A Model of Coverage Probability under Shadow Fading
北美精算师C考试内容2013-Oct-exam-C
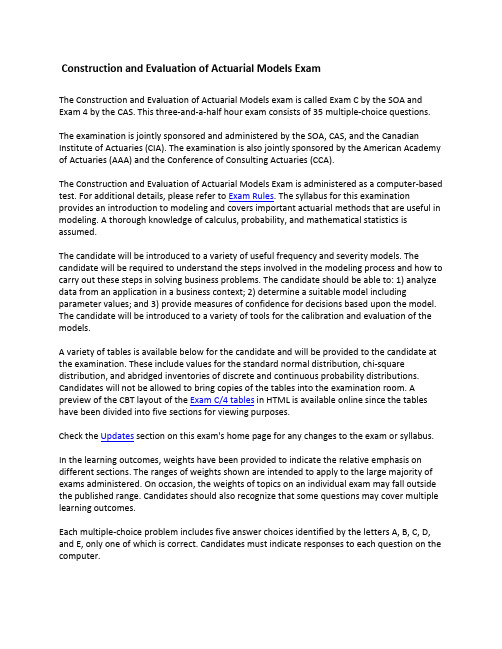
Construction and Evaluation of Actuarial Models ExamThe Construction and Evaluation of Actuarial Models exam is called Exam C by the SOA and Exam 4 by the CAS. This three-and-a-half hour exam consists of 35 multiple-choice questions. The examination is jointly sponsored and administered by the SOA, CAS, and the Canadian Institute of Actuaries (CIA). The examination is also jointly sponsored by the American Academy of Actuaries (AAA) and the Conference of Consulting Actuaries (CCA).The Construction and Evaluation of Actuarial Models Exam is administered as a computer-based test. For additional details, please refer to Exam Rules. The syllabus for this examination provides an introduction to modeling and covers important actuarial methods that are useful in modeling. A thorough knowledge of calculus, probability, and mathematical statistics is assumed.The candidate will be introduced to a variety of useful frequency and severity models. The candidate will be required to understand the steps involved in the modeling process and how to carry out these steps in solving business problems. The candidate should be able to: 1) analyze data from an application in a business context; 2) determine a suitable model including parameter values; and 3) provide measures of confidence for decisions based upon the model. The candidate will be introduced to a variety of tools for the calibration and evaluation of the models.A variety of tables is available below for the candidate and will be provided to the candidate at the examination. These include values for the standard normal distribution, chi-square distribution, and abridged inventories of discrete and continuous probability distributions. Candidates will not be allowed to bring copies of the tables into the examination room. A preview of the CBT layout of the Exam C/4 tables in HTML is available online since the tables have been divided into five sections for viewing purposes.Check the Updates section on this exam's home page for any changes to the exam or syllabus. In the learning outcomes, weights have been provided to indicate the relative emphasis on different sections. The ranges of weights shown are intended to apply to the large majority of exams administered. On occasion, the weights of topics on an individual exam may fall outside the published range. Candidates should also recognize that some questions may cover multiple learning outcomes.Each multiple-choice problem includes five answer choices identified by the letters A, B, C, D, and E, only one of which is correct. Candidates must indicate responses to each question on the computer.As part of the computer-based testing process, a few pilot questions will be randomly placed in the exam (paper and pencil and computer-based forms). These pilot questions are included to judge their effectiveness for future exams, but they will NOT be used in the scoring of this exam.1 All other questions will be considered in the scoring. All unanswered questions are scored incorrect. Therefore, candidates should answer every question on the exam. There is no set requirement for the distribution of correct answers for the SOA/CAS/CIA multiple-choice preliminary examinations. It is possible that a particular answer choice could appear many times on an examination or not at all. Candidates are advised to answer each question to the best of their ability, independently from how they have answered other questions on the examination.Because the CBT exam will be offered over a period of a few days, each candidate will receive a test form composed of questions selected from a pool of questions. Statistical scaling methods are used to ensure within reasonable and practical limits that, during the same testing period of a few days, all forms of the test are comparable in content and passing criteria. The methodology that has been adopted is used by many credentialing programs that give multiple forms of an exam.LEARNING OUTCOMESThe candidate is expected to be familiar with survival, severity, frequency and aggregate models, and use statistical methods to estimate parameters of such models given sample data. The candidate is further expected to identify steps in the modeling process, understand the underlying assumptions implicit in each family of models, recognize which assumptions are applicable in a given business application, and appropriately adjust the models for impact of insurance coverage modifications.Specifically, the candidate is expected to be able to perform the tasks listed below. Items in italic font are additions or replacements with respect to the June 2013 syllabus.Sections A–E have a combined weight of 15-20%.A. Severity Models1. Calculate the basic distributional quantities:a) momentsb) Percentilesc) Generating functions2. Describe how changes in parameters affect the distribution.3. Recognize classes of distributions and their relationships.4. Apply the following techniques for creating new families of distributions:a) Multiplication by a constant1 Beginning with the October 2013 examination there are some revised learning objectives and readings. Items covering them may appear as scored or pilot items in the same manner as items on continuing topics.b) Raising to a powerc) Exponentiation,d) Mixing5. Identify the applications in which each distribution is used and reasons why.6. Apply the distribution to an application, given the parameters.7. Calculate various measures of tail weight and interpret the results to compare the tailweights.8. Identify and describe two extreme value distributions.B. Frequency ModelsFor the Poisson, Mixed Poisson, Binomial, Negative Binomial, Geometric distribution and mixtures thereof:1. Describe how changes in parameters affect the distribution,2. Calculate moments,3. Identify the applications for which each distribution is used and reasons why,4. Apply the distribution to an application given the parameters.5. Apply the zero-truncated or zero-modified distribution to an application given theparametersC. Aggregate Models1. Compute relevant parameters and statistics for collective risk models.2. Evaluate compound models for aggregate claims.3. Compute aggregate claims distributions.D. For severity, frequency and aggregate models1. Evaluate the impacts of coverage modifications:a) Deductiblesb) Limitsc) Coinsurance2. Calculate Loss Elimination Ratios.3. Evaluate effects of inflation on losses.E. Risk Measures1. Calculate VaR, and TVaR and explain their use and limitations.Sections F and G have a combined weight of 20-25%.F. Construction of Empirical Models1. Estimate failure time and loss distributions using:a) Kaplan-Meier estimatorb) Nelson-Åalen estimatorc) Kernel density estimators2. Estimate the variance of estimators and confidence intervals for failure time and lossdistributions.3. Apply the following concepts in estimating failure time and loss distribution:a) Unbiasednessb) Consistencyc) Mean squared errorG. Estimation of decrement probabilities from large samples1. Estimate decrement probabilities using both parametric and nonparametric approachesfor both individual and interval data2. Approximate the variance of the estimatorsH. Construction and Selection of Parametric Models (25-30%)1. Estimate the parameters of failure time and loss distributions using:a) Maximum likelihoodb) Method of momentsc) Percentile matchingd) Bayesian procedures2. Estimate the parameters of failure time and loss distributions with censored and/ortruncated data using maximum likelihood.3. Estimate the variance of estimators and the confidence intervals for the parameters andfunctions of parameters of failure time and loss distributions.4. Apply the following concepts in estimating failure time and loss distributions:a) Unbiasednessb) Asymptotic unbiasednessc) Consistencyd) Mean squared errore) Uniform minimum variance estimator5. Determine the acceptability of a fitted model and/or compare models using:a) Graphical proceduresb) Kolmogorov-Smirnov testc) Anderson-Darling testd) Chi-square goodness-of-fit teste) Likelihood ratio testf) Schwarz Bayesian CriterionI. Credibility (20-25%)1. Apply limited fluctuation (classical) credibility including criteria for both full and partialcredibility.2. Perform Bayesian analysis using both discrete and continuous models.3. Apply Bühlmann and Bühlmann-Straub models and understand the relationship of theseto the Bayesian model.4. Apply conjugate priors in Bayesian analysis and in particular the Poisson-gamma model.5. Apply empirical Bayesian methods in the nonparametric and semiparametric cases. J. Simulation (5-10%)1. Simulate both discrete and continuous random variables using the inversion method.2. Simulate from discrete mixtures, decrement tables, the (a,b,0) class, and the normal andlognormal distributions using methods designed for those distributions3. Estimate the number of simulations needed to obtain an estimate with a given error anda given degree of confidence.4. Use simulation to determine the p-value for a hypothesis test.5. Use the bootstrap method to estimate the mean squared error of an estimator.6. Apply simulation methods within the context of actuarial models.Reading Selections for learning outcomes A through H and J:Text• Loss Models: From Data to Decisions, (Fourth Edition), 2012, by Klugman, S.A., Panjer,H.H. and Willmot, G.E.Chapter 3Chapter 4Chapter 5Chapter 6Chapter 8Chapter 9, Sections 9.1–9.7 (excluding 9.6.1), Sections 9.8.1–9.8.2Chapter 10Chapter 11Chapter 12Chapter 13Chapter 14, Sections 14.1 – 14.4 and 14.6Chapter 15Chapter 16Chapter 20Reading Options for learning outcome I (Credibility) will be:Option ACandidates may prepare for this exam using either the Third or Fourth Editions.• Loss Models: From Data to Decisions, (Fourth Edition), 2012, by Klugman, S.A., Panjer,H.H., and Willmot, G.E.Chapter 17, Sections 17.2 – 17.7Chapter 18Chapter 19Option B•Foundations of Casualty Actuarial Science (Fourth Edition), 2001, Casualty Actuarial SocietyChapter 8, Section 1 (background only) Sections 2–5•Topics in Credibility by Dean, C.G.Option C• Introduction to Credibility Theory (Fourth Edition), 2010, Herzog, T.N.Chapters 1-3 (background only)Chapters 4–8Chapter 9 (background only)Other ResourcesTables for Exam C/Exam 4Preview of the CBT layout of the Exam C/4 tables in HTMLAll released exam papers since 2000, can be found at:Past Exam Questions and SolutionsExam C/4 Sample Questions and Solutions. These documents will be updated to reflect the October 2013 syllabus changes. The cover page of each document will indicate any updates that have been made.。
2019年-资产负债模型(Asset Liability Model)-PPT精品文档-PPT精选文档

Interpreting Results
Results need to be interpreted and presented to the client
Recommendations may (and usually) do mean a change in strategy Examples of results:
Working at Watson Wyatt – John Ferraro
Joined Watson Wyatt in 1994
Worked in a number of departments: - IT - IT/Administration - Superannuation Consulting - Investment Consulting
Currently provide investment advice to Trustees on strategy, manager selection and monitoring.
