Introduction to Modula-2
Slides2

Creating Concurrent Processes
• FORK-JOIN construct:
Ptheads: Creation and termination
pointer to ID
pthread_create ( pthread_t *thread_handle, const pthread_attr_t *attribute, void * (*thread_function) (void * ), void *arg) pthread_join ( pthread_t thread void **ptr)
Latency Hiding
While one thread is waiting for a communication operation, others can utilize the CPU.
Scheduling and Load Balancing
Allow the programmer to specify a large number of concurrent tasks and support dynamic mapping of tasks to processors with minimal idling overheads.
forall Construct:
forall (i = 0; i < n; i++) { S1; S2; . . Sm; }
Example:
forall (i = 0; i < 5; i++) { a[i] = 0;
Cruces for Parallel Programming with Shared Memory multiprocessor system How do processes share information? How do processes synchronize? In shared-memory model, both accomplished through shared variables Creating Concurrent Processes Dealing with the Sharing Data Synchronization Problems
Modula—2和C++的比较
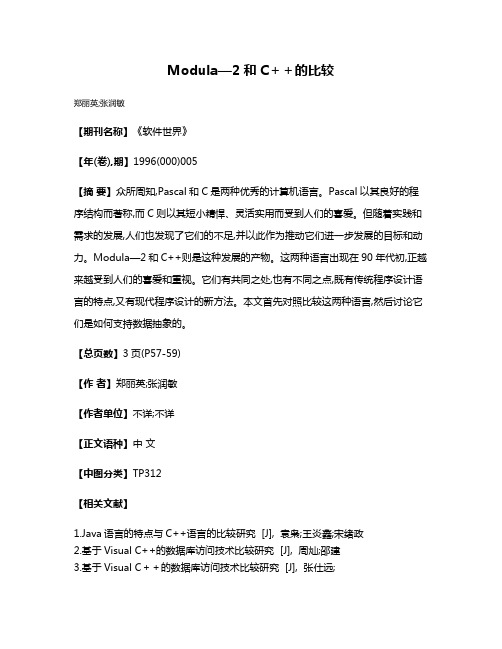
Modula—2和C++的比较
郑丽英;张润敏
【期刊名称】《软件世界》
【年(卷),期】1996(000)005
【摘要】众所周知,Pascal和C是两种优秀的计算机语言。
Pascal以其良好的程序结构而著称,而C则以其短小精悍、灵活实用而受到人们的喜爱。
但随着实践和需求的发展,人们也发现了它们的不足,并以此作为推动它们进一步发展的目标和动力。
Modula—2和C++则是这种发展的产物。
这两种语言出现在90年代初,正越来越受到人们的喜爱和重视。
它们有共同之处,也有不同之点,既有传统程序设计语言的特点,又有现代程序设计的新方法。
本文首先对照比较这两种语言,然后讨论它们是如何支持数据抽象的。
【总页数】3页(P57-59)
【作者】郑丽英;张润敏
【作者单位】不详;不详
【正文语种】中文
【中图分类】TP312
【相关文献】
1.Java语言的特点与C++语言的比较研究 [J], 袁枭;王炎鑫;宋绪政
2.基于Visual C++的数据库访问技术比较研究 [J], 周灿;邵建
3.基于Visual C++的数据库访问技术比较研究 [J], 张仕远;
4.C/C++与JAVA在ACM程序设计竞赛中的应用比较 [J], 赵豪越;靳乔乔;黄建昌
5.Microsoft C/C++和Borland C++编程环境比较 [J], 周晓莉;吴军
因版权原因,仅展示原文概要,查看原文内容请购买。
对Modula-2进行面向对象扩充

对Modula-2进行面向对象扩充
李宣东;郑国梁
【期刊名称】《计算机学报》
【年(卷),期】1996(019)001
【摘要】本文阐述了NDOOM的设计和实现的主要思想.NDOOM是一个Modula-2的面向对象扩充,是Modula-2的超集,其既充分支持面向对象程序设计,又保持了Modula-2的原有风格.
【总页数】6页(P30-35)
【作者】李宣东;郑国梁
【作者单位】南京大学计算机科学与技术系,南京,210093;南京大学计算机科学与技术系,南京,210093
【正文语种】中文
【中图分类】TP3
【相关文献】
1.利用面向对象方法实现有限元软件的扩充 [J], 尹永青
2.一种面向对象语言的扩充设计 [J], 李千目;戚湧;张宏;王宗月;刘凤玉
3.用扩充的面向对象模型表达抽象概念的方法 [J], 陆正康;蔡康明
4.面向对象语言中的持久性扩充 [J], 李清
5.一种系统依赖图的面向对象扩充方案 [J], 李必信;李宣东;郑国梁
因版权原因,仅展示原文概要,查看原文内容请购买。
无线通信 Lecture 8 Modulation II
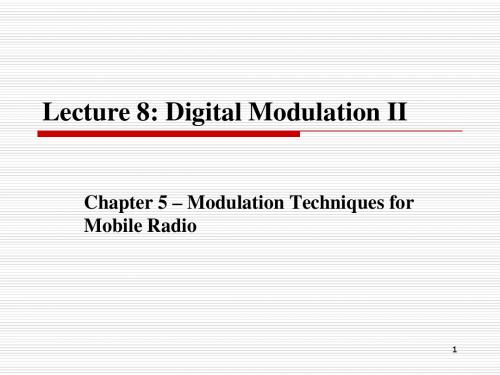
21
MSK RF signal BW MSK has lower sidelobes than QPSK → –23 dB vs. –10 dB MSK has larger null-to-null BW than QPSK → 1.5 Rb vs. 1.0 Rb But 99% RF BW is much better than QPSK (1.2 Rb vs. 8.0 Rb !!) − very low ACI Very popular modulation scheme for mobile radio
Lecture 8: Digital Modulation II
Chapter 5 – Modulation Techniques for Mobile Radio
1
Recall our picture of the overall wireless transmission and receiving system:
3
Constant Envelope Modulation Methods
Constant Envelope as compared to AM
Linear: Amplitude of the signal varies according to the message signal. Constant Envelope: The amplitude of the variation in the message signal. It is the phase that changes.
