,基于跨模态蒸馏的无监督行人重识别方法是通过使用深度神经网络分别对文本和图像提取的特征进行聚类获取伪标签,使用行人身份伪标签监督模型训练,对数据集进行一整轮训练后重新进行识别[1]。
航天遥感专业英语(中英文对照)
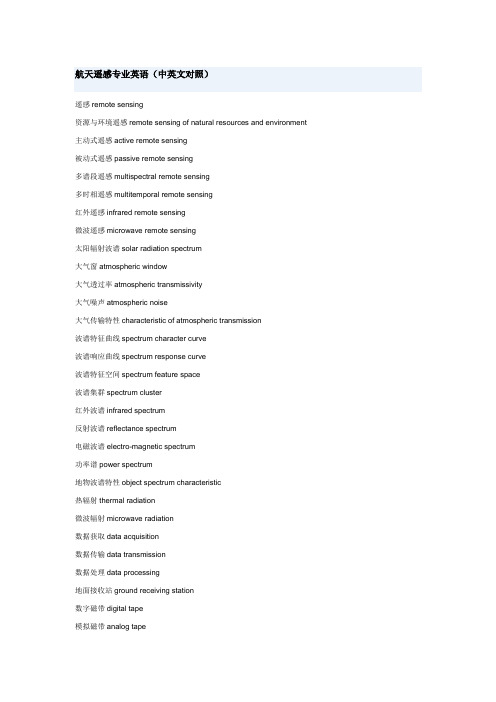
航天遥感专业英语(中英文对照)遥感remote sensing资源与环境遥感remote sensing of natural resources and environment 主动式遥感active remote sensing被动式遥感passive remote sensing多谱段遥感multispectral remote sensing多时相遥感multitemporal remote sensing红外遥感infrared remote sensing微波遥感microwave remote sensing太阳辐射波谱solar radiation spectrum大气窗atmospheric window大气透过率atmospheric transmissivity大气噪声atmospheric noise大气传输特性characteristic of atmospheric transmission波谱特征曲线spectrum character curve波谱响应曲线spectrum response curve波谱特征空间spectrum feature space波谱集群spectrum cluster红外波谱infrared spectrum反射波谱reflectance spectrum电磁波谱electro-magnetic spectrum功率谱power spectrum地物波谱特性object spectrum characteristic热辐射thermal radiation微波辐射microwave radiation数据获取data acquisition数据传输data transmission数据处理data processing地面接收站ground receiving station数字磁带digital tape模拟磁带analog tape计算机兼容磁带computer compatible tape,CCT高密度数字磁带high density digital tape,HDDT图象复原image restoration模糊影象fuzzy image卫星像片图satellite photo map红外图象infrared imagery热红外图象thermal infrared imagery,thermal IR imagery微波图象microwave imagery成象雷达imaging radar熵编码entropy coding冗余码redundant code冗余信息redundant information信息量contents of information信息提取information extraction月球轨道飞行器lunar orbiter空间实验室Spacelab航天飞机space shuttle陆地卫星Landsat海洋卫星Seasat测图卫星Mapsat立体卫星Stereosat礼炮号航天站Salyut space station联盟号宇宙飞船Soyuz spacecraftSPOT卫星SPOT satellite,systeme pro batoire d’observation de la terse(法)地球资源卫星earth resources technology satellite,ERTS环境探测卫星environmental survey satellite地球同步卫星geo-synchronous satellite太阳同步卫星sun-synchronous satellite卫星姿态satellite attitude遥感平台remote sensing platform主动式传感器active sensor被动式传感器passive sensor推扫式传感器push-broom sensor静态传感器static sensor动态传感器dynamic sensor光学传感器optical sensor微波传感器microwave remote sensor光电传感器photoelectric sensor辐射传感器radiation sensor星载传感器satellite-borne sensor机载传感器airborne sensor姿态测量传感器attitude-measuring sensor 探测器detector摄谱仪spectrograph航空摄谱仪aerial spectrograph波谱测定仪spectrometer地面摄谱仪terrestrial spectrograPh测距雷达range-only radar微波辐射计microwave radiometer红外辐射计infrared radiometer侧视雷达side-looking radar, SLR真实孔径雷达real-aperture radar合成孔径雷达synthetic aperture radar,SAR 专题测图传感器thematic mapper,TM 红外扫描仪infrared scanner多谱段扫描仪multispectral scanner.MSS 数字图象处理digital image processing光学图象处理optical image processing实时处理real-time processing地面实况ground truth几何校正geometric correction辐射校正radiometric correction数字滤波digital filtering图象几何配准geometric registration of imagery图象几何纠正geometric rectification of imagery 图象镶嵌image mosaic图象数字化image digitisation彩色合成仪additive colir viewer假彩色合成false color composite直接法纠正direct scheme of digital rectification间接法纠正indirect scheme of digital rectification 图象识别image recognition图象编码image coding彩色编码color coding多时相分析multitemporal analysis彩色坐标系color coordinate system图象分割image segmentation图象复合image overlaying图象描述image description二值图象binary image直方图均衡histogram equalization直方图规格化histogram specification图象变换image transformation彩色变换color transformation伪彩色pseudo-color假彩色false color主分量变换principal component transformation 阿达马变换Hadamard transformation沃尔什变换Walsh transformation比值变换ratio transformation生物量指标变换biomass index transformation 穗帽变换tesseled cap