Cones of matrices and successive convex relaxations of nonconvex sets
Optimization of chemically defined cell culture media – Replacing fetal bovine serum in mammalian i

ReviewOptimization of chemically defined cell culture media –Replacing fetal bovine serum in mammalian in vitro methodsJ.van der Valk a,*,D.Brunner b ,K.De Smet c ,Å.Fex Svenningsen d ,P.Honegger e ,L.E.Knudsen f ,T.Lindl g ,J.Noraberg d ,A.Price h ,M.L.Scarino i ,G.Gstraunthaler jaNCA,DWM,Fac.Veterinary Medicine,Utrecht University,Yalelaan 2,3584CM Utrecht,The Netherlandsbzet-Life Science Laboratorium,zet –Centre for Alternative and Complementary Methods to Animal Testing,Industriezeile 36/VII,4020Linz,Austria cFederal Agency for Medicines and Health Products,DG PRE Authorisation,Victor Hortaplein 40,Bus 40,B-1060Brussels,Belgium dInstitute of Molecular Medicine,Department of Neurobiology Research,University of Southern Denmark,J.B.Winslows Vej 21,DK-5000Odense C,Denmark eDepartment of Physiology,University of Lausanne,CH-1005Lausanne,Switzerland fDepartment of Public Health,Faculty of Health Sciences,University of Copenhagen,Denmark gInstitut für angewandte Zellkultur,München,Germany hIn-Vitro Methods Unit/European Centre for the Validation of Alternative Methods,Institute of Health and Consumer Protection,European Commission Joint Research Centre,Ispra (VA),Italy iINRAN,National Research Institute on Food and Nutrition,Via Ardeatina 546,00178Rome,Italy jDepartment of Physiology and Medical Physics,Innsbruck Medical University,Fritz-Pregl-Strasse 3,A-6020Innsbruck,Austriaa r t i c l e i n f o Article history:Received 10March 2010Accepted 25March 2010Available online 31March 2010Keywords:In vitro methods Fetal bovine serum Serum-freeGood cell culture practice Tissue culture 3Rsa b s t r a c tQuality assurance is becoming increasingly important.Good laboratory practice (GLP)and good manu-facturing practice (GMP)are now established standards.The biomedical field aims at an increasing reli-ance on the use of in vitro methods.Cell and tissue culture methods are generally fast,cheap,reproducible and reduce the use of experimental animals.Good cell culture practice (GCCP)is an attempt to develop a common standard for in vitro methods.The implementation of the use of chemi-cally defined media is part of the GCCP.This will decrease the dependence on animal serum,a supple-ment with an undefined and variable composition.Defined media supplements are commercially available for some cell types.However,information on the formulation by the companies is often lim-ited and such supplements can therefore not be regarded as completely defined.The development of defined media is difficult and often takes place in isolation.A workshop was organised in 2009in Copen-hagen to discuss strategies to improve the development and use of serum-free defined media.In this report,the results from the meeting are discussed and the formulation of a basic serum-free medium is suggested.Furthermore,recommendations are provided to improve information exchange on newly developed serum-free media.Ó2010Elsevier Ltd.All rights reserved.Contents 1.Introduction (1054)2.Development of a serum-free medium...................................................................................10552.1.Basal medium .................................................................................................10552.2.Supplements ..................................................................................................10550887-2333/$-see front matter Ó2010Elsevier Ltd.All rights reserved.doi:10.1016/j.tiv.2010.03.016Abbreviations:ATCC,The American Type Culture Collection;ADCF,animal-derived component-free;BSA,bovine serum albumin;CD,chemically defined;DMEM,Dulbecco minimal essential medium;DSMZ,German Collection of Microorganisms and Cell Cultures;ECACC,European Collection of Animal Cell Cultures;ECOPA,European Concensus Platform for Alternatives;ECVAM,European Centre for the Validation of Alternative Methods;EGF,epidermal growth factor;ESAC,ECVAM Scientific Advisory Committee;ESTIV,European Society of Toxicology in vitro ;FBS,fetal bovine serum;GCCP,good cell culture practice;GLP,good laboratory practice;GMP,good manufacturing practice;INVITROM,the Dutch-Belgian Society for in vitro Methods;ITS,Insulin transferrin and selenium;MEM,minimal essential medium;NGF,nerve growth factor;PET,polyethyleneterephthalate;PL,platelet lysates;SFM,serum-free medium;TEER,trans-epithelial electrical resistance;3Rs,replacement,refinement reduction of use of experimental animals.*Corresponding author.Tel.:+31302532163;fax:+31302537997.E-mail address:j.vandervalk@uu.nl (J.van der Valk).Toxicology in Vitro 24(2010)1053–1063Contents lists available at ScienceDirectToxicology in Vitroj o u r n a l ho m e p a g e :w w w.e l s e v i er.c om/locate/toxinvit2.2.1.Hormones (1055)2.2.2.Growth factors (1056)2.2.3.Protease inhibitors (1056)2.2.4.Protein hydrolysates (1056)2.2.5.Shear force protectors (1056)2.2.6.Proteins (1056)2.2.7.Vitamins (1056)2.2.8.Amino acids (1056)2.2.9.Glutamine (1056)2.2.10.Trace elements (1056)2.2.11.Lipids (1056)2.2.12.Antibiotics (1056)2.2.13.Attachment factors (1056)2.2.14.Osmolarity (1057)2.3.‘‘Building a serum-free medium (1057)2.4.Adaptation of cell lines to serum-free medium (1057)2.4.1.Reduction of serum content (1057)2.4.2.Sequential adaptation (1057)2.4.3.Adaptation with conditioned medium (1057)2.4.4.Inside adaptation (1058)3.Promoting the development and use of serum-free media (1058)rmation sources (1058)3.2.The serum-free media interactive online database(D.Brunner) (1058)3.3.Validating new media and adapted cells (1058)3.4.Other activities (1059)4.Examples of serum-free studies (1059)4.1.Human platelet lysates as a serum substitute in cell culture media(G.Gstraunthaler) (1059)4.2.Serum-free aggregating brain cell cultures(P.Honegger) (1059)anotypic brain slice cultures and defined serum-free medium Neurobasal with B27(J.Noraberg) (1059)4.4.Defined medium and serum-containing medium occasionally induce cells to use different signal transduction pathways to proliferate(Å.Fex Svenningsen) (1060)4.5.Optimization of culture conditions for human intestinal Caco-2cells to improve functional differentiation in serum-free media(M.L.Scarino) (1060)5.Conclusions (1061)6.General recommendations (1061)7.Recommendations for developing serum-free cell culture media (1061)Acknowledgements (1061)References (1061)1.IntroductionIn vitro methods are widely used tools to study physiological, biological and pharmacological activities at the cell and tissue le-vel.In addition,in vitro methods are also becoming increasingly important in the production of biological components,such as hormones and vaccines.Mammalian cells are generally grown un-der well-established conditions in incubators,where the temper-ature is typically kept at37°C with a controlled humidified gas mixture of5%CO2and95%O2.To achieve good experimental reproducibility,the composition of the cell culture medium is essential.The simplest medium is the classical Ringer’s solution (Ringer and Buxton,1887),which was developed as a solution with optimal concentrations of different salts to preserve frog heart muscle tissue.To maintain cells and tissues for longer peri-ods of time,the medium should also contain components like nutrients and pH buffering substances.This type of medium was formulated by Harry Eagle,who developed Eagle’s minimal essen-tial medium(Eagle’s MEM or MEM).MEM also contained amino acids,glucose and vitamins(Eagle,1955).A similar basal medium, MEM modified by Dulbecco(Dulbecco’s Modified Eagle’s Medium, DMEM),is still used to maintain primary cell cultures and cell lines.To keep cells alive for longer periods of time and to evaluate proliferation,migration and differentiation a basal medium must be supplemented with several factors.Serum,from animals or humans,is most commonly used to maintain and proliferate cells. Fetal bovine serum(FBS)serves most purposes and is the present standard.FBS is a complex mixture of different factors and contains a large number of components,like growth factors,proteins,vita-mins,trace elements,hormones,etc.,essential for the growth and maintenance of cells.However,the use of FBS is controversial for a number of rea-sons.First of all,the collection of serum causes unnecessary suf-fering for the unborn calf(van der Valk et al.,2004).Secondly, seasonal and continental variations in the serum composition, produces batch-to-batch variations.This,in turn,causes pheno-typical differences in the cell cultures,resulting in variations of the results.Additionally,due to the likelihood of contamination (e.g.,BSE),the use of animal products is strongly discouraged for production of new biological medicinal products(Anon, 1993;Schiff,2005;van der Valk et al.,2004).In fact,as much as20–50%of commercial FBS is virus-positive(Wessman and Levings,1999).Since in vitro methods are among the most favoured methods to replace animal methods(Hartung,2007),there is a demand for reliable and scientifically better defined cell and tissue culture methods including quality assurance(Gupta et al., 2005).Guidelines for good cell culture practice(GCCP),involving recommendations with respect to the use of serum-free media,1054J.van der Valk et al./Toxicology in Vitro24(2010)1053–1063have previously been published(Coecke et al.,2005;Hartung et al.,2002).The ECVAM Scientific Advisory Committee(ESAC) has also published a statement that strongly recommends the use of serum-free substitutes for current and new in vitro methods(ESAC,2008).Although,there is no legal basis for applying GCCP,it is recommended that GCCP becomes part of good laboratory practice(GLP)and good manufacturing practice (GMP).A workshop,to discuss the possibilities to reduce the use of FBS in cell and tissue culture was organised in2003(van der Valk et al.,2004).The report from this meeting provides clear recommendations to reduce or stop the suffering of live unborn bovine calfs from which blood is drawn for the production of FBS.Ethical,safety and scientific grounds were also given for the replacement of FBS and other animal components in cell and tissue culture methods.In2009,a follow-up workshop was organised to discuss current in vitro methods devoid of FBS,or other animal