Big-Data Architecture for Cyber Attack Graphs
Cyber attack-resilient control for smart grid
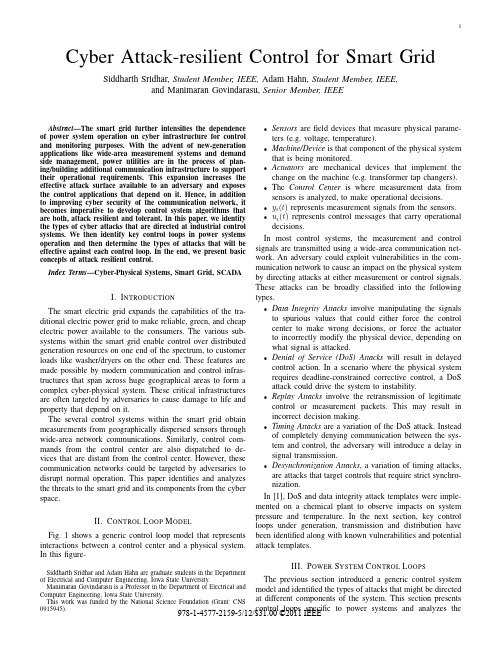
Cyber Attack-resilient Control for Smart Grid Siddharth Sridhar,Student Member,IEEE,Adam Hahn,Student Member,IEEE,and Manimaran Govindarasu,Senior Member,IEEEAbstract—The smart grid further intensifies the dependence of power system operation on cyber infrastructure for control and monitoring purposes.With the advent of new-generation applications like wide-area measurement systems and demand side management,power utilities are in the process of plan-ing/building additional communication infrastructure to support their operational requirements.This expansion increases the effective attack surface available to an adversary and exposes the control applications that depend on it.Hence,in addition to improving cyber security of the communication network,it becomes imperative to develop control system algorithms that are both,attack resilient and tolerant.In this paper,we identify the types of cyber attacks that are directed at industrial control systems.We then identify key control loops in power systems operation and then determine the types of attacks that will be effective against each control loop.In the end,we present basic concepts of attack resilient control.Index Terms—Cyber-Physical Systems,Smart Grid,SCADAI.I NTRODUCTIONThe smart electric grid expands the capabilities of the tra-ditional electric power grid to make reliable,green,and cheap electric power available to the consumers.The various sub-systems within the smart grid enable control over distributed generation resources on one end of the spectrum,to customer loads like washer/dryers on the other end.These features are made possible by modern communication and control infras-tructures that span across huge geographical areas to form a complex cyber-physical system.These critical infrastructures are often targeted by adversaries to cause damage to life and property that depend on it.The several control systems within the smart grid obtain measurements from geographically dispersed sensors through wide-area network communications.Similarly,control com-mands from the control center are also dispatched to de-vices that are distant from the control center.However,these communication networks could be targeted by adversaries to disrupt normal operation.This paper identifies and analyzes the threats to the smart grid and its components from the cyber space.II.C ONTROL L OOP M ODELFig.1shows a generic control loop model that represents interactions between a control center and a physical system. In thisfigure-Siddharth Sridhar and Adam Hahn are graduate students in the Department of Electrical and Computer Engineering,Iowa State University. Manimaran Govindarasu is a Professor in the Department of Electrical and Computer Engineering,Iowa State University.This work was funded by the National Science Foundation(Grant:CNS 0915945).•Sensors arefield devices that measure physical parame-ters(e.g.voltage,temperature).•Machine/Device is that component of the physical system that is being monitored.•Actuators are mechanical devices that implement the change on the machine(e.g.transformer tap changers).•The Control Center is where measurement data from sensors is analyzed,to make operational decisions.•y i(t)represents measurement signals from the sensors.•u i(t)represents control messages that carry operational decisions.In most control systems,the measurement and control signals are transmitted using a wide-area communication net-work.An adversary could exploit vulnerabilities in the com-munication network to cause an impact on the physical system by directing attacks at either measurement or control signals. These attacks can be broadly classified into the following types.•Data Integrity Attacks involve manipulating the signals to spurious values that could either force the control center to make wrong decisions,or force the actuator to incorrectly modify the physical device,depending on what signal is attacked.•Denial of Service(DoS)Attacks will result in delayed control action.In a scenario where the physical system requires deadline-constrained corrective control,a DoS attack could drive the system to instability.•Replay Attacks involve the retransmission of legitimate control or measurement packets.This may result in incorrect decision making.•Timing Attacks are a variation of the DoS attack.Instead of completely denying communication between the sys-tem and control,the adversary will introduce a delay in signal transmission.•Desynchronization Attacks,a variation of timing attacks, are attacks that target controls that require strict synchro-nization.In[1],DoS and data integrity attack templates were imple-mented on a chemical plant to observe impacts on system pressure and temperature.In the next section,key control loops under generation,transmission and distribution have been identified along with known vulnerabilities and potential attack templates.III.P OWER S YSTEM C ONTROL L OOPSThe previous section introduced a generic control system model and identified the types of attacks that might be directed at different components of the system.This section presents control loops specific to power systems and analyzes the978-1-4577-2159-5/12/$31.00 ©2011 IEEEFig.1:A Typical Power System Control Loopimpact of the defined attacks on these loops.Fig.2classifies key power system control loops into generation,transmission and distribution,and identifies the impact caused by specific attacks on these control loops.The automatic voltage regulator and governor control schemes are local control schemes that do not rely on SCADA telemetry for operation.Hence,attacks such as integrity,DoS, replay,timing and desynchronization are not applicable in this case.Malware attacks,attacks that are a result of infected control modules of the system,can have an impact on all the control loops in the system.The Automatic Generation Control(AGC)loop is vul-nerable to data integrity attacks as the Area Control Error (ACE),or the generation correction,that is calculated depends on these measurements.This attack was demonstrated in [2]where the attack resulted in abnormal system operating conditions.The DoS attack need not necessarily have an impact on AGC as it is only effective if implemented when AGC is operational.Timing attacks will have an impact on AGC as delayed dispatch of the generator correction could result in unstable operating conditions.Also,replay attacks will also have an impact as the ACE will be calculated based on old system state.Desynchronization attacks can be targeted on AGC operation by forcing ACE to be calculated based on frequency and tie-line measurements from different time instances.State Estimation need not necessarily be impacted by data integrity,DoS,replay and timing attacks as the algorithm performs computations even in the presence of corrupt data or in the absence of measurements from certain number of meters.However,in[3],the authors have shown that state estimation can be impacted by data integrity attacks.V AR compensation is done by Coordinating Flexible AC Transmission Systems Devices(CFDs)-Flexible AC Trans-mission Systems(FACTS)devices that communicate with one another to exchange operational information and determine setpoints.The communication is critical to stable operation of CFDs and hence,any attack on this will impact V AR compensation operation.In[4],the impact of