Abstract Dynamical Equilibrium of Interacting Ant Societies
数字示波器外文翻译文献

数字示波器外文翻译文献(文档含中英文对照即英文原文和中文翻译)原文:Design and FPGA implementation of a wireless hyperchaotic communication system for secure real-time image transmission AbstractIn this paper, we propose and demonstrate experimentally a new wireless digital encryption hyperchaotic communication system based on radio frequency (RF) communication protocols for secure real-time data or image transmission. A reconfigurable hardware architecture is developed to ensure the interconnection between two field programmable gate array developmentplatforms through XBee RF modules. To ensure the synchronization and encryption of data between the transmitter and the receiver, a feedback masking hyperchaotic synchronization technique based on a dynamic feedback modulation has been implemented to digitally synchronize the encrypter hyperchaotic systems. The obtained experimental results show the relevance of the idea of combining XBee (Zigbee or Wireless Fidelity) protocol, known for its high noise immunity, to secure hyperchaotic communications. In fact, we have recovered the information data or image correctly after real-time encrypted data or image transmission tests at a maximum distance (indoor range) of more than 30 m and with maximum digital modulation rate of 625,000 baud allowing a wireless encrypted video transmission rate of 25 images per second with a spatial resolution of 128 ×128 pixels. The obtained performance of the communication system is suitable for secure data or image transmissions in wireless sensor networks.IntroductionOver the past decades, the confidentiality of multimedia communications such as audio, images, and video has become increasingly important since communications of digital products over the network (wired/wireless) occur more frequently. Therefore, the need for secure data and transmission is increasing dramatically and defined by the required levels of security depending on the purpose of communication. To meet these requirements, a wide variety of cryptographic algorithms have been proposed. In this context, the main challenge of stream cipher cryptography relates to the generation of long unpredictable key sequences. More precisely, the sequence has to be random, its period must be large, and the various patterns of a given length must be uniformly distributed over the sequence. Traditional ciphers like DES, 3DES, IDEA, RSA, or AES are less efficient for real-time secure multimedia data encryption systems and exhibit some drawbacks and weakness in the high streamdata encryption. Indeed, the increase and availability of a high-power computation machine allow a force brute attack against these ciphers. Moreover, for some applications which require a high-levelcomputation and where a large computational time and high computing power are needed (for example, encryption of large digital images), these cryptosystems suffer from low-level efficiency. Consequently, these encryption schemes are not suitable for many high-speed applications due to their slow speed in real-time processing and some other issues such as in the handling of various data formatting. Over the recent years, considerable researches have been taken to develop new chaotic or hyperchaotic systems and for their promising applications in real-time encryption and communication. In fact, it has been shown that chaotic systems are good candidates for designing cryptosystems with desired properties. The most prominent is sensitivity dependence on initial conditions and system parameters, and unpredictable trajectories.Furthermore, chaos-based and other dynamical systembased algorithms have many important properties such as the pseudorandom properties, ergodicity and nonperiodicity. These properties meet some requirements such as sensitivity to keys, diffusion, and mixing in the cryptographic context. Therefore, chaotic dynamics is expected to provide a fast and easy way for building superior performance cryptosystems, and the properties of chaotic maps such as sensitivity to initial conditions and random-like behavior have attracted the attention to develop data encryption algorithms suitable for secure multimedia communications. Until recently, chaotic communication has been a subject of major interest in the field of wireless communications. Many techniques based on chaos have been proposed such as additive chaos masking (ACM), where the analog message signal is added to the output of the chaos generator within the transmitter. In, chaos shift keying is used where the binary message signal selects the carrier signal from two or more different chaotic attractors. Authors use chaotic modulation where the message information modulates a parameter of the chaotic generator. Chaos control methods rely on the fact that small perturbations cause the symbolic dynamics of a chaotic system to track a prescribed symbol sequence. In, the receiver system is designed in an inverse manner to ensure the recovery of theencryption signal. An impulsive synchronization scheme is employed to synchronize chaotic transmitters and receivers. However, all of these techniques do not provide a real and practical solution to the challenging issue of chaotic communication which is based on extreme sensitivity of chaotic synchronization to both the additive channel noise and parameter mismatches. Precisely, since chaos is sensitive to small variations of its initial conditions and parameters, it is very difficult to synchronize two chaotic systems in a communication scheme. Some proposed synchronization techniques have improved the robustness to parameter mismatches as reported in, where impulsive chaotic synchronization and an open-loop-closed-loopbased coupling scheme are proposed, respectively. Other authors proposed to improve the robustness of chaotic synchronization to channel noise, where a coupled lattice instead of coupled single maps is used to decrease the master-slave synchronization error. In, symbolic dynamics-based noise reduction and coding are proposed. Some research into equalization algorithms for chaotic communication systems are also proposed. For other related results in the literature, see. However, none of them were tested through a real channel under real transmission conditions. Digital synchronization can overcome the failed attempts to realize experimentally a performed chaotic communication system. In particular, when techniques exhibit any difference between the master/transmitter and slave/receiver systems, it is due to additive information or noise channel (disturbed chaotic dynamics) which breaks the symmetry between the two systems, leading to an accurate non-recovery of the transmitted information signal at the receiver. In, an original solution to the hard problem of chaotic synchronization high sensibility to channel noise has been proposed. This solution, based on a controlled digital regenerated chaotic signal at the receiver, has been tested and validated experimentally in a real channel noise environment through a realized wireless digital chaotic communication system based on zonal intercommunication global-standard, where battery life was long, which was economical to deploy and which exhibited efficient use of resources, knownas the ZigBee protocol. However, this synchronization technique becomes sensible to high channel noise from a higher transmission rate of 115 kbps, limiting the use of the ZigBee and Wireless Fidelity (Wi-Fi) protocols which permit wireless transmissions up to 250 kbps and 65 Mbps, respectively.Consequently, no reliable commercial chaos-based communication system is used to date to the best of our knowledge. Therefore, there are still plentiful issues to be resolved before chaos-based systems can be put into practical use. To overcome these drawbacks, we propose in this paper a digital feedback hyperchaotic synchronization and suggest the use of advanced wireless communication technologies, characterized by high noise immunity, to exploit digital hyperchaotic modulation advantages for robust secure data transmissions. In this context, as results of the rapid growth of communication technologies, in terms of reliability and resistance to channel noise, an interesting communication protocol for wireless personal area networks (WPANs, i.e., ZigBee or ZigBee Pro Low-Rate-WPAN protocols) and wireless local area network (WLAN, i.e., Wi-Fi protocol WLAN) is developed. These protocols are identified by the IEEE 802.15.4 and IEEE 802.11 standards and known under the name ZigBee and Wi-Fi communication protocols, respectively. These protocols are designed to communicate data through hostile Radio Frequency (RF) environments and to provide an easy-to-use wireless data solution characterized by secure, low-power, and reliable wireless network architectures. These properties are very attractive for resolving the problems of chaotic communications especially the high noise immunity property. Hence, our idea is to associate chaotic communication with theWLAN or WPAN communication protocols. However, this association needs a numerical generation of the chaotic behavior since the XBee protocol is based on digital communications.In the hardware area, advanced modern digital signal processing devices, such as field programmable gate array (FPGA), have been widely used to generate numerically the chaotic dynamics or the encryption keys. The advantage of these techniques is that the parameter mismatch problem does not existcontrary to the analog techniques. In addition, they offer a large possible integration of chaotic systems in the most recent digital communication technologies such as the ZigBee communication protocol. In this paper, a wireless hyperchaotic communication system based on dynamic feedback modulation and RF XBee protocols is investigated and realized experimentally. The transmitter and the receiver are implemented separately on two Xilinx Virtex-II Pro circuits and connected with the XBee RF module based on the Wi-Fi or ZigBee protocols. To ensure and maintain this connection, we have developed a VHSIC (very high speed integrated circuit) hardware description language (VHDL)-based hardware architecture to adapt the implemented hyperchaotic generators, at the transmitter and receiver, to the XBee communication protocol. Note that the XBee modules interface to a host device through a logic-level asynchronous serial port. Through its serial port, the module can communicate with any logic and voltage-compatible Universal