Distributed Metadata Management Scheme in Cloud Computing
数据库中英语单词

national 全国性的'næʃən! 'næʃənəlcomputer 电脑,电子计算机kəm'pjutɚkəm'pju:tərank 等级;地位ræŋk ræŋkexamination 检查,调查考试ɪg,zæmə'neʃən i g,zæmi'neiʃən information 资讯信息,ɪnfɚ'meʃən ,infə'meiʃəndata 资料,数据'detə'deitədatabase 资料库,数据库'detə,bes 'deitə,beis DB conceptual 概念上的kən'sɛptʃuəl kən'septjuəl entity 实体'ɛntətɪ'entiti 特Arelationship 关系,关联rɪ'leʃən'ʃɪp ri'leiʃənʃip attribute 属性;特性ə'trɪbjut ə'tribju:t b益Udomain 领域, 范围do'men də'mein 逃欧Akey 键;码ki ki:abstract 抽象的'æbstrækt 'æbstrækttype 集;型taɪp taipvalue 数值'vælju 'vælju:] 欧Uschema 模式'skimə'ski:mə哥instance 实例'ɪnstəns 'instəns 的external 外面的,外部的ɪk'stɝnəl eks'tə:nl 的internal 内的,内部的ɪn'tɝn! in'tə:nəlmapping 映象'mæpɪŋ'mæpiŋcomponent 子件/组件kəm'ponənt kəm'pəunəntgrid 网格grɪd grid 谷engineering 工程化,ɛndʒə'nɪrɪŋ,endʒi'niəriŋtrust 信任,信赖trʌst trʌstbenchmark 标准检查程序'bɛntʃ,mɑrk 'bentʃ,mɑ:k process 过程,进程; 处理'prɑsɛs 'prəusestopic 题目;话题'tɑpɪk 'tɔpikidentifier 标识符aɪ'dɛntə,faɪɚai'denti'faiəindependent 独立的,ɪndɪ'pɛndənt ,indi'pendənt dependent 依靠的;依赖的dɪ'pɛndənt di'pendənt] integer 整数'ɪntədʒɚ'intidʒəintegrity 完整,完全ɪn'tɛgrətɪin'tegriticonstraint 约束;限制kən'strent kən'streint 句index 索引'ɪndɛks 'indeksbucket 桶'bʌkɪt 'bʌkit 啊assertion 断语; 断定ə'sɝʃən ə'sə:ʃənprivilege 权限'prɪv!ɪdʒprivilidʒ柚河愕勒传grant 给予,授予grænt grɑ:nt 谷revoke 撤回,撤销rɪ'vok ri'vəukrole 角色rol rəulconnector 连接件kə'nɛktɚkə'nektəpreliminary 概要prɪ'lɪmə,nɛrɪpri'liminəridetail 细节'ditel 'di:teilcommit 委托kə'mɪt kə'mitrollback 回滚'rol,bæk 'rəulbækcursor 游标'kə:sə'kɝsɚfetch 获取fɛtʃfetʃ去icon 图标'aɪkɑn 'aikɔnmenu 菜单'mɛnju 'menju:pointing 指点;指向'pɔɪntɪŋ'pɔintiŋnumeric 数字的nju'mɛrɪk nju:'merikdecimal 十进位的;小数的'dɛsɪm! 'desiməlfloat 浮点数flot fləut 勒欧real 现实的,实际的'riəl 'ri:əlchar 定长字符tʃɑr tʃɑ:text 文本tɛkst t ekstbinary 二元的;二进制'baɪnərɪ'bainəriimage 影像图象'ɪmɪdʒ'imidʒmoney 货币'mʌnɪ'mʌnicreate 创建krɪ'et kri'eit A特default 默认的dɪ'fɔlt di'fɔ:ltnull 零空nʌl nʌl 愕unique 唯一的ju'nik ju:'ni:kcheck 查对,检查tʃɛk tʃekreference 提及;涉及'rɛfərəns 'refərəns 冷customer 顾客'kʌstəmɚ 'kʌstəmə啊identity 身份;本身aɪ'dɛntətɪ ai'dentitiaddress 住址,地址ə'drɛs ə'drespostcode 邮政编码'post,kod 'pəust,kəuddrop 删除drɑp drɔp 柚alter 改变;修改'ɔltɚ 'ɔ:ltə哦欧column 列'kɑləm 'kɔləmadd 添加;增加æd ædselect 选择sə'lɛkt si'lektwhere 当hwɛr hwɛəhaving 所有'hævɪŋ'hæviŋ益ascend 上升ə'sɛnd ə'senddescend 下降dɪ'sɛnd di'senddistinct 独特的dɪ'stɪŋkt di'stiŋkt D 丁count 总计kaunt kauntsum 总和sʌm sʌm 啊average 平均数'ævərɪdʒ'ævəridʒAVGmaximum 最大数'mæksəməm 'mæksiməm 帽目max minimum 最小量'mɪnəməm 'miniməm Min quantity 数量'kwɑntətɪ 'kwɔntitiamount 总数;总额ə'maunt ə'mauntinner 内部的,里面的'ɪnɚ'inəjoin 连结dʒɔɪn dʒɔinouter 在外的,外面的'autɚ'autəunion 结合'junjən 'ju:njən U呢益percent 百分率pɚ'sɛnt pə'sentcase kes keisinsert 插入ɪn'sɝt in'sə:tupdate 更新ʌp'det ʌp'deit 啊delete 删除dɪ'lit di'li:t 梨exist 存在ɪg'zɪst ig'zisttrue 真的tru tru:false 假的fɔls fɔ:lsclustered 聚集索引'klʌstɚd 'klʌstədoption 选项; 选择'ɑpʃən 'ɔpʃəntransaction 事务træn'zækʃən træn'zækʃən 树print 印刷prɪnt p rintinsufficient 资金不足,ɪnsə'fɪʃənt ,insə'fiʃəntfund 资金fʌnd fʌnd 啊atomicity 原子数consistency 一致性原则kən'sɪstənsɪkən'sistənsi C C isolation 隔离,aɪs!'eʃən ,aisə'leiʃəndurability 持久性,djurə'bɪlətɪ,djurə'bilititemp 临时'tɛmp 'tempsemaphore 信号量'sɛməfor 'seməfɔ: 哦愕lock 锁lɑk lɔklocking 封锁exclusive 排外的iks'klu:siv ɪk'sklusɪv 孤路Cunlock 开…的锁ʌn'lɑk 'ʌn'lɔkdisplay 显示dɪ'sple di'spleigranularity 锁粒度extents 区undo 撤消ʌn'du 'ʌn'du: 啊redo 重做ri'du ri:'du:logging 日志文件'lɔgɪŋ'lɔgiŋ益agent 代理人'edʒənt 'eidʒəntdistributed 分布式的dɪ'strɪbjutɪd dis'tribju:tid 句BU coordinator 协调者ko'ɔrdn,etɚkəu'ɔ:dineitə扣呢A search 检索sɝtʃsə:tʃWindows 窗口操作系统'wɪndoz 'windəuzprofessional 专家prə'fɛʃən! p rə'feʃənəlmaster 雇主'mæstɚ'mɑ:stəpage 页pedʒpeidʒfilename 文件名declare 声明dɪ'klɛr di'klɛə Dimport 输入;引用ɪm'port im'pɔ:t 哦愕export 输出ɪks'port eks'pɔ:t 抱wizard 向导'wɪzɚd 'wizədaccess 访问;存取'æksɛs 'æksesprocedure 存储过程prə'sidʒɚ prə'si:dʒəC execute 执行'ɛksɪ,kjut 'eksikju:t Cきゅfunction 函数'fʌŋkʃən 'fʌŋkʃən 啊trigger 触发器'trɪgɚ'trigəencryption 加密instead of 前触发ɪn'stɛdəv in'stedəv 益inserted 插入的ɪn'sɝtɪd in'sə:tidadministrator 管理人əd'mɪnə,stretɚəd'ministreitəlogin 注册,登录lɑg'ɪn lɔg'inpasswd 更改密码password 口令;暗语'pæs,wɝd 'pɑ:swə:dgroup 组grup gru:pdeny 否定dɪ'naɪdi'naisysadmin 系统管理员security 安全性sɪ'kjurətɪsi'kju:ritiaccount 账户ə'kaunt ə'kauntbackup 备份'bæk,ʌp 'bæk,ʌp 啊physical 物理的'fɪzɪk! 'fizikəldisk 磁盘dɪsk diskpipe 管道paɪp paiptape 磁带tep teiprestore 恢复rɪ'stor ri'stɔ:dump 转储dʌmp dʌmpdifferential 差异备份,dɪfə'rɛnʃəl ,difə'renʃəl truncate 截去'trʌŋket'trʌŋkeit啊んrecovery 复原rɪ'kʌvərɪri'kʌvəri 啊caption 标题'kæpʃən 'kæpʃənfont 字体fɑnt fɔnt 啊text 本文tɛkst t ekstprivate 个人的,私人的'praɪvɪt 'praivitsub 子sʌb sʌb 啊click 点击klɪk klikbeep 警笛声bip bi:ptextbox 文本框checkbox 复选框ListBox 列表框ComboBox 组合框enabled 启用状态dim 标注dɪm dimsucess 成功error 错误'ɛrɚ'erə第17章为空thing 事务θɪŋθiŋdiagram 图表'daɪə,græm 'daiəgræm 谷association 关联ə,sosɪ'eʃən ə,səusi'eiʃən package 打包'pækɪdʒ'pækidʒpublic 公用的'pʌblɪk 'pʌblik 柚捕protected 受保护的prə'tɛktɪd prə'tektidprivate 私人的'praɪvɪt 'praivitpersistent 持久性pɚ'sɪstənt pə'sistənt multiplicity 多样性,mʌltə'plɪsətɪ,mʌlti'plisiti aggregation 聚集,ægrɪ'geʃən ,ægri'geiʃən composition 组成,kɑmpə'zɪʃən ,kɔmpə'ziʃən generalization 继承,dʒɛnərəlai'zeʃən ,dʒenərəlai'zeiʃən cashier 出纳员kæ'ʃɪr kæ'ʃiədescription 描述dɪ'skrɪpʃən di'skripʃən D 哥provider 供应者prə'vaɪdɚprə'vaidəsalesperson 销售员'selz,pɚsn 'seilz,pə:sn universal 普遍的,junə'vɝs! ,ju:ni'və:səladaptive 适应的ə'dæptɪv ə'dæptivhierarchical 层次结构,haɪə'rɑrkɪkl ,haiə'rɑ:kikl warehouse 仓库,货栈'wɛr,haus 'wɛəhaus hierarchy 层次结构'haɪə,rɑrkɪ'haiərɑ:kilevel 层次'lɛv! 