Title Multicriteria Analysis and Visualization of Location-Allocation Problems
多维标度法介绍
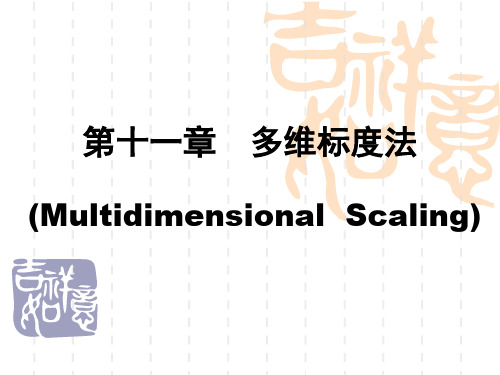
A牌 B牌
B牌
1Hale Waihona Puke C牌32▪ 各种品牌的相似次序矩阵是多维标度法的输入 资料。在第四节中我们将介绍几种建立相似次 序矩阵的方法。
多维标度法的基本思想:
▪ 用r维空间(r待定)中的点分别表示各样品,使得各 样品间距离的次序能完全反映原始输入的相似次 序(两样品间的距离越短,则越相似)。通常,要 通过两步来完成。
2.计算初步图形结构中各点之间的距离
▪ 为叙述方便,有时我们将A,B,C三种品牌分 别称为第一、第二、第三品牌。
▪ 用欧氏距离公式计算A品牌和B品牌(第一品牌 和第二品牌)的距离
d12 (10 1)2 (5 - 5)2 9
▪ 同样计算d13,d23,并将它们排成矩阵形式 (dii=dii),这个矩阵称为初步图形结构的距离矩 阵,如表3所示。
第十一章 多维标度法
(Multidimensional Scaling)
第一节 引 言
第一节 引 言
▪ 多维标度法(MDS)是著名计量心理学家谢泼 德(Shephard)和克鲁斯克 (Kruskal)分别于 1962年和1964年发展起来的一种计量心理学 技术。
▪ 多维标度法现在已经广泛应用于心理学、市 场调查、社会学、物理学、政治科学以及生 物学等领域的数据分析方法。
▪ 因此,多维标度法是用间接方法推断出品 牌有关的特性。
▪ 在许多情形中,我们可能不知道那些特性 与品牌有关,或者应答者不能够或不愿意 准确地回答,这时必须采用间接推断的方 法。
▪ 广义的MDS可以将聚类分析和对应分析 (Correspondence Analysis)也包括进 来。
▪ 根据它所利用的信息来看,多维标度法 可以分为两大类:
▪ 多维标度法可以说是上述问题的逆问题, 即给定样品两两之间的距离或相似度的排 序,反求各样品点的坐标。
journal of multivariate analysis 分区

journal of multivariate analysis 分区
一、期刊简介
《Journal of Multivariate Analysis》是一本致力于多元分析领域的研究的国际性学术期刊。
它由著名出版商Elsevier发行,是我国数学、统计学等领域的重要学术交流平台。
二、分区情况
根据我国权威的学术期刊分区体系,《Journal of Multivariate Analysis》被分为Q1区,这意味着该期刊在多元分析领域具有较高的学术地位和影响力。
三、影响因子和排名
根据最新数据,该期刊的影响因子(IF)为2.684,排名在国际同类期刊中位居前列。
这表明该期刊的文章质量和研究成果得到了广泛认可。
四、投稿要求和注意事项
1.投稿范围:该期刊主要接收关于多元分析方法、理论及其在实际应用中的研究论文。
2.语言要求:文章应使用英语撰写,确保表达清晰、论述严谨。
3.格式规范:遵循期刊提供的模板,确保图表、参考文献等符合要求。
4.原创性:文章应确保原创,避免抄袭、剽窃等行为。
5.投稿方式:通过Elsevier官网进行投稿,需注册账号并按照系统提示操作。
五、结论
《Journal of Multivariate Analysis》是一本在多元分析领域具有较高影响力的国际期刊,其分区地位和影响因子均表现出较强的学术价值和认可度。
对于从事多元分析研究的研究者来说,投稿该期刊是一个展示研究成果、提高学术地位的良好机会。
多维尺度分析课件

多维尺度分析课件
1.1方法原理
• 空间图的轴表示调查对象对刺激物形成的感知以及偏好的 潜在维度;空间图上点的位置表示不同的刺激物之间的潜 在规律性联系。多维尺度分析本质上是数据缩减技术的一 种,它试图是将刺激物间原始的相异性数据结构,转化成 一个多维度的空间感知图,个体在空间中的相对关系会与 原始输入数据保持一致。这种空间的维度可以解释并用来 进一步了解输入的原始数据。通过MDS方法可以探讨各种 刺激物之间的相似性和相异性,并于二维或三维空间表达 个体间的相对位置。
• 第三,因子数量不同。因素分析方法基于严格的线性假设,它是把变量表示 成各公因子的线性组合,分析者可以根据需要确定因子的数量;而MDS方法 不但剔除了线性结果的假设,而且因子数量受到研究对象数量的限制,所以 MDS方法更易于获得较少的维度。
• 第四,结果分析方式不同。因素分析模型源于向量之间的夹角,可以通过向 量旋转解释因子,但是需要根据结果对因子进行重新命名。MDS模型是基于 多维空间上点与点之间距离,并且最终的结果将以空间图的形式呈现,所以 MDS方法的处理结果比因素分析方法的结果更容易解释。
多维尺度分析他多元统计方法一样,首先需要对所研究的问题进行准确 界定;由于MDS允许多种类型数据的输入,所以,需要根据问题决定获取数 据的形式和方法;并在多种MDS算法中选择一种符合研究目的的方法;处理 分析结果的一个重要方面就是确定适当的空间图维数;之后需要基于空间结 构的解释,对空间图的坐标轴进行命名;最后要对评估所用方法的可靠性和 有效性。具体的分析步骤如图所示:
文献外部特征的检索语言

文献外部特征的检索语言外部特征的检索语言是指在文献检索中使用的特定术语或关键词,用于寻找与特定主题或研究领域相关的外部特征的文献。
这些外部特征可能涉及物体表面的形态、纹理、颜色、特定结构或其他性质,或者涉及人体的某些特定特征或行为。
以下是一些与外部特征相关的常用检索语言的示例:1. 表面形态特征:- 均匀性(uniformity)- 曲率(curvature)- 平滑度(smoothness)- 粗糙度(roughness)- 几何形状(geometric shape)- 表面形貌(surface topography)- 表面形态(surface morphology)2. 表面纹理特征:- 纹理特征(texture features)- 纹理描述(texture descriptors)- 纹理分析(texture analysis)- 纹理判别(texture discrimination)- 纹理识别(texture recognition)- 纹理提取(texture extraction)- 纹理模型(texture model)- 纹理分类(texture classification)3. 表面颜色特征:- 颜色特征(color features)- 颜色分布(color distribution)- 颜色模型(color model)- 颜色空间(color space)- 颜色直方图(color histogram)- 颜色特征提取(color feature extraction)- 颜色描述符(color descriptor)4. 特定结构特征:- 细胞结构(cellular structure)- 晶格结构(lattice structure)- 分子结构(molecular structure)- 生物组织结构(biological tissue structure)- 表表面结构(surface structure)- 微观结构(microstructure)5. 人体特征:- 人脸特征(facial features)- 身体形态(body shape)- 手部特征(hand features)- 骨骼结构(skeletal structure)- 步态分析(gait analysis)- 视觉注意(visual attention)- 姿势识别(pose recognition)- 表情识别(facial expression recognition)以上仅是外部特征检索语言的示例,实际应用中需要根据具体的研究领域和研究目的进行调整和进一步扩展。