data injection attacks on CFDs is presented.Phasor measurement unit-based wide-area measurement systems(W AMS)are being widely deployed in the United States.Currently,measurements provided by PMUs are not being used for real-time control.However in[5],PMU-based real-time control schemes that include HVDC and FACTS control are proposed.All attack types will have an impact on such W AMS-based applications.Load shedding is performed at the distribution level to prevent blackout-type scenarios in the system.During such contingencies,under-frequency relays are triggered and the distributed feeder opened.Modern relays support communica-tion protocols such as IEC61850over Ethernet.An attack on the relay communication infrastructure or a malicious change to the control logic could result in unexpected tripping of distribution feeders,leaving load segments unserved.DoS, Timing and Replay attacks might not necessarily have an impact on this control loop as it is operational only during rare contingencies.De-sync attacks are not applicable in this case.The Smart Meter-based Advanced Metering Infrastructure (AMI)is currently undergoing ing smart meters,utilities will be able to disable customer loads when the system demand is too high and reschedule them to hours when wind energy is available.This feature is called demand side management[6].The introduction of vast communication in-frastructure at the residential level presents adversaries with a huge attack surface.Integrity attacks on the AMI infrastructure may result in unexpected operation of loads leading to system stability problems.DoS and timing-based attacks may prevent utilities from having precise control over loads.Malware in AMI and associated infrastructure could potentially lead to loss in privacy,and unexpected operation of domestic devices.IV.A TTACK R ESILIENT C ONTROLState estimation has been the traditional technique for bad data detection in power systems.As the attackers become more sophisticated and as more resources are available to them, legacy attack detection techniques might not be sufficient.To detect attacks of the type described in the previous section, advanced cyber defense techniques that incorporate power system concepts have to be developed.Attack resilient control algorithms provide defense in depth to the control system by adding security at the applicationFig.2:Power Applications and Attack Scenarioslayer.Hence,Domain-specific anomaly detection and intrusion tolerance algorithms that are able to classify measurements and commands as good/bad have to be implemented.The defense algorithms must be developed with the assumption that the attacker has knowledge of system operation.As a basic test,the algorithm should perform a range check to see if the obtained measurements lie within acceptable values.Additional tests that are based on forecasts,histor-ical data and engineering sense should also be devised to ascertain the current state of the system.In most cases,the physical parameters of the system (e.g.generator constants)are protected by utilities.These parameters play a part in determining the state of the system and system response to an event.Hence,algorithms that incorporate such checks could help in identifying malicious data when an attacker attempts to mislead the operator into executing incorrect commands.Intelligent power system control algorithms that are able to keep the system within stability limits during contingencies are critical.Additionally,the development of enhanced power management systems capable of addressing high-impact con-tingency scenarios.V.C ONCLUSIONIn this paper,the importance of cyber security in the smart grid environment was highlighted.The types of attacks targeted at control systems were identified and their impacts on specific power system control loops were analyzed.These attacks can have a wide range of impacts ranging from system stability to financial impacts.Attack resilient controls are key to securing the smart grid.Traditional cyber security hardware and software have to be backed by such attack detection algorithms at the application layer,to further intensify security.R EFERENCES[1]Y .-L.Huang, A. A.Cardenas,S.Amin,Z.-S.Lin,H.-Y .Tsai,andS.Sastry,“Understanding the physical and economic consequences ofattacks on control systems,”International Journal of Critical Infrastruc-ture Protection ,vol.2,no.3,pp.73–83,2009.[Online].Available:/science/article/pii/S1874548209000213[2]S.Sridhar and G.Manimaran,“Data integrity attacks and their impacts on SCADA control system,”in Power and Energy Society General Meeting,2010IEEE ,Jul.2010,pp.1–6.[3]Y .Liu,P.Ning,and M.K.Reiter,“False data injection attacks against state estimation in electric power grids,”in Proceedings of the 16th ACM conference on Computer and communications security ,S ’09.New York,NY ,USA:ACM,2009,pp.21–32.[4]S.Sridhar and G.Manimaran,“Data integrity attack and its impacts on voltage control loop in power grid,”in Power and Energy Society General Meeting,2011IEEE ,Jul.2011.[5] A.Phadke and J.S.Thorp,Synchronized Phasor Measurements and Their Applications ,2008.[6]D.Callaway and I.Hiskens,“Achieving controllability of electric loads,”Proceedings of the IEEE ,vol.99,no.1,pp.184–199,Jan.2011.。
职场新概念英语:职业社交网络难以取代名片
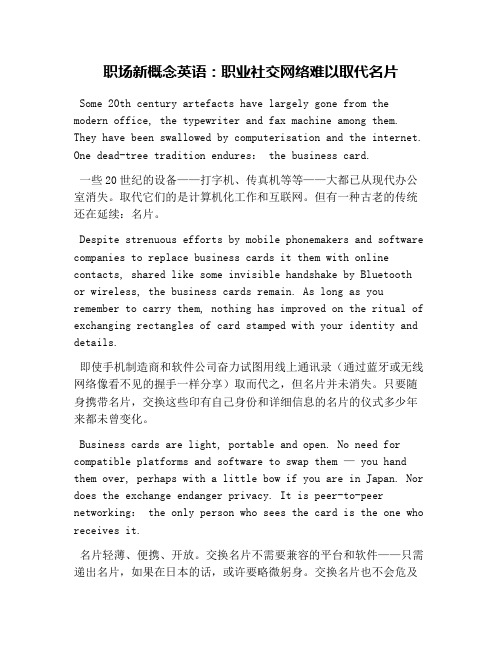
职场新概念英语:职业社交网络难以取代名片Some 20th century artefacts have largely gone from the modern office, the typewriter and fax machine among them. They have been swallowed by computerisation and the internet. One dead-tree tradition endures: the business card.一些20世纪的设备——打字机、传真机等等——大都已从现代办公室消失。
取代它们的是计算机化工作和互联网。
但有一种古老的传统还在延续:名片。
Despite strenuous efforts by mobile phonemakers and software companies to replace business cards it them with online contacts, shared like some invisible handshake by Bluetooth or wireless, the business cards remain. As long as you remember to carry them, nothing has improved on the ritual of exchanging rectangles of card stamped with your identity and details.即使手机制造商和软件公司奋力试图用线上通讯录(通过蓝牙或无线网络像看不见的握手一样分享)取而代之,但名片并未消失。
只要随身携带名片,交换这些印有自己身份和详细信息的名片的仪式多少年来都未曾变化。
Business cards are light, portable and open. No need for compatible platforms and software to swap them — you hand them over, perhaps with a little bow if you are in Japan. Nor does the exchange endanger privacy. It is peer-to-peer networking: the only person who sees the card is the one who receives it.名片轻薄、便携、开放。
网络安全风险极大英语

