Abstract Exploiting network proximity in peer-to-peer overlay networks
人工智能英文参考文献(最新120个)
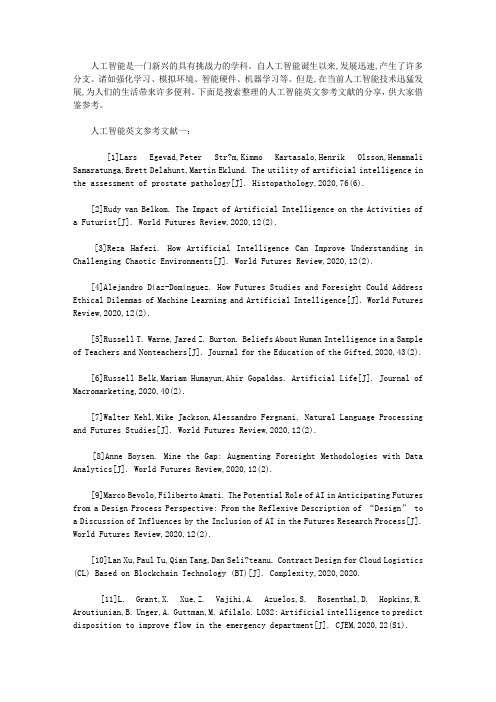
人工智能是一门新兴的具有挑战力的学科。
自人工智能诞生以来,发展迅速,产生了许多分支。
诸如强化学习、模拟环境、智能硬件、机器学习等。
但是,在当前人工智能技术迅猛发展,为人们的生活带来许多便利。
下面是搜索整理的人工智能英文参考文献的分享,供大家借鉴参考。
人工智能英文参考文献一:[1]Lars Egevad,Peter Str?m,Kimmo Kartasalo,Henrik Olsson,Hemamali Samaratunga,Brett Delahunt,Martin Eklund. The utility of artificial intelligence in the assessment of prostate pathology[J]. Histopathology,2020,76(6).[2]Rudy van Belkom. The Impact of Artificial Intelligence on the Activities ofa Futurist[J]. World Futures Review,2020,12(2).[3]Reza Hafezi. How Artificial Intelligence Can Improve Understanding in Challenging Chaotic Environments[J]. World Futures Review,2020,12(2).[4]Alejandro Díaz-Domínguez. How Futures Studies and Foresight Could Address Ethical Dilemmas of Machine Learning and Artificial Intelligence[J]. World Futures Review,2020,12(2).[5]Russell T. Warne,Jared Z. Burton. Beliefs About Human Intelligence in a Sample of Teachers and Nonteachers[J]. Journal for the Education of the Gifted,2020,43(2).[6]Russell Belk,Mariam Humayun,Ahir Gopaldas. Artificial Life[J]. Journal of Macromarketing,2020,40(2).[7]Walter Kehl,Mike Jackson,Alessandro Fergnani. Natural Language Processing and Futures Studies[J]. World Futures Review,2020,12(2).[8]Anne Boysen. Mine the Gap: Augmenting Foresight Methodologies with Data Analytics[J]. World Futures Review,2020,12(2).[9]Marco Bevolo,Filiberto Amati. The Potential Role of AI in Anticipating Futures from a Design Process Perspective: From the Reflexive Description of “Design” to a Discussion of Influences by the Inclusion of AI in the Futures Research Process[J]. World Futures Review,2020,12(2).[10]Lan Xu,Paul Tu,Qian Tang,Dan Seli?teanu. Contract Design for Cloud Logistics (CL) Based on Blockchain Technology (BT)[J]. Complexity,2020,2020.[11]L. Grant,X. Xue,Z. Vajihi,A. Azuelos,S. Rosenthal,D. Hopkins,R. Aroutiunian,B. Unger,A. Guttman,M. Afilalo. LO32: Artificial intelligence to predict disposition to improve flow in the emergency department[J]. CJEM,2020,22(S1).[12]A. Kirubarajan,A. Taher,S. Khan,S. Masood. P071: Artificial intelligence in emergency medicine: A scoping review[J]. CJEM,2020,22(S1).[13]L. Grant,P. Joo,B. Eng,A. Carrington,M. Nemnom,V. Thiruganasambandamoorthy. LO22: Risk-stratification of emergency department syncope by artificial intelligence using machine learning: human, statistics or machine[J]. CJEM,2020,22(S1).[14]Riva Giuseppe,Riva Eleonora. OS for Ind Robots: Manufacturing Robots Get Smarter Thanks to Artificial Intelligence.[J]. Cyberpsychology, behavior and social networking,2020,23(5).[15]Markus M. Obmann,Aurelio Cosentino,Joshy Cyriac,Verena Hofmann,Bram Stieltjes,Daniel T. Boll,Benjamin M. Yeh,Matthias R. Benz. Quantitative enhancement thresholds and machine learning algorithms for the evaluation of renal lesions using single-phase split-filter dual-energy CT[J]. Abdominal Radiology,2020,45(1).[16]Haytham H. Elmousalami,Mahmoud Elaskary. Drilling stuck pipe classification and mitigation in the Gulf of Suez oil fields using artificial intelligence[J]. Journal of Petroleum Exploration and Production Technology,2020,10(10).[17]Rüdiger Schulz-Wendtland,Karin Bock. Bildgebung in der Mammadiagnostik –Ein Ausblick <trans-title xml:lang="en">Imaging in breast diagnostics—an outlook [J]. Der Gyn?kologe,2020,53(6).</trans-title>[18]Nowakowski Piotr,Szwarc Krzysztof,Boryczka Urszula. Combining an artificial intelligence algorithm and a novel vehicle for sustainable e-waste collection[J]. Science of the Total Environment,2020,730.[19]Wang Huaizhi,Liu Yangyang,Zhou Bin,Li Canbing,Cao Guangzhong,Voropai Nikolai,Barakhtenko Evgeny. Taxonomy research of artificial intelligence for deterministic solar power forecasting[J]. Energy Conversion and Management,2020,214.[20]Kagemoto Hiroshi. Forecasting a water-surface wave train with artificial intelligence- A case study[J]. Ocean Engineering,2020,207.[21]Tomonori Aoki,Atsuo Yamada,Kazuharu Aoyama,Hiroaki Saito,Gota Fujisawa,Nariaki Odawara,Ryo Kondo,Akiyoshi Tsuboi,Rei Ishibashi,Ayako Nakada,Ryota Niikura,Mitsuhiro Fujishiro,Shiro Oka,Soichiro Ishihara,Tomoki Matsuda,Masato Nakahori,Shinji Tanaka,Kazuhiko Koike,Tomohiro Tada. Clinical usefulness of a deep learning‐based system as the first screening on small‐bowel capsule endoscopy reading[J]. Digestive Endoscopy,2020,32(4).[22]Masashi Fujii,Hajime Isomoto. Next generation of endoscopy: Harmony with artificial intelligence and robotic‐assisted devices[J]. Digestive Endoscopy,2020,32(4).[23]Roberto Verganti,Luca Vendraminelli,Marco Iansiti. Innovation and Design in the Age of Artificial Intelligence[J]. Journal of Product Innovation Management,2020,37(3).[24]Yuval Elbaz,David Furman,Maytal Caspary Toroker. Modeling Diffusion in Functional Materials: From Density Functional Theory to Artificial Intelligence[J]. Advanced Functional Materials,2020,30(18).[25]Dinesh Visva Gunasekeran,Tien Yin Wong. Artificial Intelligence in Ophthalmology in 2020: A Technology on the Cusp for Translation and Implementation[J]. Asia-Pacific Journal of Ophthalmology,2020,9(2).[26]Fu-Neng Jiang,Li-Jun Dai,Yong-Ding Wu,Sheng-Bang Yang,Yu-Xiang Liang,Xin Zhang,Cui-Yun Zou,Ren-Qiang He,Xiao-Ming Xu,Wei-De Zhong. The study of multiple diagnosis models of human prostate cancer based on Taylor database by artificial neural networks[J]. Journal of the Chinese Medical Association,2020,83(5).[27]Matheus Calil Faleiros,Marcello Henrique Nogueira-Barbosa,Vitor Faeda Dalto,JoséRaniery Ferreira Júnior,Ariane Priscilla Magalh?es Tenório,Rodrigo Luppino-Assad,Paulo Louzada-Junior,Rangaraj Mandayam Rangayyan,Paulo Mazzoncini de Azevedo-Marques. Machine learning techniques for computer-aided classification of active inflammatory sacroiliitis in magnetic resonance imaging[J]. Advances in Rheumatology,2020,60(1078).[28]Balamurugan Balakreshnan,Grant Richards,Gaurav Nanda,Huachao Mao,Ragu Athinarayanan,Joseph Zaccaria. PPE Compliance Detection using Artificial Intelligence in Learning Factories[J]. Procedia Manufacturing,2020,45.[29]M. Stévenin,V. Avisse,N. Ducarme,A. de Broca. Qui est responsable si un robot autonome vient à entra?ner un dommage ?[J]. Ethique et Santé,2020.[30]Fatemeh Barzegari Banadkooki,Mohammad Ehteram,Fatemeh Panahi,Saad Sh. Sammen,Faridah Binti Othman,Ahmed EL-Shafie. Estimation of Total Dissolved Solids (TDS) using New Hybrid Machine Learning Models[J]. Journal of Hydrology,2020.[31]Adam J. Schwartz,Henry D. Clarke,Mark J. Spangehl,Joshua S. Bingham,DavidA. Etzioni,Matthew R. Neville. Can a Convolutional Neural Network Classify Knee Osteoarthritis on Plain Radiographs as Accurately as Fellowship-Trained Knee Arthroplasty Surgeons?[J]. The Journal of Arthroplasty,2020.[32]Ivana Nizetic Kosovic,Toni Mastelic,Damir Ivankovic. Using Artificial Intelligence on environmental data from Internet of Things for estimating solar radiation: Comprehensive analysis[J]. Journal of Cleaner Production,2020.[33]Lauren Fried,Andrea Tan,Shirin Bajaj,Tracey N. Liebman,David Polsky,Jennifer A. Stein. Technological advances for the detection of melanoma: Part I. Advances in diagnostic techniques[J]. Journal of the American Academy of Dermatology,2020.[34]Mohammed Amoon,Torki Altameem,Ayman Altameem. Internet of things Sensor Assisted Security and Quality Analysis for Health Care Data Sets Using Artificial Intelligent Based Heuristic Health Management System[J]. Measurement,2020.[35]E. Lotan,C. Tschider,D.K. Sodickson,A. Caplan,M. Bruno,B. Zhang,Yvonne W. Lui. Medical Imaging and Privacy in the Era of Artificial Intelligence: Myth, Fallacy, and the Future[J]. Journal of the American College of Radiology,2020.[36]Fabien Lareyre,Cédric Adam,Marion Carrier,Juliette Raffort. Artificial Intelligence in Vascular Surgery: moving from Big Data to Smart Data[J]. Annals of Vascular Surgery,2020.[37]Ilesanmi Daniyan,Khumbulani Mpofu,Moses Oyesola,Boitumelo Ramatsetse,Adefemi Adeodu. Artificial intelligence for predictive maintenance in the railcar learning factories[J]. Procedia Manufacturing,2020,45.[38]Janet L. McCauley,Anthony E. Swartz. Reframing Telehealth[J]. Obstetrics and Gynecology Clinics of North America,2020.[39]Jean-Emmanuel Bibault,Lei Xing. Screening for chronic obstructive pulmonary disease with artificial intelligence[J]. The Lancet Digital Health,2020,2(5).[40]Andrea Laghi. Cautions about radiologic diagnosis of COVID-19 infection driven by artificial intelligence[J]. The Lancet Digital Health,2020,2(5).人工智能英文参考文献二:[41]K. Orhan,I. S. Bayrakdar,M. Ezhov,A. Kravtsov,T. ?zyürek. Evaluation of artificial intelligence for detecting periapical pathosis on cone‐beam computed tomography scans[J]. International Endodontic Journal,2020,53(5).[42]Avila A M,Mezi? I. Data-driven analysis and forecasting of highway traffic dynamics.[J]. Nature communications,2020,11(1).[43]Neri Emanuele,Miele Vittorio,Coppola Francesca,Grassi Roberto. Use of CT andartificial intelligence in suspected or COVID-19 positive patients: statement of the Italian Society of Medical and Interventional Radiology.[J]. La Radiologia medica,2020.[44]Tau Noam,Stundzia Audrius,Yasufuku Kazuhiro,Hussey Douglas,Metser Ur. Convolutional Neural Networks in Predicting Nodal and Distant Metastatic Potential of Newly Diagnosed Non-Small Cell Lung Cancer on FDG PET Images.[J]. AJR. American journal of roentgenology,2020.[45]Coppola Francesca,Faggioni Lorenzo,Regge Daniele,Giovagnoni Andrea,Golfieri Rita,Bibbolino Corrado,Miele Vittorio,Neri Emanuele,Grassi Roberto. Artificial intelligence: radiologists' expectations and opinions gleaned from a nationwide online survey.[J]. La Radiologia medica,2020.[46]?. ? ? ? ? [J]. ,2020,25(4).[47]Savage Rock H,van Assen Marly,Martin Simon S,Sahbaee Pooyan,Griffith Lewis P,Giovagnoli Dante,Sperl Jonathan I,Hopfgartner Christian,K?rgel Rainer,Schoepf U Joseph. Utilizing Artificial Intelligence to Determine Bone Mineral Density Via Chest Computed Tomography.[J]. Journal of thoracic imaging,2020,35 Suppl 1.[48]Brzezicki Maksymilian A,Bridger Nicholas E,Kobeti? Matthew D,Ostrowski Maciej,Grabowski Waldemar,Gill Simran S,Neumann Sandra. Artificial intelligence outperforms human students in conducting neurosurgical audits.[J]. Clinical neurology and neurosurgery,2020,192.[49]Lockhart Mark E,Smith Andrew D. Fatty Liver Disease: Artificial Intelligence Takes on the Challenge.[J]. Radiology,2020,295(2).[50]Wood Edward H,Korot Edward,Storey Philip P,Muscat Stephanie,Williams George A,Drenser Kimberly A. The retina revolution: signaling pathway therapies, genetic therapies, mitochondrial therapies, artificial intelligence.[J]. Current opinion in ophthalmology,2020,31(3).[51]Ho Dean,Quake Stephen R,McCabe Edward R B,Chng Wee Joo,Chow Edward K,Ding Xianting,Gelb Bruce D,Ginsburg Geoffrey S,Hassenstab Jason,Ho Chih-Ming,Mobley William C,Nolan Garry P,Rosen Steven T,Tan Patrick,Yen Yun,Zarrinpar Ali. Enabling Technologies for Personalized and Precision Medicine.[J]. Trends in biotechnology,2020,38(5).[52]Fischer Andreas M,Varga-Szemes Akos,van Assen Marly,Griffith L Parkwood,Sahbaee Pooyan,Sperl Jonathan I,Nance John W,Schoepf U Joseph. Comparison of Artificial Intelligence-Based Fully Automatic Chest CT Emphysema Quantification to Pulmonary Function Testing.[J]. AJR. American journal ofroentgenology,2020,214(5).[53]Moore William,Ko Jane,Gozansky Elliott. Artificial Intelligence Pertaining to Cardiothoracic Imaging and Patient Care: Beyond Image Interpretation.[J]. Journal of thoracic imaging,2020,35(3).[54]Hwang Eui Jin,Park Chang Min. Clinical Implementation of Deep Learning in Thoracic Radiology: Potential Applications and Challenges.[J]. Korean journal of radiology,2020,21(5).[55]Mateen Bilal A,David Anna L,Denaxas Spiros. Electronic Health Records to Predict Gestational Diabetes Risk.[J]. Trends in pharmacological sciences,2020,41(5).[56]Yao Xiang,Mao Ling,Lv Shunli,Ren Zhenghong,Li Wentao,Ren Ke. CT radiomics features as a diagnostic tool for classifying basal ganglia infarction onset time.[J]. Journal of the neurological sciences,2020,412.[57]van Assen Marly,Banerjee Imon,De Cecco Carlo N. Beyond the Artificial Intelligence Hype: What Lies Behind the Algorithms and What We Can Achieve.[J]. Journal of thoracic imaging,2020,35 Suppl 1.[58]Guzik Tomasz J,Fuster Valentin. Leaders in Cardiovascular Research: Valentin Fuster.[J]. Cardiovascular research,2020,116(6).[59]Fischer Andreas M,Eid Marwen,De Cecco Carlo N,Gulsun Mehmet A,van Assen Marly,Nance John W,Sahbaee Pooyan,De Santis Domenico,Bauer Maximilian J,Jacobs Brian E,Varga-Szemes Akos,Kabakus Ismail M,Sharma Puneet,Jackson Logan J,Schoepf U Joseph. Accuracy of an Artificial Intelligence Deep Learning Algorithm Implementing a Recurrent Neural Network With Long Short-term Memory for the Automated Detection of Calcified Plaques From Coronary Computed Tomography Angiography.[J]. Journal of thoracic imaging,2020,35 Suppl 1.[60]Ghosh Adarsh,Kandasamy Devasenathipathy. Interpretable Artificial Intelligence: Why and When.[J]. AJR. American journal of roentgenology,2020,214(5).[61]M.Rosario González-Rodríguez,M.Carmen Díaz-Fernández,Carmen Pacheco Gómez. Facial-expression recognition: An emergent approach to the measurement of tourist satisfaction through emotions[J]. Telematics and Informatics,2020,51.[62]Ru-Xi Ding,Iván Palomares,Xueqing Wang,Guo-Rui Yang,Bingsheng Liu,Yucheng Dong,Enrique Herrera-Viedma,Francisco Herrera. Large-Scale decision-making: Characterization, taxonomy, challenges and future directions from an Artificial Intelligence and applications perspective[J]. Information Fusion,2020,59.[63]Abdulrhman H. Al-Jebrni,Brendan Chwyl,Xiao Yu Wang,Alexander Wong,Bechara J. Saab. AI-enabled remote and objective quantification of stress at scale[J]. Biomedical Signal Processing and Control,2020,59.[64]Gillian Thomas,Elizabeth Eisenhauer,Robert G. Bristow,Cai Grau,Coen Hurkmans,Piet Ost,Matthias Guckenberger,Eric Deutsch,Denis Lacombe,Damien C. Weber. The European Organisation for Research and Treatment of Cancer, State of Science in radiation oncology and priorities for clinical trials meeting report[J]. European Journal of Cancer,2020,131.[65]Muhammad Asif. Are QM models aligned with Industry 4.0? A perspective on current practices[J]. Journal of Cleaner Production,2020,258.[66]Siva Teja Kakileti,Himanshu J. Madhu,Geetha Manjunath,Leonard Wee,Andre Dekker,Sudhakar Sampangi. Personalized risk prediction for breast cancer pre-screening using artificial intelligence and thermal radiomics[J]. Artificial Intelligence In Medicine,2020,105.[67]. Evaluation of Payer Budget Impact Associated with the Use of Artificial Intelligence in Vitro Diagnostic, Kidneyintelx, to Modify DKD Progression:[J]. American Journal of Kidney Diseases,2020,75(5).[68]Rohit Nishant,Mike Kennedy,Jacqueline Corbett. Artificial intelligence for sustainability: Challenges, opportunities, and a research agenda[J]. International Journal of Information Management,2020,53.[69]Hoang Nguyen,Xuan-Nam Bui. Soft computing models for predicting blast-induced air over-pressure: A novel artificial intelligence approach[J]. Applied Soft Computing Journal,2020,92.[70]Benjamin S. Hopkins,Aditya Mazmudar,Conor Driscoll,Mark Svet,Jack Goergen,Max Kelsten,Nathan A. Shlobin,Kartik Kesavabhotla,Zachary A Smith,Nader S Dahdaleh. Using artificial intelligence (AI) to predict postoperative surgical site infection: A retrospective cohort of 4046 posterior spinal fusions[J]. Clinical Neurology and Neurosurgery,2020,192.