[2014 Data Science and Advanced Analytics (DSAA)] SAR target recognition based on deep learning
荷兰的科学体系
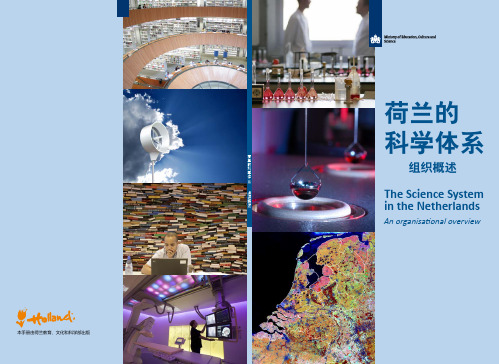
目 录1、手册介绍和内容32、科技政策要点63、科学体系的组织104、科学体系的各级组织144.1 政治和政府144.2 咨询机构154.3 科研经费的赞助机构164.4 中间组织和“临时工作组”194.5 科研的执行机构214.6 其他(支持)机构23资料表格1:部委25资料表格2:荷兰的大学30资料表格3:荷兰科学研究组织(NWO)及其机构33资料表格4:荷兰皇家科学院(KNAW)及其机构37资料表格5:荷兰应用科学研究组织 (TNO) 41资料表格6:大型技术研究院43资料表格7:农业研究院(DLO)44资料表格8:其他机构和研究院46资料表格9:关键数字48网站49缩略词50荷兰教育、文化和科学部科研政策局版本:2012年10月更多此资料的获取处:Beijing@1荷兰卫星图片图片来源: WaterWatch21、手册介绍和内容出版本手册的主要目的是帮助读者全面了解荷兰的科学体系。
手册的第2节主要介绍荷兰现行科技政策的要点; 第3节概述了荷兰科学体系的组织方式。
第4节是对第三节内容的更详细说明。
手册的最后部分列出了多个表格,表格中包含荷兰的科研项目资助方和科研执行机构的详细信息,一些关键数字、相关的荷兰网站以及一张缩略词列表。
34荷兰科学:世界一流国家荷兰科学已跻身于世界一流水平。
这一点从每位科研人员的研究成果、其成果被引用频率以及荷兰人参与科研项目的数量可见一斑。
为了保持荷兰科技的世界领先地位,荷兰政府对有才能的科研人员采取激励措施,由荷兰教育、文化和科学部科研政策处(OWB )负责提供资金和实际支持。
所有人才都应受到重视,尤其是现在荷兰已经到了与其他发达国家拼“智力之战”的最后阶段。
2010年荷兰在研发上的支出超过110亿欧元,参与研发的人员达到近10万人。
荷兰的科学研究资金荷兰的科学研究经费来自不同的渠道:其中45%由企业提供, 政府提供的占40%; 还有11%的科研经费来自国外; 其余的4%(特别是医药行业的研发经费)来自有研究经费的机构、外国公司以及欧盟提供的个人科研补助。
电脑能帮助干什么英语作文
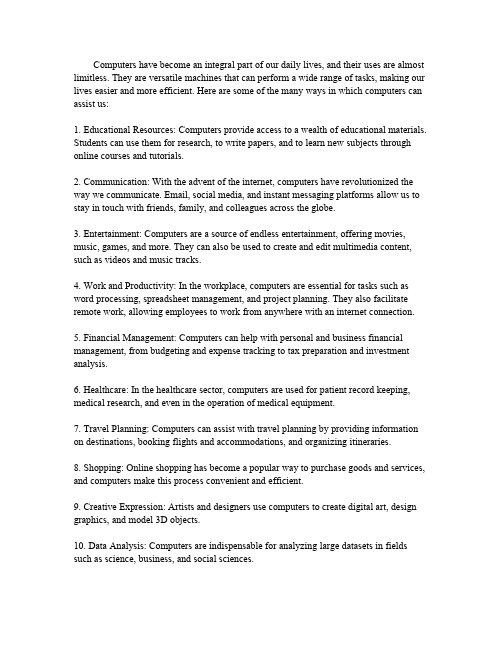
Computers have become an integral part of our daily lives,and their uses are almost limitless.They are versatile machines that can perform a wide range of tasks,making our lives easier and more efficient.Here are some of the many ways in which computers can assist us:cational Resources:Computers provide access to a wealth of educational materials. Students can use them for research,to write papers,and to learn new subjects through online courses and tutorials.munication:With the advent of the internet,computers have revolutionized the way we communicate.Email,social media,and instant messaging platforms allow us to stay in touch with friends,family,and colleagues across the globe.3.Entertainment:Computers are a source of endless entertainment,offering movies, music,games,and more.They can also be used to create and edit multimedia content, such as videos and music tracks.4.Work and Productivity:In the workplace,computers are essential for tasks such as word processing,spreadsheet management,and project planning.They also facilitate remote work,allowing employees to work from anywhere with an internet connection.5.Financial Management:Computers can help with personal and business financial management,from budgeting and expense tracking to tax preparation and investment analysis.6.Healthcare:In the healthcare sector,computers are used for patient record keeping, medical research,and even in the operation of medical equipment.7.Travel Planning:Computers can assist with travel planning by providing information on destinations,booking flights and accommodations,and organizing itineraries.8.Shopping:Online shopping has become a popular way to purchase goods and services, and computers make this process convenient and efficient.9.Creative Expression:Artists and designers use computers to create digital art,design graphics,and model3D objects.10.Data Analysis:Computers are indispensable for analyzing large datasets in fields such as science,business,and social sciences.11.Gaming:The gaming industry has exploded with the help of computers,offering immersive experiences and interactive entertainment.12.Home Automation:Computers can control home systems,such as lighting,heating, and security,making homes more comfortable and secure.nguage Learning:Language learning software and online resources can help individuals learn new languages at their own pace.14.News and Information:Computers provide instant access to news and current events from around the world,keeping us informed and uptodate.15.Legal Services:Lawyers and legal professionals use computers for case management, legal research,and document preparation.In conclusion,the computer is a powerful tool that can be harnessed for a multitude of purposes,from enhancing our personal lives to supporting complex professional tasks.As technology continues to advance,the capabilities of computers will only expand,offering even more ways to assist us in our daily endeavors.。
电脑的用途 英语作文
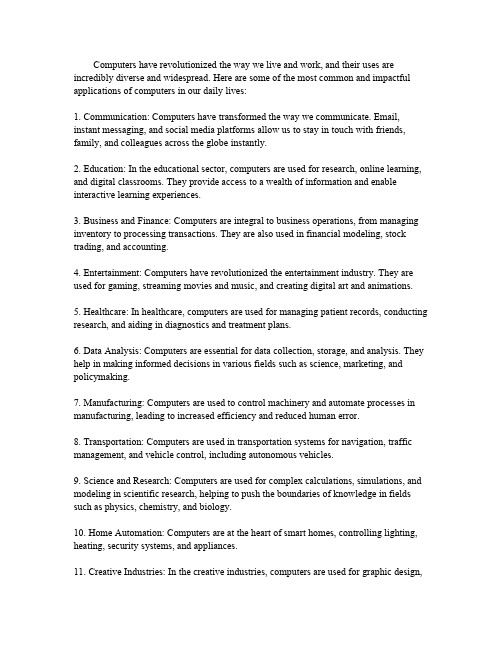
Computers have revolutionized the way we live and work,and their uses are incredibly diverse and widespread.Here are some of the most common and impactful applications of computers in our daily lives:munication:Computers have transformed the way we communicate.Email, instant messaging,and social media platforms allow us to stay in touch with friends, family,and colleagues across the globe instantly.cation:In the educational sector,computers are used for research,online learning, and digital classrooms.They provide access to a wealth of information and enable interactive learning experiences.3.Business and Finance:Computers are integral to business operations,from managing inventory to processing transactions.They are also used in financial modeling,stock trading,and accounting.4.Entertainment:Computers have revolutionized the entertainment industry.They are used for gaming,streaming movies and music,and creating digital art and animations.5.Healthcare:In healthcare,computers are used for managing patient records,conducting research,and aiding in diagnostics and treatment plans.6.Data Analysis:Computers are essential for data collection,storage,and analysis.They help in making informed decisions in various fields such as science,marketing,and policymaking.7.Manufacturing:Computers are used to control machinery and automate processes in manufacturing,leading to increased efficiency and reduced human error.8.Transportation:Computers are used in transportation systems for navigation,traffic management,and vehicle control,including autonomous vehicles.9.Science and Research:Computers are used for complex calculations,simulations,and modeling in scientific research,helping to push the boundaries of knowledge in fields such as physics,chemistry,and biology.10.Home Automation:Computers are at the heart of smart homes,controlling lighting, heating,security systems,and appliances.11.Creative Industries:In the creative industries,computers are used for graphic design,music production,film editing,and3D modeling.12.Ecommerce:Computers have enabled the growth of online shopping,making it easier for consumers to purchase goods and services from anywhere.ernment and Public Services:Governments use computers for managing public records,providing services to citizens,and ensuring national security.14.Agriculture:Computers are used in precision farming to monitor crop health, optimize irrigation,and increase yield.15.Space Exploration:In space exploration,computers are used to control spacecraft, analyze data from space missions,and simulate space environments.In conclusion,the versatility of computers is astounding,and their influence on modern society is profound.As technology continues to advance,the applications of computers are likely to expand even further,offering new opportunities and challenges for the future.。
拉曼光谱数据库及信息查询系统
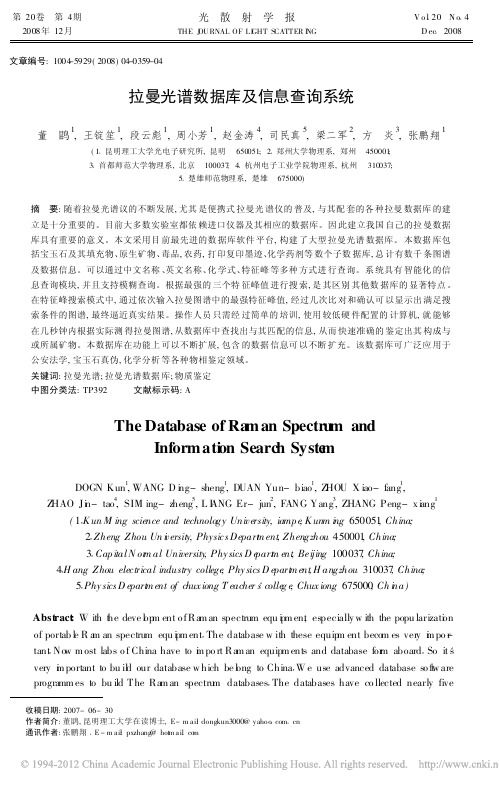
第20卷第4期光散射学报V o l120N o14 2008年12月THE J OURNAL OF LI GHT SCATTER I NG D ec12008文章编号:1004-5929(2008)04-0359-04拉曼光谱数据库及信息查询系统董鹍1,王锭笙1,段云彪1,周小芳1,赵金涛4,司民真5,梁二军2,方炎3,张鹏翔1 (11昆明理工大学光电子研究所,昆明650051;21郑州大学物理系,郑州450001;31首都师范大学物理系,北京100037;41杭州电子工业学院物理系,杭州310037;51楚雄师范物理系,楚雄675000)摘要:随着拉曼光谱议的不断发展,尤其是便携式拉曼光谱仪的普及,与其配套的各种拉曼数据库的建立是十分重要的。
目前大多数实验室都依赖进口仪器及其相应的数据库。
因此建立我国自己的拉曼数据库具有重要的意义。
本文采用目前最先进的数据库软件平台,构建了大型拉曼光谱数据库。
本数据库包括宝玉石及其填充物、原生矿物、毒品,农药,打印复印墨迹、化学药剂等数个子数据库,总计有数千条图谱及数据信息。
可以通过中文名称、英文名称、化学式、特征峰等多种方式进行查询。
系统具有智能化的信息查询模块,并且支持模糊查询。
根据最强的三个特征峰值进行搜索,是其区别其他数据库的显著特点。
在特征峰搜索模式中,通过依次输入拉曼图谱中的最强特征峰值,经过几次比对和确认可以显示出满足搜索条件的图谱,最终逼近真实结果。
操作人员只需经过简单的培训,使用较低硬件配置的计算机,就能够在几秒钟内根据实际测得拉曼图谱,从数据库中查找出与其匹配的信息,从而快速准确的鉴定出其构成与或所属矿物。
本数据库在功能上可以不断扩展,包含的数据信息可以不断扩充。
该数据库可广泛应用于公安法学,宝玉石真伪,化学分析等各种物相鉴定领域。