网络安全风险极大英语With the rapid development of the internet, the risk of cyber security is becoming increasingly serious. Cyber security threats are not only limited to individuals but also extend to businesses, governments, and national security. In this essay, I will discuss the significant risks of cyber security and their potential impact.One major risk is data breaching. Hackers can easily access personal and sensitive information such as credit card details, social security numbers, and email passwords through various methods like phishing or malware attacks. Once the hackers obtain these data, they can conduct fraudulent activities, steal money, or even commit identity theft. The consequences of data breaching can be financially devastating and emotionally traumatizing for individuals, eroding their trust in online activities.Another significant risk is the compromise of intellectual property. Companies invest a significant amount of time, money, and resources to develop and create innovative products or services. However, hackers can infiltrate their systems and steal their intellectual property, including patents, trade secrets, and business plans. The loss of intellectual property not only affects the competitiveness and profitability of the companies but also undermines innovation and hampers economic growth. Furthermore, cyber attacks can disrupt critical infrastructure. With the increasing interconnectivity of different networks, a cyber attack on essential systems such as power grids, transportation, or healthcare facilities can have severe consequences. Hospitals may not be able to provide timely medical services, transportationsystems can be paralyzed, and power outages can occur. These attacks not only pose risks to the daily lives of individuals but also to national security.Moreover, cyber security risks also extend to the realm of national security. Nation-states and hacker groups can launch sophisticated attacks on government organizations, military systems, or critical infrastructure to gain access to classified information or to disrupt operations. Cyber warfare has become a significant concern and can lead to severe consequences, including political instability, economic damage, and even physical harm.To mitigate these risks, it is crucial for individuals and organizations to implement comprehensive cyber security measures. Individuals should be aware of phishing emails, use strong passwords, and keep their software up-to-date. For businesses, investing in advanced security technologies, conducting regular security audits, and educating employees about cyber security best practices are essential. On a governmental level, enacting strict laws and regulations, collaborating with international partners, and allocating adequate resources for cyber defense are necessary.In conclusion, the risks of cyber security are significant and can have far-reaching consequences. Data breaching, intellectual property theft, infrastructure disruption, and national security threats are some of the major risks associated with cyber attacks. It is vital for individuals, businesses, and governments to take proactive measures to strengthen cyber security and protect againstthese threats. Only by working together can we create a safer digital landscape.。
网络安全相关专业术语名词解释(上篇)
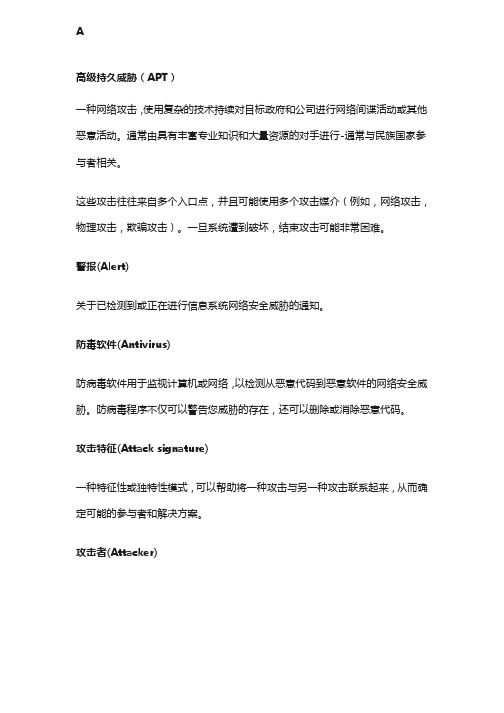
A高级持久威胁(APT)一种网络攻击,使用复杂的技术持续对目标政府和公司进行网络间谍活动或其他恶意活动。
通常由具有丰富专业知识和大量资源的对手进行-通常与民族国家参与者相关。
这些攻击往往来自多个入口点,并且可能使用多个攻击媒介(例如,网络攻击,物理攻击,欺骗攻击)。
一旦系统遭到破坏,结束攻击可能非常困难。
警报(Alert)关于已检测到或正在进行信息系统网络安全威胁的通知。
防毒软件(Antivirus)防病毒软件用于监视计算机或网络,以检测从恶意代码到恶意软件的网络安全威胁。
防病毒程序不仅可以警告您威胁的存在,还可以删除或消除恶意代码。
攻击特征(Attack signature)一种特征性或独特性模式,可以帮助将一种攻击与另一种攻击联系起来,从而确定可能的参与者和解决方案。
攻击者(Attacker)威胁的诱因:恶意角色,他们试图更改,破坏,窃取或禁用计算机系统上保存的信息,然后利用其结果。
认证方式(Authentication)验证用户,进程或设备的身份或其他属性的过程。
B行为监控(Behaviour monitoring)观察用户,信息系统和流程的活动。
可用于根据组织政策和规则,正常活动的基准,阈值和趋势来衡量这些活动。
黑名单(Blacklist)实体(用户,设备)被阻止,拒绝特权或访问的列表。
蓝队(Blue team)模拟网络安全攻击中的防御小组。
蓝队在红队攻击时捍卫企业的信息系统。
这些模拟攻击通常是由中立组织怀特(White Team)建立和监控的业务演习的一部分。
机器人(bot)连接到Internet的计算机,该计算机已受到恶意逻辑的破坏,无法在远程管理员的命令和控制下进行活动。
僵尸网络(Botnet)连接到Internet的受感染设备网络过去常常在所有者不知情的情况下进行协调的网络攻击。
违反(Breach)未经授权访问数据,计算机系统或网络。
自携设备办公(BYOD)组织允许员工将其个人设备用于工作目的的策略或政策。
网络攻击英语作文

网络攻击英语作文Title: The Peril of Cyber Attacks。
In today's interconnected world, the threat of cyber attacks looms large, casting a shadow over individuals, businesses, and even nations. Cyber attacks, facilitated by the ever-expanding reach of the internet, have become a pervasive menace, capable of wreaking havoc on a global scale. This essay delves into the nuances of cyber attacks, their implications, and the imperative need for robust cybersecurity measures.First and foremost, it's essential to understand what constitutes a cyber attack. Broadly defined, a cyber attack refers to any malicious attempt to breach digital systems, networks, or devices with the intent of stealing sensitive information, causing disruption, or inflicting damage. These attacks can take various forms, including but not limited to malware infections, phishing scams, denial-of-service (DoS) attacks, and ransomware assaults.One of the most pressing concerns regarding cyberattacks is their potential to inflict significant economic damage. For businesses, especially small and medium-sized enterprises (SMEs), a successful cyber attack can spell financial ruin. The theft of intellectual property,financial data breaches, or disruptions to essential services can result in substantial financial losses, tarnished reputations, and even bankruptcy.Furthermore, cyber attacks pose a severe threat to national security. State-sponsored hacking activities, espionage campaigns, and cyber warfare tactics have the potential to destabilize governments, undermine democratic processes, and compromise critical infrastructure. The recent surge in ransomware attacks targeting vital sectors such as healthcare, energy, and transportation underscores the vulnerability of essential services to malicious actors.In addition to the economic and security implications, cyber attacks also have far-reaching societal consequences. The proliferation of fake news, online propaganda, andsocial engineering tactics can erode trust in institutions, sow discord among communities, and manipulate public opinion. Moreover, the rise of cyberbullying, online harassment, and digital identity theft has fueled concerns about the psychological well-being and privacy of individuals in the digital age.Addressing the scourge of cyber attacks requires amulti-faceted approach encompassing technological innovation, regulatory frameworks, and public awareness campaigns. Firstly, organizations must prioritize cybersecurity as a fundamental aspect of their operations, investing in state-of-the-art security solutions, conducting regular risk assessments, and implementing robust incident response protocols.Secondly, governments play a crucial role in combating cyber threats by enacting legislation to deter cybercriminals, fostering international cooperation to address cross-border cybercrime, and investing in cybersecurity research and development. Collaborative efforts such as information sharing initiatives and jointcybersecurity exercises can enhance the collective resilience of nations against cyber attacks.Furthermore, fostering a culture of cybersecurity awareness is paramount in empowering individuals to protect themselves against online threats. Education and training programs aimed at promoting digital literacy, teaching cybersecurity best practices, and raising awareness about common cyber risks can empower users to navigate thedigital landscape safely.In conclusion, cyber attacks represent a pervasive and evolving threat that demands concerted action from all stakeholders. By embracing a proactive approach to cybersecurity, leveraging technological innovations, and fostering international collaboration, we can mitigate the risks posed by cyber attacks and safeguard the integrity of our digital ecosystem. Only through collective vigilance and resilience can we effectively thwart the adversaries lurking in the shadows of cyberspace.。
网络攻击定义及描述规范
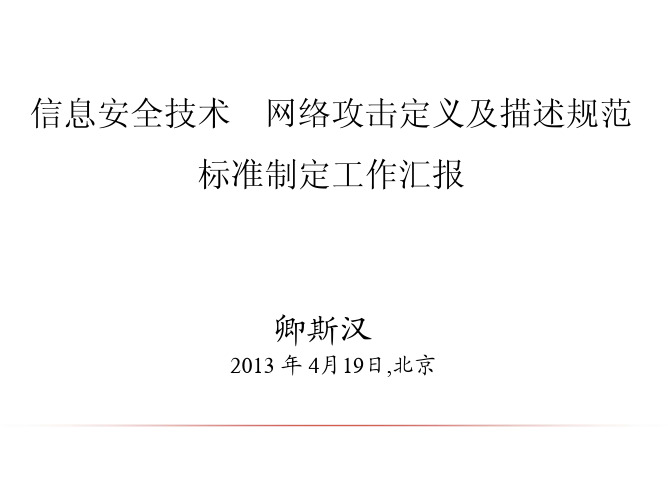
21
计算机网络利用 — 定义
计算机网络利用 — 通过使用计算机网络从目 标或敌方信息系统或网络中搜集数据而发起的操作 和情报搜集能力。简称为CNE。参看:计算机网络 攻击;计算机网络防御和计算机网络操作。
22
计算机网络操作 — 定义
computer network operations — Comprised of computer network attack, computer network defense, and related computer network exploitation enabling operations. Also called CNO. See also computer network attack; computer network defense; computer network exploitation.