'levldimension 维dɪ'mɛnʃən d i'menʃəncube 立方体kjub kju:bdrill-down 钻取roll-up 卷起'rol,ʌp 'rəul,ʌpslice 切片slaɪs slaisdice 切块daɪs dais词组意思缩写data processing 数据处理Data management 数据管理Database management system 数据库管理系统DBMS database system 数据库系统DBSdatabase administrator 数据库管理员DBAend user 最终用户;最终使用者database application system 数据库应用系统DBASdata model 数据模型conceptual data models 概念数据模型entity set 实体集relationship set 联系集relational model 关系模型Data View 数据视图Data abstract 数据抽象Divide and Conquer 分治External level 外部级Conceptual level 概念级Internal level 内部级view level 视图级logical level 逻辑级physical level 物理级external schema 外模式internal schema 内模式data definition language 数据定义语言DDLdata manipulation language 数据操纵语言DML metadata 元数据Data items 数据项data flow diagram 数据流程图DFDmanaged objects 被管对象MOtransaction processing performance council 每秒实务数TPC for exposition only 参照图identifier-independent entities 独立标识符实体集identifier-dependent entities 从属标识符实体集identifying relationships 标定型联系non-identifying relationships 非标定型联系categorization relationships 分类联系non-specific relationships 不确定联系primary key 主关键码foreign key 外部关键码specific relationship 确定型联系connection relationship 连接联系generic entity 一般实体集category entity 分类实体集attribute value 属性值userid 用户标识符integrity constraint 完整性约束user-defined integrity 用户定义约束functional dependencies 函数依赖first normal form 第一范式1NFsecond normal form 第二范式2NFthird normal form 第三范式3NFboyce-codd normal form 改进的第三范式BCNF multivalued dependency 多值依赖forth normal form 第四范式4NFjoin dependencies 连接依赖fifth normal form 第五范式5NFsearch key 查找码heap file 堆文件sequential file 顺序文件clustering file 聚集文件index file 索引文件hashing file 散列文件indexing 索引技术ordered index 有序索引index record 索引记录index entry 附标入口;索引项hash function 散列函数indexed file 被索引文件clustering index 聚集索引nonclustering index 非聚集索引index - sequential file 索引顺序文件sequential access 序列存取;顺序存取direct access 直接存取dense index 稠密索引sparse index 稀疏索引primary index 主索引secondary index 辅助索引bucket overflow 桶溢出dada dictionary 数据字典metadata 元数据system catalog 系统目录derived attribute 派生属性architectural design总体结构设计procedural design 过程设计data design 数据设计procedure design language 过程设计语言PDL pointing device 指点器structured query language 结构化查询语言SQLUnicode 统一的字符编码标准identity card 身份证group by 群组依据serial schedule 串行调度concurrent schedule 并发调度Serializable 可串行conflict serializable 冲突可串行的exclusive lock 排它锁X锁shared lock 共享锁S锁locking protocol 锁协议two-phase locking 两阶段锁2PLlog file 日志文件Distributed Transaction coordinator 分布式事务协调器DTCenterprise manager 企业管理器query analyzer 查询分析器log on 登录American Standard Code for Information Interchange 美国信息交换标准代码ASCII data transformation service 数据转换服务DTSobject linking and embeding 对象的链接与嵌入OLEOpen Database Connectivity 开放式数据库连接ODBCapplication interface 应用接口APIobject linking and embed database 对象链接与嵌入的数据库OLE DB activex data object 动态数据对象ADOapplication programming interface 应用程序编程接口APIjava database connectivity standard JDBC第17章为空unified modeling language 统一建模语言UMLmeta - meta model 元元模型层meta model 元模型层user model 用户模型层class model 类模型Type Model 类型模型object model 对象模型instance model 实例模型use - case diagram 用例视图class diagram 结构视图object diagram 对象图sequence diagram 行为视图collaboration diagram 协作图state diagram 状态图activity diagram 活动图sequence diagram 实现视图depolyment diagram 环境视图Superclass 超类communication association 通信关联sterotype 构造型share aggregation 共享聚集subsystem 子系统shared memory 共用存储器shared disk 共用磁盘shared nothing 无共享结构round robin 轮转法hash partitioning 散列划分range partitioning 范围划分operational data 操作性数据decision support system 决策支持系统operational data store 操作性数据存储materrialized view 实视图metadata repository 元数据库On-Line Analytical Processing 在线分析处理materialized views 物化视图rough set 粗糙集。
etcd模糊匹配前缀
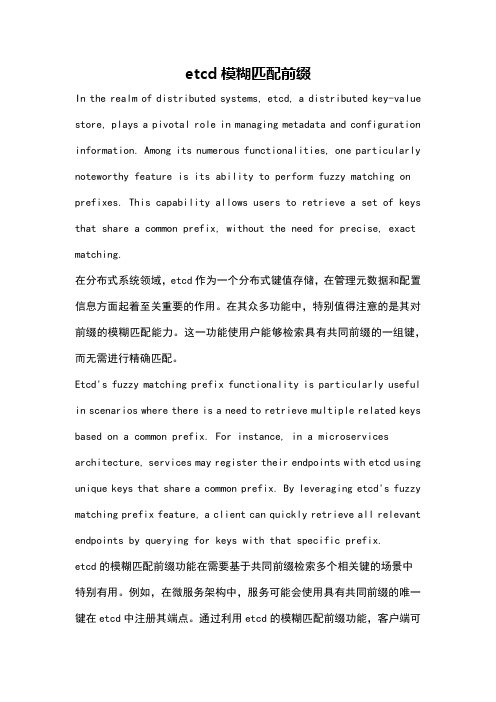
etcd模糊匹配前缀In the realm of distributed systems, etcd, a distributed key-value store, plays a pivotal role in managing metadata and configuration information. Among its numerous functionalities, one particularly noteworthy feature is its ability to perform fuzzy matching on prefixes. This capability allows users to retrieve a set of keys that share a common prefix, without the need for precise, exact matching.在分布式系统领域,etcd作为一个分布式键值存储,在管理元数据和配置信息方面起着至关重要的作用。
在其众多功能中,特别值得注意的是其对前缀的模糊匹配能力。
这一功能使用户能够检索具有共同前缀的一组键,而无需进行精确匹配。
Etcd's fuzzy matching prefix functionality is particularly useful in scenarios where there is a need to retrieve multiple related keys based on a common prefix. For instance, in a microservices architecture, services may register their endpoints with etcd using unique keys that share a common prefix. By leveraging etcd's fuzzy matching prefix feature, a client can quickly retrieve all relevant endpoints by querying for keys with that specific prefix.etcd的模糊匹配前缀功能在需要基于共同前缀检索多个相关键的场景中特别有用。
网络信息资源的特点
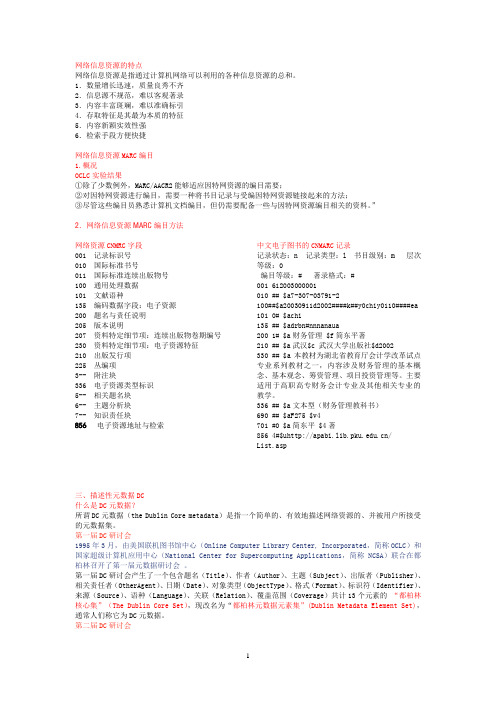
网络信息资源的特点网络信息资源是指通过计算机网络可以利用的各种信息资源的总和。
1.数量增长迅速,质量良秀不齐2.信息源不规范,难以客观著录3.内容丰富斑斓,难以准确标引4.存取特征是其最为本质的特征5.内容新颖实效性强6.检索手段方便快捷网络信息资源MARC编目1.概况OCLC实验结果①除了少数例外,MARC/AACR2能够适应因特网资源的编目需要;②对因特网资源进行编目,需要一种将书目记录与受编因特网资源链接起来的方法;③尽管这些编目员熟悉计算机文档编目,但仍需要配备一些与因特网资源编目相关的资料。
”2.网络信息资源MARC编目方法网络资源CNMRC字段001 记录标识号010 国际标准书号011 国际标准连续出版物号100 通用处理数据101 文献语种135 编码数据字段:电子资源200 题名与责任说明205 版本说明207 资料特定细节项:连续出版物卷期编号230 资料特定细节项:电子资源特征210 出版发行项225 丛编项3-- 附注块336 电子资源类型标识5-- 相关题名块6-- 主题分析块7-- 知识责任块856电子资源地址与检索中文电子图书的CNMARC记录记录状态:n 记录类型:l 书目级别:m 层次等级:0编目等级:# 著录格式:#001 612003000001010 ## $a7-307-03791-2100##$a20030911d2002####k##y0chiy0110####ea 101 0# $achi135 ## $adrbn#nnnanaua200 1# $a财务管理 $f简东平著210 ## $a武汉$c 武汉大学出版社$d2002330 ## $a本教材为湖北省教育厅会计学改革试点专业系列教材之一,内容涉及财务管理的基本概念、基本观念、筹资管理、项目投资管理等。
主要适用于高职高专财务会计专业及其他相关专业的教学。
336 ## $a文本型(财务管理教科书)690 ## $aF275 $v4701 #0 $a简东平 $4著856 4#$u/List.asp三、描述性元数据DC什么是DC元数据?所谓DC元数据(the Dublin Core metadata)是指一个简单的、有效地描述网络资源的、并被用户所接受的元数据集。
机器学习与人工智能领域中常用的英语词汇
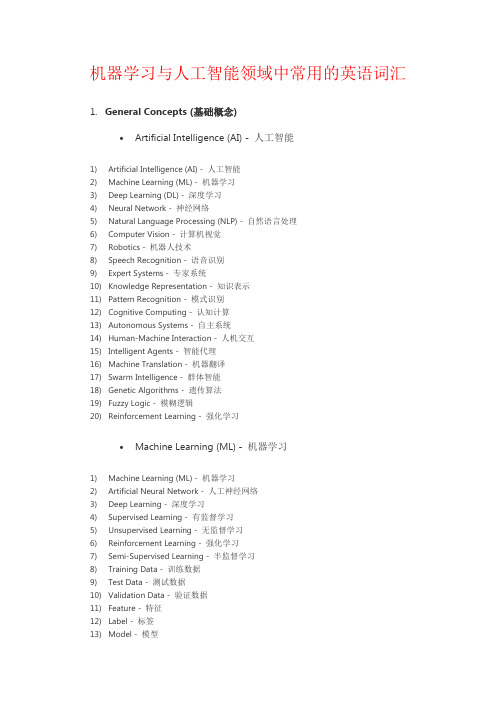