components.The workshop,held in Copen-hagen,Denmark,was organised under auspices of the European Society of Toxicology In Vitro(ESTIV),the Dutch-Belgian Society for In Vitro Methods(INVITROM)and the Danish in vitro Toxicology Network.The results from this workshop clearly demonstrate the possibilities to grow a number of different primary cell and tissue cultures as well as cell lines without the use of animal products.Furthermore,directions were provided on how to develop a serum-free,chemically defined,culture media for mammalian cell and tissue cultures in basic and applied research.This report aims at discussing the advantages of defined cell culture media and to give directions for the development of a basic defined media for a wider audience.2.Development of a serum-free mediumThe attempts to grow cells date back for at least50years (Pumper,1958;Waymouth,1955).Early attempts to grow cells in serum-free,hormone-supplemented media were performed to understand the role of serum in cell culture media.The efforts to identify all the serum components that are physiologically relevant to maintain proliferation of cells in culture,and the attempts to re-place the serum with its defined components,were not successful (Taub,1990).Since then,several different serum-free formulations have been developed where the media are supplemented with approximately10essential components(Pazos et al.,2004).About 10–20%of these strategies appeared to be successful(Pazos et al., 2004).The pioneering work by Hayashi and Sato(1976)replacing serum by the addition of selected hormones,promoting growth and stimulating differentiation of specific cells,led to the development of a good chemically defined,serum-free media (see Box1)(Barnes and Sato,1980a,b;Bjare,1992;Grillberger et al.,2009;Gstraunthaler,2003;Taub,1990).In the last10years, investigations into cell function have led to the identification of a growing number of components which have been useful in the development of modern serum-free cell culture media.Many transformed or newly transfected cell lines can successfully be maintained in these enriched serum-free media without adapta-tion and the number of cell-specific media is growing steadily. Now,more than100different available serum-free media formula-tions have been developed and can be readily used without great investments in time and money to develop one’s own(Zähringer, 2009).2.1.Basal mediumWith time,it has become clear that almost every cell type has its own requirements concerning medium supplements.Therefore, a universal(serum-free)cell and tissue culture medium may not be feasible.Different cell types have different receptors involved in cell survival,growth and differentiation and release different fac-tors to their environment.The threshold for developing or using a new(defined)medium when the current FBS containing medium works well,is high for obvious reasons.In order to aid in this process,a strategy for the development of new media will be discussed below.It is recommended to start a new formulation with a50:50(v/v) mixture of DMEM and Ham’s nutrient mixture F-12(Ham,1965). This medium formulation combines the high amino acid content of DMEM with the highly enriched Ham’s F-12(Barnes and Sato, 1980a,b;Jayme et al.,1997).Furthermore,the basal medium must contain an essential,so called,ITS supplement(insulin,transferrin and selenium).Insulin,thefirst of the components of the ITS sup-plement,has been known to be essential in cell culture from1924 and is now the most commonly used hormone in culture media (Gey and Thalhimer,1924).Transferrin is also an essential protein in culture medium where the main action is to transfer iron into the cells(Bjare,1992).Selenium is an essential trace element and acts in particular in selenoproteins which protect cells against oxi-dative stress(Helmy et al.,2000).2.2.SupplementsAlthough some cell types can be maintained in the basal medium (Bettger and McKeehan,1986;Butler and Jenkins,1989),most cells need additional supplements to survive,proliferate and/or differen-tiate.The most commonly supplied components will be discussed below.2.2.1.HormonesAll hormones of mammalian organisms are physiological con-stituents in blood circulation and are thus also present in serum Box1Culture mediaSerum-free media:serum-free media do not require sup-plementation with serum,but may contain discrete pro-teins or bulk protein fractions(e.g.,animal tissue orplant extracts)and are thus regarded as chemically unde-fined(see:chemically defined media).Protein-free media:protein-free media do not contain highmolecular weight proteins or protein fractions,but maycontain peptide fractions(protein hydrolysates),and arethus not chemically defined.Protein-free media facilitatethe down-stream processing of recombinant proteinsand the isolation of cellular products(e.g.,monoclonalantibodies),respectively.Animal-derived component-free media:media containingno components of animal or human origin.These mediaare not necessarily chemically defined(e.g.,when they con-tain bacterial or yeast hydrolysates,or plant extracts).Chemically defined media:chemically defined media donot contain proteins,hydrolysates or any other compo-nents of unknown composition.Highly purified hormonesor growth factors added can be of either animal or plantorigin,or are supplemented as recombinant products(see:animal-derived component-free media).J.van der Valk et al./Toxicology in Vitro24(2010)1053–10631055in varying amounts(Lindl and Gstraunthaler,2008;Price and Greg-ory,1982).Supplementation with hormones was therefore afirst step in the development of serum-free media(Barnes and Sato, 1980a,b;Hayashi and Sato,1976).Insulin has been shown to be obligatory in all serum-free media formulations.Other hormones most widely used in serum-free cell culture are glucocorticoids (dexamethasone and hydrocortisone),triiodothyronine(T3),and hormones that cell-specifically act by increasing intracellular cAMP levels(see Section2.3).Water-soluble complexes of steroids are commercially available.2.2.2.Growth factorsGrowth factors are generally added to the basal medium to increase cell proliferation and to stimulate specific cell functions. Traditionally,growth factors and other supplements are added as bulk in the form of fetal bovine serum(FBS).Most growth factors are highly cell type specific.Others are of more general use and can also have positive effects on several different cell types.Fibroblast growth factor-2,for example,has a positive effect on the phenotype of chondrocytes cultured in serum-free medium(Mandl et al.,2004).Some cells in culture may release growth factors thereby stimulating their own proliferation and that of other cells(Gospodarowicz and Moran,1976).2.2.3.Protease inhibitorsThe protease inhibitors that are introduced by the addition of FBS are a1-antitrypsin and a2-macroglobulin(Gstraunthaler, 2003).The inhibitors terminate the trypsination process and act beneficially by inhibiting lysosomal peptidases that may occasion-ally be released during cell turnover.Protease inhibitors thus have a protective effect on cells,but are not essential.When no protease inhibitors are supplied,one should carefully assess the trypsin concentration.2.2.4.Protein hydrolysatesProtein hydrolysates are used to deliver amino acids and small peptides.These are not essential in cell culture and the effect is somewhat controversial.In fact,some studies report a beneficial effect in cell cultures(Burteau et al.,2003;Schlaeger,1996),while other studies demonstrated that protein hydrolysates do not sup-port cell growth and that higher concentrations actually reduce cell growth(Keay,2004).Protein hydrolysates are chemically not de-fined(see Box1)and may cause problems in reproducibility and comparability of experiments.2.2.5.Shear force protectorsTurbulence in bioreactors and perfusion cultures cause shear stress in cells.Serum protects cells from this shear force(Elias et al.,1995;van der Pol and Tramper,1998).Pluronic F68has a similar effect(Zhang et al.,1992),but is not essential for ordinary cell cultures.2.2.6.ProteinsProteins are carriers for different low molecular weight compo-nents and may facilitate cell adhesion(Taub,1990).Bovine serum albumin(BSA)is often used as a lipid carrier.However,BSA is de-rived from animals and may either be contaminated or may con-tain impurities(Taub,1990).Nowadays,recombinant proteins, including albumin,are available for animal component-free cell culture(Keenan et al.,2006).2.2.7.VitaminsVitamins are provided by the basal medium.At least seven vita-mins were found to be essential for cell growth and proliferation: choline,folic acid,nicotinamide,pantothenate,pyridoxal,ribofla-vin,and thiamine(Bjare,1992;Butler and Jenkins,1989;Taub,1990).B-vitamins are necessary for cell biochemistry,and are also present in DMEM as well as in Ham-F-12.2.2.8.Amino acidsAll13essential amino acids are necessary for culturing mam-malian cells(Arg,Cys,Gln,His,Ile,Leu,Lys,Met,Phe,Thr,Trp, Tyr,and Val)and are present in high concentrations(0.5–4mM) in DMEM.The seven non-essential amino acids(Ala,Asn,Asp, Glu,Gly,Pro,and Ser)are provided by Ham’s F-12.2.2.9.GlutamineGlutamine is an essential precursor for the synthesis of proteins and ribonucleotides.It is also important respiratory fuel for rapidly dividing cells and cells that use glucose inefficiently(Glacken, 1988;Reitzer et al.,1979;Zielke et al.,1984).However,glutamine also has its drawbacks:it is unstable in solution,and glutamine breakdown and metabolism result in the production and accumu-lation of ammonia,which is toxic to cells(Schneider et al.,1996), since it is not absorbed by serum proteins in serum-free and/or protein-free media.To overcome these disadvantages,alternatives for the use of glutamine in culture media were developed.Gluta-mate,for example,can replace glutamine in cell cultures that ex-press sufficient glutamine synthetase activity.A more recent invention is the use of glutamine-containing dipeptides,alanyl-glutamine and glycyl-glutamine,commercially available under the trade name GLUTAMAX™(Christie and Butler,1994).These dipeptides are more stable and heat resistant,which even makes it possible to autoclave the media that contain these molecules. The dipeptides are intra-or