9
计算机网络利用 — 定义
计算机网络利用(CNE) — 通过使用计算机网 络从目标或敌方信息系统或网络中搜集数据而发起 的操作和情报搜集能力。 来源: CNSSI-4009
10
计算机网络操作 — 定义
Computer
Network Operations (CNO)—
Comprised of computer network attack, computer network defense, and related computer network exploitation enabling operations. SOURCE: CNSSI-4009
16
计算机网络攻击 — 定义
computer network attack — Actions taken through the use of computer networks to disrupt, deny, degrade, or destroy information resident in computers and computer networks, or the computers and networks themselves. Also called CNA. See also computer network defense; computer network exploitation; computer network operations.
FortiNDR 智能网络侦查与应对系统概述说明书
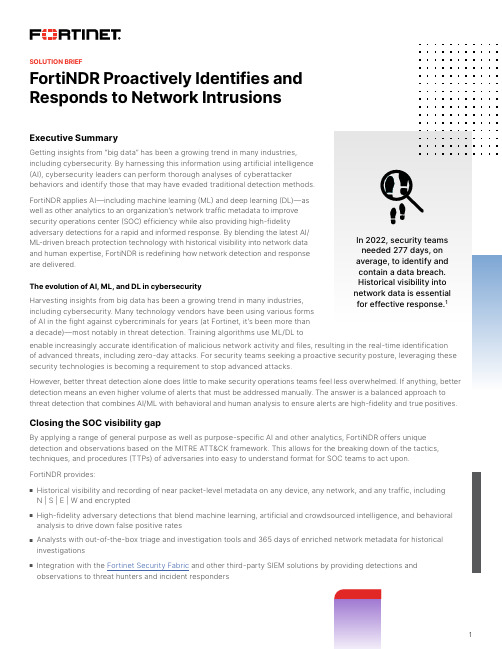
FortiNDR Proactively Identifies and Responds to Network IntrusionsExecutive SummaryGetting insights from “big data” has been a growing trend in many industries, including cybersecurity. By harnessing this information using artificial intelligence (AI), cybersecurity leaders can perform thorough analyses of cyberattacker behaviors and identify those that may have evaded traditional detection methods. FortiNDR applies AI—including machine learning (ML) and deep learning (DL)—as well as other analytics to an organization’s network traffic metadata to improve security operations center (SOC) efficiency while also providing high-fidelityadversary detections for a rapid and informed response. By blending the latest AI/ ML-driven breach protection technology with historical visibility into network data and human expertise, FortiNDR is redefining how network detection and response are delivered.The evolution of AI, ML, and DL in cybersecurityHarvesting insights from big data has been a growing trend in many industries, including cybersecurity. Many technology vendors have been using various forms of AI in the fight against cybercriminals for years (at Fortinet, it’s been more than a decade)—most notably in threat detection. Training algorithms use ML/DL to In 2022, security teams needed 277 days, on average, to identify and contain a data breach. Historical visibility into network data is essential for effective response.1SOLUTION BRIEFenable increasingly accurate identification of malicious network activity and files, resulting in the real-time identificationof advanced threats, including zero-day attacks. For security teams seeking a proactive security posture, leveraging these security technologies is becoming a requirement to stop advanced attacks.However, better threat detection alone does little to make security operations teams feel less overwhelmed. If anything, better detection means an even higher volume of alerts that must be addressed manually. The answer is a balanced approach to threat detection that combines AI/ML with behavioral and human analysis to ensure alerts are high-fidelity and true positives. Closing the SOC visibility gapBy applying a range of general purpose as well as purpose-specific AI and other analytics, FortiNDR offers unique detection and observations based on the MITRE ATT&CK framework. This allows for the breaking down of the tactics, techniques, and procedures (TTPs) of adversaries into easy to understand format for SOC teams to act upon.FortiNDR provides:n Historical visibility and recording of near packet-level metadata on any device, any network, and any traffic, includingN | S | E | W and encryptedn High-fidelity adversary detections that blend machine learning, artificial and crowdsourced intelligence, and behavioral analysis to drive down false positive ratesn Analysts with out-of-the-box triage and investigation tools and 365 days of enriched network metadata for historical investigationsn Integration with the Fortinet Security Fabric and other third-party SIEM solutions by providing detections and observations to threat hunters and incident respondersCybersecurity Analysts on Your SideCybersecurity teams are in a race against time to protect their organizations. With limited knowledge of—or time to learn—the adversary’s latest intent, tactics, techniques, or procedures and working without external guidance, the security team must often go it alone. FortiNDR offers the option of virtual or in-person expertise to ensure customers are best positioned to thwart adversaries. Let Fortinet experts do the work to keep you current on the evolving threat landscape.FortiGuard Applied Threat Research (ATR)Our seasoned advanced threat researchers monitor cybercriminal activity, perform reverse engineering, and handle detection engineering of adversary behavior with high accuracy. The first-hand knowledge from FortiGuard ATR empowers both the AI and the on-demand technical success managers (TSMs) in advance of and during high-pressure incidents.Virtual Security AnalystOperating in unsupervised mode, the FortiNDR Virtual Security Analyst helps SOC teams analyze and investigate new threats while continuously adapting to new and emerging threats.Eliminating DistractionsPurchasing a security solution should enable security professionals to focus on protecting their organization. However, all too often, security solutions create unnecessary distractions rather than positive results. Many NDR solutions have hidden costs and time tied to providing care and feeding, solution proficiency, addressing false positives, and performing detection tuning—all negating their intended value. FortiNDR includes expertise, virtual or in-person, from product and threat experts to remove distractions.Zero tuningFortiGuard Labs performs ongoing detection tuning and QA of all machine learning, behavioral analysis, and threat intelligence detection engines.Minimal maintenanceThe Fortinet TSMs and SaaS Ops teams provide sensor and traffic diagnostics, a fully managed FortiNDR Cloud web portal, and automatic software updates.Speeding ResponseRemediating the often wide-ranging spread of multi-stage cyber campaigns throughout today’s digital organizations for a return to safe operation, requires the coordination of actions across multiple security controls and infrastructures.The Fortinet Security FabricDesigned as a component of a single cybersecurity platform, FortiNDR natively integrates with the Fortinet Security Fabric and other products—from network to email to endpoint security. This facilitates automated response.Third-party technologiesAlthough 75% of organizations report consolidating cybersecurity vendors2, security infrastructures often maintain some multi-vendor elements. APIs enable additional integration beyond the Fortinet Security Fabric.FortiNDR (on premises) Architecture and IntegrationFortiNDR Cloud Architecture and DeploymentCustomer InfrastructureFortinet Guided-SaaSCopyright © 2023 Fortinet, Inc. All rights reserved. Fortinet ®, FortiGate ®, FortiCare ® and FortiGuard ®, and certain other marks are registered trademarks of Fortinet, Inc., and other Fortinet names herein may also be registered and/or common law trademarks of Fortinet. All other product or company names may be trademarks of their respective owners. Performance and other metrics contained herein were attained in internal lab tests under ideal conditions, and actual performance and other results may vary. Network variables, different network environments and other conditions may affect performance results. Nothing herein represents any binding commitment by Fortinet, and Fortinet disclaims all warranties, whether express or implied, except to the extent Fortinet enters a binding written contract, signed by Fortinet’s General Counsel, with a purchaser that expressly warrants that the identified product will perform according to certain expressly-identified performance metrics and, in such event, only the specific performance metrics expressly identified in such binding written contract shall be binding on Fortinet. For absolute clarity, any such warranty will be limited to performance in the same ideal conditions as in Fortinet’s internal lab tests. Fortinet disclaims in full any covenants, representations, and guarantees pursuant hereto, whether express or implied. Fortinet reserves the right to change, modify, transfer, or otherwise revise this publication without notice, and the most current version of the publication shall be applicable.April 1, 2023 2:03 PMConclusionFor today’s overburdened cybersecurity professionals, FortiNDR helps cybersecurityoperations teams move from a reactive to a proactive security posture, whileincreasing their efficiency and remediating threats faster. FortiNDR delivers thesekey benefits:n Improved visibility of threats. Real-time, automated investigation of networksecurity incidents and extended historical network visibility enable a faster, morecomprehensive response to threats. Since the impact of an intrusion increasesover time, real-time response is the best way to minimize damage.n Virtual or human expertise when it matters most. Virtual security analysts ortechnical success managers ease high-pressure scenarios on your cybersecurityanalysts with expertise on your side.n Fewer distractions from false positives and detection tuning. With threatanalysis and detection tuning provided in real-time, organizations are lessvulnerable while awaiting a vendor’s application patch or anti-malware signature.84% of SOC analysts rank “minimization of false positives” (detection tuning) as the most important SOC activity. Reducing false positives should be the vendor’s responsibility.31 Cost of a Data Breach Report 2022, IBM Security2 Second Annual Study on the Economics of Security Operations Centers: What is the True Cost for Effective Results? 2021 – Ponemon Institute3Ibid。
