Bioinformatics-2014-Hajirasouliha-i78-86
西红花提取物调控免疫细胞,提高程序性死亡受体-1抑制剂治疗肺腺癌效果的实验研究
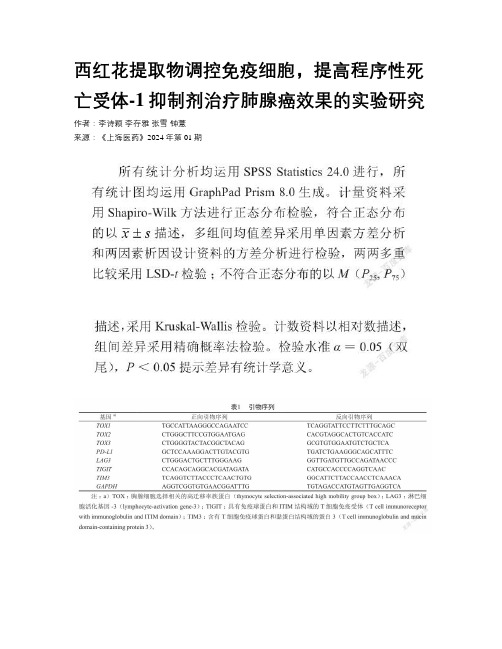
西红花提取物调控免疫细胞,提高程序性死亡受体-1抑制剂治疗肺腺癌效果的实验研究作者:李诗颖李存雅张雪钟薏来源:《上海医药》2024年第01期摘要目的:多项研究提示,西红花提取物能影响肿瘤的发展进程。
本实验探究西红花提取物在肺腺癌小鼠模型中对肿瘤免疫微环境和免疫治疗的影响,为西红花提取物抗肿瘤研究提供更多基础性数据。
方法:构建Lewis肺癌细胞和萤光素酶稳定结合的小鼠皮下瘤模型,观察西红花提取物对小鼠皮下瘤和肿瘤免疫微环境的影响:运用活体成像技术跟踪肿瘤生长情况;运用流式细胞技术检测小鼠CD4+、CD8+ T细胞的数量及占比;运用反转录-聚合酶链式反应技术检测程序性死亡受体配体1、含有T细胞免疫球蛋白和黏蛋白结构域的蛋白3(T cell immunoglobulin and mucin domaincontaining protein 3, TIM3)、淋巴细胞活化基因-3(lymphocyte-activation gene-3, LAG3)、具有免疫球蛋白和ITIM结构域的T细胞免疫受体(T cell immunoreceptor with immunoglobulin and ITIM domain, TIGIT)、胸腺细胞选择相关的高迁移率族蛋白(thymocyte selection-associated high mobility group box, TOX)1、TOX2、TOX3基因的mRNA表达情况。
结果:与对照组相比,给予西红花提取物能一定程度地抑制小鼠皮下瘤的生长(P关键词西红花免疫微环境肺腺癌免疫治疗中图分类号:R965; R282.71 文献标志码:A 文章编号:1006-1533(2024)01-0003-09引用本文李诗颖,李存雅,张雪,等. 西红花提取物调控免疫细胞,提高程序性死亡受体-1抑制剂治疗肺腺癌效果的实验研究[J]. 上海医药, 2024, 45(1): 3-11; 28.基金项目:上海市2022年度“科技创新行动计划”医学创新研究专项项目(22Y31920104);上海市虹口区第二轮“国医强优”三年行动计划(2022—2024年)中西医结合重点专科、薄弱专科建设项目(HKGYQYXM-2022-10);上海市2021年度“科技创新行动计划”扬帆计划项目(21YF444400);上海市2022年度“科技创新行动计划”启明星培育(扬帆专项)项目(22YF1444900);山东省乡村振兴基金会张秀兰慈善基金项目Experimental study of saffron extracts to modulate immune cells to improve the efficacy of a programmed death-1 inhibitor in the treatment of lung adenocarcinomaLI Shiying1, LI Cunya1, ZHANG Xue2, ZHONG Yi1(1. Department of Oncology, Shanghai TCM-Integrated Hospital, Shanghai University of Traditional Chinese Medicine, Shanghai 200082, China; 2. Shanghai Traditional Chinese Medicine Co., Ltd., Shanghai 200082, China)ABSTRACT Objective: A number of studies have shown that saffron extracts can affect the development of tumor. This study explored the effect of saffron extract on tumor immune microenvironment and immunotherapy in a mouse model of lung adenocarcinoma so as to provide more basic data for the anti-tumor research of saffron extracts. Methods: The transplanted tumor model of Lewis lung carcinoma-luciferase in mice was established to detect the effect of saffron extracts on the transplanted tumor in vivo. At the same time, the tumor growth was tracked by in vivo imaging technique. The number and proportion of CD4+ and CD8+ T cells were determined by flow cytometry. The mRNA levels of programmed death-ligand 1, T cell immunoglobulin and mucin domain-containing protein 3 (TIM3), lymphocyte-activation gene-3 (LAG3), T cell immunoreceptor with immunoglobulin and ITIM domain (TIGIT), thymocyte selection-associated high mobility group box (TOX) 1, TOX2 and TOX3 were detected by reverse transcription-polymerase chain reaction (RT-PCR) and immunohistochemical techniques to verify the effect of saffron extracts on the regulation of tumor immune microenvironment. Results:Compared with the control group, the administration of saffron extracts could inhibit the growth of subcutaneous tumor in mice to a certain extent, and the number and proportion of CD4+ and CD8+ T cells were increased (PKEY WORDS saffron; immune microenvironment; lung adenocarcinoma; immunotherapy肿瘤是一类恶性疾病,2018年全球肿瘤死亡病例数达约960万人,较2008年增加26.3%,其中男性肿瘤死亡病例数增加最多的是肺癌,增加了23.4万人[1-2]。
生物信息学英文介绍
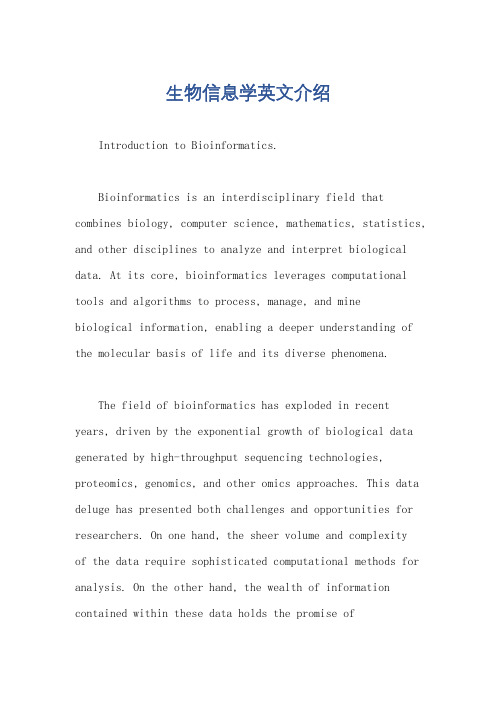
生物信息学英文介绍Introduction to Bioinformatics.Bioinformatics is an interdisciplinary field that combines biology, computer science, mathematics, statistics, and other disciplines to analyze and interpret biological data. At its core, bioinformatics leverages computational tools and algorithms to process, manage, and minebiological information, enabling a deeper understanding of the molecular basis of life and its diverse phenomena.The field of bioinformatics has exploded in recent years, driven by the exponential growth of biological data generated by high-throughput sequencing technologies, proteomics, genomics, and other omics approaches. This data deluge has presented both challenges and opportunities for researchers. On one hand, the sheer volume and complexityof the data require sophisticated computational methods for analysis. On the other hand, the wealth of information contained within these data holds the promise oftransformative insights into the functions, interactions, and evolution of biological systems.The core tasks of bioinformatics encompass genome annotation, sequence alignment and comparison, gene expression analysis, protein structure prediction and function annotation, and the integration of