origin菜单栏的中文解释
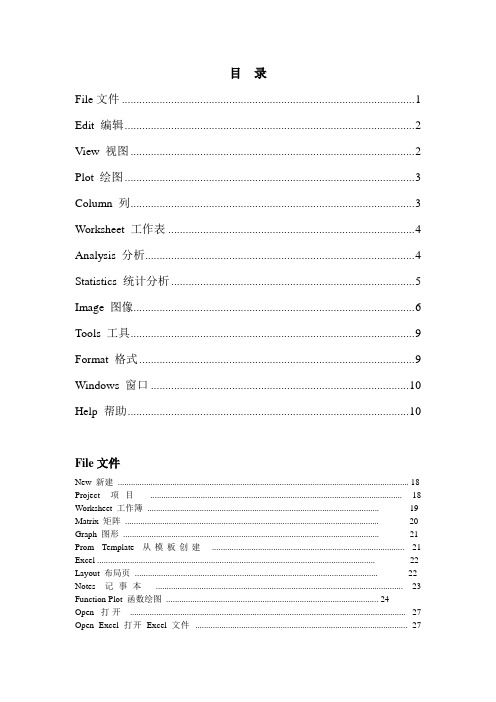
目录File文件 (1)Edit 编辑 (2)View 视图 (2)Plot 绘图 (3)Column 列 (3)Worksheet 工作表 (4)Analysis 分析 (4)Statistics 统计分析 (5)Image 图像 (6)Tools 工具 (9)Format 格式 (9)Windows 窗口 (10)Help 帮助 (10)File文件New 新建 (18)Project项目 (18)Worksheet 工作簿 (19)Matrix 矩阵 (20)Graph 图形 (21)Prom Template 从模板创建 (21)Excel (22)Layout 布局页 (22)Notes 记事本 (23)Function Plot 函数绘图 (24)Open 打开 (27)Open Excel 打开Excel文件 (27)Open Sample OPJ 打开示例项目 (28)Append 追加 (28)Close 关闭 (28)Save Project 保存项目 (28)Save Project As 项目另存为 (28)Save Window As 窗口另存为 (29)Save Template As 模板另存为 (29)Save Workbook as Analysis Template 工作簿另存为分析模板 (30)Save Project as Analysis Template项目另存为分析模板 (30)Import 导入 (30)Import Wizard 导入向导 (31)Customize 定制............................................................................................................. .. (31)Export 导出 (33)Batch Processing 批处理 (34)Database Import数据库导入 (35)Edit 编辑Copy (full precision) 精确复制 (37)Copy (including label rows) 带标识符行复制 (37)Paste Transpose 转置粘贴 (37)Paste Link 粘贴链接 (38)Paste Link Transpose粘贴链接转置 (38)Paste Special 特殊粘贴 (38)Merge (Embedded) Graphs 合并(嵌入)图形 (39)Button Edit Mode 按钮编辑模式 (39)View 视图Toolbars 工具栏 (41)Status Bar 状态栏 (41)Command Window 命令窗口 (42)Code Builder 代码创建器 (42)Quick Help 快速帮助 (43)Project Explorer 项目浏览器 (43)View Windows 视窗 (43)Results Log 结果记录 (44)View Mode (45)Messages Log 信息记录 (46)Actively Update Plots 即时更新图形 (46)Page Break Preview Lines 分页预览线 (46)Print View打印视图 (47)Page 页面视图 (47)Window 窗口视图 (47)Zoom In 放大 (48)Zoom Out 缩小 (49)Whole Page 满页 (49)Show 显示 (49)Show Data Information 显示数据信息 (51)Data Mode 数据模式 (52)Show Column/Row 显示列/行 (52)Show X/Y 显示X/Y (53)Image Mode 图像模式 (53)Show Image Thumbnails 显示缩略图 (53)Plot 绘图Line 线条 (54)Symbol 符号 (55)Line + Symbol 线条+符号 (56)Column/Bar/Pie 柱/条/饼 (56)Multi-Curve 多曲线 (57)3D XYY 三维XYY图 (59)3D XYZ 三维XYZ图 (60)3D Surface 三维曲面图 (60)3D Wire/Bar/Symbol 三维线/柱/符号 (61)Statistics 统计 (62)Area 面积 (65)Contour 等高线图 (65)Specialized 专业图 (66)Stock 股票 (68)Template Library 模板库 (70)Column 列Set as X 设为X (72)Set as Label 设为标记符号 (72)Disregard Column 忽略此列 (73)Set as Y Error 设为Y的误差 (73)Set as Categorical 设为分类数据 (73)Setting Column Values 设定列数值 (74)Fill Column with 填充列 (74)Add New Columns 添加新列 (75)Set Sampling Interval 设置采样间隔 (76)Move Columns 移动列 (76)Show X Column 显示X列 (77)Slide Show of Dependent Graphs 幻灯放映 (77)Add Sparklines 添加拆线图 (79)Worksheet 工作表Sort Range 排序范围 (80)Sort Columns列排序 (81)Sort Worksheet 工作表排序 (81)Clear Worksheet 清除工作表 (81)Worksheet Script 工作表脚本 (81)Worksheet Query 工作表查询 (82)Reset Column Short Names 重置列短名 (83)Split Worksheet 拆分工作表 (84)Split Workbooks 拆分工作簿 (84)Pivot Table 数据透视表 (84)Stack Column 堆列 (85)Unstack Column 拆堆列 (87)Remove Duplicated rows 删除重复行 (87)Reduce Rows 减少行 (88)Transpose 转置 (89)Convert to XYZ 转换为XYZ (90)Convert to Matrix转换为矩阵 (93)Analysis 分析Mathematics 数学运算 (97)Interpolate/Extrapolate Y from X 由X内插/外推求Y (98)Trace Interpolate 迹线外推 (98)Interpolate/Extrapolate 内插/外推 (99)3D Interpolation 三维内插 (100)XYZ Trace Interpolation XYZ 迹线内插 (101)XYZ Surface Area XYZ表面积 (102)Set Column Values 设置列值 (102)Normalize Column. 