信息技术常用术语中英文对照表

信息技术常用术语中英文对照表1. 互联网 (Internet)2. 网络安全 (Cybersecurity)3. 云计算 (Cloud Computing)5. 大数据 (Big Data)6. 机器学习 (Machine Learning)7. 物联网 (Internet of Things)8. 虚拟现实 (Virtual Reality)9. 增强现实 (Augmented Reality)10. 数字化转型 (Digital Transformation)11. 数据挖掘 (Data Mining)12. 信息安全 (Information Security)13. 信息技术 (Information Technology)15. 服务器 (Server)16. 客户端 (Client)17. 网络协议 (Network Protocol)18. 软件开发 (Software Development)19. 数据库 (Database)20. 编程语言 (Programming Language)21. 操作系统 (Operating System)22. 硬件 (Hardware)23. 软件 (Software)24. 网络基础设施 (Network Infrastructure)26. 数字营销 (Digital Marketing)27. 网络攻击 (Cyber Attack)28. 数据加密 (Data Encryption)29. 信息架构 (Information Architecture)30. 网络安全漏洞 (Cybersecurity Vulnerability)31. 信息系统 (Information System)32. 网络安全策略 (Cybersecurity Strategy)33. 网络安全意识 (Cybersecurity Awareness)34. 数字化战略 (Digital Strategy)35. 网络安全法规 (Cybersecurity Regulation)36. 信息安全标准 (Information Security Standard)37. 网络安全解决方案 (Cybersecurity Solution)38. 网络安全威胁 (Cybersecurity Threat)39. 信息安全事件 (Information Security Incident)40. 网络安全审计 (Cybersecurity Audit)41. 信息安全风险管理 (Information Security Risk Management)42. 网络安全监控 (Cybersecurity Monitoring)43. 信息安全培训 (Information Security Training)44. 网络安全事件响应 (Cybersecurity Incident Response)45. 信息安全政策 (Information Security Policy)46. 网络安全评估 (Cybersecurity Assessment)47. 信息安全意识提升 (Information Security Awareness)48. 网络安全培训 (Cybersecurity Training)49. 信息安全策略 (Information Security Strategy)50. 网络安全管理体系 (Cybersecurity Management System)信息技术常用术语中英文对照表51. 网络服务 (Network Service)52. 数据传输 (Data Transmission)53. 信息架构 (Information Architecture)54. 信息安全审计 (Information Security Audit)55. 信息安全认证 (Information Security Certification)56. 信息安全管理体系 (Information Security Management System)57. 信息安全策略 (Information Security Strategy)58. 信息安全培训 (Information Security Training)59. 信息安全意识 (Information Security Awareness)60. 信息安全风险管理 (Information Security Risk Management)61. 信息安全事件 (Information Security Incident)62. 信息安全标准 (Information Security Standard)63. 信息安全法规 (Information Security Regulation)64. 信息安全解决方案 (Information Security Solution)65. 信息安全威胁 (Information Security Threat)66. 信息安全监控 (Information Security Monitoring)67. 信息安全评估 (Information Security Assessment)68. 信息安全政策 (Information Security Policy)69. 信息安全审计 (Information Security Audit)70. 信息安全认证 (Information Security Certification)71. 信息安全管理体系 (Information Security Management System)72. 信息安全策略 (Information Security Strategy)73. 信息安全培训 (Information Security Training)74. 信息安全意识 (Information Security Awareness)75. 信息安全风险管理 (Information Security Risk Management)76. 信息安全事件 (Information Security Incident)77. 信息安全标准 (Information Security Standard)78. 信息安全法规 (Information Security Regulation)79. 信息安全解决方案 (Information Security Solution)80. 信息安全威胁 (Information Security Threat)81. 信息安全监控 (Information Security Monitoring)82. 信息安全评估 (Information Security Assessment)83. 信息安全政策 (Information Security Policy)84. 信息安全审计 (Information Security Audit)85. 信息安全认证 (Information Security Certification). 信息安全管理体系 (Information Security Management System)87. 信息安全策略 (Information Security Strategy)88. 信息安全培训 (Information Security Training)89. 信息安全意识 (Information Security Awareness)Management)91. 信息安全事件 (Information Security Incident)92. 信息安全标准 (Information Security Standard)93. 信息安全法规 (Information Security Regulation)94. 信息安全解决方案 (Information Security Solution)95. 信息安全威胁 (Information Security Threat)96. 信息安全监控 (Information Security Monitoring)97. 信息安全评估 (Information Security Assessment)98. 信息安全政策 (Information Security Policy)99. 信息安全审计 (Information Security Audit)100. 信息安全认证 (Information Security Certification)信息技术常用术语中英文对照表51. 网络服务 (Network Service)52. 数据传输 (Data Transmission)53. 信息架构 (Information Architecture)54. 信息安全审计 (Information Security Audit)55. 信息安全认证 (Information Security Certification)56. 信息安全管理体系 (Information Security Management System)57. 信息安全策略 (Information Security Strategy)58. 信息安全培训 (Information Security Training)59. 信息安全意识 (Information Security Awareness)Management)61. 信息安全事件 (Information Security Incident)62. 信息安全标准 (Information Security Standard)63. 信息安全法规 (Information Security Regulation)64. 信息安全解决方案 (Information Security Solution)65. 信息安全威胁 (Information Security Threat)66. 信息安全监控 (Information Security Monitoring)67. 信息安全评估 (Information Security Assessment)68. 信息安全政策 (Information Security Policy)69. 信息安全审计 (Information Security Audit)70. 信息安全认证 (Information Security Certification)71. 信息安全管理体系 (Information Security Management System)72. 信息安全策略 (Information Security Strategy)73. 信息安全培训 (Information Security Training)74. 信息安全意识 (Information Security Awareness)75. 信息安全风险管理 (Information Security Risk Management)76. 信息安全事件 (Information Security Incident)77. 信息安全标准 (Information Security Standard)78. 信息安全法规 (Information Security Regulation)79. 信息安全解决方案 (Information Security Solution)80. 信息安全威胁 (Information Security Threat)81. 信息安全监控 (Information Security Monitoring)82. 信息安全评估 (Information Security Assessment)83. 信息安全政策 (Information Security Policy)84. 信息安全审计 (Information Security Audit)85. 信息安全认证 (Information Security Certification). 信息安全管理体系 (Information Security Management System)87. 信息安全策略 (Information Security Strategy)88. 信息安全培训 (Information Security Training)89. 信息安全意识 (Information Security Awareness)90. 信息安全风险管理 (Information Security Risk Management)91. 信息安全事件 (Information Security Incident)92. 信息安全标准 (Information Security Standard)93. 信息安全法规 (Information Security Regulation)94. 信息安全解决方案 (Information Security Solution)95. 信息安全威胁 (Information Security Threat)96. 信息安全监控 (Information Security Monitoring)97. 信息安全评估 (Information Security Assessment)98. 信息安全政策 (Information Security Policy)99. 信息安全审计 (Information Security Audit)100. 信息安全认证 (Information Security Certification) 101. 数据库管理系统 (Database Management System)102. 编程语言 (Programming Language)103. 硬件 (Hardware)104. 软件 (Software)105. 操作系统 (Operating System) 106. 服务器 (Server)107. 客户端 (Client)108. 网络协议 (Network Protocol) 109. 软件开发 (Software Development) 110. 数据库 (Database)111. 编程语言 (Programming Language) 112. 操作系统 (Operating System) 113. 硬件 (Hardware)114. 软件 (Software)115. 服务器 (Server)116. 客户端 (Client)117. 网络协议 (Network Protocol) 118. 软件开发 (Software Development) 119. 数据库 (Database)120. 编程语言 (Programming Language) 121. 操作系统 (Operating System) 122. 硬件 (Hardware)123. 软件 (Software)124. 服务器 (Server)125. 客户端 (Client)126. 网络协议 (Network Protocol)127. 软件开发 (Software Development) 128. 数据库 (Database)129. 编程语言 (Programming Language) 130. 操作系统 (Operating System) 131. 硬件 (Hardware)132. 软件 (Software)133. 服务器 (Server)134. 客户端 (Client)135. 网络协议 (Network Protocol) 136. 软件开发 (Software Development) 137. 数据库 (Database)138. 编程语言 (Programming Language) 139. 操作系统 (Operating System) 140. 硬件 (Hardware)141. 软件 (Software)142. 服务器 (Server)143. 客户端 (Client)144. 网络协议 (Network Protocol) 145. 软件开发 (Software Development) 146. 数据库 (Database)147. 编程语言 (Programming Language) 148. 操作系统 (Operating System) 149. 硬件 (Hardware)150. 软件 (Software)。
- 1、下载文档前请自行甄别文档内容的完整性,平台不提供额外的编辑、内容补充、找答案等附加服务。
- 2、"仅部分预览"的文档,不可在线预览部分如存在完整性等问题,可反馈申请退款(可完整预览的文档不适用该条件!)。
- 3、如文档侵犯您的权益,请联系客服反馈,我们会尽快为您处理(人工客服工作时间:9:00-18:30)。
