Temporally Adaptive Frameless Rendering
新译林版高三总复习-必修2-思维导图-话题词汇课本逻辑梳理

Relax your mind
Take in enough carbohydrates to keep up energy
Eat food rich in protein
Drink throughout exercise to make up for water lost to sweat
Wear proper clothes
Help stretch muscles Encourage bone development
Over the long term
Stay slim
Get over negative feelings
Relieve stress
Encourage you to maintain a positive and balanced outlook
Play an energetic Twist ansdamtubrna beat
The roasted meat on the street stands
Fall on The Spring Festival
The Mid-autumn Lantern Festival The Dragon Boat Festival The Lantern Festival
effect on
Improve the efficiency of heart and lung
Be better prepared to fight off infection Make people grow stronger
strengthen immune system
Be at a lower risk of disease
Light up colorful fireworks Set off firecrackers
《6g典型场景和关键能力》 英文

6G Typical Scenarios and Key Capabilities1. Introduction6G, the next generation of mobilemunication technology, is expected to bring about revolutionary changes in various aspects of our lives. In this article, we will explore the typical scenarios and key capabilities of 6G, and discuss how they will impact the future ofmunication and technology.2. Enhanced ConnectivityOne of the key capabilities of 6G is enhanced connectivity. With the advent of 6G, we can expect to see a significant improvement in network speeds and coverage. This will enable seamless connectivity in urban areas, remote regions, and even in outer space, paving the way for new applications and services that were previously unimaginable.3. Ultra-Reliable CommunicationAnother important aspect of 6G is ultra-reliablemunication. This implies that the network will be able to provide extremely reliable and low-latencymunication, making it suitable for critical applications such as remote surgery, autonomous vehicles, and industrial automation. The ultra-reliable nature of6G will redefine what is possible in terms of mission-criticalmunication.4. Massive Internet of Things6G is also expected to support a massive Internet of Things (IoT) ecosystem, which will epass billions of devices and sensors. The network will be able to efficiently handle the massive amount of data generated by these devices, and provide the necessary connectivity and security for various IoT applications, ranging from smart cities to precision agriculture.5. Holographic CommunicationOne of the more futuristic capabilities of 6G is holographicmunication. This technology will enable users to interact with each other in a more immersive and lifelike manner, blurring the lines between physical and virtualmunication. Holographicmunication has the potential to revolutionize the way we collaborate, learn, and entertain ourselves.6. Personalized Artificial Intelligence6G will also pave the way for personalized artificial intelligence (本人) experiences. The network will be able to supportadvanced 本人 applications that are tailored to individual users, providing them with personalized rmendations, insights, and assistance. This will bring 本人 closer to users and enable a more seamless integration of 本人 into our daily lives.7. ConclusionIn conclusion, the typical scenarios and key capabilities of 6G promise to bring about a new era of connectivity,munication, and technological innovation. The enhanced connectivity, ultra-reliablemunication, massive Internet of Things, holographicmunication, and personalized 本人 experiences will revolutionize various industries and reshape the way we interact with technology. As we look forward to the deployment of 6G, it is important to consider the possibilities and implications that this new generation of technology will bring.。
超科幻未来房子英语作文
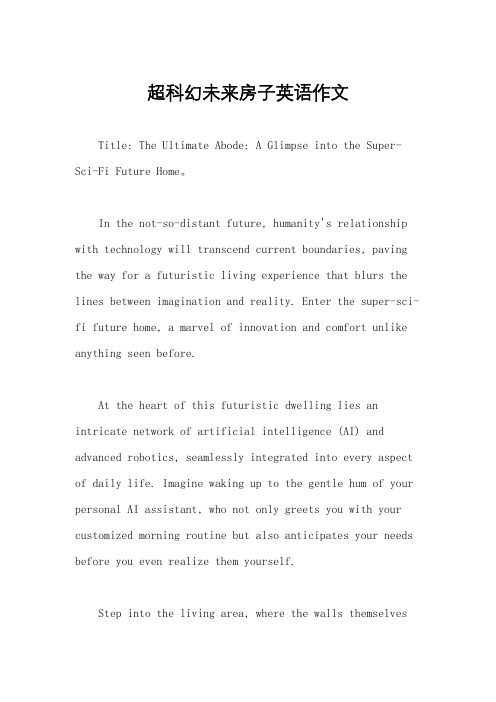
超科幻未来房子英语作文Title: The Ultimate Abode: A Glimpse into the Super-Sci-Fi Future Home。
In the not-so-distant future, humanity's relationship with technology will transcend current boundaries, paving the way for a futuristic living experience that blurs the lines between imagination and reality. Enter the super-sci-fi future home, a marvel of innovation and comfort unlike anything seen before.At the heart of this futuristic dwelling lies an intricate network of artificial intelligence (AI) and advanced robotics, seamlessly integrated into every aspect of daily life. Imagine waking up to the gentle hum of your personal AI assistant, who not only greets you with your customized morning routine but also anticipates your needs before you even realize them yourself.Step into the living area, where the walls themselvescome to life with immersive holographic displays, allowing you to transform your surroundings at will. Whether you prefer a serene forest retreat or a bustling cityscape, the possibilities are endless, limited only by your imagination.But the true magic of the super-sci-fi future home lies in its adaptive architecture. Gone are the days of rigid floor plans and static living spaces. Instead, nanotechnology-infused materials enable the home to shape-shift according to your preferences, creating dynamic environments tailored to your mood and activities.Need a cozy nook for reading or a spacious area for entertaining guests? With a simple voice command or gesture, the furniture seamlessly reconfigures itself to suit your needs, blurring the lines between form and function.Of course, no futuristic home would be complete without cutting-edge sustainability features. Advanced solar panels and energy-efficient systems ensure minimal environmental impact, while integrated recycling and waste management systems work tirelessly behind the scenes to keep the homerunning smoothly.But perhaps the most awe-inspiring feature of thesuper-sci-fi future home is its ability to transcendphysical limitations. Through the power of virtual reality and augmented reality technologies, you can explore distant worlds, attend live events, or even meet up with friendsand family from the comfort of your own home.In this hyper-connected future, distance becomes obsolete, and the boundaries between the virtual and thereal begin to blur. Yet, amidst all the technological marvels, the super-sci-fi future home remains a sanctuary,a place where comfort, convenience, and innovation converge to create an unparalleled living experience.As we peer into the future, one thing becomes clear:the possibilities are as limitless as the imaginationitself. The super-sci-fi future home is not just a dwelling; it's a glimpse into a world where the boundaries between fantasy and reality fade away, leaving only endless possibilities in their wake.。