Asynchronous Receiver/Transmitter (UART). The used hyperchaotic generator is the well-known and the most investigated hyperchaotic Lorenz system. This hyperchaotic key generator is implemented on FPGA technology using an extension of the technique developed in for three-dimensional (3D) chaotic systems. This technique is optimal since it uses directly VHDL description of a numerical resolution method of continuous chaotic system models. A number of transmission tests are carried out for different distances between the transmitter and receiver. The real-time results obtained validate the proposed hardware architecture. Furthermore, it demonstrates the efficiency of the proposed solution consisting on the association of wireless protocols to hyperchaotic modulation in order to build a reliable digital encrypted data or image hyperchaotic communication system.Hyperchaotic synchronization and encryption techniqueContrary to a trigger-based slave/receiver chaotic synchronization by the transmitted chaotic masking signal, which limits the performance of the rate synchronization transmission, we propose a digital feedback hyperchaoticsynchronization (FHS). More precisely, we investigate a new scheme for the secured transmission of information based on master-slave synchronization of hyperchaotic systems, using unknown input observers. The proposed digital communication system is based on the FHS through a dynamic feedback modulation (DFM) technique between two Lorenz hyperchaotic generators. This technique is an extension and improvement of the one developed in for synchronizing two 3D continuous chaotic systems in the case of a wired connection.The proposed digital feedback communication scheme synchronizes the master/transmitter and the slave/receiver by the injection of the transmitted masking signal in the hyperchaotic dynamics of the slave/receiver. The basic idea of the FHS is to transmit a hyperchaotic drive signal S(t) after additive masking with a hyperchaotic signal x(t) of the master (transmitter) system (x , y , z ,w ). Hyperchaotic drive signal is then injected both in the three subsystems (y , z ,w ) and (r r r w z y ,,). The subscript r represents the slave or receiver system (r r r r w z y x ,,,). At the receiver, the slave system regenerates the chaotic signal )(t x r and a synchronization is obtained between two trajectories x(t) and )(t x r if()()0||lim =-∞→t X t X r t (1) This technique can be applied to chaotic modulation. In our case, it is used for generating hyperchaotic keys for stream cipher communications, where the synchronization between the encrypter and the decrypter is very important. Therefore, at the transmitter, the transmitted signal after the additive hyperchaos masking (digital modulation) isS(t) = x(t) + d(t). (2)where d(t) is the information signal and x(t) is the hyperchaotic carrier. At the receiver, after synchronization of the regenerated hyperchaotic signal )(t x rwith the received signal )(t S r and the demodulation operation, we can recover the information signal d(t) correctly as follows:)()()(t x t S t d r r -=. (3)Therefore, the slave/receiver will generate a hyperchaotic behavior identical to that of the master/transmitter allowing to recover correctly the information signal after the demodulation operation. The advantageof this technique is that the information signal d(t) doesnot perturb the hyperchaotic generator dynamics, contraryto the ACM-based techniques of and, because d(t) is injected at both the master/transmitter and slave/receiver after the additive hyperchaotic masking. Thus, for small values of information magnitude, the information will be recovered correctly. It should be noted that we have already confirmed this advantage by testing experimentally the HS-DFM technique performances for synchronizing hyperchaotic systems (four-dimensional (4D) continuous chaotic systems) in the case of wired connection between two Virtex-II Pro development platforms. After many experimental tests and from the obtained real-time results, we concluded that the HS-DFM is very suitable for wired digital chaotic communication systems. However, in the present work, one of the objectives is to test and study the performances of the HS-DFM technique in the presence of channel noise through real-time wireless communication tests. To performthe proposed approach, a digital implementation of the master and slave hyperchaotic systems is required. Therefore, we investigate the hardware implementation of the proposed FHS-DFM technique between two Lorenz hyperchaotic generators using FPGA. To achieve this objective, we propose the following details of the proposed architecture.译文:无线超混沌通信系统安全的实时图像传输的设计和FPGA实现摘要在本文中,我们提出并论证了一种基于无线电频率通信协议对数据或图像安全实时传输的新的无线数字超混沌加密通信系统。
Statistical Mechanics of thermal denaturation of DNA oligomers
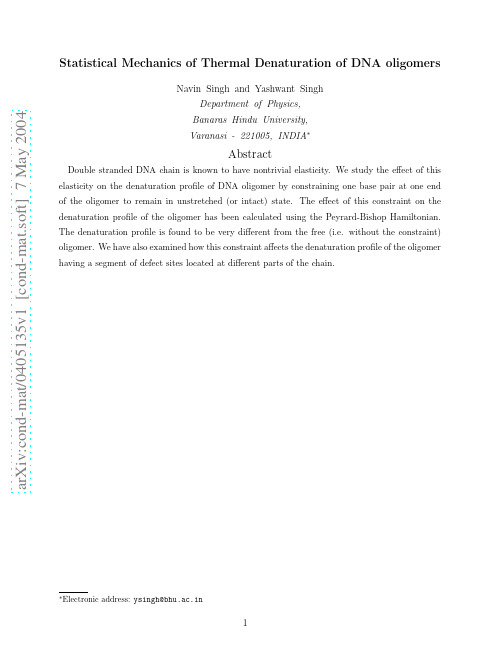
a r X i v :c o n d -m a t /0405135v 1 [c o n d -m a t .s o f t ] 7 M a y 2004Statistical Mechanics of Thermal Denaturation of DNA oligomersNavin Singh and Yashwant SinghDepartment of Physics,Banaras Hindu University,Varanasi -221005,INDIA ∗Abstract Double stranded DNA chain is known to have nontrivial elasticity.We study the effect of this elasticity on the denaturation profile of DNA oligomer by constraining one base pair at one end of the oligomer to remain in unstretched (or intact)state.The effect of this constraint on the denaturation profile of the oligomer has been calculated using the Peyrard-Bishop Hamiltonian.The denaturation profile is found to be very different from the free (i.e.without the constraint)oligomer.We have also examined how this constraint affects the denaturation profile of the oligomer having a segment of defect sites located at different parts of the chain.I.INTRODUCTIONDNA is one of the most complex and important biomolecule as it is central to all living beings.It contains all the informations needed for birth,development,living and probably sets the average life.Structurally it is a giant double stranded linear molecule with length ranging from2µm for simple viruses to3.5×107µm for more complex organism[1].How such a molecule came into being during the evolution of life and how it acquired the ability to store and transmit the genetic informations are still a mystery.The recent progress in genome mapping and availability of experimental techniques to study the physical properties of a single molecule[2,3]has,however,made thefield very active from both biological and physical point of views.A DNA molecule is not just a static object but a dynamical system having rather complex nature of internal motions[4].The structural elements such as individual atoms,group of atoms(bases,sugar rings,phosphates)fragments of double chain including several base pairs, are in constant movement and this movement plays an important role in the functioning of the molecule.The solvent in which the molecule is immersed acts as a thermal bath and provides energy for different motion.In addition,collision with the molecules of the solution which surrounds DNA,local interactions with proteins,drugs or with some other ligands also lead to internal motion.These motions are distinguished by activation energies,amplitudes and characteristic times.The motions which are of our interest here are opening of base pairs,formation of bubbles along the chain and unwinding of helix(denaturation).The energy involved for these motions is of the order of5-20kcal/mole.These motions are activated by increasing temperature,increasing pH of the solvent action of denaturation agents etc.The time scale of these motions are of the order of microseconds and is therefore generally unobservable in atomistic simulations as these simulations are restricted to time scale of nanosecond because of computational cost.The nature of thermal denaturation leading to separation of two strands has been investi-gated for several decades[5,6].Experimentally a sample containing molecules of a specific length and sequence is prepared.Then the fraction of bound base pairs as a function of temperature,referred to as the melting curve,is measured through light absorption,typ-ically at about260nm.For heterogeneous DNA,where the sequence contains both AT and GC pairs,the melting curve exhibits a multistep behaviour consisting of plateaus with different sizes separated by sharp jumps.These jumps have been attributed to the unwind-ing of domains characterized by different frequencies of AT and GC pairs.The sharpness of the jump in long DNA molecules suggests that the transition from bound to unbound isfirst-order.The understanding of this remarkable one-dimensional cooperative phenom-ena in terms of standard statistical mechanics,i.e.,a Hamiltonian model with temperatureindependent parameters is a subject of current interest[7].II.MODEL HAMILTONIAN AND ITS PROPERTIESSince the internal motion that is basically responsible for denaturation is the stretching of bases from their equilibrium position along the direction of the hydrogen bonds that connect the two bases,a DNA molecule can be considered as a quasi one dimensional lattice composed of N base pair units.The forces which stabilize the structure are the hydrogen bonds between complementary bases on opposite strand and stacking interactions between nearest neighbour bases on opposite strands.Each base pair is in one of the two states: either open(non hydrogen bonded)or intact(hydrogen bonded).A Hamiltonian model that has been found appropriate to include these interactions and describe the displacement of bases leading to denaturation is the Peyrard-Bishop model(PB model)[8].The PB modelis written asH=Ni=1H(y i,y i+1)H(y i,y i+1)=p2idtThe on-site potential V(y i)describes the interaction of the two bases of the i th pair.The Morse potentialV(y i)=D i(e−ay i−1)2(2.2) which is taken to represent the on-site interaction represents not only the hydrogen bonds connecting two bases belonging to opposite strands,but also the repulsive interactions of the phosphates and the surrounding solvent effects.Theflat part at large values of the displacement of this potential emulates the tendency of the pair”melt”at high temperatures as thermal phonons drive the molecules outside the well and towards theflat portion of the potential.The stacking interactions are contributed by dipole-dipole interactions,π-electron systems, London dispersion forces and in water solution,the hydrophobic interactions.These forces result in a complex interaction pattern between overlapping base pairs,with minimum energy distance close to3.4˚A in the normal DNA double helix.The following anharmonic potentialmodel mimic these features of the stacking energy:1W(y i,y i+1)=and the nearest neighbour interactions which lead to the confinement.The model,therefore, represents a one-dimensional system that differs from the usual one-dimensional systems that do not show phase transitions.The model Hamiltonian of Eq.