关键词:拉曼光谱;拉曼光谱数据库;物质鉴定中图分类法:TP392文献标示码:AThe Database of Ra m an Spectr u m andInform ati on Searc h Syste mDOGN Kun1,WANG D i n g-sheng1,DUAN Yun-biao1,Z HOU X iao-fang1, Z HAO Ji n-tao4,SIM ing-zheng5,L I A NG Er-jun2,FANG Yang3,ZHANG Peng-x i a ng1 (11K un M ing science and technolo gy University,iamp e,K unm i n g650051,China;21Zheng Zhou Un i v ersit y,Physics Depart m ent,Zhengzhou450001,China;31Cap italN or m al University,Phy sics D e part m ent,Beijing100037,China;41H ang Zhou electrical industry college,Phy sics D epart m ent,H angzhou310037,China;51Phy sics D epart m ent of chux iong T eacher's colle g e,Chux iong675000,Ch i n a)Abst ract:W ith t h e deve l o p m ent o fRa m an spectrum equ i p m en,t especially w ith the popu larizati o n of portab l e R a m an spectrum equ i p m ent1The database w ith these equip m ent beco m es ver y i m po r-tant1No w m ost labs o f China have to i m po rt Ra m an equipm ents and database for m aboard1So it s' very i m portant to bu il d our database w hich be l o ng to China1W e use advanced database so ft w are progra mm es to bu ild The Ra m an spectru m databases1The databases have co llected nearly five收稿日期:2007-06-30作者简介:董鹍,昆明理工大学在读博士,E-m ai:l dongkun3000@yahoo1com1cn通讯作者:张鹏翔1E-m ai:l pxzhang@hot m ai.l co m光散射学报第20卷hundreds species o f ge m s,m inera ls and filler,dr ugs,pesticide and so on1It inc l u des t h ousands of different Ra m an spectrum and re l a ted i n for m ation1Several index m ethods such as t h e chinese na m e,eng lish na m e,che m ical for m ula and o t h er key wo r ds w ere used for searching,co mpari n g and i d entify ing of the m easured or unkno w n iter m s1It has inte lligent pr ogra m to support f u zzy search。
Sample of reported job titles Statistician, Biost
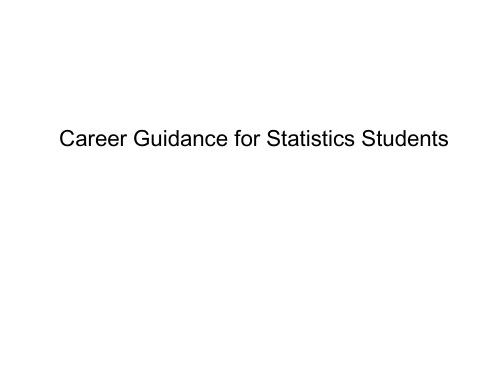
• Identify relationships and trends in data, as well as any factors that could affect the results of research.
• Analyze and interpret statistical data in order to identify significant differences in relationships among sources of information.
• Written Comprehension — The ability to read and understand information and ideas presented in writing.
Work Activities
• Analyzing Data or Information — Identifying the underlying principles, reasons, or facts of information by breaking down information or data into separate parts.
LetPub-JCR2014-SCI IF
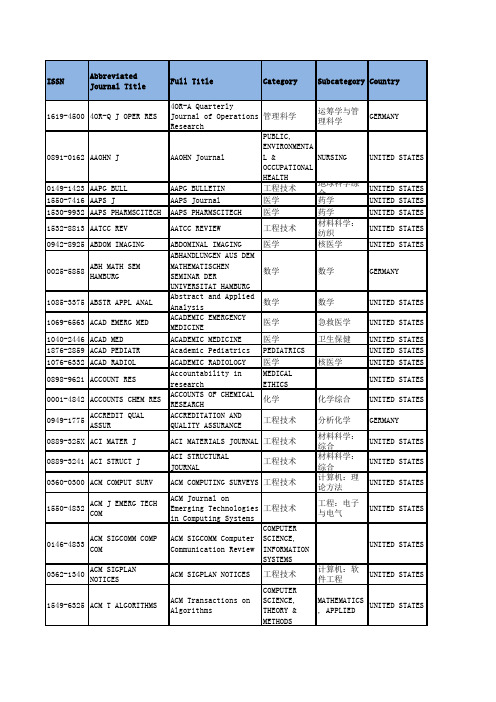
ISSN AbbreviatedJournal TitleFull Title Category Subcategory Country1619-45004OR-Q J OPER RES4OR-A Quarterly Journal 管理科学运筹学与管理科GERMANY0891-0162AAOHN J AAOHN Journal PUBLIC, ENVIRON NURSING UNITED STATES 0149-1423AAPG BULL AAPG BULLETIN工程技术地球科学综合UNITED STATES 1550-7416AAPS J AAPS Journal医学药学UNITED STATES 1530-9932AAPS PHARMSCITECH AAPS PHARMSCITECH医学药学UNITED STATES 1532-8813AATCC REV AATCC REVIEW工程技术材料科学:纺织UNITED STATES 0942-8925ABDOM IMAGING ABDOMINAL IMAGING医学核医学UNITED STATES 0025-5858ABH MATH SEM HAMBURG A BHANDLUNGEN AUS DEM MAT数学数学GERMANY1085-3375ABSTR APPL ANAL Abstract and Applied Ana数学数学UNITED STATES 1069-6563ACAD EMERG MED ACADEMIC EMERGENCY MEDIC医学急救医学UNITED STATES 1040-2446ACAD MED ACADEMIC MEDICINE医学卫生保健UNITED STATES 1876-2859ACAD PEDIATR Academic Pediatrics PEDIATRICS UNITED STATES 1076-6332ACAD RADIOL ACADEMIC RADIOLOGY医学核医学UNITED STATES 0898-9621ACCOUNT RES Accountability in resear MEDICAL ETHICS UNITED STATES 0001-4842ACCOUNTS CHEM RES ACCOUNTS OF CHEMICAL RES化学化学综合UNITED STATES 0949-1775ACCREDIT QUAL ASSURACCREDITATION AND QUALIT工程技术分析化学GERMANY0889-325X ACI MATER J ACI MATERIALS JOURNAL工程技术材料科学:综合UNITED STATES 0889-3241ACI STRUCT J ACI STRUCTURAL JOURNAL工程技术材料科学:综合UNITED STATES 0360-0300ACM COMPUT SURV ACM COMPUTING SURVEYS工程技术计算机:理论方UNITED STATES 1550-4832ACM J EMERG TECH COM A CM Journal on Emerging 工程技术工程:电子与电UNITED STATES 0146-4833ACM SIGCOMM COMP COM A CM SIGCOMM Computer Com COMPUTER SCIENCE, INFORMATI UNITED STATES 0362-1340ACM SIGPLAN NOTICESACM SIGPLAN NOTICES工程技术计算机:软件工UNITED STATES 1549-6325ACM T ALGORITHMS ACM Transactions on Algo COMPUTER SCIENC MATHEMATICS, A UNITED STATES 1544-3558ACM T APPL PERCEPT ACM Transactions on Appl工程技术计算机:软件工UNITED STATES 1544-3566ACM T ARCHIT CODE OP A CM Transactions on Arch工程技术计算机:理论方UNITED STATES 1556-4665ACM T AUTON ADAP SYS A CM Transactions on Auto工程技术计算机:理论方UNITED STATES 1529-3785ACM T COMPUT LOG ACM Transactions on Comp工程技术计算机:理论方UNITED STATES 0734-2071ACM T COMPUT SYST ACM TRANSACTIONS ON COMP工程技术计算机:理论方UNITED STATES 1073-0516ACM T COMPUT-HUM INT A CM Transactions on Comp工程技术计算机:控制论UNITED STATES 0362-5915ACM T DATABASE SYSTACM TRANSACTIONS ON DATA工程技术计算机:软件工UNITED STATES 1084-4309ACM T DES AUTOMAT EL A CM TRANSACTIONS ON DESI工程技术计算机:软件工UNITED STATES 1539-9087ACM T EMBED COMPUT S A CM Transactions on Embe工程技术计算机:软件工UNITED STATES 0730-0301ACM T GRAPHIC ACM TRANSACTIONS ON GRAP工程技术计算机:软件工UNITED STATES 1046-8188ACM T INFORM SYST ACM TRANSACTIONS ON INFO工程技术计算机:信息系UNITED STATES 1094-9224ACM T INFORM SYST SE A CM Transactions on Info工程技术计算机:信息系UNITED STATES 2157-6904ACM T INTEL SYST TEC A CM Transactions on Inte COMPUTER SCIENC COMPUTER SCIEN UNITED STATES 1533-5399ACM T INTERNET TECHN A CM Transactions on Inte工程技术计算机:软件工UNITED STATES 1556-4681ACM T KNOWL DISCOV D A CM Transactions on Know COMPUTER SCIENC COMPUTER SCIEN UNITED STATES 0098-3500ACM T MATH SOFTWAREACM TRANSACTIONS ON MATH工程技术计算机:软件工UNITED STATES 1049-3301ACM T MODEL COMPUT S A CM Transactions on Mode工程技术计算机:跨学科UNITED STATES 1551-6857ACM T MULTIM COMPUTACM Transactions on Mult工程技术计算机:理论方UNITED STATES 0164-0925ACM T PROGR LANG SYS A CM TRANSACTIONS ON PROG工程技术计算机:软件工UNITED STATES 1936-7406ACM T RECONFIG TECHN A CM transactions on reco COMPUTER SCIENCE, HARDWARE UNITED STATES 1550-4859ACM T SENSOR NETWORK A CM Transactions on Sens工程技术电信学UNITED STATES 1049-331X ACM T SOFTW ENG METH A CM TRANSACTIONS ON SOFT工程技术计算机:软件工UNITED STATES1553-3077ACM T STORAGE ACM transactions on stor COMPUTER SCIENC COMPUTER SCIEN UNITED STATES 1559-1131ACM T WEB ACM Transactions on the 工程技术计算机:软件工UNITED STATES 0814-6039ACOUST AUST Acoustics Australia物理声学AUSTRALIA1063-7710ACOUST PHYS+ACOUSTICAL PHYSICS物理声学RUSSIA1944-8244ACS APPL MATER INTER A CS Applied Materials & 工程技术材料科学:综合UNITED STATES 2155-5435ACS CATAL ACS catalysis CHEMISTRY, PHYSICAL UNITED STATES 1554-8929ACS CHEM BIOL ACS Chemical Biology生物生化与分子生物UNITED STATES 1948-7193ACS CHEM NEUROSCI ACS Chemical Neuroscienc BIOCHEMISTRY & CHEMISTRY, MED UNITED STATES 2156-8952ACS COMB SCI ACS Combinatorial Scienc CHEMISTRY, APPL CHEMISTRY, MED UNITED STATES 2161-1653ACS MACRO LETT ACS Macro Letters化学UNITED STATES 1948-5875ACS MED CHEM LETT ACS Medicinal Chemistry CHEMISTRY, MEDICINAL UNITED STATES 1936-0851ACS NANO ACS Nano工程技术材料科学:综合UNITED STATES 2168-0485ACS SUSTAIN CHEM ENG A CS Sustainable Chemistr CHEMISTRY, MULT ENGINEERING, C UNITED STATES 2161-5063ACS SYNTH BIOL ACS Synthetic Biology生物UNITED STATES 1091-5397ACSMS HEALTH FIT J ACSMS HEALTH & FITNESS J医学运动科学UNITED STATES 1610-1928ACTA ACUST UNITED AC A CTA ACUSTICA UNITED WIT物理声学GERMANY0001-5113ACTA ADRIAT ACTA ADRIATICA生物海洋与淡水生物CROATIA0906-4702ACTA AGR SCAND A-ANACTA AGRICULTURAE SCANDI农林科学奶制品与动物科ENGLAND0906-4710ACTA AGR SCAND B-S P A CTA AGRICULTURAE SCANDI农林科学农艺学NORWAY0139-3006ACTA ALIMENT HUNG ACTA ALIMENTARIA农林科学食品科技HUNGARY0001-5172ACTA ANAESTH SCAND ACTA ANAESTHESIOLOGICA S医学麻醉学DENMARK0167-8019ACTA APPL MATH ACTA APPLICANDAE MATHEMA数学应用数学NETHERLANDS 0065-1036ACTA ARITH ACTA ARITHMETICA数学数学POLAND0094-5765ACTA ASTRONAUT ACTA ASTRONAUTICA工程技术工程:宇航UNITED STATES 0001-5237ACTA ASTRONOM ACTA ASTRONOMICA地学天文天文与天体物理POLAND1672-9145ACTA BIOCH BIOPH SIN A CTA BIOCHIMICA ET BIOPH生物生化与分子生物PEOPLES R CHINA 0001-527X ACTA BIOCHIM POL ACTA BIOCHIMICA POLONICA生物生化与分子生物POLAND1509-409X ACTA BIOENG BIOMECHActa of Bioengineering a BIOPHYSICS ENGINEERING, B POLAND1726-569X ACTA BIOETH Acta Bioethica医学卫生政策Chile0001-5296ACTA BIOL CRACOV BOT A CTA BIOLOGICA CRACOVIEN生物植物科学POLAND0236-5383ACTA BIOL HUNG ACTA BIOLOGICA HUNGARICA生物生物学HUNGARY1742-7061ACTA BIOMATER Acta Biomaterialia工程技术材料科学:生物ENGLAND0001-5342ACTA BIOTHEOR ACTA BIOTHEORETICA生物生物学NETHERLANDS 0102-3306ACTA BOT BRAS Acta Botanica Brasilica P LANT SCIENCES BRAZIL0365-0588ACTA BOT CROAT Acta Botanica CroaticaPLANT SCIENCES CROATIA1253-8078ACTA BOT GALLICA ACTA BOTANICA GALLICA生物植物科学FRANCE0187-7151ACTA BOT MEX Acta Botanica Mexicana生物植物科学MEXICO0001-5385ACTA CARDIOL ACTA CARDIOLOGICA医学心血管系统BELGIUM1011-6842ACTA