三茅招聘管理软件,永久免费, HR都在用。 官方下载网站:hrloo/
University of NSW
23 September 2019 Guest Lecture John Ferraro James Fitzpatrick
Agenda
Asset Liability Modelling (ALM) Working at Watson Wyatt - James Fitzpatrick - John Ferraro
Example 1: Return Definition? Vested Benefits Index
a moden introductio to probability and statistics
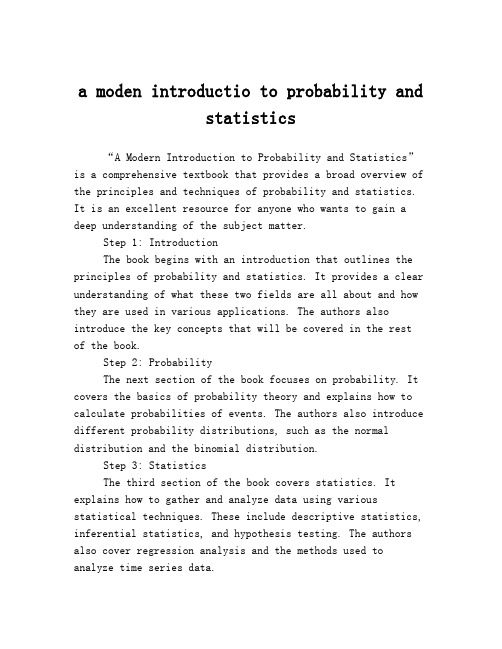
a moden introductio to probability andstatistics“A Modern Introduction to Probability and Statistics” is a comprehensive textbook that provides a broad overview of the principles and techniques of probability and statistics. It is an excellent resource for anyone who wants to gain a deep understanding of the subject matter.Step 1: IntroductionThe book begins with an introduction that outlines the principles of probability and statistics. It provides a clear understanding of what these two fields are all about and how they are used in various applications. The authors also introduce the key concepts that will be covered in the restof the book.Step 2: ProbabilityThe next section of the book focuses on probability. It covers the basics of probability theory and explains how to calculate probabilities of events. The authors also introduce different probability distributions, such as the normal distribution and the binomial distribution.Step 3: StatisticsThe third section of the book covers statistics. It explains how to gather and analyze data using various statistical techniques. These include descriptive statistics, inferential statistics, and hypothesis testing. The authors also cover regression analysis and the methods used to analyze time series data.Step 4: ApplicationsThe final section of the book provides a range of applications of probability and statistics. These include applications in finance, engineering, and the social sciences. The authors use real-world examples to demonstrate how these concepts are applied in practice.Step 5: ExercisesThroughout the book, there are numerous exercises that can be used to test your knowledge and understanding of the concepts covered. These exercises range from simple calculations to more complex applications. There are also detailed solutions provided at the back of the book.Conclusion:“A Modern Introduction to Probability and Statistics”is an excellent textbook that provides a comprehensive overview of the subject matter. The authors use clear and concise language throughout the book, making it accessible to beginners, while also providing in-depth coverage of the principles and techniques involved. The exercises andsolutions provided also make it an excellent resource for anyone who wants to test their knowledge and understanding of the material. Overall, this book is a must-read for anyonewho is serious about learning probability and statistics, and its applications to real-world problems.。
商务统计学-常用词汇整理表

商务统计学词汇表Chapter 1Categorical variables 分类变量Continuous variables 连续变量Data 数据Descriptive statistics 描述统计学Discrete variables 离散变量Focus group焦点小组方法,小组访谈法,焦点讨论方法Inferential statistics 推断统计学Numerical variables 数值变量Operational definition 运算定义Parameter 参数Population 总体Primary sources 原始(数据)来源Sample 抽样、样本secondary sources 二手(数据)来源statistic 统计量statistics 统计学、统计数据statistical packages 统计(软件)包variables 变量Chapter 2Bar chart 柱形图、条形图Cells 单元、元素Chartjunk 图表垃圾Class boundaries 组限Class grouping 分组Class midpoint 组中值Contingency table 列联表Cross-classification table 交叉分类表Cumulative percentage distribution累计百分比分布Cumulative percentage polygon累计百分比多边形图Frequency distribution 频数分布Histogram 直方图Ogive (cumulative percentage polygon)卵形图、累积曲线图Ordered array 有序的数列Pareto diagram 帕累托图Pareto principle 帕累托准则percentage distribution 百分比分布percentage polygon 百分比多边形图pie chart 饼图、圆形图range 全距relative frequency distribution相对频数分布scatter diagram 散点图stem-and-leaf display 茎叶图summary table 汇总表time-series plot 时间数列图width of class interval 组距Chapter 3Arithmetic mean 算术均值Box-and-whisker plot 箱线图Central tendency 集中趋势Chebyshev rule 切比雪夫法则Coefficient of correlation 相关系数Coefficient of variation 变异系数Covariance 协方差Dispersion 离散离差Empirical rule 经验法则Extreme value 极端值Five-number summary 五数(值)概括法Geometric mean 几何平均Interquartile range (IQR)四分间距、内距Left-skewed 左偏Mean 均值Median 中位数Midspread 中间离散、中间离差Mode 众数Outlier 异常值Population mean 总体均值Population standard deviatio总体标准差Population variance 总体方差Q1:first quartile 下四分位数Q3:third quartile 上四分位数Quartile 四分位数Range 全距Resistant measures 抗性测度值Right-skewed 右偏Sample coefficient of correlation样本相关系数Shape (分布)形状Skewed 偏斜(度)Spread 离散(分布)Standard deviation 标准差Sum of squares 平方和Symmetrical 对称的Variance 方差Variation 变异Z scores 标准分数Chapter 4A priori classical probability先验古典概率Bayes’theorem 贝叶斯定理Certain event 必然事件Collectively exhaustive 完备穷尽Combination 组合Complement of event 事件的补A’Conditional probability 条件概率Contingency table 列联表Decision tree 决策树Empirical classical probability经验古典概率Event 事件General addition rule 一般加法法则General multiplication rule一般乘法法则Impossible event 不可能事件Intersection of event 事件的交Joint event 联合事件Joint probability 联合概率Marginal probability 边际概率Multiplication rule for independent events 独立事件的乘法法则Mutually exclusive 互斥Permutation 排列Sample space 样本空间Simple event 简单事件Statistical independence 统计独立性Subjective probability 主观概率Table of cross-classifications交叉分类表Union 联合Venn diagram 韦恩图Chapter 5Area of opportunity 机会域Binomial probability distribution二项概率分布Expected value of a discrete random variable 离散随机变量的期望值Mathematical model 数学模型Poisson distribution 泊松分布Probability distribution for a discrete random variable离散随机变量的概率分布Rule of combination 组合定律Standard deviation of a discrete random variable 离散随机变量的标准差Chapter 6Continuous probability density function连续概率密度函数Cumulative standardized normal distribution 累积标准正态分布Normal distribution 正态分布Normal probability density function正态概率密度函数Normal probability plot 正态概率图Quantile-quantile plotQ-Q图,分位数图Standardized normal random variable标准正态随机变量Transformation formula 转换公式Chapter7Central Limit Theorem 中心极限定理Clusters 群Cluster sampling 整群抽样Convenience sampling 方便抽样、便利抽样Coverage error 涵盖误差Frame 抽样框Judgement sample 判断抽样(样本)Measurememnt error 测量误差Nonprobability sample 非概率抽样Nonreponse error 无回答误差Probability sample 概率抽样Sampling distribution 抽样分布Sampling distribution of the sample mean样本均值的抽样分布Sampling distribution of the sample proportion 样本比例的抽样分布Sampling error 抽样误差Sampling with replacement 重置抽样Sampling without replacement 不重置抽样Selection bias 选择偏差Simple random sample 简单随机抽样Standard error of the mean均值的标准误差nxσσ= Standard error of the proportion比例的标准误差np)1(ππσ-=Strata 层 Stratified sample 分层抽样、分类抽样 Systematic sample 系统抽样 Table of random numbers 随机数表 Unbiased 无偏的 Chapter 8Confidence interval estimat置信区间估计Critical value 临界值 Degrees of freedom 自由度 Level of confidence 置信水平 Point estimate 点估计 Sampling error 抽样误差 Chapter9Level of significance α 显著性水平 Alternative hypothesis 备择假设 Confidence coefficient 置信系数 Confidence level 置信水平 Data snooping 数据探测法 Directional test方向性检验(左或右单侧检验)Hypothesis testing 假设检验 Null hypothesis 原假设 One-tail test 单尾检验、单侧检验 P-value P 值 Power of a statistical test统计检验功效Randomization 随机性、随机选择 Region of nonrejection 非拒绝域 Region of rejection 拒绝域 Test statistic t 检验统计量 Two-tail test 双尾检验、双侧检验 Type Ⅰerror 第一类错误 Type Ⅱerror 第二类错误 Chapter 10 (第五版的10、11章)Among-group variation 组间方差 Analysis of variance (ANOVA)方差分析 Critical range 临界区域 F distribution F 分布 f-test statistic for testing the equality of two variances两个方差相等的F 检验 Factor 因素grand mean X 总平均数 homogeneity of variance 方差齐性 Levene test 方差齐性检验 Matched 匹配 Paired 配对 Mean square 均方 MSA 组间均方差 MST 总均方差 MSW 组内均方差 Multiple comparisons 多重比较 One-way ANOVA 单因素方差分析 Paired t test for the mean difference in related populations有关总体均值之差的配对t 检验Pooled-variance t test 合并方差的t 检验 Post hoc 验后比较 Random error 随机误差 Randomness and independence随机性和独立性Repeated measurements 重复测量 Robust test 稳健性检验 Separate-variance t test分离方差的t 检验Studentized range distribution学生(氏)全距或极差分布Sum of squares among groups (SSA )组间平方和Sum of squares total(SST) 总平方和 Sum of squares within groups (SSW)组内平方和Tatal variation 总方差 Treatment effect 处理效应 Tukey-Kramer multiple comparison procedureTukey-Kramer 多重比较方法 Within-group variation 组内方差 Chapter 11 (第五版的12章)Assumptions of regression 回归假设 Autocorrelation 自相关 Coefficient of determination 决定系数 Confidence interval estimate for the mean response因变量(响应变量)均值的置信区间估计 Confidence interval estimate for the slope 斜率的置信区间估计Correlation coefficient 相关系数Dependent variable 因变量Durbin-Watson statistic D-W统计量Error sum of squares (SSE)随机误差平方和Equal variance 等方差Explained variation 可解释的变差Explanatory 解释变量Homoscedasticity 同方差性,方差齐性Independence of errors随机误差的独立性Least-squares method 最小平方法Linearity 线性Normality 正态性Prediction interval for an individual response Y因变量Y个别值的预测区间Prediction line 预测线Regression line 回归线Regression analysis 回归分析Regression coefficient 回归系数Regression sum of squares(SSR)回归平方和Residual analysis 残差分析Response variable 因变量Scatter diagram 散点图Simple linear regression简单(一元)线性回归Slope 斜率Standard error of the estimate估计标准误差Y intercept 因变量Y的截距Unexplained variation不可解释的方差Chapter 12 (第五版13章)Adjusted r2修正的决定系数Coefficient of multiple determination多重决定系数Cross-product term 交叉乘积项Dummy variables 虚拟变量Interaction term 交叉项Multiple regression models多元回归模型Net regression coefficient净回归系数,偏回归系数Overall F test总体(回归方程)的F检验Quadratic regression model二次回归模型。
车辆控制系统说明书
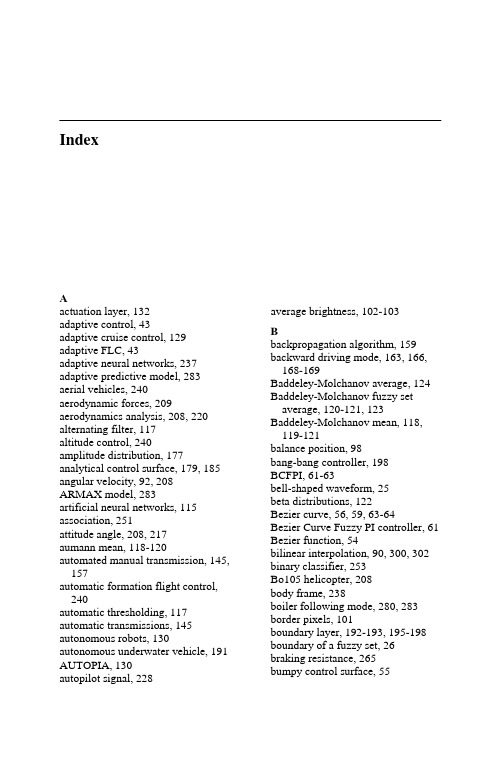