机器学习与人工智能领域中常用的英语词汇1.General Concepts (基础概念)•Artificial Intelligence (AI) - 人工智能1)Artificial Intelligence (AI) - 人工智能2)Machine Learning (ML) - 机器学习3)Deep Learning (DL) - 深度学习4)Neural Network - 神经网络5)Natural Language Processing (NLP) - 自然语言处理6)Computer Vision - 计算机视觉7)Robotics - 机器人技术8)Speech Recognition - 语音识别9)Expert Systems - 专家系统10)Knowledge Representation - 知识表示11)Pattern Recognition - 模式识别12)Cognitive Computing - 认知计算13)Autonomous Systems - 自主系统14)Human-Machine Interaction - 人机交互15)Intelligent Agents - 智能代理16)Machine Translation - 机器翻译17)Swarm Intelligence - 群体智能18)Genetic Algorithms - 遗传算法19)Fuzzy Logic - 模糊逻辑20)Reinforcement Learning - 强化学习•Machine Learning (ML) - 机器学习1)Machine Learning (ML) - 机器学习2)Artificial Neural Network - 人工神经网络3)Deep Learning - 深度学习4)Supervised Learning - 有监督学习5)Unsupervised Learning - 无监督学习6)Reinforcement Learning - 强化学习7)Semi-Supervised Learning - 半监督学习8)Training Data - 训练数据9)Test Data - 测试数据10)Validation Data - 验证数据11)Feature - 特征12)Label - 标签13)Model - 模型14)Algorithm - 算法15)Regression - 回归16)Classification - 分类17)Clustering - 聚类18)Dimensionality Reduction - 降维19)Overfitting - 过拟合20)Underfitting - 欠拟合•Deep Learning (DL) - 深度学习1)Deep Learning - 深度学习2)Neural Network - 神经网络3)Artificial Neural Network (ANN) - 人工神经网络4)Convolutional Neural Network (CNN) - 卷积神经网络5)Recurrent Neural Network (RNN) - 循环神经网络6)Long Short-Term Memory (LSTM) - 长短期记忆网络7)Gated Recurrent Unit (GRU) - 门控循环单元8)Autoencoder - 自编码器9)Generative Adversarial Network (GAN) - 生成对抗网络10)Transfer Learning - 迁移学习11)Pre-trained Model - 预训练模型12)Fine-tuning - 微调13)Feature Extraction - 特征提取14)Activation Function - 激活函数15)Loss Function - 损失函数16)Gradient Descent - 梯度下降17)Backpropagation - 反向传播18)Epoch - 训练周期19)Batch Size - 批量大小20)Dropout - 丢弃法•Neural Network - 神经网络1)Neural Network - 神经网络2)Artificial Neural Network (ANN) - 人工神经网络3)Deep Neural Network (DNN) - 深度神经网络4)Convolutional Neural Network (CNN) - 卷积神经网络5)Recurrent Neural Network (RNN) - 循环神经网络6)Long Short-Term Memory (LSTM) - 长短期记忆网络7)Gated Recurrent Unit (GRU) - 门控循环单元8)Feedforward Neural Network - 前馈神经网络9)Multi-layer Perceptron (MLP) - 多层感知器10)Radial Basis Function Network (RBFN) - 径向基函数网络11)Hopfield Network - 霍普菲尔德网络12)Boltzmann Machine - 玻尔兹曼机13)Autoencoder - 自编码器14)Spiking Neural Network (SNN) - 脉冲神经网络15)Self-organizing Map (SOM) - 自组织映射16)Restricted Boltzmann Machine (RBM) - 受限玻尔兹曼机17)Hebbian Learning - 海比安学习18)Competitive Learning - 竞争学习19)Neuroevolutionary - 神经进化20)Neuron - 神经元•Algorithm - 算法1)Algorithm - 算法2)Supervised Learning Algorithm - 有监督学习算法3)Unsupervised Learning Algorithm - 无监督学习算法4)Reinforcement Learning Algorithm - 强化学习算法5)Classification Algorithm - 分类算法6)Regression Algorithm - 回归算法7)Clustering Algorithm - 聚类算法8)Dimensionality Reduction Algorithm - 降维算法9)Decision Tree Algorithm - 决策树算法10)Random Forest Algorithm - 随机森林算法11)Support Vector Machine (SVM) Algorithm - 支持向量机算法12)K-Nearest Neighbors (KNN) Algorithm - K近邻算法13)Naive Bayes Algorithm - 朴素贝叶斯算法14)Gradient Descent Algorithm - 梯度下降算法15)Genetic Algorithm - 遗传算法16)Neural Network Algorithm - 神经网络算法17)Deep Learning Algorithm - 深度学习算法18)Ensemble Learning Algorithm - 集成学习算法19)Reinforcement Learning Algorithm - 强化学习算法20)Metaheuristic Algorithm - 元启发式算法•Model - 模型1)Model - 模型2)Machine Learning Model - 机器学习模型3)Artificial Intelligence Model - 人工智能模型4)Predictive Model - 预测模型5)Classification Model - 分类模型6)Regression Model - 回归模型7)Generative Model - 生成模型8)Discriminative Model - 判别模型9)Probabilistic Model - 概率模型10)Statistical Model - 统计模型11)Neural Network Model - 神经网络模型12)Deep Learning Model - 深度学习模型13)Ensemble Model - 集成模型14)Reinforcement Learning Model - 强化学习模型15)Support Vector Machine (SVM) Model - 支持向量机模型16)Decision Tree Model - 决策树模型17)Random Forest Model - 随机森林模型18)Naive Bayes Model - 朴素贝叶斯模型19)Autoencoder Model - 自编码器模型20)Convolutional Neural Network (CNN) Model - 卷积神经网络模型•Dataset - 数据集1)Dataset - 数据集2)Training Dataset - 训练数据集3)Test Dataset - 测试数据集4)Validation Dataset - 验证数据集5)Balanced Dataset - 平衡数据集6)Imbalanced Dataset - 不平衡数据集7)Synthetic Dataset - 合成数据集8)Benchmark Dataset - 基准数据集9)Open Dataset - 开放数据集10)Labeled Dataset - 标记数据集11)Unlabeled Dataset - 未标记数据集12)Semi-Supervised Dataset - 半监督数据集13)Multiclass Dataset - 多分类数据集14)Feature Set - 特征集15)Data Augmentation - 数据增强16)Data Preprocessing - 数据预处理17)Missing Data - 缺失数据18)Outlier Detection - 异常值检测19)Data Imputation - 数据插补20)Metadata - 元数据•Training - 训练1)Training - 训练2)Training Data - 训练数据3)Training Phase - 训练阶段4)Training Set - 训练集5)Training Examples - 训练样本6)Training Instance - 训练实例7)Training Algorithm - 训练算法8)Training Model - 训练模型9)Training Process - 训练过程10)Training Loss - 训练损失11)Training Epoch - 训练周期12)Training Batch - 训练批次13)Online Training - 在线训练14)Offline Training - 离线训练15)Continuous Training - 连续训练16)Transfer Learning - 迁移学习17)Fine-Tuning - 微调18)Curriculum Learning - 课程学习19)Self-Supervised Learning - 自监督学习20)Active Learning - 主动学习•Testing - 测试1)Testing - 测试2)Test Data - 测试数据3)Test Set - 测试集4)Test Examples - 测试样本5)Test Instance - 测试实例6)Test Phase - 测试阶段7)Test Accuracy - 测试准确率8)Test Loss - 测试损失9)Test Error - 测试错误10)Test Metrics - 测试指标11)Test Suite - 测试套件12)Test Case - 测试用例13)Test Coverage - 测试覆盖率14)Cross-Validation - 交叉验证15)Holdout Validation - 留出验证16)K-Fold Cross-Validation - K折交叉验证17)Stratified Cross-Validation - 分层交叉验证18)Test Driven Development (TDD) - 测试驱动开发19)A/B Testing - A/B 测试20)Model Evaluation - 模型评估•Validation - 验证1)Validation - 验证2)Validation Data - 验证数据3)Validation Set - 验证集4)Validation Examples - 验证样本5)Validation Instance - 验证实例6)Validation Phase - 验证阶段7)Validation Accuracy - 验证准确率8)Validation Loss - 验证损失9)Validation Error - 验证错误10)Validation Metrics - 验证指标11)Cross-Validation - 交叉验证12)Holdout Validation - 留出验证13)K-Fold Cross-Validation - K折交叉验证14)Stratified Cross-Validation - 分层交叉验证15)Leave-One-Out Cross-Validation - 留一法交叉验证16)Validation Curve - 验证曲线17)Hyperparameter Validation - 超参数验证18)Model Validation - 模型验证19)Early Stopping - 提前停止20)Validation Strategy - 验证策略•Supervised Learning - 有监督学习1)Supervised Learning - 有监督学习2)Label - 标签3)Feature - 特征4)Target - 目标5)Training Labels - 训练标签6)Training Features - 训练特征7)Training Targets - 训练目标8)Training Examples - 训练样本9)Training Instance - 训练实例10)Regression - 回归11)Classification - 分类12)Predictor - 预测器13)Regression Model - 回归模型14)Classifier - 分类器15)Decision Tree - 决策树16)Support Vector Machine (SVM) - 支持向量机17)Neural Network - 神经网络18)Feature Engineering - 特征工程19)Model Evaluation - 模型评估20)Overfitting - 过拟合21)Underfitting - 