CARDIOL SIN Acta Cardiologica Sinica医学心血管系统TAIWAN0583-6050ACTA CARSOLOGICA ACTA CARSOLOGICA地学地球科学综合SLOVENIA0567-7351ACTA CHIM SINICA ACTA CHIMICA SINICA化学化学综合PEOPLES R CHINA 1318-0207ACTA CHIM SLOV ACTA CHIMICA SLOVENICA化学化学综合SLOVENIA0001-5458ACTA CHIR BELG ACTA CHIRURGICA BELGICA医学外科BELGIUM0001-5415ACTA CHIR ORTHOP TRACTA CHIRURGIAE ORTHOPAE ORTHOPEDICS CZECH REPUBLIC 1508-1109ACTA CHIROPTEROL ACTA CHIROPTEROLOGICA生物动物学POLAND1233-2356ACTA CHROMATOGR ACTA CHROMATOGRAPHICA化学分析化学POLAND0102-8650ACTA CIR BRAS Acta Cirurgica Brasileir医学外科BRAZIL0353-9466ACTA CLIN CROAT Acta Clinica Croatica医学医学:内科CROATIA0108-7673ACTA CRYSTALLOGR A ACTA CRYSTALLOGRAPHICA S化学晶体学DENMARK0108-7681ACTA CRYSTALLOGR B ACTA CRYSTALLOGRAPHICA S化学晶体学DENMARK0108-2701ACTA CRYSTALLOGR C ACTA CRYSTALLOGRAPHICA S化学晶体学DENMARK0907-4449ACTA CRYSTALLOGR D ACTA CRYSTALLOGRAPHICA S生物晶体学DENMARK1744-3091ACTA CRYSTALLOGR F Acta Crystallographica S生物晶体学DENMARK0001-5547ACTA CYTOL ACTA CYTOLOGICA生物病理学UNITED STATES 1330-027X ACTA DERMATOVENER CR A cta Dermatovenerologica医学皮肤病学CROATIA0001-5555ACTA DERM-VENEREOL ACTA DERMATO-VENEREOLOGI医学皮肤病学NORWAY0940-5429ACTA DIABETOL ACTA DIABETOLOGICA医学内分泌学与代谢ITALY1841-0987ACTA ENDOCRINOL-BUCH A cta Endocrinologica-Buc医学内分泌学与代谢ROMANIA0374-1036ACTA ENT MUS NAT PRA A cta Entomologica Musei ENTOMOLOGY CZECH REPUBLIC 0873-9749ACTA ETHOL ACTA ETHOLOGICA生物动物学GERMANY2213-5812ACTA GEOD GEOPHYS Acta Geodaetica et Geoph GEOCHEMISTRY & GEOPHYSICS HUNGARY1214-9705ACTA GEODYN GEOMATER A cta Geodynamica et Geom地学地球化学与地球CZECH REPUBLIC 1581-6613ACTA GEOGR SLOV Acta Geographica Sloveni地学地质学SLOVENIA0001-5709ACTA GEOL POL ACTA GEOLOGICA POLONICA地学地质学POLAND1000-9515ACTA GEOL SIN-ENGL ACTA GEOLOGICA SINICA-EN地学地球科学综合PEOPLES R CHINA 1895-6572ACTA GEOPHYS Acta Geophysica地学地球化学与地球POLAND1861-1125ACTA GEOTECH Acta Geotechnica ENGINEERING, GEOLOGICAL GERMANY1854-0171ACTA GEOTECH SLOV Acta Geotechnica Sloveni地学工程:地质SLOVENIA0001-5792ACTA HAEMATOL-BASELACTA HAEMATOLOGICA医学血液学SWITZERLAND 1827-9635ACTA HERPETOL Acta Herpetologica ZOOLOGY ITALY0065-1281ACTA HISTOCHEM ACTA HISTOCHEMICA生物细胞生物学GERMANY0044-5991ACTA HISTOCHEM CYTOC A CTA HISTOCHEMICA ET CYT生物细胞生物学JAPAN0137-1592ACTA ICHTHYOL PISCAT A cta Ichthyologica et Pi FISHERIES ZOOLOGY POLAND0001-5903ACTA INFORM ACTA INFORMATICA工程技术计算机:信息系GERMANY1359-6454ACTA MATER ACTA MATERIALIA工程技术材料科学:综合UNITED STATES 0168-9673ACTA MATH APPL SIN-E A cta Mathematicae Applic数学应用数学PEOPLES R CHINA 0236-5294ACTA MATH HUNG ACTA MATHEMATICA HUNGARI数学数学HUNGARY0252-9602ACTA MATH SCI ACTA MATHEMATICA SCIENTI数学数学PEOPLES R CHINA 1439-8516ACTA MATH SIN ACTA MATHEMATICA SINICA-数学数学PEOPLES R CHINA 0001-5962ACTA MATH-DJURSHOLMACTA MATHEMATICA数学数学SWEDEN0001-5970ACTA MECH ACTA MECHANICA物理力学AUSTRIA0567-7718ACTA MECH SINICA-PRC A CTA MECHANICA SINICA物理工程:机械PEOPLES R CHINA 0894-9166ACTA MECH SOLIDA SIN A CTA MECHANICA SOLIDA SI物理材料科学:综合PEOPLES R CHINA 0386-300X ACTA MED OKAYAMA ACTA MEDICA OKAYAMA医学医学:研究与实JAPAN1646-0758ACTA MEDICA PORT Acta médica portuguesa MEDICINE, GENERAL & INTERNA PORTUGAL0412-1961ACTA METALL SIN ACTA METALLURGICA SINICA工程技术冶金工程PEOPLES R CHINA 1006-7191ACTA METALL SIN-ENGL A cta Metallurgica Sinica METALLURGY & METALLURGICAL PEOPLES R CHINA 0894-0525ACTA METEOROL SIN Acta Meteorologica Sinic地学气象与大气科学PEOPLES R CHINA 1217-8950ACTA MICROBIOL IMM H A cta Microbiologica et I IMMUNOLOGY MICROBIOLOGY HUNGARY0798-4545ACTA MICROSC MICROSCOPY VENEZUELA1335-1788ACTA MONTAN SLOVACAActa Montanistica Slovac地学地球科学综合SLOVAKIA2075-8251ACTA NATURAE Acta Naturae农林科学林学SOUTH AFRICA 0065-1400ACTA NEUROBIOL EXP ACTA NEUROBIOLOGIAE EXPE医学神经科学POLAND0001-6268ACTA NEUROCHIR ACTA NEUROCHIRURGICA医学临床神经学AUSTRIA0300-9009ACTA NEUROL BELG ACTA NEUROLOGICA BELGICA医学临床神经学BELGIUM0001-6314ACTA NEUROL SCAND ACTA NEUROLOGICA SCANDIN医学临床神经学DENMARK0001-6322ACTA NEUROPATHOL ACTA NEUROPATHOLOGICA医学病理学GERMANY1601-5215ACTA NEUROPSYCHIATRACTA NEUROPSYCHIATRICANEUROSCIENCESPSYCHIATRY DENMARK0001-6349ACTA OBSTET GYN SCAN A CTA OBSTETRICIA ET GYNE医学妇产科学DENMARK0253-505X ACTA OCEANOL SIN ACTA OCEANOLOGICA SINICA地学海洋学PEOPLES R CHINA 0001-6357ACTA ODONTOL SCAND ACTA ODONTOLOGICA SCANDI医学牙科与口腔外科NORWAY1146-609X ACTA OECOL ACTA OECOLOGICA-INTERNAT环境科学生态学FRANCE0284-186X ACTA ONCOL ACTA ONCOLOGICA医学肿瘤学NORWAY1755-375X ACTA OPHTHALMOL ACTA OPHTHALMOLOGICA医学眼科学DENMARK0001-6454ACTA ORNITHOL ACTA ORNITHOLOGICA生物鸟类学POLAND1745-3674ACTA ORTHOP Acta Orthopaedica医学整形外科ENGLAND0001-6462ACTA ORTHOP BELG Acta Orthopaedica Belgic医学整形外科BELGIUM1017-995X ACTA ORTHOP TRAUMATO A cta Orthopaedica et Tra ORTHOPEDICS TURKEY1413-7852ACTA ORTOP BRAS Acta ortopedica brasilei Orthopedics Brazil0001-6489ACTA OTO-LARYNGOL ACTA OTO-LARYNGOLOGICA医学耳鼻喉科学NORWAY0392-100X ACTA OTORHINOLARYNGO A cta Otorhinolaryngologi OTORHINOLARYNGOLOGY ITALY0803-5253ACTA PAEDIATR ACTA PAEDIATRICA医学小儿科ENGLAND0567-7920ACTA PALAEONTOL POLACTA PALAEONTOLOGICA POL地学古生物学POLAND1230-2821ACTA PARASITOL ACTA PARASITOLOGICA医学寄生虫学POLAND0103-2100ACTA PAUL ENFERM Acta Paulista de Enferma NURSING BRAZIL1000-0569ACTA PETROL SIN ACTA PETROLOGICA SINICA G EOLOGY PEOPLES R CHINA 1330-0075ACTA PHARMACEUT Acta Pharmaceutica PHARMACOLOGY & PHARMACY CROATIA1671-4083ACTA PHARMACOL SIN ACTA PHARMACOLOGICA SINI医学化学综合PEOPLES R CHINA 0587-4246ACTA PHYS POL A ACTA PHYSICA POLONICA A物理物理:综合POLAND0587-4254ACTA PHYS POL B ACTA PHYSICA POLONICA B物理物理:综合POLAND1000-3290ACTA PHYS SIN-CH EDACTA PHYSICA SINICA物理物理:综合PEOPLES R CHINA 0323-0465ACTA PHYS SLOVACA ACTA PHYSICA SLOVACA物理物理:综合SLOVAKIA1000-6818ACTA PHYS-CHIM SIN ACTA PHYSICO-CHIMICA SIN化学物理化学PEOPLES R CHINA 1748-1708ACTA PHYSIOL Acta Physiologica医学生理学ENGLAND0231-424X ACTA PHYSIOL HUNG ACTA PHYSIOLOGICA HUNGAR医学生理学HUNGARY0137-5881ACTA PHYSIOL PLANT ACTA PHYSIOLOGIAE PLANTA生物植物科学GERMANY0001-6837ACTA POL PHARM ACTA POLONIAE PHARMACEUT医学药学POLAND1000-3304ACTA POLYM SIN ACTA POLYMERICA SINICA化学高分子科学PEOPLES R CHINA 1785-8860ACTA POLYTECH HUNG ACTA POLYTECHNICA HUNGAR ENGINEERING, MULTIDISCIPLIN HUNGARY0065-1583ACTA PROTOZOOL ACTA PROTOZOOLOGICA生物微生物学POLAND0001-690X ACTA PSYCHIAT SCANDACTA PSYCHIATRICA SCANDI医学精神病学ENGLAND0284-1851ACTA RADIOL ACTA RADIOLOGICA医学核医学SWEDEN0303-464X ACTA REUMATOL PORT Acta Reumatologica Portu医学风湿病学PORTUGAL1644-0692ACTA SCI POL-HORTORU A cta Scientiarum Polonor农林科学园艺POLAND1678-0345ACTA SCI VET Acta Scientiae Veterinar VETERINARY SCIENCES BRAZIL1807-8621ACTA SCI-AGRON Acta Scientiarum. Agrono AGRONOMY BRAZIL1806-2563ACTA SCI-TECHNOL ACTA SCIENTIARUM-TECHNOL综合性期刊综合性期刊BRAZIL0001-6977ACTA SOC BOT POL ACTA SOCIETATIS BOTANICO生物植物科学POLAND0001-7051ACTA THERIOL ACTA THERIOLOGICA生物动物学POLAND0001-706X ACTA TROP ACTA TROPICA医学寄生虫学NETHERLANDS 0001-7213ACTA VET BRNO ACTA VETERINARIA BRNO农林科学兽医学CZECH REPUBLIC 0236-6290ACTA VET HUNG ACTA VETERINARIA HUNGARI农林科学兽医学HUNGARY0044-605X ACTA VET SCAND ACTA VETERINARIA SCANDIN农林科学兽医学DENMARK0567-8315ACTA VET-BEOGRAD ACTA VETERINARIA-BEOGRAD农林科学兽医学SERBIA0001-723X ACTA VIROL ACTA VIROLOGICA医学病毒学SLOVAKIA1217-8837ACTA ZOOL ACAD SCI H A CTA ZOOLOGICA ACADEMIAE生物动物学HUNGARY0324-0770ACTA ZOOL BULGAR ACTA ZOOLOGICA BULGARICA ZOOLOGY BULGARIA0001-7272ACTA ZOOL-STOCKHOLMACTA ZOOLOGICA生物动物学ENGLAND1139-9287ACTAS ESP PSIQUIATRI A CTAS ESPANOLAS DE PSIQU医学精神病学SPAIN0210-4806ACTAS UROL ESP Actas Urologicas Espanol UROLOGY & NEPHROLOGY SPAIN0964-5284ACUPUNCT MED Acupuncture in Medicine I NTEGRATIVE & COMPLEMENTARY ENGLAND0360-1293ACUPUNCTURE ELECTROACUPUNCTURE & ELECTRO-TH医学全科医学与补充UNITED STATES 1570-8705AD HOC NETW Ad Hoc Networks工程技术电信学NETHERLANDS 1551-9899AD HOC SENS WIREL NE A d Hoc & Sensor Wireless工程技术电信学UNITED STATES 1280-8571ADANSONIA ADANSONIA生物植物科学FRANCE1059-7123ADAPT BEHAV ADAPTIVE BEHAVIOR工程技术计算机:人工智ENGLAND0736-5829ADAPT PHYS ACT Q ADAPTED PHYSICAL ACTIVIT医学康复医学UNITED STATES 0306-4603ADDICT BEHAV ADDICTIVE BEHAVIORS医学药物滥用ENGLAND1369-1600ADDICT BIOL ADDICTION BIOLOGY BIOCHEMISTRY & SUBSTANCE ABUS ENGLAND0965-2140ADDICTION ADDICTION医学精神病学ENGLAND0214-4840ADICCIONES Adicciones SUBSTANCE ABUSE SPAIN0263-6174ADSORPT SCI TECHNOLADSORPTION SCIENCE & TEC工程技术工程:化工ENGLAND0929-5607ADSORPTION ADSORPTION-JOURNAL OF TH工程技术工程:化工NETHERLANDS 0065-2113ADV AGRON ADVANCES IN AGRONOMY农林科学农艺学UNITED