《2024年视觉语法视域下竖屏微电影广告的多模态隐喻构建——以华为广告《悟空》为例》范文
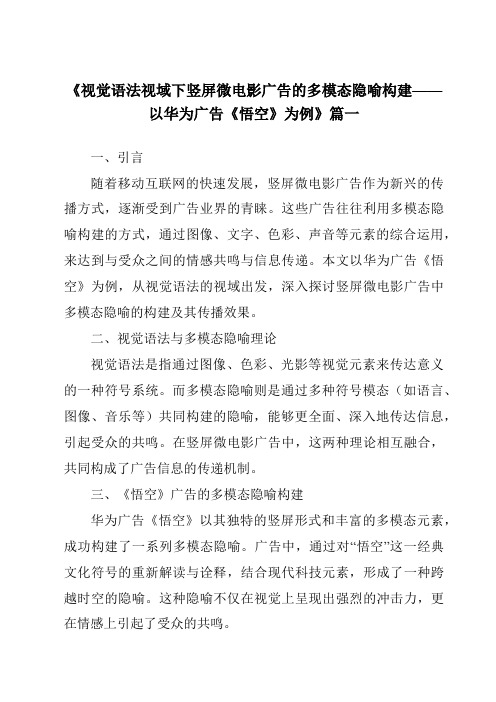
《视觉语法视域下竖屏微电影广告的多模态隐喻构建——以华为广告《悟空》为例》篇一一、引言随着移动互联网的快速发展,竖屏微电影广告作为新兴的传播方式,逐渐受到广告业界的青睐。
这些广告往往利用多模态隐喻构建的方式,通过图像、文字、色彩、声音等元素的综合运用,来达到与受众之间的情感共鸣与信息传递。
本文以华为广告《悟空》为例,从视觉语法的视域出发,深入探讨竖屏微电影广告中多模态隐喻的构建及其传播效果。
二、视觉语法与多模态隐喻理论视觉语法是指通过图像、色彩、光影等视觉元素来传达意义的一种符号系统。
而多模态隐喻则是通过多种符号模态(如语言、图像、音乐等)共同构建的隐喻,能够更全面、深入地传达信息,引起受众的共鸣。
在竖屏微电影广告中,这两种理论相互融合,共同构成了广告信息的传递机制。
三、《悟空》广告的多模态隐喻构建华为广告《悟空》以其独特的竖屏形式和丰富的多模态元素,成功构建了一系列多模态隐喻。
广告中,通过对“悟空”这一经典文化符号的重新解读与诠释,结合现代科技元素,形成了一种跨越时空的隐喻。
这种隐喻不仅在视觉上呈现出强烈的冲击力,更在情感上引起了受众的共鸣。
四、视觉元素的多模态隐喻构建在《悟空》广告中,视觉元素发挥了至关重要的作用。
画面中的色彩运用巧妙,如悟空的金箍棒与华为产品的色彩相呼应,形成了视觉上的统一与和谐。
同时,通过高超的摄影技巧和剪辑手法,将传统与现代、东方与西方的元素巧妙地融合在一起,形成了一种独特的视觉冲击力。
这些视觉元素共同构建了多模态隐喻,使得广告信息得以更加有效地传递。
五、听觉元素的多模态隐喻构建除了视觉元素外,《悟空》广告中的听觉元素也发挥了重要作用。
广告中的背景音乐既符合了广告的节奏,又为观众营造了一种强烈的情感氛围。
这种听觉元素与视觉元素的完美结合,使得多模态隐喻的构建更加完善,使得观众能够在感官上得到全面的体验。
六、结论通过对华为广告《悟空》的分析,可以看出竖屏微电影广告中多模态隐喻构建的重要性。
Arnold渲染器用户指南说明书

Arnold featuresMemory-efficient, scalable raytracer rendering software helps artists render complex scenes quickly and easily.◆ See what's new (video: 2:31 min.)◆ Get feature details in the Arnold for Maya, Houdini, Cinema 4D, 3ds Max, or Katana user guidesSubsurface scatterHigh-performance ray-traced subsurface scattering eliminates the need to tune point clouds. Hair and furMemory-efficient ray-traced curve primitives help you create complex fur and hair renders.Motion blur3D motion blur interacts with shadows, volumes, indirect lighting, reflection, or refraction. Deformation motion blur and rotational motion are also supported. VolumesThe volumetric rendering system in Arnold can render effects such as smoke, clouds, fog, pyroclastic flow, and fire.InstancesArnold can more efficiently ray trace instances of many scene objects with transformation and material overrides. Subdivision and displacementArnold supports Catmull-Clark subdivision surfaces.OSL supportArnold now features support for Open Shading Language (OSL), an advanced shading language for Global Illumination renderers. Light Path ExpressionsLPEs give you power and flexibility to create Arbitrary Output Variables to help meet the needs of production.NEW|Adaptive samplingAdaptive sampling gives users another means of tuning images, allowing them to reduce render times without jeopardizing final image quality. NEW|Toon shaderAn advanced Toon shader is part of a non-photorealistic solution provided in combination with the Contour Filter.NEW|DenoisingTwo denoising solutions in Arnold offer flexibility by allowing users to use much lower-quality sampling settings. NEW|Material assignments and overrides Operators make it possible to override any part of a scene at render time and enable support for open standard framework such as MaterialX.NEW|Alembic proceduralA native Alembic procedural allows users to render Alembic files directly without any translation.NEW|Profiling API and structured statistics An extensive set of tools allow users to more easily identify performance issues and optimize rendering processes.Standard Surface shaderThis energy-saving, physically based uber shader helps produce a wide range of materials and looks. Standard Hair shaderThis physically based shader is built to render hair and fur, based on the d'Eon and Zinke models for specular and diffuse shading.Flexible and extensible APIIntegrate Arnold in external applications and create custom shaders, cameras, light filters, and output drivers. Stand-alone command-line rendererArnold has a native scene description format stored in human-readable text files. Easily edit, read, and write these files via the C/Python API.◆ See Arnold 5.1 release notesIntegrate Arnold into your pipeline•Free plug-ins provide a bridge to the Arnold renderer from within many popular 3D applications.•Arnold has supported plug-ins available for Maya, Houdini, Cinema 4D, 3ds Max, and Katana.•Arnold is fully customizable, with a powerful API to create custom rendering solutions.◆ See Arnold plug-ins。
《2024年基于机器学习算法的超材料快速自动设计研究》范文
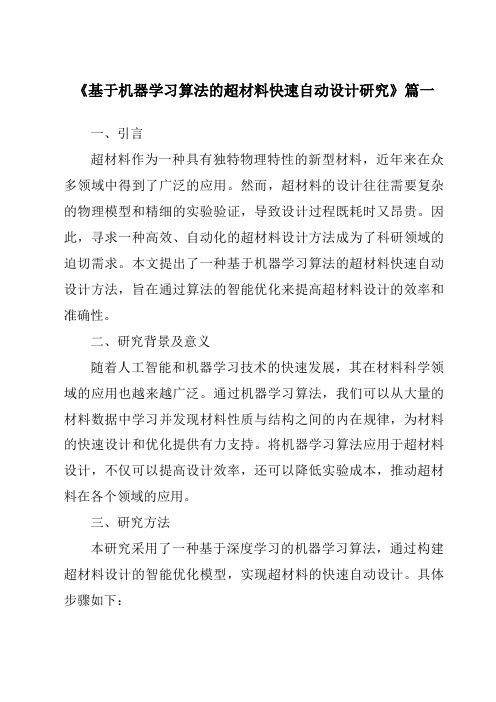
《基于机器学习算法的超材料快速自动设计研究》篇一一、引言超材料作为一种具有独特物理特性的新型材料,近年来在众多领域中得到了广泛的应用。
然而,超材料的设计往往需要复杂的物理模型和精细的实验验证,导致设计过程既耗时又昂贵。
因此,寻求一种高效、自动化的超材料设计方法成为了科研领域的迫切需求。
本文提出了一种基于机器学习算法的超材料快速自动设计方法,旨在通过算法的智能优化来提高超材料设计的效率和准确性。
二、研究背景及意义随着人工智能和机器学习技术的快速发展,其在材料科学领域的应用也越来越广泛。
通过机器学习算法,我们可以从大量的材料数据中学习并发现材料性质与结构之间的内在规律,为材料的快速设计和优化提供有力支持。
将机器学习算法应用于超材料设计,不仅可以提高设计效率,还可以降低实验成本,推动超材料在各个领域的应用。
三、研究方法本研究采用了一种基于深度学习的机器学习算法,通过构建超材料设计的智能优化模型,实现超材料的快速自动设计。
具体步骤如下:1. 数据准备:收集超材料的相关数据,包括材料的组成、结构、性质等,建立超材料数据库。
2. 特征提取:从超材料数据库中提取对设计有用的特征,如材料的组成比例、结构参数等。
3. 构建模型:利用深度学习算法,构建超材料设计的智能优化模型。
该模型可以根据提取的特征,预测材料的性质和性能。
4. 模型训练与验证:使用部分数据对模型进行训练和验证,确保模型的准确性和可靠性。
5. 自动设计:将模型应用于超材料的自动设计过程中,根据设计要求,自动寻找满足条件的材料组成和结构。
四、实验结果与分析通过实验,我们验证了基于机器学习算法的超材料快速自动设计方法的有效性和准确性。
实验结果如下:1. 设计效率:与传统的超材料设计方法相比,基于机器学习算法的设计方法可以大大提高设计效率。
在同样的时间内,可以设计出更多的超材料方案。
2. 设计准确性:机器学习模型可以准确预测超材料的性质和性能,降低实验验证的次数和成本。
PNY Quadro P1000 显卡说明书

1 VGA/DVI/HDMI support via adapter/connector/bracket |2 Windows 7, 8, 8.1 and Linux |3 Product is based on a published Khronos Specification, and is expected to pass the Khronos Conformance Testing Process when available. Current conformance status can be found at /conformance |4 GPU supports DX 12.0 API, Hardware Feature Level 12_1© 2021 NVIDIA Corporation and PNY. All rights reserved. NVIDIA, the NVIDIA logo, Quadro, nView, CUDA, and NVIDIA Pascal are trademarks and/or registered trademarks of NVIDIA Corporation in the U.S. and other countries. The PNY logotype is a registered trademark of PNY Technologies. OpenCL is a trademark of Apple Inc. used under license to the Khronos Group Inc. All other trademarks and copyrights are the property of their respective owners. J U N21Full Professional Performance and Features in a Small Form Factor . The Quadro P1000 combines a 640 CUDA core Pascal GPU, 4 GB GDDR5 on-board memory and advanced display technologies in a low-profile form factor to deliver amazing graphics performance for demanding professional applications. Support for four 4K displays (4096x2160 @ 60Hz) with HDR color gives you an expansive visual