IndexAactuation layer, 132average brightness,102-103adaptive control, 43Badaptive cruise control, 129backpropagation algorithm, 159adaptive FLC, 43backward driving mode,163,166,168-169adaptive neural networks,237adaptive predictive model, 283Baddeley-Molchanov average, 124aerial vehicles, 240 Baddeley-Molchanov fuzzy set average, 120-121, 123aerodynamic forces,209aerodynamics analysis, 208, 220Baddeley-Molchanov mean,118,119-121alternating filter, 117altitude control, 240balance position, 98amplitude distribution, 177bang-bang controller,198analytical control surface, 179, 185BCFPI, 61-63angular velocity, 92,208bell-shaped waveform,25ARMAX model, 283beta distributions,122artificial neural networks,115Bezier curve, 56, 59, 63-64association, 251Bezier Curve Fuzzy PI controller,61attitude angle,208, 217Bezier function, 54aumann mean,118-120bilinear interpolation, 90, 300,302automated manual transmission,145,157binary classifier,253Bo105 helicopter, 208automatic formation flight control,240body frame,238boiler following mode,280,283automatic thresholding,117border pixels, 101automatic transmissions,145boundary layer, 192-193,195-198autonomous robots,130boundary of a fuzzy set,26autonomous underwater vehicle, 191braking resistance, 265AUTOPIA, 130bumpy control surface, 55autopilot signal, 228Index 326CCAE package software, 315, 318 calibration accuracy, 83, 299-300, 309, 310, 312CARIMA models, 290case-based reasoning, 253center of gravity method, 29-30, 32-33centroid defuzzification, 7 centroid defuzzification, 56 centroid Method, 106 characteristic polygon, 57 characterization, 43, 251, 293 chattering, 6, 84, 191-192, 195, 196, 198chromosomes, 59circuit breaker, 270classical control, 1classical set, 19-23, 25-26, 36, 254 classification, 106, 108, 111, 179, 185, 251-253classification model, 253close formation flight, 237close path tracking, 223-224 clustering, 104, 106, 108, 251-253, 255, 289clustering algorithm, 252 clustering function, 104clutch stroke, 147coarse fuzzy logic controller, 94 collective pitch angle, 209 collision avoidance, 166, 168 collision avoidance system, 160, 167, 169-170, 172collision avoidance system, 168 complement, 20, 23, 45 compressor contamination, 289 conditional independence graph, 259 confidence thresholds, 251 confidence-rated rules, 251coning angle, 210constant gain, 207constant pressure mode, 280 contrast intensification, 104 contrast intensificator operator, 104 control derivatives, 211control gain, 35, 72, 93, 96, 244 control gain factor, 93control gains, 53, 226control rules, 18, 27, 28, 35, 53, 64, 65, 90-91, 93, 207, 228, 230, 262, 302, 304-305, 315, 317control surfaces, 53-55, 64, 69, 73, 77, 193controller actuator faulty, 289 control-weighting matrix, 207 convex sets, 119-120Coordinate Measurement Machine, 301coordinate measuring machine, 96 core of a fuzzy set, 26corner cube retroreflector, 85 correlation-minimum, 243-244cost function, 74-75, 213, 282-283, 287coverage function, 118crisp input, 18, 51, 182crisp output, 7, 34, 41-42, 51, 184, 300, 305-306crisp sets, 19, 21, 23crisp variable, 18-19, 29critical clearing time, 270 crossover, 59crossover probability, 59-60cruise control, 129-130,132-135, 137-139cubic cell, 299, 301-302, 309cubic spline, 48cubic spline interpolation, 300 current time gap, 136custom membership function, 294 customer behav or, 249iDdamping factor, 211data cleaning, 250data integration, 250data mining, 249, 250, 251-255, 259-260data selection, 250data transformation, 250d-dimensional Euclidean space, 117, 124decision logic, 321 decomposition, 173, 259Index327defuzzification function, 102, 105, 107-108, 111 defuzzifications, 17-18, 29, 34 defuzzifier, 181, 242density function, 122 dependency analysis, 258 dependency structure, 259 dependent loop level, 279depth control, 202-203depth controller, 202detection point, 169deviation, 79, 85, 185-188, 224, 251, 253, 262, 265, 268, 276, 288 dilation, 117discriminated rules, 251 discrimination, 251, 252distance function, 119-121 distance sensor, 167, 171 distribution function, 259domain knowledge, 254-255 domain-specific attributes, 251 Doppler frequency shift, 87 downhill simplex algorithm, 77, 79 downwash, 209drag reduction, 244driver’s intention estimator, 148 dutch roll, 212dynamic braking, 261-262 dynamic fuzzy system, 286, 304 dynamic tracking trajectory, 98Eedge composition, 108edge detection, 108 eigenvalues, 6-7, 212electrical coupling effect, 85, 88 electrical coupling effects, 87 equilibrium point, 207, 216 equivalent control, 194erosion, 117error rates, 96estimation, 34, 53, 119, 251, 283, 295, 302Euler angles, 208evaluation function, 258 evolution, 45, 133, 208, 251 execution layer, 262-266, 277 expert knowledge, 160, 191, 262 expert segmentation, 121-122 extended sup-star composition, 182 Ffault accommodation, 284fault clearing states, 271, 274fault detection, 288-289, 295fault diagnosis, 284fault durations, 271, 274fault isolation, 284, 288fault point, 270-271, 273-274fault tolerant control, 288fault trajectories, 271feature extraction, 256fiber glass hull, 193fin forces, 210final segmentation, 117final threshold, 116fine fuzzy controller, 90finer lookup table, 34finite element method, 318finite impulse responses, 288firing weights, 229fitness function, 59-60, 257flap angles, 209flight aerodynamic model, 247 flight envelope, 207, 214, 217flight path angle, 210flight trajectory, 208, 223footprint of uncertainty, 176, 179 formation geometry, 238, 247 formation trajectory, 246forward driving mode, 163, 167, 169 forward flight control, 217 forward flight speed, 217forward neural network, 288 forward velocity, 208, 214, 217, 219-220forward velocity tracking, 208 fossil power plants, 284-285, 296 four-dimensional synoptic data, 191 four-generator test system, 269 Fourier filter, 133four-quadrant detector, 79, 87, 92, 96foveal avascular zone, 123fundus images, 115, 121, 124 fuselage, 208-210Index 328fuselage axes, 208-209fuselage incidence, 210fuzz-C, 45fuzzifications, 18, 25fuzzifier, 181-182fuzzy ACC controller, 138fuzzy aggregation operator, 293 fuzzy ASICs, 37-38, 50fuzzy binarization algorithm, 110 fuzzy CC controller, 138fuzzy clustering algorithm, 106, 108 fuzzy constraints, 286, 291-292 fuzzy control surface, 54fuzzy damage-mitigating control, 284fuzzy decomposition, 108fuzzy domain, 102, 106fuzzy edge detection, 111fuzzy error interpolation, 300, 302, 305-306, 309, 313fuzzy filter, 104fuzzy gain scheduler, 217-218 fuzzy gain-scheduler, 207-208, 220 fuzzy geometry, 110-111fuzzy I controller, 76fuzzy image processing, 102, 106, 111, 124fuzzy implication rules, 27-28 fuzzy inference system, 17, 25, 27, 35-36, 207-208, 302, 304-306 fuzzy interpolation, 300, 302, 305- 307, 309, 313fuzzy interpolation method, 309 fuzzy interpolation technique, 300, 309, 313fuzzy interval control, 177fuzzy mapping rules, 27fuzzy model following control system, 84fuzzy modeling methods, 255 fuzzy navigation algorithm, 244 fuzzy operators, 104-105, 111 fuzzy P controller, 71, 73fuzzy PD controller, 69fuzzy perimeter, 110-111fuzzy PI controllers, 61fuzzy PID controllers, 53, 64-65, 80 fuzzy production rules, 315fuzzy reference governor, 285 Fuzzy Robust Controller, 7fuzzy set averages, 116, 124-125 fuzzy sets, 7, 19, 22, 24, 27, 36, 45, 115, 120-121, 124-125, 151, 176-182, 184-188, 192, 228, 262, 265-266fuzzy sliding mode controller, 192, 196-197fuzzy sliding surface, 192fuzzy subsets, 152, 200fuzzy variable boundary layer, 192 fuzzyTECH, 45Ggain margins, 207gain scheduling, 193, 207, 208, 211, 217, 220gas turbines, 279Gaussian membership function, 7 Gaussian waveform, 25 Gaussian-Bell waveforms, 304 gear position decision, 145, 147 gear-operating lever, 147general window function, 105 general-purpose microprocessors, 37-38, 44genetic algorithm, 54, 59, 192, 208, 257-258genetic operators, 59-60genetic-inclined search, 257 geometric modeling, 56gimbal motor, 90, 96global gain-scheduling, 220global linear ARX model, 284 global navigation satellite systems, 141global position system, 224goal seeking behaviour, 186-187 governor valves80, 2HHamiltonian function, 261, 277 hard constraints, 283, 293 heading angle, 226, 228, 230, 239, 240-244, 246heading angle control, 240Index329heading controller, 194, 201-202 heading error rate, 194, 201 heading speed, 226heading velocity control, 240 heat recovery steam generator, 279 hedges, 103-104height method, 29helicopter, 207-212, 214, 217, 220 helicopter control matrix, 211 helicopter flight control, 207 Heneghan method, 116-117, 121-124heuristic search, 258 hierarchical approaches, 261 hierarchical architecture, 185 hierarchical fuzzy processors, 261 high dimensional systems, 191 high stepping rates, 84hit-miss topology, 119home position, 96horizontal tail plane, 209 horizontal tracker, 90hostile, 223human domain experts, 255 human visual system, 101hybrid system framework, 295 hyperbolic tangent function, 195 hyperplane, 192-193, 196 hysteresis thres olding, 116-117hIIF-THEN rule, 27-28image binarization, 106image complexity, 104image fuzzification function, 111 image segmentation, 124image-expert, 122-123indicator function, 121inert, 223inertia frame, 238inference decision methods, 317 inferential conclusion, 317 inferential decision, 317 injection molding process, 315 inner loop controller, 87integral time absolute error, 54 inter-class similarity, 252 internal dependencies, 169 interpolation property, 203 interpolative nature, 262 intersection, 20, 23-24, 31, 180 interval sets, 178interval type-2 FLC, 181interval type-2 fuzzy sets, 177, 180-181, 184inter-vehicle gap, 135intra-class similarity, 252inverse dynamics control, 228, 230 inverse dynamics method, 227 inverse kinema c, 299tiJ - Kjoin, 180Kalman gain, 213kinematic model, 299kinematic modeling, 299-300 knowledge based gear position decision, 148, 153knowledge reasoning layer, 132 knowledge representation, 250 knowledge-bas d GPD model, 146eLlabyrinths, 169laser interferometer transducer, 83 laser tracker, 301laser tracking system, 53, 63, 65, 75, 78-79, 83-85, 87, 98, 301lateral control, 131, 138lateral cyclic pitch angle, 209 lateral flapping angle, 210 leader, 238-239linear control surface, 55linear fuzzy PI, 61linear hover model, 213linear interpolation, 300-301, 