[71]Mei Yang,Runze Zhou,Xiangjun Qiu,Xiangfei Feng,Jian Sun,Qunshan Wang,Qiufen Lu,Pengpai Zhang,Bo Liu,Wei Li,Mu Chen,Yan Zhao,Binfeng Mo,Xin Zhou,Xi Zhang,Yingxue Hua,Jin Guo,Fangfang Bi,Yajun Cao,Feng Ling,Shengming Shi,Yi-Gang Li. Artificial intelligence-assisted analysis on the association between exposure to ambient fine particulate matter and incidence of arrhythmias in outpatients of Shanghai community hospitals[J]. Environment International,2020,139.[72]Fatemehalsadat Madaeni,Rachid Lhissou,Karem Chokmani,Sebastien Raymond,Yves Gauthier. Ice jam formation, breakup and prediction methods based on hydroclimatic data using artificial intelligence: A review[J]. Cold Regions Science and Technology,2020,174.[73]Steve Chukwuebuka Arum,David Grace,Paul Daniel Mitchell. A review of wireless communication using high-altitude platforms for extended coverage and capacity[J]. Computer Communications,2020,157.[74]Yong-Hong Kuo,Nicholas B. Chan,Janny M.Y. Leung,Helen Meng,Anthony Man-Cho So,Kelvin K.F. Tsoi,Colin A. Graham. An Integrated Approach of Machine Learning and Systems Thinking for Waiting Time Prediction in an Emergency Department[J]. International Journal of Medical Informatics,2020,139.[75]Matteo Terzi,Gian Antonio Susto,Pratik Chaudhari. Directional adversarial training for cost sensitive deep learning classification applications[J]. Engineering Applications of Artificial Intelligence,2020,91.[76]Arman Kilic. Artificial Intelligence and Machine Learning in Cardiovascular Health Care[J]. The Annals of Thoracic Surgery,2020,109(5).[77]Hossein Azarmdel,Ahmad Jahanbakhshi,Seyed Saeid Mohtasebi,Alfredo Rosado Mu?oz. Evaluation of image processing technique as an expert system in mulberry fruit grading based on ripeness level using artificial neural networks (ANNs) and support vector machine (SVM)[J]. Postharvest Biology and Technology,2020,166.[78]Wafaa Wardah,Abdollah Dehzangi,Ghazaleh Taherzadeh,Mahmood A. Rashid,M.G.M. Khan,Tatsuhiko Tsunoda,Alok Sharma. Predicting protein-peptide binding sites with a deep convolutional neural network[J]. Journal of Theoretical Biology,2020,496.[79]Francisco F.X. Vasconcelos,Róger M. Sarmento,Pedro P. Rebou?as Filho,Victor Hugo C. de Albuquerque. Artificial intelligence techniques empowered edge-cloud architecture for brain CT image analysis[J]. Engineering Applications of Artificial Intelligence,2020,91.[80]Masaaki Konishi. Bioethanol production estimated from volatile compositions in hydrolysates of lignocellulosic biomass by deep learning[J]. Journal of Bioscience and Bioengineering,2020,129(6).人工智能英文参考文献三:[81]J. Kwon,K. Kim. Artificial Intelligence for Early Prediction of Pulmonary Hypertension Using Electrocardiography[J]. Journal of Heart and Lung Transplantation,2020,39(4).[82]C. Maathuis,W. Pieters,J. van den Berg. Decision support model for effects estimation and proportionality assessment for targeting in cyber operations[J]. Defence Technology,2020.[83]Samer Ellahham. Artificial Intelligence in Diabetes Care[J]. The American Journal of Medicine,2020.[84]Yi-Ting Hsieh,Lee-Ming Chuang,Yi-Der Jiang,Tien-Jyun Chang,Chung-May Yang,Chang-Hao Yang,Li-Wei Chan,Tzu-Yun Kao,Ta-Ching Chen,Hsuan-Chieh Lin,Chin-Han Tsai,Mingke Chen. Application of deep learning image assessment software VeriSee? for diabetic retinopathy screening[J]. Journal of the Formosan Medical Association,2020.[85]Emre ARTUN,Burak KULGA. Selection of candidate wells for re-fracturing in tight gas sand reservoirs using fuzzy inference[J]. Petroleum Exploration and Development Online,2020,47(2).[86]Alberto Arenal,Cristina Armu?a,Claudio Feijoo,Sergio Ramos,Zimu Xu,Ana Moreno. Innovation ecosystems theory revisited: The case of artificial intelligence in China[J]. Telecommunications Policy,2020.[87]T. Som,M. Dwivedi,C. Dubey,A. Sharma. Parametric Studies on Artificial Intelligence Techniques for Battery SOC Management and Optimization of Renewable Power[J]. Procedia Computer Science,2020,167.[88]Bushra Kidwai,Nadesh RK. Design and Development of Diagnostic Chabot for supporting Primary Health Care Systems[J]. Procedia Computer Science,2020,167.[89]Asl? Bozda?,Ye?im Dokuz,?znur Begüm G?k?ek. Spatial prediction of PM 10 concentration using machine learning algorithms in Ankara, Turkey[J]. Environmental Pollution,2020.[90]K.P. Smith,J.E. Kirby. Image analysis and artificial intelligence in infectious disease diagnostics[J]. Clinical Microbiology and Infection,2020.[91]Alklih Mohamad YOUSEF,Ghahfarokhi Payam KAVOUSI,Marwan ALNUAIMI,Yara ALATRACH. Predictive data analytics application for enhanced oil recovery in a mature field in the Middle East[J]. Petroleum Exploration and Development Online,2020,47(2).[92]Omer F. Ahmad,Danail Stoyanov,Laurence B. Lovat. Barriers and pitfalls for artificial intelligence in gastroenterology: Ethical and regulatory issues[J]. Techniques and Innovations in Gastrointestinal Endoscopy,2020,22(2).[93]Sanne A. Hoogenboom,Ulas Bagci,Michael B. Wallace. Artificial intelligence in gastroenterology. The current state of play and the potential. How will it affect our practice and when?[J]. Techniques and Innovations in Gastrointestinal Endoscopy,2020,22(2).[94]Douglas K. Rex. Can we do resect and discard with artificial intelligence-assisted colon polyp “optical biopsy?”[J]. Techniques and Innovations in Gastrointestinal Endoscopy,2020,22(2).[95]Neal Shahidi,Michael J. Bourke. Can artificial intelligence accurately diagnose endoscopically curable gastrointestinal cancers?[J]. Techniques and Innovations in Gastrointestinal Endoscopy,2020,22(2).[96]Michael Byrne. Artificial intelligence in gastroenterology[J]. Techniques and Innovations in Gastrointestinal Endoscopy,2020,22(2).[97]Piet C. de Groen. Using artificial intelligence to improve adequacy of inspection in gastrointestinal endoscopy[J]. Techniques and Innovations in Gastrointestinal Endoscopy,2020,22(2).[98]Robin Zachariah,Andrew Ninh,William Karnes. Artificial intelligence for colon polyp detection: Why should we embrace this?[J]. Techniques and Innovations in Gastrointestinal Endoscopy,2020,22(2).[99]Alexandra T. Greenhill,Bethany R. Edmunds. A primer of artificial intelligence in medicine[J]. Techniques and Innovations in Gastrointestinal Endoscopy,2020,22(2).[100]Tomohiro Tada,Toshiaki Hirasawa,Toshiyuki Yoshio. The role for artificial intelligence in evaluation of upper GI cancer[J]. Techniques and Innovations in Gastrointestinal Endoscopy,2020,22(2).[101]Yahui Jiang,Meng Yang,Shuhao Wang,Xiangchun Li,Yan Sun. Emerging role of deep learning‐based artificial intelligence in tumor pathology[J]. Cancer Communications,2020,40(4).[102]Kristopher D. Knott,Andreas Seraphim,Joao B. Augusto,Hui Xue,Liza Chacko,Nay Aung,Steffen E. Petersen,Jackie A. Cooper,Charlotte Manisty,Anish N. Bhuva,Tushar Kotecha,Christos V. Bourantas,Rhodri H. Davies,Louise A.E. Brown,Sven Plein,Marianna Fontana,Peter Kellman,James C. Moon. The Prognostic Significance of Quantitative Myocardial Perfusion: An Artificial Intelligence–Based Approach Using Perfusion Mapping[J]. Circulation,2020,141(16).[103]Muhammad Asad,Ahmed Moustafa,Takayuki Ito. FedOpt: Towards Communication Efficiency and Privacy Preservation in Federated Learning[J]. Applied Sciences,2020,10(8).[104]Wu Wenzhi,Zhang Yan,Wang Pu,Zhang Li,Wang Guixiang,Lei Guanghui,Xiao Qiang,Cao Xiaochen,Bian Yueran,Xie Simiao,Huang Fei,Luo Na,Zhang Jingyuan,Luo Mingyan. Psychological stress of medical staffs during outbreak of COVID-19 and adjustment strategy.[J]. Journal of medical virology,2020.[105]. Eyenuk Fulfills Contract for Artificial Intelligence Grading of Retinal Images[J]. Telecomworldwire,2020.[106]Kim Tae Woo,Duhachek Adam. Artificial Intelligence and Persuasion: A Construal-Level Account.[J]. Psychological science,2020,31(4).[107]McCall Becky. COVID-19 and artificial intelligence: protecting health-care workers and curbing the spread.[J]. The Lancet. Digital health,2020,2(4).[108]Alca?iz Mariano,Chicchi Giglioli Irene A,Sirera Marian,Minissi Eleonora,Abad Luis. [Autism spectrum disorder biomarkers based on biosignals, virtual reality and artificial intelligence].[J]. Medicina,2020,80 Suppl 2.[109]Cong Lei,Feng Wanbing,Yao Zhigang,Zhou Xiaoming,Xiao Wei. Deep Learning Model as a New Trend in Computer-aided Diagnosis of Tumor Pathology for Lung Cancer.[J]. Journal of Cancer,2020,11(12).[110]Wang Fengdan,Gu Xiao,Chen Shi,Liu Yongliang,Shen Qing,Pan Hui,Shi Lei,Jin Zhengyu. Artificial intelligence system can achieve comparable results to experts for bone age assessment of Chinese children with abnormal growth and development.[J]. PeerJ,2020,8.[111]Hu Wenmo,Yang Huayu,Xu Haifeng,Mao Yilei. Radiomics based on artificial intelligence in liver diseases: where we are?[J]. Gastroenterology report,2020,8(2).[112]Batayneh Wafa,Abdulhay Enas,Alothman Mohammad. Prediction of the performance of artificial neural networks in mapping sEMG to finger joint angles via signal pre-investigation techniques.[J]. Heliyon,2020,6(4).[113]Aydin Emrah,Türkmen ?nan Utku,Namli G?zde,?ztürk ?i?dem,Esen Ay?e B,Eray Y Nur,Ero?lu Egemen,Akova Fatih. A novel and simple machine learning algorithm for preoperative diagnosis of acute appendicitis in children.[J]. Pediatric surgery international,2020.[114]Ellahham Samer. Artificial Intelligence in Diabetes Care.[J]. The Americanjournal of medicine,2020.[115]David J. Winkel,Thomas J. Weikert,Hanns-Christian Breit,Guillaume Chabin,Eli Gibson,Tobias J. Heye,Dorin Comaniciu,Daniel T. Boll. Validation of a fully automated liver segmentation algorithm using multi-scale deep reinforcement learning and comparison versus manual segmentation[J]. European Journal of Radiology,2020,126.[116]Binjie Fu,Guoshu Wang,Mingyue Wu,Wangjia Li,Yineng Zheng,Zhigang Chu,Fajin Lv. Influence of CT effective dose and convolution kernel on the detection of pulmonary nodules in different artificial intelligence software systems: A phantom study[J]. European Journal of Radiology,2020,126.[117]Georgios N. Kouziokas. A new W-SVM kernel combining PSO-neural network transformed vector and Bayesian optimized SVM in GDP forecasting[J]. Engineering Applications of Artificial Intelligence,2020,92.[118]Qingsong Ruan,Zilin Wang,Yaping Zhou,Dayong Lv. A new investor sentiment indicator ( ISI ) based on artificial intelligence: A powerful return predictor in China[J]. Economic Modelling,2020,88.[119]Mohamed Abdel-Basset,Weiping Ding,Laila Abdel-Fatah. The fusion of Internet of Intelligent Things (IoIT) in remote diagnosis of obstructive Sleep Apnea: A survey and a new model[J]. Information Fusion,2020,61.[120]Federico Caobelli. Artificial intelligence in medical imaging: Game over for radiologists?[J]. European Journal of Radiology,2020,126.以上就是关于人工智能参考文献的分享,希望对你有所帮助。
OpenFlow Enabling Innovation in Campus Network以及中文翻译

附录A 外文原文OpenFlow: Enabling Innovation in Campus NetworksNick McKeown Stanford University Guru Parulkar Stanford UniversityTom AndersonUniversity of WashingtonLarry PetersonPrinceton UniversityHari BalakrishnanMITJennifer RexfordPrinceton UniversityScott Shenker University of California,BerkeleyJonathan Turner Washington University inSt. LouisThis article is an editorial note submitted to CCR. It has NOT been peer reviewed.Authors take full responsibility for this article’s technical ments can be posted through CCR Online.ABSTRACTThis whitepaper proposes OpenFlow: a way for researchers to run experimental protocols in the networks they use every day. OpenFlow is based on an Ethernet switch, with an internal flow-table, and a standardized interface to add and remove flow entries. Our goal is to encourage networking vendors to add OpenFlow to their switch products for deployment in college campus backbones and wiring closets. We believe that OpenFlow is a pragmatic compromise: on one hand, it allows researchers to run experiments on heterogeneous switches in a uniform way at line-rate and with high port-density; while on the other hand, vendors do not need to expose the internal workings of their switches. In addition to allowing researchers to evaluate their ideas in real-world traffic settings, OpenFlow could serve as a useful campus component in proposed large-scale testbeds like GENI. Two buildingsat Stanford University will soon run OpenFlow networks, using commercial Ethernet switches and routers. We will work to encourage deployment at other schools; and we encourage you to consider deploying OpenFlow in your university network too.Categories and Subject DescriptorsC.2 [Internetworking]: RoutersGeneral TermsExperimentation, DesignKeywordsEthernet switch, virtualization, flow-based1. THE NEED FOR PROGRAMMABLE NETWORKSNetworks have become part of the critical infrastructure of our businesses, homes and schools. This success has been both a blessing and a curse for networking researchers; their work is more relevant, but their chance of making an impact is more remote. The reduction in real-world impact of any given network innovation is because the enormous installed base of equipment and protocols, and the reluctance to experiment with production traffic, which have created an exceedingly high barrier to entry for new ideas. Today, there is almost no practical way to experiment with new network protocols (e.g., new routing protocols, or alternatives to IP) in sufficiently realistic settings (e.g., at scale carrying real traffi c) to gain the confidence needed for their widespread deployment. The result is that most new ideas from the networking research community go untried and untested; hence the commonly held belief that the network infrastructure has “ossified”.Having recognized the problem, the networking community is hard at work developing programmable networks, such as GENI [1] a proposed nationwide research facility for experimenting with new network architectures and distributed systems. These programmable networks call for programmable switches and routers that (using virtualization) can process packets for multiple isolated experimental networks simultaneously. For example, in GENI it is envisaged that a researcher will be allocated a slice of resources across the whole network, consisting of a portion of network links, packet processing elements (e.g. routers) and end-hosts; researchers program their slices to behave as they wish. A slice could extend across the backbone, into access networks, into college campuses, industrial research labs, and include wiring closets, wireless networks, and sensor networks.Virtualized programmable networks could lower the barrier to entry for new ideas, increasing the rate of innovation in the network infrastructure. But the plans for nationwide facilities are ambitious (and costly), and it will take years for them to be deployed.This whitepaper focuses on a shorter-term question closer to home: As researchers, how can we run experiments in our campus networks? If we can figure out how, we can start soon and extend the technique to other campuses to benefit the whole community.To meet this challenge, several questions need answering, including: In the early days, how will college network administrators get comfortable putting experimental equipment (switches, routers, access points, etc.) into their network? How will researchers control a portion of their local network in a way that does not disrupt others who depend on it? And exactly whatfunctionality is