归一化列 (102)Simple Math 简单数学运算 (103)Differetiate 微分 (104)Integrate 积分 (105)Polygon Area 多边形面积 (105)Average Multiple Curves 多曲线平均 (106)Data Manipulation 数据处理 (106)Subtract Reference Data 与参考值相减 (107)Reduce Duplicate X data 归纳重复的X数据 (108)Reduce by Group 按组归纳 (108)Reduce to Evenly Spaced X 归纳为均匀间隔的X (110)Linear Fit 线性拟合 (112)Fit Linear with X Error 带X误差的线性拟合 (114)Polynomial Fit 多项式拟合 (115)Multiple Linear Regression所谓多元线性回归 (115)Nonlinear Curve Fit 非线性曲线拟合 (117)Nonlinear Surface Fit 非线性表面拟合 (118)Simulate Curve 模拟曲线 (118)Simulate Surface 模拟表面 (119)Exponential Fit 指数拟合 (121)Sigmoidal Fit 反曲拟合 (122)Compare Datasets 比较数据集 (122)Compare Models 比较模型 (123)Signal Processing 信号处理 (125)Smooth 平滑 (125)FFT Filters FFT滤波 (126)FFT (127)Wavelet 小波分析 (129)Convolution 卷积 (132)Deconvolution 反卷积 (133)Coherence 相干性 (134)Correlation 相关性 (135)Hibert Transform希尔伯特变换 (135)Envelope 包络线 (136)Decimation 抽取 (136)Peaks and Baseline 峰和基线 (137)Multiple Peak Fit 多重峰拟合 (138)Single Peak Fit 单峰拟合 (141)Peak Analyzer 峰分析器 (141)Batch Peak Analysis Using Theme 使用主题批量分析 (147)Statistics 统计分析Descriptive Statistics 描述统计 (149)Correlations Coefficient 相关系数 (149)Statistics on Columns 列统计 (151)Statistics on Rows 和统计 (152)Discrete Frequency 离散频率 (154)Frequency Counts 频率计数 (155)Normality Test 正态性检验 (157)2D Frequency Counts/Binning 二维频率计数/分区 (159)Hypothesis Testing 假设检验 (160)One-Sample t-Test 单样本t-检验 (161)Pair-Sample t-Test 配对样本t-检验 (162)Two-Sample t-Test 双样本t-检验 (164)One-Sample Test for Variances 单样本方差检验 (167)Two-Sample Test for Variances 双样本方差检验 (169)ANOV A 方差分析 (170)One-Way ANOV A 单因素方差分析 (170)Two-Way ANOV A 双因素方差分析 (172)One-Way Repeated Measures ANOVA 单因素重复测量方差分析 (174)Two-Way Repeated Measures ANOV A 双因素重复测量方差分析 (176)NonParametric Tests 非参数检验 (178)One-Sample Wilcoxon Signed Rank Test单样本Wilcoxon符号秩检验 (179)Paired Sample Sign Test 配对样本符号检验 (180)Paired Sample Wilcoxon Signed Rank Test配对样本Wilcoxon符号秩检验 (181)Two-Sample Kolmogorov-Smirnov Test 双样本KS检验 (183)Mann-Whitney Test Mann-Whitney检验 (185)Kruskal-Wallis ANOV AKruskal-Wallis方差分析 (187)Moods Median Test 中位数检验 (188)Friedman ANOV A Friedman方差分析 (189)(NPH) K independent Samples K个独立样本 (191)(NPH) Paired Samples 配对样本 (193)(NPH) Two Independent Samples 两个独立样本 (195)Survival Analysis 生存分析 (197)Kaplan-Meier Estimator卡普兰-迈耶估计量 (198)Cox Model Estimator比例风险模型 (199)Weilbull fit Weibull拟合 (201)Multivariate Analysis 多元分析 (203)Principal Component Analysis 主成分分析 (203)K-Means Cluster Analysis K-均值聚类分析 (204)Hierarchical Cluster Analysis 层次聚类分析 (206)Discriminant Analysis 判别分析 (208)Power and Sample Size 功效和样本大小分析 (212)(PSS) One-Sample t-test 单样本t-检验 (212)(PSS) Two-Sample t-test 双样本t-检验 (213)(PSS)Paired t-test 配对样本t-检验 (214)(PSS)One-Way ANOV A 单因素方差分析 (215)ROC Curve受试者工作特征曲线 (216)Image 图像Image adjustments 图像调整 (220)Brightness 亮度 (221)Contrast 对比度 (221)Gamma伽玛值 (221)Hue 色调 (222)Invert 图像色彩翻转 (222)Saturation 饱和度 (223)Histcontrast 直方图对比度 (223)Histequalize 直方图均衡 (223)Auto Level 自动色阶 (224)Color Level 色阶调整 (224)Function LUT 函数搜寻表 (225)Leveling 层次调整 (226)Balance 