workspace to see your ideas come to life in stunning detail.Quadro cards are certified with a broad range of sophisticated professional applications, tested by leading workstation manufacturers, and backed by a global team of support specialists. This gives you the peace of mind to focus on doing your best work. Whether you’re developing revolutionary products or telling spectacularly vivid visual stories, Quadro gives you the performance to do it brilliantly. THE PNY ADVANTAGE PNY provides unsurpassed service and commitment to its professional graphics customers. In addition, PNY delivers a complete solution that includes the appropriate adapters, cables, brackets, driver software installation disc, and documentation to ensure a quick and successful install.FEATURES >Four mini DisplayPort 1.4 Connectors 1 >DisplayPort with Audio >NVIDIA nView ® Desktop Management Software >HDCP 2.2 Support >NVIDIA Mosaic 2 >NVIDIA Iray and MentalRay Support PACKAGE CONTENTS >NVIDIA ® Quadro ® P1000 Professional Graphics Board >Aattached full-height (ATX) bracket >Unattached low-profile (SFF) bracket >Four mDP to DP adapters >Printed Quick Start Guide >Printed Support Guide WARRANTY AND SUPPORT >3-Year Warranty >Pre- and Post-Sales Technical Support >Dedicated Field Application Engineers >Direct Tech Support Hot Lines PNY PART NUMBER VCQP1000V2-PB SPECIFICATIONS GPU Memory 4 GB GDDR5Memory Interface 128-bit Memory Bandwidth Up to 80 GB/s NVIDIA CUDA ® Cores 640 System Interface PCI Express 3.0 x16Max Power Consumption 47 W Thermal Solution Active Form Factor2.713” H x 6.061” L, Single Slot, Low Profile Display Connectors4x mDP 1.4 Max Simultaneous Displays4 direct, 4 DP 1.4 Multi-Stream Display Resolution4x 4096x2160 @ 60Hz 4x 5120x2880 @ 60Hz Graphics APIs Shader Model 5.1, OpenGL 4.53, DirectX 12.04, Vulkan 1.03Compute APIsCUDA, DirectCompute, OpenCL ™ UNMATCHED POWER.UNMATCHED CREATIVE FREEDOM. NVIDIA ® QUADRO ®P1000。
海康威视75英寸互动白板说明书
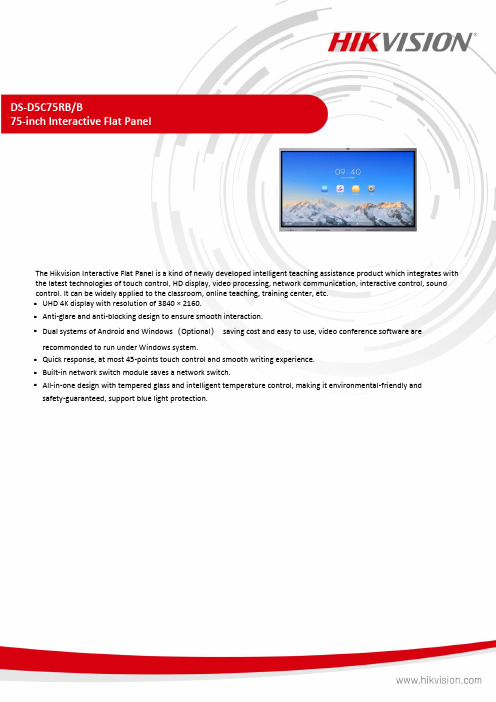
DS-D5C75RB/B75-inch Interactive Flat PanelThe Hikvision Interactive Flat Panel is a kind of newly developed intelligent teaching assistance product which integrates with the latest technologies of touch control, HD display, video processing, network communication, interactive control, sound control. It can be widely applied to the classroom, online teaching, training center, etc.⏹UHD 4K display with resolution of 3840 × 2160.⏹Anti-glare and anti-blocking design to ensure smooth interaction.⏹Dual systems of Android and Windows(Optional)saving cost and easy to use, video conference software arerecommonded to run under Windows system.⏹Quick response, at most 45-points touch control and smooth writing experience.⏹Built-in network switch module saves a network switch.⏹All-in-one design with tempered glass and intelligent temperature control, making it environmental-friendly andsafety-guaranteed, support blue light protection.SpecificationModel DS-D5C75RB/BProduct Model ProductModelDS-D5C75RB/BPanel GlassThickness3.2 mmGlass BondingMethodZero LaminationDisplay Screen Size 75 inchBacklight DLEDPixel Pitch 0.14325(H) × 0.42975(V) mm Resolution 3840 × 2160 @60 Hz Brightness 400 cd/m² with Brightness sensor Color Depth 10 bitContrast Ratio 4000 : 1ResponseTime8 msColor Gamut 72% NTSCRefresh Rate 60 HzViewing Angle 178°(H)/178°(V)Optical Aspect Ratio 16 : 9Built-in System OperationSystemAndroid 12.0Processor Quad-core Cortex-A76 × 4(2.4 GHz)and quad-core Cortex-A55 × 4(1.8 GHz)Memory 8 GBBuilt-inStorage128 GBPanel Type VAHDCP Version 2.0TouchResolution3840 × 2160Touch Type Infrared Touch Screen Touch Point 45 point multi-touchTouchResponseTime< 8 msTouchPrecision± 1 mm (≥ 90% touching area)ProcessingPerformanceDisplay Color 1.07GInternal Function Loudspeaker Built-in 2 ×15 W+25 W loudspeaker;Bluetooth Built-in BLE (Bluetooth Low Energy) module supports Bluetooth 5.1ImageProcessingSupport 3D digital noise reduction, face detection, automatic white balance, automatic purpleedge removal, automatic defogging;support shadow correction and distortion correction forTechnology lens.Audio Processing Technology Support dereverberation, adaptive noise reduction, adaptive non-stationary noise reduction, adaptive echo cancellation, automatic gain control, automatic electrical level control, sound source localization, speech dynamic detection, digital beam forming, speech detection.Interface Video &Audio InputHDMI In 2.0 × 3, VGA In × 1, DP In 1.2 × 1, Audio In × 1Video &audio outputHDMI Out 2.0 × 1, Audio Out × 1, SPDIF Out × 1ControlinterfaceTOUCH-USB 3.0 × 3, RS232 × 1NetworkInterfaceLAN (1000 Mbps) × 2WIFI AP&Station,2.4 G/5 GIEEE 802.11 a/b/g/n/ac 2 × 2 MIMO (2.4 GHz and 5 GHz) authentication protocols WEP, WPA,WPA2, PSK and 802.1X EAPDataTransmissionInterfaceUSB 3.0 × 4, USB 2.0 × 1, Type-C × 2 (Type-C supports USB 2.0, DP 1.2, and chargingfunctions; side charging 15 W, front charging 65 W)Power Standby Consumption≤ 0.5 W Max.Consumption< 250WWorking Environment WorkingTemperature0 °C to 40 °C (32 °F to 104°F) WorkingHumidity10%~90% RHGeneral VESA 800 mm × 400 mm (4 × M8-25 mm)Power Supply 100 to 240 VAC, 50/60 HzProductdimensions1713.2 × 1034.5 × 82.5AccessoryWall mount bracket, hook, user manual, touch writing pen, packing list, 3M touch USB cable,3M HDMI cable (2.0), 1.5m power cable (European standard), Bluetooth remote control. PackageDimensions(W × H × D)1937 × 264 × 1222 mmNet Weight 61.86Gross Weight 73.36NFC Support screen mirroring by Android phones.Camera Pixel 8 MPCameraFunctionSupport intelligent switching between Android system, OPS, and PC. Field Angle 120°(diagonal), 110°(horizontal), 75°(vertical)Distortion ≤2.5%CameraResolutionRatioUp to 4KMicrophone Specification Omni-directional 8-array layoutMic Function Echo reduction and smart noise-cancellation VoiceDistance12 mSampling Rate 32 KMic SamplingRate16 bit⏹Physical InterfaceInterface Description Interface Description1.SPDIF SPDIF interface2.Line OUT Audio output interface3.Line IN Audio input interface4.VGA IN Video devices connectioninterface5.TYPE-C Type-C interface6.DP IN Display input interface7.TOUCH-USB 2Peripheral touch interface8.HDMI IN 2HDMI 2 input interface9.TOUCH-USB Peripheral touch interface10.HDMI IN 1HDMI 1 input interface B USB interface12.MULTI-USB 2Multi-functional USBinterface13.MULTI-USB 1Multi-functional USBinterface14.HDMI OUT HDMI output interface⏹InstallationAvailable Model DS-D5C75RB/BDimension。