306-307, 309, 313linear interpolation method, 309 linear optimal controller, 207, 217 linear P controller, 73linear state feedback controller, 7 linear structures, 117linear switching line, 198linear time-series models, 283 linguistic variables, 18, 25, 27, 90, 102, 175, 208, 258Index 330load shedding, 261load-following capabilities, 288, 297 loading dock, 159-161, 170, 172 longitudinal control, 130-132 longitudinal cyclic pitch angle, 209 longitudinal flapping angle, 210 lookup table, 18, 31-35, 40, 44, 46, 47-48, 51, 65, 70, 74, 93, 300, 302, 304-305lower membership functions, 179-180LQ feedback gains, 208LQ linear controller, 208LQ optimal controller, 208LQ regulator, 208L-R fuzzy numbers, 121 Luenburger observer, 6Lyapunov func on, 5, 192, 284tiMMamdani model, 40, 46 Mamdani’s method, 242 Mamdani-type controller, 208 maneuverability, 164, 207, 209, 288 manual transmissions, 145 mapping function, 102, 104 marginal distribution functions, 259 market-basket analysis, 251-252 massive databases, 249matched filtering, 115 mathematical morphology, 117, 127 mating pool, 59-60max member principle, 106max-dot method, 40-41, 46mean distance function, 119mean max membership, 106mean of maximum method, 29 mean set, 118-121measuring beam, 86mechanical coupling effects, 87 mechanical layer, 132median filter, 105meet, 7, 50, 139, 180, 183, 302 membership degree, 39, 257 membership functions, 18, 25, 81 membership mapping processes, 56 miniature acrobatic helicopter, 208 minor steady state errors, 217 mixed-fuzzy controller, 92mobile robot control, 130, 175, 181 mobile robots, 171, 175-176, 183, 187-189model predictive control, 280, 287 model-based control, 224 modeless compensation, 300 modeless robot calibration, 299-301, 312-313modern combined-cycle power plant, 279modular structure, 172mold-design optimization, 323 mold-design process, 323molded part, 318-321, 323 morphological methods, 115motor angular acceleration, 3 motor plant, 3motor speed control, 2moving average filter, 105 multilayer fuzzy logic control, 276 multimachine power system, 262 multivariable control, 280 multivariable fuzzy PID control, 285 multivariable self-tuning controller, 283, 295mutation, 59mutation probability, 59-60mutual interference, 88Nnavigation control, 160neural fuzzy control, 19, 36neural networks, 173, 237, 255, 280, 284, 323neuro-fuzzy control, 237nominal plant, 2-4nonlinear adaptive control, 237non-linear control, 2, 159 nonlinear mapping, 55nonlinear switching curve, 198-199 nonlinear switching function, 200 nonvolatile memory, 44 normalized universe, 266Oobjective function, 59, 74-75, 77, 107, 281-282, 284, 287, 289-291,Index331295obstacle avoidance, 166, 169, 187-188, 223-225, 227, 231 obstacle avoidance behaviour, 187-188obstacle sensor, 224, 228off-line defuzzification, 34off-line fuzzy inference system, 302, 304off-line fuzzy technology, 300off-line lookup tables, 302 offsprings, 59-60on-line dynamic fuzzy inference system, 302online tuning, 203open water trial, 202operating point, 210optical platform, 92optimal control table, 300optimal feedback gain, 208, 215-216 optimal gains, 207original domain, 102outer loop controller, 85, 87outlier analysis, 251, 253output control gains, 92 overshoot, 3-4, 6-7, 60-61, 75-76, 94, 96, 193, 229, 266Ppath tracking, 223, 232-234 pattern evaluation, 250pattern vector, 150-151PD controller, 4, 54-55, 68-69, 71, 74, 76-77, 79, 134, 163, 165, 202 perception domain, 102 performance index, 60, 207 perturbed plants, 3, 7phase margins, 207phase-plan mapping fuzzy control, 19photovoltaic power systems, 261 phugoid mode, 212PID, 1-4, 8, 13, 19, 53, 61, 64-65, 74, 80, 84-85, 87-90, 92-98, 192 PID-fuzzy control, 19piecewise nonlinear surface, 193 pitch angle, 202, 209, 217pitch controller, 193, 201-202 pitch error, 193, 201pitch error rate, 193, 201pitch subsidence, 212planetary gearbox, 145point-in-time transaction, 252 polarizing beam-splitter, 86 poles, 4, 94, 96position sensor detectors, 84 positive definite matrix, 213post fault, 268, 270post-fault trajectory, 273pre-defined membership functions, 302prediction, 251, 258, 281-283, 287, 290predictive control, 280, 282-287, 290-291, 293-297predictive supervisory controller, 284preview distance control, 129 principal regulation level, 279 probabilistic reasoning approach, 259probability space, 118Problem understanding phases, 254 production rules, 316pursuer car, 136, 138-140 pursuer vehicle, 136, 138, 140Qquadrant detector, 79, 92 quadrant photo detector, 85 quadratic optimal technology, 208 quadrilateral ob tacle, 231sRradial basis function, 284 random closed set, 118random compact set, 118-120 rapid environment assessment, 191 reference beam, 86relative frame, 240relay control, 195release distance, 169residual forces, 217retinal vessel detection, 115, 117 RGB band, 115Riccati equation, 207, 213-214Index 332rise time, 3, 54, 60-61, 75-76road-environment estimator, 148 robot kinematics, 299robot workspace, 299-302, 309 robust control, 2, 84, 280robust controller, 2, 8, 90robust fuzzy controller, 2, 7 robustness property, 5, 203roll subsidence, 212rotor blade flap angle, 209rotor blades, 210rudder, 193, 201rule base size, 191, 199-200rule output function, 191, 193, 198-199, 203Runge-Kutta m thod, 61eSsampling period, 96saturation function, 195, 199 saturation functions, 162scaling factor, 54, 72-73scaling gains, 67, 69S-curve waveform, 25secondary membership function, 178 secondary memberships, 179, 181 selection, 59self-learning neural network, 159 self-organizing fuzzy control, 261 self-tuning adaptive control, 280 self-tuning control, 191semi-positive definite matrix, 213 sensitivity indices, 177sequence-based analysis, 251-252 sequential quadratic programming, 283, 292sets type-reduction, 184setting time, 54, 60-61settling time, 75-76, 94, 96SGA, 59shift points, 152shift schedule algorithms, 148shift schedules, 152, 156shifting control, 145, 147shifting schedules, 146, 152shift-schedule tables, 152sideslip angle, 210sigmoidal waveform, 25 sign function, 195, 199simplex optimal algorithm, 80 single gimbal system, 96single point mass obstacle, 223 singleton fuzzification, 181-182 sinusoidal waveform, 94, 300, 309 sliding function, 192sliding mode control, 1-2, 4, 8, 191, 193, 195-196, 203sliding mode fuzzy controller, 193, 198-200sliding mode fuzzy heading controller, 201sliding pressure control, 280 sliding region, 192, 201sliding surface, 5-6, 192-193, 195-198, 200sliding-mode fuzzy control, 19 soft constraints, 281, 287space-gap, 135special-purpose processors, 48 spectral mapping theorem, 216 speed adaptation, 138speed control, 2, 84, 130-131, 133, 160spiral subsidence, 212sporadic alternations, 257state feedback controller, 213 state transition, 167-169state transition matrix, 216state-weighting matrix, 207static fuzzy logic controller, 43 static MIMO system, 243steady state error, 4, 54, 79, 90, 94, 96, 98, 192steam turbine, 279steam valving, 261step response, 4, 7, 53, 76, 91, 193, 219stern plane, 193, 201sup operation, 183supervisory control, 191, 280, 289 supervisory layer, 262-264, 277 support function, 118support of a fuzzy set, 26sup-star composition, 182-183 surviving solutions, 257Index333swing curves, 271, 274-275 switching band, 198switching curve, 198, 200 switching function, 191, 194, 196-198, 200switching variable, 228system trajector192, 195y,Ttail plane, 210tail rotor, 209-210tail rotor derivation, 210Takagi-Sugeno fuzzy methodology, 287target displacement, 87target time gap, 136t-conorm maximum, 132 thermocouple sensor fault, 289 thickness variable, 319-320three-beam laser tracker, 85three-gimbal system, 96throttle pressure, 134throttle-opening degree, 149 thyristor control, 261time delay, 63, 75, 91, 93-94, 281 time optimal robust control, 203 time-gap, 135-137, 139-140time-gap derivative, 136time-gap error, 136time-invariant fuzzy system, 215t-norm minimum, 132torque converter, 145tracking error, 79, 84-85, 92, 244 tracking gimbals, 87tracking mirror, 85, 87tracking performance, 84-85, 88, 90, 192tracking speed, 75, 79, 83-84, 88, 90, 92, 97, 287trajectory mapping unit, 161, 172 transfer function, 2-5, 61-63 transient response, 92, 193 transient stability, 261, 268, 270, 275-276transient stability control, 268 trapezoidal waveform, 25 triangular fuzzy set, 319triangular waveform, 25 trim, 208, 210-211, 213, 217, 220, 237trimmed points, 210TS fuzzy gain scheduler, 217TS fuzzy model, 207, 290TS fuzzy system, 208, 215, 217, 220 TS gain scheduler, 217TS model, 207, 287TSK model, 40-41, 46TS-type controller, 208tuning function, 70, 72turbine following mode, 280, 283 turn rate, 210turning rate regulation, 208, 214, 217two-DOF mirror gimbals, 87two-layered FLC, 231two-level hierarchy controllers, 275-276two-module fuzzy logic control, 238 type-0 systems, 192type-1 FLC, 176-177, 181-182, 185- 188type-1 fuzzy sets, 177-179, 181, 185, 187type-1 membership functions, 176, 179, 183type-2 FLC, 176-177, 180-183, 185-189type-2 fuzzy set, 176-180type-2 interval consequent sets, 184 type-2 membership function, 176-178type-reduced set, 181, 183-185type-reduction,83-1841UUH-1H helicopter, 208uncertain poles, 94, 96uncertain system, 93-94, 96 uncertain zeros, 94, 96underlying domain, 259union, 20, 23-24, 30, 177, 180unit control level, 279universe of discourse, 19-24, 42, 57, 151, 153, 305unmanned aerial vehicles, 223 unmanned helicopter, 208Index 334unstructured dynamic environments, 177unstructured environments, 175-177, 179, 185, 187, 189upper membership function, 179Vvalve outlet pressure, 280vapor pressure, 280variable structure controller, 194, 204velocity feedback, 87vertical fin, 209vertical tracker, 90vertical tracking gimbal, 91vessel detection, 115, 121-122, 124-125vessel networks, 117vessel segmentation, 115, 120 vessel tracking algorithms, 115 vision-driven robotics, 87Vorob’ev fuzzy set average, 121-123 Vorob'ev mean, 118-120vortex, 237 WWang and Mendel’s algorithm, 257 WARP, 49weak link, 270, 273weighing factor, 305weighting coefficients, 75 weighting function, 213weld line, 315, 318-323western states coordinating council, 269Westinghouse turbine-generator, 283 wind–diesel power systems, 261 Wingman, 237-240, 246wingman aircraft, 238-239 wingman veloc y, 239itY-ZYager operator, 292Zana-Klein membership function, 124Zana-Klein method, 116-117, 121, 123-124zeros, 94, 96µ-law function, 54µ-law tuning method, 54。