欠拟合22)Bias-Variance Tradeoff - 偏差-方差权衡•Unsupervised Learning - 无监督学习1)Unsupervised Learning - 无监督学习2)Clustering - 聚类3)Dimensionality Reduction - 降维4)Anomaly Detection - 异常检测5)Association Rule Learning - 关联规则学习6)Feature Extraction - 特征提取7)Feature Selection - 特征选择8)K-Means - K均值9)Hierarchical Clustering - 层次聚类10)Density-Based Clustering - 基于密度的聚类11)Principal Component Analysis (PCA) - 主成分分析12)Independent Component Analysis (ICA) - 独立成分分析13)T-distributed Stochastic Neighbor Embedding (t-SNE) - t分布随机邻居嵌入14)Gaussian Mixture Model (GMM) - 高斯混合模型15)Self-Organizing Maps (SOM) - 自组织映射16)Autoencoder - 自动编码器17)Latent Variable - 潜变量18)Data Preprocessing - 数据预处理19)Outlier Detection - 异常值检测20)Clustering Algorithm - 聚类算法•Reinforcement Learning - 强化学习1)Reinforcement Learning - 强化学习2)Agent - 代理3)Environment - 环境4)State - 状态5)Action - 动作6)Reward - 奖励7)Policy - 策略8)Value Function - 值函数9)Q-Learning - Q学习10)Deep Q-Network (DQN) - 深度Q网络11)Policy Gradient - 策略梯度12)Actor-Critic - 演员-评论家13)Exploration - 探索14)Exploitation - 开发15)Temporal Difference (TD) - 时间差分16)Markov Decision Process (MDP) - 马尔可夫决策过程17)State-Action-Reward-State-Action (SARSA) - 状态-动作-奖励-状态-动作18)Policy Iteration - 策略迭代19)Value Iteration - 值迭代20)Monte Carlo Methods - 蒙特卡洛方法•Semi-Supervised Learning - 半监督学习1)Semi-Supervised Learning - 半监督学习2)Labeled Data - 有标签数据3)Unlabeled Data - 无标签数据4)Label Propagation - 标签传播5)Self-Training - 自训练6)Co-Training - 协同训练7)Transudative Learning - 传导学习8)Inductive Learning - 归纳学习9)Manifold Regularization - 流形正则化10)Graph-based Methods - 基于图的方法11)Cluster Assumption - 聚类假设12)Low-Density Separation - 低密度分离13)Semi-Supervised Support Vector Machines (S3VM) - 半监督支持向量机14)Expectation-Maximization (EM) - 期望最大化15)Co-EM - 协同期望最大化16)Entropy-Regularized EM - 熵正则化EM17)Mean Teacher - 平均教师18)Virtual Adversarial Training - 虚拟对抗训练19)Tri-training - 三重训练20)Mix Match - 混合匹配•Feature - 特征1)Feature - 特征2)Feature Engineering - 特征工程3)Feature Extraction - 特征提取4)Feature Selection - 特征选择5)Input Features - 输入特征6)Output Features - 输出特征7)Feature Vector - 特征向量8)Feature Space - 特征空间9)Feature Representation - 特征表示10)Feature Transformation - 特征转换11)Feature Importance - 特征重要性12)Feature Scaling - 特征缩放13)Feature Normalization - 特征归一化14)Feature Encoding - 特征编码15)Feature Fusion - 特征融合16)Feature Dimensionality Reduction - 特征维度减少17)Continuous Feature - 连续特征18)Categorical Feature - 分类特征19)Nominal Feature - 名义特征20)Ordinal Feature - 有序特征•Label - 标签1)Label - 标签2)Labeling - 标注3)Ground Truth - 地面真值4)Class Label - 类别标签5)Target Variable - 目标变量6)Labeling Scheme - 标注方案7)Multi-class Labeling - 多类别标注8)Binary Labeling - 二分类标注9)Label Noise - 标签噪声10)Labeling Error - 标注错误11)Label Propagation - 标签传播12)Unlabeled Data - 无标签数据13)Labeled Data - 有标签数据14)Semi-supervised Learning - 半监督学习15)Active Learning - 主动学习16)Weakly Supervised Learning - 弱监督学习17)Noisy Label Learning - 噪声标签学习18)Self-training - 自训练19)Crowdsourcing Labeling - 众包标注20)Label Smoothing - 标签平滑化•Prediction - 预测1)Prediction - 预测2)Forecasting - 预测3)Regression - 回归4)Classification - 分类5)Time Series Prediction - 时间序列预测6)Forecast Accuracy - 预测准确性7)Predictive Modeling - 预测建模8)Predictive Analytics - 预测分析9)Forecasting Method - 预测方法10)Predictive Performance - 预测性能11)Predictive Power - 预测能力12)Prediction Error - 预测误差13)Prediction Interval - 预测区间14)Prediction Model - 预测模型15)Predictive Uncertainty - 预测不确定性16)Forecast Horizon - 预测时间跨度17)Predictive Maintenance - 预测性维护18)Predictive Policing - 预测式警务19)Predictive Healthcare - 预测性医疗20)Predictive Maintenance - 预测性维护•Classification - 分类1)Classification - 分类2)Classifier - 分类器3)Class - 类别4)Classify - 对数据进行分类5)Class Label - 类别标签6)Binary Classification - 二元分类7)Multiclass Classification - 多类分类8)Class Probability - 类别概率9)Decision Boundary - 决策边界10)Decision Tree - 决策树11)Support Vector Machine (SVM) - 支持向量机12)K-Nearest Neighbors (KNN) - K最近邻算法13)Naive Bayes - 朴素贝叶斯14)Logistic Regression - 逻辑回归15)Random Forest - 随机森林16)Neural Network - 神经网络17)SoftMax Function - SoftMax函数18)One-vs-All (One-vs-Rest) - 一对多(一对剩余)19)Ensemble Learning - 集成学习20)Confusion Matrix - 混淆矩阵•Regression - 回归1)Regression Analysis - 回归分析2)Linear Regression - 线性回归3)Multiple Regression - 多元回归4)Polynomial Regression - 多项式回归5)Logistic Regression - 逻辑回归6)Ridge Regression - 岭回归7)Lasso Regression - Lasso回归8)Elastic Net Regression - 弹性网络回归9)Regression Coefficients - 回归系数10)Residuals - 残差11)Ordinary Least Squares (OLS) - 普通最小二乘法12)Ridge Regression Coefficient - 岭回归系数13)Lasso Regression Coefficient - Lasso回归系数14)Elastic Net Regression Coefficient - 弹性网络回归系数15)Regression Line - 回归线16)Prediction Error - 预测误差17)Regression Model - 回归模型18)Nonlinear Regression - 非线性回归19)Generalized Linear Models (GLM) - 广义线性模型20)Coefficient of Determination (R-squared) - 决定系数21)F-test - F检验22)Homoscedasticity - 同方差性23)Heteroscedasticity - 异方差性24)Autocorrelation - 自相关25)Multicollinearity - 多重共线性26)Outliers - 异常值27)Cross-validation - 交叉验证28)Feature Selection - 特征选择29)Feature Engineering - 特征工程30)Regularization - 正则化2.Neural Networks and Deep Learning (神经网络与深度学习)•Convolutional Neural Network (CNN) - 卷积神经网络1)Convolutional Neural Network (CNN) - 卷积神经网络2)Convolution Layer - 卷积层3)Feature Map - 特征图4)Convolution Operation - 卷积操作5)Stride - 步幅6)Padding - 填充7)Pooling Layer - 池化层8)Max Pooling - 最大池化9)Average Pooling - 平均池化10)Fully Connected Layer - 全连接层11)Activation Function - 激活函数12)Rectified Linear Unit (ReLU) - 线性修正单元13)Dropout - 随机失活14)Batch Normalization - 批量归一化15)Transfer Learning - 迁移学习16)Fine-Tuning - 微调17)Image Classification - 图像分类18)Object Detection - 物体检测19)Semantic Segmentation - 语义分割20)Instance Segmentation - 实例分割21)Generative Adversarial Network (GAN) - 生成对抗网络22)Image Generation - 图像生成23)Style Transfer - 风格迁移24)Convolutional Autoencoder - 卷积自编码器25)Recurrent Neural Network (RNN) - 循环神经网络•Recurrent Neural Network (RNN) - 循环神经网络1)Recurrent Neural Network (RNN) - 循环神经网络2)Long Short-Term Memory (LSTM) - 长短期记忆网络3)Gated