- 1、下载文档前请自行甄别文档内容的完整性,平台不提供额外的编辑、内容补充、找答案等附加服务。
- 2、"仅部分预览"的文档,不可在线预览部分如存在完整性等问题,可反馈申请退款(可完整预览的文档不适用该条件!)。
- 3、如文档侵犯您的权益,请联系客服反馈,我们会尽快为您处理(人工客服工作时间:9:00-18:30)。
Computer Science DepartmentTechnical ReportNWU-CS-04-47Oct 28, 2004Temporally Adaptive Frameless RenderingAbhinav Dayal, Cliff Woolley, David Luebke, Benjamin WatsonAbstractRecent advances in computational power and algorithmic sophistication have made ray tracing an increasingly viable and attractive algorithm for interactive rendering. Assuming that these trends will continue, we are investigating novel rendering strategies that exploit the unique capabilities of interactive ray tracing. Specifically, we propose a system that adapts rendering effort spatially, sampling some image regions more densely than others, and temporally, sampling some regions more often than others. Our system revisits and extends Bishop et al.'s frameless rendering with new approaches to sampling and reconstruction. We make sampling both spatially and temporally adaptive, using closed loop feedback from the current state of the image to continuously guide sampling toward regions of the image with significant change over space or time. We then send these frameless samples in a continuous stream to a temporally deep buffer, which stores all the samples created over a short time interval. The image to be displayed is reconstructed from this deep buffer. Reconstruction is also temporally adaptive, responding both to sampling density and color gradient. Where the displayed scene is static, spatial color change dominates and older samples are given significant weight in reconstruction, resulting in sharper images. Where the scene is dynamic, more recent samples are emphasized, resulting in a possibly blurry but up-to-date image. We describe a CPU-based implementation that runs at near-interactive rates on current hardware, and analyze simulations of the real-time performance we expect from future hardware-accelerated implementations. Our analysis accounts for temporal as well as spatial error by comparing displayed imagery across time to a hypothetical ideal renderer capable of instantaneously generating optimal frames. From these results we argue that the temporally adaptive approach is not only more accurate than frameless rendering, but also more accurate than traditional framed rendering at a given sampling rate.Keywords:[Computer Graphics]: Display algorithms, Raytracing, Virtual reality, frameless rendering, adaptive rendering, reconstruction, sampling, global illumination.Technical Report(NWU-CS-04-47)Northwestern University(Evanston,IL)Temporally Adaptive Frameless RenderingAbhinav Dayal†,Cliff Woolley‡,David Luebke‡,and Benjamin Watson††Department of Computer Science,Northwestern University‡Department of Computer Science,University of VirginiaAbstractRecent advances in computational power and algorithmic sophistication have made ray tracing an increasingly viable and attractive algorithm for interactive rendering.Assuming that these trends will continue,we are inves-tigating novel rendering strategies that exploit the unique capabilities of interactive ray tracing.Specifically,we propose a system that adapts rendering effort spatially,sampling some image regions more densely than others, and temporally,sampling some regions more often than others.Our system revisits and extends Bishop et al.’s frameless rendering with new approaches to sampling and reconstruction.We make sampling both spatially and temporally adaptive,using closed loop feedback from the current state of the image to continuously guide sampling toward regions of the image with significant change over space or time.We then send these frameless samples ina continuous stream to a temporally deep buffer,which stores all the samples created over a short time interval.The image to be displayed is reconstructed from this deep buffer.Reconstruction is also temporally adaptive,re-sponding both to sampling density and color gradient.Where the displayed scene is static,spatial color change dominates and older samples are given significant weight in reconstruction,resulting in sharper images.Where the scene is dynamic,more recent samples are emphasized,resulting in a possibly blurry but up-to-date image.We describe a CPU-based implementation that runs at near-interactive rates on current hardware,and analyze sim-ulations of the real-time performance we expect from future hardware-accelerated implementations.Our analysis accounts for temporal as well as spatial error by comparing displayed imagery across time to a hypothetical ideal renderer capable of instantaneously generating optimal frames.From these results we argue that the temporally adaptive approach is not only more accurate than frameless rendering,but also more accurate than traditional framed rendering at a given sampling rate.Categories and Subject Descriptors(according to ACM CCS):I.3.3[Computer Graphics]:Picture/Image Generation1.Improving interactive renderingIn recent years a number of traditionally offline rendering al-gorithms have become feasible in the interactive realm.The sudden appearance of programmable high-precision graph-ics processors(GPUs)has drastically expanded the range of algorithms that can be employed in real-time graphics; meanwhile,the steady progress of Moore’s Law has made techniques such as ray tracing,long considered a slow algo-rithm suited only for offline realistic rendering,feasible in real-time rendering settings[23]:.These trends are related; indeed,some of the most promising research on interactive global illumination performs algorithms such as ray tracing and photon mapping directly on the GPU[18,19].Future hardware should provide even better support for these algo-rithms,quickening the day when ray-based algorithms are an accepted and powerful component of every production ren-dering system.What makes interactive ray tracing attractive? Researchers in the area have commented on the ray tracing’s ability to model physically correct global illumination phe-nomena,its easy applicability to different shaders and primi-tive types,and its output-sensitive running time,only weakly dependent on scene complexity[25].We focus on another unique