Big-Data Architecture for Cyber Attack Graphs Representing Security Relationships in NoSQL Graph DatabasesSteven Noel, Eric Harley, Kam Him Tam, and Greg GyorCyber Security DivisionThe MITRE CorporationMcLean, Virginia, USA[snoel, eharley, ktam, ggyor]@Abstract—We introduce a new modeling framework for mapping vulnerability paths through networks and associating them with observed attacker activities. We merge a complex blend of network relationships and events, such as topology, firewall policies, host configurations, vulnerabilities, attack patterns, intrusion alerts, and logs. Our persistence layer includes Neo4j, a Not Only SQL (NoSQL) database optimized for graphs. We explore how the Neo4j property-graph data model supports analysis and queries within our problem domain. For interoperability with other tools, we employ standardized cyber-security data exchange language. We show that our approach supports the same kinds of graph analytics as an existing attack graph tool, through the application of the Neo4j Cypher query language. We then extend those analytics through a much richer model of the network environment and attacker/defender activities. Our work represents the first investigation of cyber attack graph analysis based on graph databases –an important class of NoSQL database.Keywords-attack graphs; topological vulnerability analysis; network attack modeling; cyber security standards; cluster computing; NoSQL databasesI.I NTRODUCTIONAs defenders, it is crucial to understand our networks from the adversary’s perspective. Network relationships are complex and can often be combined in unexpected ways. We need to understand how attackers can leverage initial footholds to extend their reach through the network. Rather than relying on perimeter defenses and being overwhelmed by many point sources, a unified picture of attacker movement through the network is needed.We must also extend our notion of vulnerabilities, beyond the usual assumption of system flaws. Once inside a network, attackers can behave in ways that would otherwise be considered legitimate, while still achieving their goals. Furthermore, attacker movement does not depend on host vulnerabilities and configurations alone. The location of hosts within the overall network topology, security mechanism such as firewalls (both perimeter and internal), and specific access policy rules can all play roles.Building such a comprehensive web of network attack relationships poses significant challenges. We must correlate data from numerous sources (topology, vulnerabilities, client/server configurations, policy rules, trust relationships, security events, etc.) into a common model. An architecture for data representation and persistence must be flexible and easy to extend, and map naturally to network attack relationships. For interoperability with other tools, we should leverage security data standards where applicable.Such a model has potential application to a variety of use cases (offensive/defensive, proactive/reactive, etc.), suggesting the need for ad hoc queries. In many cases, our query and analytic results are complex graph relationships, motivating the need for interactive graph visualization.The discovery of network attack relationships represents a significantly different computational challenge than simply analyzing data at rest. Testing combinations of source and destination machines against firewall policy rules is highly iterative. Popular architectures for big-data analytics based on the MapReduce programming model are batch-oriented and file-based, which performs poorly for iterative computations.To address these challenges, we introduce an approach for mapping multi-step attack vulnerability and event dependencies through networks. We build a predictive model of possible attack paths, which we correlate with network events (intrusion alerts, sensor logs, etc.). We consider vulnerabilities not only in the sense of exploitable system flaws, but also legitimate accesses that can be leveraged once an attacker has established a foothold inside the network.Correlating detected attack events with potential attack paths gives the best options for response, especially for protecting critical assets, and improves situational awareness, e.g., inferring missed attack steps and identifying false positives. We store the resulting attack graph in Neo4j [1], a NoSQL graph database. This is much more flexible for our problem domain than a relational database, and provides significantly better performance for graph analytics.II.P REVIOUS W ORKA variety of approaches have been proposed for analyzing multi-step attacks through cyber networks. A predominant meta-model in this problem domain is to represent attack relationships as graphs or trees. The abstractions and semantics for these kinds of graphs vary depending on thenetwork©2014 The MITRE Corporation. ALL RIGHTS RESERVED.entities under consideration and the kinds of analytics supported.For example, graphs can represent network state transitions leading to attack goals [2][3], attacker exploitation steps related by preconditions and postconditions [4][5], intrusion alert sequences [6][7], logical dependencies for attack goals [8][9], or host attack reachability [10][11][12]. Attack graphs have also been implemented with the relational model [13]. We show results for the attack graph tool Cauldron [14] as a baseline of comparison for our much richer model.A common theme in these kinds of approaches has been to focus on a limited set of entity types in the graph model. However, security concerns in real environments are much more complex. Our aim is to introduce an extensible and scalable modeling framework that can incorporate a rich variety of interrelated data about the network environment and how they relate to potential and actual adversary activities. Our data model incorporates standardized languages and processes for cyber security, developed by MITRE and others, known collectively as Making Security Measurable ™ [15]. This includes Common Vulnerabilities and Exposures (CVE)® [16], Common Vulnerability Scoring System (CVSS) [17], Common Weakness Enumeration (CWE)™ [18], Common Platform Enumeration (CPE)™ [19], Common Attack Pattern Enumeration and Classification (CAPEC)™ [20], Cyber Observable eXpression (CybOX)™ language [21], and others.III. A PPROACHFigure 1 shows the components of our system for mapping attack relationships through a network. We ingest data from various sources, map them to a common environment model. We analyze elements of the environment model for potential attack paths, and associate them with network events (alerts, logs, etc.). We store the resultingattack graph in a Neo4j database.EnvironmentDatabaseAttackMappingGraph DatabaseQueryVisualizationAnalystsOtherToolsAgnostic ModelVulnerability ScansPort Scans Host Inventories Firewall RulesNetflow Intrusion AlertsHostflowNeo4jFigure 1. Components for cyber attack mapping.We ingest data from various sources relevant to attacks (both potential and actual). For this, rely primarily on the Splunk platform [22]. The ingest process maps the data to an agnostic (vendor-neutral) model using standardized language. The Cauldron tool ingests data from vulnerability scans andfirewall configurations. We include additional sources for a much richer data model, including network flows, IDS/IPS alerts, anti-virus logs, operating system logs, host inventory agents, and proxy server logs. For mapping network attack relationships we leverage Apache Spark [23], which has an in-memory compute model optimized for iterative computation on Apache Hadoop [24] clusters. As input, we build a model of the network environment and events, stored in MongoDB [25]. The result of our iterative analysis is a graph of potential attack steps and associated network events, which we store in a Neo4j graph database. Graph databases represent node adjacency without indices, via direct pointers. The relational model relies on expensive join operations (Cartesian products) when traversing graph edges, having complexity O (n d ) for traversals of depth d over n graph nodes. In Neo4j, traversals follow direct links (edges only) so that complexity depends only on the size of query results sub-graph, independent of the total graph.As a simple example, consider Figure 2. This illustrates the attack graph model employed by the Cauldron tool. It defines protection domains (e.g., a subnet or enclave), which contain machines with vulnerabilities. Implicitly, a machine can reach the vulnerabilities of all other machines in its domain. Graph edges across domains (exploits) are vulnerabilities that are