Recurrent Unit (GRU) - 门控循环单元4)Sequence Modeling - 序列建模5)Time Series Prediction - 时间序列预测6)Natural Language Processing (NLP) - 自然语言处理7)Text Generation - 文本生成8)Sentiment Analysis - 情感分析9)Named Entity Recognition (NER) - 命名实体识别10)Part-of-Speech Tagging (POS Tagging) - 词性标注11)Sequence-to-Sequence (Seq2Seq) - 序列到序列12)Attention Mechanism - 注意力机制13)Encoder-Decoder Architecture - 编码器-解码器架构14)Bidirectional RNN - 双向循环神经网络15)Teacher Forcing - 强制教师法16)Backpropagation Through Time (BPTT) - 通过时间的反向传播17)Vanishing Gradient Problem - 梯度消失问题18)Exploding Gradient Problem - 梯度爆炸问题19)Language Modeling - 语言建模20)Speech Recognition - 语音识别•Long Short-Term Memory (LSTM) - 长短期记忆网络1)Long Short-Term Memory (LSTM) - 长短期记忆网络2)Cell State - 细胞状态3)Hidden State - 隐藏状态4)Forget Gate - 遗忘门5)Input Gate - 输入门6)Output Gate - 输出门7)Peephole Connections - 窥视孔连接8)Gated Recurrent Unit (GRU) - 门控循环单元9)Vanishing Gradient Problem - 梯度消失问题10)Exploding Gradient Problem - 梯度爆炸问题11)Sequence Modeling - 序列建模12)Time Series Prediction - 时间序列预测13)Natural Language Processing (NLP) - 自然语言处理14)Text Generation - 文本生成15)Sentiment Analysis - 情感分析16)Named Entity Recognition (NER) - 命名实体识别17)Part-of-Speech Tagging (POS Tagging) - 词性标注18)Attention Mechanism - 注意力机制19)Encoder-Decoder Architecture - 编码器-解码器架构20)Bidirectional LSTM - 双向长短期记忆网络•Attention Mechanism - 注意力机制1)Attention Mechanism - 注意力机制2)Self-Attention - 自注意力3)Multi-Head Attention - 多头注意力4)Transformer - 变换器5)Query - 查询6)Key - 键7)Value - 值8)Query-Value Attention - 查询-值注意力9)Dot-Product Attention - 点积注意力10)Scaled Dot-Product Attention - 缩放点积注意力11)Additive Attention - 加性注意力12)Context Vector - 上下文向量13)Attention Score - 注意力分数14)SoftMax Function - SoftMax函数15)Attention Weight - 注意力权重16)Global Attention - 全局注意力17)Local Attention - 局部注意力18)Positional Encoding - 位置编码19)Encoder-Decoder Attention - 编码器-解码器注意力20)Cross-Modal Attention - 跨模态注意力•Generative Adversarial Network (GAN) - 生成对抗网络1)Generative Adversarial Network (GAN) - 生成对抗网络2)Generator - 生成器3)Discriminator - 判别器4)Adversarial Training - 对抗训练5)Minimax Game - 极小极大博弈6)Nash Equilibrium - 纳什均衡7)Mode Collapse - 模式崩溃8)Training Stability - 训练稳定性9)Loss Function - 损失函数10)Discriminative Loss - 判别损失11)Generative Loss - 生成损失12)Wasserstein GAN (WGAN) - Wasserstein GAN(WGAN)13)Deep Convolutional GAN (DCGAN) - 深度卷积生成对抗网络(DCGAN)14)Conditional GAN (c GAN) - 条件生成对抗网络(c GAN)15)Style GAN - 风格生成对抗网络16)Cycle GAN - 循环生成对抗网络17)Progressive Growing GAN (PGGAN) - 渐进式增长生成对抗网络(PGGAN)18)Self-Attention GAN (SAGAN) - 自注意力生成对抗网络(SAGAN)19)Big GAN - 大规模生成对抗网络20)Adversarial Examples - 对抗样本•Encoder-Decoder - 编码器-解码器1)Encoder-Decoder Architecture - 编码器-解码器架构2)Encoder - 编码器3)Decoder - 解码器4)Sequence-to-Sequence Model (Seq2Seq) - 序列到序列模型5)State Vector - 状态向量6)Context Vector - 上下文向量7)Hidden State - 隐藏状态8)Attention Mechanism - 注意力机制9)Teacher Forcing - 强制教师法10)Beam Search - 束搜索11)Recurrent Neural Network (RNN) - 循环神经网络12)Long Short-Term Memory (LSTM) - 长短期记忆网络13)Gated Recurrent Unit (GRU) - 门控循环单元14)Bidirectional Encoder - 双向编码器15)Greedy Decoding - 贪婪解码16)Masking - 遮盖17)Dropout - 随机失活18)Embedding Layer - 嵌入层19)Cross-Entropy Loss - 交叉熵损失20)Tokenization - 令牌化•Transfer Learning - 迁移学习1)Transfer Learning - 迁移学习2)Source Domain - 源领域3)Target Domain - 目标领域4)Fine-Tuning - 微调5)Domain Adaptation - 领域自适应6)Pre-Trained Model - 预训练模型7)Feature Extraction - 特征提取8)Knowledge Transfer - 知识迁移9)Unsupervised Domain Adaptation - 无监督领域自适应10)Semi-Supervised Domain Adaptation - 半监督领域自适应11)Multi-Task Learning - 多任务学习12)Data Augmentation - 数据增强13)Task Transfer - 任务迁移14)Model Agnostic Meta-Learning (MAML) - 与模型无关的元学习(MAML)15)One-Shot Learning - 单样本学习16)Zero-Shot Learning - 零样本学习17)Few-Shot Learning - 少样本学习18)Knowledge Distillation - 知识蒸馏19)Representation Learning - 表征学习20)Adversarial Transfer Learning - 对抗迁移学习•Pre-trained Models - 预训练模型1)Pre-trained Model - 预训练模型2)Transfer Learning - 迁移学习3)Fine-Tuning - 微调4)Knowledge Transfer - 知识迁移5)Domain Adaptation - 领域自适应6)Feature Extraction - 特征提取7)Representation Learning - 表征学习8)Language Model - 语言模型9)Bidirectional Encoder Representations from Transformers (BERT) - 双向编码器结构转换器10)Generative Pre-trained Transformer (GPT) - 生成式预训练转换器11)Transformer-based Models - 基于转换器的模型12)Masked Language Model (MLM) - 掩蔽语言模型13)Cloze Task - 填空任务14)Tokenization - 令牌化15)Word Embeddings - 词嵌入16)Sentence Embeddings - 句子嵌入17)Contextual Embeddings - 上下文嵌入18)Self-Supervised Learning - 自监督学习19)Large-Scale Pre-trained Models - 大规模预训练模型•Loss Function - 损失函数1)Loss Function - 损失函数2)Mean Squared Error (MSE) - 均方误差3)Mean Absolute Error (MAE) - 平均绝对误差4)Cross-Entropy Loss - 交叉熵损失5)Binary Cross-Entropy Loss - 二元交叉熵损失6)Categorical Cross-Entropy Loss - 分类交叉熵损失7)Hinge Loss - 合页损失8)Huber Loss - Huber损失9)Wasserstein Distance - Wasserstein距离10)Triplet Loss - 三元组损失11)Contrastive Loss - 对比损失12)Dice Loss - Dice损失13)Focal Loss - 焦点损失14)GAN Loss - GAN损失15)Adversarial Loss - 对抗损失16)L1 Loss - L1损失17)L2 Loss - L2损失18)Huber Loss - Huber损失19)Quantile Loss - 分位数损失•Activation Function - 激活函数1)Activation Function - 激活函数2)Sigmoid Function - Sigmoid函数3)Hyperbolic Tangent Function (Tanh) - 双曲正切函数4)Rectified Linear Unit (Re LU) - 矩形线性单元5)Parametric Re LU (P Re LU) - 参数化Re LU6)Exponential Linear Unit (ELU) - 指数线性单元7)Swish Function - Swish函数8)Softplus Function - Soft plus函数9)Softmax Function - SoftMax函数10)Hard Tanh Function - 硬双曲正切函数11)Softsign Function - Softsign函数12)GELU (Gaussian Error Linear Unit) - GELU(高斯误差线性单元)13)Mish Function - Mish函数14)CELU (Continuous Exponential Linear Unit) - CELU(连续指数线性单元)15)Bent Identity Function - 弯曲恒等函数16)Gaussian Error Linear Units (GELUs) - 高斯误差线性单元17)Adaptive Piecewise Linear (APL) - 自适应分段线性函数18)Radial Basis Function (RBF) - 径向基函数•Backpropagation - 反向传播1)Backpropagation - 反向传播2)Gradient Descent - 梯度下降3)Partial Derivative - 偏导数4)Chain Rule - 链式法则5)Forward Pass - 前向传播6)Backward Pass - 反向传播7)Computational Graph - 计算图8)Neural Network - 神经网络9)Loss Function - 损失函数10)Gradient Calculation - 梯度计算11)Weight Update - 权重更新12)Activation Function - 激活函数13)Optimizer - 优化器14)Learning Rate - 学习率15)Mini-Batch Gradient Descent - 小批量梯度下降16)Stochastic Gradient Descent (SGD) - 随机梯度下降17)Batch Gradient Descent - 批量梯度下降18)Momentum - 动量19)Adam Optimizer - Adam优化器20)Learning Rate Decay - 学习率衰减•Gradient Descent - 梯度下降1)Gradient Descent - 梯度下降2)Stochastic Gradient Descent (SGD) - 随机梯度下降3)Mini-Batch Gradient Descent - 小批量梯度下降4)Batch Gradient Descent - 批量梯度下降5)Learning Rate - 学习率6)Momentum - 动量7)Adaptive Moment Estimation (Adam) - 自适应矩估计8)RMSprop - 均方根传播9)Learning Rate Schedule - 学习率调度10)Convergence - 收敛11)Divergence - 发散12)Adagrad - 自适应学习速率方法13)Adadelta - 自适应增量学习率方法14)Adamax - 自适应矩估计的扩展版本15)Nadam - Nesterov Accelerated Adaptive Moment Estimation16)Learning Rate Decay - 学习率衰减17)Step Size - 步长18)Conjugate Gradient Descent - 共轭梯度下降19)Line Search - 线搜索20)Newton's Method - 牛顿法•Learning Rate - 学习率1)Learning Rate - 学习率2)Adaptive Learning Rate - 自适应学习率3)Learning Rate Decay - 学习率衰减4)Initial Learning Rate - 初始学习率5)Step Size - 步长6)Momentum - 动量7)Exponential Decay - 指数衰减8)Annealing - 退火9)Cyclical Learning Rate - 循环学习率10)Learning Rate Schedule - 学习率调度11)Warm-up - 预热12)Learning Rate Policy - 学习率策略13)Learning Rate Annealing - 学习率退火14)Cosine Annealing - 余弦退火15)Gradient Clipping - 梯度裁剪16)Adapting Learning Rate - 适应学习率17)Learning Rate Multiplier - 学习率倍增器18)Learning Rate Reduction - 学习率降低19)Learning Rate Update - 学习率更新20)Scheduled Learning Rate - 定期学习率•Batch Size - 批量大小1)Batch Size - 批量大小2)Mini-Batch - 小批量3)Batch Gradient Descent - 批量梯度下降4)Stochastic Gradient Descent (SGD) - 随机梯度下降5)Mini-Batch Gradient Descent - 小批量梯度下降6)Online Learning - 在线学习7)Full-Batch - 全批量8)Data Batch - 数据批次9)Training Batch - 训练批次10)Batch Normalization - 批量归一化11)Batch-wise Optimization - 批量优化12)Batch Processing - 批量处理13)Batch Sampling - 批量采样14)Adaptive Batch Size - 自适应批量大小15)Batch Splitting - 批量分割16)Dynamic Batch Size - 动态批量大小17)Fixed Batch Size - 固定批量大小18)Batch-wise Inference - 批量推理19)Batch-wise Training - 批量训练20)Batch Shuffling - 批量洗牌•Epoch - 训练周期1)Training Epoch - 训练周期2)Epoch Size - 周期大小3)Early Stopping - 提前停止4)Validation Set - 验证集5)Training Set - 训练集6)Test Set - 测试集7)Overfitting - 过拟合8)Underfitting - 欠拟合9)Model Evaluation - 模型评估10)Model Selection - 模型选择11)Hyperparameter Tuning - 超参数调优12)Cross-Validation - 交叉验证13)K-fold Cross-Validation - K折交叉验证14)Stratified Cross-Validation - 分层交叉验证15)Leave-One-Out Cross-Validation (LOOCV) - 留一法交叉验证16)Grid Search - 网格搜索17)Random Search - 随机搜索18)Model Complexity - 模型复杂度19)Learning Curve - 学习曲线20)Convergence - 收敛3.Machine Learning Techniques and Algorithms (机器学习技术与算法)•Decision Tree - 决策树1)Decision Tree - 决策树2)Node - 节点3)Root Node - 根节点4)Leaf Node - 叶节点5)Internal Node - 内部节点6)Splitting Criterion - 分裂准则7)Gini Impurity - 基尼不纯度8)Entropy - 熵9)Information Gain - 信息增益10)Gain Ratio - 增益率11)Pruning - 剪枝12)Recursive Partitioning - 递归分割13)CART (Classification and Regression Trees) - 分类回归树14)ID3 (Iterative Dichotomiser 3) - 迭代二叉树315)C4.5 (successor of ID3) - C4.5(ID3的后继者)16)C5.0 (successor of C4.5) - C5.0(C4.5的后继者)17)Split Point - 分裂点18)Decision Boundary - 决策边界19)Pruned Tree - 剪枝后的树20)Decision Tree Ensemble - 决策树集成•Random Forest - 随机森林1)Random Forest - 随机森林2)Ensemble Learning - 集成学习3)Bootstrap Sampling - 自助采样4)Bagging (Bootstrap Aggregating) - 装袋法5)Out-of-Bag (OOB) Error - 袋外误差6)Feature Subset - 特征子集7)Decision Tree - 决策树8)Base Estimator - 基础估计器9)Tree Depth - 树深度10)Randomization - 随机化11)Majority Voting - 多数投票12)Feature Importance - 特征重要性13)OOB Score - 袋外得分14)Forest Size - 森林大小15)Max Features - 最大特征数16)Min Samples Split - 最小分裂样本数17)Min Samples Leaf - 最小叶节点样本数18)Gini Impurity - 基尼不纯度19)Entropy - 熵20)Variable Importance - 变量重要性•Support Vector Machine (SVM) - 支持向量机1)Support Vector Machine (SVM) - 支持向量机2)Hyperplane - 超平面3)Kernel Trick - 核技巧4)Kernel Function - 核函数5)Margin - 间隔6)Support Vectors - 支持向量7)Decision Boundary - 决策边界8)Maximum Margin Classifier - 最大间隔分类器9)Soft Margin Classifier - 软间隔分类器10) C Parameter - C参数11)Radial Basis Function (RBF) Kernel - 径向基函数核12)Polynomial Kernel - 多项式核13)Linear Kernel - 线性核14)Quadratic Kernel - 二次核15)Gaussian Kernel - 高斯核16)Regularization - 正则化17)Dual Problem - 对偶问题18)Primal Problem - 原始问题19)Kernelized SVM - 核化支持向量机20)Multiclass SVM - 多类支持向量机•K-Nearest Neighbors (KNN) - K-最近邻1)K-Nearest Neighbors (KNN) - K-最近邻2)Nearest Neighbor - 最近邻3)Distance Metric - 距离度量4)Euclidean Distance - 欧氏距离5)Manhattan Distance - 曼哈顿距离6)Minkowski Distance - 闵可夫斯基距离7)Cosine Similarity - 余弦相似度8)K Value - K值9)Majority Voting - 多数投票10)Weighted KNN - 加权KNN11)Radius Neighbors - 半径邻居12)Ball Tree - 球树13)KD Tree - KD树14)Locality-Sensitive Hashing (LSH) - 局部敏感哈希15)Curse of Dimensionality - 维度灾难16)Class Label - 类标签17)Training Set - 训练集18)Test Set - 测试集19)Validation Set - 验证集20)Cross-Validation - 交叉验证•Naive Bayes - 朴素贝叶斯1)Naive Bayes - 朴素贝叶斯2)Bayes' Theorem - 贝叶斯定理3)Prior Probability - 先验概率4)Posterior Probability - 后验概率5)Likelihood - 似然6)Class Conditional Probability - 类条件概率7)Feature Independence Assumption - 特征独立假设8)Multinomial Naive Bayes - 多项式朴素贝叶斯9)Gaussian Naive Bayes - 高斯朴素贝叶斯10)Bernoulli Naive Bayes - 伯努利朴素贝叶斯11)Laplace Smoothing - 拉普拉斯平滑12)Add-One Smoothing - 加一平滑13)Maximum A Posteriori (MAP) - 最大后验概率14)Maximum Likelihood Estimation (MLE) - 最大似然估计15)Classification - 分类16)Feature Vectors - 特征向量17)Training Set - 训练集18)Test Set - 测试集19)Class Label - 类标签20)Confusion Matrix - 混淆矩阵•Clustering - 聚类1)Clustering - 聚类2)Centroid - 质心3)Cluster Analysis - 聚类分析4)Partitioning Clustering - 划分式聚类5)Hierarchical Clustering - 层次聚类6)Density-Based Clustering - 基于密度的聚类7)K-Means Clustering - K均值聚类8)K-Medoids Clustering - K中心点聚类9)DBSCAN (Density-Based Spatial Clustering of Applications with Noise) - 基于密度的空间聚类算法10)Agglomerative Clustering - 聚合式聚类11)Dendrogram - 系统树图12)Silhouette Score - 轮廓系数13)Elbow Method - 肘部法则14)Clustering Validation - 聚类验证15)Intra-cluster