extracellularly cleaved by peptidases, thereby releasing glutamine and either alanine or glycine.The availability of glutamine is therefore dependent on the peptidase activity,which results in lower rates of glutamine consumption and ammonia production.GLUTAMAX™can be substituted for glutamine on a1:1M basis.2.2.10.Trace elementsMost trace elements are available in the basal medium since Ham’s F-12is qualitatively rich in necessary trace elements (Ham,1965).2.2.11.LipidsThe role of fatty acids and lipids in cell culture has long been ne-glected.Lipids serve as energy stores,as structural constituents of cellular membranes,and in transport and signalling systems.Some lipids are available in the basal medium.However,essential fatty acids and ethanolamine are recommended as supplements. Water-soluble supplements are commercially available.Serum albumin is a carrier of fatty acids and lipids(see Section2.2.6).Essential fatty acids are components of several serum-free med-ia formulations.2.2.12.AntibioticsWherever possible,the use of antibiotics should be avoided (Kuhlmann,1996).Antibiotic-resistant microorganism may develop,and antibiotics may also have adverse effects on cell growth and function.2.2.13.Attachment factorsMost mammalian cells need a special culture substratum for cell attachment in order to survive and grow in vitro.The plastic culture dish,that is specifically treated to introduce charge and hydrophilicity into the polystyrol surface,e.g.,with poly-L(or D)-ly-sine or ornithine,is the most commonly used substrate for cell attachment.Coating the plastic dishes with other substrates like extracellular matrix components(Kleinman et al.,1987)or1056J.van der Valk et al./Toxicology in Vitro24(2010)1053–1063collagenous matrices (Kleinman et al.,1981)further facilitates the adhesion of anchorage-dependent cells.2.2.14.OsmolarityAlthough mammalian cells express a reasonable wide tolerance to osmolarity,osmolarity should always be carefully checked and compensated for when adapting to a new cell culture formulations.2.3.‘‘Building ”a serum-free mediumAs shown above,to exclude FBS from a cell and tissue culture medium,and still maintain cell adhesion,growth and proliferation it is important to include a large number of several components in the cell culture medium.In Fig.1,a schematic modular approach for the development of serum-free media is shown as a ‘‘media pyramid”.The bottom of the pyramid contains the basal medium,which includes DMEM/Ham’s F-12(50:50,v/v),supplemented with insulin–transferrin–selenium (ITS).To make adherent cells stick to the bottom of the culture vessel,coating with components of the extracellular matrix should be considered.Cell attachment factors are often required for serum-free culture.The next step in media formulation development is the addition of specific hormones and growth factors.Epidermal growth factor (EGF)and glucocorticoids (hydrocortisone and dexamethasone),for example,are present in most media.Depending on the cell type,additional cell-specific growth factors may also be needed,like nerve growth factor (NGF)for neurons.It has been demon-strated that cultures of epithelial cells need a supplementation with agonists,that specifically elevates cellular cAMP levels (Gstraunthaler,2003).In this respect,also forskolin and cholera toxin,although acting as strong pharmacological agents,were used as in vitro mitogens.The tip of the pyramid represents increased specificity in ser-um-free media composition:the addition of lipids,antioxidants and/or specific vitamins.Retinoic acid (vitamin A)is an additive re-quired in cell culture media for a number of epithelial cell types.Vitamin E (a -tocopherol)and ascorbate (vitamin C)are presum-ably acting as antioxidants.Other antioxidants found in serum-freemedia formulations are b -mercaptoethanol (b -ME)and selenium (see above).2.4.Adaptation of cell lines to serum-free mediumThere are several approaches to adapt cultured cells to a serum-free medium (Fig.2).Typically,a cell culture has to undergo a gradual weaning process which involves progressive adaptation to lower serum concentrations until serum-free conditions are reached.The cultures to be adapted should be in the logarithmic phase of growth and should have viability over 90%.However,one should also keep in mind that an unwanted selection of a change in the population of cells,during the adaptation process,by indirectly selecting cells capable to grow in serum-free media,may occur.Therefore,it is necessary to check the performance of cultures and to monitor cellular morphology and function during weaning.In order to aid the process of weaning several adaption proto-cols are listed below (and in Fig.2):2.4.1.Reduction of serum contentIn this protocol,serum content is reduced at each passage until 0.1%serum is reached.After cultivation in normal medium con-taining 10%FBS,the consequent serum reduction steps (from 5%to 0.1%FBS)are carried out in serum-free,hormone-supplemented medium.2.4.2.Sequential adaptationSimilar to protocol 1,cells are passaged into mixtures of serum-containing and serum-free media,until complete serum-free condition is reached.If the last step,changing from 75%to 100%serum-free,is too stressful for the cells,it is recommended to keep the cell culture in a 10%serum-containing and 90%ser-um-free medium mixture for 2–3passages,before switching to a complete serum-free medium.2.4.3.Adaptation with conditioned mediumThis adaptation follows protocol 2,however,cells are passaged into decreasing mixtures of conditioned media from the passage before.in serum-free culture media pre-coating of culture vessels with: collagen type I, type IV , laminin, fibronectin,Basement Membrane Matrigel™basal medium: DMEM / Ham F-12 (50:50, v/v) + ITSgrowth factors: EGF, FGF, NGF, IGF-1, PDGF, VEGF, TGF-βhormones:glucocorticoids, thyroid hormones, cell- specific agonists that signal via cAMP (ADH, PTH, PGE2, glucagon)lipids:fatty acids, cholesterol, ethanolamine vitamins acting as anti-oxidants: α-tocopherolascorbic acid, vitamins: retinoic acidchemically defined, serum-free media:β-MEJ.van der Valk et al./Toxicology in Vitro 24(2010)1053–106310572.4.4.Inside adaptationIn this protocol,freshly seeded cells are weaned in serum-free medium,and cultures are grown to confluence.The confluent monolayer is then passaged into serum-free medium.3.Promoting the development and use of serum-free media rmation sourcesBefore using the experimental approach to set up a serum-free medium for a given cell type,cell lines or tissue culture,a search for already existing media formulations should be performed This can be done by a thorough literature survey,or by a search in a re-cently established serum-free media online database(see Section 3.2).There are several databases that contain information on com-mercially available serum-free media formulations and supple-ments(Anon,2009a,b,c).Approximately450different serum-free cell culture media formulations are now commercially available, but only for a limited number of cell types(Anon,2009a,b,c). Regrettably,the formulations of specific supplements for the com-mercially available media are generally not available,and those can therefore not be considered as fully defined media.Such for-mulations have also been changed without informing the users (Chen et al.,2008;Cressey,2009),and supplements with the same name may differ in formulation between suppliers.Today,the information on available serum-free media formula-tions,particularly when these are not commercially available,is unfortunately scarce.Nevertheless,development of serum-free media and cell adaptation protocols are ongoing processes in sev-eral laboratories,often without knowledge about research pro-cesses,experiences and results of other laboratories regarding this topic.This is partly due to a lack in communication between labs and particularly the lack of a common forum where such for-mulations could be posted.It is recommended that these obstacles must be overcome in order to encourage future use and develop-ment of serum-free media.It is further recommended to collect formulations of‘‘in lab developed”media in databases,where access to reliable protocols including detailed formulations,should be free.It is also recom-mended to publish established protocols in dedicated online databases like Springer Protocols and Nature Protocols.When pub-lishing studies with newly developed serum-free formulations spe-cific keywords should be used in the publication to enable easy retrieval of the publications.Key words like3R,serum-free media or defined media are recommended.3.2.The serum-free media interactive online database(D.Brunner)To make the search for serum-free media easier,a new collec-tion of commercially available serum-free media has been devel-oped in a free accessible unique interactive online database (Brunner et al.,2010;Falkner et al.,2006).Specifications of serum-free media(i.e.,ability to maintain cells of specific organism,organs,tissue,cell type and disease)were col-lected and systematically arranged with respect to specific stan-dards(ICD2007of WHO and ITIS).Additional commercially available cell lines,hybridoma and primary cells from ATCC,ECACC and DSMZ are included in the database to allow a‘‘reverse search”by specifying the used cells to gain a serum-free medium.This search modus is based upon comparison of specifications and can also be used tofind most similar serum-free media.Furthermore,the degree of chemical definition,e.g.,serum-free (SFM),animal-derived component-free(ADCF)or chemically de-fined(CD),and the kind of medium,e.g.,basal media,media sup-plements,or full replacement media can be selected.Presently, 452serum-free media and4817continuous cell lines,hybridoma lines and primary cultures from ATCC,ECACC and DSMZ that are commercially available are included in the database.Despite extensive search for serum-free media and adapted cell lines,there is still a lack of detailed information from companies and suppliers. It is intended to open the database for interactive exchange of information and guidelines from experts in thefield in order to continuously improve and extend the serum-free online database. The database is accessible at .3.3.Validating new media and adapted cellsIn a statement on the use of FBS and other animal-derived supplements(ESAC,2008),the ECVAM Scientific Advisory Commit-tee strongly argues for the development of new serum-free in vitro culture methods.Furthermore,when an in vitro method using serum-containing media is presented to ECVAM for validation,a justification for using serum must be provided.To promote the use of serum-free media,ECVAM(European Centre for the Valida-tion of Alternative Methods)will encourage the submitters of new tests systems for validation studies to make their protocols public if their model is designed under serum-free conditions.Existing culture methods where animal components are being replaced, should be validated against serum-containing media to ensure that1058J.van der Valk et al./Toxicology in Vitro24(2010)1053–1063。