multi-omic data. These tasks require a range of computational tools and algorithms, often developed by bioinformatics experts in collaboration with biologists and other researchers.Genome annotation, for example, involves the identification of genes and other genetic elements within a genome and the prediction of their functions. This process involves the use of bioinformatics algorithms to identify protein-coding genes, non-coding RNAs, and regulatory elements based on sequence patterns and other features. The resulting annotations provide a foundation forunderstanding the genetic basis of traits and diseases.Sequence alignment and comparison are crucial for understanding the evolutionary relationships betweenspecies and for identifying conserved regions within genomes. Bioinformatics algorithms, such as BLAST and multiple sequence alignment tools, are widely used for these purposes. These algorithms enable researchers to compare sequences quickly and accurately, revealing patterns of conservation and divergence that inform our understanding of biological diversity and function.Gene expression analysis is another key area of bioinformatics. It involves the quantification of thelevels of mRNAs, proteins, and other molecules within cells and tissues, and the interpretation of these data to understand the regulation of gene expression and its impact on cellular phenotypes. Bioinformatics tools and algorithms are essential for processing and analyzing the vast amounts of data generated by high-throughput sequencing and other experimental techniques.Protein structure prediction and function annotation are also important areas of bioinformatics. The structure of a protein determines its function, and bioinformatics methods can help predict the three-dimensional structure ofa protein based on its amino acid sequence. These predictions can then be used to infer the protein'sfunction and to understand how it interacts with other molecules within the cell.The integration of multi-omic data is a rapidly emerging area of bioinformatics. It involves theintegration and analysis of data from different omics platforms, such as genomics, transcriptomics, proteomics, and metabolomics. This approach enables researchers to understand the interconnectedness of different biological processes and to identify complex relationships between genes, proteins, and metabolites.In addition to these core tasks, bioinformatics also plays a crucial role in translational research and personalized medicine. It enables the identification of disease-associated genes and the development of targeted therapeutics. By analyzing genetic and other biological data from patients, bioinformatics can help predict disease outcomes and guide treatment decisions.The future of bioinformatics is bright. With the continued development of high-throughput sequencing technologies and other omics approaches, the amount of biological data available for analysis will continue to grow. This will drive the need for more sophisticated computational methods and algorithms to process and interpret these data. At the same time, the integration of bioinformatics with other disciplines, such as artificial intelligence and machine learning, will open up new possibilities for understanding the complex systems that underlie life.In conclusion, bioinformatics is an essential field for understanding the molecular basis of life and its diverse phenomena. It leverages computational tools and algorithms to process, manage, and mine biological information, enabling a deeper understanding of the functions, interactions, and evolution of biological systems. As the amount of biological data continues to grow, the role of bioinformatics in research and medicine will become increasingly important.。
生物学数据库及其检索精选ppt
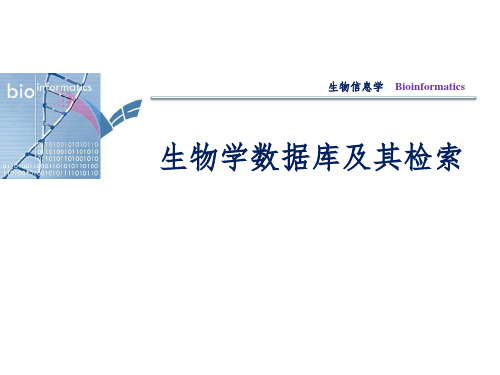
Genbank 由 美国国立生物 技术信息中心 (NCBI)建立维 护,其主页如 图所示。
NCBI 简介
• NCBI全称National Center of Biotechnology Information(美国国家生物技术信息中心)
• NCBI是美国国立卫生研究院(NIH)的美国 国立医学图书馆(NLM)的一个分支。
模式生物
Ureaplasma urealyticum
Bacillus subtilis
Drosophila melanogaster
Rickettsia prowazekii
Helicobacter pylori
Buchnerasp. APS
Escherichia coli
human
Arabidopsis
网
址
结构
分类 蛋白质组 蛋白质组
一次数据库
PDB MMDB
二次数据库 DSSP
HSSP
FSSP
PSdb
结构分类
SCOP
CATH
PDBsum
二次数据库
ProtoMap
氨基酸索引
AAindex
蛋白质间功能关 Predictome 系
蛋白质组分析
Proteome Analysis
二维凝胶电泳
GELBANK SWISS-2DPAGE
生物信息学 Bioinformatics
生物学数据库及其检索
第一节 生物学数据库简介
Chapter 2
一、什么是数据库?