(2.1)has extensively been used to study the melting profile of a very long(N→∞)homogeneous DNA chain using both statistical mechanical calcu-lations and constrained temperature molecular dynamics[9,10].Analytical investigation of nonlinear dynamics of the model suggests that intrinsic energy localization can initiate the denaturation[11].The model for a long homogeneous chain exhibits a peculiar type offirst-order transition withfinite melting entropy,a discontinuity in the fraction of bound pairs and divergent correlation lengths.However,as the value of the stacking parameterαincreases and the range of the”entropy barrier”becomes shorter than or comparable to the range of the Morse potential the transition changes to second order.The crossover is seen atα/a=0.5[7].Though the PB model seems capable of explaining the multistep melting in a sequence-specific disorder[12],how this disorder affect the nature of transition has yet to be understood.In other work the PB model has been used to understand the melting profile of short chains[13]and effect of defects on this profile[14].III.DENATURATION PROFILEIn a given system of DNA chains the average fractionθof bonded base pairs can be written asθ=θextθint.θext represents the average fraction of the strands forming duplexes(double strands),whileθint is the average fraction of unbroken bonds in the duplexes.The equilib-rium dissociation of the duplexes C2to single strand C1may be represented by the relation C2⇀↽2C1.The dissociation equilibrium can be neglected in the case of long chains asθext is practically1whileθint and thereforeθgoes to zero.This is because in the case of long DNA chains whenθgoes practically from1to zero near the denaturation transition,while most bonds are disrupted and the DNA has denatured,few bonds still remaining prevent the two strands getting apart from each other.It is only at T>>T m(T m being the melting temperature at which half of the bonds are broken)there will be real separation.Therefore at the transition the double strand is always a single molecule and in calculation based on PB model one has to calculate onlyθint(≡θ).On the contrary,in the case of short chains the processes of single bond disruption and strand dissociation tend to happen in the same temperature range,therefore,the computation ofθext in addition toθint is essential. Unfortunately,at present,we do not have any reliable method for calculatingθext.The method which has been used is based on the partition function of rigid molecules and ad-justable parameters[5,13]to be determined from experimental data.To avoid this shortcom-ing of the theory we in the present article discuss the denaturation profile of oligonucleotidesof given sequence with a base pair at one end of the chain held in such a way that it remains at its equilibrium seperation(i.e.there is no stretching)at all temperatures.This can be done by creating a deep potential well for this base pair or attaching the one end of both strand to a substrate.This will be referred to as chain with constraint in order to distinguish it form the”free chain”.One of the advantage of having a constraint of this type is obvious; the problem of divergence of the partition function for the PB model for short chains no more exists.The DNA molecule is known to have non trivial elastic properties:When the two strands of DNA molecule are pulled apart by applying a force at one end of the chain,a novel phase transition is found(in case of infinitely long chain)to take place at which the two strands are pulled completely apart[15].The phase digram plotted in the plane of temperature and force reveals the elastic properties of the DNA chain.Our study reported in this paper differs from the situation just described in two ways;(i)a short chain of21given base sequence is considered and(ii)instead of pulling the chain apart the end base pair is constrained to be in unstreched or intact position.The oligonucleotide which we consider has the following sequence given by:5′ACGCT AT ACT CACGT T AACAG3′′(3.1)3′T GCGAT AT GAGT GCAAT T GT C5The denaturation profile of this oligonucleotide has been studied by Campa and Giansanti [13]and by us[14].We take the same parameters as in the previous study.Thus,D AT=0.05 eV,D GC=0.075eV,a AT=4.2˚A−1,a GC=6.9˚A−1,k=0.025eV˚A−2,ρ=2andα= 0.35˚A−1.When one end of chain is heldfixed at y=0distance,the fraction of intact bonds is found using the relation1θ=200250300350400450500T(K)00.10.20.30.40.50.60.70.80.9θ200250300350400450500T(K)00.020.040.06d θ/dT(a)(b)FIG.2:Variation of θand dθ/dT as a function of temperature for free (solid line)and constrained chain(dashed line)in the constrained chain it is over a wide temperature range.The value of temperature at which dθ/dT is maximum shifts about 450C and the peak is much wider and smaller compared to that of free chain.Next we calculated the denaturation profile for the following two nucleotides having a seg-ment of chain with defect sites:(a )5′ACGCT AT ACT CACGT T AACAG 3′3′T CGCT AT ACT CT GCAAT T GT C 5′(3.3)(b )5′ACGCT AT ACT CACGT T AACAG 3′3′T GCGAT T ACT CACGT T T T GT C 5′(3.4)While both oligonucleotide have ten defect sites their locations differ.In (a)the defects are on the left end from site 2to 11while in (b)it is in middle from site 6to 16.The position of the base pair is counted from left.The purpose is to see how these defects affect the denaturation profile and the formation of loop and stem as often seen in a single strand DNA or RNA.In Fig.3we plot the variation of θand dθ/dT as a function of temperature.While the denaturation takes place at low temperature the shift is more when the defects are in the middle.The other quantities of interest are the mean value of displacement of n th base pairs defined200250300350400450500T(K)0.20.30.40.50.60.70.80.9θ200250300350400450500T(K)00.0020.0040.0060.008d θ/dT (a)(b)FIG.3:Fig.(a):Variation of θas a function of temperature for the constrained chain without defect (solid line)with 10defect at one end of the chain (dashed line)and 10defects in the middle(dot-dashed line).Fig.(b):Plot of dθ/dT for the same.asy n =1ZN i =1dy i (y n − y n )2exp[−βN i =1H (y i ,y i +1)](3.6)Here Z = N i =1dy i exp[−βN i =1H (y i ,y i +1)]is the partition function of the chain.Because of the constraint that the first base pair of the chain is in unstreched condition the value of y n as well as |δy n |2 depend on the site n .In Fig.4we plot the value of y n as a function of n at several temperatures for all three constrained chain as described above.We find in all cases the opening of the chain starts from the open end of the chain.In Fig.5we plot |δy n |2 as a function of temperature for several base pairs.The quantity |δy n |2 measures the transverse correlation length for the base pair n .This correlation length remains almost zero for all n when the oligonucleotide is in the native state but at the denaturation its value increases.At a given temperature the value of |δy n |2 depends on n and it increases with n .For a long chain we expect it to diverge for large value of n .In conclusion we wish to emphasize that the effect of constraining a base pair at one end of a given oligonucleotide has very significant effect on the denaturation profile.135791113151719012345613579111315171901234560246810121416182001234561357911131517190123456360 K 370 K 380 K 390 K FIG.4:Plot of y n vs.n (site position)at four different temperatures.Circles denotes the constrained chain without defect,square denotes the chain with 10defect at one end while diamond denotesthe chain with 10defect in the middle.The work has been supported through research grant by Department of Science and Tech-nology and Council of Scientific and Industrial Research,New Delhi,Government of India.200250300350400450500T(K)02468101214<δy n 2>n = 2n = 5n = 10n = 15n = 20FIG.5:Plot showing the variation of transverse correlation length with temperature for different value ofn .[1]L.Stryer,Biochemistry ,(W.H.Freeman and Company,New York 1995)[2]S.B.Smith,L.Finzi and C.Bustamante,Science 258,1122(1992);T.R.Strick et al ibid ,271,1835(1996)[3]U.Bockelmann,B.Essevaz-Roulet and F.Heslot,Phys.Rev.Lett.79,4489(1997)and refer-ences therein[4]L.V.Yakushevich,Non-linear Physics of DNA ,(John-Wiley &sons,1998)[5]R.M.Wartell and A.S.Benight,Phys.Rep.126,67(1985).[6]N.Theodorakopoulos,cond-mat/0210188and refernce therin[7]N.Theodorakopoulos,T.Dauxois and M.Peyrard,Phys.Rev.Lett.85,6(2000)[8]M.Peyrard and A.R.Bishop,Phys.Rev.Lett.62,2755(1989)[9]T.Dauxois and M.Peyrard,Phys.Rev.Lett.70,3935(1993)[10]T.Dauxois and M.Peyrard and A.R.Bishop,Phys.Rev.E 47,R44(1993)[11]T.Dauxois and M.Peyrard,Phys.Rev.E 51,4027(1995)[12] D.Cule and T.Hwa,Phys.Rev.Lett.79,2375(1997)[13] A.Campa and A.Giansanti,Phys.Rev.E 58,3585(1998)[14]Navin Singh and Yashwant Singh,Phys.Rev.E 64,42901(2001)[15]David K.Lubensky and David R.Nelson,Phys.Rev.Lett.85,1572(2000);S.M.Bhattachar-jee,J.Phys.A 33,L423(2000).。
equilibrium英文解释
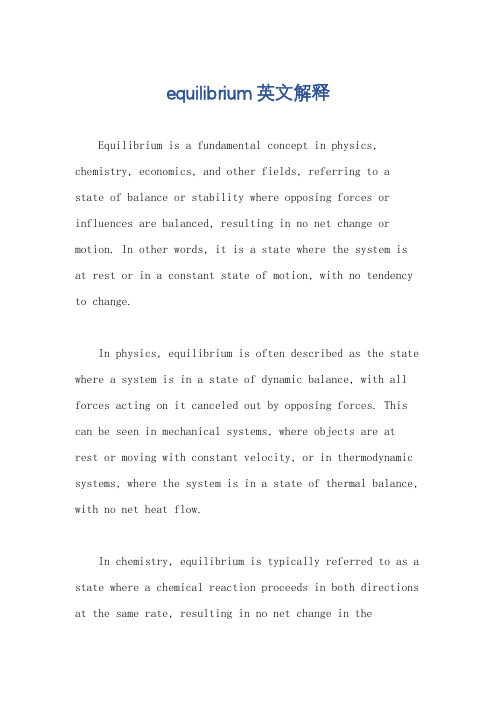