色彩平衡 (227)Color Replace 颜色替换 (228)Arithmetic transformation 算术变换 (228)Alpha Blend 透明混合 (229)Simple Math 简单数学运算 (230)Math Function 数学函数 (232)Pixel Logic 像素逻辑 (233)Subtract Background 减背景 (234)Extract to XYZ 图像转数据 (235)Morphological Filter 形态学过滤 (236)Replace Background 背景替换 (238)Subtract Interpolated Background 内插背景减影 (238)Conversion 转换 (239)Convert to Data 转成数值 (239)Color to Gray 转换成灰度图....................................................................................... 240 Convert to Image 转换成图像. (241)Binary 转换成二值图 (242)Dynamic Binary 转换成动态二值图 (243)Threshold 阈值 (244)RGB Merge 三原色合并 (245)RGB Splist 三原色拆分 (246)Image Scale 图像比例尺 (247)Geometric Transforms 几何变换 (248)Flip 翻转 (248)Rotate 旋转 (248)Shear 修剪 (249)Resize 调整尺寸 (251)AutoTrim 自动修整 (251)Offset 图像偏移 (252)Spatial Filtering 空间过滤 (254)Average 平均过滤 (255)Gaussian 高斯过滤 (255)Median 中值过滤 (256)Noise 噪音 (257)Edge 边缘.................................................................................................................... 258 Sharpen 锐化................................................................................................................ 258 Unsharpen Mask USM 锐化. (259)User Filter 用户滤镜 (260)Graph 图形 (262)Layer Contents 图层内容 (262)Plot Setup 绘图设置 (263)Add Plot to Layer 层中加图 (263)Add Error Bars 添加误差条 (264)Add Function Graph 添加函数曲线 (265)Rescale to Show All 重置坐标显示全部 (266)Layer Management 图层管理 (266)Add Axis Scrollbar 添加坐标滚动条 (266)New Layer (Axes) 新图层(坐标) (267)Extract to Graphs 提取图层 (268)Apply Palette to Color Map 应用调色板 (270)Merge Graph Windows (271)Speed Mode (271)Update Legend 更新图例 (272)New Legend 新图例 (273)New Enhanced Legend (273)New Table (274)New Color Scale (274)New XY Scaler 新XY比例尺............................................................................................ 275 Set Active Layer By Layer Icon Only ................................................................................... 275 Fit Layer to Graph 图层适合图形....................................................................................... 276 Fit Page to Layers 页面适合图层. (276)Exchange X-Y Axes 交换X-Y坐标 (277)Offset Grouped Data in Layer 偏移图层中的分组数据 (277)Convert to Standard Font Size (277)Data 数据 (278)Set Display Range 设置显示范围 (278)Reset to Full Range 显示全部数据 (280)Mark Data Range 标记数据范围 (280)Clear Data Markers 清除数据标记 (280)Analysis Marker 分析标记 (281)Lock Position 锁定位置 (282)Mask Data Point 给数据点加蒙板 (282)Move Data Point 移动数据点 (283)Remove Bad Data Points 清除坏数据 (285)Gadget 小工具 (285)Quick Fit 快速拟合 (285)Quick Sigmoidal Fit 快速反曲拟合 (287)Quick Peaks 快速峰拟合 (288)Rise Time 上升时间 (289)Cluster 聚类 (289)Statistics (291)Differentiate 微分 (292)Integrate 积分 (293)Interpolate 内插 (293)Intersect 交叉点 (294)FFT 快速傅立叶变换 (295)Vertical Cursor 垂直光标 (295)Layout 布局 (296)Add Graph 添加图形 (297)Add Worksheet 添加工作 (298)Set Picture Holder 开启图像定位 (298)Clear Picture Holder 清除图像定位 (299)New Table 新表格 (299)Global Speed Control 全局速度控制 (300)Tools 工具Options 选项 (301)System Variables 系统变量 (302)Protection 保护 (303)Fitting Function Builder 拟合函数创建器 (304)Fitting Function Organizer 拟合函数管理器 (305)Template Library 模板库 (306)Theme Organizer 主题管理 (306)Import Filters Manager 滤镜导入管理器 (307)Package Manager 包管理器 (308)Customer