transformation参照数据reference data图象增强image enhancement边缘增强edge enhancement边缘检测edge detection反差增强contrast enhancement纹理增强texture enhancement比例增强ratio enhancement纹理分析texture analysis彩色增强color enhancement模式识别pattern recognition特征feature特征提取feature extraction特征选择feature selection特征编码feature coding距离判决函数distance decision function概率判决函数probability decision function模式分析pattern analysis分类器classifier监督分类supervised classification非监督分类unsupervised classification盒式分类法box classifier method模糊分类法fuzzy classifier method最大似然分类maximum likelihood classification 最小距离分类minimum distance classification 贝叶斯分类Bayesian classification机助分类computer-assisted classification 图象分析image analysis。
基于地震资料有效信息约束的深度网络无监督噪声压制方法

第49卷第1期2021年2月Vol. 49 No.l Feb. 2021煤田地质与勘探COAL GEOLOGY & EXPLORATION 移动阅读陈文超,刘达伟,魏新建,等.基于地震资料有效信息约束的深度网络无监督噪声压制方法[J].煤田地质与勘探,2021, 49(1): 249-256. doi : 10.3969/j.issn. 1001-1986.2021.01.027CHEN Wenchao , LIU Dawei , WEI Xinjian , et al. Unsupervised noise suppression method for depth network seismicdata based on prior information constraintfJ]. Coal Geology & Exploration , 2021, 49(1): 249-256. doi: 10.3969/j.issn.1001-1986.2021.01.027基于地震资料有效信息约束的深度网络无监督噪声压制方法陈文超1,刘达伟1,魏新建2,王晓凯1,陈德武彳,李书平2,李冬2(1.西安交通大学信息与通信工程学院,陕西西安710049;2.中国石油勘探开发研究院西北分院,甘肃兰州730020)摘要:地震资料处理是地震勘探中的关键环节,由于地下构造和地表条件的复杂性,地震资料的处理需要经过一系列复杂流程,从而形成多种不同类型的地震数据。
不同种类的地震数据具有不同的 数据特征,充分利用和发掘其中的数据特征,不仅可以充分发挥处理方法的技术潜力,消除各类非地质因素对地震资料处理质量的影响,同时可以增强地震资料处理的可靠性,改善地震资料的资料 信噪比及分辨率,在复杂油气藏勘探开发中具有非常重要的基础作用。
叠前地震成像道集(CRP)中的有效信号同相轴近似水平,叠后地震成像数据因为地层沉积的规律性,有效信号相比于随机噪声、成像画弧噪声等干扰具有规律、简单等特点。
基于GAN的无监督域自适应行人重识别
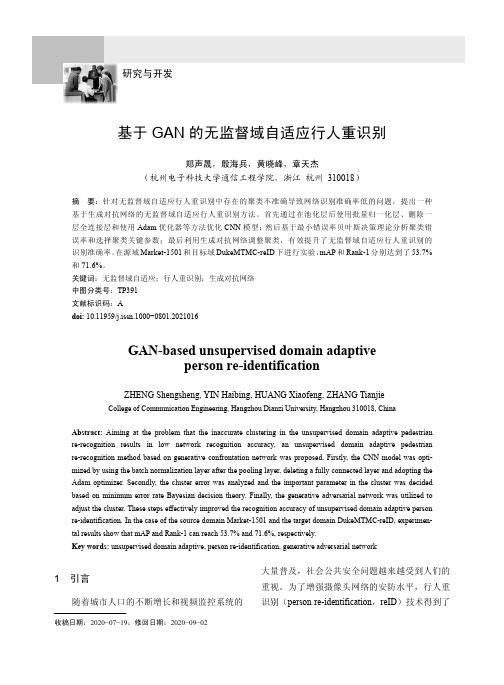
研究与开发基于GAN的无监督域自适应行人重识别郑声晟,殷海兵,黄晓峰,章天杰(杭州电子科技大学通信工程学院,浙江杭州 310018)摘 要:针对无监督域自适应行人重识别中存在的聚类不准确导致网络识别准确率低的问题,提出一种基于生成对抗网络的无监督域自适应行人重识别方法。
首先通过在池化层后使用批量归一化层、删除一层全连接层和使用Adam优化器等方法优化CNN模型;然后基于最小错误率贝叶斯决策理论分析聚类错误率和选择聚类关键参数;最后利用生成对抗网络调整聚类,有效提升了无监督域自适应行人重识别的识别准确率。
在源域Market-1501和目标域DukeMTMC-reID下进行实验,mAP和Rank-1分别达到了53.7%和71.6%。
关键词:无监督域自适应;行人重识别;生成对抗网络中图分类号:TP391文献标识码:Adoi: 10.11959/j.issn.1000−0801.2021016GAN-based unsupervised domain adaptiveperson re-identificationZHENG Shengsheng, YIN Haibing, HUANG Xiaofeng, ZHANG Tianjie College of Communication Engineering, Hangzhou Dianzi University, Hangzhou 310018, ChinaAbstract: Aiming at the problem that the inaccurate clustering in the unsupervised domain adaptive pedestrian re-recognition results in low network recognition accuracy, an unsupervised domain adaptive pedestrian re-recognition method based on generative confrontation network was proposed. Firstly, the CNN model was opti-mized by using the batch normalization layer after the pooling layer, deleting a fully connected layer and adopting the Adam optimizer. Secondly, the cluster error was analyzed and the important parameter in the cluster was decided based on minimum error rate Bayesian decision theory. Finally, the generative adversarial network was utilized to adjust the cluster. These steps effectively improved the recognition accuracy of unsupervised domain adaptive person re-identification. In the case of the source domain Market-1501 and the target domain DukeMTMC-reID, experimen-tal results show that mAP and Rank-1 can reach 53.7% and 71.6%, respectively.Key words: unsupervised domain adaptive, person re-identification, generative adversarial network1 引言随着城市人口的不断增长和视频监控系统的大量普及,社会公共安全问题越来越受到人们的重视。