equilibrium英文解释Equilibrium is a fundamental concept in physics, chemistry, economics, and other fields, referring to a state of balance or stability where opposing forces or influences are balanced, resulting in no net change or motion. In other words, it is a state where the system is at rest or in a constant state of motion, with no tendency to change.In physics, equilibrium is often described as the state where a system is in a state of dynamic balance, with all forces acting on it canceled out by opposing forces. This can be seen in mechanical systems, where objects are atrest or moving with constant velocity, or in thermodynamic systems, where the system is in a state of thermal balance, with no net heat flow.In chemistry, equilibrium is typically referred to as a state where a chemical reaction proceeds in both directions at the same rate, resulting in no net change in theconcentrations of the reactants and products. This state is known as chemical equilibrium, and it is described by the law of mass action, which states that the rate of a chemical reaction is proportional to the product of the concentrations of the reactants.In economics, equilibrium is often described as a state where the supply and demand for a particular good or service are balanced, resulting in a stable price. This state is known as market equilibrium, and it is described by the law of supply and demand, which states that the price of a good or service will adjust to balance the quantity supplied with the quantity demanded.In all these fields, equilibrium is an important concept because it represents a state of stability and predictability. When a system is in equilibrium, it is generally easier to understand and predict its behavior than when it is in a state of flux or change. Additionally, equilibrium states often correspond to optimal or most efficient conditions, making them important targets for engineering, economic policy, and other applications.However, it is important to note that equilibrium is not always a static or unchanging state. In many systems, equilibrium is a dynamic state, with small fluctuations or perturbations constantly occurring. These fluctuations can be caused by external influences, internal fluctuations in the system, or random events. In these cases, the system will continually adjust to maintain the balance orstability of the equilibrium state.Additionally, it is worth noting that achieving or maintaining an equilibrium state can often be challenging. In many cases, it requires careful control or management of the system, as well as an understanding of the interactions and dependencies within the system. For example, in economic systems, maintaining market equilibrium often requires government intervention or regulation to prevent market failures or excesses. In physical systems, achieving equilibrium may require precise control of external conditions or the manipulation of system parameters.In conclusion, equilibrium is a fundamental conceptthat describes a state of balance or stability in various fields. It represents a state where opposing forces or influences are balanced, resulting in no net change or motion. While equilibrium may be seen as a static or unchanging state in some cases, it is often a dynamic state that requires careful management and control to maintain. Understanding and manipulating equilibrium states iscrucial in many fields, including physics, chemistry, economics, and engineering, and has important applications in real-world scenarios.。
An Economist's Perspective on Probability Matching 1 by
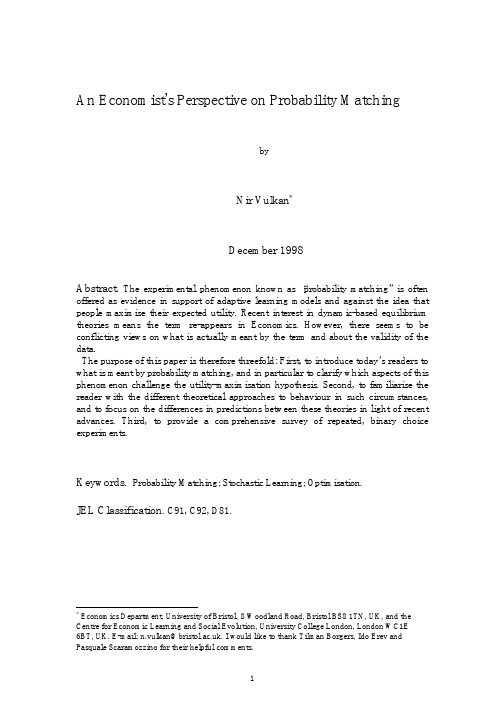
An Economist's Perspective on Probability MatchingbyNir Vulkan*December 1998Abstract. The experimental phenomenon known as “probability matching”is often offered as evidence in support of adaptive learning models and against the idea that people maximise their expected utility. Recent interest in dynamic-based equilibrium theories means the term re-appears in Economics. However, there seems to be conflicting views on what is actually meant by the term and about the validity of the data.The purpose of this paper is therefore threefold: First, to introduce today’s readers to what is meant by probability matching, and in particular to clarify which aspects of this phenomenon challenge the utility-maximisation hypothesis. Second, to familiarise the reader with the different theoretical approaches to behaviour in such circumstances, and to focus on the differences in predictions between these theories in light of recent advances. Third, to provide a comprehensive survey of repeated, binary choice experiments.Keywords. Probability Matching; Stochastic Learning; Optimisation.JEL Classification. C91, C92, D81.* Economics Department, University of Bristol, 8 Woodland Road, Bristol BS8 1TN, UK, and the Centre for Economic Learning and Social Evolution, University College London, London WC1E6BT, UK. E-mail: n.vulkan@. I would like to thank Tilman Borgers, Ido Erev and Pasquale Scaramozzino for their helpful comments.1IntroductionDo people make choices that maximise their expected utility? By and large, economists believe that they do, especially in those cases where the underlying decision situation is simple and is repeated often enough. Somehow people learn how to choose optimally. In introductory courses we teach our students that equilibrium is reached by a process of bayesian-style belief updating, or a process of imitation, or reinforcement-type learning, or even by the replicator dynamics. However, with the notable exception of Cross (1973), it is only recently that economists have started to study seriously these models and have attempted to explain behaviour in terms of the underlying dynamic process which may, or may not, lead to equilibrium. Experimental game theory, behavioural economics, and evolutionary economics all focus on the learning process and on the effects it might have on behaviour in the steady state. An explosion of models in which an individual learner is faced with an uncertain environment had recently been developed (e.g. McKelvey & Palfrey (1995), Fudenberg & Levine (1997), Erev & Roth (1997), Roth & Erev (1995), Camerer & Ho (1996), Chen & Tang (1996), and Tang (1996)). A one-player decision problem with a move of Nature often provides a simple case where the basics of these learning models can be expressed and tested. In this paper I restrict attention to this seemingly simple case.Naturally, the study of human learning falls within the scope of another social science, psychology. Furthermore, mathematical learning theories date back to the early 1950s (starting with Estes’seminal 1950 paper), when, for about two decades, they have played a major role in the research agenda of experimental and theoretical psychology. During the 1950s and 1960s a large data set was collected about the behaviour of humans, rats and pigeons in repeated choice experiments. At a typical experiment each subject at each trial has to predict whether a light would appear on his left or his right, whether the next card will be blue of yellow, or any other of many mutually-exclusive choice situations. Which light actually appears (or card etc.) depends on a random device operating with fixed probabilities which are independent of the history of outcomes and of the behaviour of the subject. The experiment then continues for many trials. In some experiments subjects received (small) monetary rewards for making the correct prediction, and in a few of those experiments, had to pay a small penalty for making the wrong prediction.A striking feature of this data is that subjects match the underlying probabilities of the two outcomes. Denote by p(L) the (fixed) probability with which the random device picks the option Left (alternatively, if the sequence of outcomes is predetermined, p(L) denotes the proportion of Left), then after a period of learning, subjects choose Left in approximately p(L) of the trials. Notice that matching suggests that subjects had learnt the underlying probabilities. But if those probabilities are known then the strategy that maximises expected utility is to always choose side which is chosen with probability greater than one half. Now if people are not able to maximise utility in this simple setting, can we reasonably expect them to do so in more complicated situation? This was recognised as a chllange to economists aleady in 1958 by Kenneth Arrow who wrote: “We have here an experimental situation which is essentially of an economic nature in the sense of seeking to achieve a maximum of expected reward, and yet theindividual does not in fact, at any point, even in a limit, reach the optimal behaviour. I suggest that this result points out strongly the importance of learning theory, not only in the greater understanding of the dynamics of economic behaviour, but even in suggesting that equilibria maybe be different from those that we have predicted in our usual theory.”(Arrow, 1958, p. 14). Moreover, stochastic learning theories (like Bush and Mosteller (1955)) which assume only that a person is more likely to choose an option in the future if he receives a positive feedback (the so called “Law of Effect”) do predict this kind of behaviour (the “probability matching theorem”of Estes and Suppes (1959)). So who is right?The purpose of this survey is threefold: First, to introduce today’s readers to what is meant by probability matching, and in particular to clarify which aspects of this phenomenon challenge the utility-maximisation hypothesis. Second, to familiarise the reader with the different theoretical approaches to behaviour in such circumstances, and to focus on the differences in predictions between these theories in light of recent advances (such as Borgers & Sarin (1993, 1995), and Borgers, Morales and Sarin (1997)). Third, to provide a comprehensive survey of repeated, binary choice experiments. Although these experiments have been surveyed before, my goal is to provide a complete and unbiased “survey of surveys”of these results (previous surveys, like Edwards (1956), Fiorina (1971) and Brackbill & Bravos (1962) focus only on specific set of experiments, and within the context of their own theories).With respect to my first objective, I note that the terms “probability matching”and “matching law”are sometimes confused: The behaviour known as probability matching is explained in details in sections 2 and 3. The “matching law”and other types of behaviour associated in the literature with the term “matching”, but which do not conflict with the assumption of utility (or reward) maximisation, are described in some detailed in the appendix.The results, in section 3, show that if the experiment is repeated often enough and/or if subjects are paid enough, they tend to asymptotically chose the side which maximises their expected reward, although humans appear to be very slow learners. Moreover, looking at the group’s average behaviour over a relatively small number of trials is likely to generate results supporting the matching hypothesis. Probability matching is therefore not a robust prediction of asymptotic behaviour in these settings.I show that this experimental setting is not as simple as one might think. First, we do not have a learning theory which predicts optimisation with probability 1 in this setting - impatient, but rational decision makers could end up choosing the wrong side forever. Second, although the data supports the fact that subjects condition their behaviour on the outcomes of the last trial, it also suggests that they condition it on additional features, like the outcomes of the trial before last. Subjects have no problems