capability available in a ray-based renderer but not a depth-buffered rasterizer.We believe that the ability of in-teractive ray tracing to selectively sample the image plane enables a new approach to rendering that is more interactive, more accurate,and more portable.To achieve these goals, we argue that the advent of real-time ray tracing demandsc Northwestern University NWU-CS-04-47.1Figure1:Adaptive frameless sampling of a moving car in a static scene.Left,the newest samples in each pixel region.Middle, the tiles in the spatial hierarchy.Note small tiles over car and high contrast edges.Right,the per-tile derivative term,which very effectively focuses on the car.a rethinking of the fundamental sampling strategies used in computer graphics.The topic of sampling in ray tracing,and related ap-proaches such as path tracing,may seem nearly exhausted, but almost all previous work has focused on spatial sam-pling,or where to sample in the image plane.In an interac-tive setting,the question of temporal sampling,or when to sample with respect to user input,becomes equally impor-tant.Temporal sampling in traditional graphics is bound to the frame:an image is begun in the back buffer incorporat-ing the latest user input,but by the time the frame is swapped to the front buffer for display,the image reflects stale input. To mitigate this,interactive rendering systems increase the frame rate by reducing the complexity of the scene,trad-ing offfidelity for performance.We consider this tradeoff in terms of spatial error and temporal error.Spatial error is caused by rendering coarse approximations for speed,and includes such factors as resolution of the rendered image and geometric complexity of the rendered models.Tempo-ral error is caused by the delay imposed by rendering,and includes such factors as how often the image is generated (frame rate)and how long the image takes to render and dis-play(latency).In this paper we investigate novel sampling schemes for managing thefidelity-performance tradeoff.Our approach has two important implications.First,we advocate adaptive temporal sampling,analogous to the adaptive spatial sam-pling that takes place in progressive ray tracing[16,2,14]. Just as spatially adaptive renderers display detail where it is most important,adaptive temporal sampling displays de-tail when it is most important.Second,we advocate frame-less rendering[3],in which samples are not collected into coherent frames for double-buffered display,but instead are incorporated immediately into the image.Frameless render-ing,which requires a per-sample rendering algorithm such as real-time ray tracing,decouples spatial and temporal updates and thus enables veryflexible adaptive spatial and temporal sampling.Our prototype adaptive frameless render is broken into three primary sub-systems.An adaptive sampler directs ren-dering to image regions undergoing significant change(in space and/or time).The sampler produces a stream of sam-ples scattered across space-time;recent samples are col-lected and stored in a temporally deep buffer.An adap-tive reconstructor repeatedly reconstructs the samples in the deep buffer into an image for display,adapting the recon-structionfilter to local sampling density and color gradients. Where the displayed scene is static,spatial color change dominates and older samples are given significant weight in reconstruction,resulting in sharper images.Where the scene is dynamic,only more recent samples are emphasized,re-sulting in a possibly blurry but up-to-date image.We describe the design of an interactive system built on these principles,and show in simulation that this system achieves superior rendering accuracy and responsiveness. We evaluate our system with a“gold standard”analysis that compares displayed imagery to the ideal image that would be displayed by a hypothetical ideal renderer,evaluating the im-age difference at using mean RMS error and and show that it outperforms not only the pseudorandom frameless sam-pling of Bishop et al.[3],but also traditional framed sam-pling strategies with the same overall sampling rate.Since our approach is self-monitoring,we also argue that it can achieve a new level of portability and adaptivity to changes in platform and load.2.Related work2.1.Interactive ray tracingRecent years have seen interactive ray tracing go from an oxymoron to a reality.Interactive ray tracers have been demonstrated on supercomputers[17],PC clusters[26],onc Northwestern University NWU-CS-04-47.2the SIMD instruction sets of modern CPUs[24],and on graphics hardware[18][4].Wald et al.provide a good sum-mary of the state of the art[25].To build an interactive ray tracer,while hardly simple,is becoming a matter of engi-neering.Looking forward,hardware and software systems are being developed[21][10]that help harness the render-ing power of PC clusters,and consumer graphics hardware is growing more powerful and moreflexible at an astonishing rate.Real-time ray tracing is currently feasible;we believe it will soon become commonplace even on commodity desk-top hardware.2.2.Interruptible renderingRecent work on temporally adaptive sampling includes a new approach tofidelity control called interruptible render-ing[30]that adaptively controls frame rate to minimize the sum of spatial and temporal error.They propose a progres-sive rendering framework that renders a coarse image into the back buffer and continuously refines it,while tracking the error introduced by subsequent input(such as changes in viewpoint).When this temporal error exceeds the spatial er-ror caused by coarse rendering,there is no longer any reason to refine further,since any improvement to the appearance of objects in the image will be overwhelmed by their incor-rect position and/or size.In other words,further refinement becomes pointless when the error due to the image being late is greater than the error due to the image being coarse. The front and back buffers are then swapped and rendering begins again into the back buffer for the most recent view-point.The resulting system produces coarse,high frame-rate display when input is changing rapidly,andfinely detailed, low frame rate display when input is static.2.3.Frameless renderingInterruptible rendering retains a basic underlying assump-tion of interactive computer graphics:all