Distance - 类内距离16)Inter-cluster Distance - 类间距离17)Cluster Cohesion - 类内连贯性18)Cluster Separation - 类间分离度19)Cluster Assignment - 聚类分配20)Cluster Label - 聚类标签•K-Means - K-均值1)K-Means - K-均值2)Centroid - 质心3)Cluster - 聚类4)Cluster Center - 聚类中心5)Cluster Assignment - 聚类分配6)Cluster Analysis - 聚类分析7)K Value - K值8)Elbow Method - 肘部法则9)Inertia - 惯性10)Silhouette Score - 轮廓系数11)Convergence - 收敛12)Initialization - 初始化13)Euclidean Distance - 欧氏距离14)Manhattan Distance - 曼哈顿距离15)Distance Metric - 距离度量16)Cluster Radius - 聚类半径17)Within-Cluster Variation - 类内变异18)Cluster Quality - 聚类质量19)Clustering Algorithm - 聚类算法20)Clustering Validation - 聚类验证•Dimensionality Reduction - 降维1)Dimensionality Reduction - 降维2)Feature Extraction - 特征提取3)Feature Selection - 特征选择4)Principal Component Analysis (PCA) - 主成分分析5)Singular Value Decomposition (SVD) - 奇异值分解6)Linear Discriminant Analysis (LDA) - 线性判别分析7)t-Distributed Stochastic Neighbor Embedding (t-SNE) - t-分布随机邻域嵌入8)Autoencoder - 自编码器9)Manifold Learning - 流形学习10)Locally Linear Embedding (LLE) - 局部线性嵌入11)Isomap - 等度量映射12)Uniform Manifold Approximation and Projection (UMAP) - 均匀流形逼近与投影13)Kernel PCA - 核主成分分析14)Non-negative Matrix Factorization (NMF) - 非负矩阵分解15)Independent Component Analysis (ICA) - 独立成分分析16)Variational Autoencoder (VAE) - 变分自编码器17)Sparse Coding - 稀疏编码18)Random Projection - 随机投影19)Neighborhood Preserving Embedding (NPE) - 保持邻域结构的嵌入20)Curvilinear Component Analysis (CCA) - 曲线成分分析•Principal Component Analysis (PCA) - 主成分分析1)Principal Component Analysis (PCA) - 主成分分析2)Eigenvector - 特征向量3)Eigenvalue - 特征值4)Covariance Matrix - 协方差矩阵。
TDSQL核心架构
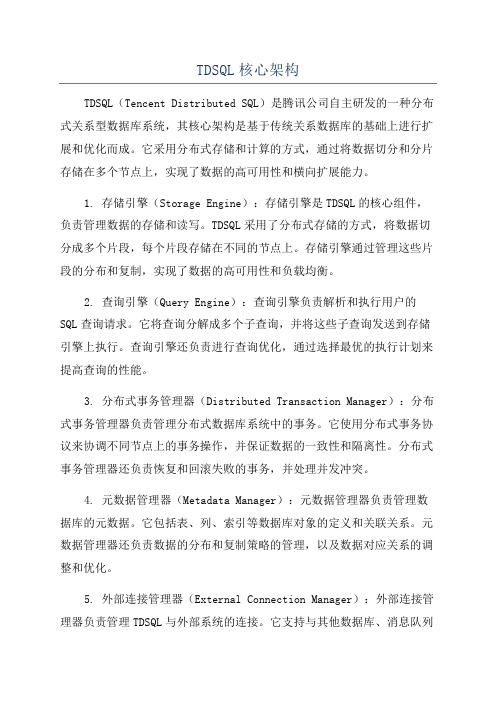
TDSQL核心架构TDSQL(Tencent Distributed SQL)是腾讯公司自主研发的一种分布式关系型数据库系统,其核心架构是基于传统关系数据库的基础上进行扩展和优化而成。
它采用分布式存储和计算的方式,通过将数据切分和分片存储在多个节点上,实现了数据的高可用性和横向扩展能力。
1. 存储引擎(Storage Engine):存储引擎是TDSQL的核心组件,负责管理数据的存储和读写。
TDSQL采用了分布式存储的方式,将数据切分成多个片段,每个片段存储在不同的节点上。
存储引擎通过管理这些片段的分布和复制,实现了数据的高可用性和负载均衡。
2. 查询引擎(Query Engine):查询引擎负责解析和执行用户的SQL查询请求。
它将查询分解成多个子查询,并将这些子查询发送到存储引擎上执行。
查询引擎还负责进行查询优化,通过选择最优的执行计划来提高查询的性能。
3. 分布式事务管理器(Distributed Transaction Manager):分布式事务管理器负责管理分布式数据库系统中的事务。
它使用分布式事务协议来协调不同节点上的事务操作,并保证数据的一致性和隔离性。
分布式事务管理器还负责恢复和回滚失败的事务,并处理并发冲突。
4. 元数据管理器(Metadata Manager):元数据管理器负责管理数据库的元数据。
它包括表、列、索引等数据库对象的定义和关联关系。
元数据管理器还负责数据的分布和复制策略的管理,以及数据对应关系的调整和优化。
5. 外部连接管理器(External Connection Manager):外部连接管理器负责管理TDSQL与外部系统的连接。
它支持与其他数据库、消息队列等系统的数据交互,并提供数据同步和数据迁移的功能。
外部连接管理器还支持分布式事务和跨节点查询的功能。
总之,TDSQL的核心架构是基于传统关系数据库的基础上扩展和优化而成的分布式关系数据库系统。
通过将数据切分和分片存储在多个节点上,以及采用分布式事务管理和查询优化的技术,实现了数据的高可用性和横向扩展能力。
PMS_overview_chs_REVISED_pics
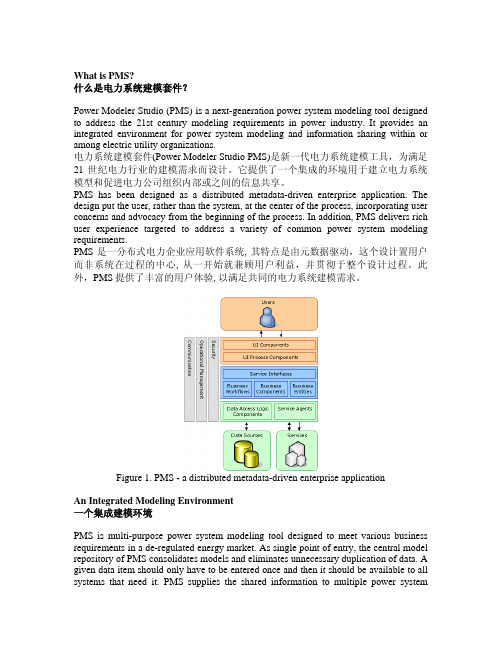
What is PMS?什么是电力系统建模套件?Power Modeler Studio (PMS) is a next-generation power system modeling tool designed to address the 21st century modeling requirements in power industry. It provides an integrated environment for power system modeling and information sharing within or among electric utility organizations.电力系统建模套件(Power Modeler Studio PMS)是新一代电力系统建模工具,为满足21世纪电力行业的建模需求而设计。
它提供了一个集成的环境用于建立电力系统模型和促进电力公司组织内部或之间的信息共享。
PMS has been designed as a distributed metadata-driven enterprise application. The design put the user, rather than the system, at the center of the process, incorporating user concerns and advocacy from the beginning of the process. In addition, PMS delivers rich user experience targeted to address a variety of common power system modeling requirements.PMS是一分布式电力企业应用软件系统, 其特点是由元数据驱动,这个设计置用户而非系统在过程的中心, 从一开始就兼顾用户利益,并贯彻于整个设计过程。
CLOUD STORAGE SYSTEM WITH DISTRIBUTED METADATA
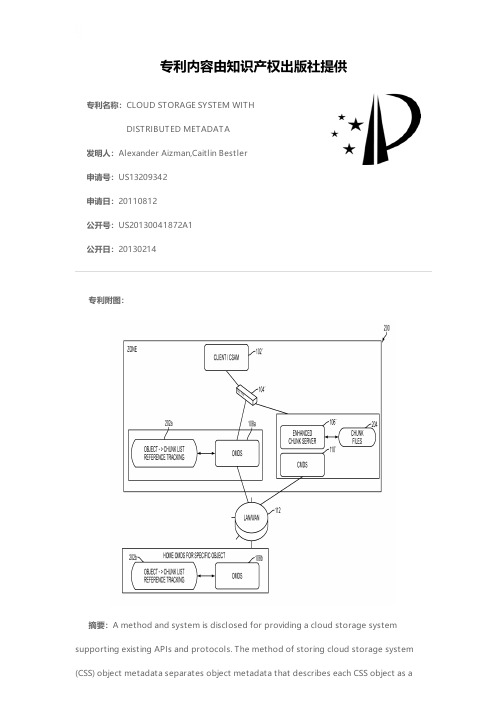
专利名称:CLOUD STORAGE SYSTEM WITHDISTRIBUTED METADATA发明人:Alexander Aizman,Caitlin Bestler申请号:US13209342申请日:20110812公开号:US20130041872A1公开日:20130214专利内容由知识产权出版社提供专利附图:摘要:A method and system is disclosed for providing a cloud storage systemsupporting existing APIs and protocols. The method of storing cloud storage system (CSS) object metadata separates object metadata that describes each CSS object as acollection of named chunks with chunk locations specified as a separate part of the metadata. Chunks are identified using globally unique permanent identifiers that are never re-used to identify different chunk payload. While avoiding the bottleneck of a single metadata server, the disclosed system provides ordering guarantees to clients such as guaranteeing access to the most recent version of an object. The disclosed system also provides end-to-end data integrity protection, inline data deduplication, configurable replication, hierarchical storage management and location-aware optimization of chunk storage.申请人:Alexander Aizman,Caitlin Bestler地址:Mountain View CA US,Sunnyvale CA US国籍:US,US更多信息请下载全文后查看。
tidb 基础概念
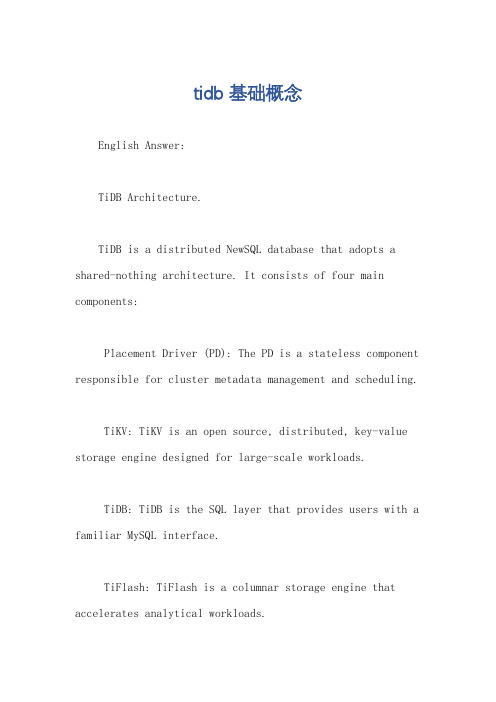
tidb 基础概念English Answer:TiDB Architecture.TiDB is a distributed NewSQL database that adopts a shared-nothing architecture. It consists of four main components:Placement Driver (PD): The PD is a stateless component responsible for cluster metadata management and scheduling.TiKV: TiKV is an open source, distributed, key-value storage engine designed for large-scale workloads.TiDB: TiDB is the SQL layer that provides users with a familiar MySQL interface.TiFlash: TiFlash is a columnar storage engine that accelerates analytical workloads.TiDB Features.TiDB offers several key features that make it suitable for various applications:High performance: TiDB can handle high-throughput workloads with low latency, making it suitable for mission-critical systems.Scalability: TiDB can be scaled horizontally to meet growing data and workload demands, allowing businesses to handle spikes in traffic.High availability: TiDB ensures data availability and reliability by replicating data across multiple nodes.SQL compatibility: TiDB supports the MySQL protocol, allowing developers to use familiar tools and applications.Cloud-native: TiDB is designed to run in cloud environments, making it easy to deploy and manage.TiDB Use Cases.TiDB is widely used in various industries and applications, including:E-commerce: Handling high-volume transactions and customer data for online shopping platforms.Finance: Managing financial data, processing transactions, and performing analytics.Healthcare: Storing and analyzing medical records, providing real-time insights for patient care.Government: Handling large-scale data, providing citizen services, and conducting performance monitoring.Media: Processing large amounts of content, including images, videos, and social media data.中文回答:TiDB 的基础概念。