方差协方差矩阵中文翻译
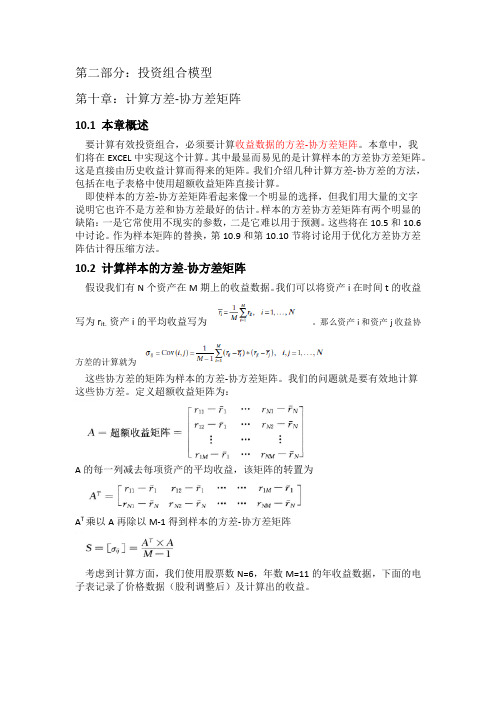
第二部分:投资组合模型第十章:计算方差-协方差矩阵10.1 本章概述要计算有效投资组合,必须要计算收益数据的方差-协方差矩阵。
本章中,我们将在EXCEL中实现这个计算。
其中最显而易见的是计算样本的方差协方差矩阵。
这是直接由历史收益计算而得来的矩阵。
我们介绍几种计算方差-协方差的方法,包括在电子表格中使用超额收益矩阵直接计算。
即使样本的方差-协方差矩阵看起来像一个明显的选择,但我们用大量的文字说明它也许不是方差和协方差最好的估计。
样本的方差协方差矩阵有两个明显的缺陷:一是它常使用不现实的参数,二是它难以用于预测。
这些将在10.5和10.6中讨论。
作为样本矩阵的替换,第10.9和第10.10节将讨论用于优化方差协方差阵估计得压缩方法。
10.2 计算样本的方差-协方差矩阵假设我们有N个资产在M期上的收益数据。
我们可以将资产i在时间t的收益写为r it。
资产i的平均收益写为。
那么资产i和资产j收益协方差的计算就为这些协方差的矩阵为样本的方差-协方差矩阵。
我们的问题就是要有效地计算这些协方差。
定义超额收益矩阵为:A的每一列减去每项资产的平均收益,该矩阵的转置为A T乘以A再除以M-1得到样本的方差-协方差矩阵考虑到计算方面,我们使用股票数N=6,年数M=11的年收益数据,下面的电子表记录了价格数据(股利调整后)及计算出的收益。
我们用数字例子来说明计算方差-协方差矩阵的矩阵方法。
我们通过减去资产各自的平均收益,得到超额收益矩阵(下面的表格中42到52行)在第55-61行中我们计算样本方差-协方差矩阵。
10.2.1一个稍微更有效率的替代方法正如你所期望的那样,的确存在其他计算方差-协方差矩阵的可选方法。
这里讲的方法跳过了超额收益的计算,并且直接使用单元格B71:B76中的公式进行计算。
他通过使用数组函数=MMULT(TRANSPOSE(B23:G33-B35:G35),B23:G33-B35:G35)/10.通过写B23:G33-B35,我们直接将每项收益减去平均收益得到超额收益向量。
ActivityTheory活动理论
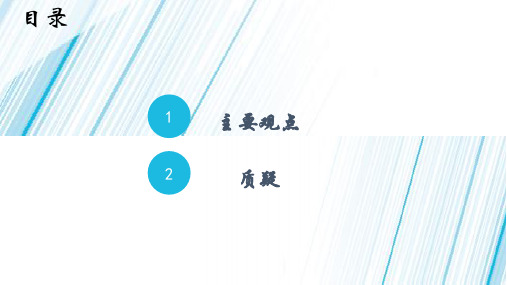
多重声音思想
多重声音思想认为各种不同观点的话语都要被融合接纳与利用, 而在传统的课堂中,一切学习活动目标与过程都已经被预先设定, 难以倾听学习者的多重声音,难以考虑学习者的不同差异,无法包 含多种持有不同理解、不同观点的主体与共同体,也就无法相互争 论、协商与融合。活动理论关注的不是知识传递,而是人们参与的 学习活动的过程,所以应该充分给予学生表达自己观点的机会,并 且构建课程学习活动共同体,建立教师之间、师生之间、学生之间 互助的学习共同体,通过沟通学习的模式,分享经验、表达观点, 促进多元声音融合。
理解:在拓展性学习中,学习者所学习的知识时并不是固定的, 先前就已经存在的,而是学习者通过集体活动,一边创造知识一 边学习知识,随后再将习得的知识运用于实践。这与一般意义上 的学习是有较大差别的。一般我们所说的学习的知识是比较固定 的,并且已经被他人证明过是正确且合理的。而拓展性学习主要 是探索“未知领域”,根据自己的实践总结出知识并学习。比如 说,陶行知先生所提出的“生活教育”就提倡拓展性学习。
举例:
在课堂中,老师正在讲解新课,突然有两个学生发生了争执。在 这种情况下,学生的争执就是进入课堂教学这个活动系统的新因素, 打破了原先正常教学的“平衡”,产生了矛盾。这时,活动系统就 因为这个矛盾而发生变化,从先前的讲课活动转化为处理学生争执 的活动。
媒介工具
从活动理论看,工具是学 习者和学习内容的中介,是学 习内容的制品,工具改变学习 活动中知识传递、呈现、处理 等的方式。工具是支持活动开 展的条件,在活动中使用媒介 工具,不仅使学习者获取知识, 也能培养他们的思维方式和价 值观念。如电化教学,慕课等 的推广,不仅帮助学生获取记 忆知识,而且也能使他们意识 到科技在生活中的作用。
拓展性学习
Projective Nonnegative Matrix Factorization for Image Compression and Feature Extraction
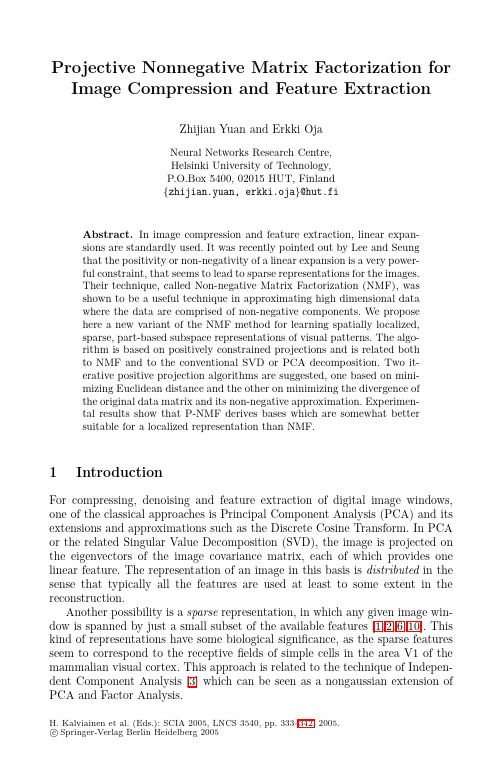
Projective Nonnegative Matrix Factorization for Image Compression and Feature ExtractionZhijian Yuan and Erkki OjaNeural Networks Research Centre,Helsinki University of Technology,P.O.Box5400,02015HUT,Finland{zhijian.yuan,erkki.oja}@hut.fiAbstract.In image compression and feature extraction,linear expan-sions are standardly used.It was recently pointed out by Lee and Seungthat the positivity or non-negativity of a linear expansion is a very power-ful constraint,that seems to lead to sparse representations for the images.Their technique,called Non-negative Matrix Factorization(NMF),wasshown to be a useful technique in approximating high dimensional datawhere the data are comprised of non-negative components.We proposehere a new variant of the NMF method for learning spatially localized,sparse,part-based subspace representations of visual patterns.The algo-rithm is based on positively constrained projections and is related bothto NMF and to the conventional SVD or PCA decomposition.Two it-erative positive projection algorithms are suggested,one based on mini-mizing Euclidean distance and the other on minimizing the divergence ofthe original data matrix and its non-negative approximation.Experimen-tal results show that P-NMF derives bases which are somewhat bettersuitable for a localized representation than NMF.1IntroductionFor compressing,denoising and feature extraction of digital image windows, one of the classical approaches is Principal Component Analysis(PCA)and its extensions and approximations such as the Discrete Cosine Transform.In PCA or the related Singular Value Decomposition(SVD),the image is projected on the eigenvectors of the image covariance matrix,each of which provides one linear feature.The representation of an image in this basis is distributed in the sense that typically all the features are used at least to some extent in the reconstruction.Another possibility is a sparse representation,in which any given image win-dow is spanned by just a small subset of the available features[1,2,6,10].This kind of representations have some biological significance,as the sparse features seem to correspond to the receptivefields of simple cells in the area V1of the mammalian visual cortex.This approach is related to the technique of Indepen-dent Component Analysis[3]which can be seen as a nongaussian extension of PCA and Factor Analysis.H.Kalviainen et al.(Eds.):SCIA2005,LNCS3540,pp.333–342,2005.c Springer-Verlag Berlin Heidelberg2005334Z.Yuan and E.OjaRecently,it was shown by Lee and Seung[4]that positivity or non-negativity of a linear expansion is a very powerful constraint that also seems to yield sparse representations.Their technique,called Non-negative Matrix Factoriza-tion(NMF),was shown to be a useful technique in approximating high di-mensional data where the data are comprised of non-negative components.The authors proposed the idea of using NMF techniques tofind a set of basis func-tions to represent image data where the basis functions enable the identification and classification of intrinsic“parts”that make up the object being imaged by multiple observations.NMF has been typically applied to image and text data [4,9],but has also been used to deconstruct music tones[8].NMF imposes the non-negativity constraints in learning the basis images. Both the values of the basis images and the coefficients for reconstruction are all non-negative.The additive property ensures that the components are combined to form a whole in the non-negative way,which has been shown to be the part-based representation of the original data.However,the additive parts learned by NMF are not necessarily localized.In this paper,we start from the ideas of SVD and NMF and propose a novel method which we call Projective Non-negative Matrix Factorization(P-NMF), for learning spatially localized,parts-based representations of visual patterns. First,in Section2,we take a look at a simple way to produce a positive SVD by truncating away negative parts.Section3briefly reviews Lee’s and Seung’s ing this as a baseline,we present our P-NMF method in Section4. Section5gives some experiments and comparisons,and Section6concludes the paper.2Truncated Singular Value DecompositionSuppose that our data1is given in the form of an m×n matrix V.Its n columns are the data items,for example,a set of images that have been vectorized by row-by-row scanning.Then m is the number of pixels in any given image.Typically, n>m.The Singular Value Decomposition(SVD)for matrix V isV=UDˆU T,(1) where U(m×m)andˆU(n×m)are orthogonal matrices consisting of the eigenvectors of VV T and V T V,respectively,and D is a diagonal m×m matrix where the diagonal elements are the ordered singular values of V.Choosing the r largest singular values of matrix V to form a new diagonal r×r matrixˆD,with r<m,we get the compressive SVD matrix X with given rank r,X=UˆDˆU T.(2)1For clarity,we use here the same notation as in the original NMF theory by Lee and SeungProjective Nonnegative Matrix Factorization335 Now both matrices U andˆU have only r columns corresponding to the r largest eigenvalues.The compressive SVD gives the best approximation X of the matrix V with the given compressive rank r.In many real-world cases,for example,for images,spectra etc.,the original data matrix V is non-negative.Then the above compressive SVD matrix X fails to keep the nonnegative property.In order to further approximate it by a non-negative matrix,the following truncated SVD(tSVD)is suggested.We simply truncate away the negative elements byˆX=12(X+abs(X)).(3)However,it turns out that typically the matrixˆX in(3)has higher rank than X. Truncation destroys the linear dependences that are the reason for the low rank. In order to get an equal rank,we have to start from a compressive SVD matrix X with lower rank than the given r.Therefore,tofind the truncated matrix ˆX with the compressive rank r,we search all the compressive SVD matrices X with the rank from1to r and form the corresponding truncated matrices.The one with the largest rank that is less than or equal to the given rank r is the truncated matrixˆX what we choose as thefinal non-negative approximation. This matrix can be used as a baseline in comparisons,and also as a starting point in iterative improvements.We call this method truncated SVD(t-SVD).Note that the tSVD only produces the non-negative low-rank approximation ˆX to the data matrix V,but does not give a separable expansion for basis vectors and weights as the usual SVD expansion.3Non-negative Matrix FactorizationGiven the nonnegative m×n matrix V and the constant r,the Nonnegative Matrix Factorization algorithm(NMF)[4]finds a nonnegative m×r matrix W and another nonnegative r×n matrix H such that they minimize the following optimality problem:minW,H≥0||V−WH||.(4) This can be interpreted as follows:each column of matrix W contains a basis vector while each column of H contains the weights needed to approximate the corresponding column in V using the basis from W.So the product WH can be regarded as a compressed form of the data in V.The rank r is usually chosen so that(n+m)r<nm.In order to estimate the factorization matrices,an objective function defined by the authors as Kullback-Leibler divergence isF=mi=1nµ=1[V iµlog(WH)iµ−(WH)iµ].(5)This objective function can be related to the likelihood of generating the images in V from the basis W and encodings H.An iterative approach to336Z.Yuan and E.Ojareach a local maximum of this objective function is given by the following rules[4,5]:W ia←W iaµV iµ(WH)iµH aµ,W ia←W iajW ja(6)H aµ←H aµi W iaV iµ(WH)iµ.