如何成为一名优秀的志愿者英文作文
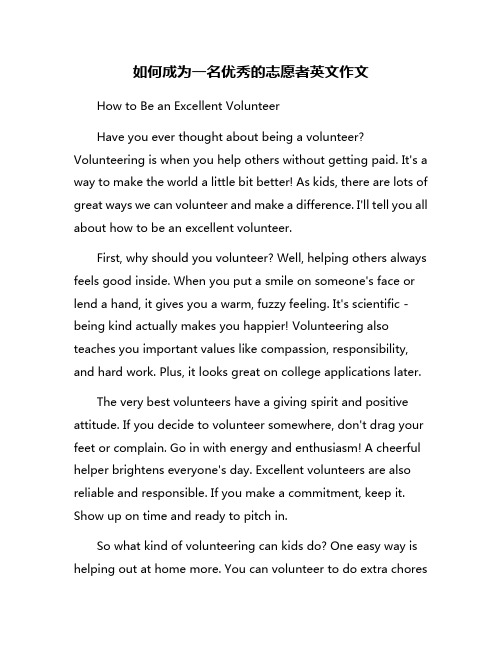
如何成为一名优秀的志愿者英文作文How to Be an Excellent VolunteerHave you ever thought about being a volunteer? Volunteering is when you help others without getting paid. It's a way to make the world a little bit better! As kids, there are lots of great ways we can volunteer and make a difference. I'll tell you all about how to be an excellent volunteer.First, why should you volunteer? Well, helping others always feels good inside. When you put a smile on someone's face or lend a hand, it gives you a warm, fuzzy feeling. It's scientific - being kind actually makes you happier! Volunteering also teaches you important values like compassion, responsibility, and hard work. Plus, it looks great on college applications later.The very best volunteers have a giving spirit and positive attitude. If you decide to volunteer somewhere, don't drag your feet or complain. Go in with energy and enthusiasm! A cheerful helper brightens everyone's day. Excellent volunteers are also reliable and responsible. If you make a commitment, keep it. Show up on time and ready to pitch in.So what kind of volunteering can kids do? One easy way is helping out at home more. You can volunteer to do extra choresaround the house, like taking out the trash or doing dishes. This gives your parents a break and helps out the family. Or make a home-cooked meal to bring to an elderly neighbor. The possibilities are endless!At school, there are tons of ways to volunteer too. You can be a classroom helper, collecting papers or running errands for the teacher. Or join the safety patrol to assist kids at drop-off and pick-up times. Some schools have a recycling team that collects cans and bottles. If your school has a garden, pitch in by weeding, watering plants, or harvesting vegetables.In the community, look for opportunities like park clean-ups, food drives, or beach restoration projects. Animal shelters usually need kid volunteers to feed pets, groom them, or just play and socialize. Hospitals and nursing homes really appreciate when youth come read stories and play games with patients. Get creative about using your unique talents!Volunteering teaches you discipline and commitment. If you sign up for a recurring volunteer role, make sure you can stick with it long-term. The organizers are counting on you. Show up every scheduled day with a positive mindset and a readiness to work hard. Come prepared with any supplies needed. Pay attention, follow instructions, and give it your best effort.Being an excellent volunteer also means being safe and responsible. Don't try to lift anything too heavy, use sharp tools unsupervised, or go into any unsafe situations. Always listen to the adult leaders. And of course, be polite and respectful at all times - say please and thank you, don't goof around, and avoid bothering others with loud voices or rude behavior.Finally, excellent volunteers make the most of their experience by staying curious. Ask questions if you're not sure about something. Try to learn new skills, like gardening, organizing a clothes drive, or using tools properly. Observe role