needed in network switches to enable experiments? Our goal here is to propose a new switch feature that can help extend programmability into the wiring closet of college campuses.One approach -that we do not take -is to persuade commercial “name-brand” equipment vendors to provide an open, programmable, virtualized platform on their switches and routers so that researchers can deploy new protocols, while network administrators can take comfort that the equipment is well supported. This outcome is very unlikely in the short-term. Commercial switches and routers do not typically provide an open software platform, let alone provide a means to virtualize either their hardware or software. The practice of commercial networking is that the standardized external interfaces are narrow (i.e., just packet forward ing), and all of the switch’s internalflexibility is hidde n. The internals differ from vendor to vendor, with no standard platform for researchers to experiment with new ideas. Further, network equipment vendors are understandably nervous about opening up interfaces inside their boxes: they have spent years deploying and tuning fragile distributed protocols and algorithms, and they fear that new experiments will bring networks crashing down. And, of course, open platforms lower the barrier-to-entry for new competitors.A few open software platforms already exist, but do not have the performance or port-density we need. The simplest example is a PC with several network interfaces and an operating system. All well-known operating systems support routing of packets between interfaces, and open-source implementations of routing protocols exist (e.g., as part of the Linux distribution, or from XORP [2]); and in most cases it is possible to modify theoperating system to process packets in almost any manner (e.g., using Click [3]). The problem, of course, is performance: A PC can neither support the number of ports needed for a college wiring closet (a fan out of 100+ ports is needed per box), nor the packet-processing performance (wiring closet switches process over 100Gbits/s of data, whereas a typical PC struggles to exceed 1Gbit/s; and the gap between the two is widening).Existing platforms with specialized hardware for line-rate processing are not quite suitable for college wiring closets either. For example, an ATCA-based virtualized programmable router called the Supercharged Planet Lab Platform [4] is under development at Washington University, and can use network processors to process packets from many interfaces simultaneously at line-rate. This approach is promising in the long-term, but for the time being is targeted at large switching centers and is too expensive for widespread deployment in college wiring closets. At the other extreme is NetFPGA [5] targeted for use in teaching and research labs. NetFPGA is a low-cost PCI card with a user-programmable FPGA for processing packets, and 4 ports of Gigabit Ethernet. NetFPGA is limited to just four network interfaces—insufficient for use in a wiring closet.Thus, the commercial solutio ns are too closed and inflex ible and the research solutions either have insufficient performance or fan out, or are too expensive. It seems unlikely that the research solutions, with their complete generality, can overcome their performance or cost limitations. A more promising approach is to compromise on generality and to seek a degree of switch flexibility that is:Figure 1 Idealized OpenFlow Switch. The Flow Table is controlled by a remote controllervia the Secure Channel.•Amenable to high-performance and low-cost implementations.•Capable of supporting a broad range of research.•Assured to isolate experimental traffic from production traffic. •Consistent with vendors’ need for closed platforms.This paper describes the OpenFlow Switch—a specification that is an initial attempt to meet these four goals.2. THE OPENFLOW SWITCHThe basic idea is simple: we exploit the fact that most modern Ethernet switches and routers contain flow-tables (typically built from TCAMs) that run at line-rate to im plement firewalls, NAT, QoS, and to collect statistics. While each vendor’s flow-table is different, we’ve identified an interesting common set of functions that run in many switches and routers. OpenFlow exploits this common set of functions.OpenFlow provides an open protocol to program the flow-table in different switches and routers. A network administrator can partition traffic into production and research flows. Researchers can control their own flows -by choosing the routes their packets follow and the processing they receive. Inthis way, researchers can try new routing protocols, security models, addressing schemes, and even alternatives to IP. On the same network, the production traffic is isolated and processed in the same way as today.The datapath of an OpenFlow Switch consists of a Flow Table, and an action associated with each flow entry. The set of actions supported by an OpenFlow Switch is extensible, but below we describe a minimum requirement for all switches. For high-performance and low-cost the data-path must have a carefully prescribed degree of flexibility. This means forgoing the ability to specify arbitrary handling of each packet and seeking a more limited, but still useful, range of actions. Therefore, later in the pape r, define a basic required set of actions for all OpenFlow switches.An OpenFlow Switch consists of at least three parts: (1) A Flow Table, with an action associated with each flow entry, to tell the switch how to process the flow, (2) A Secure Channel that connects the switch to a remote control process (called the controller), allowing commands and packets to be sent between a controller and the switch using (3) The OpenFlow Protocol, which provides an open and standard way for a controller to communicate with a switch. By specifying a standard interface (the OpenFlow Protocol) through which entries in the Flow Table can be defined externally, the OpenFlow Switch avoids the need for researchers to program the switch.It is useful to categorize switches into dedicated OpenFlow switches that do not support normal Layer 2 and Layer 3 processing, and OpenFlow-enabled general purpose commercial Ethernet switches and routers, to which the Open-Flow Protocol and interfaces have been added as a new feature.Dedicated OpenFlow switches. A dedicated OpenFlow Switch is a dumb datapath element that forwards packets between ports, as defined b y a remote control process. Figure 1 shows an example of an OpenFlow Switch.In this context, flows are broadly defined, and are limit ed only by the capabilities of the particular implementation of the Flow Table. For example, a flow could be a TCP con nection, or all packets from a particular MAC address or IP address, or all packets with the same VLAN tag, or all packets from the same switch port. For experiments involving non-IPv4 packets, a flow could be defined as all packets matching a specific (but non-standard) header.Each flow-entry has a simple action associated with it; the three basic ones (that all dedicated OpenFlow switches must support) are:1 Forward this flow’s packets to a given port (or ports). This allows packets to be routed through the network. In most switches this is expected to take place at line-rate.2 Encapsulate and forw ard this flow’s packets to a con troller. Packet is delivered to Secure Channel, where it is encapsulated and sent to a controller. Typically used for the first packet in a new flow, so a controller can decide if the flow should be added to the Flow Table. Or in some experiments, it could be used to forward all packets to a controller for processing.3 Drop this flow’s packets. Can be used for security, to curb denial of service attacks, or to reduce spurious broadcast discovery traffic from end-hosts.An entry in the Flow-Table has three fields: (1) A packet header that defines the flow, (2) The action, which defines how the packets should be processed, and (3) Statistics, which keep track of the number of packets and bytes foreach flow, and the time since the last packet matched the flow (to help with the removal of inactive flows).In the first generation “Type 0” switches, the flow header is a 10-tuple shown in T able 1. A TCP flow could be specified by all ten fields, whereas an IP flow might not include the transport ports in its definition. Each header field can be a wildcard to allow for aggregation of flows, such as flows in which only the VLAN ID is defined would apply to all traffic on a particular VLAN.Table 1 The header fields matched in a “Type 0” OpenFlow switch.The detailed requirements of an OpenFlow Switch are defined by the OpenFlow Switch Specification [6].OpenFlow-enabled switches. Some commercial switches, routers and access points will be enhanced with the OpenFlow feature by adding the Flow Table, Secure Channel and OpenFlow Protocol (we list some examples in Section 5). Typically, the Flow Table will re-use existing hardware, such as a TCAM; the Secure Channel and Proto col will be ported to run on the switch’s operating system. Figure 2 shows a network of OpenFlow-enabled commercial switches and access points. In this example, all the Flow Tables are managed by the same controller; the OpenFlow Protocol allows a switch to be controlled by two or more controllers for increased performance or robustness. Our goal is to enable experiments to take place in an existing production network alongside regular traffic and applications. Therefore, to win theconfidence of network administrators, OpenFlow-enabled switches must isolate experimental traffic (processed by the Flow Table) from productiontraffic that is to be processed by the normal Layer 2 and Layer 3 pipeline of the switch. There are two ways to achieve this separation. One is to add a fourth action:4. Forward this flow’s packets through the switch’s nor mal processing pipeline.The other is to define separat e sets of VLANs for experimental and production traffic. Both approaches allow normal production traffic that isn’t part of an experiment to be processed in the usual way by the switch. All OpenFlow-enabled switches are required to support one approach or the other; some will support both.Additional features. If a switch supports the header formats and the four basic actions mentioned above (and detailed in the OpenFlow SwitchSpecification), then we call it a “Type 0” switch. We expect that many switches will support additional actions, for example to rewrite portions of the packet header (e.g., for NAT, or to obfuscate addresses on intermediate links), and to map packets to a priority class. Likewise, some Flow Tables will be able to match on arbitrary fields in the packet header, enabling experiments with new non-IP protocols. As a particular set of features emerges, we willdefine a “Type 1” switch.Controllers.A controller adds and removes flow-entries from the Flow Table on behalf of experiments. For example, a static controller might be a simple application running on a PC to statica lly establish flows to interconnect a set of test computers for the duration of an experiment. In this case the flows resemble VLANs in current networks— providing a simple mechanism toisolate experimental traffic from the production network. Viewed this way, OpenFlow is a generalization of VLANs.One can also imagine more sophisticated controllers that dynamicallyadd/remove flows as an experiment progresses. In one usage model, a researcher might control the complete network of OpenFlow Switches and be free to decide how all flows are processed.Figure 2 Example of a network of OpenFlow-enabled commercial switches and routers.A more sophisticated controller might support multiple researchers, each with different accounts and permissions, enabling them to run multiple independent experiments on different sets of flows. Flows identified as under the control of a particular researcher (e.g., by a policy table running in a controller) could be delivered to a researcher’s user-level control program which then decides if a new flow-entry should be added to the network of switches.3. USING OPENFLOWAs a simple example of how an OpenFlow Switch might be used imagine that Amy (a researcher) invented Amy-OSPF as a new routing protocol toreplace OSPF. She wants to try her protocol in a network of OpenFlow Switches, without changing any end-host software. Amy-OSPF will run in a controller; each time a new application flow starts Amy-OSPF picks a route through a series of OpenFlow Switches, and adds a flow-entry in each switch along the path. In her experiment, Amy decides to use Amy-OSPF for thetraffic entering the OpenFlow network from her own desktop PC— so she doesn’t disrupt the network for others. To do this, she defines one flow to be all the traffic entering the Open-Flow switch through the switch port her PC is connected to, and adds a flow-entry with the action “Enca psulate and forward all packets to a controller”. When her packets reach a controller, her new protocol chooses a route and adds a new flow-entry (for the application flow) to every switch along the chosen path. When subsequent packets arrive at a switch, they are processed quickly (and at line-rate) by the Flow Table. There are legitimate questions to ask about the performance, reliability and scalability of a controller that dynam ically adds and removes flows as an experiment progresses: Can such a centralized controller be fast enough to process new flows and program the Flow Switches? What happens when a controller fails? To some extent these questions were addressed in the context of the Ethane prototype, which used simple flow switches and a central controller [7]. Preliminary results suggested that an Ethane controller based on a low-cost desktop PC could process over 10,000 new flows per second —enough for a large college campus. Of course, the rate at which ne w flows can be processed will depend on the complexity of the processing required by the re searcher’s experiment. But it gives us confidence that mean ingful experiments can be run. Scalability and redundancy are possible by making acontroller (and the experiments) stateless, allowing simple load-balancing over multiple separate devices.3.1 Experiments in a Production NetworkChances are, Amy is testing her new protocol in a network used by lots of other people. We therefore want the network to have two additional properties:1 Packets belonging to users other than Amy should be routed using a standard and tested routing protocol running in the switch or router from a “name-brand” v endor.2 Amy should only be able to add flow entries for her traffic, or for any traffic her network administrator has allowed her to control.Property 1 is achieved by OpenFlow-enabled switches. In Amy’s experiment, the default action for all packets that don’t come from Amy’s PC could be to forward them through the normal processing pipeline. Amy’s own packets would be forwarded directly to the outgoing port, without being processed by the normal pipeline.Property 2 depends on the controller. The controller should be seen as a platform that enables researchers to implement various experiments, and the restrictions of Property 2 can be achieved with the appropriate use of permissions or other ways to limit the powers of individual researchers to control flow e ntries. The exact nature of these