But this still does not alleviate congestion.
GSM uses a constant envelope modulation scheme (discussed below) that is not bandwidth efficient.
MOD.209 MODULO 2

/ / .IT 40. IN DATA
gg
mm
aaaa
社区 EN 41. SEZIONE 4 - LAVORO AUTONOMO 这里是老板居留要填写的 公司名字
R 42. DENOMINAZIONE SOCIALE
人 IA 个人税号 家 IJ 43. CODICE FISCALE
公司增值税号
意 .Y 44. PARTITA IVA inps注册号
34. CONTRATTO DI SOGGIORNO SOTTOSCRITTO PRESSO LO SPORTELLO UNICO IMMIGRAZIONE (c)
35. DELLA PREFETTURA/UTG DI (b)
36. DATA SOTTOSCRIZIONE
//
(sigla provincia)
W 日期
/ / 50. IN DATA
aaaa
gg
mm
aaaa
51. SEZIONE 5 - DATI REDDITO 收入资料
打钩表示前年的年收入 52. ANNUALE PERCEPITO (c)
年收入 53. IMPORTO
最后一年又收入的年份,除了申请失业居留一般都是写去年
54. ULTIMO ANNO DI PRESENTAZIONE DELLA DICHIARAZIONE DEI REDDITI
62. IMPORTO MENSILE
,
EURO
60和61分别是月工资单和月税单,打工者选60,老板选61,62是月收入
Mod. 209 Modulo 2 - Pagina 2 di 3
MOD. 209 MODULO 2
MINISTERO DELL’INTERNO 1. SCRIVERE IN STAMPATELLO CON PENNA NERA
J2000 TFS - Interval type-2 fuzzy logic systems - theory and design

IEEE TRANSACTIONS ON FUZZY SYSTEMS, VOL. 8, NO. 5, OCTOBER 2000535Interval Type-2 Fuzzy Logic Systems: Theory and DesignQilian Liang and Jerry M. Mendel, Fellow, IEEEAbstract—In this paper, we present the theory and design of interval type-2 fuzzy logic systems (FLSs). We propose an efficient and simplified method to compute the input and antecedent operations for interval type-2 FLSs; one that is based on a general inference formula for them. We introduce the concept of upper and lower membership functions (MFs) and illustrate our efficient inference method for the case of Gaussian primary MFs. We also propose a method for designing an interval type-2 FLS in which we tune its parameters. Finally, we design type-2 FLSs to perform time-series forecasting when a nonstationary time-series is corrupted by additive noise where SNR is uncertain and demonstrate improved performance over type-1 FLSs. Index Terms—Interval type-2 fuzzy sets, nonsingleton fuzzy logic systems, time-series forecasting, tuning of parameters, type-2 fuzzy logic systems, upper and lower membership functions.I. INTRODUCTION FUZZY logic system (FLS) (also known as a fuzzy system, fuzzy logic controller, etc) includes fuzzifier, rules, inference engine, and defuzzifier [22]. Quite often, the knowledge that is used to construct the rules in a FLS is uncertain. Three ways in which such rule uncertainty can occur are: 1) the words that are used in antecedents and consequents of rules can mean different things to different people [23]; 2) consequents obtained by polling a group of experts will often be different for the same rule because the experts will not necessarily be in agreement; and 3) noisy training data. Antecedent or consequent uncertainties translate into uncertain antecedent or consequent membership functions. Type-1 FLSs, whose membership functions are type-1 fuzzy sets, are unable to directly handle rule uncertainties. Type-2 FLSs, the subject of this paper, in which antecedent or consequent membership functions are type-2 fuzzy sets, can handle rule uncertainties. The concept of type-2 fuzzy sets was introduced by Zadeh [41] as an extension of the concept of an ordinary fuzzy set, i.e., a type-1 fuzzy set. Type-2 fuzzy sets have grades of membership that are themselves fuzzy [4]. A type-2 membership —the primary membership; grade can be any subset in and, corresponding to each primary membership, there is a sec) that defines ondary membership (which can also be in the possibilities for the primary membership. A type-1 fuzzy set is a special case of a type-2 fuzzy set; its secondary membership function is a subset with only one element—unity. Type-2AManuscript received August 20, 1999; revised May 30, 2000. The authors are with the Signal and Image Processing Institute, Department of Electrical Engineering Systems, University of Southern California, Los Angeles, CA 90089-2564 USA (e-mail: mendel@). Publisher Item Identifier S 1063-6706(00)08455-1.fuzzy sets allow us to handle linguistic uncertainties, as typified by the adage “words can mean different things to different people.” A fuzzy relation of higher type (e.g., type-2) has been regarded as one way to increase the fuzziness of a relation and, according to Hisdal, “increased fuzziness in a description means increased ability to handle inexact information in a logically correct manner [6].” Mizumoto and Tanaka [25] studied the set theoretic operations of type-2 sets, properties of membership grades of such sets, and examined the operations of algebraic product and algebraic sum for them [26]. More details about algebraic structure of type-2 sets are given in [28]. Dubois and Prade [2]–[4] discussed fuzzy valued logic and give a formula for the composition of type-2 relations as an extension of the type-1 sup-star composition, but this formula is only for minimum -norm. A general formula for the extended sup-star composition of type-2 relations is given by Karnik and Mendel [11], [12], [17]. Based on this formula, Karnik and Mendel [10]–[12], [17] established a complete type-2 FLS theory to handle uncertainties in FLS parameters. Similar to a type-1 FLS, a type-2 FLS includes fuzzifier, rule base, fuzzy inference engine, and output processor. The output processor includes type-reducer and defuzzifier; it generates a type-1 fuzzy set output (from the type-reducer) or a crisp number (from the defuzzifier). A type-2 FLS is again characterized by IF–THEN rules, but its antecedent