STATES 0301-5556ADV ANAT EMBRYOL CEL A DVANCES IN ANATOMY EMBR生物发育生物学UNITED STATES 1072-4109ADV ANAT PATHOL ADVANCES IN ANATOMIC PAT医学病理学UNITED STATES 1743-6753ADV APPL CERAM Advances in Applied Cera工程技术材料科学:硅酸ENGLAND0188-7009ADV APPL CLIFFORD AL A dvances in Applied Clif数学物理:数学物理SWITZERLAND 0196-8858ADV APPL MATH ADVANCES IN APPLIED MATH数学应用数学UNITED STATES 2070-0733ADV APPL MATH MECH Advances in Applied Math MATHEMATICS, AP MECHANICS PEOPLES R CHINA 0065-2156ADV APPL MECH ADVANCES IN APPLIED MECH工程技术工程:机械UNITED STATES 0065-2164ADV APPL MICROBIOL ADVANCES IN APPLIED MICR生物生物工程与应用UNITED STATES 0001-8678ADV APPL PROBAB ADVANCES IN APPLIED PROB数学统计学与概率论ENGLAND1687-7969ADV ASTRON Advances in Astronomy ASTRONOMY & ASTROPHYSICS UNITED STATES 0256-1530ADV ATMOS SCI ADVANCES IN ATMOSPHERIC 地学气象与大气科学PEOPLES R CHINA 1049-250X ADV ATOM MOL OPT PHY A DVANCES IN ATOMIC MOLEC物理光学UNITED STATES 0724-6145ADV BIOCHEM ENG BIOT A DVANCES IN BIOCHEMICAL 工程技术生物工程与应用UNITED STATES 0065-2296ADV BOT RES Advances in Botanical Re生物植物科学UNITED STATES 1864-8258ADV CALC VAR Advances in Calculus of MATHEMATICS, AP MATHEMATICS GERMANY0065-230X ADV CANCER RES ADVANCES IN CANCER RESEA医学肿瘤学UNITED STATES 0065-2318ADV CARBOHYD CHEM BI A DVANCES IN CARBOHYDRATE生物生化与分子生物UNITED STATES 0360-0564ADV CATAL ADVANCES IN CATALYSIS化学物理化学UNITED STATES 0951-7197ADV CEM RES ADVANCES IN CEMENT RESEA工程技术材料科学:综合ENGLAND0065-2385ADV CHEM PHYS Advances in Chemical Phy PHYSICS, ATOMIC, MOLECULAR UNITED STATES 1548-5595ADV CHRONIC KIDNEY D A DVANCES IN CHRONIC KIDN医学泌尿学与肾脏学UNITED STATES 0065-2423ADV CLIN CHEM ADVANCES IN CLINICAL CHE医学医学实验技术UNITED STATES 1899-5276ADV CLIN EXP MED Advances in Clinical and MEDICINE, RESEARCH & EXPERI POLAND0001-8686ADV COLLOID INTERFAC A DVANCES IN COLLOID AND 化学物理化学NETHERLANDS 0219-5259ADV COMPLEX SYST ADVANCES IN COMPLEX SYST综合性期刊数学跨学科应用SINGAPORE0924-3046ADV COMPOS MATER ADVANCED COMPOSITE MATER工程技术材料科学:复合NETHERLANDS 0065-2458ADV COMPUT ADVANCES IN COMPUTERS工程技术计算机:软件工UNITED STATES 1019-7168ADV COMPUT MATH ADVANCES IN COMPUTATIONA数学应用数学NETHERLANDS 1687-8108ADV COND MATTER PHYS A dvances in Condensed Ma PHYSICS, CONDENSED MATTER UNITED STATES 1862-5347ADV DATA ANAL CLASSI A dvances in Data Analysi STATISTICS & PROBABILITY GERMANY1687-1847ADV DIFFER EQU-NY Advances in Difference E MATHEMATICS, AP MATHEMATICS UNITED STATES 1079-9389ADV DIFFERENTIAL EQU B ULLETIN OF SYMBOLIC LOG MATHEMATICS, AP MATHEMATICS UNITED STATES0169-409X ADV DRUG DELIVER REV A DVANCED DRUG DELIVERY R医学药学NETHERLANDS 0065-2504ADV ECOL RES ADVANCES IN ECOLOGICAL R环境科学生态学UNITED STATES 1582-7445ADV ELECTR COMPUT EN A dvances in Electrical a工程技术工程:电子与电ROMANIA1614-6832ADV ENERGY MATER Advanced Energy Material CHEMISTRY, PHYS ENERGY & FUELS GERMANY1474-0346ADV ENG INFORM ADVANCED ENGINEERING INF工程技术工程:综合ENGLAND1438-1656ADV ENG MATER ADVANCED ENGINEERING MAT工程技术材料科学:综合GERMANY0965-9978ADV ENG SOFTW ADVANCES IN ENGINEERING 工程技术计算机:跨学科ENGLAND1616-301X ADV FUNCT MATER ADVANCED FUNCTIONAL MATE工程技术材料科学:综合GERMANY0065-2660ADV GENET ADVANCES IN GENETICS生物遗传学UNITED STATES 1615-715X ADV GEOM ADVANCES IN GEOMETRY数学数学GERMANY0065-2687ADV GEOPHYS Advances in GeophysicsGEOSCIENCES, MULTIDISCIPLIN UNITED STATES 1382-4996ADV HEALTH SCI EDUCADVANCES IN HEALTH SCIEN医学卫生保健UNITED STATES 2192-2640ADV HEALTHC MATER Advanced healthcare mate工程技术生物材料Germany?0065-2725ADV HETEROCYCL CHEMADVANCES IN HETEROCYCLIC化学有机化学UNITED STATES 1687-7357ADV HIGH ENERGY PHYS A dvances in High Energy PHYSICS, PARTICLES & FIELDS UNITED STATES 1076-5670ADV IMAG ELECT PHYSAdvances in Imaging and 物理物理:应用UNITED STATES 0065-2776ADV IMMUNOL ADVANCES IN IMMUNOLOGY医学免疫学UNITED STATES 0898-8838ADV INORG CHEM ADVANCES IN INORGANIC CH化学无机化学与核化UNITED STATES 0065-2806ADV INSECT PHYSIOL ADVANCES IN INSECT PHYSI生物昆虫学UNITED STATES 0065-2881ADV MAR BIOL Advances in Marine Biolo MARINE & FRESHWATER BIOLOGY UNITED STATES 0935-9648ADV MATER ADVANCED MATERIALS工程技术材料科学:综合UNITED STATES 0882-7958ADV MATER PROCESS ADVANCED MATERIALS & PRO工程技术材料科学:综合UNITED STATES 1687-8434ADV MATER SCI ENG Advances in Materials Sc MATERIALS SCIENCE, MULTIDIS UNITED STATES 0001-8708ADV MATH ADVANCES IN MATHEMATICS数学数学UNITED STATES 1930-5346ADV MATH COMMUN Advances in Mathematics 工程技术计算机:理论方UNITED STATES 1687-9120ADV MATH PHYS Advances in Mathematical数学应用数学United States 1687-8132ADV MECH ENG Advances in Mechanical E工程技术机械工程United States 1896-1126ADV MED SCI-POLAND Advances in Medical Scie医学医学:研究与实POLAND1687-9309ADV METEOROL Advances in Meteorology地学天文气象与大气科学UNITED STATES 0065-2911ADV MICROB PHYSIOL ADVANCES IN MICROBIAL PH生物生化与分子生物UNITED STATES 1536-1365ADV NONLINEAR STUD ADVANCED NONLINEAR STUDI数学数学UNITED STATES 0161-9268ADV NURS SCI ADVANCES IN NURSING SCIE医学护理UNITED STATES 2161-8313ADV NUTR Advances in nutrition (B医学营养学UNITED STATES 2195-1071ADV OPT MATER Advanced Optical Materia MATERIALS SCIEN OPTICS GERMANY1943-8206ADV OPT PHOTONICS Advances in Optics and P OPTICS UNITED STATES 0065-3055ADV ORGANOMET CHEM ADVANCES IN ORGANOMETALL化学无机化学与核化UNITED STATES 0065-308X ADV PARASIT ADVANCES IN PARASITOLOGY医学寄生虫学UNITED STATES 0001-8732ADV PHYS ADVANCES IN PHYSICS物理物理:凝聚态物ENGLAND0065-3160ADV PHYS ORG CHEM ADVANCES IN PHYSICAL ORG化学物理化学UNITED STATES 1043-4046ADV PHYSIOL EDUC ADVANCES IN PHYSIOLOGY E医学生理学UNITED STATES 0065-3195ADV POLYM SCI ADVANCES IN POLYMER SCIE化学高分子科学GERMANY0730-6679ADV POLYM TECH ADVANCES IN POLYMER TECH工程技术高分子科学UNITED STATES 0921-8831ADV POWDER TECHNOL ADVANCED POWDER TECHNOLO工程技术工程:化工JAPAN1876-1623ADV PROTEIN CHEM STR A dvances in Protein Chem BIOCHEMISTRY & MOLECULAR BI UNITED STATES 0065-3276ADV QUANTUM CHEM ADVANCES IN QUANTUM CHEM化学物理化学UNITED STATES 0169-1864ADV ROBOTICS ADVANCED ROBOTICS工程技术机器人学JAPAN1527-7941ADV SKIN WOUND CAREAdvances in Skin & Wound DERMATOLOGY NURSING UNITED STATES 0273-1177ADV SPACE RES ADVANCES IN SPACE RESEAR地学天文地球科学综合ENGLAND1816-112X ADV STEEL CONSTR Advanced Steel Construct CONSTRUCTION & ENGINEERING, C PEOPLES R CHINA1369-4332ADV STRUCT ENG ADVANCES IN STRUCTURAL E工程技术工程:土木UNITED STATES 0065-3454ADV STUD BEHAV ADVANCES IN THE STUDY OF医学行为科学UNITED STATES 1615-4150ADV SYNTH CATAL ADVANCED SYNTHESIS & CAT化学应用化学GERMANY1095-0761ADV THEOR MATH PHYSAdvances in Theoretical 物理物理:粒子与场UNITED STATES 0741-238X ADV THER ADVANCES IN THERAPY医学药学ENGLAND0972-5768ADV VIB ENG ADVANCES IN VIBRATION EN ENGINEERING, ME MECHANICS INDIA0065-3527ADV VIRUS RES Advances in Virus Resear VIROLOGY UNITED STATES 0309-1708ADV WATER RESOUR ADVANCES IN WATER RESOUR环境科学水资源ENGLAND1875-9637AEOLIAN RES Aeolian Research GEOGRAPHY, PHYSICAL ENGLAND0001-9054AEQUATIONES MATH Aequationes Mathematicae MATHEMATICS, AP MATHEMATICS SWITZERLAND 0393-5965AEROBIOLOGIA AEROBIOLOGIA环境科学环境科学NETHERLANDS 0001-9240AERONAUT J AERONAUTICAL JOURNAL工程技术工程:宇航ENGLAND1680-8584AEROSOL AIR QUAL RES A erosol and air quality ENVIRONMENTAL SCIENCES China0278-6826AEROSOL SCI TECH AEROSOL SCIENCE AND TECH环境科学工程:化工UNITED STATES 1270-9638AEROSP SCI TECHNOL AEROSPACE SCIENCE AND TE工程技术工程:宇航FRANCE0740-722X AEROSPACE AM AEROSPACE AMERICA工程技术工程:宇航UNITED STATES 0364-216X AESTHET PLAST SURG AESTHETIC PLASTIC SURGER医学外科UNITED STATES 1090-820X AESTHET SURG J Aesthetic Surgery Journa SURGERY UNITED STATES 1434-8411AEU-INT J ELECTRON C A EU-INTERNATIONAL JOURNA工程技术电信学GERMANY0001-9704AFINIDAD AFINIDAD化学化学综合SPAIN1021-3589AFR ENTOMOL AFRICAN ENTOMOLOGY生物昆虫学SOUTH AFRICA 1680-6905AFR HEALTH SCI African Health Sciences M EDICINE, GENERAL & INTERNA UGANDA1681-5556AFR INVERTEBR AFRICAN INVERTEBRATES生物动物学SOUTH AFRICA 1608-5914AFR J AQUAT SCI African Journal of Aquat MARINE & FRESHWATER BIOLOGY SOUTH AFRICA 0141-6707AFR J ECOL AFRICAN JOURNAL OF ECOLO环境科学生态学ENGLAND2156-4574AFR J HERPETOL AFRICAN JOURNAL OF HERPE ZOOLOGY SOUTH AFRICA 1814-232X AFR J MAR SCI AFRICAN JOURNAL OF MARIN生物海洋与淡水生物SOUTH AFRICA 1994-8220AFR J PSYCHIATRY African Journal of Psych PSYCHIATRY SOUTH AFRICA 1022-0119AFR J RANGE FOR SCIAfrican Journal of Range ECOLOGY ENVIRONMENTAL SOUTH AFRICA 0189-6016AFR J TRADIT COMPLEM A frican Journal of Tradi医学全科医学与补充NIGERIA2305-7963AFR NAT HIST African Natural History B IODIVERSITY CONSERVATION