pixels in a given image represent a single moment in time(or possibly afixed duration surrounding that moment,e.g.the“shutter speed”used for motion blur).When the system swaps buffers,all pixels in the image are simultaneously replaced with pix-els representing a different moment in time.In interactive settings,this coherent temporal sampling strategy has sev-eral unfortunate perceptual consequences:temporal alias-ing,delay,and temporal discontinuity.Temporal aliasing re-sults when the sampling rate is inadequate to capture high speed motion.Motion blur techniques can compensate for this aliasing,but are generally so expensive that in interac-tive settings they actually worsen the problem.Delay is a byproduct of double buffering,which avoids tearing(simul-taneous display of two partial frames)at the cost of ensuring that each displayed scene is at least two frames old before it is swapped out.Even at a60Hz frame rate,this introduces 33ms of delay–a level that human factors researchers have consistently shown can harm task performance[29][20].Fi-nally,when frame rates fall below60Hz,the perceptual sen-sation of image continuity is broken,resulting in display of choppy or“jerky”looking motion.Interruptible rendering performs adaptive temporal sam-pling to achieve higher accuracy,but that sampling is still coherent:all pixels(or,more generally,spatial samples) still represent the same moment in time.We have since fo-cused our research on the unique opportunities for tempo-rally adaptive rendering presented by Bishop et al.’s frame-less rendering[3].This novel rendering strategy replaces the coherent,simultaneous,double-buffered update of all pixels with stochastically distributed spatial samples,each repre-senting the most current input when the sample was taken. Frameless rendering thus decouples spatial and temporal sampling,so that the pixels in a frameless image represent many moments in time.We build on and improve the original frameless rendering approach.Our rendering system samples rapidly changing regions of an image coarsely but frequently to reduce tempo-ral error,while refining static portions of the image to reduce spatial error.We improve the displayed image by perform-ing a reconstruction step,filtering samples in space and time so that older samples are weighted less than recent samples; reconstruction adapts to the local sampling density and color gradient to optimize thefilter width for different parts of the scene.In the following sections we describe our temporally adaptive sampling and reconstruction strategies,and discuss their implementation in a simulated prototype system.We next describe the“gold standard”evaluation technique and analyze our prototype against traditional rendering as well as an“oracle”system that knows which samples are valid and which contain stale information.We close by discussing fu-ture directions and argue that frameless,temporally adaptive systems will ultimately provide more interactive,accurate, portable rendering.3.Temporally adaptive,closed-loop samplingWhile traditional frameless sampling is unbiased,we make our frameless renderer adaptive to improve rendering qual-ity.Sampling is both spatially adaptive,focusing on regions where color changes across space;and temporally adaptive, focusing on regions where color changes over time(Fig-ure1).As in previous spatially adaptive rendering methods [16][2][14][9],adaptive bias is added to sampling with the use of a spatial hierarchy of tiles superimposed over the view.However,while previous methods operated in the static context of a single frame,we operate in a dynamic frameless context.This is has several implications.First, rather than operating on a frame buffer,we send samples to a temporally deep buffer that collects samples scattered across space-time.Our tiles therefore partition a space-time vol-ume using planes parallel to the temporal axis.As in framedc Northwestern University NWU-CS-04-47.3schemes,color variation within each tile guides rendering bias,but variation represents change over not just space but also time.Moreover,variation is not monotonically decreas-ing as the renderer increases the number of tiles,but con-stantly changing in response to user interaction and anima-tion.Therefore the hierarchy is also constantly changing, with tiles continuously merged and split in response to dy-namic changes in the contents of the deep buffer.We implement our dynamic spatial hierarchy using a K-D tree.Given a target number of tiles,the tree is managed to en-sure that the amount of color variation per unit space-time in each tile is roughly equal:the tile with the most color varia-tion is split and the two tiles with the least summed variation are merged,until all tiles have roughly equal variation.As a result,small tiles are located over buffer regions with signif-icant change orfine spatial detail,while large tiles emerge over static or coarsely detailed regions(Figure1). Sampling then becomes a biased,probabilistic process. Since time is notfixed as in framed renderers,we cannot simply iteratively sample the tile with the most variation per unit space-time–in doing so,we would overlook newly emerging motion and detail.At the same time,we cannot leave rendering unbiased and unimproved.Our solution is to sample each tile with equal probability,and select the sampled location within the tile using a uniform distribu-tion.Because tiles vary in size,sampling is biased towards those regions of the image which exhibit high spatial and/or temporal variance.Because all tiles are sampled,we remain sensitive to newly emerging motion and detail.This sampler is in fact a closed loop control system[7], capable of adapting to user input with greatflexibility.In control theory,the plant is the process being directed by the compensator,which must adapt to external disturbance. Output from the plant becomes input for the compensator, closing the feedback loop.In a classic adaptive framed sam-pler,the compensator chooses the rendered location,the ray tracer is the plant that must be controlled,and disturbance is Figure2:Adaptive frameless sampling as closed loop con-trol.Output sample from the ray tracer(plant)is sent to an error tracker,which adjusts the spatial tiling or error map. As long as the error map is not zero everywhere,the