permission-like mechanisms will depend on how the controller is implemented. We expect that a variety of controllers will emerge. As an example of a concrete realization of a controller, some of the authors are working on a controller called NOX as a follow-on to the Ethane work [8]. A quite different controller might emerge by extending the GENI management software to OpenFlow networks.3.2 More ExamplesAs with any experimental platform, the set of experiments will exceed those we can think of up-front — most experiments in OpenFlow networks are yet to be thought of. Here, for illustration, we offer some examples of how OpenFlow-enabled networks could be used to experiment with new network applications and architectures.Example 1: Network Management and Access Con trol. We’ll use Ethane as our first example [7] as it was the research that inspired OpenFlow. In fact, an OpenFlow Switch can be thought of as a generalization of Ethane’s datapath switch. Ethane used a specific implementation of a controller, suited for network management and control, that manages the admittance and routing of flows. The basic idea of Ethane is to allow network managers to define a network-wide policy in the central controller, which is enforced directly by making admission control decisions for each new flow. A controller checks a new flow against a set of rules, such as “Guests can communicate using HTTP, but only via a web proxy” or “VoIP phones are not allowed to communicate with laptops.” A controller associates pack ets with their senders by managing all the bindings between names and addresses — it essentially takes over DNS, DHCP and authenticates all users when they join, keeping track of which switch port (or access point) they are connected to. One could envisage an extension to Ethane in which a policy dictates that particular flows are sent to a user’s process in a controller, hence allowing researcher-specific processing to be performed in the network.Example 2: VLANs. OpenFlow can easily provide users with their own isolated network, just as VLANs do. The simplest approach is to statically declare a set of flows which specify the ports accessible by traffic on a givenVLAN ID. Traffic identified as coming from a single user (for example, originating from specific switch ports or MAC addresses) is tagged by the switches (via an action) with the appropriate VLAN ID.A more dynamic approach might use a controller to manage authentication of users and use the knowledge of the users’ locations for tagging traffic at runtime.Example 3: Mobile wireless VOIP clients. For this example consider an experiment of a new call-handoff mechanism for WiFi-enabled phones. In the experiment VOIP clients establish a new connection over the OpenFlow-enabled network. A controller is implemented to track the location of clients, re-routing connections — by reprogramming the Flow Tables — as users move through the network, allowing seamless handoff from one access point to another.Example 4: A non-IP network. So far, our examples have assumed an IP network, but OpenFlow doesn’t require packets to be of any one format — so long as the Flow Table is able to match on the packet header. This would allow experiments using new naming, addressing and routing schemes. There are several ways an OpenFlow-enabled switch can support non-IP traffic. For example, flows could be identified using their Ethernet header (MAC src and dst addresses), a new EtherType value, or at the IP level, by a new IP Version number. More generally, we hope that future switches will allow a controller to create a generic mask (offset + value + mask), allowing packets to be processed in a researcher-specified way.Example 5: Processing packets rather than flows.The examples above are for experiments involving flows — where a controller makes decisions when the flow starts. There are, of course, interesting experiments to be performed that require every packet to be processed. For example, an intrusion detection system that inspects every packet, an explicit congestion control mechanism, or when modifying the contents of packets, such as when converting packets from one protocol format to another.Figure 3: Example of processing packets through anexternal line-rate packet-processing device, such as a programmable NetFPGA router.There are two basic ways to process packets in an OpenFlow-enabled network. First, and simplest, is to force all of a flow’s packets to pass t hrough a controller. To do this, a controller doesn’t add a new flow entry into the Flow Switch — it just allows the switch to default to forwarding every packet to a controller. This has the advantage of flexibility, at the cost of performance. It might provide a useful way to test the functionality of a new protocol, but is unlikely to be of much interest for deployment in a large network.The second way to process packets is to route them to a programmable switch that does packet processing — for example, a NetFPGA-based programmable router. The advantage is that the packets can be processed at line-rate in a user-definable way; Figure 3 shows an example of how this could be done, in which the OpenFlow-enabled switch operates essentially as a patch-panel to allow the packets to reach the NetFPGA. In some cases, the NetFPGA board (a PCI board that plugs into a Linux PC) might be placed in the wiring closet alongside the OpenFlow-enabled switch, or (more likely) in a laboratory.4. THE OPENFLOW CONSORTIUMThe OpenFlow Consortium aims to popularize OpenFlow and maintain the OpenFl ow Switch Specification. The Con sortium is a group of researchers and network administrators at universities and colleges who believe their research mission will be enhanced if OpenFlow-enabled switches are installed in their network.Membership is open and free for anyone at a school, college, university, or government agency worldwide. The OpenFlow Consortium welcomes individual members who are not employed by companies that manufacture or sell Ethernet switches, routers or wireless access points (because we want to keep the consortium free of vendor influence). To join, send email to***********************.The Consortium web-site contain s the OpenFlow Switch Specification, a list of consortium members, and reference implementations of OpenFlow switches.Licensing Model: The OpenFlow Switch Specification is free for all commercial and non-commercial use. (The exact wording is on the web-site.) Commercial switches and routers claiming to be “OpenFlow-enabled” must conform to the requirements of an OpenFlow Type 0 Switch, as defined in the OpenFlow Switch Specification. OpenFlow is a trademark of Stanford University, and will be protected on behalf of the Consortium.5. DEPLOYING OPENFLOW SWITCHESWe believe there is an interesting market opportunity for network equipment vendors to sell OpenFlow-enabled switches to the research community. Every building in thousands of colleges and universities contains wiring closets with Ethernet switches and routers, and with wireless access points spread across campus.We are actively working with several switch and router manufacturers who are adding the OpenFlow feature to their products by implementing a Flow Table in existing hardware; i.e. no hardware change is needed. The switches run the Secure Channel software on their existing processor.We have found network equipment vendors to be very open to the idea of adding the OpenFlow feature. Most vendors would like to support the research community without having to expose the internal workings of their products. We are deploying large OpenFlow networks in the Computer Science and Electrical Engineering departments at Stanford University. The networks in two buildings will be replaced by switches running OpenFlow. Eventually, all traffic will run over the OpenFlow network, with production traffic and experimental traffic being isolated on different VLANs under the control of。
General Definition

© Zoran Despotovic, Karl Aberer EPFL-I&C, Laboratoire de systèmes d'informations répartis
CEC 2004, San Diego July 19, 2004
slide 15
Peer Behavior Examples
slide 25
Maximum Likelihood Estimation
© Zoran Despotovic, Karl Aberer EPFL-I&C, Laboratoire de systèmes d'informations répartis
CEC 2004, San Diego July 19, 2004
CEC 2004, San Diego July 19, 2004
slide 5
Structured P2P Networks
© Zoran Despotovic, Karl Aberer EPFL-I&C, Laboratoire de systèmes d'informations répartis
© Zoran Despotovic, Karl Aberer EPFL-I&C, Laboratoire de systèmes d'informations répartis
CEC 2004, San Diego July 19, 2004
slide 32
Repeated Games
slide 29
Strategic Game
© Zoran Despotovic, Karl Aberer EPFL-I&C, Laboratoire de systèmes d'informations répartis
Research Interests

Bin Wu2916 S. Shields Ave. #1N Email: bwu3@ Chicago, IL60616 Cell Phone: 312-203-5012 ObjectiveTo find a challenging full-time research/development position in the areas of P2P networking and Web protocol design.Education (GPA: 3.75/4.0) Ph.D. in Computer Science expected 06/2006 University of Illinois at Chicago Chicago,ILMaster in Computer Science 2002 University of Illinois at Chicago Chicago,ILMaster of Engineering 1995 ZheJiang University ZheJiang, China Bachelor of Science 1992 ZheJiang University ZheJiang, China Research InterestsDistributed Systems, Peer-to-Peer Networks, Networks Protocols and Algorithms, Web-BasedMedication Systems.Publications1. B. Wu, A. D. Kshemkalyani, “Objective-Optimal Algorithms for Long-Term Web Prefetching,”IEEE Transactions on Computers, December 2005.2. B. Wu, A.D. Kshemkalyani, “Objective-Greedy Algorithms for Long-Term Web Prefetching”,3rd IEEE Conference on Network Computing and Applications (NCA), 61-68, August 2004.3. A.D. Kshemkalyani, B. Wu, “Nonintrusive Snapshots Using Thin Slices”, The 2005 IFIP InternationalConference on Embedded And Ubiquitous Computing (EUC-05), Japan, 6-9 December 2005.4. B. Wu, A.D. Kshemkalyani, “Evaluation of Analysis Models for Unguided Search in UnstructuredP2P Networks”, IFIP International Symposium on Network-Centric Ubiquitous Systems (NCUS2006), LNCS 4097, Springer, 163-172, 2006.5. B. Wu, A.D. Kshemkalyani, “Analysis Models for Blind Search in Unstructured Overlays”, 5th IEEESymposium on Network Computing and Applications (NCA), 223-226, 2006.Reviewer•Computer Network (COMNET) Journal.•4th IEEE International Conference of Peer-to-Peer (P2P) Network.•7th International Workshop on Distributed Computing (IWDC), 2005.Honors and Certificates•UIC Provost’s Award for Graduate Research, 2005.•UIC University Fellowship, 1999-2000 Academic Year.Technical Skills•Language: , , , ASP, C/C++, C#, Java, Visual Basic 6, DHTML, VB Script, Java Bean, JavaScript, XML/XSLT, SQL/Transact-SQL, WML•Operating Systems: Unix, Windows 2000 Server/98/XP•Programming Platforms: Microsoft Visual Studio, Microsoft Visual , Microsoft Office, J-Builder•Programming Skills: MFC, OLEDB, ActiveX, COM/COM+, IIS 5.0/6.0 Programming (Server Extension, ISAPI Filter)•DBMS: SQL Server 2000, Oracle 8/8i, MS Access/FoxProWorking Experience1. Software Engineer University of Illinois Medical College 01/2000-PresentAs a core team member, I designed and developed the AvidCare Tele-Medicine system. This web-based system is used for physicians and medical professionals to provide medical services to registered patients. Physicians log on to the AvidCare Tele-Medicine system to monitor and advise theirpatients who may reside all over the world. In addition, this system organizes patients, physicians, carecenters and monitoring devices with full consideration of security, efficiency, scalability and robustness.This system has been submitted to FDA examination. My contributions to this project include: •Designed the system database using SQL Server 2000.•Designed and implemented the Web application for AvidCare Tele-Medicine system•Developed an Expert Diagnostic Toolkit.•Built a real time Alarm Paging system.•Implemented a wireless version of the Tele-Medicine system with WAP protocol and WML language.2. Research Assistant Department of Mechanical Engineering at UIC 01/1998-01/2000Modified and enhanced an academic program for combustion computation (OPPDIF by Sandia Lab) to perform micro-gravity combustion radiation computation for a NASA founded micro-gravityproject.3. Software Developer ZheJiang University, China 04/1995-11/1997•Designed, implemented and maintained a real-time monitoring and control network (MCN) for Power Industrial processes. The tasks of data collection, data analysis and generationof control commands are distributed over the network which is composed of datacollectors, PCs and industrial control units. Compared with centralized control systemsthat were widely used in industry processes, our distributed version was especiallyadvantageous with regards to cost, reliability and maintenance. Since the time this projectwas completed in 1997, it has been installed and used in a large number of power plantsand industrial companies in China.•Designed a Computer Aided Instruction Tool Kit using C++, VB, Microsoft Access and Microsoft Graphic User Interface. This set of software tools are used by course instructorsor professors to create a series of interactive lectures in the form of user-friendly computerprograms that help students better understand course materials with various approaches.ReferencesAvailable on demand。
太赫兹波段的吸收、衍射和自由空间路径损耗模型(IJEM-V10-N1-5)
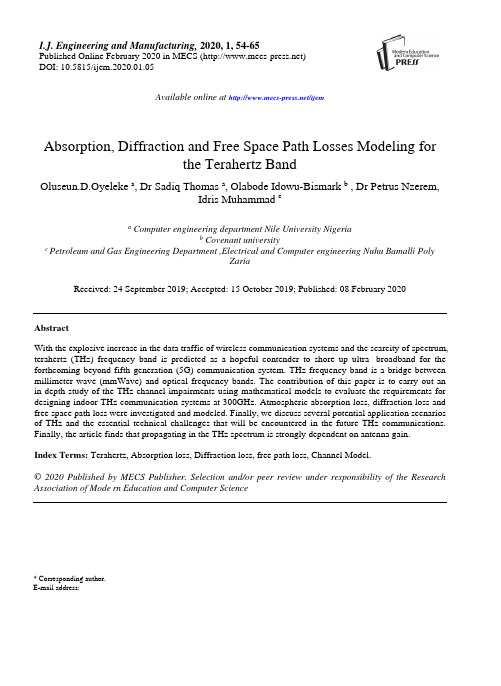
Absorption, Diffraction and Free Space Path Losses Modeling for the Terahertz Band
55
1. Introduction
Machine-type communications such as the Virtual reality applications, Internet of Things, Internet of Everything, vehicles Ado networks, Artificial intelligence, etc are expected to play an important role in the future wireless networks [1,2]. Especially in industry requiring automation, communication, safety for increased productivity. Edholm notes, is that we may someday see the end of wire line [3] and Moore's law is the observation that the number of transistors in a dense integrated circuit doubles about every two year [4]. This implies bandwidth scarcity in future due to the escalating rate of interconnected devices. Also, the current long term evolution advance (LTE/LTE-A) cellular networks cannot handle such gigantic connections since user terminals at the cell boundary experience very high interference, and therefore perform badly [5]. Furthermore, orthodox cellular systems are designed mainly for human users [1]. The use of a wide range of spectrum in the forth coming fifth generation (5G) communication network including the millimeter wave (MmWave) with its ability to provide large bandwidth from hundreds of MHz to several GHz range is able to offer much more bandwidth and connectivity than the traditional microwave communication below the 6GHz band [2]. Although the data rate provided by mmWave band can attain several Gbps, it is still not adequate to meet the requirement of the increasing data traffics in future wireless communications such as the future wireless local area networks (WLAN) and the wireless personal area networks (WPAN) systems with data rate of 10X Gbps [6]. Also, the least data rate of virtual reality (VR) devices is in the range of 10 Gbps while the data rate of uncompressed ultra-high-definition videos and 3D videos will reach 24 Gbps and 100 Gbps, respectively [6] leading to the need for an urgent research for much higher frequency resources.