SOUTH AFRICA 1562-7020AFR ZOOL AFRICAN ZOOLOGY生物动物学SOUTH AFRICA 0161-9152AGE AGE医学老年医学UNITED STATES 0002-0729AGE AGEING AGE AND AGEING医学老年医学ENGLAND1568-1637AGEING RES REV AGEING RESEARCH REVIEWS医学老年医学IRELAND0096-140X AGGRESSIVE BEHAV AGGRESSIVE BEHAVIOR医学行为科学UNITED STATES 1474-9718AGING CELL AGING CELL生物老年医学ENGLAND1594-0667AGING CLIN EXP RES AGING CLINICAL AND EXPER医学老年医学ITALY1368-5538AGING MALE Aging Male医学泌尿学与肾脏学ENGLAND1360-7863AGING MENT HEALTH AGING & MENTAL HEALTH医学精神病学ENGLAND1945-4589AGING-US Aging CELL BIOLOGY UNITED STATES 0169-5150AGR ECON-BLACKWELL AGRICULTURAL ECONOMICS管理科学农业经济与政策NETHERLANDS 0139-570X AGR ECON-CZECH Agricultural Economics-Z AGRICULTURAL ECONOMICS & PO CZECH REPUBLIC 0167-8809AGR ECOSYST ENVIRONAGRICULTURE ECOSYSTEMS &环境科学环境科学NETHERLANDS 1459-6067AGR FOOD SCI AGRICULTURAL AND FOOD SC农林科学农业综合FINLAND1461-9555AGR FOREST ENTOMOL AGRICULTURAL AND FOREST 农林科学昆虫学ENGLAND0168-1923AGR FOREST METEOROLAGRICULTURAL AND FOREST 农林科学林学NETHERLANDS 0002-1482AGR HIST AGRICULTURAL HISTORY农林科学科学史与科学哲UNITED STATES 0889-048X AGR HUM VALUES AGRICULTURE AND HUMAN VA农林科学科学史与科学哲NETHERLANDS1671-2927AGR SCI CHINA Agricultural Sciences in AGRICULTURE, MULTIDISCIPLIN PEOPLES R CHINA 0308-521X AGR SYST AGRICULTURAL SYSTEMS农林科学农业综合NETHERLANDS 0378-3774AGR WATER MANAGE AGRICULTURAL WATER MANAG农林科学农艺学NETHERLANDS 1663-7852AGRARFORSCH SCHWEIZ+A GRARFORSCH SCHWEIZ AGRICULTURE, MULTIDISCIPLIN SWITZERLAND 0303-1853AGREKON Agrekon AGRICULTURAL ECONOMICS & PO SOUTH AFRICA 0742-4477AGRIBUSINESS Agribusiness农林科学食品科技UNITED STATES 1722-6996AGRO FOOD IND HI TEC A GRO FOOD INDUSTRY HI-TE工程技术生物工程与应用ITALY0002-1857AGROCHIMICA AGROCHIMICA农林科学土壤科学ITALY1405-3195AGROCIENCIA-MEXICO AGROCIENCIA农林科学农业综合MEXICO2168-3565AGROECOL SUST FOOD Agroecology and Sustaina AGRICULTURE, MULTIDISCIPLIN UNITED STATES 0167-4366AGROFOREST SYST AGROFORESTRY SYSTEMS农林科学林学NETHERLANDS 0002-1962AGRON J AGRONOMY JOURNAL农林科学农艺学UNITED STATES 1774-0746AGRON SUSTAIN DEV Agronomy for Sustainable农林科学农艺学FRANCE0921-7126AI COMMUN AI COMMUNICATIONS工程技术计算机:人工智NETHERLANDS 0890-0604AI EDAM AI EDAM-ARTIFICIAL INTEL工程技术工程:制造UNITED STATES 0738-4602AI MAG AI MAGAZINE工程技术计算机:人工智UNITED STATES 0001-1452AIAA J AIAA JOURNAL工程技术工程:宇航UNITED STATES 0001-1541AICHE J AICHE JOURNAL工程技术工程:化工UNITED STATES 0269-9370AIDS AIDS医学病毒学UNITED STATES 1087-2914AIDS PATIENT CARE ST A IDS PATIENT CARE AND ST医学传染病学UNITED STATES 0889-2229AIDS RES HUM RETROVAIDS RESEARCH AND HUMAN 医学病毒学UNITED STATES 1742-6405AIDS RES THER AIDS Research and Therap INFECTIOUS DISEASES ENGLAND1139-6121AIDS REV AIDS REVIEWS医学传染病学SPAIN2158-3226AIP ADV AIP Advances NANOSCIENCE & N MATERIALS SCIE UNITED STATES 1873-9318AIR QUAL ATMOS HLTHAir Quality, Atmosphere ENVIRONMENTAL SCIENCES NETHERLANDS 1748-8842AIRCR ENG AEROSP TEC A IRCRAFT ENGINEERING AND工程技术工程:宇航ENGLAND1608-5906AJAR-AFR J AIDS RESAJAR-African Journal of 医学公共卫生、环境SOUTH AFRICA 0341-051X AKTUEL RHEUMATOL AKTUELLE RHEUMATOLOGIE医学风湿病学GERMANY0001-7868AKTUEL UROL AKTUELLE UROLOGIE医学泌尿学与肾脏学GERMANY0311-5518ALCHERINGA ALCHERINGA地学古生物学ENGLAND0741-8329ALCOHOL ALCOHOL医学毒理学UNITED STATES 0735-0414ALCOHOL ALCOHOLISM ALCOHOL AND ALCOHOLISM医学药物滥用ENGLAND0145-6008ALCOHOL CLIN EXP RES A LCOHOLISM-CLINICAL AND 医学药物滥用UNITED STATES 0002-5100ALDRICHIM ACTA ALDRICHIMICA ACTA化学有机化学UNITED STATES 1980-0436ALEA-LAT AM J PROBAB L atin American Journal o STATISTICS & PROBABILITY BRAZIL2211-9264ALGAL RES Algal Research-Biomass B BIOTECHNOLOGY & APPLIED MIC NETHERLANDS 1005-3867ALGEBR COLLOQ ALGEBRA COLLOQUIUM数学数学PEOPLES R CHINA 1472-2739ALGEBR GEOM TOPOL Algebraic and Geometric 数学数学ENGLAND0002-5232ALGEBR LOG+Algebra and Logic数学数学RUSSIA1937-0652ALGEBR NUMBER THEORY A lgebra and Number Theor MATHEMATICS UNITED STATES 1386-923X ALGEBR REPRESENT THALGEBRAS AND REPRESENTAT数学数学NETHERLANDS 0002-5240ALGEBR UNIV ALGEBRA UNIVERSALIS数学数学SWITZERLAND 1748-7188ALGORITHM MOL BIOL Algorithms for Molecular生物生化研究方法ENGLAND0178-4617ALGORITHMICA ALGORITHMICA工程技术计算机:软件工UNITED STATES 0269-2813ALIMENT PHARM THER ALIMENTARY PHARMACOLOGY 医学胃肠肝病学ENGLAND0971-4693ALLELOPATHY J ALLELOPATHY JOURNAL农林科学农艺学INDIA0301-0546ALLERGOL IMMUNOPATHALLERGOLOGIA ET IMMUNOPA医学过敏SPAIN0344-5062ALLERGOLOGIE ALLERGOLOGIE医学过敏GERMANY0105-4538ALLERGY ALLERGY医学过敏ENGLAND2092-7355ALLERGY ASTHMA IMMUN T he American Academy of ALLERGY IMMUNOLOGY SOUTH KOREA 1088-5412ALLERGY ASTHMA PROCALLERGY AND ASTHMA PROCE医学过敏UNITED STATES 0002-5852ALLG FORST JAGDZTG ALLGEMEINE FORST UND JAG农林科学林学GERMANY1664-2201ALPINE BOT Alpine Botany PLANT SCIENCES SWITZERLAND 1078-6791ALTERN THER HEALTH M A lternative therapies in INTEGRATIVE & COMPLEMENTARY United States 1868-596X ALTEX-ALTERN ANIM EX A LTEX-ALTERNATIVES TO AN MEDICINE, RESEARCH & EXPERI GERMANY0893-0341ALZ DIS ASSOC DIS ALZHEIMER DISEASE & ASSO医学病理学UNITED STATES 1552-5260ALZHEIMERS DEMENT Alzheimers & Dementia医学临床神经学UNITED STATES 1758-9193ALZHEIMERS RES THERAlzheimer\'s Research an医学神经病学United Kingdom 0002-7626AM BEE J AMERICAN BEE JOURNAL生物昆虫学UNITED STATES 0002-7685AM BIOL TEACH The American Biology Tea BIOLOGY EDUCATION, SCI UNITED STATES 0002-7812AM CERAM SOC BULL AMERICAN CERAMIC SOCIETY工程技术材料科学:硅酸UNITED STATES 0002-838X AM FAM PHYSICIAN AMERICAN FAMILY PHYSICIA医学医学:内科UNITED STATES 0002-8444AM FERN J AMERICAN FERN JOURNAL生物植物科学UNITED STATES 0002-8703AM HEART J AMERICAN HEART JOURNAL医学心血管系统UNITED STATES 0002-9092AM J AGR ECON AMERICAN JOURNAL OF AGRI管理科学农业经济与政策UNITED STATES 1533-3175AM J ALZHEIMERS DISAmerican Journal of Alzh GERIATRICS & GE CLINICAL NEURO UNITED STATES 1059-0889AM J AUDIOL American Journal of Audi AUDIOLOGY & SPE OTORHINOLARYNG UNITED STATES 1526-5161AM J BIOETHICS AMERICAN JOURNAL OF BIOE社会科学科学史与科学哲UNITED STATES 0002-9122AM J BOT AMERICAN JOURNAL OF BOTA生物植物科学UNITED STATES 2156-6976AM J CANCER RES American Journal of Canc ONCOLOGY UNITED STATES 0002-9149AM J CARDIOL AMERICAN JOURNAL OF CARD医学心血管系统UNITED STATES 1175-3277AM J CARDIOVASC DRUG A merican Journal of Card医学心血管系统NEW ZEALAND 0192-415X AM J CHINESE MED AMERICAN JOURNAL OF CHIN医学全科医学与补充UNITED STATES 1175-0561AM J CLIN DERMATOL AMERICAN JOURNAL OF CLIN医学皮肤病学NEW ZEALAND 0002-9165AM J CLIN NUTR AMERICAN JOURNAL OF CLIN医学营养学UNITED STATES 0277-3732AM J CLIN ONCOL-CANC A MERICAN JOURNAL OF CLIN医学肿瘤学UNITED STATES 0002-9173AM J CLIN PATHOL AMERICAN JOURNAL OF CLIN医学病理学UNITED STATES 1062-3264AM J CRIT CARE AMERICAN JOURNAL OF CRIT医学护理UNITED STATES 0894-8275AM J DENT AMERICAN JOURNAL OF DENT医学牙科与口腔外科UNITED STATES 0193-1091AM J DERMATOPATH AMERICAN JOURNAL OF DERM医学皮肤病学UNITED STATES 0095-2990AM J DRUG ALCOHOL AB A MERICAN JOURNAL OF DRUG SUBSTANCE ABUSE UNITED STATES 0735-6757AM J EMERG MED AMERICAN JOURNAL OF EMER医学急救医学UNITED STATES 0002-9254AM J ENOL VITICULT AMERICAN JOURNAL OF ENOL农林科学生物工程与应用UNITED STATES 0002-9262AM J EPIDEMIOL AMERICAN JOURNAL OF EPID医学公共卫生、环境UNITED STATES 0195-7910AM J FOREN MED PATHAMERICAN JOURNAL OF FORE医学病理学UNITED STATES 0002-9270AM J GASTROENTEROL AMERICAN JOURNAL OF GAST医学胃肠肝病学UNITED STATES 1064-7481AM J GERIAT PSYCHIAT A MERICAN JOURNAL OF GERI医学精神病学UNITED STATES 1543-5946AM J GERIATR PHARMAC A merican Journal Geriatr GERIATRICS & GE PHARMACOLOGY &UNITED STATES 1079-2082AM J HEALTH-SYST PHAMERICAN JOURNAL OF HEAL医学药学UNITED STATES 0361-8609AM J HEMATOL AMERICAN JOURNAL OF HEMA医学血液学UNITED STATES 1049-9091AM J HOSP PALLIAT ME A merican Journal of Hosp HEALTH CARE SCIENCES & SERV UNITED STATES 1042-0533AM J HUM BIOL AMERICAN JOURNAL OF HUMA生物生物学UNITED STATES 0002-9297AM J HUM GENET AMERICAN JOURNAL OF HUMA生物遗传学UNITED STATES 0895-7061AM J HYPERTENS AMERICAN JOURNAL OF HYPE医学外周血管病UNITED STATES 0271-3586AM J IND MED AMERICAN JOURNAL OF INDU医学公共卫生、环境UNITED STATES 0196-6553AM J INFECT CONTROLAMERICAN JOURNAL OF INFE医学传染病学UNITED STATES 0272-6386AM J KIDNEY DIS AMERICAN JOURNAL OF KIDN医学泌尿学与肾脏学UNITED STATES 1088-0224AM J MANAG CARE AMERICAN JOURNAL OF MANA医学卫生保健UNITED STATES。
文献检索试题和答案

《大学生信息检索概论》模拟试题一、填空题1、文献的级次分为零次文献、一次文献、二次文献、三次文献2、《中图法》有五个基本部类,分别是马克思主义、列宁主义、毛泽东思想_、哲学;社会科学;自然科学和综合性图书,在此基础上又划分为_22_个大类。
3、按内容可将计算机检索系统的数据库类型分为:文献书目型数据库、事实型数据库、数值型数据库和全文型数据库。
4、我国标准可分为国家标准、部标准和企业标准三大类。
5、在实际检索中,文献的检索方法主要有:直查法、追溯法、工具法和综合法。