(7)The convergence of the process is ensured2.The initialization is performed using positive random initial conditions for matrices W and H.4The Projective NMF Method4.1Definition of the ProblemThe compressive SVD is a projection method.It projects the data matrix V onto the subspace of the eigenvectors of the data covariance matrix.Although the truncated method t-SVD outlined above works and keeps nonnegativity, it is not accurate enough for most cases.To improve it,for the given m×n nonnegative matrix V,m<n,let us try tofind a subspace B of R m,and an m×m projection matrix P with given rank r such that P projects the nonnegative matrix V onto the subspace B and keeps the nonnegative property, that is,PV is a nonnegative matrix.Finally,it should minimize the difference ||V−PV||.This is the basic idea of the Projective NMF method.We can write any symmetrical projection matrix of rank r in the formP=WW T(8) with W an orthogonal(m×r)matrix3.Thus,we can solve the problem by searching for a nonnegative(m×r)matrix W.Based on this,we now introduce a novel method which we call Projective Non-negative Matrix Factorization(P-NMF)as the solution to the following optimality problemminW≥0||V−WW T V||,(9)where||·||is a matrix norm.The most useful norms are the Euclidean dis-tance and the divergence of matrix A from B,defined as follows:The Euclidean distance between two matrices A and B is2The matlab program for the above update rules is available at under the”Computational Neuroscience”discussion category.3This is just notation for a generic basis matrix;the solution will not be the same as the W matrix in NMF.Projective Nonnegative Matrix Factorization337||A−B||2=i,j(A ij−B ij)2,(10) and the divergence of A from BD(A||B)=i,j (A ij logA ijB ij−A ij+B ij).(11)Both are lower bounded by zero,and vanish if and only if A=B.4.2AlgorithmsWefirst consider the Euclidean distance(10).Define the functionF=12||V−WW T V||2.(12)Then the unconstrained gradient of F for W,∂F∂w ij,is given by∂F∂w ij=−2(VV T W)ij+(WWT VV T W)ij+(VVT WW T W)ij.(13)Using the gradient we can construct the additive update rule for minimization,W ij←W ij−ηij∂F∂w ij(14)whereηij is the positive step size.However,there is nothing to guarantee that the elements W ij would stay non-negative.In order to ensure this,we choose the step size as follows,ηij=W ij(WW T VV T W)ij+(VV T WW T W)ij.(15)Then the additive update rule(14)can be formulated as a multiplicative update rule,W ij←W ij(VV T W)ij(WW T VV T W)ij+(VV T WW T W)ij.(16)Now it is guaranteed that the W ij will stay nonnegative,as everything on the right-hand side is nonnegative.For the divergence measure(11),we follow the same process.First we calcu-late the gradient∂D(V||WW T V)∂w ij=k(W T V)jk+lW lj V ik(17)−kV ik(W T V)jk/(WW T V)ik(18)−k V iklW lj V lk/(WW T V)lk.(19)338Z.Yuan and E.OjaUsing the gradient,the additive update rule becomesW ij ←W ij +ζij ∂D (V ||WW T V )∂w ij(20)where ζij is the step size.Choosing this step size as following,ζij =W ij k V ik [(W T V )jk /(WW T V )ik + l W lj V lk /(WW T V )lk ].(21)we obtain the multiplicative update ruleW ij ←W ij k (W T V )jk + l W lj V ik k V ik ((W T V )jk /(WW T V )ik + l W lj V lk /(WW T V )lk ).(22)It is easy to see that both multiplicative update rules (16)and (22)can ensure that the matrix W is non-negative.4.3The Relationship Between NMF and P-NMFThere is a very obvious relationship between our P-NMF algorithms and the original paring the two optimality problems,P-NMF (9)and the original NMF (4),we see that the weight matrix H in NMF is simply replaced by W T V in our algorithms.Both multiplicative update rules (16)and (22)are obtained similar to Lee and Seung’s algorithms [5].Therefore,the convergence of these two algorithms can also be proved following Lee and Seung [5]by noticing that the coefficient matrix H is replaced by WV .4.4The Relationship Between SVD and P-NMFThere is also a relationship between the P-NMF algorithm and the SVD.For the Euclidean norm,note the similarity of the problem (9)with the conventional PCA for the columns of V .Removing the positivity constraint,this would be-come the usual finite-sample PCA problem,whose solution is known to be an orthogonal matrix consisting of the eigenvectors of VV T .But this is the matrix U in the SVD of eq.(1).However,now with the positivity constraint in place,the solution will be something quite different.5Simulations 5.1Data PreparationAs experimental data,we used face images from the MIT-CBCL database and derived the NMF and P-NMF expansions for them.The training data set con-tains 2429faces.Each face has 19×19=361pixels and has been histogram-equalized and normalized so that all pixel values are between 0and 1.ThusProjective Nonnegative Matrix Factorization339 the data matrix V which now has the faces as columns is361×2429.This matrix was compressed to rank r=49using either t-SVD,NMF,or P-NMF expansions.5.2Learning Basis ComponentsThe basis images of tSVD,NMF,and P-NMF with dimension49are shown in Figure1.For NMF and P-NMF,these are the49columns of the corresponding matrices W.For t-SVD,we show the49basis vectors of the range space of the rank-49nonnegative matrixˆX,obtained by ordinary SVD of this matrix.Thus the basis images for NMF and P-NMF are truly non-negative,while the t-SVD only produces a non-negative overall approximation to the data but does not give a separable expansion for basis vectors and weights.All the images are displayed with the matlab command”imagesc”without any extra scale.Both NMF and P-NMF bases are holistic for the training set. For this problem,the P-NMF algorithm converges about5times faster than NMF.Fig.1.NMF(top,left),t-SVD(bottom,left)and the two versions of the new P-NMF method(right)bases of dimension49.Each basis component consists of19×19pixels340Z.Yuan and E.OjaFig.2.The original face image(left)and its reconstructions by NMF(top row),the two versions of the new P-NMF method under100iterative steps(second and third rows),and t-SVD(bottom row).The dimensions in columns2,3,and4are25,49and 81,respectively5.3Reconstruction AccuracyWe repeated the above computations for ranks r=25,49and81.Figure2 shows the reconstructions for one of the face images in the t-SVD,NMF,and P-NMF subspaces of corresponding dimensions.For comparison,also the original face image is shown.As the dimension increases,more details are recovered. Visually,the P-NMF method is comparable to NMF.The recognition accuracy,defined as the Euclidean distance between the orig-inal data matrix and the recognition matrix,can be used to measure the perfor-mance quantitatively.Figure3shows the recognition accuracy curves of P-NMF and NMF under different iterative steps.NMF converges faster,but when the number of steps increases,P-NMF works very similarly to NMF.One thing to be noticed is that the accuracy of P-NMF depends on the initial values.Al-though the number of iteration steps is larger in P-NMF for comparable error with NMF,this is compensated by the fact that the computational complexity for one iteration step is considerably lower for P-NMF,as only one matrix has to be updated instead of two.Projective Nonnegative Matrix Factorization341Fig.3.Recognition accuracies(unit:108)versus iterative steps using t-SVD,NMF and P-NMF with compressive dimension496ConclusionWe proposed a new variant of the well-known Non-negative Matrix Factorization (NMF)method for learning spatially localized,sparse,part-based subspace rep-resentations of visual patterns.The algorithm is based on positively constrained projections and is related both to NMF and to the conventional SVD decompo-sition.Two iterative positive projection algorithms were suggested,one based on minimizing Euclidean distance and the other on minimizing the divergence of the original data matrix and its pared to the NMF method, the iterations are somewhat simpler as only one matrix is updated instead of two as in NMF.The tradeoffis that the convergence,counted in iteration steps, is slower than in NMF.One purpose of these approaches is to learn localized features which would be suitable not only for image compression,but also for object recognition. Experimental results show that P-NMF derives bases which are better suitable for a localized representation than NMF.It remains to be seen whether they would be better in pattern recognition,too.342Z.Yuan and E.OjaReferences1. A.Bell and T.Sejnowski.The”independent components”of images are edgefilters.Vision Research,37:3327–3338,1997.2. A.Hyv¨a rinen and P.Hoyer.Emergence of phase and shift invariant features bydecomposition of natural images into independent feature subspaces.Neural Com-putation,13:1527–1558,2001.3. A.Hyv¨a rinen,J.Karhunen,and E.Oja.Independent Component Analysis.Wiley,New York,2001.4. D.D.Lee and H.S.Seung.Learning the parts of objects by non-negative matrixfactorization.Nature,401:788–791,1999.5. D.D.Lee and H.S.Seung.Algorithms for non-negative matrix factorization.InNIPS,pages556–562,2000.6. B.A.Olshausen and D.J.Field.Natural image statistics and efficient coding.Network,7:333–339,1996.7.P.Paatero and U.Tapper.Positive Matrix Factorization:A non-negative factormodel with optimal utilization of error estimations of data values.Environmetrics, 5,111-126,1997.8.T.Kawamoto,K.Hotta,T.Mishima,J.Fujiki,M.Tanaka and T.Kurita.Esti-mation of single tones from chord sounds using non-negative matrix factorization.Neural Network World,3,429-436,July2000.9.L.K.Saul and D.D.Lee.Multiplicative updates for classification by mixture mod-ela.In Advances in Neural Information Processing Systems14,2002.10.J.H.van Hateren and A.van der Schaaf.Independent componentfilters of natu-ral images compared with simple cells in primary visual cortex.Proc.Royal Soc.London B,265:2315–2320,1998.。
Integrating Symmetric Nonnegative Matrix Factorization and Normalized Cut