- 1、下载文档前请自行甄别文档内容的完整性,平台不提供额外的编辑、内容补充、找答案等附加服务。
- 2、"仅部分预览"的文档,不可在线预览部分如存在完整性等问题,可反馈申请退款(可完整预览的文档不适用该条件!)。
- 3、如文档侵犯您的权益,请联系客服反馈,我们会尽快为您处理(人工客服工作时间:9:00-18:30)。
Abstract
Metadata management is critical to distributed file system. Many large-scale storage systems such as HDFS involve the decoupling of metadata management from file data access. In such architecture, a single master server manages all metadata, while a number of data servers store file data. This architecture can't meet the exponentially increased storage demand in cloud computing, as the single master server may become a performance bottleneck. This paper presents a metadata management scheme based on HDFS for cloud computing. Our scheme employs multiple Namenodes, and divides the metadata into "buckets" which can be dynamically migrated among Namenodes according to system workloads. To maintain reliability, metadata is replicated in different Namenodes with log replication technology, and Paxos algorithm is adopted ቤተ መጻሕፍቲ ባይዱo keep replication consistency. Besides, caching and prefetching technology is integrated to improve system performance.
operations [1]. So the metadata management is critically important for file system performance. There have been many distributed storage systems, and most involve the decoupling of metadata management from data access, such as GFS[2] and HDFS[3]. The Hadoop Distributed File System (HDFS) is an open source distributed file system with master/slave architecture. A file is split into one or more blocks and these blocks are stored in a set of DataNodes. A single Namenode manages file system namespace, determines the mapping of file to blocks, and regulates access to files. In HDFS, all metadata is kept in the memory of the single Namenode, so it may become performance bottleneck as metadata number increases. So we researched HDFS, changed the single Namenode architecture to multiple Namenodes, and proposed a metadata management scheme that fulfills the following goals: (1) High performance: We distribute metadata among multiple Namenodes, and provide an efficient route for metadata requests to appropriate Namenodes. Caching and prefetching strategy is also adopted to improve system performance. (2) Well balanced load: We adopt a variant of consistent hashing to assign metadata among multiple Namenodes according to each Namenode's processing ability, and redistribute metadata when system workloads change. (3) Reliability: To guarantee system fault-tolerance, we propose a group-based log replication technology, and integrate Paxos algorithm to maintain replication consistency under the occurrence of failures. (4) Scalability: A Namenode can be added to or removed from the cluster without system restarting, and the metadata can be redistributed to keep load balance. The rest of the paper is organized as follows: We begin by discussing related work in Section 2. In Section 3, we present the architecture of our metadata management system, and discuss some detailed design and optimization issues. Performance test based on a prototype implementation are given in section 4. Finally, we conclude our paper in section 5.
978-1-4577-0208-2/11/$26.00 ©2011 IEEE
32
2. Related Work
Currently, Sub Tree Partitioning [4] [5] and Hashing [6] [7] [8] are two common techniques to distribute metadata among mUltiple servers. In sub tree partitioning, namespace is divided into many directory sub trees, each of which is managed by individual metadata servers. This strategy provides a good locality because metadata in the same sub tree is assigned to the same metadata server, but metadata may not be evenly distributed, and the computing and transferring of metadata may generate a high time and network overhead. Hashing technique uses a hash function on the path name to get metadata location. In this scheme, metadata can be distributed uniformly among cluster, but the directory locality feature is lost, and if the path is renamed, some metadata have to migrate. Hierarchical Bloom Filter Arrays (HBA) [9] is a special hash strategy that uses Bloom Filter to map metadata to metadata servers. We can check whether a metadata is in a server by hashing the path into positions in Bloom Filter and checking the values of those positions. HBA is fast and space-efficient, but each server has to keep as many Bloom filters as possible in order to maintain all metadata hash results, so a large memory is consumed. Consistent hashing [10] is another hash method used in Amazon Dynamo [11]. In basic consistent hashing, the output range of the hash function is treated as a ring. Not only the data is hashed, but also each node is hashed to a value in the ring. Each node is responsible for the data in the range between it and its predecessor node. In consistent hashing, the addition and removal of a node only affects its neighbor nodes. An optimization of consistent hashing is the introduction of "virtual node". Instead of mapping a physical node to a single point in the ring, each physical node is assigned to multiple positions, each of which is called a virtual node. With virtual node, data and workload is distributed over nodes more uniformly.