6、国际标准化组织简称:ISO 、本标准每5 年修订一次二、选择题1、如果需要检索某位作者的文献被引用的情况,应该使用(C )检索。
A.分类索引B.作者索引C.引文索引D.主题索引2、利用图书馆的据库检索期刊论文时,可供选择的中文数据库是(D )。
A.超星数字图书馆B.万方学位论文C.国研网D.维普科技期刊 E.高校财经库3、如果检索有关多媒体网络传播方面的文献,检索式为(A D)。
A.多媒体and 网络传播B.多媒体+网络传播C.多媒体or 网络传播D.多媒体*网络传播4、如果对某个课题进行主题检索时,可选择的检索字段有( A D E )。
A.关键词B.作者C.刊名D.题名E.文摘5、二次文献又称检索工具,包括:(A CD )。
A.书目B.百科C.索引D.文摘E.统计数据三、名词解释题1、文献用文字、图形、符号、声频、视频等技术手段记录人类知识的一种载体,或理解为固化在一定物质载体上的知识。
也可以理解为古今一切社会史料的总称。
2、体系分类语言体系语言是以科学分类为基础,运用概念的划分与概括的逻辑方法,形成一个概念等级体系,按知识门类的逻辑次序,按照从总到分,从一般到具体,从低级到高级,从简单到复杂的原则进行概念的综分,层层划分,累累隶属,逐步展开而形成的一个等级体系。
3、引文语言引文语言是根据文献所附参考或引用文献的特征进行检索的语言。
Data Science专业全解读
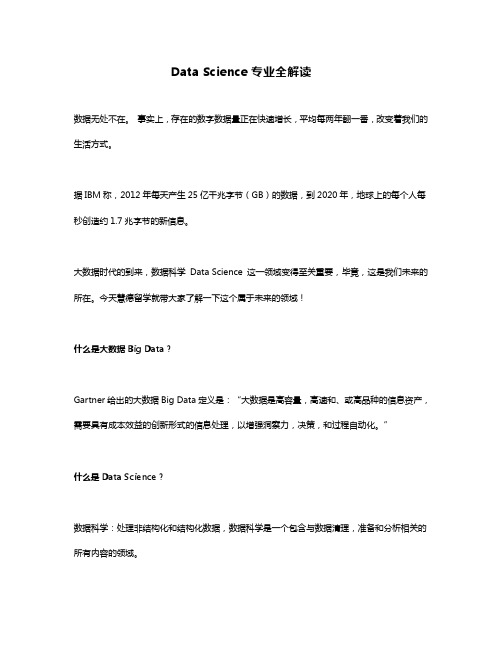
Data Science专业全解读数据无处不在。
事实上,存在的数字数据量正在快速增长,平均每两年翻一番,改变着我们的生活方式。
据IBM称,2012年每天产生25亿千兆字节(GB)的数据,到2020年,地球上的每个人每秒创造约1.7兆字节的新信息。
大数据时代的到来,数据科学Data Science 这一领域变得至关重要,毕竟,这是我们未来的所在。
今天慧德留学就带大家了解一下这个属于未来的领域!什么是大数据Big Data?Gartner给出的大数据Big Data定义是:“大数据是高容量,高速和、或高品种的信息资产,需要具有成本效益的创新形式的信息处理,以增强洞察力,决策,和过程自动化。
”什么是Data Science?数据科学:处理非结构化和结构化数据,数据科学是一个包含与数据清理,准备和分析相关的所有内容的领域。
数据科学是统计学,数学,编程,解决问题,以巧妙方式捕获数据,以不同方式查看事物的能力以及清理,准备和对齐数据的活动的组合。
简单来说,它是尝试从数据中提取见解和信息时使用的技术方式。
这就衍生了其中一种专业叫数据分析Data Analytics数据分析Data Analytics是检查原始数据的科学,目的是得出有关该信息的结论。
数据分析涉及应用算法或机械过程来获得洞察力。
例如,运行大量数据来查找彼此之间的相关性。
它被用于许多行业,使组织和公司能够做出更好的决策,并验证和反驳现有的理论或模型。
数据分析的重点在于推理,即推断结论的过程完全基于研究人员已经知道的内容。
Data Science可以应用在哪些领域?互联网搜索Internet search搜索引擎利用数据科学算法在几秒钟内为搜索查询提供最佳结果。
数字广告Digital Advertisement整个数字营销使用数据科学算法- 从显示横幅到数字广告牌。
这也是数字广告获得比传统广告更高点击率的原因。
推荐系统Recommender systems推荐系统不仅可以轻松找到数十亿产品中的相关产品,还可以增添很多用户体验。
- 1、下载文档前请自行甄别文档内容的完整性,平台不提供额外的编辑、内容补充、找答案等附加服务。
- 2、"仅部分预览"的文档,不可在线预览部分如存在完整性等问题,可反馈申请退款(可完整预览的文档不适用该条件!)。
- 3、如文档侵犯您的权益,请联系客服反馈,我们会尽快为您处理(人工客服工作时间:9:00-18:30)。
SAR Target Recognition Based on Deep LearningSizhe Chen, Haipeng WangKey Laboratory for Information Sciences of Electromagnetic Waves (MoE)Fudan University, Shanghai, ChinaEmail: hpwang@Abstract— Deep learning algorithms such as convolutional neural networks (CNN) have been successfully applied in computer vision. This paper attempts to adapt the optical camera-oriented CNN to its microwave counterpart, i.e. synthetic aperture radar (SAR). As a preliminary study, a single layer of convolutional neural network is used to automatically learn features from SAR images. Instead of using the classical backpropagation algorithm, the convolution kernel is trained on randomly sampled image patches using unsupervised sparse auto-encoder. After convolution and pooling, an input SAR image is then transformed into a series of feature maps. These feature maps are then used to train a final softmax classifier. Initial experiments on MSTAR public data set show that an accuracy of 90.1% can be achieved on three types of targets classification task, and an accuracy of 84.7% is achievable on ten types of targets classification task.Keywords—Convolutional Neural Network; sparse auto-encoder; Synthetic Aperture Radar; Automatic Target RecognitionI.I NTRODUCTIONSynthetic aperture radar (SAR) is a kind of airborne or spaceborne two-dimensional high resolution imaging radar. Unlike optical remote sensing, which cannot work in poor weather conditions and night, SAR can operate regardless of weather conditions, day and night, so it is of high value in military and civil applications. But the interpretation of SAR images needs specialists, since unlike natural images, SAR images reflect the backscattering intensity of electromagnetic wave. Moreover, searching targets of interest in the massive SAR images by human is time-consuming and extremely difficult, which justifies the need for efficient SAR automatic target recognition (ATR) algorithms.MIT Lincoln Laboratory has pioneered research on this topic, as evidenced in their two SAR ATR system: Template-based SAIP (semi-automated image intelligence processing system) program [1, 2] and Model-based MSTAR (moving and stationary target acquisition and recognition) program [3]. The main component of SAIP system is standard pattern recognition architecture. The researchers collected several hundreds of thousands airborne SAR images, designed five different features extraction algorithms, and finally use these feature vectors as inputs to train a classifier. The performance is nearly perfect when testing conditions are similar to the training conditions, however, when testing conditions changed the recognition rate will decrease drastically [2]. The reason is that SAR images are very sensitive to all kinds of variations, such as articulation, obscuration, and camouflage on military targets, and changes of background environment. Uncertainties in the real world are so large that it is unrealistic to collect sample images covering all possible variations. To solve these problems, a model-based module was later added on the back-end of MSTAR system. The front-end of MSTAR system is still a typical pattern recognition system, while targets unable to be recognized by front-end will be sent into back-end, which is an iterative searching classifier. In each iteration, features extracted from test images are compared with features predicted from target CAD model using electromagnetic signature prediction code. The needs for another iteration are determined according to the degree of matching. In both of these SAR ATR systems, designing and selecting useful features plays an important role in recognition accuracy.Just like machine learning used in speech recognition and computer vision, the most important thing is feature engineering: designing better features to represent targets and then use these features as input to train a classifier. Previously, researchers in computer vision community developed many powerful features for object recognition, such as SIFT (Scale-invariant feature transform) and HOG (Histogram of Oriented Gradients). Over the last few years, considerable efforts have been made to design multi-stage architectures to automatically learn representations or features from data instead of hand-crafted features in speech recognition and computer vision domain. In many speech recognition or natural images object recognition benchmark dataset, these feature learning algorithms have achieved superior performance.Nowadays, deep architecture with convolution and pooling are found to be highly effective and commonly used incomputer vision and object recognition [4-14]. The most impressive result was achieved in 2012 ImageNet contest, where 1.2 million images in the training set with 1000 different object classes. On the test data set including 150,000 images, the deep Convolutional Neural Network (CNN) approach described in [4] achieved the error rates considerably lower than the previous state-of-the-art results. Furthermore, CNN has achieved superior classification accuracy on different tasks, such as handwritten digits or Latin and Chinese character recognition [5, 6], traffic sign recognition [7], face detection and recognition [8]. Deep networks are shown to be powerful for computer vision and image recognition tasks because they extract appropriate features while jointly performing discrimination [9].A few researchers in SAR recognition area have tried to use multi-layer network to learn features for classification [15, 16]. However, in their work, complex image pre-processing and hand-crafted feature extraction on the first layer are needed. Fully unsupervised feature learning algorithm begins from extracted features instead of raw input.This paper attempts to adapt the optical camera-oriented CNN to its microwave counterpart, i.e. synthetic aperture radar (SAR). As a preliminary study, a single layer of convolutional neural network is used to automatically learn features from SAR images. It will be shown that although SAR images are extremely noisy and identifying targets is not an easy task even for humans, features learned from raw input pixels can still be useful for classification.II.A LGORITHMSMost successful and commonly used feature learning models typically consist of multi-stage trainable feature extractor, greedily layer-wise training