models in action and aim to develop those qualities yourself, like diligence, resourcefulness, or patience. See if there are ways to take on more responsibilities over time. The goal is to soak up every lesson!Volunteering allows you to explore different fields and discover new interests. Maybe you'll find a calling in social services, education, environmentalism, or something totally unexpected. Or maybe not - but at least you'll gain exposure and build a variety of capabilities that'll help in the future. Who knows, you may even get inspired to start your own community initiative someday!I encourage you to get out there and start volunteering pronto. Ask around to find opportunities through your school, place of worship, clubs, or neighborhood organizations. With an eager and diligent spirit, you can make a positive impact and develop into an excellent volunteer. It feels wonderful helping others and contributing to great causes. Plus, it's awesome practice for building leadership skills for life. So what are you waiting for? There's a world of good to do out there! Let's get volunteering.。
unsupervised-domain-adaptation训练时候的损失函数
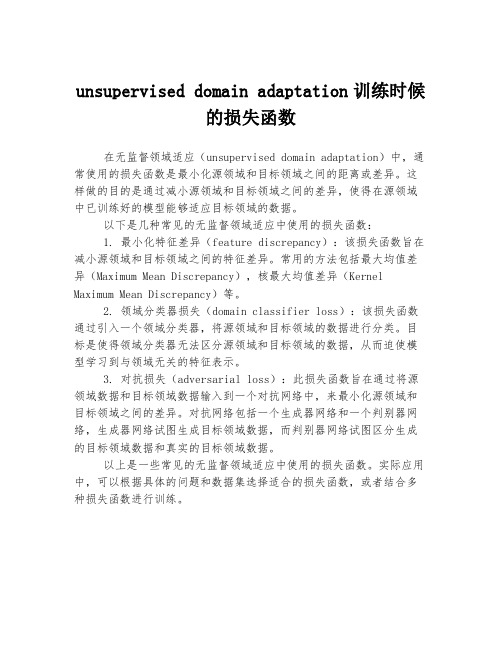
unsupervised domain adaptation训练时候
的损失函数
在无监督领域适应(unsupervised domain adaptation)中,通常使用的损失函数是最小化源领域和目标领域之间的距离或差异。
这样做的目的是通过减小源领域和目标领域之间的差异,使得在源领域中已训练好的模型能够适应目标领域的数据。
以下是几种常见的无监督领域适应中使用的损失函数:
1. 最小化特征差异(feature discrepancy):该损失函数旨在减小源领域和目标领域之间的特征差异。
常用的方法包括最大均值差异(Maximum Mean Discrepancy),核最大均值差异(Kernel Maximum Mean Discrepancy)等。
2. 领域分类器损失(domain classifier loss):该损失函数通过引入一个领域分类器,将源领域和目标领域的数据进行分类。
目标是使得领域分类器无法区分源领域和目标领域的数据,从而迫使模型学习到与领域无关的特征表示。
3. 对抗损失(adversarial loss):此损失函数旨在通过将源领域数据和目标领域数据输入到一个对抗网络中,来最小化源领域和目标领域之间的差异。
对抗网络包括一个生成器网络和一个判别器网络,生成器网络试图生成目标领域数据,而判别器网络试图区分生成的目标领域数据和真实的目标领域数据。
以上是一些常见的无监督领域适应中使用的损失函数。
实际应用中,可以根据具体的问题和数据集选择适合的损失函数,或者结合多种损失函数进行训练。
- 1、下载文档前请自行甄别文档内容的完整性,平台不提供额外的编辑、内容补充、找答案等附加服务。
- 2、"仅部分预览"的文档,不可在线预览部分如存在完整性等问题,可反馈申请退款(可完整预览的文档不适用该条件!)。
- 3、如文档侵犯您的权益,请联系客服反馈,我们会尽快为您处理(人工客服工作时间:9:00-18:30)。
1. Introduction
The aim of this paper is to propose original techniques of hidden signal restorations that use simultaneously copulas and hidden Markov chains. Let Y ¼ ðY 1; . . . ; Y mÞ be a random vector taking its values in Rm. The theory of copulas
2. Hidden and pairwise Markov chains
Received 24 June 2004; received in revised form 14 January 2005 Available online 10 May 2005
Abstract
This paper deals with the statistical restoration of hidden discrete signals, extending the classical methodology based on hidden Markov chains. The aim is to take into account the hidden signal and complex relationships between the noises which can be from different parametric models, non-independent, and of class-varying nature. We discuss some possibilities of managing it using copulas. Further, we propose a parameter estimation method and apply resulting unsupervised restoration methods in variety of situations. It is also validated by experiments performed in supervised and unsupervised context. r 2005 Elsevier B.V. All rights reserved.