adap-tive sampler(compensator)selects one tile to render,and one location in the tile.Constantly changing user input(dis-turbance)makes it very difficult to limiterror.Figure3:A snapshot of color gradients in the car scene. Green and red are spatial gradients,blue is the temporal gradient.Here the spatial gradients dominate,and the num-ber of tiles is fairly high.provided by the scene as viewed at the time being rendered. Our frameless sampler(Figure2)faces a more difficult chal-lenge:view and scene state may change after each sample. Unfortunately,a ray tracer is extremely nonlinear and highly multidimensional,and therefore very difficult to analyze us-ing control theoretic techniques.Nevertheless,more pragmatic control engineering tech-niques may be applied.One such technique is the use of PID controllers,in which control may respond in proportion to error itself(P),to its integral(I),and to its derivative(D). In our sampler,error is color variation–if it were small enough,we could assume that rendering was complete.In biasing sampling toward variation,we are already respond-ing in proportion to error.However,we have also found it useful to respond to error’s derivative.By biasing sampling toward regions in which variation is changing,we compen-sate for delay in our control system and direct sampling to-ward changing regions of the deep buffer,such as the edges of the car in Figure1.We accomplish this by tracking vari-ation change d,and adding it to variation itself p to form a new summed control error:e=kp+(1−k)d,where k in the range[0,1]is the weight applied to the proportional term.The right image in Figure1visualizes d for each tile by mapping high d values to high gray levels.Our prototype adaptive sampler will be less effective when the rendered scene is more dynamic.In control the-ory,one way of compensating for varying rates of change in the target signal is to adjust gain,thereby damping or am-c Northwestern University NWU-CS-04-47.4plifying the impact of control on the plant.A similar sort of compensation is possible in our sampling control system by restricting or increasing the ability of the sampler to adapt to deep buffer content.We implement this approach by ad-justing the number of tiles in the K-D tree according to the ratio of color change over time to color change across space. We achieve this by ensuring that dC/dsS=dC/dtT,where dC/ds and dC/dt are color change over space and time(Fig-ure3),S is the average width of the tiles,and T the average age of the samples in each tile.By solving for S we can de-rive the current number of tiles that would be appropriate.4.Space-time reconstruction for interactive rendering Frameless sampling strategies demand a rethinking of the traditional computer graphics concept of an“image”,since at any given moment the samples in an image plane repre-sent many different moments in time.The original frame-less work[3]simply displayed the most recent sample at every pixel,a strategy we refer to as traditional reconstruc-tion of the frameless sample stream.The result is a noisy, pixelated image which appears to sparkle or scintillate as the underlying scene changes(see Figure4).Instead we store a temporally deep buffer of recent frameless samples, and continuously reconstruct images for display by convolv-ing the samples with a space-timefilter.This is similar to the classic computer graphics problem of reconstruction of an image from non-uniform samples[14],but with a tem-poral element:since older samples may represent“stale”data,they are treated with less confidence and contribute less to nearby pixels than more recent samples.The resulting images greatly improve over traditional reconstruction(see again Figure4).The key question is what shape and sizefilter to use.A temporally narrow,spatially broadfilter(i.e.afilter which falls off rapidly in time but gradually in space)will give very little weight to relatively old samples;such afilter em-phasizes the newest samples and leads to a blurry but very current image.Such afilter provides low-latency response to changes and should be used when the underlying image is changing rapidly(Figure4,right member of leftmost pair).A temporally broad,spatially narrowfilter will give nearly as much weight to relatively old samples as to recent sam-ples;such afilter accumulates the results of many samples and leads to afinely detailed,antialiased image when the underlying scene is changing slowly(Figure4,right mem-ber of rightmost pair).However,often different regions of an image change at different rates;for example,in a sta-tionary view in which an object is moving across a static background.A scene such as this demands spatially adap-tive reconstruction,in which thefilter width varies across the image.What should guide this process?We use local sampling density and space-time gradient information to guidefilter size.The sampler provides an es-timate of sampling density for an image region,based on the overall sampling rate and on the tiling used to guide sampling.We size ourfilter–which can be interpreted as a space-time volume–as if we were reconstructing a regular sampling with this local sampling density,and while pre-serving the total volume of thefilter perturb thefilter widths according to local gradient information.We reason that a large spatial gradient implies an edge,which should be re-solved with a narrowfilter to preserve the underlying high frequencies.Similarly,a large temporal gradient implies a “temporal edge”such as an occlusion event,which should be resolved with a narrowfilter to avoid including stale sam-ples from before the event.What function to use for thefilter kernel remains an open question.Signal theory tells us that for a regularly sampled bandlimited function,ideal reconstruction should use a sinc function,but our deep buffer is far from regularly sampled and the underlying signal(an image of a three-dimensional scene)contains high-frequency discontinuities such as oc-clusion boundaries.We currently use an inverse exponential filter so that the relative contribution of two samples does not change as both grow older;however,the bandpass properties of thisfilter are less than ideal.We would like to investigate multistage approaches inspired by the classic Mitchellfilter [14].Our implementation of a deep buffer stores