one layer at a time, treating the features learned from previous layer as the input to the next layer, using unsupervised feature learning algorithm. For each of these layers, a number of parameters are chosen from cross-validation or empirically: the size of convolution receptive field, the number of feature maps in each layer, dimension of pooling region. Results in [12] demonstrate that with large number of hidden nodes and dense feature extraction, using simple unsupervised learning algorithm and only a single layer of features, state-of-art results on both CIFAR-10 and NORB datasets can be achieved. Because our goal is firstly to find whether features learned from raw input SAR images would be useful for target recognition or not, for simplicity, we choose a single layer convolutional neural network (CNN).Our approach consists of the following steps to learn a feature representations: (1) Extract random patches from unlabeled images, the dimension of these patches is the same as convolution kernel. (2) Subtract mean value and then apply ZCA whitening pre-processing to the sampled patches. (3) Instead of training purely supervised from labeled image data using classical backpropagation and stochastic gradient decent, our convolutional layer is trained using sparse auto-encoder on randomly sampled image patches.Given the learned convolution kernel and a set of labeled training images, we can perform feature extraction and classification: (1) Convolve the input images with learned convolution kernel. (2) Pool features over local neighborhoods using max operation, reducing the dimension of feature values and achieving invariance to small distortion. (3) Train a softmax regression classifier to predict the labels given the pooled convolved feature vectors, the weights on this final layer is trained supervised using backpropagation. Fig. 1 shows the flowchart of the algorithm.Fig. 1 Flowchart of the algorithmA.Generate image patches for unsupervised learningOur approach begins by resizing all of the SAR images to 128 128pixels. And then extract a large number of image patches from unlabeled training input images. The size of each image patches is w w, where w referred to the size of convolution receptive field. Next convert each image patch into an N –dimensional vector, where N w w. Suppose the total number of sampled image patches is m, and concatenate all of them into N m matrix: X , , , , where .SAR imagedatasetB.Pre-processingIn this step, each patch is normalized by subtracting the mean value of each image patch and then apply Zero Component Analysis (ZCA) Whitening [17]. This pre-processing approach is commonly used in deep learning. The main idea here is that since adjacent pixel values are highly correlated, the raw input is highly redundant. The goal of ZCA whitening is to make the input less correlated with each other, and to make all input elements have the same variance. We can compute the ZCA whitened data as: , whereT UP / U T and U and P are the eigenvectors and eigenvalues of the covariance matrix ofX: Σ∑ T.C.Sparse auto-encoderThe sparse auto-encoder is a kind of one hidden layer neural network that sets its output target value to be the same as the input data. In other word, it tries to learn an identity function , . By minimizing the reconstruction error with an extra sparse penalty term that encourage the hidden units to keep low average activation, convolution kernel W and biases b will be learned using backpropagation from unlabeled data. Imposing a sparsity constraint on the hidden layer, then structure of input data will be discovered by the auto-encoder.For each training sample , the activation value on the hidden layer is given by:(1) where , denotes to connection weightsand bias respectively, and 1/ 1 is the logistic sigmoid function. However, sigmoid function cannot be used on the output layer, since the output of logistic sigmoid function is in the range of [0, 1], while input data are not rescaled in the range [0, 1]. So a linear activation function are chosen for the output layer:(2) where , . With a training set of m examples, the overall cost function is defined as:W,b 112,(3)The first term is an average sum of squared error term, tryingto minimize reconstruction error. The second term isregularization term, which is commonly used to preventoverfitting.Sparsity constraint means that for each inputs , most ofthe corresponding hidden units are close to 0, only afew of them will be activated. Given a training set with msamples, the average activation value of hidden unit j is:1(4)The sparsity penalty term is based on the Kullback-Leibler(KL) divergence between a Bernoulli random variable withmean and a Bernoulli random variable with mean . KL-divergence is used to measure the extent of discrepancybetween two different distributions. This penalty term can bewritten as:KL log1 log11 (5)where is sparsity parameter, typically a small value aroundzero (say 0.02 .KL 0, if , and increasesmonotonically as deviates from . This penalty term seeksto enforce the average activation of hidden units to be equalto .Suppose the number of hidden units is K, the overall costfunction is:, W,b KL(6)D.Convolutional feature extraction and poolingA single stage of convolution network consists of three layers:a convolution filter layer, a non-linearity layer, a featurepooling layer. At the output, each input image is represented bysets of arrays called feature maps. Each feature maps representsa particular feature extracted at all locations on the wholeimages. Fig. 2 shows the Architecture of the 1-layer CNN.Convolution filter layer: given an input image ofpixels, the output y composed of K feature maps of size nw 1 n w 1 . K corresponds to the number of hiddenunits in the sparse auto-encoder trained on small patchespreviously. All trainable convolution kernel have sizeand convolve them with large input images. The modelcomputes ∑where is the 2D discreteconvolution operator, is a trainable bias, andmeans flipping the matrix horizontally and vertically. Both convolution kernel and biases are unsupervised trained using sparse auto-encoder.Fig. 2 Architecture of the 1-layer CNNNon-linearity Layer: Simply apply logistic sigmoidfunction 1/ 1 point-wise. Then a methodcalled Local Contrast Normalization was employed to performlocal subtractive and divisive normalizations, which enforceslocal competition between adjacent features in a feature map, and between features at the same spatial location in different feature maps [10]. The subtractive normalization operationremoves the weighted average of neighboring neurons from thecurrent neuron: ∑ , , , whereis a Gaussian weighting window (typically of size 9 9)normalized so that ∑ 1. The divisive normalizationcomputes /max , where∑, ,/ .Feature pooling layer: Max pooling operation is chosen,which computes the maximum value over a neighborhood region in each feature map: , , , . This process not only reduce the dimension of output feature mapsbut also introduce small translation invariance, which helps to build robust features for classification. E. Softmax regression classifierIn this step, pooled features and their corresponding labels are used to train the final softmax classifier. Softmax regression model focuses on multi-class classification. Given a test input , output of softmax classifier will be a k dimensional vector, each element of which refers to the estimated probability of the class label equal to each of the k different values conditioned on this input feature: | ; , for each value of 1, , . The output k dimensional hypothesis takes the form:1 ;2 ; ;1∑(7)Here, , , ,are the parameters to be learned supervised. The cost function would be:1 1log ∑(8)where 1 · is the indicator function, so that 1{a true statement}= 1, and 1{a false statement} = 0. For example, 1{2 + 2 =4} =1, whereas 1{1 + 1 = 3} = 0. The second term is weightdecay term which prevents overfitting. The minimum ofneeds to be solved by gradient decent. The gradient of costfunction