or consequent sets are now type-2. Type-2 FLSs can be used when the circumstances are too uncertain to determine exact membership grades such as when training data is corrupted by noise—a case studied later in this paper. General type-2 FLSs are computationally intensive because type-reduction is very intensive. Things simplify a lot when secondary membership functions (MFs) are interval sets (in this case, the secondary memberships are either zero or one and we call them interval type-2 sets) and this is the case studied in this paper. When the secondary MFs are interval sets, we call the type-2 FLSs “interval type-2 FLSs.” For some other discussions on the use of interval sets in fuzzy logic (see Hisdal [6], Schwartz [32], and Turksen [34]). The most commonly used fuzzifier is a singleton; but, such a fuzzifier is not adequate when data is corrupted by measurement noise. In this case, a nonsingleton fuzzifier that treats each measurement as a fuzzy number should be used. The theory and applications of a type-1 FLS with nonsingleton fuzzifier are presented in [27], where the input is fuzzified into a type-1 fuzzy set (e.g., Gaussian) whose parameters are based on the measured input and the mean and variance of the measurement noise. This assumes that the statistical knowledge (mean and variance) of1063–6706/00$10.00 © 2000 IEEE536IEEE TRANSACTIONS ON FUZZY SYSTEMS, VOL. 8, NO. 5, OCTOBER 2000the noise is given or can be estimated; but, in many cases, these values are not known ahead of time and can not be estimated from the data. Instead, we only have some linguistic knowledge about the noise, such as very noisy, moderately noisy, or approximately no noise. In this case, we cannot fuzzify the crisp input as a type-1 fuzzy set, because type-1 MFs cannot fully represent the uncertainty associated with this linguistic knowledge. We believe that in this important case, the input should be fuzzified into a type-2 fuzzy set for use in a nonsingleton type-2 FLS. This case is also studied in this paper. In this paper, we also provide a design method for an interval type-2 FLS, where IF–THEN fuzzy rules are obtained from given input–output (I/O) data. Two primary design tasks are structure identification and parameter adjustment [7]. The former determines input/output (I/O) space partition, antecedent and consequent variables, the number of IF–THEN rules (which are determined by the I/O space partition), and the number and initial locations of membership functions. The latter identifies a feasible set of parameters under the given structure. In this paper, we focus on parameter adjustments in an interval type-2 FLS. Tuning the parameters of a type-1 FLS is possible because can be expressed as a closed-form mathematical its output formula. Optimization methods for doing this have been extensively studied (for example, [7], [19], [22], [24], and [36]). Unfortunately, the output of a type-2 FLS cannot be represented by a closed-form mathematical formula; hence, there is an additional level of complexity associated with tuning its parameters. To date, type-2 sets and FLSs have been used in decision making [1], [40], solving fuzzy relation equations [35], survey processing, [12], [13], time-series forecasting [12], [14], function approximation [12], time-varying channel equalization [17], control of mobile robots [39], and preprocessing of data [9]. In the sequel, results for a general type-2 nonsingleton fuzzy logic system (NSFLS) are given in Section II; meet and join operations for interval sets are given in Section III; upper and lower membership functions that characterize a type-2 MF are introduced in Section IV; an efficient and simplified method to compute the input and antecedent operations for interval type-2 FLSs is given in Section V; type-reduction and defuzzification for an interval type-2 FLS are reviewed in Section VI; a method for designing an interval type-2 FLS is given in Section VII; an application of our design method is given in Section VIII for time-series forecasting of a nonstationary time-series that is corrupted by additive noise whose SNR is uncertain; and, finally, the conclusions and topics for future research are given in Section IX. denotes In this paper, denotes a type-1 fuzzy set; the membership grade of in the type-1 fuzzy set ; dedenotes the membership grade notes a type-2 fuzzy set; , of in the type-2 fuzzy set , i.e., ; denotes meet operation; and, denotes join operation. Meet and join are defined and explained in great detail in [10]–[12], [15]. II. TYPE-2 FLSS: GENERAL RESULTS In a type-2 FLS with a rule base of rules in which each rule has antecedents, let the th rule be denoted by , where : IF is , is , , and is , THEN is . Themembership function of a fired rule can be expressed by the following extended sup-star composition [12], [17]: (1) where is a -dimensional Cartesian product space, , is the measurement domain of input ); and is given by,((2) Additionally (3) Substituting (3) and (2) into (1), the latter becomes(4) Let (5) then(6) rules in the FLS fire, where Suppose that any of the ; then, the output fuzzy set, for a type-2 FLS is (7) For later use, we define (8) and (9) so that (6) can be re-expressed as (10) General type-2 FLSs are computationally intensive. Things simplify a lot when secondary MFs are interval sets, in which case secondary memberships are either zero or one and, as we demonstrate below, such simplifications