learning, for example, the pattern Left, Left, Left, Right, Left, Left, Left, Right, etc. which requires a memory of at least 4 trials back. However, stochastic learning theories restrict attention to memories of size one, hence ruling out front the possibility of any such pattern matching. In sections 3 and 4 I look in some more details on what exactly is being reinforced.Moreover, subjects do not like to believe outcomes occur in random (for example,they are more likely to guess Left after 3 consecutive Right s). Subjects try to look for patterns, even in situations when there are not any. This is supported by experiments showing that behaviour changes when subjects actually observe the random selection process. When these types of behaviour are averaged over a whole group, the matching hypothesis could artificially appear to outperform the utility maximisation hypothesis.The rest of the paper is organised in the following way. Section 2 provides the theoretical background. Section 3 surveys many of the known experiments. In section 4 I discuss the results and some of their implications. Section 5 concludes.2Theoretical BackgroundFor simplicity, I refer to the two options as Left and Right throughout this paper.Denote by p(L) the fixed probability with which Left is chosen by the random device. If we normalise to zero the utility of making the wrong prediction, then the expected payoff from choosing Left with probability (or frequency) p* is p p L U R p p L U R L R **()()()(())()⋅⋅+−⋅−⋅11, where U R i () is the utility of the reward received from correctly predicting i . If the utility of both rewards is constant, then the expression is maximised by p* =0 or p*=1, depending on which of the two expected rewards is greater. This is a static decision rule (no learning). Other static decision rules relevant to this experimental setting include Edwards' Relative Expected Loss Minimisation rule (Edwards 1961, 1962) and Simon's Minimal Regret (Simon 1976),where the decision maker either maximises, or minimises an expression based on his regret (rather than payoff) matrix (see Savage 1972). In the setting considered in this paper, the predictions of all three static rules are identical.Static rules neglect any effect that the learning process might have. Even if subjects learn and understand that Left and Right are chosen randomly and independently, they still have to learn the value of p(L). We can, therefore, go one step “down” in our rationality assumptions, and look at the learning process of a mathematician who perfectly understands the structure of the problem and who is trying to maximise his expected utility. The length of the learning process will depend on how time is dicounted, and its outcome will depend on the actual outcomes of the trials. These types of maximisation problems are known as the bandit problems (where a bandit is a nickname for a slot machine). If p(L)+p(R)=1 then the decision maker is, in effect,estimating only one probability. Hence, this is the one-armed bandit problem . If p(L)≠1-p(R) then this becomes the two-armed bandit problem . In general, the solution to these problems involves a period of experimenting followed by convergence to one side (this is sometimes known as the Gittins indices , see (Gittens 1989)). The multi-arm bandit problem was first introduced to Economics by Rothschild (1974), who pointed out that it is possible that a rational, but impatient decision maker will end up choosing the wrong side forever. To see why, consider a setting where p(L)=0.7 and a subject who’s impatience leads her to experiment for only three periods. Then with probability 0.216 she will end up choosing Right (because this is the probability that Right was chosen by the random device at least twice).From a descriptive point of view, the Gittins indices imply that the decision maker had somehow figured out the structure of the problem, that she experiments and that she keep statistics of all the outcomes of these experiments. These are obviously very strong assumptions. An alternative route was taken by mathematical psychologists (and some economists) which makes only a minimal assumption - that people are more likely to repeat a certain action if it proved successful in some sense in the past (what Erev & Roth call the “law of effect” and which dates back at least to Thorndike (1898)). More specifically, the decision maker is characterised in every given moment in time by a distribution over her strategy space (which represents the probability with which each strategy will be played in the next stage), and by an updating rule based on reinforcement. Theories which follow this general structure, where no beliefs, or beliefs-updating rules, are specified, are known as stochastic learning theories . These theories differ only with respect to the specifis of this updating rule. Predictions can now be made with regards to transitory behaviour and to behaviour in the limit. An attractive feature of stochastic learning theories is that, under certain conditions, they are equivalent to the replicator dynamic 1, another favourite metaphor of modern economists.A typical stochastic learning model is Bush and Mosteller’s (1955), where learning is assumed to be linear in the reinforcement. More specifically, assuming that the decision maker a priori prefers choosing Left (alt. Right) when the outcome is Left (alt. Right),the transition rule can be written as: p n p n L L ()()()+=−⋅+1111θθ when the outcome is Left, or p n p n L L ()()()+=−⋅112θ otherwise, where θ1 and θ2 are learning constants.In the limit, p p L p L p L L ()()()(())∞=+−⋅121θθ (see Bush & Mosteller (1955) for the exact conditions under which this limit exists). Notice that if the ratio of the Tetas is close to one, the model predicts probability matching in the limit. This is no coincidence: all stochastic learning models predict matching, under some conditions (different conditions for the different models).In general these models can be divided to two broad classes:1. Models where players always play a pure strategy (e.g. Right) but use a probabilistic updating rule (as in Suppes (1960) or Suppes and Atkinson (1960)).For example, “start with Left; stick with your strategy when you made a correct predictions; otherwise switch with probability ε), and2. Models in which agents use a deterministic updating rule to choose between the set of all mix strategies (like the Bush-Mosteller model mentioned above).In a recent paper, Borgers, Morales and Sarin (1997) show that no learning (updating)rule specified for class (a) can lead to optimal choice, and conjecture that a similar result holds for models in class (b). Leaving aside for the moment the question whether 1 Some work is needed before comparison can be made between the two interpretations: In a learning model the decision maker can choose between a continuum of strategies. In the biological model there is a continuum of agents, each with a fixed rule of behaviour. See Borgers and Sarin (1993) for more details.such models provide a realistic description of human learning, their result serves as an important benchmark for any theorems which might be proven in such settings. To be blunt, if we start with a rule that does not converge to the optimal behaviour in a simple setting, we should not be surprised when it does not converge in more complicated settings, like multiplayer games.In experimental settings, we can only guess what exactly is being reinforced. The typical approach, implicit to our discussion so far, is (a) that the set of strategies consists of one-shot strategies only, i.e. what to chose next, bearing in mind that these could be mixed, and (b) that the strength of the reinforcement is directly related to the payoffs (typically linear). Despite their intuitive appeal, these two assumptions are very strong and their experimental validity remains, still today, in doubt. As for the first assumption, it was repeatedly shown that subjects are able (quite easily) to respond differently to events which are four or five trials back in the sequence (see, for example, Anderson 1960). To this, Goodnow (1955), and Nicks (1959) suggested that subjects do not react to the outcome of the last trial, but instead, to runs of consecutive Lefts or Rights. These idea was further developed by Restle (1961). As for the second assumption; several effects (like the framing effect, and the negativity effect) have been identified in probability learning experiments. There is no simple solution to these problems. Several attempts have been made recently to account for the second set of effects (for example, Erev & Roth (1997), Tang (1996), Chen & Tang (1996)) with some success.Some experimenters suggested that subjects get bored with always choosing the same option, therefore switching between Left and Right throughout the trials. The most formal attempt is Brackbill & Bravos's (1962) model where subjects receive a greater utility by guessing correctly the outcome of the less frequent option. In these types of models utility-maximising individuals will not choose one strategy with probability 1 in the limit. Under some such utility structures, subjects may optimally end up matching p(L).An even more daring explanation is that subjects believe in the existence of some sort of regularities, or patterns, in the sequence of outcomes. Such a belief is the reason why they disregard their own experience and keep looking for rules and patterns. Of course, if a pattern exists, it is worth spending some time trying to find it. Once it is found, the subject can get 100 per cent of the rewards (compared to only p(L) in the static optimal rule described above). Restle (1961) discusses some of the typical attitudes of subjects suggesting that “..the subject seems to think that he is responding to patterns. Such attempts are natural. The subject has no way of knowing that the events occur in random, and even if he is told that the sequence is random he does not understand this information clearly, nor is there any strong reason for him to believe it.”