the last n sam-ples within each pixel;typical values of n range from1to8. As samples arrive they are bucketed into pixels and added to the deep buffer,displacing the oldest sample in that pixel; average gradient information is also updated incrementally as samples arrive.At display time a reconstruction process adjusts thefilter size and widths at each pixel as described (using gradient and local sample density)and gathers sam-ples“outwards”in space and time until the maximum possi-ble incremental contribution of additional samples would be less than some thresholdε(ε=1%in our case).Thefinal color at that pixel is computed as the normalized weighted average of sample colors.This process is expensive–our simulation requires reconstruction times of a few hundred ms for small(256×256)image sizes–so we are investi-gating several techniques to accelerate reconstruction.One currentlykey technique will be to implement the reconstruc-tion process directly on the graphics hardware,and we have a prototype implementation of a GPU-based reconstructor in which the deep buffer is represented as a texture with sam-ples interleaved in columns;samples are added to the buffer by rendering points with a special pixel shader enabled.At display time the system reconstructs an image by drawing a single screen-sized quad with a(quite elaborate)pixel shader that reads andfilters samples from the deep buffer texture. Though not yet fully adaptive,our initial GPU implementa-tion provides a promising speedup(more than an order of magnitude)over the CPU version.We plan to revisit this implementation,which is far from optimized,and hope for another order of magnitude to allow the system to achieve interactive frame rates on realistic image resolutions.c Northwestern University NWU-CS-04-47.5Figure4:Adaptive reconstructions.The left pair shows a dynamic scene,with the traditional frameless reconstruction on the left and the adaptive reconstruction on the right.The right pair shows a static scene,with the traditional reconstruction once more on the left and the adaptive reconstruction on the right.5.Gold standard evaluationUsing the gold standard validation described in[30],wefind that our adaptive frameless renderer consistently out-performs other renders that have the same sampling rates.Gold standard validation uses as its standard an ideal ren-derer I capable of rendering imagery in zero time.To per-form comparisons to this standard,we create n ideal imagesI j(j in[1,n])at60Hz for a certain animation A using a sim-ulated ideal renderer.We then create n more images R j foranimation A using an actual interactive renderer R.We nextcompare each image pair(I j,R j)using an image comparisonmetric comp,and average the resulting image differences:1/nΣn1comp(I j,R j).Note that if this comparison metric isroot mean squared(RMS)error,this result is very closely re-lated to the peak signal-to-noise ratio(PSNR),a commonlyused measure of video quality.We report the results of our gold standard evaluation us-ing PSNR in Figure5below.In thefigure,we compare sev-eral rendering methods.Two framed renderings either max-imize Hz at the cost of spatial resolution(lo-res),or spatialresolution at the cost of Hz(hi-res).The traditional frame-less rendering uses a pseudorandom non-adaptive samplerand simply displays the newest sample at a given pixel.Theadaptive frameless renderings come in two groups:one thatuses afixed number of tiles(256),and one that uses a vari-able number of tiles,as determined by balance between spa-tial and temporal change in the sample stream.In both thefixed and variable groups,there are three biases in responseto sampling:biased toward color change itself(k=.8)(P),to-ward the derivative of color change(k=.2)(D),or balanced.Rendering methods were tested in3different animations:thepublicly available BART testbed[12];a closeup of a toy carin the same testbed,and a dynamic interactive recording.Allof these animations were rendered at sampling rates of100Kpixels per second,two at1M pixels per second.Adaptive frameless rendering is the clear winner,withhigh PSNRs throughout.In the largely static Toycar stream,it made almost no difference whether adaptive frameless ren-dering used afixed or variable number of tiles.But in theother more dynamic streams,the variable number of tilesconsistently had a small edge.Responding to the deriva-tive of color change was slightly more effective when thescene was static,but the pattern here was less clear.In allcases however,adaptive frameless rendering was better thanframed or traditional frameless rendering.A quick glance at Figure6confirms this impression.These graphs show frame by frame comparisons using RMSbetween many of these rendering techniques and the idealrendering.Adaptive frameless rendering is the consistentwinner with traditional frameless and framed renderings al-most always with more error.The lone exception is in theBART100K stream,when the animation begins with a verydynamic content and uses sampling rate20x too slow to sup-port60Hz at full resolution.In addition to evaluating the ability of our entire adap-tive renderer to approximate a hypothetical ideal renderer,we can evaluate the ability of our reconstruction sub-systemto approximate a hypothetical ideal reconstructor.This sam-ple oracle evaluation generates crucial and detailed feedbackfor the development of both the reconstruction sub-systemand by extension,the entire rendering system.Sample ora-cle evaluation is identical to gold standard evaluation in allrespects save one:rather than comparing the reconstructedinteractive image R j to a corresponding ideal image I j,itRender Method Toycar 100K Toycar 1M BART 100K BART 1M Dynamic 100KFramed: lo-res11.2615.8210.1612.6910.35Framed: hi-res9.0115.38 4.587.517.54Traditional frameless16.7118.07 6.319.9710.54Adaptive: fixed (k=0.2)18.111910.3515.0813.95Adaptive: fixed (k=0.5)18.0118.9910.415.3914.17Adaptive: fixed (k=0.8)17.8618.9310.2115.5814.13Adaptive: var (k=0.2)18.1518.9710.8615.0714.04Adaptive: var (k=0.5)18.0118.961115.4214.31Adaptive: var (k=0.8)17.8518.9210.9515.714.36Animation / Sampling RateFigure5:A comparison of several rendering techniques toan ideal rendering using peak signal to noise ratio(PSNR),across three animations and two sampling rates.c Northwestern University NWU-CS-04-47. 6。