make the use of type-2 FLSs practical. III. MEET AND JOIN FOR INTERVAL SETS The meet and join operations, which are needed in (5)–(10), can be greatly simplified for interval type-1 sets. Theorem 1 (Meet of Interval Sets Under Minimum or Product -Norms): and be two interval a) Let type-1 sets (often called interval sets) with domainsLIANG AND MENDEL: INTERVAL TYPE-2 FUZZY LOGIC SYSTEMS537( ), and ( ), respectively. The meet between and , ( ), under minimum or product -norms (i.e., ) is given by (11) . where b) The meet under minimum or product -norms of in, , having domains terval type-1 sets , , , respectively, where is an interval set with domain , . The proof of Theorem 1a), based on minimum or product operations between two interval sets, is given in [18] and [32]. The extension to part b) (via mathematical induction) is so straightforward, we leave it to the reader. Theorem 2 (Join of Interval Sets): a) Let and be as defined in part (a) of Theorem 1. The join ( ), is given by between and , (12) . where ( ) be as defined in Theorem 1(b). b) Let Then the join of these interval type-1 sets is an interval , . set with domain The proof of Theorem 2(a), based on maximum operation between two interval sets, is given in [18], [12], and [32]. The extension to part (b) (via mathematical induction) is also so straightforward we leave it to the reader. In this paper, we always assume that the operation is the maximum operation. Observe from Theorems 1 and 2, that meet and join operations of interval sets are determined just by the two end-points of each interval set. In a type-2 FLS, the two end-points are associated with two type-1 MFs that we refer to as upper and lower MFs. IV. UPPER AND LOWER MFS FOR TYPE-2 FLSS For convenience in defining the upper and lower MFs of a type-2 MF, we first give the definition of footprint of uncertainty of a type-2 MF. Definition 1 (Footprint of Uncertainty of a Type-2 MF): Uncertainty in the primary membership grades of a type-2 MF consists of a bounded region that we call the footprint of uncertainty of a type-2 MF (e.g., see Fig. 1). It is the union of all primary membership grades. Definition 2 (Upper and Lower MFs): An upper MF and a lower MF are two type-1 MFs that are bounds for the footprint of uncertainty of an interval type-2 MF. The upper MF is a subset that has the maximum membership grade of the footprint of uncertainty; and the lower MF is a subset that has the minimum membership grade of the footprint of uncertainty.Fig. 1. The type-2 MFs for (a) Example 1 and (b) Example 2. The thick solid lines denote upper MFs and the thick dashed lines denote lower MFs. The shaded regions are the footprints of uncertainty for interval secondaries. In (a), the centers of Gaussian MFs vary from 4.5–5.5; in (b), the center of the Gaussian MFs is 5 and the variance varies from 1.0–2.0.We use an overbar (underbar) to denote the upper (lower) MF. are For example, the upper and lower MFs of and , respectively, so that (13)Similarly, we will representandas (14) (15)538IEEE TRANSACTIONS ON FUZZY SYSTEMS, VOL. 8, NO. 5, OCTOBER 2000Example 1: Gaussian Primary MF with Uncertain Mean: Consider the case of a Gaussian primary MF having a and an uncertain mean that takes fixed standard deviation , i.e., on values inExample 2: Gaussian Primary MF with Uncertain Standard Deviation: Consider the case of a Gaussian primary MF having a fixed mean and an uncertain standard deviation that takes , i.e., on values in(16) where ; number of antecedents; ; and, number of rules. is [see Fig. 1(a)] The upper MF where ; number of antecedents; ; number of rules. is [see Fig. 1(b)] The upper MF(19)(20) (17) and the lower MF is [see Fig. 1(b)] (21) where, for example Note that the upper and lower membership functions are simpler for Example 2 than for Example 1. These examples illustrate how to define and so it is clear how to define these membership functions for other situations (e.g., triangular, trapezoidal, bell MFs). V. INTERVAL TYPE-2 FLSS (18) Our major result for interval type-2 FLSs is given in: Theorem 3: In an interval type-2 nonsingleton FLS with type-2 fuzzification and meet under minimum or product -norm: 1) the result of the input and antecedent operations, in (9), is an interval type-1 set, i.e., , whereThe lower MFis [see Fig. 1(a)]From this example, we see that sometimes an upper (or a lower) MF cannot be represented by one mathematical function over its entire domain. It may consist of several branches each defined over a different segment of the entire domain. When the input is located in one domain-segment, we call its corresponding MF branch an active branch, e.g., in Example 1, , the active branch for when is . When an upper (or lower) MF is represented in different segments, its left-hand and right-hand derivatives at the segment for ] may not end point [e.g., be equal, so the upper (or lower) MF may not be differentiable over the entire domain; however, it is piecewise differentiable, i.e., each branch is differentiable over its segment domain. This fact will be used by us when we tune the parameters of a type-2 FLS. Some upper and lower MFs can be represented by one function and are differentiable over their entire domain as we demonstrate in the following example.(22) and(23) the supremum is attained when each term in brackets attains its fired output consequent set in supremum; 2) the rule (10) is (24) and are the lower and upper membership where ; and 3) the output fuzzy set in (7) is grades of (25), as shown at the bottom of the page.