(Restle, 1961, p. 109). A theory which accounts for pattern matching is clearly an attractive idea. Unfortunately, it is also an extremely hard idea to formalise, because of the size of the set of all patterns. Restle’s own theory (Restle 1961), which only accounts for one class of patterns (namely for consecutive runs), already becomes very complicated analytically when he considers the behaviour of subjects who get paid, or those who face decisions with more than two choices.3Survey of Experimental ResultsSubjects: Subjects in most experiments are undergraduate students (mostly psychology students). In Neimark (1956), Gardner (1958) and Edwards (1956, 1961) subjects are army recruits. Children were the subjects of Derks & Paclisanu (1967), Brackbill et al. (1962) and Brackbill & Bravos (1962) experiments.Apparatus: The most popular setting is the light guessing experiment: Subjects face two lights, their task being to predict which of the two would illuminate at the end of the trial. Otherwise, pre-prepared multiple choice answer sheets were used (as in Edwards 1961). Here, subjects choose one of two options (Left or Right) and then revealed a third column to find out whether it matched their choice. Sheets are prepared in advanced according to fixed probabilities. Finally, in the setting of Mores and Randquist (1960), subjects collectively observed a random event after individually predicting its outcome.Instructions: Subjects were instructed to maximise the number of correct predictions. In most experiments they were told that their actions could not affect the outcome of the next trials (this was obvious when pre-prepared sheets were used). Otherwise, instructions vary: some mention probabilities and others did not. I tried to exclude those experiments were subjects knew “too much”, for example, those experiments where they were told that the probabilities are fixed.Experimental Design: The important features (see discussion below) are: group size, number of trials, size of the last block of trials (where asymptotic behaviour is measured) and the size of reward(s). These details, whenever available, are provided in the tables below.Tables: The first table summarises results from experiments where subjects did not receive any payoff, but were still informed about the outcome of the trial. Table 2 lists the results of those experiments with monetary payoffs. The payoffs, in cents, appear in the fourth column where the leftmost number describes the payoff. For example, (1,0) means that subjects receive 1 cent for each correct guess, and 0 otherwise. p(L) is as before, and the group means are obtained by taking the group's average frequency of choosing Left over the last block of trials. The third table contains some individual results, taken from Edwards (1961) where the mean was measured over the last 80, out of 1000, trials. Each column contains the results for one of four groups which faced different p(L)’s. For example, in the group which faced p(L)=0.7 two subjects chose Left in all of the last 80 trials. Five (out of 20) chose Left 70 percent of the time or less.The fourth table summarises the results of Brackbill, Kappy & Starr (1962), and Barckbill & Bravos (1962). The left most column describes the ratio between the two rewards: for correctly predicting M (the most frequent event, with p(M)=0.75), and L.The main difference between this table and the previous three is that here the frequencies of choosing Left (p(L)=0.75 throughout) in the n th trial are given as a function of the prediction and outcome in the n-1th trial. For example, if subjects predicted M in the n-1th trial and the actual outcome of that trial was L, then the mean frequency with which M was chosen in the n th trial is given in the ML column.The fifth and final table is reproduced from Derks and Paclisanu (1967). It examines the relationship between probability matching and age (this is a part of a more general study into the relationship between cognitive development and decision making). 200 trials were used and the group average was measured over the last 100. p(L) equals 0.75 for all groups.Experimenter(s)Group Size Trials p(L)Group MeanGrant et al. (51)37600.250.150.750.85Jarvik (51)29870.600.65210.670.70280.750.80Hake & Hyman (53)102400.750.80Burke et al. (54)721200.90.87Estes & Straughan (54)162400.300.251200.150.13Gardner (58)244500.600.620.700.72Engler (58)201200.750.71Neimark & Shuford (59)361000.670.63Rubinstein (59)371000.670.78Anderson & Whalen (60)183000.650.670.800.82Suppes & Atkinson (60)302400.600.59Edwards (61)1010000.300.110.400.310.600.700.700.83Myers et al. (63)204000.600.620.700.750.800.87Friedman et al. (64)802880.800.81Table 1: Experiments with no Monetary PayoffsExperimenter(s)Group Size Trials Payoffs p(L)Group Mean Goodnow (55)14120(-1,1)0.700.820.900.99 Edwards (56)24150(10,-5)0.300.190.800.966150(4,-2)0.700.850.800.966150(4 or 12, -2)20.700.460.900.95Nicks (59)144380(1,0)0.670.7172380(1,0)0.750.79 Siegel & Goldstein (59)4300(0,0)0.750.75(5,0)0.750.86(5,-5)0.750.95 Suppes & Atkinson (60)2460(1,0)0.600.63(5,-5)0.600.64(10,-10)0.600.69 Siegel (61)20300(5,-5)0.650.750.750.93 Myers et al. (63)20400(1,-1)0.600.650.700.870.800.93(10,-10)0.600.710.700.870.800.9542500(0,-4)0.700.89Berby-Meyer & Erev(97)3(4,0)0.700.85(2,-2)0.700.95Table 2: Experiments with Monetary Payoffs2 Asymmetric payoffs here: Subjects received 12 cents for correctly predicting the right light, 4 cents for correctly predicting the left light, and -2 cents otherwise.3 Payoffs in Israeli agorot (In 1997, 1 Agora-= 0.01 of Israeli Shekel was approximately equal to$0.003).π = 0.7π = 0.6π = 0.4π = 0.3100914926100904826978547209681462095774317937643139174431388744013887135138770311285693111856629118064268806422875632147061204656116060591505856110564600µ = 83µ = 70µ = 0.31µ = 0.11Table 3: Individual asymptotics in 1000 trials (Edwards 1961)None45321-4000.770.710.560.68None123101-2000.740.820.380.56(1, 1)123101-2000.800.830.650.85(3, 3)123101-2000.820.870.670.73(5, 5)123101-2000.890.870.640.78(1, 4)104121-2000.760.790.500.75(1, 3)104121-2000.830.700.680.76(2, 3)104121-2000.800.840.580.85(1, 4)1012121-2000.740.570.710.61(1, 3)1012121-2000.720.620.630.76(2, 3)1012121-2000.840.880.700.72Table 4: Probability of guessing “Left” as a function of last outcome and last guess (Brackbill, Kappy & Starr, 1962, and Barckbill & Bravos, 1962).Nursery223429Kindergarten532129First Grade551020Second Grade48820Third Grade310720Fifth Grade213520Seventh Grade213320College413320Table 5: Matching and Age (Derks and Paclisanu, 1967)Finally, consider Morse and Rundquist (1960) experiment, where 16 subjects are instructed to guess whether a small rod dropped to the floor would intersect with a crack in the floor. Then, the same subjects went through a standard light guessing experiment, were the sequence of Lefts and Rights was determined by the outcomes of the first part of the experiment (that is, the same sequence as before, with “Left”replacing the outcome “No intersection”in the first round). In the second stage subjects, who are not aware of how the second sequence had been generated, are not able to watch the random move. In the first stage Morse and Rundquist reported that 5 subjects adopt a ''maximising'' strategy, and the group average was much higher than predicted by the probability matching hypothesis. Matching behaviour was observed in the second stage.3.1. Comments on the quality of the experimentsFirst important observation is that the sequences of Left’s and Right’s in some experiments were not statistically independent from the outcomes of the previous trials. For example, in some places randomisation took place within small blocks of trials: say 7 of every 10 consecutive trials were Lefts and the other 3 Rights. Also common practice was to exclude from the experiment three or more (or four or more) consecutive Lefts. Although I excluded most obvious forms of such violation of the non-contingency condition (which is assumed by the theoretical discussion so far) from the above tables, the sequences used by Grant et al., Jarvik, Gardner, Anderson & Whalen, Goodnow, and Galanter & Smith are not i.i.d. either. This is partially the fault of the technology that was available in those years for generating random sequences and partially because some experimenters did not appreciate the importance of such considerations. Of course, it then becomes possible that attentive subjects noticed the contingencies and act accordingly. For example if 7 out of each 10 trials are Lefts and in the first 8 you have counted 7 Lefts, it is optimal to guess Right in the remaining two trials. In general, optimising subjects will, in such circumstances, sometime guess the less frequent option. For similar considerations Fiorina (1971) concluded that the whole psychological literature on probability matching should be disregarded, and that the gambler’s fallacy might not be a fallacy after all. I leave it for the reader to draw his own conclusions from the above results and the discussion below.Second, asymptotic behaviour is estimated by taking the group average over the last block of trials. For this to be justified, it is required, at a minimum, that individual behaviour has already stabilised. Once again, I excluded those experiments where this was clearly not happening, but I suspect that the individual learning curves have not yet converges in Estes & Straughan (1954) and Neimark & Shuford (1959) experiments, where behaviour seems to still be changing in the last block of trials.4Discussion。
Abstract Pure bigraphs structure and dynamics

Robin Milner
University of Cambridge Computer Laboratory, Cambridge CB3 0FD, UK
Abstract Bigraphs are graphs whose nodes may be nested, representing locality, independently of the edges connecting them. They may be equipped with reaction rules, forming a bigraphical reactive system (Brs) in which bigraphs can reconfigure themselves. Following an earlier paper describing link graphs, a constituent of bigraphs, this paper is a devoted to pure bigraphs, which in turn underlie various more refined forms. Elsewhere it is shown that behavioural analysis for Petri nets, π -calculus and mobile ambients can all be recovered in the uniform framework of bigraphs. The paper first develops the dynamic theory of an abstract structure, a wide reactive system (Wrs), of which a Brs is an instance. In this context, labelled transitions are defined in such a way that the induced bisimilarity is a congruence. This work is then specialised to Brss, whose graphical structure allows many refinements of the theory. The latter part of the paper emphasizes bigraphical theory that is relevant to the treatment of dynamics via labelled transitions. As a running example, the theory is applied to finite pure CCS, whose resulting transition system and bisimilarity are analysed in detail. The paper also mentions briefly the use of bigraphs to model pervasive computing and biological systems.