(仅供参考)2-医学实验室-测量不确定度的评定与表达

临床实验室测量不确定度评估卫生部临床检验中心张传宝cbzhang@参考文献•CNAS-CL06:2006 化学分析中不确定度的评估指南•CNAS-GL05:2011 测量不确定度要求的实施指南•CNAS-TRL-001:2012 医学实验室-测量不确定度的评定与表达15189认可要求的改变•ISO 15189:2007 5.6.2 使用且可能时,实验室应确定检验结果的不确定度。
应考虑所有重要的不确定度分量。
…… •ISO 15189:2012“测量不确定度”的评定改为强制要求,要求实验室确定每一个定量检验程序的测量不确定度,规定测量不确定度的性能标准并定期评审测量不确定度的评估结果,解释测量结果时应考虑测量不确定度,应在实验室用户要求时为其提供测量不确定度的评估结果。
不确定度的应用例:成年男性ALT的参考区间为(9~50)U/L(WS/T4041-2012 临床常用生化检验项目参考区间第1部分1);三位患者A、B、C的测得值如下所示,判断是否正常:A:40U/LB:45U/LC:48U/L术语测量不确定度measurement uncertainty•简称不确定度(uncertainty)•VIM:2008 根据所用到的信息,表征赋予被测量量值分散性的非负参数。
•GUM:表征合理地赋予被测量之值的分散性,与测量结果相联系的参数。
测量不确定度的A类评定Type A evaluation of measurement uncertainty•简称A 类评定(Type A evaluation)•对在规定测量条件下测得的量值用统计分析的方法进行的测量不确定度分量的评定。
•注:规定测量条件是指重复性测量条件、期间精度度测量条件或复现性测量条件。
测量不确定度的B类评定Type B evaluation of measurement uncertainty•简称B类评定(Type B evaluation)•用不同于测量不确定度A类评定的方法对测量不确定度分量进行的评定。
AISSVOL5VOL1-AICIT
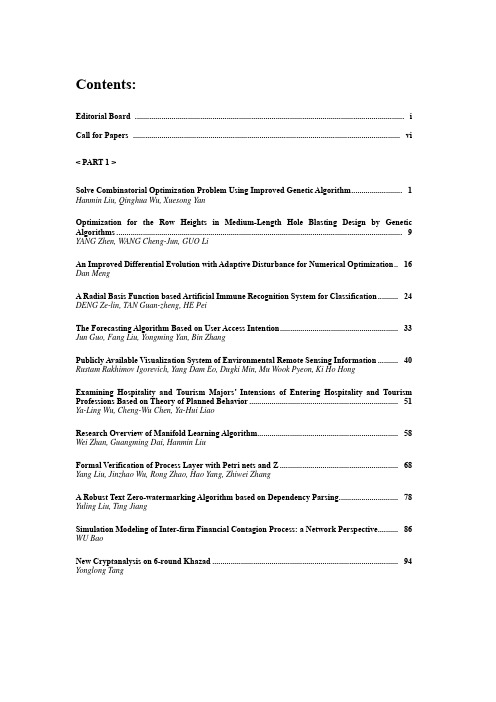
Contents:Editorial Board (i)Call for Papers (vi)< PART 1 >Solve Combinatorial Optimization Problem Using Improved Genetic Algorithm (1)Hanmin Liu, Qinghua Wu, Xuesong YanOptimization for the Row Heights in Medium-Length Hole Blasting Design by Genetic Algorithms (9)YANG Zhen, WANG Cheng-Jun, GUO LiAn Improved Differential Evolution with Adaptive Disturbance for Numerical Optimization .. 16 Dan MengA Radial Basis Function based Artificial Immune Recognition System for Classification (24)DENG Ze-lin, TAN Guan-zheng, HE PeiThe Forecasting Algorithm Based on User Access Intention (33)Jun Guo, Fang Liu, Yongming Yan, Bin ZhangPublicly Available Visualization System of Environmental Remote Sensing Information (40)Rustam Rakhimov Igorevich, Yang Dam Eo, Dugki Min, Mu Wook Pyeon, Ki Ho HongExamining Hospitality and Tourism Majors’ Intensions of Entering Hospitality and Tourism Professions Based on Theory of Planned Behavior (51)Ya-Ling Wu, Cheng-Wu Chen, Ya-Hui LiaoResearch Overview of Manifold Learning Algorithm (58)Wei Zhan, Guangming Dai, Hanmin LiuFormal Verification of Process Layer with Petri nets and Z (68)Yang Liu, Jinzhao Wu, Rong Zhao, Hao Yang, Zhiwei ZhangA Robust Text Zero-watermarking Algorithm based on Dependency Parsing (78)Yuling Liu, Ting JiangSimulation Modeling of Inter-firm Financial Contagion Process: a Network Perspective (86)WU BaoNew Cryptanalysis on 6-round Khazad (94)Yonglong TangA New Risk Assess Model for Urban Rail Transit Projects (104)Zhu Xiangdong, Xiao Xiang, Wu ChaoranAnt Colony Algorithm Optimized by Vaccination (111)He Haitao, Xin NingComputer Network Security and Precaution Evaluation based on Incremental Relevance Vector Machine Algorithm and ACO (120)Guangyuan SongRealization of an Embedded and Automated Performance Testing System for a MEMS Transducer (128)LIAO Hai-yang, XIONG Kui, WEN Zhi-yuMulti-level Cache Prediction and Partitioning Mechanism in CMP (135)Shuo Li, Gaochao Xu, Xiaozhong Geng, Xiaolin Qiao, Feng WuNew Classes of Sequences for Encryption Procedures in Symmetric Cryptography (145)Amparo Fuster-SabaterExplore and Analysis of Environmental Policy Based on Green Industry Development (152)Chunhong Zhu, Zhe Liu, Yue Zhou, Xuehua ZhangResearch on Service Encapsulation of Manufacturing Resources Based on SOOA (158)Lingjun Kong, Wensheng Xu, Nan Li, Jianzhong ChaA Twice Ant Colony Algorithm Based on Simulated Annealing for Solving Multi-constraints QoS Unicast Routing (167)Yongteng Lv, Yongshan Liu, Wei Chen, Xuehui Shang, Yuanyuan Han, Chang LiuA Two-phase Multi-Constraint Web Service Selection Approach for Web Service Composition (176)Zhongjun Liang, Hua Zou, Fangchun Yang, Rongheng LinAdvanced Coupled Map Lattice Model for the Cascading Failure on Urban Street Network . 186 ZHENG Li, SONG Rui, Xiao YunTransition Probability Matrix Based Tourists Flow Prediction (194)Yuting Hu, Rong Xie, Wenjun ZhangA Study on the Macro-Control Policy of China Real Estate Development (202)Lu ShiAn Effective Construction of a Class of Hyper-Bent Functions (212)Yu Lou, Feng Zhou, Chunming TangSpeaker-independent Recognition by Using Mel Frequency Cepstrum Coefficient and Multi-dimensional Space Bionic Pattern Recognition (221)Guanglin Xian, Guangming XianIntelligent Decision Support System (IDSS) for Cooling/Heating Sources Scheme Selection of City Buildings Based on AHP Method (228)Liu Ying, Jiang Kun, Jiang ShaModeling of Underwater Distributed Target Based on FDTD and Its Scale Characteristic Extraction (237)P AN Yu-Cheng, SHAO Jie, ZHAO Wei-Song, ZHONG Ya-QinMethod for Dynamic Multiple Attribute Decision Making under Interval Uncertainty and its Application to Supplier Selection (246)Xu JingStudy on Multi-Agent Information Retrieval Based on Concern Domain (254)Sun JianmingA New Method for Solving Numerical Solution of Fractional Differential Equations (263)Jianping Liu, Xia Li, HuiQuan Ma, XueZhi Mao Guoping ZhenAssessment and Analysis of Hierarchical and Progressive Bilingual English Education Based on Neuro-Fuzzy approach (269)Hao Xin< PART 2 >Computer-based Case Simulations in China: 2001-2011 (277)Tianming Zuo, Peng Wang, Baozhi Sun, Jin Shi, Yang Zhang, Hongran BiHybrid Monte Carlo Sampling Implementation of Bayesian Support Vector Machine (284)Zhou Yatong, Li Jin, Liu LongA Face Recognition Method based on PCA and GEP (291)WANG Xue-guang, CHEN Shu-hongResearch and Application of Higher Vocational College Library Personalized Information Service Based on Cloud Computing (298)Meiying Nie, XinJuan Zhou,Qingzhi WenA Calculation Model for the Rover’s Coverage Boundary on the Lunar Surface Based on Elevation (307)Hu Yasi, Meng Xin, Pan Zhongshi, Li Dalin, Liang Junmin, Yang YiAn Evaluation of firms’ Best Strategies with the ANP, AHP and Sensitivity Test Approaches 316 Catherine W. Kuo, Shun-Chiao ChangDesign of Embedded Vehicle Safety Monitoring System (326)Jing-Lian, Lin-Hui Li, Hu-Han, Ya-Fu Zhou, Feng-Hu, Ze-Quan ZengThe Approach to Obtain the Accurate TOA of VHF Lightning Signals Based on FastICA Algorithm (334)Xuquan Chen, Wenguang ZhaoIntegrating Augmented Reality into Consumer’ Tattoo Try-on Experience (341)Wen-Cheng Wang, Hao-Hsiang Ku, Yen-Wu TiResearch on Location for Emergency Logistics Center Based on Node Cost (348)Wang ShouqiangLogistics Terminal Facility Location Model Based on Customer Value in Competitive Environment (354)Han Shuang Wang XiaoxiaA Decision Support Model for Risk Analysis with Interval-valued Intuitionistic Fuzzy Information (362)Guoqing WuPeak to Average Power Ratio Reduction with Bacterial Foraging Algorithm for OFDM Systems (370)Jing Gao, Jinkuan Wang, Bin WangUsing Linear and Nonlinear Inversion Algorithm Combined with Simple Dislocation Model Inversion of Coal Mine Subsidence Mechanism (379)Yu-Feng ZHU,Qin-Wei WU,Tie-Ding Lu, Yan LuoApplication of Multi- media in Education of Schoolgirl’s Public P. E. in College and University—set Popular Aerobics as Example (388)Yuanchao zhouA Fuzzy Control System for Trailers Driven by Multiple Motors in Side Slipway to Launch and Pull Out Ships (395)Nyan Win Aung, Wei HaijunA Novel Image Encryption Algorithm based on Virtual Optical Imaging and Hyper-chaos .. 403 Wei Zhu , Geng Yang, Lei Chen, Zheng-Yu ChenThe Structure Character of Market Sale Price in the Coordinating Supply Chain (412)Jun Tian, Zhichao WangDesign Parameter Analysis for Inducers (419)Wei Li, Weidong Shi, Zhongyong Pan, Xiaoping Jiang, Ling ZhouAutomatic Recognition of Chinese Traffic Police Gesture Based on Max-Covering Scheme .. 428 Fan Guo, Jin Tang, Zixing CaiDetermination of Acrylamide Contents in Fried Potato Chips Based on Colour Measurement 437 Peng He, Xiao-Qing Wan, Zhen zhou, Cheng-Lin WangAn Efficient Method of Secure Startup and Recovery for Linux (446)Lili Wu, Jingchao Liu,Research on Life Signals Detection Based on Parallel Filter Bank and Higher Order Statistics (454)Jian-Jun LiResearch for Enterprise Logistics Dynamic Optimization Based on the Condition of Production Ability Limited (462)Guo QiangTechnological Progress in Macroeconomic Volatility and Employment Impact analysis - Based on Endogenous Labor RBC Model (469)Wang Qine, Hu honghaiResearch on Bottleneck Identification in Multiple Products Small lot Production Logistics of Manufacturing Enterprise Based on TOC (476)Jian Xu, Hongbo WangThe Spiral Driven and Control Method Research of the Pipe Cleaning Robot (484)Quanyu Yu, Jingyuan Yu, Jun Wang, Jie LiuHoisting Equipment of Coal mine Condition Monitoring and Early Warning Based on BP Neural Network (491)Shu-Fang Zhao, Li-Chao ChenSpatial Temporal Index-based Historic Closing Event Query or Moving Objects (497)Xianbiao Ji, Hong Mi, Zheping ShaoResearch on the Risk-sharing Mechanism of Energy Management Contract Project in Building Sector (510)CaiWeiguang, Ren Hong, Qin BeibeiFinancial Crisis and Financial Index Structure Break (516)Keng-Hsin Lo, Yen-Chang Chen, Yi -Wei ChuangThe Research of Cooling System for the High-Energy Storage Flywheel (522)Wang Wan, He LinNumerical Models and Seismic Design of Steel Frames Equipped with Supplemental Fluid Viscous Devices (528)Marco ValenteStudy on Positive and Dynamic Enterprise Crisis Management