(25)LIANG AND MENDEL: INTERVAL TYPE-2 FUZZY LOGIC SYSTEMS539A. Proof of Theorem 3 1) Applying Theorem 1(a) to (5) for an interval type-2 FLS with type-2 fuzzifier and using (14) and (15), we findThe suprema in (31) and (32) are, overall, in . By the monotonicity property of a -norm [42], [27], the supremum is attained when each term in brackets attains its supremum. 2) Based on (9), (31), (32), and Theorem 2(a), we evaluate (10) as(26) . So, the meet between an input type-2 where set and an antecedent type-2 set just involves the -norm operation between the points in two upper or lower MFs. are The upper and lower MFs of (27) (28) The meet operations in (8) are in a -dimensional Cartesian product space so the meet operation is over all , . Based on Theorem 1(b), points , we know that the upper membership grades of (a type-1 MF) are obtained from the -norm of ; hence, from (27), we find membership grades in ( 3) Because straightforward to obtain 2(b). The result is (25).(33) ) are interval sets, it is in (7) using TheoremIn evaluating (22) and (23), the supremum is attained when each term in brackets attains its supremum; so, in the inference of a type-2 FLS, we will examine (34) (35) where re-expressed as , and is a -norm; then, and can be(29) (36) (a type-1 MF), are The lower membership grades ; hence, the -norm of the membership grades in from (28) we find (37) where denotes -norm. We illustrate (36) and (37) below in Section V-C. B. Corollaries to Theorem 3 (30) The join operation in (9) is over all points in . Based on Theorem 2, we know that the right-most point of the ) interval sets is the maximum value join of ( of all the right-most points in the interval sets; so, the right-most point of comes from the maximum value (the right-most point of interval set (supremum) of for each value of ); hence, from (29) we find When the input is fuzzified to a type-1 fuzzy set so that ( ), the upper and lower MFs of merge into one MF in which case Theorem 3 simplifies to the following. Corollary 1: In an interval type-2 FLS with nonsingleton type-1 fuzzification and meet under minimum or product -norm, and in (22) and (23) simplify to(38) (31) Similarly, the left-most point maximum value (supremum) of we find of comes from the ; hence, from (30) and(39) ( ) is the type-1 fuzzified input. where When a singleton fuzzifier is used, the upper and lower MFs merge into one crisp value, namely one, in which of case Theorem 3 simplifies further to the following.(32)540IEEE TRANSACTIONS ON FUZZY SYSTEMS, VOL. 8, NO. 5, OCTOBER 2000Fig. 2. Type-2 FLS: input and antecedent operations. (a) Singleton fuzzification with minimum t-norm; (b) singleton fuzzification with product t-norm; (c) NS type-1 fuzzification with minimum t-norm; (d) NS type-1 fuzzification with product t-norm; (e) NS type-2 fuzzification with minimum t-norm; and (f) NS type-2 fuzzification with product t-norm. The dark shaded regions depict the meet between input and antecedent [computed using Theorem 1(a)].Corollary 2: In an interval type-2 FLS with singleton fuzzification and meet under minimum or product -norm and in (22) and (23) simplify to (40) and (41) ) denotes the location of the singleton. where ( The proofs of these corollaries are so simple, we leave them for the reader.C. Illustrative Examples Example 3—Pictorial Representation of Input and Antecedent Operations: In Fig. 2, we plot the results of input and antecedent operations with singleton, type-1 nonsingleton, and type-2 nonsingleton fuzzifications. The number of antecedents is . In all cases, the firing stength is an interval type-1 , where and . For set, denotes the singleton fuzzification [Fig. 2(a) and (b)], and , namely ; firing strength between input denotes the firing strength between input and , andLIANG AND MENDEL: INTERVAL TYPE-2 FUZZY LOGIC SYSTEMS541xTABLE I FOR EXAMPLE 4xFORTABLE II EXAMPLE 4 BASED ON PRODUCT t-NORMnamely , , as established by Corollary 2. For nonsingleton type-1 fuzzification [Fig. 2(c) and (d)], denotes the supremum of the firing strength between the -norm of membership functions and ; and denotes the supremum of the firing strength between the -norm of and , , as established membership functions by Corollary 1. For nonsingleton type-2 fuzzification [Fig. 2(e) denotes the supremum of the firing strength and (f)], and between the -norm of upper membership functions ; and, denotes the supremum of the firing strength and between the -norm of lower membership functions , , as established by Theorem 3. The main thing to observe from these figures is that regardless of singleton or nonsingleton fuzzification and minimum or product -norm, the result of input and antecedent operations is an interval type-1 set that is determined by its left-most point and right-most point . Example 4—Input is a Gaussian Primary MF with Uncertain Standard Deviation and Antecedents are Gaussian Primary MFs with Uncertain Means: In this example, we compute and when a Gaussian primary MF with an uncertain standard deviation (as in Example 2) is used as input fuzzy sets and Gaussian primary MFs with uncertain means (as in Example 1) are used as antecedent MFs. This case is important to our time-series forecasting application in Section VIII. In this caseby , and lowing form:by. The th antecedent MF has the fol-(43) and its upper and