石墨炉原子吸收光谱法测定血浆中铂

工作简报石墨炉原子吸收光谱法测定血浆中铂王世亮1,叶红杨2,马晓芹2,许媛媛2,储成顶2*(1.安徽职业技术学院,合肥230051; 2.合肥工业大学控释药物研究所,合肥230009)摘 要:提出了石墨炉原子吸收光谱法测定顺铂人血浆中铂浓度。
采集人静脉血作样品,用浓硝酸及过氧化氢消解。
冷却至4 ,使脂肪固化并过滤除去。
收集滤液,蒸干,用200 L盐酸(1+99)溶液浸取残渣,所得溶液供石墨炉原子吸收光谱分析。
方法的线性范围为0.02~1.00m gL-1,检出限(3 )为0.016mg L-1,回收率在94.6%~120.0%之间,相对标准偏差(n=5)小于7%。
关键词:石墨炉原子吸收光谱法;铂(!);顺铂;血浆中图分类号:O657.31 文献标志码:A 文章编号:1001 4020(2010)03 0257 03GF AAS Determination of Platinum in Hu man Plasma WANG Shi liang1,YE Hong yang2,MA Xiao qin2,XU Yuan yuan2,CHU C hen ding2*(1.A nhui T echno Vocational College,H ef ei230051,China;2.Resear ch I nstitute of S us tained R eleas e D r ugs,H ef ei Univ er sity of I nd ustr y,H ef ei230009,China)Abstract:A method fo r deter mination of platinum in human plasma r eleased fro m t he sustained release implant o f cis platinum by GF A AS w as pr oposed.Veino us blo od was taken as sample and dig est ed w ith conc.H NO3and H2O2.T he clear digested solut ion w as coo led to4 to so lidify fat which w as then separ ated byfiltrat ion.T he filtr ate was collected and evapor at ed to dr yness,the r esidue w as taken up w ith200 L of H Cl(1+99)and used for G F A A S analysis under the optimized co nditions.L inear relationship was obtained between valuesof absor bance and mass co ncentration o f P t(!)in the r ang e f rom0.02-1.00mg L-1.Detect ion lim it(3 )o f the method was found to be0.016mg L-1.R eco very and pr ecision w ere tested on the base of simulated samples by standar d addition metho d,giv ing values of recov ery in the r ang e fr om94.6%to120.0%,and values o f R SD∀s(n=5)less than7%.Keywords:GF A AS;Platinum(!);cis Platinum;Blood plasma缓释顺铂植入剂是一种新型抗肿瘤长效制剂,标称缓释期15d,可在组织器官中植入给药或外科术中给药,实现局部的药物高浓度及较小的周边血药浓度,明显减轻治疗的副作用和不良反应。
反应扩散方程
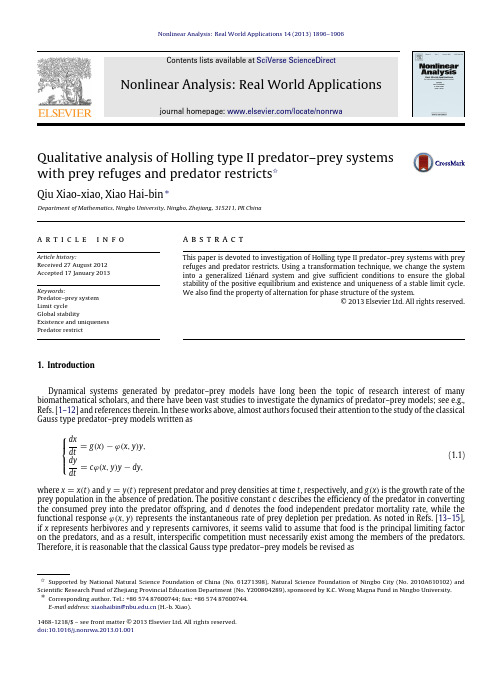
Article history: Received 27 August 2012 Accepted 17 January 2013 Keywords: Predator–prExistence and uniqueness Predator restrict
Qiu Xiao-xiao, Xiao Hai-bin ∗
Department of Mathematics, Ningbo University, Ningbo, Zhejiang, 315211, PR China
article
info
abstract
This paper is devoted to investigation of Holling type II predator–prey systems with prey refuges and predator restricts. Using a transformation technique, we change the system into a generalized Liénard system and give sufficient conditions to ensure the global stability of the positive equilibrium and existence and uniqueness of a stable limit cycle. We also find the property of alternation for phase structure of the system. © 2013 Elsevier Ltd. All rights reserved.
journal homepage: /locate/nonrwa
Abstract

Permissionto copy without fee all or part of this materilalis granted provided that the copies are not made or distributed for direct
commercial advantage, the ACM copyright notice and the title of the publication and its date appear, and notice is given that copying is by permission of the Association for Computing Machinery. To copy otherwise, or to republish, requires a fee and/or specfic permission.
- 1、下载文档前请自行甄别文档内容的完整性,平台不提供额外的编辑、内容补充、找答案等附加服务。
- 2、"仅部分预览"的文档,不可在线预览部分如存在完整性等问题,可反馈申请退款(可完整预览的文档不适用该条件!)。
- 3、如文档侵犯您的权益,请联系客服反馈,我们会尽快为您处理(人工客服工作时间:9:00-18:30)。
Dynamical Equilibrium of Interacting Ant SocietiesMelvin Boon-Tiong LeokMSC609,Caltech,Pasadena,CA91126-0609,USAmleok@(626)744-9985AbstractThe sustainable biodiversity associated with a speci…c ecological niche as a function of land area is analysed computationally by considering the interaction of ant societies over a collection of islands.A power law relationship between sustainable species and land area is observed.We will further consider the e¤ect a perturbative in‡ow of ants has upon the model.1IntroductionIt has been observed qualitatively that the biodiversity of a landmass varies as a function of the area raised to a power less than unity.We will attempt to develop a numerical model of the interaction of ant societies which are constantly perturbed by the external in‡ow of new ants,and determine the sustain-able number of species as a function of area in the dynamically stable equilibrium condition.2Model RepresentationThe conventions which will be utilized in the development of the numerical model of ant society interaction are described herein.They generally serve to constrain the scope and com-plexity of the model.2.1Phase and State VariablesThe model will utilize phase variables to represent the inde-pendent parameters such as land area,and rate of in‡ow of new ants.State variables will represent the dynamic state of the simulation at a given time.These variables are described in the following section.2.1.1Discretization of timeThis model will represent the dynamic state of ant populations using discrete time,thus the model will be formulated in the form of a set of coupled mappings.2.1.2Normalization of variablesCertain phase and state variables,such as the land area,pop-ulation density will be normalized to the interval[0;1]2R as a method of simplifying the analysis of the model.2.1.3Representation of PopulationsThe state of the ant societies will be represented in matrix form,with the rows corresponding to the distinct ant species, and the columns corresponding to the island upon which they reside.As such the population state at a given time can be represented in the following matrix,P(t)=B@p11¢¢¢p1m.........p n1¢¢¢p nm1C A(1)where p ij=population of i-th ant species on the j-th island, t2N.Associated with this population matrix,is the population density matrix,which is,Pd(t)=B@pd11¢¢¢pd1m.........pd n1¢¢¢pd nm1C A(2)where pd ij=population density of i-th ant species on the j-th island,t2N.The population density is related to the population by,pd ij=p ijArea j(3) where Area j=maximum population for j-th island.2.2Initial conditionsThe islands are assumed to be initially uninhabited,with the ant population on each island due to both self-propagation and the in‡ux of new ants from the continent.In addition, the following conditions are assumed for the ant in‡ux,and the self-interaction on each island.2.2.1Distribution of in‡ow populationIt shall be assumed that the perturbative in‡ow of new ants from the continent will be randomly chosen from uniformly distributed pool of ant species.We shall further assume that the number of ants which are derived from immigration from the continent is proportional to the area of the island.Due to the negligible size of the islands compared to that of the continent,the e¤ect of back‡ow of ant populations to the continent shall be neglected.2.2.2Genetic StasisWe shall assume that the biodiversity is completely derived from the in‡ow of a variety of ant species from the continent and that the ant species do not adapt and deviate genetically from species obtained from the continent.2.3Population InteractionsThe problem can be considered to be a generalization of the insect population model considered by May[1],in his paper which introduces the notion of the logistic mapping.The lo-gistic map was originally used to represent the e¤ect limited resources have upon the growth of insect populations.The addition of interaction between competing ant popu-lations can be modelled with the use of an inter-population attrition factor,which is described further below.2.3.1Inter-island InteractionThe mode of inter-island interaction will be that of travel over water.Without loss of generality,the attrition of ant popula-tions due to travel over water can be assumed to be50%unit length¡1,p(d)=p0(0:5)d(4) This relationship will serve to a¤ect terms in the interaction matrix for ant populations residing on di¤erent islands.The inter-island distances can be represented as a distance matrix as follows,D=0B@d11¢¢¢d1m.........d m1¢¢¢d mm1C A(5)where d ij=distance between i-th and j-th island.From the distance matrix,we can obtain the associated dis-sipation matrix,which determines the percentage of ants sur-viving the trip between the i-th and j-th island.f ij=p(d ij) p0=(0:5)d ij(6)2.3.2Inter-species InteractionThe ant societies will interact according to an interaction ma-trix.The intensity of the attrition will be dependent upon the population density of the island,such that an increase in the population density would increase both the possibility of confrontation between populations on the same island,as well as the possibility that a portion of the ant population ventures out in search of other island to colonize.As each species potentially interacts with all other ant colonies both within the same island,and on di¤erent islands, we can de…ne the following mapping,p ij(t+1)=f oj¢NµArea jn;Area j4n¶+Xk=1::nl=1::ma ijkl p kl(t)(7)where f oj corresponds to the dissipation due to travel from the continent to the j-th island.N³Area jn;Area j4n´is a random variable from a normal distribution.The normally distributed random term corresponds