abstract-sample

Requirements for the abstract 摘要的要求several paragraphs (within 350 words))methodology; (一般过去时)findings; (一般现在时)recommendations (conclusion, discussion, evaluation, limitation, awareness, suggestion, confidence, direction, significance, prospect, further investigation) (一般现在时)impersonal 物称passive 被动complex 复杂句The glass partition: Obstacles to cross-sex friendships at workCross-sex friendships can be difficult to develop and maintain under any circumstances (O’Meara, 1989). (introduce the topic) Issues stemming from male-female differences in friendship norms and interests, sexual attraction, and concerns that a cross-sex friendship may be misperceived as sexual all pose potential challenges(Martin, 1997; Swain, 1992). (problem) Within the workplace/work unit,where friendships are particularly important for career development (Kram & Isabella, 1985), physical proximity亲近,接近(e.g. having desks or offices in close proximity to one another) and job requirements may facilitate/promote cross-sex friendship formation. (narrow the topic: importance & benefits/advantages/positive impact) On the contrary,other organizational influences such as fear that a cross-sex friendship may be misperceived as a romantic relationship or fear of sexual harassment charges may decrease interactions between cross-sex co-workers/colleagues/associates, (thus) limiting the pool of一拨人potential friends潜在的朋友圈. (narrow the topic: defects/disadvantages/negative impact) The present analysis of interviews with professionals investigates the impact of the workplace on the development and maintenance of these friendships.(purpose & method)Workplace friendships are important because they can provide benefits which promote career success. (topic sentence: importance) Lincoln and Miller (1979) defined friendship networks in organizations as ‘systems for making decisions, mobilizing resources调动资源, concealing 隐瞒or transmitting 传达concept-defining) Friends in the workplace 社交网络support that are invaluable for both job performance and satisfaction (Kram & Isabella, 1985; Lincoln & Miller, 1979). (supporting sentence 2 by statement)Bridge and Baxter (1992) found that work friends provided career support in three forms. (topic sentence) Friends provided information access途径, serving ‘as a second pair of eyes and ears for one another.’ Friends also provided work-related assistance, helping each other accomplish their job tasks.Third, friends gave psychological support to one another, ‘providing understanding, empathy移情作用;同理心(站在对方立场思考的一种方式be in somebody’s shoes); 共感; 心灵相通and comfort’ (Bridge & Baxter, 1992: 216). (supporting sentences 1-3 by statement & quotation) language feature: static静态; sentence structure: parallelism平行结构While/Although friendships with peers同龄人are important, relationships with those more senior in the organization can also be valuable to the careers of junior employees. (topic sentence) Sometimes these mentor良师益友【希腊神话】门托耳(奥德修斯的忠实朋友和贤明顾问)relationships are formally initiated and managed by the organization, but more often they are informal relationships similar to friendships (Ragins & Cotton, 1999). (supporting sentence 1) Mentors can provide advice and information, offer protection, and promote the mentee’s学员career by making his or her accomplishments more visible (Burke, 1984; Kram & Isabella, 1985; Mobley et al., 1994). (supporting sentence 2) Given the substantial advantages that friendships in the workplace offer, those not able to form friendships are at a career disadvantage.(topic sentence) A preference for same-sex friendships restricts an individual’s pool of potential friends. This may have the most impact for women in male-dominated work environments who need to befriend交友men in order todevelop both peer friendships and mentor-like relationships. Extending the previous research 扩展研究on cross-sex friendships to the workplace may therefore illuminate/illustrate阐明an important barrier to women’s career advancement. (concluding/link sentence承上启下) Cross-sex friendshipsTo date, research on barriers to the development of cross-sex friendships has typically been conducted outside the context of work. Nonetheless/However/Nevertheless,this research suggests several important factors that may be relevant to workplace friendships. (topic sentence) First,gender differences in recreational interests and preferred topics of conversation result in a preference for same-sex friends (Martin, 1997; Swain, 1992). (supporting sentence 1)In addition, gender differences in norms规范and expectations of friendships could cause problems for cross-sex friends. For example, men often disapprove of their same and cross-sex friends crying while women approve of this behavior (Felmlee, 1999). (supporting sentence 2)Workplace environment (subtitle)Since little research has examined cross-sex friendship in the workplace, the effects/outcomes/consequences of organizational influences on these friendships remains unknown. (background: introduce the topic) Certain aspects of the work environment may facilitate cross-sex friendship development while others may hinder the development of new friendships. (thesis & plan of development)Many workplaces encourage teamwork (Beyerlein et al., 1995) which leads to greater interaction between employees. Therefore, the workplace potentially provides an environment where men and women can work closely together to achieve common goals. Consequently/As a result,the workplace offers coworkers proximity, familiarity, and common interests, all of which have been shown to promote liking and friendship (Berscheid & Reis, 1998). This suggests that cross-sex friendships may be easier to develop within the workplace than outside of work. (benefits)Other current workplace trends may inhibit cross-sex friendship. (topic sentence: (defects/disadvantages) For example, since workplace romances are often discouraged, some may want to avoid any possibility that their co-workers misperceive误解a friendship as a romance. Even co-workers who are participants in workplace romances typically attempt to keep their romance a secret (Anderson & Hunsaker, 1985). Unlike friendships and other work-sanctioned relationships, workplace romances can be disruptive to the organization and typically lead to negative gossip (Bowes-Sperry & Tata, 1999; Mainiero, 1986; Powell & Graves, 2003). The romantic label can be particularly damaging when the alleged所谓的,被断言的couple are not peers in the organization. For example, Powell (2001) found that when those perceived to be in a romantic relationship are not peers, and the subordinate is female, other employees tend to believe that the relationship is utilitarian功利的(where sex is traded for career advancement). Once a relationship is deemed utilitarian, outsiders may question the basis for work-related rewards from the higher-level participant. To date, there is no research on how this fear of third-party misperception of cross-sex friendship impacts friendships at work. (supporting sentences by example, statement & quotation)Similarly, the increased awareness of sexual harassment within organizations may impact cross-sex friendship development. (topic sentence: defects/disadvantages) A recentsurvey by the Society for Human Resources Management (SHRM) found that 97 percent of organizations had sexual harassment policies, and 62 percent had formal training in dealing with sexual harassment (SHRM, 1999). However, little research has addressed how increased awareness of sexual harassment has impacted interactions between cross-sex co-workers. One survey of university professors found that 68 percent were concerned about being unjustly accused of sexual harassment, and 45 percent had modified their behavior toward students due to that concern (Nicks, 1996). In addition, Gutek (1997) suggests that ‘the adversarial敌对的,紧张的nature of the formal complaint procedures正式提出申诉程序has had the effect of polarizing两极分化men and women in the workplace’ (p. 196). (supporting sentences by statistics)Finally, an examination of the literature文献on social networks and mentor relationships provides material relevant to cross-sex friendships at work. The preference of men to associate with other men has been well-established in the literature on social networks (see McPherson et al., 2001 for a review). Among managers, men tend to have more gender homo phil ous喜欢同性的networks than do women (Ibarra, 1992, 1995). The causes for same-gender preference in these work networks have included similarity (individuals choose to be close to those who are similar to themselves) and contact (people are most likely to come into contact with others like themselves).The purpose of this exploratory study was to investigate friendships within the professional workplace and to examine what impact, if any, the workplace environment has on cross-sex friendship formation.(purpose) Structured interviews结构式访谈(又称标准化访谈Standardized Interview,它是一种对访谈过程高度控制的访问。
Abstract Faster Network Design with Scenario Pre-filtering

Faster Network Design with Scenario Pre-filteringDebojyoti Dutta,Ashish Goel,John Heidemannddutta@,agoel@,johnh@Information Sciences InstituteSchool of EngineeringUniversity of Southern California4676Admiralty WayMarina Del Rey,CA90292(ISI-Technical-Report-550)November15,2001AbstractDesign and engineering of networks requires the consid-eration of many possible configurations(different networktopologies,bandwidths,traffic and policies).Network en-gineers may use network simulation to evaluate changesin network configuration,but detailed,packet-level simu-lation of many alternatives would be extremely time con-suming.This paper introduces the concept of scenario pre-filtering—rather than perform detailed simulation of eachscenario,we propose to quickly evaluate(pre-filter)all sce-narios in order to select only the relevant scenarios and dis-card those that are clearly too over-or under-provisioned.To rapidly evaluate scenarios,we have developed severalnew analytical techniques to quickly determine the steady-state behavior of the network with both bulk and short termTCPflows.These techniques apply to arbitrary topologiesand routers that use both drop-tail and RED queuing poli-cies.Since we are only interested in selecting the inter-esting scenarios for detailed simulation,the answers needonly be approximate.However,we show that accuracy istypically within10%of detailed simulation.More impor-tantly,these techniques are10–300faster than detailedsimulation,and,hence,pre-filtering is a promising tech-nique to reduce the total simulation time when many sce-narios must be considered.vide an understanding of the network bounds,or,they are under-provisioned.Only a few scenarios are critical i.e. provide a good balance to define the operating limits of the network.Another fact about simulations with proto-cols such as TCP[23]is that they often need to be run for several seconds in order to reach a steady state.Such restrictions further add to the simulation time for each sce-nario.This paper addresses the second problem,i.e.to eval-uate a wide range of simulation scenarios tofind the rel-evant ones and discard the undesired ones quickly.Our work is based on the observation that there is no need to simulate the uninteresting scenarios in detail.We propose Approx-sim,a design tool that can very quickly evaluate the steady-state behavior of scenarios and pre-filter them by user-supplied criteria.It allows uninteresting scenarios to be dismissed quickly and the interesting ones to be eval-uated in detail.Approx-sim identifies the steady-state behavior of sce-narios using a hybrid queuing theoretic approach for drop-tail routers,a new approach to RED modeling,and,an approximatefixed-point algorithm.It makes use of well known equations for bulk TCP behavior[22]and a new ap-proach to approximate the short lived TCPflows.We show that approx-sim can evaluate scenarios an order of magni-tude faster than what is possible with packet-level simula-tors.At the same time,the accuracy is typically within10% with the largest observed error being30%.In approx-sim, scenarios consist of a mix of long and short lived TCP traf-fic(“elephants”and“mice”respectively),and,have both drop-tail and RED queuing at routers over arbitrary topolo-gies.Packet level simulators are inherently deterministic. This is appropriate for simulation studies.However,this can lead to synchronization of traffic.Our analytical simu-lation engine,approx-sim,does not run into such problems. In fact,comparisons with approx-sim helped us to identify scenarios where the ns-2simulations were getting synchro-nized.In later sections,we demonstrate how we removed the synchrony in ns-2by adding short livedflows and by using RED gateways.2Related WorkOur work is related to other approaches for fast simulation, either through parallelism or approximation.It also builds on analytical approaches to understanding network perfor-mance.2.1Rapid simulationParallelism has been used for many years to improve sim-ulation performance[4,16].Several parallel network simulators are currently available,such as Parsec[1], SSFNET[6],and parallel versions of ns-2[13,24].Our work is complementary to these efforts—while parallelism can improve the performance of detailed simulation by up to the number CPUs devoted to the task(typically4–8to-day),pre-filtering can improve performance many-fold by never simulating the uninteresting scenarios in detail. RPI has proposed the use of experimental factoring,that combines multiple sequential simulations on a network of workstations with search algorithms to choose the scenar-ios that should be considered[26].This work is similar to ours in goal(rapidly exploring the design space),and largely complementary in result:their approach could gain further performance improvement by using pre-filtering techniques.In contrast to our approach,they do a random search of the parameter space while we have a determinis-tic algorithm.2.2Analytical approachesQueuing theoretic approaches have long been used to eval-uate network performance(for example,[18]).Although it is necessary to understand fundamental performance lim-its,these approaches must be applied to the Internet with care because of the complexity of the protocols and the networks in use there.Our approach seeks to get the best from both queuing theory and detailed packet-level simu-lation by using the former for a rapid approximation while using the later for detailed evaluation.Recently,there has been extensive work influid-flow-based approaches to network simulation[19,20,21]. These approaches are promising,and,some such as Misra et al.’s[20]approach can capture the transient behavior. However,further work is needed to understand the perfor-mance of these approaches for large networks.Our work complements their strategy;we look at a different problem offinding the steady state values of the network state. 2.3Fixed pointFixed point approximations have been studied by Bu et al.[2].They present formalisms to compute thefixed point for a single congested RED router with reasonable accuracy.They do not,however,have strong results for complex scenarios with both RED and drop-tail routers, and,with both long and short lived TCPflows(elephants and mice).We show that our approach allows complex mixed scenarios to be solved,approximately.Also,the for-malisms used in our approach are extremely simple.2.4ModelingRecent work has developed increasingly accurate analyti-cal models for the steady state of bulk TCP[22].Our workNSFigure1:The structure of the pre-filtering tool builds upon these results.Other work has modeled short TCPflows;we build upon the work there by Cardwell et al.[3]and Huang et al.[15].More recently,Ben Fredj et al.