数据库(database) 是一类用于存储和管理数据的
计算机文档,是统一管理的相关数 据的集合,其储存形式有利于数据 信息的检索与调用。
bioxm使用说明
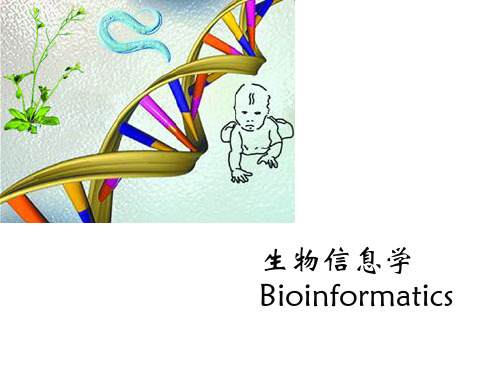
(domain);
第四章 DNA与蛋白质序列分析
第一节 序列比对
第二节 Blast应用
第三节 序列功能分析
Question1:
1. 我刚刚分离一个水稻基因片段序列,大概250bp, 我想初步分析一下它是什么基因,编码什么产物以 及是否已经被别人克隆,应该采用什么工具和数据 库? A. Blastn E. blastx B.Blastp F. nr C.tblastn, D.tblastx,
酶切位点分析(载体构建)
基因结构分析/启动子序列分析
Part 1. 初级序列分析
序列的组成/分子量/等电点分析
/
点击“BioXM version 2.6 ” 点击“运行”进行安装
序列组成分析
序列组成分析
序列组成分析
A/G/C/T的组成,尤其是G+C含量的预测(进化?探针设计?)
/Blast.cgi
具体步骤
1.登陆blast主页
/BLAST/ 2.根据数据类型,选择合适的程序 3.填写表单信息 4.提交任务 5.查看和分析结果
第3节 序列功能分析的内容
序列组成/分子量/等电点---初级分析
Part 3. 基因结构分析/启动子序列分析
Genomic DNA 1)基因结构分析: cDNA
生物信息学资源
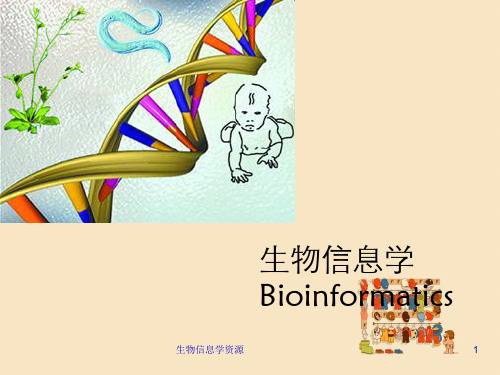
逻辑符的运算次序是从左至右,括号内的检索式可作为一个 单元,优先运行。
布尔逻辑检索允许在检索词后面附加字段标识
例如:rice[ti] AND Bao YM[au] AND 2008:2009[dp]
生物信息学资源
15
生物信息学资源
16
Question1:
如何查找由Zhu J实验室于2005以后发表的, 题目中显示关于 水稻的文献.
名称 生物信息学引论 生物信息学的生物学基础 生物信息学数据库资源 DNA和蛋白质序列分析 系统发生分析 基因表达数据分析 其他常用生物信息学工具 电子克隆的原理和应用 基本生物信息学工具的开发与应用
生物信息学资源
3
第三章 生物信息学数据库资源
--数据库查询
生物信息学资源
4
GenBank
生物信息学资源
生物信息学资源
17
Question 2:
如:我要查找BaoYM在Nature或Science上发表的论文
1 Bao YM[au] AND (Nature[Journal] OR Science[Journal]) 2 Bao YM[au] AND Nature OR Science[Journal] 3 Bao YM[au] AND Nature[Journal] OR Science[Journal] 4 Bao YM[au] AND (Nature OR Science)[Journal] 哪一个检索语言是正确的?
29
生物信息学资源
30
生物信息学资源
31
查找蛋白质序列:
生物信息学资源
32
查找EST序列:
生物信息学资源
33
查找Structure:
Bioinformatics
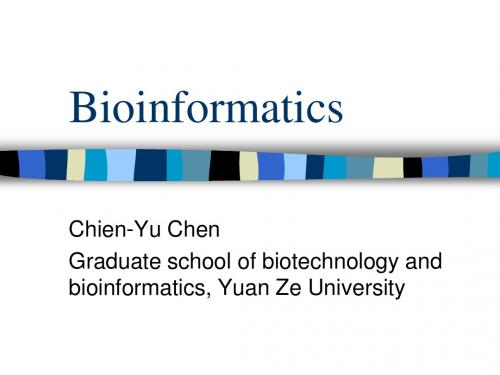
Chien-Yu Chen Graduate school of biotechnology and bioinformatics, Yuan Ze University
Course Information
Web page
– .tw/~cychen/course/922BioInfo/922-BioInfo-CourseInformation.htm
12
Functional Genomics – Research Issues
Which genes are expressed in which tissues? How is the expression of a gene affected by extracellular influences? Which genes are expressed during the development of an organism? What is the effect of misregulated expression of a gene? What patterns of gene expression cause a disease or lead to disease progression? What patterns of gene expression influence response to treatmen Reading Frames
Consider the double-stranded DNA sequence below
5' CAATGGCTAGGTACTATGTATGAGATCATGATCTTTACAAATCCGAG 3' 3' GTTACCGATCCATGATACATACTCTAGTACTAGAAATGTTTAGGCTC 5'
Bioinformatics
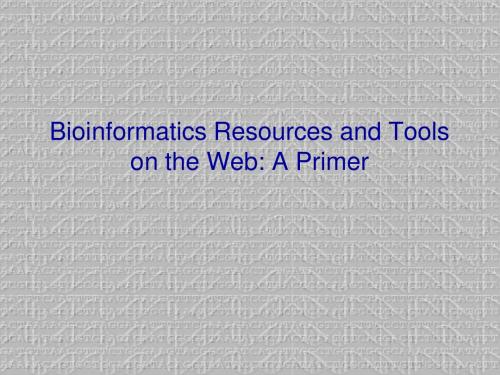
– Tblastn search with BLOSUM45 produces an unexpected exon
• Conclusion: Incomplete (as opposed to incorrect) annotation
A few more resources to be aware of
• Human Genome Working Draft
– /
• TIGR (The Institute for Genomics Research)
– /
What is bioinformatics?