to the contribution of immigration from the continent.For the second term of the expression,a ijkl=interaction factor between i-th ant species on j-th island,and k-th ant species on l-th island.2.3.3Strength of InteractionWe will be concerned with four possible sources of population change,1.internal population growth2.attrition due to interaction with other species3.population decrease due to emigration4.population increase due to immigration,both from thecontinent and other islandsThe following functions serve to represent the intensity of population interactions.All of these functions will de…nedin terms of the total population density of the island,x= P nq=1pd qj.Growth Function The logistic mapping considers the inter-action of a population with limiting environmental resources. We shall thus base the growth function on the same concept. We shall represent the total population density at time t on the j-th island as T j(t)=P nq=1pd qj(t),and the next iterate of the total population density due to interaction only withthe environment at time t+1on the j-th island as T j(t+1)= P nq=1pd qj(t+1).Then by analogy to the logistic mapping,the following equa-tion should be satis…ed,T j(t+1)=¸¢T j(t)¢[1¡T j(t)](8) Where¸2[0;1].We would like the growth function to satisfy the following,p ij (t +1)=p ij (t )¢T j (t +1)T j (t )=p ij (t )¢G (x )(9)From which,we can derive the growth function as,G (x )=T j (t +1)T j (t )=¸¢[1¡T j (t )]=¸¢(1¡x )(10)Attrition Function A is the attrition function ,de…ned in this fashion,A (x )=k 1¢x®(11)Emigration Function E is the emigration function ,which is related to the attrition function (11)by,E (x )=¹¢A (x )(12)where ¹is a parameter within the range [0;1]2R .2.3.4Interaction Factors There are generally four classes of inter-population interaction,1.self-interaction2.interaction with di¤erent species on the same island3.interaction with same species on di¤erent islands4.interaction with di¤erent species on the same islandThe interaction factor for each of these cases will be consid-ered in the following section.Self-Interaction The interaction factor for self-interactioncan be de…ned as the increase due to interaction populationgrowth,less the decrease due to emigration.Thus,a ijij =G Ãn X q =1pd qj !¡E Ãn X q =1pd qj !(13)Interaction with di¤erent species on the same islandThe e¤ect interaction with di¤erent species on the same island have upon the population of a species in question,would be adecrease in population due to attrition.a ijkj =¡A Ãn Xq =1pd qj !(14)Interaction with same species on di¤erent islands The increase due to immigration of the same species from di¤erent islands would be the portion of the immigrants multiplied by the dissipation due to travel.a ijil =f jl ¢EÃn Xq =1pd ql !(15)Interaction with di¤erent species on di¤erent islandsThe attrition due to interaction with hostile immigrant species would be the attrition factor,multiplied by the portion of im-migrants,multiplied by the dissipation due to travel.a ijkl =¡f jl ¢E Ãn X q =1pd ql !¢A Ãn Xq =1pd qj !(16)Complete Formulation of the Interaction Factor Wecan collate the interaction factors for the individual cases inthis complete formulation,a ijkl =8>>>>>><>>>>>>:G ³P n q =1pd qj ´¡E ³P n q =1pd qj ´i =k;j =l f jl ¢E ³P n q =1pd ql ´i =k;j =l ¡A ³P n q =1pd qj ´i =k;j =l ¡f jl ¢E ³P n q =1pd ql ´¢A ³P n q =1pd qj´i =k;j =l (17)Thus,the above equation,in conjunction with the mapping (7),de…nes a mapping,M :P (t )!P (t +1)(18)3Implementation of the modelThe formulation above describes the mathematical framework for the model of interacting ant populations.In order to im-plement the model,certain parameters and initial conditionsneed to be speci…ed.These are described in the following sec-tion..3.1User-de…ned parameters The following parameters need to be speci…ed to uniquely de-…ne the simulation.3.1.1Stability of Population Fluctuations The stability of population ‡uctuations is in‡uenced by the choice of the parameter ¸.Recall that this parameter is anal-ogous to the control parameter in the logistic mapping.As we’re more concerned with inter-species interaction,we shall limit ¸to values in which the single population case results ina stable population,thus¸is within the range(1;3)2R.For the purpose of the simulation,we shall let¸=2.3.1.2Competition between speciesThe competition between species results in attrition of the ant populations.k1corresponds to the percentage of the popula-tion of the island lost to con‡ict when the island is…lled to capacity.®speci…es the power law relation which the intensity of attrition increases as a function of population density.We shall be using k1=0:05,®=2:5.3.1.3EmigrationThe emigration parameter¹determines the proportion of the population which are driven o¤the island due to population pressure.Since we can assume that the population out‡ow is relatively insubstantial,we shall take¹=0:1.3.2Initial ConditionsInitial conditions determine the physical conditions which the simulation evolves under.These include the distance from the continent,inter-island distances,and the areas of the individ-ual islands.3.2.1Distance from the continentDistances from the continent should be su¢ciently large that the in‡ux of immigrant ants from the population do not over-whelm the inter-island dynamics of the system,we shall set the continental distance to a minimum of5.3.2.2Area of islandsThe area of the individual islands will be randomly assigned to provide a representative sample of the phase space of sim-ulations.3.2.3Inter-island distancesThe inter-island distances will be kept constant to reduce the e¤ect of the placement of the randomly sized islands.Further-more,to isolate the contribution of distances from the conti-nent,all the inter-island distances will be set to1.4Results from the simulationThe simulation was constrained to n=10;m=5.The…rst 100iterations were discarded from the analysis,as they were assumed to exhibit transient as opposed to the characteristic dynamics of the system.The average number of species on each island over the next100turns was taken as the number of species which the island would support.We then…t the data to the power law relation to determine the scaling exponent,°.species j_Area j°(19) The scaling exponent is obtained as the gradient of a log-log plot of species j versus area j.This was repeated over20initial area con…gurations,and the mean and standard deviation of the scaling exponents were obtained.4.1Scaling exponent as a function of dis-tanceThe scaling exponent for the number of species changes as a function of distance from the continent.The tabulated results are as follows.distance°¾5.000.36560.07325.250.31620.09215.500.27910.08395.750.23830.08626.000.20010.05706.500.17180.05327.000.15270.08128.000.12930.110910.000.12100.086914.000.13050.113418.000.11900.0470The results are illustrated graphically in the plot below.0.00000.05000.10000.15000.20000.25000.30000.35000.40000.45000.50004.0000 6.00008.000010.000012.000014.000016.000018.000020.0000Scaling exponent versus distanceNote that there are two modes of saturation in the simu-lation.In the case of extremely low values of distance,the in‡ow of new ants is so substantial as to cause the number of species on each island to saturate at the maximum number of species available.In the extreme of high values of distance, the external perturbation is so small that the islands can be considered to be independent of the in‡uence of the continent once the dynamics settle onto the attractor of the phase space.0.01000.10001.00001.000010.0000log-log plot of scaling exponent versus distanceThe power law regression for the 8data points with distances between 5and 8gives the following relationship for the scaling exponent and distance,°_distance ¡0:0766(20)The r 2=0:8442does suggest that the power law relation between the scaling exponent and distance from the continent is a reasonable approximation.5ConclusionThe results of our simulation tends to indicate that the dy-namical equilibrium of interacting ant societies follows a power law relation between the number of species and the area of the island.The scaling exponent is less than unity,and varies ac-cording to the power law,°_distance ¡0:0766,for moderate values of distance.The exact form of the power law relation between the scal-ing exponents and the distance is likely to vary depending on the user parameters speci…ed for the simulation.However,the quality of the power law regression does suggest that for dynamics with qualitatively similar behaviour,an analogous power law relationship is likely to arise for distances which do not result in saturation.References[1]Simple mathematical models with very complicated dynam-ics.Robert M.May,Nature 261,459-467(1976)。