[12]describe shortflows as inelastic traffic and demon-strate that simple queuing models like are rea-sonably accurate for modeling drop-tail routers.We build upon their results and extend them to RED routers.Al-though RED has been studied in detail[10,7,9],and Hol-lot et al.presented a control theoretic model of RED[14], we believe that our work is thefirst to evaluate RED using a Markovian queuing model.3Our ApproachWe designed a pre-filtering framework where we integrated a a fast approximate network simulator,a decider(to deter-mine whether to discard a scenario)and a detailed packet-level simulator using the structure shown in Figure1.The user feeds a regular ns-2script into our version of ns-2that has the embedded approx-solver,approx-sim.Approx-sim would do a fast approximate simulation of the network sce-nario and would present to the user the drop probabilities of the routers,the delays and the approximate aggregate throughput of the links.The user can then decide to either simulate the scenario in detail by using the packet level en-gine in ns-2or discard it.The data structures of approx-sim are populated by a module within the Tcl space of ns-2[25].The pre-filtering tool thus reduces to a simple Tcl script that runs the approx-sim module within the ns-2framework.The output of the approx-sim would be detailed steady state statistics of the network that can be accessed by the Tcl scripts.This in-formation can be used to write simple pre-filtering tools in Tcl itself.Currently,the approx-sim tool is not tightly in-tegrated into the ns-2framework.It runs in a stand-alone mode.There were certain design choices that went into the de-sign of the pre-filtering framework.Our initial approach was to build a pre-filtering tool along with approx-sim for the user.We wanted it to have features such as to deter-mine whether a scenario has congestion above a threshold. That needed a careful designing of the query language that the user would use and features that we could possibly sup-port.This approach could have limited the capabilities of the tool itself.As a better design choice,we decided to build just the approx-sim and a good user interface to the data structures and the results of the simulation.The user would query the data structure and would take decisions based on the response.This approach has a couple of advantages.First of all,the user has completeflexibility to design the query that would suit his design.Also,the user would not have to learn yet another query-language.From the designers standpoint,it is redundant to redesign a query language that would imitate the functionality of a standard scripting lan-guage like Perl or Tcl,and,at the same time be different. Another important design choice was to build the whole simulator in a plug-and-play fashion so that it is easily scal-able and it is easy to incorporate newer models of network elements and traffic agents.Also,this ensures that inte-grating approx-sim into ns-2is easy.We hope that such a modular design will make the system more suitable for rapid validation of new protocols.4Solving for the Steady-state behav-iorThe approximate-solver is the heart of our pre-filtering tool.It evaluates characteristics of long-lived and short-lived TCP connections,including the throughput of the flows and the delays,drop probabilities and the aggregate throughputs at each router.We next describe how these are accomplished,quickly and approximately.Figure2shows theflowchart we use to solve for the steady-state behavior of the network.We begin with a topology and the details of traffic agents in the topology from the user in the form of a ns-2script.Our module would parse the ns-2script and populate the internal data structures of approx-sim.The user can invoke the approx-sim module by a simple Tcl command from his ns-2script. In step1,the engine,in its simulate procedure,first cal-culates the drop probability and the queuing delays at each router from the previous iteration or the initial conditions (See Figure2).In step2,it uses these router statistics to calculate the end-to-end drop probability and delays en-countered by eachflow which are then used to obtain the per-flow throughput.Step3calculates the total through-put for each link by adding the per-flow throughputs of eachflow that pass through it.Then the algorithm checksFigure2:The structure of the Approx-sim simulator whether convergence of a new network state is achieved. If the network state converges,we terminate.Otherwise we use a scaling algorithm in step4to scale theflows to meet the network constraints,and,we use the results to run another iteration of the procedure,simulate.In step1,we use simple queuing theory for drop tail routers and a new,very simple analytical model for RED[10]gateways.We describe these models below in Section4.1.At the end of this step,we know the drop probabilities,,and the average queuing delay,at each router.The state of router is defined by.The calculations in step2are dependent on whether the flows are short lived or not.For bulkflows,we calcu-late the throughput of eachflow(Section4.2.1).For short livedflows,we calculate their aggregate throughput(Sec-tion4.2.2).An important question is how to guarantee that this process converges and leads to the steady state.We discuss this issue in Section4.4.4.1Modeling network elementsApprox-sim considers models of two different packet queu-ing disciplines:drop-tail and RED[10].For drop-tail routers,we use the M/M/1/K model[17].Let us consider a server processing packets from the TCP connection.Packets arrive at the server at a particular rate(). The server consumes packets at the rate of,which is given by the bandwidth of the link.Packet losses are given by the of the system.Hence we can plot a curve of throughput versus drop probability.Call this.Note that we could have used the more accurateor but that would have added to the complexity to our formalism.We have found the simple models to be fairly accurate as long as the net-work is not very heavily loaded.RED gateways represent a common AQM strategy used in today’s routers.We have developed a simple model to describe the steady state behavior of a RED router.We as-sume that the traffic thatflows into this gateway has also reached its steady state.In Bu et al.’s work[2]the authors used the RED model directly into the TCP equations of Padhye et al.[22].Our approach is different.We deter-mine the drop probability and the average queue length as a function of the steady-state utilization at the router. Without loss of generality,if the service rate of a RED router is unity,the throughput at the router,,will be the same as the utilization of the router queuing system,i.e..Let the queue length at the router be and the drop probability at the router be.The RED characteristics can be expressed byif(2)Lemma4.2.In the steady state,the average queue length will never be larger thanTheorem4.3.If the drop probability seen at this router is and the steady state queue length is between the interval and,the drop probability at this router is given by(3)The above theorem ensures that we can obtain a quadratic equation in.Hence,given a value of(or), we canfind a drop probability.Then,using the value of,we can use Lemma4.1to calculate the queue length and the queuing delay.Next,we define,for every RED router,two parameters and.is the so-lution to the equation2and corresponds to the throughput that causes the buffer length of the RED router to beand the drop probability to be0.Similarly,we can define to be the throughput that causes the buffer length of the RED router to be and the drop probability tobe.By Theorem4.3and the above definitions of and,we can easily calculate the queue length and the drop probability in the following way:If the link throughput is less than,the drop probability is0and the queue length is given by the model.Simi-larly,when the throughput is greater than,the queue length is exactly and the drop probability can be calculated from Theorem4.3.Note that we cannot use Equation1to obtain the drop probability because we are interested in the average drop probability and not the in-stantaneous one.Instead,we are try tofind the average state.If,the drop probability can be computed using Theorem4.3.We should note that we can adapt the above analysis to obtain an iterative method in order to calculate the state of RED routers that use more complex variations like Gentle RED[8].4.2Modeling theflowsIn our work,we assume that allflows are TCPflows.This is reasonable because TCP traffic is known to account for the bulk of the traffic in the Internet.In this section,we refer to the analytical models that have been used in our approx-sim.4.2.1Modeling elephantsPadhye et al.[22]gave the throughput of bulk TCPflows (),or elephants),as a function of the probabil-ity of a loss event()and the round trip time as(4)where is a constant and is the maximum value of the timeout.4.2.2Modeling miceShort lived TCPflows,or mice,have been extensively stud-ied in[3]and[15].These efforts have focused onfind-ing very detailed models to accurately depict the behav-ior of individual short termflows.In contrast,we con-centrated on a much simpler model for aggregates of short termflows.We have refined Ben Fredj et al.[12]model of mice to incorporate the drop probabilities.They validated their work on simple topologies while we validated their model(and our refinement)on more general topologies. We approximate aggregates of shortflow between the same source-destination pair as a smoothfluid.The ratio-nale for this kind of idea is that aggregates being inelastic traffic will have less correlation to the complex feedback mechanism and will be easier to model at a higher level.It is also much faster to determine the behavior of the aggre-gate.Lemma4.4.If the rate of arrival of short livedflows be-tween any source-destination pair is and the data transferred by theflow is,then the rate of shortflow traf-fic between the same source-destination pair is given by(5)For now,we assume that short TCP connections come according to some arrival pattern that is Markovian and each connection transfers a constant amount of data. Lemma4.5.Let the end-to-end drop probability between any source-destination pair be and the arrival rate for shortflows be.The throughput of the mice is given byproofs and arguments.Consider the throughput character-istic of each TCPflow,.We call this curve .It is natural to question the existence of afixed point as the throughput is a surface.We now show that afixed point indeed exists.Lemma4.6.When the probability of loss()or the increases,the throughput of bulk TCPflows on a single link decreases.Proof.Follows from Equation4.Lemma4.7.When the aggregate throughput on a link in-creases,both and increase.Proof.Increasing the throughput implies a higher rate of arrival into the queue.That results in higher queuing delays and increases the and the drop probability.Theorem4.8.The intersection of the curve of the router with has a uniquefixed point.Proof.Assume the contrary.Since our curve as-sumes afixed RTT,it is a straight line.It must intersect the curve at least once.Assume that it inter-sects at two points and.Lemmas4.6,4.7indicate the monotonicity of TCP performance.Assume. By Lemma4.7,drop probabilities increase as we increase from to.But,by Lemma4.6,as drop probabili-ties increase throughput should increase.Thus there is a contradiction.Hence the theorem.Note that we cannot say that the above holds for a net-work with many congested links.Now we discuss the exact procedure to calculate the fixed point of each TCPflow in the network.Let there be a network with edges/links and TCP connections. Let each connection be and let each link be denoted as .Assume that connection passes through edges and denotes the edge set of this connection.Let the delays of each link be,the drop probability be.Also if we assume that the connection goes through the link,it will occur a drop of and a delay a of and this link is denoted by.Now is equal to,is equal to for some link.Intuitively,the procedure is as follows:we use the ap-propriate model at each router to calculate the link delays and drops experienced at each router by all theflows go-ing through that link.These link characteristics are used to estimate the end-to-end round trip time and the drop prob-abilities seen by eachflow at the sender.We can then use either Equation4or Lemma4.5to calculate the throughput of the TCPflows.Hence we have(8)For example,is the link in the path of connection ,and,hence,it can be denoted by for some.Now we can write asfor some(9) where denotes the queuing delay of the link and is the propagation delay of the link.The RTT seen from the end-point,i.e.the sender,for a connection is denoted by and is given by(10)where is the number of links traversed by connection. If we make the assumption that packet losses are inde-pendent on each link,the following theorem is obvious Lemma4.9.The drop probability seen by the connection ,is given by(11)Hence,the throughput of a TCPflow,can now be calculated by(12) 4.4Initial conditions and convergenceIt is trivial to design an algorithm to calculate the approx-imate steady state throughput from the discussion in the preceding sub-section.Thus the algorithm is shown in Fig-ure3.The above algorithm requires us to start with the correct initial values of for each link.But,we do not want to make any assumptions apriori on the state of the network. Without such a restriction we can always solve the network in the following fashion:Run ns-2for a few seconds in virtual time.The throughput of each link will give us the initial s for approx-sim.A more elegant solution is not to use any prior knowledge of the intermediate ns-2results. This is our approach.4.4.1Our approach to convergenceInitially we assume that the links are not loaded when there are only bulkflows or elephants present.We argue that the load due to the elasticflows is such that they will share all the available bandwidth.In thisfirst iteration of ourfixed point algorithm,the bulk TCPflows get what Equation4 with low drop probabilities.That may result in window limited or large throughput.Now we run the algorithm andset initial conditionswhile(convergence not reached)doif(not initial)then scale connectionsfor i=1to nLinks docalculate the queueing delays and drop probabilities endforfor j=i to nConnections dosum the drops and delays from the link listof edge for connection icalculate throughput from the TCP equationsendforfor i=1to nLinks docalculate the total throughput of each linkendforendwhileFigure3:Aggregation algorithm after every round offixed pointcompute the new throughput of each connection and sum them up tofind the new s of each of the links.This gives us the initial throughput.The throughput of a bulk connection(elephant)is very sensitive to small changes in probability,which makes it hard to achieve convergence using the iterative process de-scribed earlier.Specifically,if the drop probability is very low,then the computed throughput of the bulk connections on a link can be much higher than the capacity of the link. To speed up convergence,we scale down the computed throughput of bulk connections so that link capacities are not exceeded.A brief description of the scaling algorithm is given below.The scaling algorithm:Let represent the computed throughput of the bulkflows after each iter-ation of thefixed point algorithm.Initially,we mark each bulkflow as being unscaled.For each link define,the unscaled capacity,as the capacity of the link minus the throughput of all the shortflows(mice)on this link.Also, for each link,define to be the combined throughput of all the unscaled bulkflows on the link.Define the conges-tion as.Now,we repeat the following process. while there exists a link with:Let denote the link with the largest value of.Scale down the throughput of all the unscaled bulkflows using this link by a factor, and mark all theseflows as being scaled.Now the total throughput of this link exactly matches the capacity of the link and hence=1.For each newly scaledflow,and each link such thatflow uses link,we reduce the unscaled capacity of link by the new throughput of flow and the combined throughput of link by the old unscaled throughput offlow..When the above algorithm terminates,the throughput on any link does not exceed its capacity.In practice,we found the scaling step to be critical for fast convergence.We call this step Link capping.This step ensures that a particular link is put back into a stable state before the averaging pro-cess in the convergence algorithm discussed in the previous subsection.The performance of the scaling algorithm is given by the following theorem:Theorem4.10.The worst case running time,,of the scaling algorithm on a network of size connections, links is given(13) where is the average number of links traversed by each connectionProof.Finding the most congested link takestime with suitable data structures.When we scale each connection,we need to change the unscaled capacity of links.This takes time with suitable datastructures.where is the number of links the connection traverses.Hence the theorem.5Evaluation and ResultsWe next evaluate how well approx-sim meets its three goals:speed,accuracy,and generality.First,we con-sider its performance relative to packet-level simulation. Second,we show that it is reasonably accurate,typically