lower MFs and are obtainedby , from (17) and (18), respectively, by replacing by and by . Observe that there are six pa, rameters that determine these two type-2 Gaussian MFs: , , , , and . In this example, as in [27], we assume that (44) and our objective is to evaluate (34) and (35). Equation (44) means that uncertainty in each input set is always no more than the uncertainty in the antecedent. at which the supremum of (34) We denote the value of and the value of at which the supremum of occurs as . The results for and of this (35) occurs as example are carried out in Appendix A, and are summarized in Tables I–III. From these results, it is straightforward to compute and using (34) and (35), i.e., (45) (46)(42) and and its upper and lower MFs from (20) and (21), respectively, by replacing are obtained by , When the input is fuzzified to a type-1 Gaussian MF, then , and we can easily obtain and based on Tables I–III. When a singleton fuzzifier is used, the542IEEE TRANSACTIONS ON FUZZY SYSTEMS, VOL. 8, NO. 5, OCTOBER 2000xFORTABLE III EXAMPLE 4 BASED ON MINIMUM t-NORMresults in Tables I–III simplify even further since . VI. TYPE REDUCTION AND DEFUZZIFICATION . After fuzzification, A type-2 FLS is a mapping fuzzy inference, type-reduction, and defuzzification, we obtain a crisp output. For an interval type-2 FLS, this crisp output is the center of the type-reduced set. Based on Theorem 3 and Corollaries 1 and 2, we know that for an interval type-2 FLS, regardless of singleton or nonsingleton fuzzification and minimum or product -norm, the result of input and antecedent operations (firing strength) is an interval type-1 set, which is determined by and (e.g., see Fig. 2). its left-most and right-most points of rule can be obThe fired output consequent set tained from the fired interval strength using (24) or Corollaries 1 or 2 and (24). Then the fired combined output consequent set can be computed using (25). Type-reduction was proposed by Karnik and Mendel [11], [12], [17]. It is an “extended version” [using the extension principle [41] of type-1 defuzzification methods and is called typereduction because this operation takes us from the type-2 output sets of the FLS to a type-1 set that is called the “type-reduced set.” This set may then be defuzzified to obtain a single crisp number; however, in many applications, the type reduced set may be more important than a single crisp number since it conveys a measure of uncertainties that have flown through the type-2 FLS. There exist many kinds of type-reduction, such as centroid, center-of-sets, height, and modified height, the details of which are given in [11], [12], and [17]. In this paper, for illustrative purposes, we focus on center-of-sets type-reduction, which can be expressed ascentroid of the type-2 interval conse(the centroid of a type-2 quent set fuzzy set is described in [11], [16], and [12]); . Observe, that each set on the right-hand side (RHS) of (47) is an interval type-1 set, hence, is also an interval type-1 set. So, to find , we just need to compute the two end-points of this interval. Unfortunately, no closed-form . formula is available for , can be represented as For any value and(48)and the minimum value of is the maximum value of is . From (48), we see that is a monotonic increasing function with respect to ; so is associated only with and, similarly, is associated only with . In the center of sets (COS)-typereduction method, Karnik and Mendel [12], [17] have shown , , and depend only on a that the two end points of mixture of or values, since . In this case, and can each be represented as a fuzzy basis function (FBF) expansion, i.e.,(49)(47)where [eitherdenotes the firing strength membership grade or ] contributing to the left-most point and is the FBF. Similarlywhere interval set determined by two end points and ; ; (50)LIANG AND MENDEL: INTERVAL TYPE-2 FUZZY LOGIC SYSTEMS543denotes the firing strength membership grade (either ) contributing to the right-most point and is another FBF. Note that whereas a type-1 FLS is characterized by a single FBF expansion [22], [37], an interval type-2 FLS is characterized by two FBF expansions. A general type-2 FLS is characterized by a huge number of FBF expansions [12], [17]; hence, we have demonstrated that by choosing secondary membership functions to be interval sets, the complexity of a general type-2 FLS is vastly reduced. In order to compute and , we need to compute and . This can be done using the exact computational procedure given in [12], [16], and [17]. Here, we briefly provide the computation procedure for . Without loss of generality, assume the s are arranged in . ascending order, i.e., 1) Compute for in (50) by initially setting , where and have been previously . . andwhere orVII. DESIGNING INTERVAL TYPE-2 FLSS BASED ON TUNING , and Given an input–output training pair , we wish to design an interval type-2 FLS with output (53) so that the error function (54) is minimized. Based on the analysis in Section VI, we know that (the only the upper and lower MFs and the two endpoints of . So we want to center of the consequent set) determine tune the upper and lower MFs and the consequent parameters . Since an interval type-2 FLS can be characterized by two FBF expansions that generate the points and , respectively, we can focus on tuning the parameters of just these two type-1 FLSs. input–output training samples , Given ), we wish to update the design parameters ( so that (54) is minimized for training epochs (updating the parameters using all the training samples one time is called “one epoch”). A general method for doing this is as follows. 