exposed over the network (e.g., through firewalls) and reachable by machines in other domains.Figure 2. Protection domains, machines, vulnerabilities, and exploits.Figure 3 shows our Neo4j representation of the attack graph in Figure 2. Neo4j employs the property-graph model [26]. A property graph contains nodes and nodes (vertices) and relationships (edges). Nodes and relationships are named; relationships are directed. Nodes and relationships can also contain properties, which are key-value pairs. A particular kind of property are labels, which define groups (types) of nodes or relationships.Figure 3 has four node labels {Exploit , Machine , Vulnerability , Domain } and four relationship labels {IN , ON , LAUNCHES , AGAINST , VICTIM }. Machine-domain membership isd :Domainm :Machine:IN:INThis represents that machine m is in protection domain d . The edges are needed in both directions to support graph traversalqueries, which are directional. This allows traversal in both directions (from machine to domain and domain to machine), as needed to follow a particular flow of attack, including attacksteps implicitly possible within a domain.Figure 3. Attack graph represented as property graph.This explicitly encodes the domain membership that is implicit in Cauldron. An advantage of the protection domain abstraction is that the number of edges among machines in a domain is linear in the number of machines rather than quadratic [27]. Our property-graph representation retains this advantage. However, in Cauldron, the implicit domain membership is built into the system, and cannot be changed for cases in which the assumption of full access among machines does not hold. In our flexible property-graph representation, we can simply omit protection domains, and explicitly connect machines through exploits.Here is the representation for vulnerability v on machine m : v :Vulnerabilitym :Machine:ONAn exploit e from machine m 1 against vulnerability v on machine m 2 is thenm 1:Machine e :Exploit :LAUNCHES e :Exploitv :Vulnerability :AGAINST e :Exploitm 2:Machine:VICTIMWith this basic attack graph representation as a property graph, we can perform the same kinds of analysis as the Cauldron tool. For this we leverage the Cypher query language [26] for Neo4j. For example, this Cypher query traverses the graph of Figure 3, finding all paths of exploitable vulnerabilities between a particular pair of machines:MATCH path =(start:Machine {name:'Machine 1.1'}) -[r:LAUNCHES |VICTIM |IN *]->(end:Machine {name:'Machine 3.1'}) RETURN pathFigure 4 shows the result of this query.This query seeks a sub-graph starting on Machine 1.1 (in Domain 1) and ending on Machine 3.1 (in Domain 3). Itconstrains the matching sub-graph to relationships of type LAUNCHES , VICTIM , or IN . This yields paths of Machine , Domain , and Exploit nodes. That is, it finds paths from the start machineto the end machine, through and across domains as required.Figure 4. Query result for paths between two chosen machines.IV. I LLUSTRATIVE E XAMPLEIn this section, we examine our approach to our cyber attack modeling. We first compare it to an existing attack graph tool (Cauldron). We then extend the baseline attack graph model with richer content for more in-depth analysis. Figure 5 shows the network for this illustrative example.External FirewallFirewallIDS SensorIDS SensorFigure 5. Network for illustrative example.The internal network is segmented into three protection domains (DMZ, mission client workstations, and data center). The externalfirewall protects the internal network from the outside, and the internal firewall protects the critical data-center servers. Intrusion detection sensors monitor traffic crossing through the firewalls. Figure 6 is the resulting attack graph generated by the Cauldron tool.Figure 6. Cauldron attack graph for Figure 5.This shows that the DNS server has a known vulnerability, which is exposed (through the external firewall) to the outside. The client workstation has two vulnerabilities, one of which is exposed to the outside. In the data center, a database has a vulnerability, although it is blocked (by the internal firewall) from the other domains. While not shown here, Cauldron supports drilldown into the details for the vulnerabilities within and across domains. Assume those details are supplied by a vulnerability scanner in terms of CVE.We build our model containing domains, machines, vulnerabilities, and exploits as described in the previous section. We then extend the model as additional nodes and relationships of our property graph. This includes the network topology (placement of firewalls between domains), attack patterns from CAPEC, vulnerability details from NVD, andintrusion alerts. Figure 7 is the resulting Neo4j property graph.Figure 7. Neo4j property-graph model for Figure 5.The property graph of Figure 7 is relatively complex. However, the intention is not to necessarily view the full graph. Rather, the property graph provides a rich framework for analytics and queries. For example, consider this query:MATCH path = (start)-[r:IN |ROUTES *]->(end) RETURN pathThis query finds the IN and ROUTES relationships of the property graph, which define the network topology (domains, machines, and firewall devices). Figure 8 is the result of this query. This shows how firewalls separate domains, e.g., to identify theappropriate firewalls for network hardening.Figure 8. Query result showing network topology.Next, assume that intrusion alerts are generated (red nodes in Figure 7). While Cauldron does not support such real-time events, this is a straightforward extension to our model. Consider then this query:MATCH path = (start:Alert )-[r:ALERT |AGAINST |VICTIM |ON *]->(end) RETURN pathThis query starts on alert nodes, and then traverses the ALERT , AGAINST , VICTIM , and ONrelationships, resulting in Figure 9.Figure 9. Vulnerable path between intrusion alerts.By including the AGAINST , VICTIM , and ON relationships in our query, traversal leads away from alerts along paths of potential exploitation (via Exploit nodes). The LAUNCHES relationships is included because it spans the set of query result nodes. This query shows that the “client-side buffer overflow ” alert (against a QuickTime vulnerability on the mission client) leads along an exploitable path to the “web application fingerprinting ” alert (against the database front-end). From this, we might surmise that the alerts are potentially multiple attack steps by the same adversary.We can expand the scope of exploits through this query:MATCH path = (start:Alert )-[r:ALERT |AGAINST |VICTIM |ON |LAUNCHES *]->(end) RETURN pathThis query adds the LAUNCHES relationship, to show all exploits launched when traversing away from alerts, not just immediatevictims. The result is Figure 10.Figure 10. Vulnerable paths in the vicinity of alerts.This shows that there is in fact a larger chain of potential exploits near the alerts: ∙ Client-side buffer overflow against mission client. ∙ Lifting of database login credentials on client.∙ Logging in to database (via web front-end) from client. ∙ Fingerprinting to discover back-end database details. ∙ SQL injection attack against the database.This ability to discover vulnerability paths between near alerts is especially important because in many cases key attacker behaviors go undetected.We can further extend the search for exploitation paths by ignoring the alerts:MATCH path = (start)-[r:LAUNCHES |VICTIM |PREPARES *]->(end) RETURN pathFigure 11 is the query result. This finds all paths involving LAUNCHES , VICTIM , and PREPARES relationships. The PREPARES relationship represents the idea that one exploit prepares for another in a chain of attack. The LAUNCHES and VICTIM thendefine the attacker and victim machines for each exploit.Figure 11. Chain of potential exploits with attackers and victims.This query result introduces two preparatory exploits into the chain: cache poisoning by an outside attacker against the DNS server in the DMZ, followed by a pharming attack by a malicious web site. This leverages a vulnerability in the DNS server that allows an attacker to cause domain names to be resolved to a server under the attacker’s control. The resulting pharming attack lets the malicious web site serve content that exploits the client-side vulnerability, compromising the client. Our cyber-attack model leverages various standards defined under the Making Security