Examples of Bioinformatics
• Database interfaces
– Genbank/EMBL/DDBJ, Medline, SwissProt, PDB, …
• Sequence alignment
– BLAST, FASTA
• Now we have only one hit
– How are they related?
Conclusion: Incorrect gene prediction/annotation
• The two predicted proteins have essentially identical annotation • The protein-protein alignments are disjoint and consecutive on the protein • The protein-nucleotide alignment includes both protein-protein alignments in the proper order • Why/how does this happen?
bioinformatics analysis is a technique
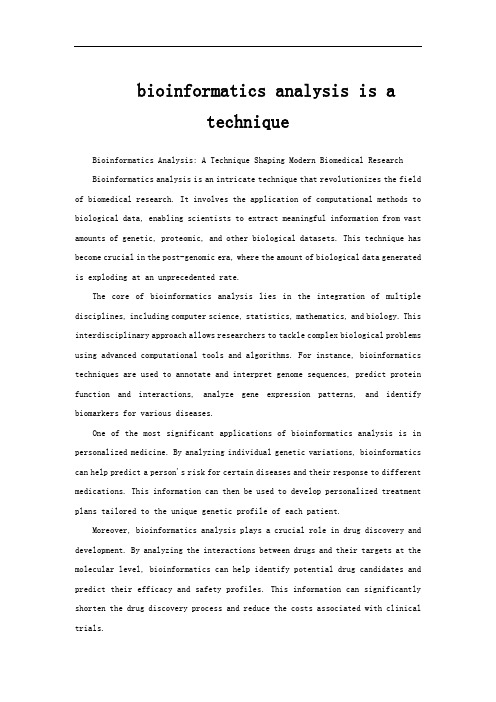
bioinformatics analysis is atechniqueBioinformatics Analysis: A Technique Shaping Modern Biomedical ResearchBioinformatics analysis is an intricate technique that revolutionizes the field of biomedical research. It involves the application of computational methods to biological data, enabling scientists to extract meaningful information from vast amounts of genetic, proteomic, and other biological datasets. This technique has become crucial in the post-genomic era, where the amount of biological data generated is exploding at an unprecedented rate.The core of bioinformatics analysis lies in the integration of multiple disciplines, including computer science, statistics, mathematics, and biology. This interdisciplinary approach allows researchers to tackle complex biological problems using advanced computational tools and algorithms. For instance, bioinformatics techniques are used to annotate and interpret genome sequences, predict protein function and interactions, analyze gene expression patterns, and identify biomarkers for various diseases.One of the most significant applications of bioinformatics analysis is in personalized medicine. By analyzing individual genetic variations, bioinformatics can help predict a person's risk for certain diseases and their response to different medications. This information can then be used to develop personalized treatment plans tailored to the unique genetic profile of each patient.Moreover, bioinformatics analysis plays a crucial role in drug discovery and development. By analyzing the interactions between drugs and their targets at the molecular level, bioinformatics can help identify potential drug candidates and predict their efficacy and safety profiles. This information can significantly shorten the drug discovery process and reduce the costs associated with clinical trials.In addition to its applications in personalized medicine and drug discovery, bioinformatics analysis also has numerous other uses. It can be used to study the evolution of species, the mechanisms of gene regulation, and the interactions between different biological systems. Bioinformatics analysis is also essential in the field of epidemiology, where it helps track the spread of diseases and identify potential outbreaks.In conclusion, bioinformatics analysis is a technique that has revolutionized biomedical research. Its interdisciplinary nature and the use of advanced computational methods have enabled researchers to extract meaningful information from vast amounts of biological data. This information has led to breakthroughs in personalized medicine, drug discovery, and other areas of biomedical research, promising better health outcomes and improved quality of life for millions of people.。
- 1、下载文档前请自行甄别文档内容的完整性,平台不提供额外的编辑、内容补充、找答案等附加服务。
- 2、"仅部分预览"的文档,不可在线预览部分如存在完整性等问题,可反馈申请退款(可完整预览的文档不适用该条件!)。
- 3、如文档侵犯您的权益,请联系客服反馈,我们会尽快为您处理(人工客服工作时间:9:00-18:30)。
Vol.30ISMB2014,pages i78–i86 BIOINFORMATICS doi:10.1093/bioinformatics/btu284 A combinatorial approach for analyzing intra-tumor heterogeneity from high-throughput sequencing dataIman Hajirasouliha1,2,y,Ahmad Mahmoody1,y and Benjamin J.Raphael1,2,*1Department of Computer Science and2Center for Computational Molecular Biology,Brown University,Providence,RI, 02906,USAABSTRACTMotivation:High-throughput sequencing of tumor samples has shown that most tumors exhibit extensive intra-tumor heterogeneity, with multiple subpopulations of tumor cells containing different som-atic mutations.Recent studies have quantified this intra-tumor hetero-geneity by clustering mutations into subpopulations according to the observed counts of DNA sequencing reads containing the variant allele.However,these clustering approaches do not consider that the population frequencies of different tumor subpopulations are correlated by their shared ancestry in the same population of cells. Results:We introduce the binary tree partition(BTP),a novel com-binatorial formulation of the problem of constructing the subpopula-tions of tumor cells from the variant allele frequencies of somatic mutations.We show that finding a BTP is an NP-complete problem; derive an approximation algorithm for an optimization version of the problem;and present a recursive algorithm to find a BTP with errors in the input.We show that the resulting algorithm outperforms existing clustering approaches on simulated and real sequencing data. Availability and implementation:Python and MATLAB implementa-tions of our method are available at / software/Contact:braphael@Supplementary information:Supplementary data are available at Bioinformatics online.1INTRODUCTIONCancer is a disease driven by somatic mutations that accumu-late in the genome during the lifetime of an individual. High-throughput sequencing technologies now provide an un-precedented ability to measure these somatic mutations in tumor samples(Ding et al.,2013).Application of these technol-ogies to cohorts of cancer patients has revealed a number of new cancer-causing mutations and cancer genes(Kandoth et al., 2013;Lawrence et al.,2013;Vogelstein et al.,2013).Cancer sequencing studies have also demonstrated that most tumors ex-hibit extensive intra-tumor heterogeneity characterized by individ-ual cells in the same tumor harboring different complements of somatic mutations(Ding et al.,2012;Gerlinger et al.,2012;Nik-Zainal et al.,2012;Schuh et al.,2012;Shah et al.,2012).Such heterogeneity is a consequence of the fact that cancer is an evo-lutionary process in a population of cells.The clonal theory of cancer evolution(Nowell,1976)posits that the cells of a tumor descended from a single founder cell.This founder cell contained an advantageous mutation leading to a clonal expansion