Integrating Symmetric Nonnegative Matrix Factorization and Normalized CutSpectral ClusteringZhichen Xia a and Chris Ding ba College of Electronic Science&Engineering,Jilin University,Changchun,Chinab Department of Comp.Sci.&Eng.,University of Texas,Arlington,Texas,USAEmails:xiazhichenjlu@,chqding@AbstractIn this paper,we integrate symmetric NMF and nor-malized cut into a single clustering framework and derive the computational algorithm.Another contribution is to provide a new matrix inequality which is useful for the analysis of4-th order matrix polynomials.We perform experiments on three real-life data sets to show the effec-tiveness of the proposed algorithm.We also demonstrate the importance of the orthogonality among matrix factors.I.IntroductionNonnegative Matrix Factorization(NMF)has been pop-ularly studied in data mining and machine learning areas since the initial work of Lee and Seung[9].Originally proposed as method forfinding matrix factors with parts-of-whole interpretations[9],NMF has been applied to a number of applied areas,environmetrics[13],chemo-metrics[19],pattern recognition[10],multimedia data analysis[3],text mining[14]and DNA gene expression analysis[1].Algorithmic extensions of NMF have been developed to accommodate a variety of objective func-tions[4],[6]and a variety of data analysis problems,in-cluding classification[15]and collaborativefiltering[17], constrained clustering[11],[18].One of the important features of NMF is its clustering capabilities.It was shown [5],[7]that NMF essentially solves a matrix clustering problem.Symmetric NMF(SNMF)[5],[12]deals with nonneg-ative factorization of a symmetric matrix.In its simple form,SNMF essentially solves a graph clustering problem with a pairwise similarity matrix W.On the other hand, Laplacian-based spectral clustering such as normalized cut[16]is now a standard approach for graph clustering.In this paper,we integrate the SNMF graph clustering and normalized cut graph clustering into a single frame-work.We derive a computational algorithm with rigorous analysis.As another contribution of this paper,we provide a new matrix inequality which is useful for analysis of4-th order matrix polynomials involved in the SNMF problem.We mention that combining NMF with Laplacian reg-ularization have been studied in[2],[8].However,both papers study one matrix factor at a time which involves a2nd order matrix polynomials.The symmetric case is harder to analysis due to the4th order matrix polynomial. Our new matrix inequality resolves this problem.The rest of the paper is organized as follows.Section 2we discuss integrating SNMF and Normalized Cut and computational algorithm.Section3we present the new matrix inequality and its proof.Section4provides a detailed analysis of algorithm in Section2.Section5-7 presents experimental results.Section8gives a summary.II.Integrating NMF and Normalized CutGiven input pairwise similarity matrix W,the symmet-ric NMFminH∥W−HH T∥2,s.t.H≥0(1)has been a popular clustering algorithm.The essence of the symmetric NMF is to discover the block structure in W as represented by HH T.On the other hand,the Lapplacian based normalized cut clustering algorithm solve the following optimization minHTr H T(D−W)H,s.t.H≥0,H T DH=I(2) In this paper,we integrate these two approaches into a unified formulationminH∥W−HH T∥2+2αTr H T(D−W)H,(3) s.t.H≥0,H T DH=I (4)2010 IEEE International Conference on Data Mining WorkshopsOne contribution of this paper is to derive a computational algorithm to solve the integrated clustering formulation.The iterative algorithm is given belowH ik ←H ik[(1+α)(W H )ik(HH T H +DH Λ)ik ]1/4(5)where Λis the Lagrangian multiplier that enforce the condition H T DH =I and its value is given byΛ=[(1+α)H T W H −H T HH T H ]+(6)Here A +=(A +abs (A ))/2is the positive part of A .The correctness and convergence of the above algorithm can be rigorously proved.Details are provided in §4.III.A useful matrix inequalityAnother contribution of this paper is to provide a new generic matrix inequality:Tr (HAH T HBH T )(7)≤∑ik(H ′AH ′T H ′B +H ′BH ′T HA 2)ik H 4ikH ′3ikwhere A,B ∈ℜK ×K +,H,H ′∈ℜn ×K+are nonnegative matrices;and A,B are symmetric:A =A T ,B =B T .This inequality is useful when analyzing objective func-tions involving 4-th order matrix polynomials,such as the symmetric NMF of Eq.(1).We will use this inequality to prove the convergence of the algorithm of Eq.(6).Proof of the inequality Eq.(7)Let H ik =H ′iku ik .The 2nd term in RHS of Eq.(7)is ∑ik(H ′BH ′TH ′A )ik H 4ikH ′3ik=∑ijkrpqH ′ip B pq H ′jq H ′jr A rk H ′ik u 4ik(8)Now,switching indexes:i <=>j,p <=>q,r <=>k ,we obtain∑ik (H ′BH ′T H ′A )ik H 4ik H ′3ik =∑ijkrpqH ′jq B qp H ′ip H ′ik A kr H ′jr u 4jr(9)The 1st term in RHS of Eq.(7)is∑ik(H ′AH ′TH ′B )ik H 4ikH ′3ik=∑ijkrpqH ′jr A rk H ′ik H ′ip B pq H ′jq u 4jq(10)Now,switching indexes:i <=>j,p <=>q,r <=>k ,we obtain∑ik (H ′AH ′T H ′B )ik H 4ik H ′3ik=∑ijkrpqH ′ik A kr H ′jr H ′jq B qp H ′ip u 4ip (11)Carefully examination of the RHS of Eqs.(8-11)show thatthey are identical except u 4terms.Adding Eqs.(8-11),we obtain that the RHS of Eq.(7)is equal to ∑ijkrpqH ′ip B pq H ′jq H ′jr A rk H ′iku 4ik +u 4jr +u 4jq +u 4ip4(12)The LHS of Eq.(7)is equal to∑ijkrpqH ′ip B pq H ′jq H ′jr A rk H ′ik u ik u jr u jq u ip(13)Therefore,if we can establishu ik u jr u jq u ip ≤u 4ik +u 4jr +u 4jq +u 4ip4,(14)then the inequality Eq.(7)holds.For any a,b,c,d >0,we havea 4+b 4≥2a 2b 2,c 4+d 4≥2c 2d 2,(ab )2+(cd )2≥2(ab )(cd ).Thusa 4+b 4+c 4+d 4≥2(a 2b 2+c 2d 2)≥4(ab )(cd ).This is Eq.(14).QED.IV .Analysis of Algorithm Eq.(5)Here we prove the correctness and convergence of Algorithm Eq.(5).We note that since ∥W −HH T ∥2=Tr (W 2−2H T W H +HH T HH T ),the optimization of Eq.(3)becomesmin Tr [−2(1+α)H T W H+HH T HH T (15)+W 2+2αH TDH ]The last two terms are constants.Thus the optimizationbecomesmax HTr [2(1+α)H T W H −HH T HH T ](16)s.t.H ≥0,H T DH =I(17)Correctness .We first prove the correctness of the algorithm,i.e.,we haveTheorem 1.At convergence,the solution of Eq.(5)satisfies the KKT condition of optimization theory.Proof .Introducing Lagrangian multipliers Λto enforce H T DH =I ,we maximizeℒ=Tr [2(1+α)H T W H −HH T HH T −2Λ(H T DH −I )]The KKT optimality condition for complementary slack-ness for the constraint H ≥0is(∂ℒ∂H )ik H ik=4[(1+α)W H −HH T H −DH Λ]ik H ik =0(18)At convergence,Eq.(5)can be written as[(1+α)W H −HH TH −DH Λ]ik H 4ik=0(19)Eq.(18)is identical to Eq.(17).This is because for Eq.(18)to hold,at least one of the two factors must be zero.If the first factor is zero,then Eq.(17)also holds.If the secondfactor H 4ik=0,then H ik =0and Eq.(17)holds again.We can see that the inverse is true,i.e.,if Eq.(17)holds,so does Eq.(18).This proves that if the algorithm Eq.(5)converges,the solution satisfies the KKT condition,i.e.,the solution is an optimal solution.QED.From the KKT condition Eq.(17),summing over i ,we obtainΛkk =[(1+α)H T W H −H T HH T H ]kk(20)This gives Lagrangian multipliers the values on the diag-onal.For non-diagonal elements,we use the Lagrangianwithout nonnegativity bining these two together,we obtain Eq.(6).Convergences .We now prove that the algorithm converges.We have Theorem 2.The Lagrangian functionℒ=Tr [2(1+α)H T W H −HH T HH T −2ΛH T DH ](21)increase monotonically under successive update of thealgorithm Eq.(5).Using this theorem,we have ℒ(H 0)≤ℒ(H 1)≤ℒ(H 2)≤⋅⋅⋅≤ℒ(H t)≤ℒ(Ht +1)⋅⋅⋅Because ℒ(H )is bounded from above due to H T DH =I .Therefore,algorithm Eq.(5)converges.Proof of Theorem 2.We use the auxiliary function approach (Lee and Seung,2001b).If a function satisfies G (H,H )=ℒ(H );G (H,H ′)≤ℒ(H ),we say G (H,H ′)is an aux-iliary function of L (H ).We defineH (t +1)=arg max HG (H,H (t ))(22)Then by construction,we haveℒ(H (t ))=G (H (t ),H (t ))≤G (H (t +1),H (t ))≤ℒ(H (t +1))(23)This proves that L (H (t ))is monotonically increasing.The key steps in the remainder of the proof are:(1)Find an appropriate auxiliary function;(2)Find the global maxima of the auxiliary function.Now we show that an auxiliary function of ℒ(H )isG (H,H ′)=2(1+α)∑ijkH ′ik W ij H ′jk (1+log H ik H jk H ′ik H ′jk)−∑ik(H ′H ′TH ′)ik H 4ikH ′3ik(24)−∑ik (DH ′Λ)ik H 4ik +H ′4ikH ′3ik.(25)where 1st term uses inequality z ≥1+log z with z =H ik H jk /H ′ik H ′jk ,the 2nd term uses the inequality Eq.(7),and 3rd term usesTr (ΛH T DH )≤∑ik(DH ′Λ)ik H 2ik H ′ik(26)and2H 2ik≤H 4ik +H ′4ik H ′2ikThis inequality is just 2ab <a 2+b 2and Eq.(23)is from[7].Now,we solve the optimization of Eq.(21)by setting ∂G (H,H ′)/∂H =0gives(1+α)H ′ik (W H ′)ikH ik=(H ′H ′T H ′)ik H 3ik H ′3ik +(DH ′Λ)ik H 3ik H ′3ik(27)To show that this is a maxima,we compute the 2nd order derivatives,∂2G (H,H ′)∂H ik ∂H jl=−A ik δij δklwhere A ik=(1+α)H ′ik (W H ′)ikH 2ik+3(H ′H ′T H ′)ik H 2ik H ′2ik +3(DH ′Λ)ik H 2ik H ′2ik(28)Therefore,∂2G (H,H ′)∂H ik ∂H jl is negative definite.This implies function F (H )=G (H,H ′)is a concave function and there is a unique global maxima.The maxima is obtained by solving Eq.(27)which is equivalent toH 4ik=H ′4ik(1+α)(W H ′)ik(H ′H ′T H ′)ik +(DH ′Λ)ik(29)Noting H (t +1)←H and H (t )←H ′,the above recovers the algorithm Eq.(5).This proves that under the updating algorithm Eq.(5)which is Eq.(29),ℒ(H )monotonically increasing.QED.V.The importance of orthogonalityOrthogonality in NMF plays an important role[5].A rigorous orthogonality among columns of H provides a hard clustering that each data object belongs to only one cluster(due to orthogonality and nonnegativity,each row of H has only one nonzero element).Without orthogo-nality,however,each row of H could have more nonzero elements and thus NMF provides a soft clustering.Here we note another important role of orthogonality. In the spectral clustering formulation of Eq.(2),without orthogonality constraint H T DH=I,the optimal solution H∗will contains identical columns.This can be seen asfollows.The objective can be written asTr H T(D−W)H=K∑k=1ℎT k(D−W)ℎkWithout the orthogonality constraint,different columns become independent of each other.and thus reach the same minimum with the same solution,i.e.,ℎ∗1=⋅⋅⋅=ℎ∗k.Notice that in SNMF formulation of Eq.(1),orthogonal-ity is not necessary:without orthogonality constraint,the optimal solution H∗will have different columns.The main reason for this desirable feature[5]is due to the matrix approximation nature of Eq.(1),as opposed to the trace minimization of Eq.(2).To summarize,we have show that(A)Orthogonality is not a necessary constraint for SNMF of Eq.(1)and(B)Orthogonality is a necessary constraint for spectral clustering Eq.(2).A.An investigation of orthogonalityIn our approach of integrating SNMF and Spectral Clus-tering,we enforce the orthogonality.Here,we provide an investigation of this issue,since in previous work[2],[8], orthogonality is not incorporated into their formulations.To see the exact difference due to incorporating orthog-onality or not,we adopt our main SNMF-Ncut formulation Eqs.