1) Initialize all the parameters including the parameters in antecedent and consequent MFs and input sets. . 2) Set the counter of training epoch . 3) Set the counter of training data sample input to the type-2 FLS, and compute the 4) Apply total firing degree for each rule, i.e., compute and ( ) using Theorem 3. 5) Compute and , as described in Section VI (which rules; but, they are then leads to a reordering of the renumbered 1, 2, , ). This will establish and , so that and can be expressed ascomputed using (22) and (23) and let ) such that 2) Find ( for 3) Compute in (50) withfor and let . , then go to Step 5). If , then stop and 4) If . set and return to Step 2). 5) Set equal to This four-step computation procedure [Step 1) is an initialization step] has been proven to converge to the exact solution iterations [12]. Observe that in this procein no more than , , and dure, the number is very important. For , ; so can be represented as for (51) The procedure for computing is very similar. Just replace by and, in Step 2), find ( ) such that and, in step 3, let for , and for . Then can be represented as (52)(55)(56) is an interval set, we defuzzify it using the avBecause erage of and ; hence, the defuzzified output of an interval type-2 FLS is , which is the defuzzified 6) Compute output of the type-2 FLS. and on 7) Determine the explicit dependence of and obtained in membership functions (because Step 5) may have changed from one iteration to the next, the dependence of and on MFs may also have changed). To do this, first determine the dependence of and on membership functions, using (34)–(37), is determined by , , , i.e.,(53) , where is the deA perfect FLS should have sired output but, generally, there exist errors between the desired output and actual output. We, therefore, need a design procedure for tuning the parameters of the FLS in order to minimize such errors.。
Introduction to Molecular Dynamics Simulation
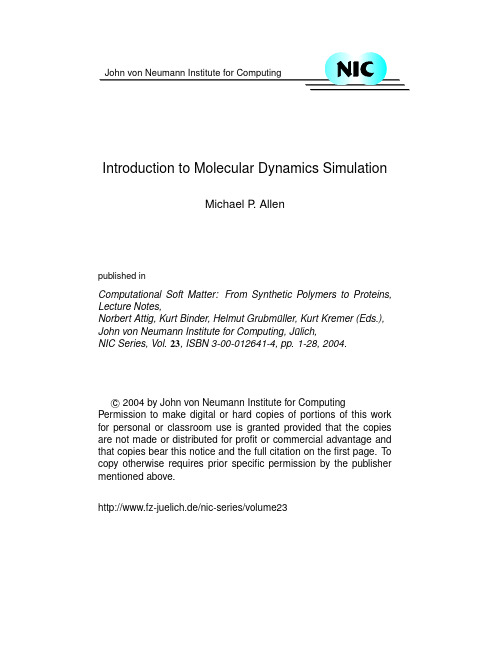
Introduction to Molecular Dynamics Simulation
Michael P. Allen
published in
Computational Soft Matter: From Synthetic Polymers to Proteins, Lecture Notes, Norbert Attig, Kurt Binder, Helmut Grubmuller, Kurt Kremer (Eds.), ¨ John von Neumann Institute for Computing, Julich, ¨ NIC Series, Vol. 23, ISBN 3-00-012641-4, pp. 1-28, 2004.
1
P Intermolecular potential v(r) r c(t) g(r)
Phases s l g T Structure
Experimental Results Complex Fluid (real system)
Make model Test model
Simulation Results
In this chapter a summary is given of the key ingredients necessary to carry out a molecular dynamics simulation, with particular emphasis on macromolecular systems. We discuss the form of the intermolecular potential for molecules composed of atoms, and of non-spherical sub-units, giving examples of how to compute the forces and torques. We also describe some of the MD algorithms in current use. Finally, we briefly refer to the factors that influence the size of systems, and length of runs, that are needed to calculate statistical properties.
咨询方法与工具资料库MOD2【精品文档】

Module 2: QUALITY FRAMEWORKINSTRUCTOR OVERVIEWObjectivesAfter completing this module, participants will be able to:☐Apply the following four components of the Arthur Andersen Quality Framework to an organization:o Driverso Mindseto Processo EnablersAgendaSection Strategy Estimated TimeI. Introduction Lecture5 II. Overview of Framework Discussion 10 III. Drivers Discussion 10 IV. Mindset Discussion 5 V. Process Lecture/Discussion 5 VI. Enablers Lecture 15 VII. Summary Lecture 545BreakMaterials ListHardware☐Overhead projector☐Overhead screen❑FlipchartsSupplies☐Overheads M-1 to M-8❑Flipchart markers☐ Overhead markers❑Masking tapeOther☐AAQF laminated cards☐Quality Framework wallchart☐Strategy PlannerM-1I. Introduction (5 minutes)Present overhead M-1.State: In this second module, we will discuss our Quality Framework. This framework illustrates our approach to implementing quality. Before beginning this module, I would like you to identify key elements of implementing Quality.Instruct participants to record their ideas on page 4 of their Strategy Planner.Allow 3 minutes for participants to write.Ask: What are key elements of implementing Quality?Record responses on a flipchart.State: After I introduce our framework, we will return to this list to check how robust our framework is. We will compare our framework against this list of key elementswhich we, as a class, created.M-2I. Introduction (cont.)Present overhead M-2.State: The purpose of this module is to provide you with an overview of Arthur Andersen's Quality framework and process.Allow participants time to read the objectives.Ask: What questions do you have regarding the objectives?。
- 1、下载文档前请自行甄别文档内容的完整性,平台不提供额外的编辑、内容补充、找答案等附加服务。
- 2、"仅部分预览"的文档,不可在线预览部分如存在完整性等问题,可反馈申请退款(可完整预览的文档不适用该条件!)。
- 3、如文档侵犯您的权益,请联系客服反馈,我们会尽快为您处理(人工客服工作时间:9:00-18:30)。
Practicum I
25
Structured types
Most types are built-in:
CHAR, REAL, BOOLEAN, CARDINAL, INTEGER
Self defined types:
Enumeration Subrange
Until now simple types i.e. one variable = one value at a time
:=
11
27/02/2011
Variables vs. Literals
Variable can have several values (not at one time) Literals are the values themselves:
-3 123 ‘z’ 4.342 TRUE
27/02/2011 Practicum I 12
..