Menu Organizer 用户菜单管理器 (308)x-Function Builder x-函数创建器 (309)x-Function Script Samples x-函数脚本样本 (309)Copy Origin Sub-VI to LabVIEW User.lib (310)Set Group Folder Location 设置组文件夹位置 (310)Group Folder Manager 组文件夹管理器 (311)Transfer User Files 传递用户文件 (311)Pallet Editor 调色板编辑器 (312)Digitizer 数字转换器 (312)MATLAB Console MATLAB控制台 (313)Mathematica Link 连接Mathematica (314)Format 格式Menu 菜单 (315)Worksheet 工作表 (315)Column 列 (316)Cell 单元格 (317)Snap to Grid 对齐栅格 (317)Programming Control 编程管理 (318)Object Properties 对象属性 (319)Page Properties 页面属性 (320)Layer Properties 图层属性 (320)Plot Properties 图形属性 (321)Snap Layer to Grid 图层对齐栅格 (321)Snap Objects to Grid 对象对齐栅格 (321)Axes 坐标 (321)Axis Tick Labels 坐标刻度 (323)Axis Titles 坐标名称 (323)Windows 窗口Cascade 层叠窗口 (324)Tile Horizontally 水平显示 (324)Tile Vertically 垂直显示 (325)Arrange Ions 排列图标 (325)Refresh 刷新 (325)Duplicate副本 (326)Split 分割 (326)Properties 属性 (327)Command Window 命令窗口 (327)Script Window 脚本窗口 (328)Help 帮助Origin Toolbars 工具栏 (329)Standard 标准 (329)Edit 编辑 (330)Graph 图形 (330)2D Graph 二维图形 (331)3D and Contour Graph 三维图和等高线图 (333)3D Rotation 三维旋转 (334)Worksheet Data 工作表数据 (334)Column 列 (335)Layout 布局 (335)Mask 蒙板 (336)Tools 工具 (336)Object Edit 对象编辑 (337)Arrow 箭头 (338)Style 样式 (338)Format 格式 (339)Auto Update 自动更新 (339)Database 数据库 (340)Markers & Locks 标记和锁定 (340)。
功能语言学视角的翻译质量评估模式与诗歌翻译质量评估
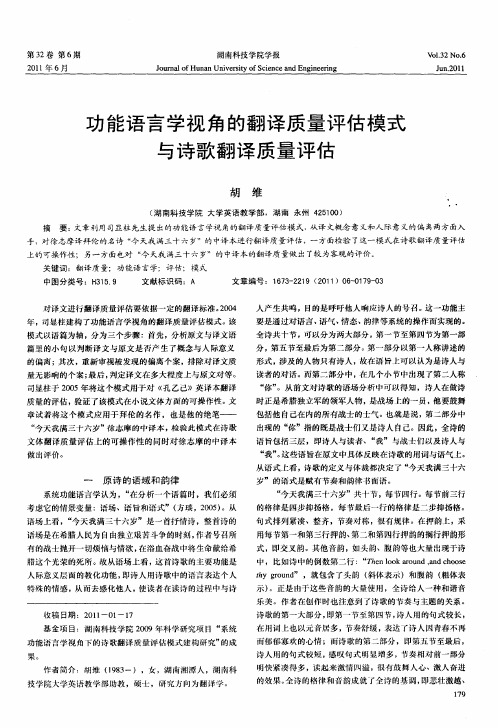
译文 :恋爱 的灵感与苦痛与蜜 甜,
An p d owe e a rofl ,Ic nno ha e ov ts r ,
新信息依次出现 ,先是刀剑、军旗,再是整个战场 ,然后 目 光投向远 方,似乎看见荣光映照下的希腊 , 犹如镜头 由远及 近, 给人身临其境 的感觉。 但译文没有保留原文的主位结构,
腊 这 个 光 荣 的死 所 。故从 语 场 上 看 , 首 诗 歌 的主 要 功 能 是 这 人 际 意义 层 面 的教 化 功 能 , 即诗 人 用诗 歌 中 的语 言 表 达 个 人
旬 式排列紧凑 、整齐,节奏对称 ,很有规律 。在押 韵上 ,采 用每 节第一和第三行押韵、 第二和第四行押韵的搁行押韵形
的偏离 ;其次 ,重新审视被发现的偏离个案 ,排除对 泽文质 量无 影响的个案 ; 后, 最 判定译文在多大程度上与原文对 等。 司显柱于 2 0 0 5年将这个模式用于 对 孔乙 已 英译 本翻译 质量 的评估 , 验证 了该模式在小说文体 方面的可操作性 。 文 章试着将这个模 式应 用于 拜伦 的名 作 ,也是 他的绝笔——
全 诗 共 十 节 , 以分 为 两 大 部 分 , 一 节 至第 四 节 为第 一 部 可 第
模式 以语篇为轴 , 分为三个步骤 :首先 ,分析原文 与译文语
篇里 的小 旬 以 判 断 译 文 与原 文 是 否 产 生 了概 念 与 人 际意 义
分, 第五节至最后为第二部分。第一部分以第 一人称讲述的 形式 , 涉及 的人物只有诗人 , 故在语 旨上可以认为 是诗人与 读者 的对话 。而第二部分中 , 在几个小节中出现了第二人称 “ 。从前文对 诗歌的语场分析 中可以得 知,诗人在做诗 你” 时正是希腊独立军的领军人物,是战场 上的一员, 他要鼓舞
multivariate statistical analysis
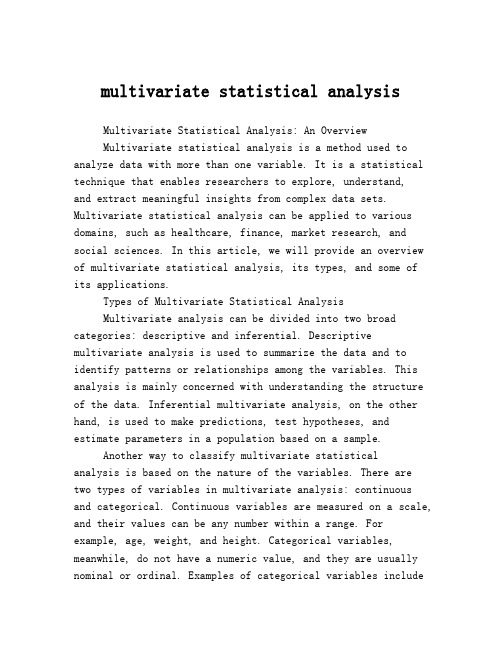
multivariate statistical analysisMultivariate Statistical Analysis: An OverviewMultivariate statistical analysis is a method used to analyze data with more than one variable. It is a statistical technique that enables researchers to explore, understand,and extract meaningful insights from complex data sets. Multivariate statistical analysis can be applied to various domains, such as healthcare, finance, market research, and social sciences. In this article, we will provide an overview of multivariate statistical analysis, its types, and some ofits applications.Types of Multivariate Statistical AnalysisMultivariate analysis can be divided into two broad categories: descriptive and inferential. Descriptive multivariate analysis is used to summarize the data and to identify patterns or relationships among the variables. This analysis is mainly concerned with understanding the structure of the data. Inferential multivariate