of a large population of cells descended from the founder. Subsequent clonal expansions occur as additional advantageous mutations accumulate in descendent cells.A sequenced tumor sample thus consists of multiple subpopulations of tumor cells from the most recent clonal expansions(Fig.1).Nearly all cancer sequencing efforts thus far sequence DNA from a single sample of a tumor at a single time.This is because of technical limitations:the most cost-effective DNA sequencing technologies(e.g.Illumina)require input DNA from many tumor cells,and such samples are typically available only when patients undergo surgery(Occasionally,paired samples from two time points,such as a primary tumor and a metastasis,are also sequenced.).While sequencing of multiple samples from the same tumor(Gerlinger et al.,2012;Newburger et al.,2013;Salari et al.,2013)or single-cell sequencing(Hou et al.,2012;Navin et al.,2011;Xu et al.,2012)might eventually provide even better datasets to assess intra-tumor heterogeneity,technical consider-ations have limited their applicability utility thus far.Thus,there is tremendous interest in methods that infer the relative propor-tion of different subpopulations of tumor cells in a single sample. Several recent studies(Ding et al.,2012;Nik-Zainal et al., 2012;Shah et al.,2012)have demonstrated that it is possible to infer the subpopulations of tumor cells by counting the number of DNA sequence reads that contain a somatic muta-tion.For single-nucleotide mutations,or variants,the variant allele frequency(VAF)is defined as the fraction of DNA sequence reads covering the variant position that contains the variant allele rather than the reference/germ line allele.The VAF provides an estimate of the fraction of tumor chromosomes con-taining the mutation,but with error due to the stochastic nature of the sequencing process(Fig.1).In addition,technologies cur-rently used in cancer sequencing studies produce short reads that rarely contain more than one somatic mutation.Thus,for any pair of somatic mutations,the only information available to distinguish their subpopulation of origin is the VAF.To overcome substantial variability in measured VAFs,a common approach is to cluster VAFs and from these clusters infer the number and proportion of various subpopulations of tumor cells in the sample.A number of techniques have been introduced to perform this clustering,with Dirichlet Process Mixture models and related non-parametric models being par-ticularly popular,as they do not fix the number of clusters in advance(Miller et al.,forthcoming;Nik-Zainal et al.,2012;Shah et al.,2012).VAF clusters correspond to tumor subpopulations, and the cellular fraction,or fraction of tumor cells containing the cluster of somatic mutation,is derived from the VAF of the clusters.In the simplest case,the VAF directly determines the cellular fraction:e.g.a cluster with VAF=0.5corresponding to homozygous mutations in50%of tumor cells,or*To whom correspondence should be addressed.y The authors wish it to be known that in their opinion,the first twoauthors should be regarded as Joint First Authors.ßThe Author2014.Published by Oxford University Press.This is an Open Access article distributed under the terms of the Creative Commons Attribution Non-Commercial License(/licenses/ by-nc/3.0/),which permits non-commercial re-use,distribution,and reproduction in any medium,provided the original work is properly cited.For commercial re-use,please contact journals.permissions@ by guest on September 2, 2015 /Downloaded fromheterozygous mutations in 100%of tumor cells.However,in practice,the inference of cellular fraction is complicated by copy number aberrations and normal admixture (percentage of the tumor sample that is normal cells),and these two factors are themselves correlated (Carter et al.,2012;Oesper et al.,2013;Strino et al.,2013).We will not consider such complicationshere;rather we restrict attention to heterozygous somatic muta-tions outside of copy number aberrations;assumptions that were made in sequencing studies including Ding et al.(2012).With two exceptions (Jiao et al.,2014;Strino et al.,2013),current techniques for clustering VAFs treat each subpopulation independently and do not consider that these frequencies are correlated by the fact that they are partitions of the same cellular population.Thus,these approaches do not explicitly construct an evolutionary history of the accumulated somatic mutations in the cells.Strino et al.(2013)performs a heuristic search over possible trees,and we discuss Jiao et al.(2014)below.Contributions .In this article,we formulate the problem of infer-ring subpopulations of tumor cells from VAF data obtained from a single tumor sample as the combinatorial problem of construct-ing a Binary Tree Partition (BTP).We show that the problem of finding a BTP is NP-complete and present a 23Ào ð1Þ-approxima-tion algorithm for a related max -BTP problem.The approxima-tion algorithm is based on Local Search and we use the well-known packing bound of Hurkens and Schrijver (1989)for the purpose of analysis.Next,we define "-BTP,a generalization of the BTP problem that allows for the possibility that VAFs are observed with errors and some VAFs are not observed.We pre-sent a straightforward recursive algorithm to find an "-BTP and show that this algorithm outperforms existing VAF clustering approaches on simulated and real data.This recursive algorithm is fast in practice and runs in less than a minute,on a single CPU,for each of our simulated or real samples.2TUMOR SUBPOPULATIONS AND THE BTP In this section,we formulate the problem of determining tumor subpopulations from VAF clusters.The clonal theory of cancerevolution proposes that the cancerous cells in a tumor are the re-sult of multiple waves of somatic mutation and clonal expansion.Given the relationship between sequence coverage (1000–10000Âfor targeted studies)and number of tumor cells that are sequenced in a tumor sample (millions),we first assume that any somatic mutation reported in the data must be present in an appreciable fraction of tumor cells.This implies that the observed somatic mutations were present in at least one clonal expansion.Second,as in other recent studies (Jiao et al.,2014;Salari et al.,2013),we assume that somatic mutations follow the infinite sites assumption such that at most one single mutation occurs at a genomic locus (e.g.single position)during the evolu-tion of the tumor.It follows from this assumption that if a mu-tation occurs in a tumor cell subsequent to a mutation ,then the fraction of cells containing mutation must be at least as large as the fraction of cells containing .This condition was also recently noted in Jiao et al.