(3,4)but without orthogonality constraint:minH≥0∥W−HH T∥2+2TrαH T(D−W)H.(30)Repeat the derivation of the computational algorithm of Eq.(5),we obtain the following iterative algorithmH ik←H ik [(1+α)(W H)ik(HH T H+αDH)ik]1/4(31)The correctness and convergence of this algorithm are established in the same fashion as the algorithm Eq.(5). Details are skipped here.We provide extensive experiments in later sections.A general picture is the following:whenαis small (α≃0.01),the solution and performance of NonOrthog-onal formulation of Eq.(3)is similar to the Orthogonal formulation of Eqs.(3,4)(but generally the accuracy is not as good);Whenαis medium or large(α≃1),the solution to NonOrthogonal formulation gives poor accuracy;in this case,different columns of obtained optimal H∗are very similar as explained above.Details are given in later sections.VI.Data Sets DescriptionWe demonstrate the clustering ability of the integrated SNMF and Ncut on three real-life datasets,including facial expression,hand-written digits,and hand-written letters. Human face images.In the AT&T database1,there are 400human face images for40human subjects and ten different images of each subject were taken at different times,varying the lighting,facial expression,and facial details.The original112×92images are resized to32×32 and form1024=32×32dimensional vectors.The weight matrix W is constructed asW ij=exp(−∥x i−x j∥2/r2),(32) where the parameter r isfixed tor=0.7×(average distances between all pairs).Hand-written English Alphabet letters.Hand-written capital English letters“A”-“Z”are clustered into26 clusters.Each letter is a20×16binary image.The input data are26×20=520images.They are from the BinAlha dataset which can be downloaded from “/roweis/data.html”.The weight matrix W is constructed as Eq.(32).Hand-written Digits.Hand-written digits of“0”through “9”are clustered into10clusters.Each digit is a20×16 binary image.The input data are10×39=390images. They are from the same BinAlha dataset above for the hand-written capital English letters.The weight matrix W is constructed as Eq.(32).VII.Experiment Results DemonstrationTo illustrate the clustering result,we show a typical clustering solution in Figure1for the alphabet data.Here we should all26clusters.For this clustering solution,the accuracy as evaluated against the ground truth is60.0%.For the digits data,we show a typical clustering solution in Figure 2.Here we should all10clusters.For this clustering solution,the accuracy as evaluated against the ground truth is72.3%.1/research/dtg/attarchive/facedatabase.htmlFor the human face data,we show a typical clustering solution in Figure3.Here we should10clusters out of the40clusters.For this clustering solution,the accuracy as evaluated against the ground truth is65.8%.VIII.Experiment Results Comparison In Tables1-3,we list the clustering accuracy as evalu-ated according to the ground true of the original images. It should benoted that because of the large variations of real data,many objects from different class mix,as seen from Figures1-3.For algorithm comparison,we also run the symmetric NMF algorithm(settingα=0.01in the Algorithm Eq.(5)) and the pure Ncut algorithm(settingα=10in the Algorithm Eq.(5)).For each clustering method,we run50runs starting from randomly initialized H.We average the obtained accuracy and list them in Tables1-3.We also list the maximum accuracy and minimum accuracy from these runs.From the tables,one can see the integrated approach consistantly perform better than SNMF and Ncut,although not very significantly.It is amazing that the algorithm is very stable with respect to the parameterα.methods average maximum minimumNew75.681.568.8Ncut74.179.268.2SNMF70.076.365.8Table I.Clustering accuracy(in percentage)for face data.methods average maximum minimumNew53.856.747.5Ncut52.155.546.3SNMF41.145.837.5Table II.Clustering accuracy(in percentage)for alphabet data.methods average maximum minimumNew62.074.453.9Ncut60.874.152.9SNMF54.360.549.5Table III.Clustering accuracy(in percentage)for digits data.A.Importance of orthogonalityHere we perform the same experiments using the non-orthogonal SNMF-Ncut formulation of Eqs.(30,31).Theresults on the three data sets are listed in Tables4-6.For the AT&T Face data,the non-orthogonal SNMF-Ncut performs reasonable at smallα=0.01:the clusteraccuracy isη=61.17vs70.0for orthogonal SNMF-Ncut(see Tables1,4).Asαincrease gradually toα=1,the non-orthogonal SNMF-Ncut performance gradually decreases;Atα=1,clustering accuracy is rather low:η=3.03.Thisis because the Laplacian term becomes dominant here andin the solution H∗,different columns become very similar—they are all close to the trivial solution of the Laplacian: H∗≈(e,⋅⋅⋅,e),because e is the lowest eigenvector solution for the Ncut.Thus the cluster assignment at thiscase is rather random,resulting low accuracy.This same trend also occur in Alphabet data(Table5)and Digits data(Table6).Asαincreases fromα=0.01toα=1,the average clustering accuracy of the non-orthogonal SNMF-Ncut decreases fromη=39.62to η=3.93for the Alphabet data(see Table5);For the Digits data,the performance(average clustering accuracy) decreases fromη=53.89toη=10.69(see Table6).In conclusion,the mutual orthogonality of columnsof H are necessary for the integration of NMF andLaplacian-based clustering.Without the orthogonality,theperformance of the integrated SNMF-Ncut deteriorate sig-nificantly at even moderateα=1.αaverage maximum minimum0.0161.1766.7555.00.0254.1961.7549.50.0537.1142.7530.50.126.8232.0020.50.217.0122.2512.750.57.5110.50 5.01.0 3.038.252.5Table IV.Non-orthogonal SNMF-Ncut Cluster-ing accuracy for AT&T Face data at different α.αaverage maximum minimum0.0139.6245.7733.850.0238.1843.6532.310.0531.8236.3528.080.121.3518.4627.50.212.3513.857.690.5 4.258.08 3.851.0 3.93 5.38 3.85Table V.Non-orthogonal SNMF-Ncut Cluster-ing accuracy for Alphabet data at differentα.αaverage maximum minimum0.0153.8959.4948.210.0254.3663.0847.690.0553.9462.3147.440.149.6957.4441.540.232.9144.3628.720.511.4827.1810.001.010.6918.4610.00Table VI.Non-orthogonal SNMF-Ncut Cluster-ing accuracy for Digits data at differentα.IX.SummaryWe integrate symmetric NMF and normalized cut into a single clustering framework,derive the computational algorithm.and prove rigorously the correctness and con-vergence of the proposed algorithm.We also provide a new matrix inequality which is useful for the analysis of 4-th order matrix polynomials.We perform experiments on three real-life data sets to show the effectiveness of the proposed algorithm.We also demonstrate the importance of the orthogonality among matrix factors. Acknowledgments.This research is supported by NSF CCF-0830780,NSF CCF-0939187,NSF CCF-0917274, NSF DMS-0915228.References[1]J.-P.Brunet,P.Tamayo,T.Golub,and J.Mesirov.Metagenes and molecular pattern discovery using matrix factorization.Proc.Nat’l Academy of Sciences USA, 102(12):4164–4169,2004.[2] D.Cai,X.He,X.Wu,and J.Han.Non-negative matrixfactorization on manifold.In ICDM,pages63–72,2008.[3]M.Cooper and J.Foote.Summarizing video using non-negative similarity matrix factorization.In Proc.IEEE Workshop on Multimedia Signal Processing,pages25–28, 2002.[4]I.Dhillon and S.Sra.Generalized nonnegative matrixapproximations with Bregman divergences.In Advances in Neural Information Processing Systems17,Cambridge, MA,2005.MIT Press.[5] C.Ding,X.He,and H.Simon.On the equivalence ofnonnegative matrix factorization and spectral clustering.Proc.SIAM Data Mining Conf,2005.[6] C.Ding,T.Li,and W.Peng.Nonnegative matrix factoriza-tion and probabilistic latent semantic indexing:Equivalence, chi-square statistic,and a hybrid method.Proc.National Conf.Artificial Intelligence,2006.[7] C.Ding,T.Li,W.Peng,and H.Park.Orthogonal nonneg-ative matrix tri-factorizations for clustering.In Proceedings of ACM SIGKDD,pages126–135,2006.[8]Q.Gu and J.Zhou.Co-clustering on manifolds.In KDD,pages359–368,2009.[9] D.D.Lee and H.S.Seung.Algorithms for non-negativematrix factorization.In NIPS,2000.[10]S.Li,X.Hou,H.Zhang,and Q.Cheng.Learning spatiallylocalized,parts-based representation.In Proc.IEEE Conf.Computer Vision and Pattern Recognition,pages207–212, 2001.[11]T.Li, C.Ding,and M.I.Jordan.Solving consensusand semi-supervised clustering problems using nonnegative matrix factorization.In ICDM,pages577–582,2007. [12] B.Long,Z.M.Zhang,X.Wu,and P.S.Yu.Relationalclustering by symmetric convex coding.In ICML,pages 569–576,2007.[13]P.Paatero and U.Tapper.Positive matrix factorization:Anon-negative factor model with optimal utilization of error estimates of data values.Environmetrics,5:111–126,1994.[14]V.P.Pauca,F.Shahnaz,M.Berry,and R.Plemmons.Textmining using non-negative matrix factorization.In Proc.SIAM Int’l conf on Data Mining,pages452–456,2004. [15] F.Sha,L.K.Saul,and D.D.Lee.Multiplicative updatesfor nonnegative quadratic programming in support vector machines.In Advances in Neural Information Processing Systems15.MIT Press,Cambridge,MA,2003.[16]J.Shi and J.Malik.Normalized cuts and image segmen-tation.IEEE.Trans.on Pattern Analysis and Machine Intelligence,22:888–905,2000.[17]N.Srebro,J.Rennie,and T.Jaakkola.Maximum marginmatrix factorization.In Advances in Neural Information Processing Systems,Cambridge,MA,2005.MIT Press. [18] F.Wang,T.Li,and C.Zhang.Semi-supervised clusteringvia matrix factorization.In SDM,pages1–12,2008. [19]Y.-L.Xie,P.Hopke,and P.Paatero.Positive matrixfactorization applied to a curve resolution problem.Journal of Chemometrics,12(6):357–364,1999.clusters are shown as26rows;all member of the same cluster are shown in the same row.Figure4.Face data.Figure5.Clustering results for the Face data using the proposed algorithm.There are total40 discovered clusters.Here we show only10clusters,as10rows;all member of the same clusterare shown in the same row.。
kraus算子分解 -回复
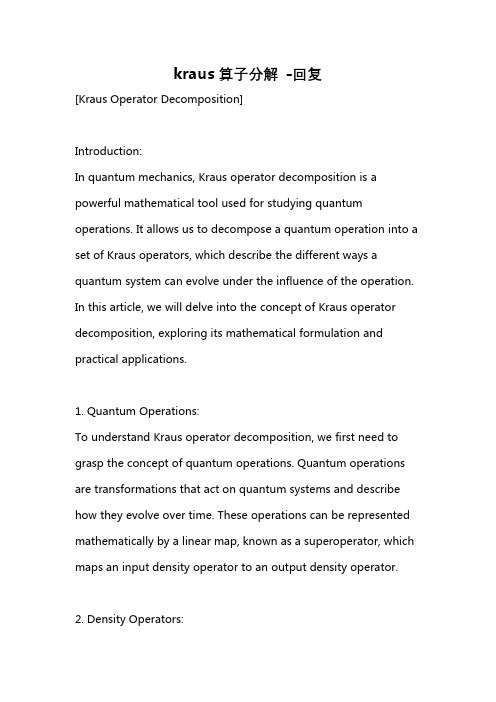