analysis, on the other hand, is used to make predictions, test hypotheses, and estimate parameters in a population based on a sample.Another way to classify multivariate statisticalanalysis is based on the nature of the variables. There are two types of variables in multivariate analysis: continuous and categorical. Continuous variables are measured on a scale, and their values can be any number within a range. For example, age, weight, and height. Categorical variables, meanwhile, do not have a numeric value, and they are usually nominal or ordinal. Examples of categorical variables includegender, marital status, and education level.Applications of Multivariate Statistical AnalysisMultivariate statistical analysis is a valuable tool in various fields. Here are some examples:1. Healthcare: Multivariate analysis is used to identify risk factors associated with disease and to analyze the effectiveness of treatments. For example, researchers can use multivariate analysis to investigate the relationship between smoking, age, gender, and lung cancer incidence.2. Market research: Multivariate analysis is used to segment customers, to identify their preferences, and to understand their behavior. For example, multivariate analysis is used to cluster customers based on their purchasing habits, demographic characteristics, and lifestyle.3. Finance: Multivariate analysis is used to analyze financial data and to make investment decisions. For example, analysts can use multivariate analysis to identify thefactors that affect stock prices or to evaluate the performance of a portfolio.4. Social sciences: Multivariate analysis is used to investigate the relationships among variables in social sciences. For example, researchers can use multivariate analysis to study the relationship between income, education level, and job satisfaction.In conclusion, multivariate statistical analysis is a powerful method for analyzing complex data sets. It is usedto identify relationships among variables, to understand the structure of the data, and to make predictions. Multivariate analysis is applicable to various domains and is a valuable tool for researchers, professionals, and decision-makers. Ifyou are dealing with complex data sets, multivariate analysis might be the right approach for you.。
多维标度法

多维标度法内容丰富、方法较多。 按相似性(距离)数据测量尺度的不同MDS可分为: 度量MDS:当利用原始相似性(距离)的实际数值为间隔尺 度和比率尺度时称为度量MDS(metric MDS) 非度量MDS:当利用原始相似性(距离)的等级顺序(即有 序尺度)而非实际数值时称为非度量MDS(nonmetric MDS) 按相似性(距离)矩阵的个数和MDS模型的性质MDS可分
2
Shepard和Kruskal等人进一步加以发展完善。多维标度法 现在已经成为一种广泛用于心理学、市场调查、社会学、物 理学、政治科学及生物学等领域的数据分析方法。 多维标度法解决的问题是:当n个对象(object)中各对对象 之间的相似性(或距离)给定时,确定这些对象在低维空间 中的表示(感知图Perceptual Mapping),并使其尽可能与 原先的相似性(或距离)“大体匹配”,使得由降维所引起 的任何变形达到最小。多维空间中排列的每一个点代表一个 对象,因此点间的距离与对象间的相似性高度相关。也就是 说,两个相似的对象由多维空间中两个距离相近的点表示, 而两个不相似的对象则由多维空间两个距离较远的点表示。 多维空间通常为二维或三维的欧氏空间,但也可以是非欧氏 三维以上空间。
整数 r 和 R r 中的 n 个点 X1 , X 2 ,
2 dij ( X i X j )( X i X j )
, X n ,使得
i, j 1,2,
,n
则称 D 为欧氏距离阵 3.相似系数阵
定义 10.3 一个 n n 阶的矩阵 C (cij )nn ,如果满足条件:
定义10.1 一个n n阶的矩阵D=(dij ) n n ,如果满足条件:
8
( 1) D D ( 2) dij 0, dii 0,
- 1、下载文档前请自行甄别文档内容的完整性,平台不提供额外的编辑、内容补充、找答案等附加服务。