(2014).We assume that at any particular time in the cancer progres-sion at most one cell in the tumor population acquires a new mutation leading to a clonal expansion.We emphasizethatFig.1.(a )The cells in a tumor descend from a single founder cell via multiple waves of clonal expansion.Each circle represents a population,each dot corresponds to a mutation and the shaded sections indicate the cells descended from each founder cell of the clonal expansion.(b )Under mild assumptions,these clonal expansions give rise to a BTP,with nodes representing populations of tumor cells with specific subsets of somatic mutations.(c )The variant allele frequencies (VAFs)of somatic mutations are determined from sequencing data and used to infer tumor subpopulations and/or the BTP.Here the clusters correspond to clonal expansions,and center of each cluster estimates the frequency of the newly formed subpopulation (denoted with colored marker).Note that the size of each cluster depends on the number of mutations that accumulate before its expansioni79A combinatorial approach for analyzing intra-tumor heterogeneityby guest on September 2, 2015/Downloaded fromthis assumption restricts only the number of clonal expansions that begin at a given time and not the number of clonal expan-sions that are ongoing at one time.Under this assumption,each clonal expansion splits the present tumor cell population into exactly two subpopulations:the subpopulation P of cells con-taining the newly acquired somatic mutation and the subpopula-tion P 0of cells without the mutation (and possibly with a different set of new mutations occurring later in time).Thus,the ancestral history of the sequenced tumor cell population is represented by a rooted binary tree with nodes corresponding to populations of cells at each clonal expansion,and edges indicat-ing the ancestral relationships between these populations.Consequently,each node v in the tree has a set M v of somatic mutations that accumulate along the unique edge p v that con-nects v to its parent (As the root r does not have a parent,the set M r represents the somatic mutations that accumulate before the first clonal expansion.Alternatively,we can let p r be a hidden edge that connects the founder cell of the tumor (represented by the root r )to the normal cell from which it is derived.).Each node v also has a frequency a v representing the proportion of sequenced tumor cells with mutations M v (Fig.1).Note that most tumor samples contain admixture by normal cells with no detectable somatic mutations,and thus in general a r 51.A consequence of these assumptions is that every internal node v in the tree satisfies the children sum to parents (CSP)condition:P u child of v a u =a v .We make the following definition:D EFINITION 1..Given a multiset L =f a 1;...;a n g with 05a i 1,aBTP for L is a complete rooted weighted binary tree T =(V ,E )with nodes V =v 1;...;v n f g such that v i has weight a i and everyinternal node satisfies the CSP condition.Figure 1shows a simple example of a complete binary tree in which the CSP conditions are satisfied for every internal node (also see Supplementary Appendix D.6for a more general ex-ample).Recall that a complete rooted binary tree is a binary treewherein there is a unique node of degree 2(the root),and every node in the tree is either a leaf or has exactly two children.Our goal is to construct such a tree from the measured VAF data.We define the following.D EFINITION 2(BTP problem).Given a multiset L =f a 1;...;a n g ,find a BTP for L if one exists.Note that in some cases,at the split defined by a clonalexpansion,cells from P 0may survive to the present,without undergoing additional clonal expansions (e.g.such cells may cease dividing,or senesce).In this case,there are no mutations that exclusively occur in P 0.We discuss this case in Section 5below.3COMPLEXITY OF THE BTP PROBLEM In this section,we outline the proof of the following theorem.T HEOREM 3.The BTP problem is NP-complete.The proof of this theorem relies on the idea of finding a set of conflict-free triangles in the multiset L .This idea is also useful below for deriving an approximation for a related problem of finding a max -BTP,and so we now define the relevant concepts.Suppose L =a 1;...;a n f g is a multiset of n elements.For any distinct i ,jand k such that a i +a j =a k ,we define the orderedpair ðk ;i ;j ÈÉÞas a triangle in the multiset.See SupplementaryAppendix A.1for an example.We call k as the peak of the tri-angle and i ,j as the tails of thetriangle.We say that trianglest =ðk ;i ;j ÈÉÞand t 0=ðk 0;i 0;j 0ÈÉÞare inconflict if k =k 0or i ;j ÈÉ\i 0;j 0Èɼ;.In other words,two tri-angles are in conflict if and only if they either share a common peak or a common tail.A set Z of triangles is conflict-free if no pair of triangles in Z are in conflict.If T is a BTP for L ,for each internal node a k and itschildren a i and a j ,we have a k =a i +aj ,by CSP.Therefore,ðk ;i ;j ÈÉÞis a triangle in L ,and thus,T cor-responds to a set of conflict-free triangles in L :each internal node and its children form a triangle in L ,and no two triangles share a common peak or a common tail.Because the number of nodes in a complete binary tree is always odd,jLj being an odd number is a necessary condition for the existence of a BTP for L .For a multiset L ,with jLj =2q À1,the size of a conflict-free set of triangles is at most q À1.This is because each triangle has exactly two tails,and in a set of conflict-free triangles,all the tails must be distinct elements.We have the following theorem,whose proof is in Supplementary Appendix A.2.T HEOREM 4.Suppose L =a 1;...;a 2q À1ÈÉ.L has a BTP if and only if there exists a set of q À1conflict-free triangles in L .The proof of NP-completeness of the BTP problem (Theorem 3)is by a reduction from the Numerical Matching with TargetSums (NMTS)problem,(Garey and Johnson,1979).An instanceof NMTS is a tripleI =ðX ;Y ;B Þwhere X ;Y ;B &Z +,and X =x 1;...;x m f g ;Y =y 1;...;y m ÈÉand B =b 1;...;b m f g .Thegoal is to find two permutations X and Y on 1;...;m f g such that x X ði Þ+y Y ði Þ=b i for i =1...,m .T HEOREM 5.Let I =ðX ;Y ;B Þbe an instance of NMTS.Then,I has a solution if and only if a particular multiset L I has a BTP (i.e.a set of 2m À1conflict-free triangles).Moreover,each solu-tion of I can be obtained (in polynomial time)from a BTP of L ,and vice versa.P ROOF .For a given instance I =ðX ;Y ;B Þ,let ^x i =4ðm +1Þx i+1;^y i =4ðm +1Þy i +3and ^b i =4ðm +1Þb i +4;1 i m .Moreover,let j =X jk =1^b k ;2 j m .Now we construct aninstance L I of BTP (i.e.a multiset),with 4m À1elements asfollows:L I =^x 1;...;^x m f g [^y 1;...;^y m ÈÉ[f ^b 1;...;^b m g[2;...; m ÈÉ:())First assume that we have two permutations X ; Y on1;...;m f g such that x X ði Þ+y Y ði Þ=b i :By definitionof ^x i ;^y i and^b i we have also ^x X ði Þ+^y Y ði Þ=^b i .Now we construct a set of con-flict-free triangles forL I of size 2m À1:for each i 21;...;m f g ,add the triangle ð^b i ;f ^x X ði Þ;^y Y ði ÞgÞ.In addition,for eachi 21;...;m À1f g ,we add all triangles ð i +1;f ^b i ;^b i +1gÞ.Thus,by Theorem 4we obtain a BTP from X and Y in poly-nomial time.(()Suppose S is a set of 2m À1conflict-freetriangles for L I .We claim that for each ^x i a triangle ð^b ði Þ;f ^x i ;^y ði ÞgÞexists,whereand are two permutations on {1,...,n }.Note that this i80I.Hajirasouliha et al.by guest on September 2, 2015/Downloaded fromcompletes the proof,by taking X= À1and Y=ð À1* Þfor the instance I.Node^x i cannot be the root,as the largest number in L I is m.Therefore,^x i has a sibling s and a parent p.For all i21;...;mf g,we have^x i=1;^y i=3;^b i=4and i=4i,all in mod4(m+1).Thus,if^x i+s=p2L I,we have s=3 mod4ðm+1ÞðÞand p=4mod4ðm+1ÞðÞ.This implies s=^y ðiÞand p=^b ðiÞfor some (i)and (i).Finally,because all the elem-ents of L I are presented uniquely in T, and are two permu-tations on1;...;mf g.Note that we construct X and Y from T in polynomial time,and the proof is complete.h We note that the proof above shows that the BTP problem in NP-complete in the strong sense,i.e.the problem is still NP-complete if the elements of the multiset are polynomially bounded.In addition,the analogous partition problem for non-binary trees is also NP-complete by reduction from the subset sum problem.See Supplementary Appendix A.6.4A23Àoð1ÞAPPROXIMATION FOR MAX-BTPIn the previous section,we showed that for a given multiset L of 2qÀ1elements,each BTP for L corresponds to a collection of qÀ1conflict-free triangles.Because L can have at most qÀ1 conflict-free triangles,we define the max-BTP problem to be the problem of finding the maximum sized set of conflict-free tri-angles.This is a closely related problem to the BTP problem: in the context of VAF data,the maximum sized set of conflict-free triangles denotes partial information about the ancestral re-lationships among mutations.Moreover,for a multiset of m elements if max-BTP has a solution of size"then a BTP with k=mÀ1À2"additional nodes can be found.See Supplementary Appendix A.7.We derive a23Àoð1Þapproximation algorithm for the max-BTP problem for L.The algorithm is based on Local Search.We start with any collection of conflict-free triangles in L as a solu-tion and iteratively add another triangle as follows.For a fixed constant t!1,we iteratively replace any subcollection of s t triangles in the solution with s+1triangles of L such that the new collection still contains only conflict-free triangles.It is easy to see that the above local search terminates in poly-nomial time.Let OPT be the size of the optimal solution. Because we cannot have more than qÀ1conflict-free triangles in a solution,OPT qÀ1.After each iteration,the size of the collection increases by1,and because t is a constant,the search procedure at each iteration is polynomial time.Similar to the technique that was used in Hajirasouliha et al.(2007),we use the packing bound of Hurkens and Schrijver(1989)to prove the following theorem(Proof in Supplementary Appendix A.7).T HEOREM 6.There exists a polynomial time algorithm that gives an approximated solution to the problem of finding max-imum set of conflict-free triangles within a factor of23À forany 40.5THE"-BTP PROBLEMTypically on real data,a BTP will not exist—either because the frequencies a i are determined with some error or the VAF data does not capture the frequency of a subpopulation that does nothave mutations that exclusively occur in that subpopulation(VAFs provide information only about the proportion of cellswith a mutation,and do not provide information about propor-tions of cells that have a specific mutation and lack another mutation.).In this section,we introduce the"-BTP to accountfor these scenarios.Suppose we have the multiset~L=~a1;...;~a mf g of observed frequencies and a corresponding VAFerror vector"=("1,...,"m)for~L,where"i is the maximum pos-sible error in observing~a i for1i m.To account for subpo-pulations without distinguishing mutations,we may need to add auxiliary frequencies to~L that correspond to the missing sub-population frequencies.We make the following definitions.D EFINITION7("-BTP).Given a multiset~L=~a1;...;~a mf g with associated VAF error vector =ð 1;...; mÞ,an"-BTP withk!0auxiliary nodes is a BTP for a multiset L=a1;a2;...a m+kf g such that for all i m:j a iÀ~a i j i.We callthe nodes a m+1,...,a m+k the auxiliary nodes of the"-BTP.D EFINITION8(The"-BTP problem).Given a multiset~L and an associated VAF error vector",find an"-BTP of~L with min-imum number of auxiliary nodes such that two auxiliary nodesare not siblings.The constraint on auxiliary nodes in the definition of"-BTP problem follows from the assumptions in our model of cancer progression:each branching in the cancer progression happensonly when at least one clonal expansion starts.So,the VAF data captures the frequency of the newly formed subpopulation(seeSection2).Thus,at least one of the children of the current sub-population node is not an auxiliary node.It is straightforward to show that for any multiset L of size m,it is always possible to obtain an"-BTP with k=mÀ1auxiliarynodes(proof in Supplementary Appendix A.3).Also,when"i=0for