based on Sustainable Business Model Innovation (535)Shi-chang Fu, Hui-fen Wang, Dalen ChiangQuantitatively Study on the Mechanism of Cooperating Profit Distribution within Business Ecosystem (544)Bin HuStudy on the Landscape Design of Urban Commemorative Squares Based on Sustainable Development (552)Wenting Wu, Ying Li, Yi Ren< PART 3 >SRPMS: A web-based Project Management System for Scientific Research (559)Yanbao Ji, Xiaopeng Yun, Zhao Jun, Quanjiang Bai, Lingwang GaoEmpirical Study on Influence Factors of Carbon Dioxide Emissions in Liaoning Province based on PLS (567)Yu-xi Jiang, Su-yan He, Xiang-chao WeiHarmony Factor Considered Evaluation of Science Popularization Talents Based on Grey Relational Analysis Model (575)Li MingStudy on the Safety of High-Speed Trains under Crosswind (582)Xian-Liang Sun, Bin-Jie Wang, Ming Gong, San-San Ding, Ai-Qing TianControl Method of Giant Magnetostrictive Precise Actuator Based on the Preisach Hysteresis Theory (589)Yu Zhang, Huifang Liu, Feng SunDynamic Modeling and Characteristics Analysis of Rolls along Axial Direction for Four High Mill Based on Timoshenko Theory (602)Jian-Liang Sun, Yan PengConstruct on Maintenance Requirement Analysis Model of Pavement Management System 610 Xiu-shan Wang, Yun-fang YangAutomatically Generate Test Data Based on Intelligent Algorithms Method (617)Jian Ni, Ning-NingYangAnalysis of the Functions of a High-Speed Railway Station in China (623)Li-Juan Wang, Tian-Wei Zhang, Fan Wang, Qing-Dong ZhouEconometric Analysis of Expectation in Savings-to-Investment in Capital Market Converting Process (630)Wang Yantao , Yu Lihua ,Mao BeibeiAnalysis Model and Empirical Research on Product Innovation Process of Manufacturing Enterprises Based on Entropy-Topsis Method (637)Hang Yin, Bai-Zhou Li, Tao Guo, Jian-Xin ZhuThe Market Analysis and Prediction of Chinese Iron and Steel Industry (645)Li Xiaohan, Sun Qiubai , Li HuaSystem Dynamics Mode Construction and System Simulation in the Product Innovation Process (653)Jian-Xin Zhu, Jun DuResearch on Organization Innovation of Enterprise Based on Complexity Theory (664)Yu Zheng, Tao GuoThe Study on TFP of Iron and Steel Industry inChina Based on DEA-Malmquist Productivity Index Model (672)Xiaodong Dong, Yuzhi ShenResearch and Implementation of Energy Balance Control System in Metallurgy Industry (681)Qiu DongA Study of Opportunities and Threats in the Implementation of International Marketing for Production Design of Corporate Brand Licensing – A Case Study of POP 3D Co., Ltd. (689)Min-Wei Hsu,Tsai-Yun Lo,Liang,K.C.Research on Risk Forming Mechanism and Comprehensive Evaluation of the Enterprise Group (695)Dayong XUMode Construction of Dining Reform in Universities Based on Theory of Institutional Transformation (704)Li PingjinResearch on QR Decomposition and Algorithm of Linguistic Judgment Matrix (711)Lu YuanA Comparison of the Mahalanobis-Taguchi System to A Selective Naïve Bayesian Algorithm for Semiconductor Chemical Vapor Deposition Process (720)Jui-Chin Jiang, Tai-Ying LinStudy of Policy-making Model for Producer Service: Empirical Research in Harbin (730)Xin Xu, Yunlong DingKrein space H∞ filtering for initial alignment of SINS with large azimuth misalignment (738)Jin Feng, Fei Yu, 3Meikui Zou, Heming JiaInternet Word-Of-Mouth on Consumer Online Purchasing Behavior Analysis in China (747)Jie Gao, Weiling YeConvex Relaxation for Array Gain/Phase Calibration in ULAs and UCAs with Unknown Mutual Coupling (758)Shu CaiFinancial-Industrial Integration Risk Management Model of Listed Companies Base on Logistic (767)Ke WenBehavior Equilibrium Analysis for The Cross-Organizational Business Process Reengineering in Supply Chain (775)Jianfeng Li, Yan ChenA Secure Scheme with Precoding Approach in Wireless Sensor Networks (782)Bin Wang, Xiao Wang, Wangmei GuoA New Method on Fault Line Detection for Distribution Network (789)Bo LiManagers’ Power and Earnings Manipulating Preference (796)J. Sun, X. F. Ju, Y. M. Peng, Y. ChangStudy and Application of the Consistency of Distributed Heterogeneous Database Based on Mobile Agent (804)Zhongchun Fang, Hairong Li, Xuyan Tu。
计算保险索赔的概率英语

计算保险索赔的概率英语全文共四篇示例,供读者参考第一篇示例:Calculating the Probability of Insurance ClaimsInsurance is a crucial financial tool that helps individuals and businesses mitigate risks and protect their assets against potential losses. When an insured event occurs, policyholders may file an insurance claim to seek compensation from their insurance provider. In order to assess the financial impact of insurance claims and determine appropriate premium rates, insurance companies rely on calculating the probability of claims.The probability of an insurance claim can be defined as the likelihood that a specific insured event will occur during the policy period. By analyzing historical data and statistical models, insurance companies can estimate the probability of various types of claims and use this information to price their insurance policies accurately. In this article, we will explore the key factors that influence the probability of insurance claims and the methods used to calculate this probability.Factors Influencing the Probability of Insurance ClaimsSeveral factors can influence the probability of insurance claims, including:1. Type of Insurance Coverage: The type of insurance coverage will have a significant impact on the probability of claims. For example, health insurance policies may have a higher probability of claims than property insurance policies, as healthcare costs are more frequent and unpredictable.2. Policyholder Demographics: The demographics of policyholders, such as age, gender, and occupation, can also affect the probability of claims. For instance, young drivers may have a higher probability of auto insurance claims due to their lack of driving experience.3. Geographic Location: The location of insured properties or vehicles can impact the probability of claims. Areas prone to natural disasters or high crime rates may experience more frequent insurance claims compared to low-risk locations.4. Policy Limits and Deductibles: The coverage limits and deductibles set in the insurance policy can influence the probability of claims. Policies with higher limits and lower deductibles may result in more frequent claims, while policieswith lower limits and higher deductibles may lead to fewer claims.5. Claim History: The insured's previous claim history can also impact the probability of future claims. Policyholders with a history of filing frequent claims may be considered higher risk by insurance companies.Methods for Calculating the Probability of Insurance ClaimsInsurance companies use various mathematical and statistical methods to calculate the probability of insurance claims, including:1. Historical Data Analysis: Insurance companies analyze historical data on claims frequency and severity to estimate the probability of future claims. By examining patterns and trends in past claims data, insurers can make informed predictions about the likelihood of future claims.2. Actuarial Models: Actuarial science is the discipline of using mathematical and statistical methods to assess risk and uncertainty in the insurance industry. Actuaries develop models that take into account various risk factors to calculate the probability of claims and determine appropriate premium rates.3. Risk Assessment Tools: Insurance companies may use risk assessment tools and algorithms to evaluate the likelihood of insurance claims. These tools examine a range of risk factors, such as credit scores, driving records, and property values, to calculate the probability of claims for individual policyholders.4. Machine Learning Algorithms: With advancements in technology, insurance companies are increasingly using machine learning algorithms to analyze vast amounts of data and predict the probability of insurance claims. These algorithms can identify complex patterns and correlations in data to make more accurate predictions.ConclusionCalculating the probability of insurance claims is a critical function for insurance companies to manage risk and set appropriate premium rates. By analyzing factors such as the type of coverage, policyholder demographics, claim history, and geographic location, insurers can estimate the likelihood of future claims and make data-driven decisions. Using mathematical and statistical methods, including historical data analysis, actuarial models, risk assessment tools, and machine learning algorithms, insurance companies can optimize theirunderwriting processes and provide financial protection to policyholders effectively.