allows one to define the distribution pðyÞ from given marginal distributions pðy1Þ, y, pðymÞ and dependence structure [1]. Firstly introduced by Sklar [2], this theory is widely used in various fields like linguistics [3], biology [4], decision sciences [5], finance and insuring [6], or still psychology [7], among others. We only mentioned recent papers, each of which contain numerous references for these different fields of research. In a more theoretical context, copulas can also be useful to measure the dependence among random variables [2,8,38], and to construct a general model for
2305
stationary Markov process, with non-linear dependence, as it is shown in [9,37], or in [10] for financial applications.
Otherwise, the hidden Markov chains model allows one to recover a hidden random vector X ¼ ðX 1; . . . ; X nÞ from an observed one Y ¼ ðY 1; . . . ; Y nÞ, even for a very large n. Since the seminal papers [11,12], hundreds of papers described different applications in various domains like image processing [13–15], biology [16], finance [17], among others. However, apart [18] there are no results, to our knowledge, concerning a simultaneous use of copulas and hidden Markov chains. Extending the first results presented in [18], the aim of this paper is to describe some possibilities of such a simultaneous use. In particular, the latter allows one to consider complex noise such that (i) its nature can vary with the class, and (ii) it is not independent conditionally on the hidden states. Indeed, instead of assuming that the observed process is the simplest stationary process with fixed marginals conditionally on the state process, we use recent advances in stationary Markov chain modeling based on copulas for specifying processes with prescribed margins. The first point (i) can be seen as a generalization of ideas in [15], where the noise follows the usual assumption of conditional independence of the classical hidden Markov chain model. Concerning the second point (ii), the approach presented here is a different one, and can be seen as complementary, to the method studied in [19]. The main difference is that the present method does respect the given marginal distributions of the noise, when the previous method does not. We also present some numerical results which attest the interest of the new models, with potential application to the different domains mentioned above. The practical applications are made possible with an original parameter estimation method we propose, based on the general Expectation-Maximization (EM) principle. Finally, some possible extensions of the proposed models and techniques to pairwise and triplet Markov chains [20,21], which generalize hidden Markov chains, are also mentioned.
ARTICLE IN PRESS
Signal Processing 85 (2005) 2304–2315
/locate/sigpro
Unsupervised signal restoration using hidden Markov chains with copulas
The paper is organized as follows. Hidden and pairwise Markov chains are recalled in Section 2
and the new models using copulas are described in Section 3. Section 4 is devoted to the parameter estimation problem. Experiments are described in Section 5 and the Section 6 contains some concluding remarks and perspectives.
Keywords: Hidden Markov chains; Pairwise Markov chains; Triplet Markov chains; Copulas; Parameter estimation; Bayesian restoration; Stochastic expectation-maximization; Statistical image segmentation