kraus算子分解-回复[Kraus Operator Decomposition]Introduction:In quantum mechanics, Kraus operator decomposition is a powerful mathematical tool used for studying quantum operations. It allows us to decompose a quantum operation into a set of Kraus operators, which describe the different ways a quantum system can evolve under the influence of the operation. In this article, we will delve into the concept of Kraus operator decomposition, exploring its mathematical formulation and practical applications.1. Quantum Operations:To understand Kraus operator decomposition, we first need to grasp the concept of quantum operations. Quantum operations are transformations that act on quantum systems and describe how they evolve over time. These operations can be represented mathematically by a linear map, known as a superoperator, which maps an input density operator to an output density operator.2. Density Operators:In quantum mechanics, a density operator is a mathematical object that represents the state of a quantum system. It is a positive semi-definite Hermitian matrix with trace equal to one. Density operators capture both the pure and mixed states of a quantum system, allowing us to describe both deterministic and probabilistic quantum phenomena.3. Kraus Operators:Now, let's delve into Kraus operators. A Kraus operator is a set of matrices that completely characterize a quantum operation. These matrices act on the input density operator, transforming it into the output density operator. Mathematically, a quantum operation E can be represented as:E(ρ) = ∑iKiρKi†Here, ρ represents the input density operator, Ki are the Kraus operators, and Ki† represents the adjoint of Ki. The sum is taken over all possible values of i, which represents the different ways the quantum system can evolve under the operation.4. Mathematical Formulation:To find the Kraus operators for a given quantum operation, we need to solve the following equation:E(ρ) = ∑iKiρKi†This equation requires that the Kraus operators satisfy the completeness relation:∑iKi†Ki = IHere, I represents the identity operator. The completeness relation ensures that the quantum operation preserves the probability and unitarity of the system.5. Applications:Kraus operator decomposition has several practical applications in quantum information science. It is commonly used in quantum error correction, where it helps to describe and correct quantum errors in quantum computing systems. Additionally, Kraus operators play a crucial role in studying the effects of noise and decoherence in quantum systems, allowing researchers to model and understand the behavior of quantum systems in realisticscenarios.Conclusion:In conclusion, Kraus operator decomposition is a powerful tool in quantum mechanics for understanding quantum operations. By representing a quantum operation as a set of Kraus operators, we can gain valuable insights into the behavior of quantum systems under the influence of the operation. The mathematical formalism of Kraus operators provides a precise framework for studying various quantum phenomena and has numerous applications in the field of quantum information science.。
模式识别与机器学习第一章
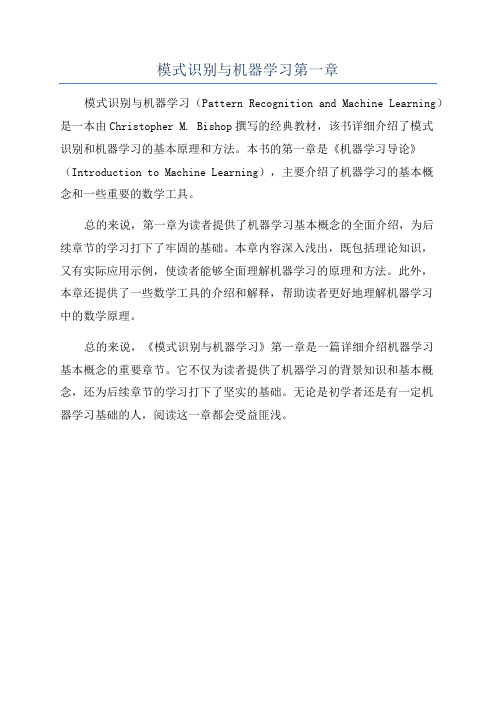
模式识别与机器学习第一章
模式识别与机器学习(Pattern Recognition and Machine Learning)是一本由Christopher M. Bishop撰写的经典教材,该书详细介绍了模式
识别和机器学习的基本原理和方法。
本书的第一章是《机器学习导论》(Introduction to Machine Learning),主要介绍了机器学习的基本概
念和一些重要的数学工具。
总的来说,第一章为读者提供了机器学习基本概念的全面介绍,为后
续章节的学习打下了牢固的基础。
本章内容深入浅出,既包括理论知识,
又有实际应用示例,使读者能够全面理解机器学习的原理和方法。
此外,
本章还提供了一些数学工具的介绍和解释,帮助读者更好地理解机器学习
中的数学原理。
总的来说,《模式识别与机器学习》第一章是一篇详细介绍机器学习
基本概念的重要章节。
它不仅为读者提供了机器学习的背景知识和基本概念,还为后续章节的学习打下了坚实的基础。
无论是初学者还是有一定机
器学习基础的人,阅读这一章都会受益匪浅。
数形结合思想在解题中的应用外文文献翻译

勾股定理(外文翻译从原文第一段开始翻译,翻译了约 2000字)勾股定理是已知最早的古代文明定理之一。
这个著名的定理被命名为希腊的数学家和哲学家毕达哥拉斯。
毕达哥拉斯在意大利南部的科托纳创立了毕达哥拉斯学派。
虽然其中一些可能实际上一直是他学生的工作。
毕达哥拉斯定理是毕达哥拉斯最著名的数学贡 献。
据传说,毕达哥拉斯在得出此定理很高兴,曾宰杀了牛来祭神,以酬谢神灵的启示。
后 来又发现2的平方根是不合理的,因为它不能表示为两个整数比,极大地困扰毕达哥拉斯和他的追随者。
他们在自己的认知中,二是一些单位长度整数倍的长度。
因此 理的,他们就尝试了知识压制。
它甚至说,谁泄露了这个秘密在海上被淹死。
毕达哥拉斯定理是关于包含一个直角三角形的发言。
毕达哥拉斯定理指出,对一个直角三角形斜边为边长的正方形面积,等于剩余两直角为边长正方形面积的总和根据勾股定理,在两个红色正方形的面积之和 A 和B ,等于蓝色的正方形面积,正方形三区他在数学上有许多贡献,2的平方根被认为是不合2Area Square B =bArea Square C = /的数字显示。
在广场的地方就可以表现在两个不同的方式: 1。
由于两个长方形和正方形面积的总和:2。
作为一个正方形的面积之和四个三角形:2 2 /ab\ 2 (a +b) = c 1= c + 2ab因此,毕达哥拉斯定理指出的代数式是:2 2 2 a +b = c对于一个直角三角形的边长 a ,b 和c . 其中c 是斜边长度。
虽然记入史册的是著名的毕达哥拉斯定理, 但是巴比伦人知道某些特定三角形的结果比毕达哥拉斯早一千年。
现在还不知道希腊人最初如何体现了勾股定理的证明。
如果用欧几里德的算法使用,很可能这是一个证明解剖类型类似于以下内容: "一个大广场边a+ b 是分成两个较小的正方形的边a 和b 分别与两个矩形 A 和B ,这两个矩形各 可分为两个相等的直角三角形,有相同的矩形对角线c 。
- 1、下载文档前请自行甄别文档内容的完整性,平台不提供额外的编辑、内容补充、找答案等附加服务。
- 2、"仅部分预览"的文档,不可在线预览部分如存在完整性等问题,可反馈申请退款(可完整预览的文档不适用该条件!)。
- 3、如文档侵犯您的权益,请联系客服反馈,我们会尽快为您处理(人工客服工作时间:9:00-18:30)。
1. Introduction. Consider a maximization problem with a linear objective function cT x: (1.1) maximize cT x subject to x ∈ F,
Байду номын сангаас
where c denotes a constant vector in the n-dimensional Euclidean space Rn and F a subset of Rn . We can reduce a more general maximization problem with a nonlinear objective function f (x) to a maximization problem having a linear objective function represented by a new variable, xn+1 , if we replace f (x) by xn+1 and then add the inequality f (x) ≥ xn+1 to the constraint. Thus (1.1) covers such a general optimization problem. Throughout the paper we assume that F is compact. Then the problem (1.1) has a global maximizer whenever the feasible region F is nonempty. For any compact convex set C containing F , the maximization problem (1.2) maximize cT x subject to x ∈ C
¸ EL‡ MASAKAZU KOJIMA† AND LEVENT TUNC Abstract. Let F be a compact subset of the n-dimensional Euclidean space Rn represented by (finitely or infinitely many) quadratic inequalities. We propose two methods, one based on successive semidefinite programming (SDP) relaxations and the other on successive linear programming (LP) relaxations. Each of our methods generates a sequence of compact convex subsets Ck (k = 1, 2, . . . ) of Rn such that (a) the convex hull of F ⊆ Ck+1 ⊆ Ck (monotonicity), (b) ∩∞ k=1 Ck = the convex hull of F (asymptotic convergence). Our methods are extensions of the corresponding Lov´ asz–Schrijver lift-and-project procedures with the use of SDP or LP relaxation applied to general quadratic optimization problems (QOPs) with infinitely many quadratic inequality constraints. Utilizing descriptions of sets based on cones of matrices and their duals, we establish the exact equivalence of the SDP relaxation and the semiinfinite convex QOP relaxation proposed originally by Fujie and Kojima. Using this equivalence, we investigate some fundamental features of the two methods including (a) and (b) above. Key words. semidefinite programming, nonconvex quadratic optimization problem, linear matrix inequality, bilinear matrix inequality, semi-infinite programming, global optimization AMS subject classifications. 15A48, 52A47, 49M39, 90C05, 90C25, 90C26, 90C30, 90C34 PII. S1052623498336450
serves as a convex relaxation problem, which satisfies the properties that (i) the maximum objective value ζ of the problem (1.2) gives an upper bound for the maximum objective value ζ ∗ of the problem (1.1), i.e., ζ ≥ ζ ∗ , and
∗ Received by the editors March 31, 1998; accepted for publication (in revised form) July 19, 1999; published electronically March 21, 2000. /journals/siopt/10-3/33645.html † Department of Mathematical and Computing Sciences, Tokyo Institute of Technology, 2-12-1 Oh-Okayama, Meguro-ku, Tokyo 152-8552, Japan (kojima@is.titech.ac.jp). ‡ Department of Combinatorics and Optimization, Faculty of Mathematics, University of Waterloo, Waterloo, Ontario N2L 3G1, Canada (ltuncel@math.uwaterloo.ca). This work was completed while this author was visiting Tokyo Institute of Technology, Department of Mathematical and Computing Sciences, on a sabbatical leave from University of Waterloo. The research of this author was supported in part by Tokyo Institute of Technology and by a research grant from NSERC of Canada.
SIAM J. OPTIM. Vol. 10, No. 3, pp. 750–778
c 2000 Society for Industrial and Applied Mathematics
CONES OF MATRICES AND SUCCESSIVE CONVEX RELAXATIONS OF NONCONVEX SETS∗
750
SUCCESSIVE CONVEX RELAXATIONS OF NONCONVEX SETS
751
ˆ ∈ C of (1.2) lies in F , it is a maximizer of (1.1). (ii) if a maximizer x Since the objective function of (1.1) is linear, we know that if we take the convex hull c.hull(F ) (defined as the intersection of all the convex sets containing F ) for C in (1.2), then (i)′ ζ = ζ ∗ , and (ii)′ the set of the maximizers of (1.2) forms a compact convex set whose extreme points are maximizers of (1.1). Therefore, if we solve the relaxation problem (1.2) with a convex feasible region C which closely approximates c.hull(F ), we can expect to get not only a good upper bound ζ for the maximum objective value ζ ∗ but also an approximate maximizer of the problem (1.1). We can further prove that for almost every c ∈ Rn (in the sense of measure), any maximizer x′ ∈ C = c.hull(F ) of (1.2) is an extreme point of c.hull(F ), which also lies in F ; hence x′ is a maximizer of (1.1). This follows from a result due to Ewald, Larman, and Rogers [5] for consequences of related results; see also [17]. ˆ ∈ C , we can Furthermore, for many representations of various convex sets C , given x ˆ. very efficiently find x∗ , an extreme point of C , such that cT x∗ ≥ cT x Indeed, the relaxation technique mentioned above has been playing an essential role in practical computational methods for solving various problems in the fields of combinatorial optimization and global optimization. It is often used in hybrid schemes with the branch-and-bound and branch-and-cut techniques in those fields. See, for instance, [2]. The aim of this paper is to present a basic idea on how we can approximate the convex hull of F . This is a quite difficult problem, and also too general. Before making further discussions, we at least need to provide an appropriate (algebraic) representation for the compact feasible region F of the problem (1.1) and the compact convex feasible region C of the relaxation problem (1.2). We employ quadratic inequalities for this purpose. n Let S n and S+ ⊂ S n denote the set of n × n symmetric matrices and the set of n × n symmetric positive semidefinite matrices, respectively. Given Q ∈ S n , q ∈ Rn , and γ ∈ R, we write a quadratic function on Rn with the quadratic term xT Qx, the linear term 2q T x, and the constant term γ as p(·; γ, q , Q): Then the set Q of quadratic functions on Rn and the set Q+ of convex quadratic functions are defined as Q ≡ {p(·; γ, q , Q) : Q ∈ S n , q ∈ Rn and γ ∈ R} and