第二篇示例:Introduction第三篇示例:Calculating the Probability of Insurance ClaimsInsurance claims are a vital component of the insurance industry. They represent the financial costs that insurers incur when policyholders experience an insured event. The ability to accurately assess the probability of insurance claims is critical for insurance companies to properly manage their risks and set appropriate premiums. In this article, we will explore the methods used by insurers to calculate the probability of insurance claims.There are several factors that insurers consider when calculating the probability of insurance claims. These factors can be broadly categorized into two main groups: external factors and policyholder-specific factors. External factors include things like geographical location, weather patterns, and economicconditions, while policyholder-specific factors include age, gender, health status, and past insurance claims history.To calculate the probability of insurance claims, insurers use a variety of statistical models and tools. One common method is to use historical data to estimate the likelihood of a claim occurring. This can involve analyzing past claims data to identify patterns and trends that can help predict future claims. Insurers also use actuarial tables, which provide statistical data on the likelihood of different events happening, such as accidents or illnesses.Another important tool that insurers use to calculate the probability of insurance claims is risk assessment. This involves evaluating the risk factors associated with an insured event and determining the likelihood of that event occurring. Insurers may conduct risk assessments using various methods, such as probability theory, statistical analysis, or expert judgment.In addition to statistical models and risk assessment, insurers also use computer simulations and predictive analytics to estimate the probability of insurance claims. These tools allow insurers to simulate different scenarios and predict the likelihood of a claim occurring under various conditions. By using these advanced techniques, insurers can better understand their risksand make more informed decisions about pricing and underwriting policies.It's important to note that the probability of insurance claims is not an exact science. There are inherent uncertainties and variables that can affect the accuracy of these calculations. Insurers must continually monitor and update their models to account for changing conditions and emerging risks.In conclusion, calculating the probability of insurance claims is a complex and multifaceted process that requires the use of various statistical tools and techniques. By accurately assessing the likelihood of claims, insurers can better manage their risks and ensure the financial stability of their businesses. As the insurance industry continues to evolve, the ability to calculate the probability of insurance claims will remain a critical skill for insurers to master.第四篇示例:In the field of insurance, calculating the probability of a claim is a crucial aspect for both insurance companies and policyholders. By determining the likelihood of an event occurring and resulting in a claim, insurers can set premiums accurately and manage risks effectively. In this article, we willexplore how insurance companies calculate the probability of a claim and the factors that influence this calculation.Insurance companies use actuarial science to analyze data and assess risk. Actuaries collect and analyze historical data on similar events to determine the probability of a claim occurring in the future. They take into account various factors such as the insured individual's age, health status, occupation, lifestyle, and geographical location. By using mathematical models and statistics, actuaries can estimate the likelihood of a claim and set premiums accordingly.One of the key factors in calculating the probability of a claim is the loss ratio. The loss ratio is the ratio of the total amount of claims paid out by an insurance company to the total premiums it receives. A high loss ratio indicates that the insurance company is paying out more in claims than it is receiving in premiums, which can lead to financial difficulties. By monitoring the loss ratio and adjusting premiums accordingly, insurers can ensure their financial stability and profitability.Another factor that influences the probability of a claim is the type of insurance coverage. For example, life insurance policies have different risk factors compared to health insurance or property insurance policies. Insurers take into account thespecific risks associated with each type of insurance coverage when calculating the probability of a claim. They also consider external factors such as changes in the economy, regulatory changes, and natural disasters that could impact the likelihood of a claim.In addition to analyzing data and assessing risk factors, insurers also use predictive modeling to calculate the probability of a claim. Predictive modeling involves using algorithms and machine learning techniques to analyze large data sets and predict future outcomes. By using predictive modeling, insurers can identify trends and patterns in claims data and make more accurate predictions about the likelihood of a claim.It is important for insurance companies to continuously monitor and evaluate the probability of a claim to ensure they are adequately pricing their policies and managing risks effectively. By staying up-to-date with the latest data and trends, insurers can make informed decisions about setting premiums, underwriting policies, and managing their overall risk exposure.In conclusion, calculating the probability of a claim is a complex process that involves analyzing data, assessing risk factors, and using predictive modeling techniques. By accurately estimating the likelihood of a claim, insurance companies can setpremiums appropriately, manage risks effectively, and ensure their financial stability and profitability. By understanding the factors that influence the probability of a claim, both insurance companies and policyholders can make informed decisions about their insurance coverage.。
- 1、下载文档前请自行甄别文档内容的完整性,平台不提供额外的编辑、内容补充、找答案等附加服务。
- 2、"仅部分预览"的文档,不可在线预览部分如存在完整性等问题,可反馈申请退款(可完整预览的文档不适用该条件!)。
- 3、如文档侵犯您的权益,请联系客服反馈,我们会尽快为您处理(人工客服工作时间:9:00-18:30)。
A Model of Coverage Probability under ShadowFadingKenneth L.Clarkson John D.HobbyAugust25,2003AbstractWe give a simple analytic model of coverage probability for CDMA cel-lular phone systems under lognormally distributed shadow fading.Prioranalyses have generally considered the coverage probability of a singleantenna;here we consider the probability of coverage by an ensembleof antennas,using some independence assumptions,but also modeling alimited form of dependency among the antenna fades.We use the Fenton-Wilkinson approach of approximating the external interference I0as log-normally distributed.We show that our model gives a coverage probabilitythat is generally within a few percent of Monte Carlo estimates,over awide regime of antenna strengths and other relevant parameters.1IntroductionIn modeling a spread-spectrum cellular phone system,we are interested in the conditions under which the quality of the radio link between the mobile(phone) and the base station antenna is adequate.An important measure of that quality is the E c/I0of the pilot signal,since important decisions in starting a call are based on it.Here E c/I0for a given mobile m and antenna a is the ratio of the signal strength E c received by m from a to the interference I0received by m from all other sources;such interference is due to external noise,and to the power received from all antennas.(As measured,the interference includes all the power received from a itself,but this only approximates the fact that some power received from a is interference for this mobile.)If E c/I0is too low,then the call may not be carried by a,or only carried with poor quality.If the E c/I0from a at a particular location is above a quality threshold,then we say that the location is“covered”by a,and in a given cellular market,it is important to know what the probability that locations are covered.The situation is complicated by the phenomenon of fading,where motion of the mobile results in variation of the received signal strength.We will ignore here fast fading,the rapid variation due to constructive and destructive interference of signals arriving via different paths to the phone,and concentrate on shadow1fading,a slower variation due to obstructions.It is common to model shadow fading as a lognormally distributed random variable[Gud91].Such a model would imply that at a given location,we are interested inthe ratio of a lognormally distributed random variable E c to an interference term I0that is the sum of such random variables,together with some noise. The coverage probability is the probability that such a ratio is above a given threshold.In particular,we are interested in the probability that there is some antenna above threshold,which provides a certain“gain”:if fading increasesthe E c of some antenna,that not only reduces the chance that other antennasare above threshold,by increasing I0,but it also,of course,increases the chance that the given antenna is above threshold.We will derive an expression for coverage probability that conservatively accounts for such gain.Our analysis reduces the ensemble-coverage problem to the problem of es-timating the probability that a given antenna is above threshold.Here thereis a substantial related literature,mostly concerned with approximating the probability distribution of I0,the sum of lognormally-distributed random vari-ables.See,for example,the paper of Abu-Dayya and Beaulieu for references inthe wireless literature[ADB94],the paper of Datey,Gauthier and Simonato for references in the computationalfinance literature[DGS03],and the paper of Ras-musson for further references and an application in network design.[Ras02]The techniques applied to this problem include cumulant matching[JR82,Sch77],ap-proximation using the Inverse Gamma distribution[MS98],upper bounds[Sli01], and characteristic function or moment-generating function techniques[ATB01, Zha99].