Measurable framework. This includes CVE for known vulnerabilities, CVSS for vulnerability scoring and categorization, CWE for software weaknesses, and CPE for vulnerable software versions. Standardized language for security data helps close the gap for automated model generation. For example, many vulnerability scanners are CVE compliant, and the National Vulnerability Database (NVD) [28] maintained by NIST reports CVSS scores, CWE weaknesses, and CPE software versions for each CVE vulnerability.For example, this query finds NVD content relevant to intrusion alerts:MATCH path =(start:Alert )-[r:ALERT |AGAINST |CVE |CVSS |SEVERITY | METRICS |CWE |CPE |REFERENCES | REFERENCE |CONTAINS *]->(end) RETURN pathFigure 12 is the query result. A CVE node is the root of a sub-tree for a vulnerability, and nodes for CVSS score, CWE software weaknesses, and references (e.g., vendor bulletins). In this example, there is no CVE vulnerability associated withthe fingerprinting exploit.Figure 12. Incorporating NVD content in property graph.Our model also incorporates CAPEC, a community-developed catalog of common cyber attack patterns. CAPEC currently contains over 450 attack patterns, organized in a classification hierarchy of general to specific. CAPEC attack patterns capture knowledge about classes of attacks against cyber resources. Each attack pattern includes details about specific phases of the attack, the vulnerable attack surface, the resources required by the attacker, and ways to mitigate the attack. This provides a frame of reference for correlating various relevant information about attacks.This query finds the CAPEC attack pattern content relevant to a particular intrusion alert:MATCH path =(start:Alert{name:'Client-Side BOF Alert '}) -[r: ALERT |AGAINST |CAPEC |PATTERN | CHALLENGE |PHASE |CONTEXT | MECHANISM |CONSEQUENCE |MITIGATION |IMPACT *]->(end) RETURN pathFigure 13 is the query result.Figure 13. CAPEC attack pattern associated with an intrusion alert.V.S UMMARYWe describe a modeling and analytical framework for tracing cyber-attack vulnerability paths through networks, correlated with observed security events. We consider a variety of relationships, such as network topology, firewalls, host vulnerabilities, potential attack patterns, and intrusion alerts. Our model leverages a property-graph formalism, implemented through Neo4j, a NoSQL graph database. We examine how property graphs support cyber-attack analysis through ad hoc queries. Where appropriate, our modeling framework employs standardized data representations under the Making Security Measurable suite of languages.We demonstrate how our approach supports the same kinds of analytics as an existing attack graph tool (Cauldron). We then extend the baseline analytics, developing a much richer model of the network environment and attacker activities. Through the application of the Neo4j Cypher query language, we iteratively explore attack relationships, building a more complete picture of security state.Our work represents the first investigation of cyber-attack graph analysis based on NoSQL graph databases. These database architectures are optimized for graph analytics, especially traversals. This provides a flexible, scalable, high-performance persistence layer for our approach.We provide the necessary context for responding to attacks, based on known vulnerability paths. We consider vulnerabilities not only in the usual sense of exploitable system flaws, but also legitimate accesses that can be leveraged once an attacker has established a foothold inside the network, e.g., capturing login credentials. In this way, we analyze system interdependencies to map how an attacker can incrementally penetrate a network. This makes our results particularly relevant to advanced persistent threats and malicious insider behaviors.Our analysis guides the hardening of the network in advance of attacks. Potential attack paths are then correlated with detected attack events. We address the problem of missing alerts (false negatives), and extrapolate observed attacker presence to trace attack origins and next possible steps. This context gives the best options for response, especially for protecting critical assets, and improves situational awareness. Overall, we assemble disparate data sources into a global picture of overall security posture.A CKNOWLEDGMENTSThis work was supported by the MITRE Innovation Program (project number EPF-14-00341), with Vipin Swarup as Information Area Lead.R EFERENCES[1] J. Partner, A. Vukotic, N. Watt, Neo4j in Action, Manning, 2014.[2] R. Ritchey and P. Ammann, “Using model checking to analyze networkvulnerabilit ies,” IEEE Symposium on Security and Privacy, 2000.[3] O. Sheyner, J. Haines, S. Jha, R. Lippmann, and J. Wing, “Automatedgeneration and analysis of attack g raphs,” IEEE Symposium on Security and Privacy, 2002.[4] P. Ammann, D. Wijesekera, and S. Kaushik, “Scalable, graph-basednetwork vulnerability a nalysis,” ACM Conference on Computer and Communications Security (CCS), 2002.[5] S. Jajodia, S. Noel, B. O’Berry, “Topological analysis of network attackvulnerability,”Managing Cyber Threats: Issues, Approaches and Challenges, Springer, 2005.[6] P. Ning, Y. Cui, and D. Reeves, “Constructing attack scenarios throughcorrelation of intrusion al erts,” ACM Conference on Computer and Communications Security (CCS), 2002.[7] S. Noel, E. Robertson, and S. Jajodia, “Correlating intrusion events andbuilding attack scenarios through attack graph d istances,” Annual Computer Security Applications Conference (ACSAC), 2004.[8] S. Mauw, M. Oostdijk, “Foundations of attack trees,” Lecture Notes inComputer Science Volume 3935, 2006.[9] S. Noel, S. Jajodia, L. Wang, A. Singhal, “Measuring security risk ofnetworks using attack graphs,” International Journal of Next-Generation Computing, 1(1), 2010.[10] K. Ingols, C. Scott, K. Piwowarski, K. Kratkiewicz, M. Artz, R.Cunningham, “Validating and restoring defense in depth using attack graphs,” Military Communications Conference (MILCOM), 2006. [11] S. Jajodia, S. Noel, “Topological vulnerability analysis,”CyberSituational Awareness, Springer, 2010.[12] J. Hong and D. Kim, “Scalable security models for assessingeffectiveness of moving target defenses,” IEEE/IFIP International Conference on Dependable Systems and Networks (DSN), 2014. [13] L. Wang, C. Yao, A. Singhal, S. Jajodia, “Implementing interactiveanalysis of attack graphs using relational databases,” IFIP WG 11.3 Working Conference on Data and Applications Security, 2008.[14] S. Jajodia, S. Noel, P. Kalapa, M. Albanese, J. Williams, “Cauldron:mission-centric cyber situational awareness with defense in depth,”Military Communications Conference (MILCOM), 2011.[15] R. Martin, “Making security measurable and manageable,” CrossTalk:The Journal of Defense Software Engineering, September/October 2009.[16] The MITRE Corporation, Common Vulnerabilities and Exposures: TheStandard for Information Security Vulnerability Names, https:///.[17] FIRST, Common Vulnerability Scoring System (CVSS-SIG),/cvss.[18] The MITRE Corporation, Common Weakness Enumeration: ACommunity-Developed Dictionary of Software Weakness Types, /.[19] National Institute of Standards and Technology, Official CommonPlatform Enumeration (CPE) Dictionary, /cpe.cfm. [20] The MITRE Corporation, Common Attack Pattern Enumeration andClassification: A Community Resource for Identifying and Understanding Attacks, https:///.[21] The MITRE Corporation, Cyber Observable eXpression: A StructuredLanguage for Cyber Observables, https:///.[22] D. Carasso, Exploring Splunk, CITO Research, 2012.[23] M. Zaharia, M. Chowdhury, T. Das, A. Dave, J. Ma, M. McCauley, M.Franklin, S. Shenker, and I. Stoica, “Fast and interact ive analytics over Hadoop data with Spark,” ;login: Magazine, 37(4), 2012.[24] M. Bhandarkar, “MapReduce programming with apache Hadoop,” IEEEInternational Symposium on Parallel & Distributed Processing, 2010.[25] K. Chodorow, MongoDB: The Definitive Guide, O’Reilly Media, 2013.[26] I. Robinson, J. Webber, and E. Eifrem, Graph Databases, O’Reilly,2013.[27] S. Noel, S. Jajodia, “Managing attack graph complexity through visualhierarchical aggregation,”ACM CCS Workshop on Visualization and Data Mining for Computer Security (VizSec), 2004.[28] National Institute of Standards and Technology, National VulnerabilityDatabase: Automating Vulnerability Management, Security Measurement, and Compliance Checking, /.。