all1i m,a BTP exists for L if and only if the corresponding"-BTP has a solution with k=0auxiliary nodes.To outline our algorithm,we need the following definitions.D EFINITION9("-CSP tree).Given a VAF error vector",an"-CSP tree is a(weighted)binary tree,such that for each internalnode~a i we have~a j+~a k2½~a iÆð i+ j+ kÞ ,where~a j and~a k arethe children of~a i.We say that an"-CSP tree~T for a multiset~L is acceptable if wecan obtain a BTP, ð~TÞ,by replacing each~a i by a value a i wherej a iÀ~a i j i.Note that ð~TÞis an"-BTP for~L.Also,note thatan"-CSP tree is not necessarily acceptable(See Supplementary Appendix D.8).However,one can easily check whether a given"-CSP tree~T is acceptable by finding a collection of e i’s,wherej e i j i,satisfying the following constraints:ð~a i+e iÞ=ð~a j+e jÞ+ð~a k+e kÞ;for each internal nodes~a i and its children~a j;~a k.Thiscan be easily done via a linear program,which we denote byLPð~TÞ.Our Rec-BTP algorithm(Algorithm1)uses a recursivemethod that works as follows:at each recursion during the al-gorithm,we have(i)a partially constructed"-CSP tree^T,(ii)amultiset of remaining frequencies^L and(iii)the number of re-maining auxiliary nodes that we are allowed to use.We check if^T can be extended by attaching two elements of^L,or one elem-ent from^L and an auxiliary node,to one of the leaves in^T(weassign the auxiliary node’s weight accordingly).If^L is empty,iti81A combinatorial approach for analyzing intra-tumor heterogeneityby guest on September 2, 2015/Downloaded frommeans the algorithm has constructed an "-CSP tree.So we output ð^T Þn o if LP ð^T Þhas a feasible solution.Finally,Rec-BTP outputs all the "-BTPs.Iterating over all values of k from 0to m À1,the algorithm will find the smallest k such that there exists an "ter in Section 6,for the purpose of benchmarking our re-sults,in case of multiple "-BTP outputs,we choose only the tree whose list of node frequencies has the minimum root mean square deviation (RMSD)from the original VAFs data (defined below in Section 6).6EXPERIMENTAL RESULTS6.1Simulated dataWe generate simulated mutation data from all complete rootedbinary trees with 3,5,7and 9nodes (Supplementary AppendixD.7).For each tree topology,we generate 1000random BTPs byassigning a weight a i to each node i ,as follows.For the root r ,weset a r =1,assuming that our tumor sample is pure,i.e.is notcontaminated with normal cells.Next,we proceed down the tree:for each parent with weight a i ,we select a pair of real numbers a jand a k for the children uniformly at random such that the CSPcondition (a i =a j +a k )is satisfied.Finally,we generate a set M iof somatic mutations for each node,with j M i j selected uniformlyfrom [50400]independently for each node.We assume all som-atic mutations in the set M i happened independently because theparental cell was created.For each such BTP and set of muta-tions,we generate a VAF data corresponding to each tumorsubpopulation.Ideally,the VAF of a tumor subpopulationfrom node v equals a v .However,because the observed frequen-cies are estimated from alignments of sequencing data,theobserved frequencies will deviate from the true values.Weassume that the observed VAFs for mutations of a subpopula-tion v are normally distributed with mean a v and standard devi-ation .Specifically,for each node v ,let X v be a set of j M v jsamples from N ða v ; Þ.Here,we present the results when=2,with results on =1,4in Supplementary Appendix B.The VAF data for the tumor sample is thus X =[v X v .Note thatwe simulate VAFs directly rather than the number of mappedreads containing a mutation.Because we assume that our se-quence coverage is high (41000Â),it follows that the correspond-ing binomial (or negative binomial)distribution of read counts is well approximated by the normal distribution.For lower cover-ages,the asymptotic normal approximation may not model the data as accurately;nevertheless,the simulations provide a com-parison of the different methods on high-quality data.For each simulated VAF dataset X ,we estimate the number and frequencies of tumor subpopulations using two non-parametric clustering algorithms:(i)Accelerated variational Dirichlet process Gaussian mixture model (AVDPM;Kurihara et al.,2006),as implemented in https:///site/kenichikurihara/academic-software/variational-dirichlet-process-gaussian-mixture-model,which is a general clustering method that we apply directly on VAF data,and (ii)SciClone (Miller et al.,forthcoming),which is a recent algorithm (with available software but no published paper)that estimates tumor composition from VAF data by clustering the data using a mixture of Gaussian model.Parameter settings for each method are given in Supplementary Appendix C.Also,be-cause SciClone runtimes were extremely long,we down sampled the mutation data by a factor of 20.For each of our synthetic dataset,we implanted the fraction of the mutations (together with their corresponding VAF)on a synthetic chromosome with neutral copy number compatible with the SciClone input format (See Supplementary Appendix C).We ran SciClone with default parameters on each dataset and then extracted the means of reported clusters fromSciClone.From the output of each clustering algorithm,we obtain the input for our Rec-BTP algorithm.We compute the sample mean ~a i and standard deviation i for each cluster C i ,and set the VAF error "i for the subpopulation frequency of cluster C i equalto 1:96Ác iffiffiffiffiffiffii p ,where c is a constant set to 3.Note that1:96Á i =ffiffiffiffiffiffiffiffij C i j p is the radius of the empirical 95%confidenceinterval in estimating the true subpopulation frequency.We set L =~a 1;...;~a m f g and =ð 1;...; m Þas the input to Rec-BTP.We find the minimum k for which there exists a "-BTP for L with k auxiliary nodes.In many cases,there are multiple BTPs for L with exactly k auxiliary nodes.In these cases,weselect a single BTP with the minimum costcost X ðTÞ=X s i =1P x 2D i j x Àa i j 2,where s =n Àk ;X is theVAF dataset and D i =f x 2X j arg min j j a j Àx j =i g is the subsetof elements of X that are closest to a i .We compare the results of our Rec-BTP algorithm (applied to clusters from both AVDPM and SciClone)to the original i82I.Hajirasouliha et al.by guest on September 2, 2015/Downloaded from。