is:11; (9) Then plug it into gradient decent algorithm, for eachiteration the parameter will perform the update:.III. E XPERIMENTAL RESULTSA. DatasetThe experiment data set was collected by the Sandia National Laboratory (SNL) SAR sensor platform. The collection was jointly sponsored by Defense AdvancedInput imageContrastNormalizationPoolingResearch Projects Agency (DARPA) and Air Force Research Laboratory as part of the Moving and Stationary Target Acquisition and Recognition (MSTAR) program. They collected hundreds of thousands of SAR images containing ground military targets, and only a small subset of which are public available on the website [18]. The public released datasets include 10 different types of ground military targets (BMP2, BRDM2, BTR60, BTR70, D7, T62, T72, ZIL131, ZSU234, 2S1), and was collected by using an X-band SAR sensor in one foot resolution spotlight mode, full aspect coverage (in the range of 0 to 360 degree). The data collected at 17 degree depression angles are used for training, and the data collected at 15 degree depression angles are used for testing.Fig. 3 Ten types of military targets in SAR images (left) andtheir corresponding optical images (right).Fig. 4 Visualization of learned convolutional kernels from SAR images (top) vs. that learned from optical images asgiven in [17] (bottom).The ten types of targets in SAR images are shown in Fig. 3 with the corresponding optic images given for comparison. As we can see, the same target appears to be very different than optical image. The most prominent difference lies to the ‘speckle’ appearance of SAR image. Fig. 4 compares the learned convolutional kernels of SAR images vs. conventional optical images. Interestingly, the SAR features looks very different from optical features which are basically edge detector templates. The SAR features looks more like ‘sinc’ shaped responses probabaly due to SAR speckle.B. Classification ResultsThe algorithms are tested on two classification experiments. The first one is classifying three types of military targets. The details of training and testing data set are shown in TABLE Ι. In the experiment, deformation targets are included in the test set. For example, T72_132, T72_812, and T72_s7 are all belong to T72 tank, but they have different militaryconfigurations such as whether contain machine guns, antenna,(2) BMP2(1) BRDM2(4) BTR60(3) BTR70(6) D7(5) 2S1(8) T62(7) T72(10) ZIL131(9) ZSU234protection armor or not. The classification accuracy of three types of target is 90.1% (error rate 9.9%).TABLE I. SAMPLE NUMBER OF TRAINING AND T ESTING SET FOR THREETYPESTraining set Sample number Testing set Sample numberBMP2_c21 BTR70_c71 T72_132 233233232BMP2_c21BMP2_9563BMP2_9566BTR70_c71T72_132T72_812T72_s7196195196196196195191The second one is classifying ten types of military targets. In this experiment, all of the targets are in standard configuration, deformation targets are not included. The details of training and testing data set are shown in TABLE Ⅱ. In this experiment, after adjust hyper parameters by cross-validation, we achieve a classification accuracy of 84.7% (error rate 15.3%). The detailed ambiguity matrix is shown in TABLE III.TABLE II. SAMPLE NUMBER OF TRAINING AND T ESTING SET FOR TENTYPESTraining set Sample number Testing set Sample numberBMP2BRDM2BTR60BTR70D72S1T62T72ZIL131ZSU234233298256233299299299232299299BMP2BRDM2BTR60BTR70D72S1T62T72ZIL131ZSU234195274195196274274273196274274For comparison, similar studies using handcrafted features [19] to classify the same set of MSTAR datasets have achieved accuracy of 96.79% for three types and 90.5% for ten types, respectively. It can be seen that the fully automatic single-layer CNN is able to achieve reasonable performance (i.e. ~5% lower than manually designed classifier) which makes us confident that a multi-layer ‘deep’ CNN might achieve comparable or better performance than hand-crafted algorithms.TABLE III. CLASSIFICATION A MBIGUITY M ATRIXTest setClassification ResultsBMP2 BRDM2 BTR60 BTR70 D7 2S1 T62 T72 ZIL131 ZSU234Classificationaccuracy (%)BMP2 157 9 2 9 0 4 0 4 6 4 80.5 BRDM2 9 220 6 18 0 3 1 2 15 0 80.2 BTR60 0 11 168 4 4 4 1 2 1 0 86.1 BTR70 3 4 3 181 0 4 0 0 1 0 92.3 D7 0 0 0 0 252 0 8 2 5 7 91.9 2S1 14 9 5 5 0 190 7 22 21 1 69.3 T62 2 1 5 0 4 7 2423 7 2 88.6T72 3 3 1 1 0 8 2168 9 1 85.7 ZIL131 5 6 5 7 1 12 3 9 226 0 82.4 ZSU234 1 1 3 0 4 1 2 7 6 249 90.8average classification rate: 84.7%IV.C ONCLUSION AND FUTURE WORKAs a preliminary study, a single stage of convolutional network is adapted to automatically learn features useful for SAR target recognition. Using learned conolutional features, an accuracy of 90.1% and 84.7% are achieved on three and ten types of targets MSTAR dataset classifcation, respectively. The next step is to learn hierarchical features by using multi-stage convolutional network, considering the scarcity of SAR image samples, data augmentation technique and overfitting reduction methods must be utilized.R EFERENCES[1]Novak L M, Owirka G J, Brower W S, et al. “The automatic target-recognition system in SAIP,” Lincoln Laboratory Journal, 1997, 10(2). [2]Novak L M. “State-of-the-art of SAR automatic target recognition,”Radar Conference, 2000. The Record of the IEEE 2000 International.IEEE, 2000: 836-843.[3]Wissinger J, Ristroph R, Diemunsch J R, et al. “MSTAR's extensiblesearch engine and model-based inferencing toolkit,” AeroSense'99.International Society for Optics and Photonics, 1999: 554-570.[4]Krizhevsky A, Sutskever I, Hinton G E. “ImageNet Classification withDeep Convolutional Neural Networks,” NIPS. 2012, 1(2): 4.[5]Ciresan D C, Meier U, Gambardella L M, et al. “Deep, big, simpleneural nets for handwritten digit recognition,” Neural computation, 2010, 22(12): 3207-3220.[6]Ciresan D C, Meier U, Schmidhuber J. “Transfer learning for Latin andChinese characters with deep neural networks,” Neural Networks (IJCNN), The 2012 International Joint Conference on. IEEE, 2012: 1-6. [7]Cireşan D, Meier U, Masci J, et al. “Multi-column deep neural networkfor traffic sign classification,” Neural Networks, 2012, 32: 333-338. [8]Taigman Y, Yang M, Ranzato M, et al. “Deep-Face: Closing the Gap toHuman-Level Performance in Face Verification,” IEEE CVPR. 2014. [9]Yann LeCun. “Learning invariant feature hierarchies,” Computervision–ECCV 2012. Workshops and demonstrations. Springer Berlin Heidelberg, 2012: 496-505.[10]Jarrett K, Kavukcuoglu K, Ranzato M, et al. “What is the best multi-stage architecture for object recognition?” Computer Vision, 2009 IEEE 12th International Conference on. IEEE, 2009: 2146-2153.[11]Kavukcuoglu K, Sermanet P, Boureau Y L, et al. “LearningConvolutional Feature Hierarchies for Visual Recognition,” NIPS. 2010, 1(2): 5. [12]Coates A, Ng A Y, Lee H. “An analysis of single-layer networks inunsupervised feature learning,” International Conference on Artificial Intelligence and Statistics. 2011: 215-223.[13]Zeiler M D, Krishnan D, Taylor G W, et al. “Deconvolutionalnetworks,” Computer Vision and Pattern Recognition (CVPR), 2010 IEEE Conference on. IEEE, 2010: 2528-2535.[14]Zeiler M D, Taylor G W, Fergus R. “Adaptive deconvolutional networksfor mid and high level feature learning,” Computer Vision (ICCV), 2011 IEEE International Conference on. IEEE, 2011: 2018-2025.[15]Diemunsch J R, Wissinger J. “Moving and stationary target acquisitionand recognition (MSTAR) model-based automatic target recognition: search technology for a robust ATR.” Aerospace/Defense Sensing and Controls. International Society for Optics and Photonics, 1998: 481-492.[16]Sun Z, Xue L, Xu Y, et al. “Marginal Fisher Analysis Feature ExtractionAlgorithm Based on Multilayer Auto-encoder,” Journal of Information and Computational Science, 2012, 9(18): 5897-5906.[17]Deep learning tutorial: /wiki/index.php/UFLDL_Tutorial[18]MSTAR public targets dataset: https:///index.php?collection=mstar[19]ZHANG Xinzheng, HUANG Peikang. “SAR ATR based on Bayesiancompressive sensing,” Systems Engineering and Electronics, 2013, 35(1): 40-44. [in Chinese]。