- 2、"仅部分预览"的文档,不可在线预览部分如存在完整性等问题,可反馈申请退款(可完整预览的文档不适用该条件!)。
- 3、如文档侵犯您的权益,请联系客服反馈,我们会尽快为您处理(人工客服工作时间:9:00-18:30)。
Tiivistelmä: Sijaintiongelmissa tehtävänä on sijoittaa optimaalinen määrä palvelupisteitä tarkasteltavalle alueelle sekä jakaa asiakkaat näihin palvelupisteisiin. Tarkasteltava sijaintialue voi myös koostua pienemmistä osa-alueista. Optimoinnin tavoitteena voi olla esimerkiksi kustannusten minimointi tai voiton maksimointi, ja optimointitehtävä voi myös sisältää erilaisia rajoituksia. Sijaintiongelmalla voi olla useita mahdollisia skenaarioita, jotka vastaavat esimerkiksi erityyppisiä budjettirajoitteita, kustannusfunktioita tai markkina-analyysejä. Tämän työn painopisteenä ovat sijaintiongelmat vähittäiskaupassa ja aihealueelta esitellään myös yksi havainnollistava esimerkki. Kun tavoitteena on löytää paras sijainti-allokaatiovaihtoehto, on välttämätöntä verrata erilaisille skenaarioille tuotettuja ratkaisuja. Tämä työn ensimmäinen tavoite on määritellä erityisesti vähittäiskaupan sijaintiongelmiin soveltuvia päätöskriteerejä. Ehdotettujen kriteerien joukossa on sekä taloudellisia että asiakasvirtoja ja palveluverkon ominaisuuksia luonnehtivia attribuutteja. Palvelupisteiden sijaintien ja asiakasvirtojen lisäksi sijaintiongelman ratkaisuille voidaan johtaa erilaisia lisäominaisuuksia, kuten esimerkiksi saavutettu markkinaosuus, palvelupisteiden etäisyys kilpailijoihin tai tyydyttämättömän kysynnän määrä. Myös määritellyt päätöskriteerit kuuluvat näihin johdettuihin ominaisuuksiin. Kun sijaintialue on jaettu osa-alueisiin, tarkasteltavat attribuutit saavat maantieteellisen ulottuvuuden. Vaihtoehtojen ominaisuuksien visualisointi on usein päätöksenteon kannalta tärkeää, ja siksi sopivien visualisointimenetelmien löytäminen on työn toinen päätavoite. Paikkatietojärjestelmiä voidaan käyttää avuksi visualisoidessa vähittäiskaupan sijaintiongelmien maantieteellisiä ominaisuuksia. Paikkatietojärjestelmien sisältämät työkalut eivät kuitenkaan ole riittäviä, kun tehtävänä on visualisoida samanaikaisesti useita monidimensioisia sijaintiratkaisuja. Ongelma voidaan kuitenkin osittain ratkaista tarkastelemalla vaihtoehtojoukon tilastollisia ominaisuuksia yksittäisten ratkaisujen sijasta. Toinen vaihtoehto on jakaa ratkaisujoukko esimerkiksi ryhmittelyanalyysin avulla osajoukkoihin, joiden ominaisuuksia voidaan verrata. Tämän lisäksi pääkomponenttianalyysi voi helpottaa visualisointia vähentämällä tarkasteltavien muuttujien määrää. Sivumäärä: 86 Avainsanat: sijaintiongelma, sijainti-allokaatio-ongelma, vähittäiskauppa, visualisointi, paikkatietojärjestelmä Täytetään osastolla Hyväksytty: Kirjasto:
HELSINKI UNIVERSITY OF TECHNOLOGY Author: Emilia Suomalainen
ABSTRACT OF MASTER’S THESIS
Department: Department of Engineering Physics and Mathematics Major subject: Systems and Operations Research Minor subjects: Environmental Strategies and Technology Assessment, Reliability and Environment Title: Multicriteria Analysis and Visualization of Location-Allocation Problems Chair: Mat-2 Applied Mathematics Supervisor: Professor Ahti Salo Instructors: Ulrich Bartling, Dr. Angi Voss