within10–15%of packet-level simulation for the scenar-ios we consider.Only some scenarios were20%accurate but they were under very heavy load.A very high level of accuracy is not required for approx-sim because we expect final simulation results to be done with packet-level simu-lation;approx-sim merely selects those scenarios.Finally,A BFigure4:The linetopologyFigure5:The symmetric tree topology:a sample binary tree of height with four clients at each leaf.we evaluate the generality of approx-sim by showing that it is applicable to increasingly complex scenarios in terms of traffic mix,topology and network elements.In this entire section,we use a particular terminology. Long livedflows and elephants are used interchangeably. Similarly we refer to short lived TCPflows as mice.For throughput,units of packets/s and kB/s are used inter-changeably since all our simulations use a packet size of1kB.We start with simple topologies topologies(lines and symmetric trees)and move to more complex topolo-gies(asymmetric trees and circular topologies)to validate approx-sim progressively.5.1Elephant traffic aloneFirst we consider results that we obtained for the experi-ments with elephant-only traffic.We evaluated approx-sim on the line topology(Figure4)as well as symmetric(Fig-ure5)and asymmetric trees(Figure8).This gradual in-crease in the complexity of the topologies will help us to evaluate approx-sim with just bulkflows.The line topology shows good accuracy between ns-2 and approx-sim so we jump directly to symmetric trees. Symmetric trees were initially chosen because it allows us to study the effect of many similarflows passing through a bottleneck link.Figure5shows the symmetric tree topol-ogy.We place the TCP sinks at the bottleneck link i.e.at the root of the tree,and four bulk TCP sources at each of the leaves of the tree.All the links are assumed to have a capacity of1Mb/s.Figure6shows the run-time performance of approx-simFigure6:Running time comparisons between approx-sim and ns-2for the symmetric tree topology with elephanttraffic onlycompared to packet-level simulation with ns-2for symmet-ric trees as a function of tree height.Approx-sim is10-300faster than packet-level simulation.Although the performance of both approx-sim and ns-2is linear withnetwork size(and increases exponentially as a function of tree height),the very large difference in constant factor makes approx-sim one to two orders of magnitude faster than packet-level simulation.Speed is not useful if the simulation is completely inac-curate.Figure7compares approx-sim and ns-2accuracy by evaluating meanflow bandwidth for the bottleneck link. (No error bars are shown in this case because approx-sim is deterministic and the standard deviation between the ns-2flows is less than5%.)This graph shows that approx-sim is quite accurate compared to ns-2.The simulators are typ-ically with10–20%;the worst case is with a hight of8 when the network is very heavily loaded where they are 40%apart.approx-sim is more accurate when we look at aggregates of manyflows.The accuracy is much higher for the links close to the root.At the root bottleneck link, the accuracy was7.6%.We have also conducted exper-iments for high link capacities and the results have been better with less utilization.Next we consider asymmetric trees(as shown in Fig-ure8)to avoid biases in evaluation due to symmetry.We examine asymmetric trees of varying heights.Figure8 shows a tree with height two.In general,we construct an asymmetric tree of height by expanding the leftmost node of a tree of height to have two children.All traffic terminates at the lower-left-most node of the tree;traffic begins at all the other leaves of the tree with elephants. Early comparisons of results for asymmetric trees show large differences between approx-sim and ns-2.In ns-2all long RTTflows(eg.between nodes,,Figure8)had very low throughput while short RTTflows(eg.between。
硕士论文_无线传感器网络定位算法的研究
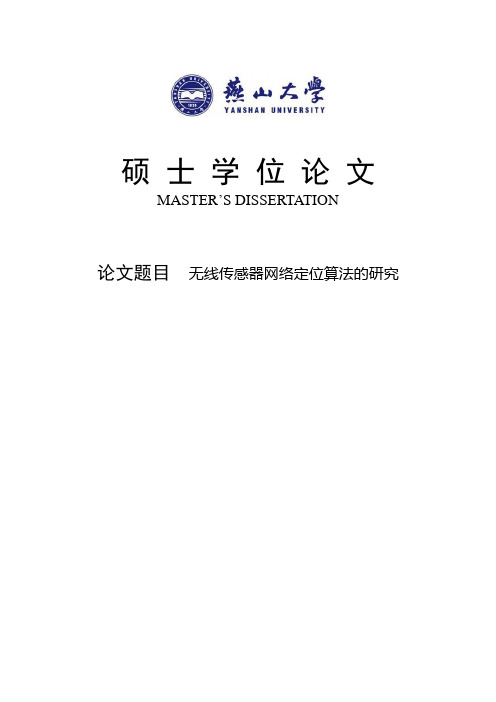
硕士学位论文MASTER’S DISSERTATION论文题目无线传感器网络定位算法的研究A Dissertation in Computer Application TechnologySTUDY ON LOCALIZATION ALGORITHM OF WIRELESS SENSOR NETWORKby Hu YulanSupervisor: Professor Wang XinshengYanshan University2011.12燕山大学硕士学位论文原创性声明本人郑重声明:此处所提交的硕士学位论文《无线传感器网络定位算法的研究》,是本人在导师指导下,在燕山大学攻读硕士学位期间独立进行研究工作所取得的成果。
据本人所知,论文中除已注明部分外不包含他人已发表或撰写过的研究成果。
对本文的研究工作做出重要贡献的个人和集体,均已在文中以明确方式注明。
本声明的法律结果将完全由本人承担。
作者签字日期:年月日燕山大学硕士学位论文使用授权书《无线传感器网络定位算法的研究》系本人在燕山大学攻读硕士学位期间在导师指导下完成的硕士学位论文。
本论文的研究成果归燕山大学所有,本人如需发表将署名燕山大学为第一完成单位及相关人员。
本人完全了解燕山大学关于保存、使用学位论文的规定,同意学校保留并向有关部门送交论文的复印件和电子版本,允许论文被查阅和借阅。
本人授权燕山大学,可以采用影印、缩印或其他复制手段保存论文,可以公布论文的全部或部分内容。
保密□,在年解密后适用本授权书。
本学位论文属于不保密□。
(请在以上相应方框内打“√”)作者签名:日期:年月日导师签名:日期:年月日摘要摘要传感器节点的位置信息在无线传感器网络的监测活动等应用中起着至关重要的作用。
而取得节点位置信息较简便、快捷、精确的方法是通过手动设定或携带GPS 定位设备等手段,但通过这种方式获取的成本很高。
因此,较好的方法是采用定位算法进行估计。
本文将主要研究基于多维标度的无线传感器网络定位算法。
- 1、下载文档前请自行甄别文档内容的完整性,平台不提供额外的编辑、内容补充、找答案等附加服务。
- 2、"仅部分预览"的文档,不可在线预览部分如存在完整性等问题,可反馈申请退款(可完整预览的文档不适用该条件!)。
- 3、如文档侵犯您的权益,请联系客服反馈,我们会尽快为您处理(人工客服工作时间:9:00-18:30)。
Exploiting network proximity in peer-to-peer overlaynetworksMiguel Castro Peter Druschel Y.Charlie Hu Antony Rowstron Microsoft Research,7J J Thomson Close,Cambridge,CB30FB,UK.Rice University,6100Main Street,MS-132,Houston,TX77005,USA.Purdue University,1285EE Building,West Lafayette,IN47907,USA.AbstractGeneric peer-to-peer(p2p)overlay networks like CAN, Chord,Pastry and Tapestry offer a novel platform for a va-riety of scalable and decentralized distributed applications. These systems provide efficient and fault-tolerant routing, object location and load balancing within a self-organizing overlay network.One important aspect of these systems is how they exploit network proximity in the underlying Inter-net.In this paper,we present a comprehensive study of the network locality properties of a p2p overlay network.Results obtained via analysis and via simulation of two large-scale topology models indicate that it is possible to efficiently ex-ploit network proximity in self-organizing p2p substrates.A simple heuristic measures a scalar proximity metric among a small number of nodes,incurring only a modest additional overhead for organizing and maintaining the overlay net-work.The resulting locality properties improve application performance and reduce network usage in the Internet sub-stantially.Finally,we study the impact of proximity-based routing on the load balancing in the p2p overlay.1IntroductionSeveral recent systems(CAN[6],Chord[10],Pastry[7]and Tapestry[14])provide a self-organizing substrate for large-scale peer-to-peer applications.These systems can be viewed as providing a scalable,fault-tolerant distributed hash table, in which any item can be located within a bounded number of routing hops,using a small per-node routing table.While there are algorithmic similarities among each of these sys-tems,one important distinction lies in the approach they take to considering and exploiting proximity in the underlying In-ternet.Chord,for instance,does not currently consider net-work proximity at all.As a result,its protocol for maintaining the overlay network is very light-weight,but messages may travel arbitrarily long distances in the Internet in each routing hop.In CAN,each node measures its network delay to a set of landmark nodes,in an effort to determine its relative position in the Internet and to construct an Internet topology aware overlay.Tapestry and Pastry exploit locality by measuring a proximity metric among pairs of nodes,and by choosing nearby nodes for inclusion in their routing tables.Early re-sults for the resulting locality properties are promising.In Tapestry and Pastry,for instance,the average total“distance”traveled by a message is only a small and constant factor larger than the“distance”between source and destination in the underlying network.However,these results come at the expense of more expensive overlay maintenance protocol, relative to Chord.Also,proximity based routing may com-promise the load balance in the p2p overlay network.More-over,it remains unclear to what extent the locality properties hold in the actual Internet,with its complex,dynamic,and non-uniform topology.As a result,the cost and effectiveness of proximity based routing in these p2p overlays remain un-clear.To address these questions,this paper presents results of a comprehensive study of Pastry’s locality properties via anal-ysis and via simulations based on two large-scale Internet topology models.Moreover,we propose an improved node join and failure protocol that substantially decreases the over-lay maintenance cost relative to the original implementa-tion[7],at the expense of a negligible reduction in the quality of the Pastry’s routing properties.The results indicate that the locality properties are robust on a variety of network topol-ogy models.Moreover,the load imbalance caused by the proximity based routing is modest,and hot spots can be eas-ily dispersed without affecting overall route quality.While our analysis and simulations are based on Pastry,many of our results apply to Tapestry and Chord as well.We conclude that it is possible to exploit network proximity in p2p overlay networks with low overhead and without compromising their self-organizing and load balancing properties.The rest of this paper is organized as follows.Related work is discussed in Section2.In Section3,we provide a brief overview of the Pastry protocol.Pastry’s locality properties, and the new protocols for node joining and failure recovery are presented in Section4.An analysis of Pastry’s localityproperties follows in the Section5.Section6presents exper-imental results,and we conclude in Section7.2Related workCAN[6],Chord[10]and Tapestry[14]are closely related to Pastry.Each of these protocols form a self-organizing over-lay network and provide a load-balanced,fault-tolerant dis-tributed hash table,in which items can be inserted and looked up in a bounded number of forwarding hops.CAN,Tapestry and Pastry each use heuristics to exploit proximity in the In-ternet,and the resulting properties have been studied in prior work.To the best of our knowledge,this paper is thefirst study that looks at both costs and benefits of proximity based routing in a p2p overlay,and considers the impact of node failures on those costs and benefits.Pastry and Tapestry are related to the work by Plaxton et al.[5]and to routing in the landmark hierarchy[12].The approach of routing based on address prefixes,which can be viewed as a generalization of hypercube routing,is common to all these schemes.However,neither Plaxton nor the land-mark approach are fully self-organizing.Pastry and Tapestry differ in their approach to locating the numerically closest node in the sparsely populated nodeId space,and for man-aging replication.Pastry uses overlapping sets of neighbor-ing nodes in the nodeId space(leaf sets),both to locate the destination in thefinal routing hop,and to store replicas of data items for fault tolerance.Tapestry uses a different con-cept called surrogate routing to locate the destination,and it inserts replicas of data items using different keys.The ap-proach to achieving network locality is very similar in both systems.The Chord protocol is closely related to Pastry and Tapestry,but instead of routing based on address prefixes, Chord forwards messages based on numerical difference with the destination address.Unlike Pastry and Tapestry,Chord currently makes no explicit effort to exploit network proxim-ity.However,locality heuristics similar to the ones used in Pastry could presumably be added to Chord.CAN routes messages in a-dimensional space,where each node maintains a routing table with entries and any node can be reached in routing hops.Un-like Pastry,Tapestry and Chord,the CAN routing table does not grow with the network size,but the number of routing hops grows faster than.The work on CAN[6]ex-plored two techniques to improve routing performance by us-ing information about the network topology.In thefirst tech-nique,each node measures the RTT to each of its neighbors and messages are forwarded to the neighbor with the max-imum ratio of progress to RTT.This technique differs from the one used in Pastry because the set of neighbors of a node is chosen without regard to their proximity;this has the dis-advantage that all neighbors may be quite far from the node.The second technique measures the distances between each node and a set of landmark servers to compute the coordi-nates of the node in the CAN space such that neighbors inthe CAN space are topologically close.This technique can achieve good performance but it has the disadvantage that itis not fully self-organizing;it requires a set of well-known landmark servers.In addition,it may cause significant im-balances in the distribution of nodes in the CAN space thatlead to hotspots.Existing applications built on top of Pastry includePAST[8]and SCRIBE[9].Other peer-to-peer applicationsthat were built on top of generic routing and location sub-strates are OceanStore[3](Tapestry)and CFS[2](Chord). 3PastryPastry is described in detail in[7],and a brief summary isprovided here.Pastry is a generic,efficient,scalable,fault re-silient,and self-organizing peer-to-peer substrate.Each Pas-try node has a unique,uniform randomly assigned nodeId in a circular128-bit identifier space.Given a128-bit key,Pas-try routes an associated message towards the live node whosenodeId is numerically closest to the key.Moreover,each Pas-try node keeps track of its neighboring nodes in the names-pace and notifies application of changes in the set.Thesecapabilities can be used to build a distributed,fault-tolerant hashtable,which in turn can be used to support a variety ofdecentralized,distributed applications.Assuming a network consisting of nodes,the expectednumber of forwarding hops to deliver a messages with arandom key is(is a configuration parameter with typical value4).The tables required in each node haveonly entries,where each entry maps an existingnodeId to the associated node’s IP address.Upon a node fail-ure or the arrival of a new node,the invariants in all affected tables can be restored by exchanging messages. In the following paragraphs,we briefly sketch the Pastry rout-ing scheme.Node state:For the purposes of routing,nodeIds and keys are thought of as a sequence of digits in base.A node’s routing table is organized into rows and columns.The entries in row of the routing table refer to nodes whose nodeIds share thefirst digits with the present node’s nodeId;the th nodeId digit of a node in column of row equals.The column in row corre-sponding to the value of the’s digits of the local node’s nodeId remains empty.Figure1depicts an example routing table.A routing table entry is also left empty if no node with the appropriate nodeId prefix is known.The uniform ran-dom distribution of nodeIds ensures an even population of the nodeId space;thus,on average only levels areFigure1:Routing table of a Pastry node with nodeId,.Digits are in base16, represents an arbitrary suffix.The IP address associated with each entry is not shown.d467c4d471f1OFigure2:Routing message fromwith key The dotsin Pastry’s circular namespace.populated in the routing table.Each node maintains IP ad-dresses for the nodes in its leaf set.The leaf set is the set of nodes with nodeIds that are numerically closest to the present node’s nodeId,with larger and smaller nodeIds than the current node’s id.A typical value for is approximately.Message routing:At each routing step,a node normally forwards the message to a node whose nodeId shares with the key a prefix that is at least one digit(or bits)longer than the prefix that the key shares with the present node’s id.If no such node is known,the message is forwarded to a node whose nodeId shares a prefix with the key as long as the current node,but is numerically closer to the key than the present node’s id.Such a node is guaranteed to exist in the leaf set unless the message has already arrived at the node with numerically closest nodeId,or its immediate neighbor1. And,unless all nodes in one half of the leaf set have failed simultaneously,at least one of those nodes must be live. The Pastry routing procedure is shown in Figure3.Fig-ure2shows the path of an example message.Analysis shows that the expected number of forwarding hops is slightly below ,with a distribution that is tight around the mean.A deterministic upper bound for the number of routing hops is 128/b+1,assuming correct routing tables and no concurrent node failures.Moreover,simulation shows that the routing is highly resilient to node failures.To achieve self-organization,Pastry must dynamically maintain its node state,i.e.,the routing table and leaf set, in the presence of new node arrivals,node failures,node re-coveries,and network partitions.to the initial members of its leaf set,which in turn updatetheir own leaf sets and routing tables.Given an appropriate leaf set size(e.g.,),one can show that,with high prob-ability,all nodes whose routing tables are affected by’sarrival are notified.Node failure:When a node fails,the leaf sets and routing tables of a number of other nodes must be updated.Leaf set memberships is actively maintained.The members of each leaf set periodically exchange keep-alive messages.If a node is unresponsive for a period,it is presumed failed.All members of the failed node’s leaf set are then notified and they update their leaf sets.Since the leaf sets of nodes with adjacent nodeIds overlap,this update is trivial.Routing table entries that refer to failed nodes are repaired lazily.During message forwarding,when a routing table en-try is found that is either empty or the referenced node has failed,Pastry routes the message to another node with numer-ically closer nodeId(lines11-13in Figure3).If the down-stream node has a routing table entry that matches the next digit of the message’s key,it automatically informs the up-stream node of that entry.Node recovery:The node recovery protocol is optimized to reduce the overhead of temporary node failures.A recov-ering Pastry nodefirst attempts to contact nodes in its last known leaf set and obtains their leaf sets.If the numerical range of nodeIds in one of those sets still includes the recov-ering node’s nodeId,the node updates its own leaf set based on the information it receives and then notifies the current members of its leaf set of its presence.Otherwise,the node follows the normal protocol for node addition.Network partitions:A network partition can cause the ap-parent simultaneous failure of a large number of nodes at random points in the nodeId space.In extreme cases,such a partition could cause the failure of nodes with adjacent nodeIds.This(rare)case requires a special recovery proce-dure,since live nodes that are separated by or more failed nodes in the nodeId space are not aware of each other.Briefly, the live nodes at the edges of such a sequence of failed nodes locate each other by sending messages towards the other us-ing their remaining live routing table entries,then form a new leaf set.4Pastry locality propertiesThis section focuses on Pastry’s locality properties.Pastry seeks to exploit proximity in the underlying Internet,by rout-ing through as short a path as possible,finding nearest copies of objects,etc.It relies on a scalar proximity metric that indi-cates the“distance”between any given pair of Pastry nodes.It is assumed that each Pastry node can measure or other-wise obtain the distance between itself and any node with a known IP address.Furthermore,it is assumed that the prox-imity metric reflects static properties of the underlying phys-ical network,rather than prevailing traffic conditions.The choice of a proximity metric depends on the desired qualities of the resulting overlay(e.g.,low delay,high band-width,low network utilization).In practice,metrics such as round-trip time(minimum of a series of pings),bandwidth (measured,for instance,using packet pair techniques),the number of IP routing hops(measured using traceroute),or some combination thereof could be used.Choosing an ap-propriate proximity metric for p2p overlay networks is the subject of future work and beyond the scope of this paper. Pastry’s locality properties derive from its attempt to mini-mize the distance,according to the proximity metric,to each of the nodes that appear in a node’s routing table,subject to the constraints imposed on nodeId prefixes.It is expensive to achieve this goal precisely in a large system because it re-quires communication.Therefore,Pastry uses heuris-tics that require only communication but only en-sure that routing table entries are close but not necessarily the closest.More precisely,Pastry ensures the following invari-ant for each node’s routing table:Proximity invariant:Each entry in a node’s routing ta-ble refers to a node that is near,according to the proxim-ity metric,among all live Pastry nodes with the appropriate nodeId prefix.In Section4.1,we show how Pastry’s node joining pro-tocol maintains the proximity invariant.Next,we consider the effect of the proximity invariant on Pastry’s routing.Ob-serve that as a result of the proximity invariant,a message is normally forwarded in each routing step to a nearby node, according to the proximity metric,among all nodes whose nodeId shares a longer prefix with the key.Moreover,the ex-pected distance traveled in each consecutive routing step in-creases exponentially,because the density of nodes decreases exponentially with the length of the prefix match.From this property,one can derive three distinct properties of Pastry with respect to network locality:Total distance traveled The expected distance of the last routing step tends to dominate the total distance traveled by a message.As a result,the average total distance traveled by a message exceeds the distance between source and destination node only by a small constant value.Analysis and simula-tions on two Internet topology models presented in Section6 confirm this.Local route convergence The paths of two Pastry messages sent from nearby nodes with identical keys tend to converge at a node near the source nodes,in the proximity space.To see this,observe that in each consecutive routing step,the messages travel exponentially larger distances towards an ex-ponentially shrinking set of nodes.Thus,the probability of a route convergence increases in each step,even in the casewhere earlier(smaller)routing steps have moved the mes-sages farther apart.This result has significance for cachingapplications layered on Pastry.Popular objects requested bya nearby node and cached by all nodes along the route are likely to be found when another nearby node requests the ob-ject.Also,this property is exploited in Scribe[9]to achieve low link stress in an application level multicast system. Locating the nearest replica If replicas of an object arestored on nodes with adjacent nodeIds,Pastry messages re-questing the object have a tendency tofirst reach a node near the client node.To see this,observe that Pastry messages ini-tially take small steps in the proximity space,but large stepsin the nodeId space.Applications can exploit this property to make sure that client requests for an object tend to be handled by a replica that is near the client.Exploiting this property is application-specific,and is discussed in[8].An analysis of these properties follows in Section5.Sim-ulation results that confirm and quantify these properties ontwo Internet topology models follow in Section6.4.1Node addition and failureNext,we present the Pastry node join protocol and show howthis protocol maintains the proximity invariant.First,recall from Section3that a new node must con-tact an existing Pastry node when joining the system. then routes a message using as the key,and the new node obtains the th row of its routing table from the node encoun-tered along the path from to whose nodeId matches in thefirst digits.We will show that the proximity in-variant holds on’s resulting routing table,if node is near ,according to the proximity metric.First,consider the top row of’s routing table,obtainedfrom node.Assuming the triangulation inequality holds in the proximity space,it is easy to see that the entries in the top row of’s routing table are also close to.Next,consider the th row of’s routing table,obtained from the node encountered along the path from to.By induction,this node is Pastry’s approximation to the node closest to that matches’s nodeId in thefirst digits.Therefore,if the triangulation inequality holds,we can use the same argument to conclude that the entries of the th row of’s routing table should be close to.At this point,we have shown that the proximity invariantholds in’s routing table.To show that the node join pro-tocol maintains the proximity invariant globally in all Pastry nodes,we must next show how the routing tables of other affected nodes are updated to reflect’s arrival.Once has initialized its own routing table,it sends the th row of its routing table to each node that appears as an entry in that row.This serves both to announce its presence and to propa-gate information about nodes that joined previously.Each of the nodes that receives a row then inspects the entries in the row,performs probes to measure if or one of the entries is nearer than the corresponding entry in its own routing table, and updates its routing table as appropriate.To see that this procedure is sufficient to restore the prox-imity invariant in all affected nodes,consider that and the nodes that appear in row of’s routing table form a group of nearby nodes whose nodeIds match in thefirst digits.It is clear that these nodes need to know of’s arrival,since may displace a more distant node in one of the node’s rout-ing tables.Conversely,a node with identical prefix in thefirst digits that is not a member of this group is likely to be more distant from the members of the group,and therefore from; thus,’s arrival is not likely to affect its routing table and, with high probability,it does not need to be informed of’s arrival.Node failure Recall that failed routing tables entries are re-paired lazily,whenever a routing table entry is used to route a message.Pastry routes the message to another node with numerically closer nodeId(lines11-13in Figure3).If the downstream node has a routing table entry that matches the next digit of the message’s key,it automatically informs the upstream node of that entry.We need to show that the entry supplied by this procedure satisfies the proximity invariant.If a numerically closer node can be found in the routing table,it must be an entry in the same row as the failed node.If that node supplies a substi-tute entry for the failed node,its expected distance from the local node is therefore low,since all three nodes are part of the same group of nearby nodes with identical nodeId prefix. On the other hand,if no replacement node is supplied by the downstream node,we trigger the routing table maintenance task(described in the next section)tofind a replacement en-try.In either case,the proximity invariant is preserved.4.2Routing table maintenanceThe routing table entries produced by the node join protocol and the repair mechanisms are not guaranteed to be the clos-est to the local node.Several factors contribute to this,in-cluding the heuristic nature of the node join and repair mech-anisms with respect to locality.Also,many practical proxim-ity metrics do not strictly satisfy the triangulation inequality and may vary over time.However,limited imprecision is consistent with the proximity invariant,and as we will show in Section6,it does not have a significant impact on Pastry’s locality properties.However,one concern is that deviations could cascade, leading to a slow deterioration of the locality properties over time.To prevent a deterioration of the overall route qual-ity,each node runs a periodic routing table maintenance task (e.g.,every20minutes).The task performs the following procedure for each row of the local node’s routing table.It selects a random entry in the row,and requests from the as-sociated node a copy of that node’s corresponding routingtable row.Each entry in that row is then compared to the corresponding entry in the local routing table.If they differ, the node probes the distance to both entries and installs the closest entry in its own routing table.The intuition behind this maintenance procedure is to ex-change routing information among groups of nearby nodes with identical nodeId prefix.A nearby node with the ap-propriate prefix must be know to at least one member of the group;the procedure ensures that the entire group will even-tually learn of the node,and adjust their routing tables ac-cordingly.Whenever a Pastry node replaces a routing table entry be-cause a closer node was found,the previous entry is kept in a list of alternate entries(up to ten such entries are saved in the implementation).When the primary entry fails,one of the alternates is used until and unless a closer entry is found during the next periodic routing table maintenance.4.3Locating a nearby nodeRecall that for the node join algorithm to preserve the prox-imity invariant,the starting node must be close to the new node,among all live Pastry nodes.This begs the question of how a newly joining node can detect a nearby Pastry node. One way to achieve this is to perform an“expanding ring”IP multicast,but this assumes the availability of IP multicast.In Figure4,we present an efficient algorithm by which a node may discover a nearby Pastry node,given that it has knowl-edge of some Pastry node at any location.Thus,a joining node is only required to obtain knowledge of any Pastry node through out-of-band means,as opposed to obtaining knowl-edge of a nearby node.The algorithm exploits the property that location of the nodes in the seeds’leaf set should be uni-formly distributed over the network.Next,having discovered the closest leaf set member,the routing table distance proper-ties are exploited to move exponentially closer to the location of the joining node.This is achieved bottom up by picking the closest node at each level and getting the next level from it.The last phase repeats the process for the top level until no more progress is made.In this section,we have shown at an intuitive level why the Pastry node join protocol preserves the proximity invari-ant,and how Pastry’s locality properties can be derived from the proximity invariant.However,as part of this argument, we have relied on a few assumptions that do not generally hold in the Internet.For instance,the triangulation inequality does not generally hold for most practical proximity metrics in the Internet.Also,nodes are not uniformly distributed in the resulting proximity space.Therefore,it is necessary to confirm the robustness of Pastry’s locality properties using simulations on Internet topology models.Results of simula-tions based two Internet topology models will be presented in Section6.(1)discover(seed)(2)nodes=getLeafSet(seed)(3)forall node in nodes(4)nearNode=closerToMe(node,nearNode)(5)depth=getMaxRoutingTableLevel(nearNode)(6)while(depth0)(7)nodes=getRoutingTable(nearNode,depth--)(8)forall node in nodes(9)nearNode=closerToMe(node,nearNode)(10)end while(11)do(12)nodes=getRoutingTable(nearNode,0)(13)currentClosest=nearNode(14)forall node in nodes(15)nearNode=closerToMe(node,nearNode)(16)while(currentClosest!=nearNode)(17)return nearNodeFigure4:Simplified nearby node discovery algorithm.seed is the Pastry node initially known to the joining node.5AnalysisIn this section,we present analytical results for Pastry’s rout-ing properties.First,we analyze the distribution of the num-ber of routing hops taken when a Pastry message with a ran-domly chosen key is sent from a randomly chosen Pastry node.This analysis then forms the basis for an analysis of Pastry’s locality properties.Throughout this analysis,we as-sume that each Pastry node has a perfect routing table.That is,a routing table entry may be empty only if no node with an appropriate nodeId prefix exists,and all routing table entries point to the nearest node,according to the proximity metric, with the appropriate nodeId prefix.In practice,Pastry does not guarantee perfect routing tables.Simulation results pre-sented in Section6show that the performance degradation due to this inaccuracy is minimal.Due to space constraints, the details of the analysis and the proofs are omitted here; they are avaliable at :8080.5.1Route probability matrixThe analysis of the distribution of the number of routing hops is based on the statistical population of the nodeId space. Since the assignment of nodeIds is assumed to be randomly uniform,this population can be captured by the binomial dis-tribution(see,for example,[1]).For instance,the distribution of the number of nodes with a given value of the most signif-icant nodeId digit,out of nodes,is given by. Recall from Figure3that at each node,a message can be forwarded using one of three branches in the forwarding pro-cedure.In case,the message is forwarded using the leaf set(line3);in case using the routing table(line8);。