(Note that the lognormal distribution,alone,has no moment-generating function,so the latter techniques are applied to fading models where the log-normal is compounded with some other distribution.)Here we will use the approximation due to Fenton[Fen60]and to Wilkinson[SY82], where the sum is approximated as a single lognormal distribution,whose pa-rameters are such that its mean and variance match those of the original sum.We compare our overall coverage probability estimate to the results of Monte Carlo experiments.By exploring the space of relevant parameters for such a comparison,we show that our estimate is generally accurate within a few percent absolute error.Therefore,a Monte Carlo coverage probability estimate can be replaced with our analytic expression.This has the advantage of a very large speedup in time needed for evaluation,and also that the resulting function ofthe parameters is much smoother than a Monte Carlo estimate would be.2The modelFirst,we will define some notation,and give some simplifying assumptions.We have signals E j from antenna j to a location,for j=1...m,and additional external interference termη.We will use the following assumptions:1.The values ln E j are normally distributed with meanµj;2.The values ln E j all have the same varianceσ2;23.The random variables E j and ηare all independent.4.We can regard the value ln ηas normally distributed,with mean µηand variance ση;As noted above,assumption (1)is common in the literature.It is based on experimental evidence,and is suggested by the Central Limit Theorem,as ap-plied to the sequence of semi-independent obstructions and terrain variations between the location and the antenna.Assumptions (2)and (3)are due to ignorance:there may be some correla-tions among the signals,and each signal will have a different variance,but often we will not have such data.Assumption (4)is non-physical,but simply reflects per-location,“correlated”fading:it is equivalent to such fading since we are interested in E c /I 0ratios E k /(η+j E j ).Such correlations are treated with greater generality by some authors,using a general covariance matrix A .Note,however,that a model often tested is one where the off-diagonal entries of A have a single common value,and the diagonal entries of A have another common value.(For example,the distributions tested by Abu-Dayya and Beaulieu all have this property[ADB94])Our model satisfies those conditions.The means µj are due to the path loss from the antenna to the location,and also the antenna pattern and the antenna power level.3Estimating the coverage probabilityWe are interested in the probability that a location is uncovered,so thatIE k>t k for all k,where I ≡η+j E j .(We write I 0as just I here.)To simplify the discussion we will assume that all t k =t for some t ,but it is easy to remove this assumption.The desired probability is equal tokProb I >tE k |I ≥t max j<kE j .(1)LetI k ≡η+j>kE j .The conditions for given k imply that tI =t j<kE j +tE k +tI k ≤(k −1)I +tE k +tI k ,so thatI ≤tt −k +1(E k +I k ).3We will use the estimateProbI>tE k|I≥t maxj<kE j≤Probt(E k+I k)>tE k|I≥t maxj<kE j≈Probtt−k+1(E k+I k)>tE k=ProbI kE k>t−k.Here we have approximated in two ways:the upper bound on non-coverage in one step,and the more questionable approximation in the next step,where we assume the condition I≥t max j<k E j does not affect our revised condi-tion too much.We will use Monte Carlo simulation to check our severe these approximations were.It seems to be better,based on our Monte Carlo experiments,as discussed below,to use t−t d k in place of t−k in the above,where the best value of t d, found experimentally,is0.4.We can estimate the probabilities Prob{I k/E k>t−t d k},under the as-sumption that each I k is lognormal.Letˆµk andˆσ2k denote the mean and varianceof ln I k;these values can be readily determined.[ADB94]The mean of ln(I kE k)is thenˆµk−µk,while the variance of ln(I kE k )isˆσ2k+σ2,since I k and E k areindependent.We use these quantities,and the error function,to estimate the coverage probability.3.1HandlingσηThis method of estimating the coverage probability heuristically and experi-mentally accurate whenση=0.It is not accurate whenσηis large,but it can be extended forση=0by using numerical integration:take a weighted combi-nation of probability estimates for trial valuesµtηand trial assumptionση=0, for values ofµtη=µη−mση/2,...µη+mση/2,where m is ten or so.Plainly this integration can be refined and extended to be as accurate as desired,up to the accuracy of the underlying estimates.4Experimental resultsWhile the derivation of the coverage probability estimate was rigorous“most of the time,”it used several approximations,beyond the assumptions mentioned in Section2.We can,however,check its accuracy by means of comparison to Monte Carlo computations.Here we do many such computations,over a broad range of values of the relevant parameters:µj,σ,µη,and the threshold t.Note that,for the purpose of checking the usability of our estimate,that these are the relevant input values.In all the experiments the noise variation4ση0dBµη-1dBM1000t2,7dBt d0.4σ3,5,7dB∆µ00to12step1dB∆µ10to12step1dB∆µ20,3,6,9dB∆µ30,3,6,9dB∆µ40,5,10dB∆µ50,5,10dB∆µ60,8,16dB∆µ70,8,16dBFigure1:Range of experimental parameters,Study1ση=0because,as noted in S3.1,a non-zeroσηcan be handled using a single numerical integration.Ourfirst results show the range of errors in using our estimate.In Figure2, we show a histogram of the differences between Monte Carlo calculations and our estimates,for all the combinations of values shown in Table1.Here for given values of the∆µi,we haveµi set toµi−1−∆µi,for i>0. We also restrict the evaluations to values ofµi that are not too small:if some µj is less than20dB belowµ0,we only considerµj =µj for j ≥j.In Figure3,we show the range of probabilities associated with the combi-nations of values in Table1.We want to make sure that we are not considering combinations of conditions for which the coverage probability is“easily”zero or one,and indeed,while the probabilities are skewed a bit toward the high end, a broad range of probabilities is found.Table4shows the combinations of conditions for a second round of com-parisons.Here we are trying to more closely monitor the effect of variations in antennas that are closer together in power levels.The histograms in Figures5 and6show the general pattern of results.In Table7are the combinations of conditions for a set of experiments in-tended to helpfind the best value of t d,the amount by which the threshold is reduced in the uncoverage calculation,as discussed in§3.Figure8shows the distribution of errors for different values of t d,and shows that t d=0.4seems, by a narrow margin,to be the best.The conditions explored in experiment4are the same as for experiment1, but onlyσ=0.5is considered.Here the errors are typically larger,and the limits of the applicability of our estimates may be visible.The parameters considered are shown in FigureTable9,the errors in Figure10,and the range of probabilities in Figure11.52468Absolute Error,PercentPercent occurrenceFigure 2:Error of our analytic estimate vs.Monte Carlo,Study10.511.52Monte Carlo Probability,PercentPercent occurrenceFigure 3:Distribution of Monte Carlo Probabilities,Study 16ση0dB µη-1dB M 1000t 2,7dB t d0.4σ2,4dB ∆µ00to 4step 1dB ∆µ10to 4step 1dB ∆µ20to 4step 1dB ∆µ30to 4step 1dB ∆µ40to 4step 1dB ∆µ50to 4step1dB ∆µ60dB ∆µ7dBFigure 4:Range of experimental parameters,Study 20510152025Absolute Error,PercentPercent occurrence Figure 5:Error of our analytic estimate vs.Monte Carlo,Study 2751015Monte Carlo Probability,PercentPercent occurrenceFigure 6:Distribution of Monte Carlo Probabilities,Study 2ση0dB µη-1dB M 1000t 12dB t d 0.2to 1.2step 0.2σ4dB ∆µ00to 12step 1dB ∆µ10to 12step 1dB ∆µ20,3,6,9dB ∆µ30,3,6,9dB ∆µ40,5,10dB ∆µ50,5,10dB ∆µ60,8,16dB ∆µ70,8,16dBFigure 7:Range of experimental parameters,Study 380.20.40.60.811.2−20246810t dAbsolute Error,PercentFigure 8:Distribution of probability errors vs.t d ,Study 3ση0dB µη-1dB M 1000t 2,7dB t d 0.4σ0.5dB ∆µ00to 12step 1dB ∆µ10to 12step 1dB ∆µ20,3,6,9dB ∆µ30,3,6,9dB ∆µ40,5,10dB ∆µ50,5,10dB ∆µ60,8,16dB ∆µ70,8,16dBFigure 9:Range of experimental parameters,Study 4920406080Absolute Error,PercentPercent occurrenceFigure 10:Error of our analytic estimate vs.Monte Carlo,Study41020304050Monte Carlo Probability,PercentPercent occurrenceFigure 11:Distribution of Monte Carlo Probabilities,Study 410References[ADB94]A.A.Abu-Dayya and N.C.Beaulieu.Outage probabilities in the presence of correlated lognormal interferers.IEEE Trans.VehicularTechnology,43(1),February1994.[ATB01] A.Annamalai,C.Tellambura,and V.K.Bhargava.Simple and ac-curate methods for outage analysis in cellular mobile radio systems–a unified approach.IEEE munications,49(2):303–316,2001.[DGS03]J.-Y.Datey,G.Gauthier,and J.-G.Simonato.The performance of analytical approximations for the computation of asian quanto-basketoption prices.Multinational Finance Journal,7(1),2003.[Fen60]L.Fenton.The sum of lognormal probability distributions in scat-ter transmission systems.IEEE Trans.on Comm.Sys.,CS-8:57–67,March1960.[Gud91]M.Gudmundson.Correlation model for shadow fading in mobile radio systems.Electronics Letters,27(23):387–421,1991.[JR82]R.Jarrow and A.Rudd.Approximate option valuation for arbitrary stochastic processes.J.of Financial Economics,10:347–369,1982. [MS98]evsky and n options,the sum of lognormals and the reciprocal gamma distribution.J.Financial and QuantitativeAnal.,33:409–422,1998.[Ras02]Lars Rasmusson.Evaluating the cdf for m weighted sums of n corre-lated lognormal random variables.In Proc.of the8th Int.Conf.onComputing in Economics and Finance,June2002.[Sch77] D.Scheher.Generalized gram-charlier series with application to the sum of lognormal variates.IEEE rm.Theory,pages275–280,March1977.[Sli01]S.Ben Slimane.Bounds on the distribution of a sum of indepen-dent lognormal random variables.IEEE munications,49(6):975–978,2001.[SY82]S.C.Schwartz and Y.S.Yeh.On the distribution function and mo-ments of power sums with lognormal components.Bell Syst.Tech.J,61(7),Sept.1982.[Zha99]Q.T.Zhang.Co-channel inference analysis for mobile radio suf-fering lognormal shadowed nakagami fading.IEEE Proceedings-Communications,pages49–54,1999.11。