27/02/2011
Not for real valued intervals!!
Practicum I 24
Subrange
MODULE demo; VAR
a, b, c : [2..15];
BEGIN
a := 2; b := 8; c := a * b;
END demo.
27/02/2011
27/02/2011 Practicum I 26
Structured types
Store more than one value per variable
RECORD: possible to combine different types in one variable ARRAY: represent several values of the same type in one variable
((13 DIV 4) = 3) = TRUE)
27/02/2011 Practicum I 18
Characters
Built-in type: CHAR Values: all the entries of the ASCII table Character literals have to be surrounded by single quotes :
Precedence rules
Define which operator is evaluated first.
Multiplication, division, sum, …
13 DIV 4 = 3 = TRUE
Is this correct? What is the value then?
Use brackets to ensure the order of evalution =>
27/02/2011 Practicum I 10
Variables
MODULE demo; VAR a : CHAR; b : INTEGER; c : REAL; d : CARDINAL; e : BOOLEAN: BEGIN a := ‘z’; b := -345; c := 0.35E-3; d := 345; e := FALSE; END demo. Practicum I
Johan@info.vub.ac.be 4K216
27/02/2011
Practicum I
2
Modula 2 : why?
Why not Java, C, C++, C# ?! Learning
Looks like English Very structured Safe (easy?) Has all the modern features
RECORD
day : [1..31]; month : [1..12]; year : [1900..3000];
27/02/2011 Practicum I 14
Conversions
Numbers can not be mixed! CARDINAL ≠ REAL ≠ INTEGER Conversion is needed
TRUNC(real) => CARDINAL FLOAT(cardinal/integer) => REAL
27/02/2011 Practicum I 6
Syntax
Keywords completely in CAPITAL letters Spaces do not matter White lines do not matter Signal the end of a command with semi colon ; End of program with a period .
27/02/2011
Practicum I
27
Record
Delcaration VAR name: RECORD field1 : type1;
. .
fieldN : typeN; END;
27/02/2011 Practicum I 28
Record
Declaration VAR
date :
27/02/2011 Practicum I 5
Program structure
Program is called a MODULE MODULE name;
FROM …. IMPORT …; CONST pi = 3.1459; TYPE euro = REAL;
BEGIN …. (* comment *) END name.
27/02/2011
Practicum I
22
Enumeration
MODULE demo; VAR continent : ( europe, asia, africa, antartica, america); BEGIN continent := antartica; END demo. Danger: values of enumeration can not be used as name for other constants or variable
Practicum I
8
Variables
Need to be declared:
Name Type
:
:=
VAR
X : CARDINAL;
Change the value (assignment)
X := 451;
27/02/2011 Practicum I 9
Variables
Single types:
Modulo using MOD
27/02/2011 Practicum I 13
Working with numbers
MODULE demo; VAR a, b : INTEGER; d : REAL; f : CARDINAL; BEGIN a := -3; b := a * 2; d := 5.0 / 3.0; f := 5 DIV 3; END demo.
Working with numbers
INTEGER, CARDINAL and REAL Operators:
Sum using + Substract using – Multiply using *
Divide using
DIV for CARDINAL and INTEGER / for REAL
27/02/2011 Practicum I 7
Variables
Convenient way to:
Store values Manipulate values
Closely related to the variable concept used in mathematics!
27/02/2011
27/02/2011
Practicum I
16
BOOLEAN algebra
MODULE demo; VAR a, b : INTEGER; c, d : BOOLEAN; BEGIN a := -3; b := 33; c := a > b; d := NOT c; END demo.
27/02/2011 Practicum I 17
27/02/2011 Practicum I 21
Enumeration
First flexible type: defined by you Explicit definition of the possible values of a variable during the declaration VAR x : ( value1, value2, …, valueN); Variable x can only have the values listed between brackets
All programming languages are equal!!
27/02/2011 Practicum I 3
Programming
Way to control a computer
Write down the program (editor) Translate using special program (compiler) Execution by the computer
Characters (‘a’) : CHAR Integers (-40) : INTEGER Reals (2.7) : REAL Pos number (3) : CARDINAL Logical (TRUE/FALSE) : BOOLEAN
Why the name « single types »?
27/02/2011
Practicum I