TEKNILLINEN KORKEAKOULU Tekijä: Emilia Suomalainen Osasto: Teknillisen fysiikan ja matematiikan osasto Pääaine: Systeemi- ja operaatiotutkimus
DIPLOMITYÖN TIIVISTபைடு நூலகம்LMÄ
Sivuaineet: Ympäristöstrategiat ja teknologian arviointi, Reliability and Environment Työn nimi: Monitavoiteoptimointi ja visualisointi sijaintiongelmien ratkaisussa Title in English: Multicriteria Analysis and Visualization of Location-Allocation Problems Professuurin koodi ja nimi: Mat-2 Sovellettu matematiikka Työn valvoja: Professori Ahti Salo Työn ohjaajat: Ulrich Bartling, Dr. Angi Voss
Abstract: In a location-allocation problem (LAP), an optimal number of facilities has to be placed in an area of interest in order to satisfy the customer demand. The optimization objective can be, e.g., to minimize costs or to maximize profit and the situation can be characterized by various constraints. The total location area may also be divided into regions which have each their special characteristics. There are often several plausible scenarios for an LAP representing, for example, different budgets limitations, cost functions, or estimates for market growth. In this Thesis, the emphasis is on retail location-allocation problems and it is illustrated with a case study in retailing. When dealing with a large number of location-allocation solutions for various scenarios, it is necessary to examine which option is the best one according to specified criteria. The first objective of this Thesis is therefore to define decision criteria for retail applications. The proposed criteria include several monetary, facility related, and customer related attributes. Besides the facility locations and customer flows, location-allocation solutions are characterized by various derived properties, for example market share, distance to competitors, and the amount of unassigned demand. These derived properties also include the chosen decision criteria. When the total location area is composed of regions, these properties can be expressed for each region and thus have a spatial distribution. In decision support, it is often important to be able to visualize the solutions and their derived properties: finding suitable visualization techniques is also the second main objective of this Thesis. The visualization of geographic properties can be assisted by geographic information systems (GIS). However, existing tools of GIS software are not sufficient for the visualization of dozens of multi-dimensional location-allocation solutions. This problem can be circumvented either by concentrating on the statistical properties of the solution set instead of examining and comparing the options one by one, or by trying to classify the solutions into groups whose properties can then be more easily compared. The methods of cluster analysis and subgroup discovery could prove useful in grouping the solutions. Also, principal component analysis can be used to reduce the dimensions of the problem and to facilitate visualization. Number of pages: 86 Keywords: location-allocation, facility location, retail, visualization, geographic information system Department fills Approved: Library code: