BME studies of stochastic differential equations representing physical laws-part II
中西方饮食文化差异英语图文
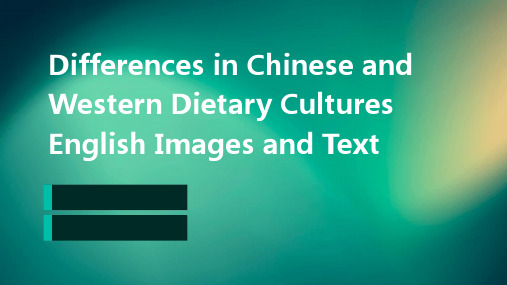
Introduction to Regional Specialty Cuisine
Chinese cuisine has many regional specialties, such as Sichuan cuisine knowledge for its flavor and numbering flags, Cantonese cuisine knowledge for its light and fresh vegetables, and Beijing cuisine knowledge for its rich and healthy dishes
02 Ingredients selection and cooking methods
Main Western ingredients and cooking techniques
Key Western talents include while, before, day products, and variable vegetables and fruits
Economic and technological developments
Changes in economic conditions and technological advancements have affected food production, processing, and consumption patterns, leading to differences in dietary concepts
Characteristics of Chinese dietary concepts
Emphasis on overall balance
主观社会地位与身心健康评述
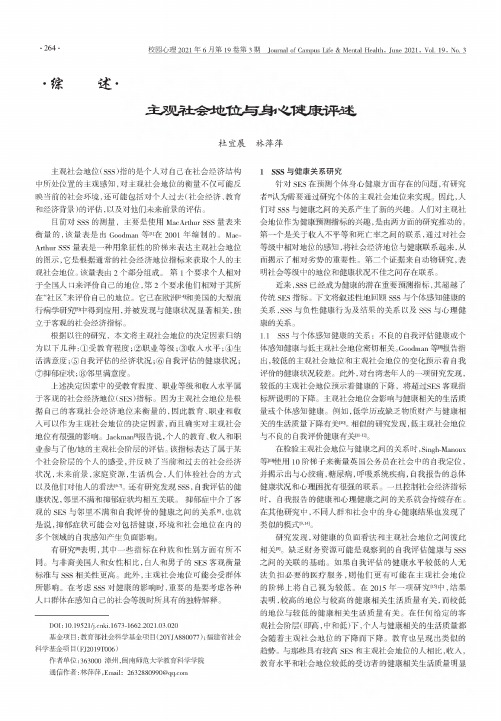
主观社会地位的 致 ,
的
难。而
的
,对社会、
对主观社
会地位的影响知
, 先的
SSS与
身心健康相关的 。此,先的
对少数
,
其
,可能
其
。因此,主观社会地位是否一定与个体的身心健
康有关,能下。
2.2青 年SSS的
主观社会地位和社会经济地位
是青年心理健康问题的重要决因,
对它如何
相互作用的
。
,这2个 与心理健康问
题 存独立的联系,对它 青年心理健康问题中
以促进
长期 -
更 的研究来了解SSS与长期
间
的 在 制,以
地
以促 较 SSS 吸
者的 -
1.4 SSS与心理健康的关系:心理健康困扰是低SSS的潜在
结果-SSS考虑了 SES法解释的社会和心理因-如果考
虑客观数据,社会弱势对健康的 影响可能会
-研
究 合表 ,与其他人相比, 不 的社会地位的认
可能会导致相对 的 , 致
衡量方法所 的心理社会果和社会-例与 经
有 的社会心理因 可能会对他 的主观社会地位产生
影响,
受 , 社会 , 以及对生活的期
望未到等-
主观社会地位可能导致抑郁性思维-在对拉丁裔年轻
人的一项 研究中 冋,与高主观社会地位的情况下相
比,参与者
,在主观社会地位的况下,自心和力
量 显下降-可能反映了主观社会地位与心理健康关系
的
质量
在
的
观社会阶 ( 高,中 ) ,个人
的 质量
会 主观社会地位的
教育也
的
势
些具 高ses 主观社会地位的人比, ,
Bayesian representation of stochastic processes under learning De finetti revisited
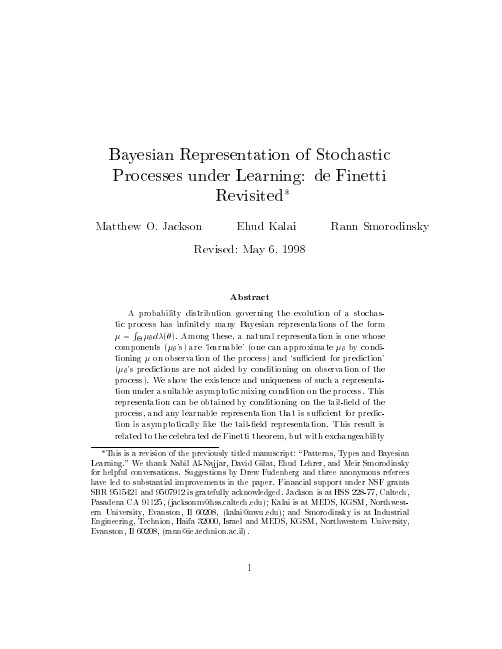
Matthew O. Jackson Ehud Kalai Revised: May 6, 1998
Abstract
Rann Smorodinsky
1
weakened to an asymptotic mixing condition, and with his conclusion of a decomposition into iid component distributions weakened to components that are learnable and su cient for prediction.
R R R
0 0
1 For example, Nyarko 1996 argues that it is important for learning results in incomplete information games to be robust to equivalent reformulations of type spaces. He discusses examples which are not robust to such reformulations. In the language of this paper the reformulations are di erent representations of the process associated with the same game and strategies.
A probability distribution governing the evolution of a stochastic process has in nitely many Bayesian representations of the form R = d . Among these, a natural representation is one whose components 's are `learnable' one can approximate by conditioning on observation of the process and `su cient for prediction' 's predictions are not aided by conditioning on observation of the process. We show the existence and uniqueness of such a representation under a suitable asymptotic mixing condition on the process. This representation can be obtained by conditioning on the tail- eld of the process, and any learnable representation that is su cient for prediction is asymptotically like the tail- eld representation. This result is related to the celebrated de Finetti theorem, but with exchangeability
与年龄相关的具有扩散的随机种群系统数值解的收敛性_英文_
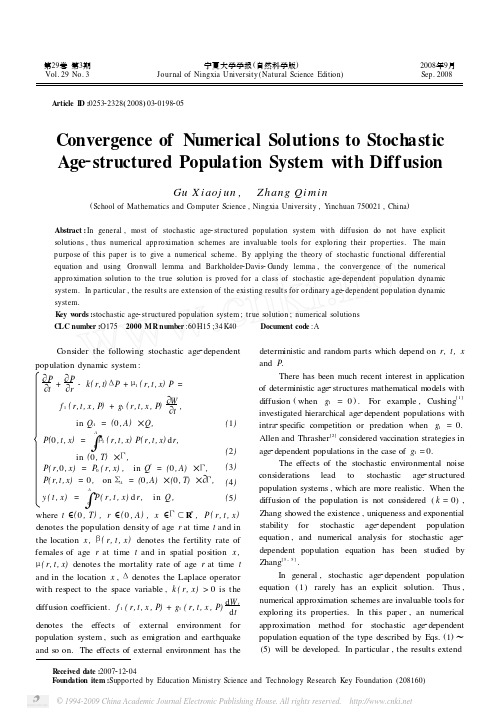
1 Preliminaries
Consider stochastic age2structured population system with diffusion (1) ~ (5) . A is the maximal age of the population species , so P ( r , t , x ) = 0 , Π r ≥A . By (5) , integrating on [ 0 , A ] to Eq. (1) and Eq. (3) with respect to r , then we obtain the following system
a family of nonlinear operators , Ft2measurable almost surely in t. g ( t , x , ・ ) :L 2H → L ( M , H) is the family of nonlinear operator , Ft2measurable almost surely in t. The integral version of Eq. ( 7 ) is given by the
Received date :2007212204 Foundation item :Suppo rted by Education Minist ry Science and Technology Research Key Foundatio n ( 208160)
© 1994-2009 China Academic Journal Electronic Publishing House. All rights reserved.
Δy d t + μ( t , x) y d t - β ( t , x) y d t = dt y - k f ( t , x , y) d t + g ( t , x , y) dW t , Γ, in Q = ( 0 , T) × y ( 0 , x) = y0 ( x) , in Γ,
质性研究方法与量化研究方法之初步比较
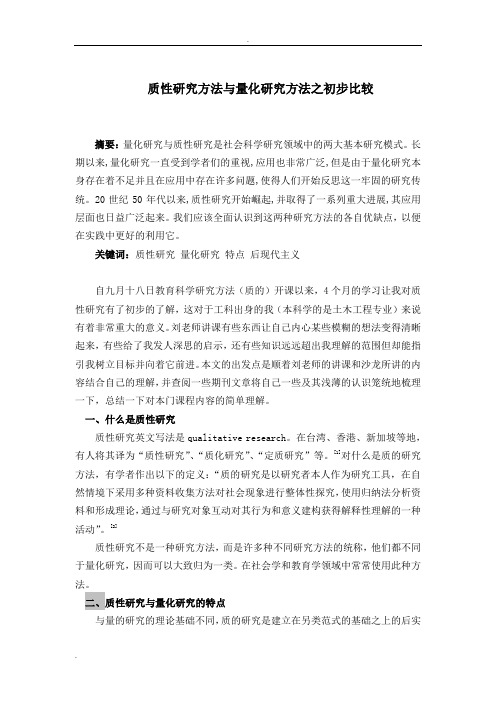
质性研究方法与量化研究方法之初步比较摘要:量化研究与质性研究是社会科学研究领域中的两大基本研究模式。
长期以来,量化研究一直受到学者们的重视,应用也非常广泛,但是由于量化研究本身存在着不足并且在应用中存在许多问题,使得人们开始反思这一牢固的研究传统。
20世纪50年代以来,质性研究开始崛起,并取得了一系列重大进展,其应用层面也日益广泛起来。
我们应该全面认识到这两种研究方法的各自优缺点,以便在实践中更好的利用它。
关键词:质性研究量化研究特点后现代主义自九月十八日教育科学研究方法(质的)开课以来,4个月的学习让我对质性研究有了初步的了解,这对于工科出身的我(本科学的是土木工程专业)来说有着非常重大的意义。
刘老师讲课有些东西让自己内心某些模糊的想法变得清晰起来,有些给了我发人深思的启示,还有些知识远远超出我理解的范围但却能指引我树立目标并向着它前进。
本文的出发点是顺着刘老师的讲课和沙龙所讲的内容结合自己的理解,并查阅一些期刊文章将自己一些及其浅薄的认识笼统地梳理一下,总结一下对本门课程内容的简单理解。
一、什么是质性研究质性研究英文写法是qualitative research。
在台湾、香港、新加坡等地,有人将其译为“质性研究”、“质化研究”、“定质研究”等。
[1]对什么是质的研究方法,有学者作出以下的定义:“质的研究是以研究者本人作为研究工具,在自然情境下采用多种资料收集方法对社会现象进行整体性探究,使用归纳法分析资料和形成理论,通过与研究对象互动对其行为和意义建构获得解释性理解的一种活动”。
[2]质性研究不是一种研究方法,而是许多种不同研究方法的统称,他们都不同于量化研究,因而可以大致归为一类。
在社会学和教育学领域中常常使用此种方法。
二、质性研究与量化研究的特点与量的研究的理论基础不同,质的研究是建立在另类范式的基础之上的后实证主义、批判理论和建构主义。
质的研究方法采取的对世界探究的态度和方式源于自然主义、解释学和后现代主义,它继承了自然主义对自然研究情境的追求,注重对研究结果的“真实性”和可靠性进行探究,它吸纳了解释学对主体间性的重视,它也发扬了后现代理论对边缘性知识尊重的态度。
An algorithmic introduction to numerical simulation of stochastic differential equations

Our intended readership includes
• undergraduate and beginning graduate students in mathematics, statistics, physics, economics, finance, business, computer science, engineering and the life sciences, who, perhaps having been exposed to SDE models, wish to learn more about how to simulate them,
polished. Please feel free to email comments/lists of typos to: djh “at” .
Our intention in this book is to provide a punchy, accessible introduction to the numerical solution of stochastic differential equations (SDEs). With the aim of making this topic available to the widest possible readership, we have kept the prerequisites to a minimum. We assume only a competence in algebra and calculus at the level reached by a typical first year undergraduate mathematics class. Some familiarity with basic concepts from numerical analysis and probability is also desirable, but not absolutely necessary.
随机微分方程(stochastic differential equation,sde)
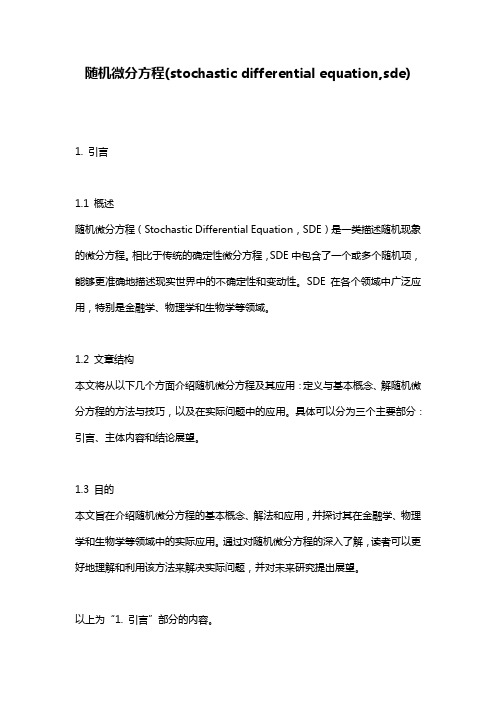
随机微分方程(stochastic differential equation,sde) 1. 引言1.1 概述随机微分方程(Stochastic Differential Equation,SDE)是一类描述随机现象的微分方程。
相比于传统的确定性微分方程,SDE中包含了一个或多个随机项,能够更准确地描述现实世界中的不确定性和变动性。
SDE在各个领域中广泛应用,特别是金融学、物理学和生物学等领域。
1.2 文章结构本文将从以下几个方面介绍随机微分方程及其应用:定义与基本概念、解随机微分方程的方法与技巧,以及在实际问题中的应用。
具体可以分为三个主要部分:引言、主体内容和结论展望。
1.3 目的本文旨在介绍随机微分方程的基本概念、解法和应用,并探讨其在金融学、物理学和生物学等领域中的实际应用。
通过对随机微分方程的深入了解,读者可以更好地理解和利用该方法来解决实际问题,并对未来研究提出展望。
以上为“1. 引言”部分的内容。
2. 随机微分方程的定义与基本概念2.1 随机过程简介随机过程是一类描述随着时间推移而随机变化的数学模型。
它可以看作是时间参数上的一族随机变量的集合。
随机过程常用于描述具有随机性质的现象,如金融市场中的股票价格、天气预报中的温度变化等。
2.2 随机微分方程的定义随机微分方程是一类描述含有随机项(通常为噪声)的微分方程。
它通常采用以下形式表示:dX(t) = a(X(t), t)dt + b(X(t), t)dW(t)其中,X(t)是未知函数,a(X(t), t)和b(X(t), t)是已知函数,dW(t)表示Wiener 过程(也称为布朗运动或白噪声)。
这个方程表示了X在无穷小时间段dt内发生微小变化dX(t),其中包含一个确定性项a(X(t), t)dt和一个随机项b(X(t), t)dW(t)。
2.3 常见的随机微分方程模型在实际应用中,有许多不同类型的随机微分方程模型被广泛使用。
- Ornstein-Uhlenbeck 过程:该模型描述了维持平衡状态的粒子在受到随机扰动时的演化过程。
Some Recent Aspects of Differential Game Theory
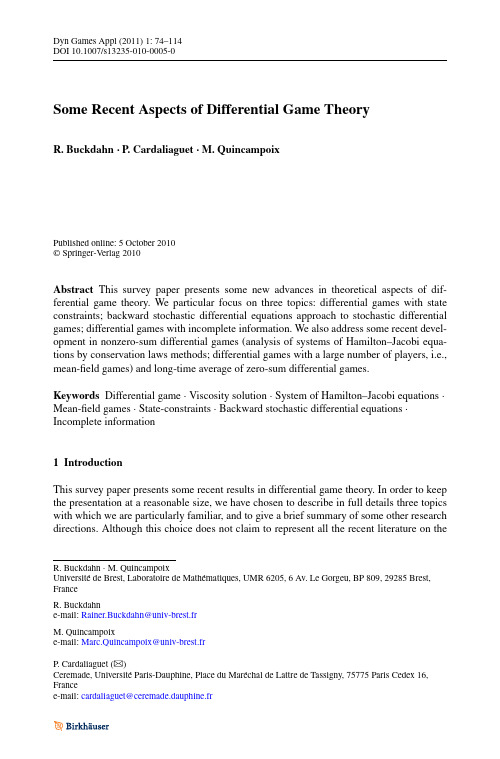
Dyn Games Appl(2011)1:74–114DOI10.1007/s13235-010-0005-0Some Recent Aspects of Differential Game TheoryR.Buckdahn·P.Cardaliaguet·M.QuincampoixPublished online:5October2010©Springer-Verlag2010Abstract This survey paper presents some new advances in theoretical aspects of dif-ferential game theory.We particular focus on three topics:differential games with state constraints;backward stochastic differential equations approach to stochastic differential games;differential games with incomplete information.We also address some recent devel-opment in nonzero-sum differential games(analysis of systems of Hamilton–Jacobi equa-tions by conservation laws methods;differential games with a large number of players,i.e., mean-field games)and long-time average of zero-sum differential games.Keywords Differential game·Viscosity solution·System of Hamilton–Jacobi equations·Mean-field games·State-constraints·Backward stochastic differential equations·Incomplete information1IntroductionThis survey paper presents some recent results in differential game theory.In order to keep the presentation at a reasonable size,we have chosen to describe in full details three topics with which we are particularly familiar,and to give a brief summary of some other research directions.Although this choice does not claim to represent all the recent literature on the R.Buckdahn·M.QuincampoixUniversitéde Brest,Laboratoire de Mathématiques,UMR6205,6Av.Le Gorgeu,BP809,29285Brest, FranceR.Buckdahne-mail:Rainer.Buckdahn@univ-brest.frM.Quincampoixe-mail:Marc.Quincampoix@univ-brest.frP.Cardaliaguet( )Ceremade,UniversitéParis-Dauphine,Place du Maréchal de Lattre de Tassigny,75775Paris Cedex16, Francee-mail:cardaliaguet@ceremade.dauphine.frmore theoretic aspects of differential game theory,we are pretty much confident that it cov-ers a large part of what has recently been written on the subject.It is clear however that the respective part dedicated to each topic is just proportional to our own interest in it,and not to its importance in the literature.The three main topics we have chosen to present in detail are:–Differential games with state constraints,–Backward stochastic differential equation approach to differential games,–Differential games with incomplete information.Before this,we also present more briefly two domains which have been the object of very active research in recent years:–nonzero-sum differential games,–long-time average of differential games.Thefirst section of this survey is dedicated to nonzero-sum differential games.Although zero-sum differential games have attracted a lot of attention in the80–90’s(in particular, thanks to the introduction of viscosity solutions for Hamilton–Jacobi equations),the ad-vances on nonzero-sum differential games have been scarcer,and mainly restricted to linear-quadratic games or stochastic differential games with a nondegenerate diffusion.The main reason for this is that there was very little understanding of the system of Hamilton–Jacobi equations naturally attached to these games.In the recent years the analysis of this sys-tem has been the object of several papers by Bressan and his co-authors.At the same time, nonzero-sum differential games with a very large number of players have been investigated in the terminology of mean-field games by Lasry and Lions.In the second section we briefly sum up some advances in the analysis of the large time behavior of zero-sum differential games.Such problems have been the aim of intense re-search activities in the framework of repeated game theory;it has however only been re-cently investigated for differential games.In the third part of this survey(thefirst one to be the object of a longer development) we investigate the problem of state constraints for differential games,and in particular,for pursuit-evasion games.Even if such class of games has been studied since Isaacs’pioneer-ing work[80],the existence of a value was not known up to recently for these games in a rather general framework.This is mostly due to the lack of regularity of the Hamiltonian and of the value function,which prevents the usual viscosity solution approach to work(Evans and Souganidis[63]):Indeed some controllability conditions on the phase space have to be added in order to prove the existence of the value(Bardi,Koike and Soravia[18]).Following Cardaliaguet,Quincampoix and Saint Pierre[50]and Bettiol,Cardaliaguet and Quincam-poix[26]we explain that,even without controllability conditions,the game has a value and that this value can be characterized as the smallest supersolution of some Hamilton–Jacobi equation with discontinuous Hamiltonian.Next we turn to zero-sum stochastic differential games.Since the pioneering work by Fleming and Souginidis[65]it has been known that such games have a value,at least in a framework of games of the type“nonanticipating strategies against controls”.Unfortunately this notion of strategies is not completely satisfactory,since it presupposes that the players have a full knowledge of their opponent’s control in all states of the world:It would be more natural to assume that the players use strategies which give an answer to the control effectively played by their opponent.On the other hand it seems also natural to consider nonlinear cost functionals and to allow the controls of the players to depend on events of the past which happened before the beginning of the game.The last two points have beeninvestigated in a series of papers by Buckdahn and Li[35,36,39],and an approach more direct than that in[65]has been developed.Thefirst point,together with the two others,will be the object of the fourth part of the survey.In the last part we study differential games with incomplete information.In such games, one of the parameters of the game is chosen at random according to some probability mea-sure and the result is told to one of the players and not to the other.Then the game is played as usual,players observing each other’s control.The main difference with the usual case is that at least one of the players does not know which payoff he is actually optimizing.All the difficulty of this game is to understand what kind of information the informed player has interest in to disclose in order to optimize his payoff,taking thus the risk that his opponent learns his missing information.Such games are the natural extension to differential games of the Aumann–Maschler theory for repeated games[11].Their analysis has been developed in a series of papers by Cardaliaguet[41,43–45]and Cardaliaguet and Rainer[51,52].Throughout these notes we assume the reader to be familiar with the basic results of dif-ferential game theory.Many references can be quoted on this subject:A general introduction for the formal relation between differential games and Hamilton–Jacobi equations(or sys-tem)can be found in the monograph Baçar and Olsder[13].We also refer the reader to the classical monographs by Isaacs[80],Friedman[67]and Krasovskii and Subbotin[83]for early presentations of differential game theory.The recent literature on differential games strongly relies on the notion of viscosity solution:Classical monographs on this subject are Bardi and Capuzzo Dolcetta[17],Barles[19],Fleming and Soner[64],Lions[93]and the survey paper by Crandall,Ishii and Lions[56].In particular[17]contains a good introduc-tion to the viscosity solution aspects of deterministic zero-sum differential games:the proof of the existence and the characterization of a value for a large class of differential games can be found there.Section6is mostly based on the notion of backward stochastic differential equation(BSDE):We refer to El Karoui and Mazliak[60],Ma and Yong[96]and Yong and Zhou[116]for a general presentation.The reader is in particular referred to the work by S.Peng on BSDE methods in stochastic control[101].Let usfinally note that,even if this survey tries to cover a large part of the recent literature on the more theoretical aspects of differential games,we have been obliged to omit some topics:linear-quadratic differential games are not covered by this survey despite their usefulness in applications;however,these games have been already the object of several survey ck of place also prevented us from describing advances in the domain of Dynkin games.2Nonzero-sum Differential GamesIn the recent years,the more striking advances in the analysis of nonzero-sum differential games have been directed in two directions:analysis by P.D.E.methods of Nash feedback equilibria for deterministic differential games;differential games with a very large number of small players(mean-field games).These topics appear as the natural extensions of older results:existence of Nash equilibria in memory strategies and of Nash equilibria in feedback strategies for stochastic differential games,which have also been revisited.2.1Nash Equilibria in Memory StrategiesSince the work of Kononenko[82](see also Kleimenov[81],Tolwinski,Haurie and Leit-mann[114],Gaitsgory and Nitzan[68],Coulomb and Gaitsgory[55]),it has been knownthat deterministic nonzero-sum differential games admit Nash equilibrium payoffs in mem-ory strategies:This result is actually the counterpart of the so-called Folk Theorem in re-peated game theory[100].Recall that a memory(or a nonanticipating)strategy for a player is a strategy where this player takes into account the past controls played by the other play-ers.In contrast a feedback strategy is a strategy which only takes into account the present position of the system.Following[82]Nash equilibrium payoffs in memory strategies are characterized as follows:A payoff is a Nash equilibrium payoff if and only if it is reach-able(i.e.,the players can obtain it by playing some control)and individually rational(the expected payoff for a player lies above its min-max level at any point of the resulting trajec-tory).This result has been recently generalized to stochastic differential games by Buckdahn, Cardaliaguet and Rainer[38](see also Rainer[105])and to games in which players can play random strategies by Souquière[111].2.2Nash Equilibria in Feedback FormAlthough the existence and characterization result of Nash equilibrium payoffs in mem-ory strategies is quite general,it has several major drawbacks.Firstly,there are,in general, infinitely many such Nash equilibria,but there exists—at least up to now—no completely satisfactory way to select one.Secondly,such equilibria are usually based on threatening strategies which are often non credible.Thirdly,the corresponding strategies are,in general, not“time-consistent”and in particular cannot be computed by any kind of“backward in-duction”.For this reason it is desirable tofind more robust notions of Nash equilibria.The best concept at hand is the notion of subgame perfect Nash equilibria.Since the works of Case[54]and Friedman[67],it is known that subgame perfect Nash equilibria are(at least heuristically)given by feedback strategies and that their corresponding payoffs should be the solution of a system of Hamilton–Jacobi equations.Up to now these ideas have been successfully applied to linear-quadratic differential games(Case[54],Starr and Ho[113], ...)and to stochastic differential games with non degenerate viscosity term:In thefirst case,one seeks solutions which are quadratic with respect to the state variable;this leads to the resolution of Riccati equations.In the latter case,the regularizing effect of the non-degenerate diffusion allows us to usefixed point arguments to get either Nash equilibrium payoffs or Nash equilibrium feedbacks.Several approaches have been developed:Borkar and Ghosh[27]consider infinite horizon problems and use the smoothness of the invari-ant measure associated to the S.D.E;Bensoussan and Frehse[21,22]and Mannucci[97] build“regular”Nash equilibrium payoffs satisfying a system of Hamilton–Jacobi equations thanks to elliptic or parabolic P.D.E techniques;Nash equilibrium feedbacks can also be built by backward stochastic differential equations methods like in Hamadène,Lepeltier and Peng[75],Hamadène[74],Lepeltier,Wu and Yu[92].2.3Ill-posedness of the System of HJ EquationsIn a series of articles,Bressan and his co-authors(Bressan and Chen[33,34],Bressan and Priuli[32],Bressan[30,31])have analyzed with the help of P.D.E methods the system of Hamilton–Jacobi equations arising in the construction of feedback Nash equilibria for deter-ministic nonzero-sum games.In state-space dimension1and for thefinite horizon problem, this system takes the form∂V i+H i(x,D V1,...,D V n)=0in R×(0,T),i=1,...,n,coupled with a terminal condition at time T(here n is the number of players and H i is the Hamiltonian of player i,V i(t,x)is the payoff obtained by player i for the initial condition (t,x)).Setting p i=(V i)x and deriving the above system with respect to x one obtains the system of conservation laws:∂t p i+H i(x,p1,...,p n)x=0in R×(0,T).This system turns out to be,in general,ill-posed.Typically,in the case of two players(n= 2),the system is ill-posed if the terminal payoff of the players have an opposite monotonicity. If,on the contrary,these payoffs have the same monotony and are close to some linear payoff (which is a kind of cooperative case),then the above system has a unique solution,and one can build Nash equilibria in feedback form from the solution of the P.D.E[33].Still in space dimension1,the case of infinite horizon seems more promising:The sys-tem of P.D.E then reduces to an ordinary differential equation.The existence of suitable solutions for this equation then leads to Nash equilibria.Such a construction is carried out in Bressan and Priuli[32],Bressan[30,31]through several classes of examples and by various methods.In a similar spirit,the papers Cardaliaguet and Plaskacz[47],Cardaliaguet[42]study a very simple class of nonzero-sum differential games in dimension1and with a terminal payoff:In this case it is possible to select a unique Nash equilibrium payoff in feedback form by just imposing that it is Pareto whenever there is a unique Pareto one.However,this equilibrium payoff turns out to be highly unstable with respect to the terminal data.Some other examples of nonlinear-quadratic differential games are also analyzed in Olsder[99] and in Ramasubramanian[106].2.4Mean-field GamesSince the system of P.D.Es arising in nonzero-sum differential games is,in general,ill-posed,it is natural to investigate situations where the problem simplifies.It turns out that this is the case for differential games with a very large number of identical players.This problem has been recently developed in a series of papers by Lasry and Lions[87–90,94] under the terminology of mean-field games(see also Huang,Caines and Malhame[76–79] for a related approach).The main achievement of Lasry and Lions is the identification of the limit when the number of players tends to infinity.The typical resulting model takes the form⎧⎪⎨⎪⎩(i)−∂t u−Δu+H(x,m,Du)=0in R d×(0,T),(ii)∂t m−Δm−divD p H(x,m,Du)m=0in R d×(0,T),(iii)m(0)=m0,u(x,T)=Gx,m(T).(1)In the above system,thefirst equation has to be understood backward in time while the second one is forward in time.Thefirst equation(a Hamilton–Jacobi one)is associated with an optimal control problem and its solution can be regarded as the value function for a typical small player(in particular the Hamiltonian H=H(x,m,p)is convex with respect to the last variable).As for the second equation,it describes the evolution of the density m(t)of the population.More precisely,let usfirst consider the behavior of a typical player.He controls through his control(αs)the stochastic differential equationdX t=αt dt+√2B t(where(B t)is a standard Brownian motion)and he aims at minimizing the quantityET12LX s,m(s),αsds+GX T,m(T),where L is the Fenchel conjugate of H with respect to the p variable.Note that in this cost the evolving measure m(s)enters as a parameter.The value function of our average player is then given by(1-(i)).His optimal control is—at least heuristically—given in feedback form byα∗(x,t)=−D p H(x,m,Du).Now,if all agents argue in this way,their repartition will move with a velocity which is due,on the one hand,to the diffusion,and,one the other hand,to the drift term−D p H(x,m,Du).This leads to the Kolmogorov equation(1-(ii)).The mean-field game theory developed so far has been focused on two main issues:firstly,investigate equations of the form(1)and give an interpretation(in economics,for instance)of such systems.Secondly,analyze differential games with afinite but large num-ber of players and interpret(1)as their limiting behavior as the number of players goes to infinity.Up to now thefirst issue is well understood and well documented.The original works by Lasry and Lions give a certain number of conditions under which(1)has a solution,discuss its uniqueness and its stability.Several papers also study the numerical approximation of this solution:see Achdou and Capuzzo Dolcetta[1],Achdou,Camilli and Capuzzo Dolcetta[2], Gomes,Mohr and Souza[71],Lachapelle,Salomon and Turinici[85].The mean-field games theory has been used in the analysis of wireless communication systems in Huang,Caines and Malhamé[76],or Yin,Mehta,Meyn and Shanbhag[115].It seems also particularly adapted to modeling problems in economics:see Guéant[72,73],Lachapelle[84],Lasry, Lions,Guéant[91],and the references therein.As for the second part of the program,the limiting behavior of differential games when the number of players tend to infinity has been understood for ergodic differential games[88].The general case remains mostly open.3Long-time Average of Differential GamesAnother way to reduce the complexity of differential games is to look at their long-time be-havior.Among the numerous applications of this topic let us quote homogenization,singular perturbations and dimension reduction of multiscale systems.In order to explain the basic ideas,let us consider a two-player stochastic zero-sum dif-ferential game with dynamics given bydX t,ζ;u,vs =bX t,ζ;u,vs,u s,v sds+σX t,ζ;u,v,u s,v sdB s,s∈[t,+∞),X t=ζ,where B is a d-dimensional standard Brownian motion on a given probability space (Ω,F,P),b:R N×U×V→R N andσ:R N×U×V→R N×d,U and V being some metric compact sets.We assume that thefirst player,playing with u,aims at minimizing a running payoff :R N×U×V→R(while the second players,playing with v,maximizes). Then it is known that,under some Isaacs’assumption,the game has a value V T which is the viscosity solution of a second order Hamilton–Jacobi equation of the form−∂t V T(t,x)+Hx,D V T(t,x),D2V T(t,x)=0in[0,T]×R N,V T(T,x)=0in R N.A natural question is the behavior of V T as T→+∞.Actually,since V T is typically of linear growth,the natural quantity to consider is the long-time average,i.e.,lim T→+∞V T/T.Interesting phenomena can be observed under some compactness assumption on the un-derlying state-space.Let us assume,for instance,that the maps b(·,u,v),σ(·,u,v)and (·,u,v)are periodic in all space variables:this actually means that the game takes place in the torus R N/Z N.In this framework,the long-time average is well understood in two cases:either the dif-fusion is strongly nondegenerate:∃ν>0,(σσ∗)(x,u,v)≥νI N∀x,u,v,(where the inequality is understood in the sense of quadratic matrices);orσ≡0and H= H(x,ξ)is coercive:lim|ξ|→+∞H(x,ξ)=+∞uniformly with respect to x.(2) In both cases the quantity V T(x,0)/T uniformly converges to the unique constant¯c forwhich the problem¯c+Hx,Dχ(x),D2χ(x)=0in R Nhas a continuous,periodic solutionχ.In particular,the limit is independent of the initial condition.Such kind of results has been proved by Lions,Papanicoulaou and Varadhan[95] forfirst order equations(i.e.,deterministic differential games).For second order equations, the result has been obtained by Alvarez and Bardi in[3],where the authors combine funda-mental contributions of Evans[61,62]and of Arisawa and Lions[7](see also Alvarez and Bardi[4,5],Bettiol[24],Ghosh and Rao[70]).For deterministic differential games(i.e.,σ≡0),the coercivity condition(2)is not very natural:Indeed,it means that one of the players is much more powerful than the other one. However,very little is known without such a condition.Existing results rely on a specific structure of the game:see for instance Bardi[16],Cardaliaguet[46].The difficulty comes from the fact that,in these cases,the limit may depend upon the initial condition(see also Arisawa and Lions[7],Quincampoix and Renault[104]for related issues in a control set-ting).The existence of a limit for large time differential games is certainly one of the main challenges in differential games theory.4Existence of a Value for Zero-sum Differential Games with State Constraints Differential games with state constraints have been considered since the early theory of differential games:we refer to[23,28,66,69,80]for the computation of the solution for several examples of pursuit.We present here recent trends for obtaining the existence of a value for a rather general class of differential games with constraints.This question had been unsolved during a rather long period due to problems we discuss now.The main conceptual difficulty for considering such zero-sum games lies in the fact that players have to achieve their own goal and to satisfy the state constraint.Indeed,it is not clear to decide which players has to be penalized if the state constraint is violated.For this reason,we only consider a specific class of decoupled games where each player controls independently a part of the dynamics.A second mathematical difficulty comes from the fact that players have to use admissible controls i.e.,controls ensuring the trajectory to fulfilthe state constraint.A byproduct of this problem is the fact that starting from two close initial points it is not obvious tofind two close constrained trajectories.This also affects the regularity of value functions associated with admissible controls:The value functions are,in general,not Lipschitz continuous anymore and,consequently,classical viscosity solutions methods for Hamilton–Jacobi equations may fail.4.1Statement of the ProblemWe consider a differential game where thefirst player playing with u,controls afirst systemy (t)=gy(t),u(t),u(t)∈U,y(t0)=y0∈K U,(3) while the second player,playing with v,controls a second systemz (t)=hz(t),v(t),v(t)∈V,z(t0)=z0∈K V.(4)For every time t,thefirst player has to ensure the state constraint y(t)∈K U while the second player has to respect the state constraint z(t)∈K V for any t∈[t0,T].We denote by x(t)= x[t0,x0;u(·),v(·)](t)=(y[t0,y0;u(·)](t),z[t0,z0;v(·)](t))the solution of the systems(3) and(4)associated with an initial data(t0,x0):=(t0,y0,z0)and with a couple of controls (u(·),v(·)).In the following lines we summarize all the assumptions concerning with the vectorfields of the dynamics:⎧⎪⎪⎪⎪⎪⎪⎪⎪⎪⎪⎪⎪⎪⎪⎪⎪⎪⎪⎪⎪⎪⎪⎪⎨⎪⎪⎪⎪⎪⎪⎪⎪⎪⎪⎪⎪⎪⎪⎪⎪⎪⎪⎪⎪⎪⎪⎪⎩(i)U and V are compact subsets of somefinitedimensional spaces(ii)f:R n×U×V→R n is continuous andLipschitz continuous(with Lipschitz constant M)with respect to x∈R n(iii)uf(x,u,v)andvf(x,u,v)are convex for any x(iv)K U={y∈R l,φU(y)≤0}withφU∈C2(R l;R),∇φU(y)=0ifφU(y)=0(v)K V={z∈R m,φV(z)≤0}withφV∈C2(R m;R),∇φV(z)=0ifφV(z)=0(vi)∀y∈∂K U,∃u∈U such that ∇φU(y),g(y,u) <0(vii)∀z∈∂K V,∃v∈V such that ∇φV(z),h(z,v) <0(5)We need to introduce the notion of admissible controls:∀y0∈K U,∀z0∈K V and∀t0∈[0,T]we defineU(t0,y0):=u(·):[t0,+∞)→U measurable|y[t0,y0;u(·)](t)∈K U∀t≥t0V(t0,z0):=v(·):[t0,+∞)→V measurable|z[t0,z0;v(·)](t)∈K V∀t≥t0.Under assumptions(5),the Viability Theorem(see[9,10])ensures that for all x0= (y0,z0)∈K U×K VU(t0,y0)=∅and V(t0,z0)=∅.Throughout the paper we omit t0in the notations U(t0,y0)and U(t0,y0)whenever t0=0.We now describe two quantitative differential games.Let us start with a game with an integral cost:Bolza Type Differential Game Given a running cost L:[0,T]×R N×U×V→R and afinal costΨ:R N→R,we define the payoff associated to an initial position(t0,x0)= (t0,y0,z0)and to a pair of controls(u,v)∈U(t0,y0)×V(t0,z0)byJt0,x0;u(·),v(·)=Tt0Lt,x(t),u(·),v(·)dt+Ψx(T),(6)where x(t)=x[t0,x0;u(·),v(·)](t)=(y[t0,y0;u(·)](t),z[t0,z0;v(·)](t))denotes the solu-tion of the systems(3)and(4).Thefirst player wants to maximize the functional J,while the second player’s goal is to minimize J.Definition1A mapα:V(t0,z0)→U(t0,y0)is a nonanticipating strategy(for thefirst player and for the point(t0,x0):=(t0,y0,z0)∈R+×K U×K V)if,for anyτ>0,for all controls v1(·)and v2(·)belonging to V(t0,z0),which coincide a.e.on[t0,t0+τ],α(v1(·)) andα(v2(·))coincide almost everywhere on[t0,t0+τ].Nonanticipating strategiesβfor the second player are symmetrically defined.For any point x0∈K U×K V and∀t0∈[0,T]we denote by A(t0,x0)and by B(t0,x0)the sets of the nonanticipating strategies for thefirst and the second player respectively.We are now ready to define the value functions of the game.The lower value V−is defined by:V−(t0,x0):=infβ∈B(t0,x0)supu(·)∈U(t0,y0)Jt0,x0;u(·),βu(·),(7)where J is defined by(6).On the other hand we define the upper value function as follows:V+(t0,x0):=limε→0+supα∈A(t0,x0)infv(·)∈V(t0,z0)Jεt0,x0;αv(·),v(·)(8)withJεt0,x0;u(·),v(·):=Tt0Lt,x(t),u(t),v(t)dt+Ψεx(T),where x(t)=x[t0,x0;u(·),v(·)](t)andΨεis the lower semicontinuous function defined byΨε(x):=infρ∈R|∃y∈R n with(y,ρ)−x,Ψ(x)=ε.The asymmetry between the definition of the value functions is due to the fact that one assumes that the terminal payoffΨis lower semicontinuous.WhenΨis continuous,one can check that V+can equivalently be defined in a more natural way asV+(t0,x0):=supα∈A(t0,x0)infv(·)∈V(t0,z0)Jt0,x0;αv(·),v(·).We now describe the second differential game which is a pursuit game with closed target C⊂K U×K V.Pursuit Type Differential Game The hitting time of C for a trajectory x(·):=(y(·),z(·)) is:θCx(·):=inft≥0|x(t)∈C.If x(t)/∈C for every t≥0,then we setθC(x(·)):=+∞.In the pursuit game,thefirst player wants to maximizeθC while the second player wants to minimize it.The value functions aredefined as follows:The lower optimal hitting-time function is the mapϑ−C :K U×K V→R+∪{+∞}defined,for any x0:=(y0,z0),byϑ−C (x0):=infβ(·)∈B(x0)supu(·)∈U(y0)θCxx0,u(·),βu(·).The upper optimal hitting-time function is the mapϑ+C :K U×K V→R+∪{+∞}de-fined,for any x0:=(y0,z0),byϑ+ C (x0):=limε→0+supα(·)∈A(x0)infv(·)∈V(z0)θC+εBxx0,αv(·),v(·).By convention,we setϑ−C (x)=ϑ+C(x)=0on C.Remarks–Note that here again the definition of the upper and lower value functions are not sym-metric:this is related to the fact that the target assumed to be closed,so that the game is intrinsically asymmetric.–The typical pursuit game is the case when the target coincides with the diagonal:C= {(y,z),|y=z}.We refer the reader to[6,29]for various types of pursuit games.The formalism of the present survey is adapted from[50].4.2Main ResultThe main difficulty for the analysis of state-constraint problems lies in the fact that two trajectories of a control system starting from two—close—different initial conditions could be estimated by classical arguments on the continuity of theflow of the differential equation. For constrained systems,it is easy to imagine cases where the constrained trajectories starting from two close initial conditions are rather far from each other.So,an important problem in order to get suitable estimates on constrained trajectories,is to obtain a kind of Filippov Theorem with ly a result which allows one to approach—in a suitable sense—a given trajectory of the dynamics by a constrained trajectory.Note that similar results exist in the literature.However,we need here to construct a constrained trajectory in a nonanticipating way[26](cf.also[25]),which is not the case in the previous constructions.Proposition1Assume that conditions(5)are satisfied.For any R>0there exist C0= C0(R)>0such that for any initial time t0∈[0,T],for any y0,y1∈K U with|y0|,|y1|≤R,。
- 1、下载文档前请自行甄别文档内容的完整性,平台不提供额外的编辑、内容补充、找答案等附加服务。
- 2、"仅部分预览"的文档,不可在线预览部分如存在完整性等问题,可反馈申请退款(可完整预览的文档不适用该条件!)。
- 3、如文档侵犯您的权益,请联系客服反馈,我们会尽快为您处理(人工客服工作时间:9:00-18:30)。
BME STUDIES OF STOCHASTIC DIFFERENTIAL EQUATIONS REPRESENTING PHYSICAL LAWS -PART IIM.L. Serre and G. ChristakosEnvironmental Modelling Program, Department of Environmental Sciences & Engineering School of Public Health, University of North Carolina, Chapel Hill, NC 27599-7400, USA Classical Geostatistics methods have been designed to use mainly statistical knowledge about natural variables and they lack the ability to incorporate important forms of knowledge like physical laws and scientific theories into the mapping process. On the other hand, the powerful and versatile Bayesian maximum entropy (BME) method of Modern Geostatistics can accomplish such a task, rigorously and efficiently. In this work, BME is used to incorporate the Darcy law of subsurface hydrology in the spatial mapping of a hydraulic head field. The hydraulic map thus obtained is physically meaningful as well as numerically more accurate than that obtained using classical methods (e.g., kriging). Moreover, taking advantage of the Darcy law the BME hydraulic head mapping can involve other related soil properties, like hydraulic conductivity. The approach leads to very accurate hydraulic head solutions, and may be also applied to study the inverse problem, in which one seeks to estimate hydraulic conductivity from hydraulic head measurements.1. INTRODUCTIONThe Bayesian Maximum Entropy (BME) method of Modern Geostatistics is a method which offers a rigorous framework to account for a large class of general and specificatory knowledge bases [1-6]. The general knowledge G includes physical laws, scientific theories, empirical relationships, and statistical moments. The specificatory knowledge S includes hard data (exact measurement) and soft data (such as intervals, probability assessments, uncertain observations and fuzzy sets) that are specific to the mapping situation. Previous studies have shown that the BME method is very useful and numerically efficient in a number of practical situations in which various forms of soft data are available [5, 6]. In the first part of this work ([4]) we discussed two methods used by BME analysis in order to account for physical knowledge in the form of physical laws. In this work, the BME formalism is used to incorporate the Darcy law of groundwater flow in porous media, which relates changes in hydraulic head with soil properties such as the hydraulic conductivity or resistivity. Darcy law is considered part of the general knowledge G which leads to a prior G-based pdf that describes the joint spatial distribution of hydraulic head and resistivity. Then, the specificatory knowledge S is used to update the prior pdf leading to the posterior pdf of the hydraulic head map. Numerical examples show that, by accounting for the Darcy law BME provides predictions that are physically meaningful and numerically more accurate than classical data analysis that does not account for the physical law.2. INCORPORATING DARCY LAW IN BME MAPPINGConsider the Darcy law of one-dimensional flow usually expressed as K(s)∇H(s)=−q(s), where the specific discharge q(s) is considered deterministic while the hydraulic head H(s) and the hydraulic conductivity K(s) are random fields. It is convenient to define the hydraulic resistivity R(s)=1/K(s) and rewrite Darcy law asdH(s)/ds=−q(s)R(s).(1)Let H0 be the known value of H(s) at the spatial origin s=0, let Hmap=[H1,...,H nH]T be thevector of random variables representing the hydraulic head H(s) at points s1,...,sn H, and assumethat R data =[R n H +1,...,R n ]T is the vector of random variables representing the hydraulic resistivity R (s ) at points s n H +1,...,s n . At the prior stage of the BME analysis we seek to obtain the prior pdff G (H map ,R data ;s 1...s n ) given general knowledge G (which includes the Darcy law),where H map and R data represent a realization of the random vectors H map and R data ,respectively. The general knowledge G is transformed in a suitable set of moment equations as followsd H map ∫d R data ∫g α(H map ,R data )f G (H map ,R data ;s 1...s n )=g α (α=1,...,N α), where g α isa set of functions chosen such that their stochastic expectations g α are either known statistical moments or they can be inferred from Darcy law. For example, we assume that the hydraulic resistivity has a known mean m R (s )=R (s ), non-centered covariance C R (s ,s ')=R (s )R (s '), and 4th-order non-centered moment m R ,4(s )=R 4(s ). These moments are incorporated in the general knowledge by letting g i (R i ;s i )=R i and g i (s i )=m R (s i ), i ∈J R ={n H +1,...,n };g ij (R i ,R j ;s i ,s j )=R i R j and g ij (s i ,s j )=C R (s i ,s j ) for i ,j ∈J R ; and finally g n +i (R i ;s i )=R i 4and g n +i (s i )=m R ,4(s i ) for n +i ∈J R . On the other hand the moments describing the hydraulic head are not known a priori, but they may be inferred from Darcy law, Eq. (1).Using the Darcy law we may obtain expressions for the mean m H (s )=H (s ) and non-centered covariance function C H (s ,s ')=H (s )H (s ') of the hydraulic head, as well as the non-centered cross-covariance function C RH (s ,s ')=R (s )H (s '). Clearly, if we take the stochastic expectation of Eq. (1) and solve with respect to the boundary condition H 0 we obtain m H (s )=H o −du 0s∫q (u )m R (u ), which is readily incorporated into G by choosingg i (H i ;s i )=H i and g i (s i )=m H (s i ) for i ∈J H , where J R ={n H +1,...,n }. Similarly,multiplying Eq. (1) by R (s '), taking the stochastic expectation and solving, we obtain C HR (s ,s ')=H 0m R (s ')−duq (u )C R (u ,s ')0s∫, which we incorporate in G by choosingg ij (H i ,R j ;s i ,s j )=H i R j and g ij (s i ,s j )=C HR (s i ,s j ) for i ∈J H , j ∈J R . Other moments may be obtained in a similar way. For example, multiplying Eq. (1) by H (s ') and taking the stochasticexpectation leads C H (s ,s ')=du 0s∫du '0s '∫q (u )q (u ')C R (u ,u ')−H 02+H 0[m H (s )+m H (s ')], whichwe also incorporate in G by choosing g ij (H i ,H j ;s i ,s j )=H i H j and g ij (s i ,s j )=C H (s i ,s j ) for i ,j ∈J H . The selected g α functions, summarized in Table 1, represent a set of constraints which incorporates the general knowledge consisting of statistical moments and the Darcy ing these constraints under the epistemic goal of information maximization leads to the following solution for the prior pdf [3]f G (H map ,R data ;s 1...s n )=Z −1exp[µi i ∈J H ∑H i +µi i ∈J R ∑R i +µij j ∈J H∑i ∈J H ∑H i H j +µij j ∈J R∑i ∈J R ∑R i R j+µij j ∈J R∑i ∈J H ∑H i R i +µi +n i ∈J R∑R i 4](2)where the Lagrange coefficients µi and µij are calculated by solving the system of equations obtained from Table (1).In the posterior stage of the BME method, the specificatory knowledge S is used to update the prior pdf and lead to the posterior pdf f K (H k ) which completely describes the hydraulicTable 1: General knowledge constraintsαg αα=i , i ∈J g (H ;s )=H g (s )=m (s )α=i , i ∈J Rg i (R i ;s i )=R i g i (s i )=m R (s i )α=(ij ), i ∈J H , j ∈J Hg ij (H i ,H j ;s i ,s j )=H i H j g ij (s i ,s j )=C H (s i ,s j )α=(ij ), i ∈J R , j ∈J Rg ij (R i ,R j ;s i ,s j )=R i R j ;g ij (s i ,s j )=C R (s i ,s j )α=(ij ), i ∈J H , j ∈J Rg ij (H i ,R j ;s i ,s j )=H i R j ;g ij (s i ,s j )=C HR (s i ,s j )α=n +i , i ∈J Rg n +i (R i ;s i )=R i 4;g n +i (s i )=m R ,4(s i )head distribution. Let H hard T =[H 1,...,H m Hh ], H soft T=[H m Hh +1,...,H m H ], and H k represent H (s )at the hard data points, soft data points and estimation points, respectively, such that H map T =[H hard T H soft T H k ]. Similarly let R hard T =[R 1,...,R m Rh ] and R soft T =[R m Rh +1,...,R m R ]represent R (s ) at the hard and soft data points, respectively, such that R data T =[R hard T R soft T ]. For soft data of the interval type, the BME posterior pdf is given by ([3,4])f K (H k )=A −1d H soft l H u H ∫d R soft l Ru R∫f G (H map ,R data ), where l H and u H are the lower and upperbounds of the soft data for the hydraulic head such that l H ≤H soft ≤u H , and l R and u R are the lower and upper bounds of the soft data for the hydraulic resistivity so that l R ≤R soft ≤u R .3. SIMULATED CASE STUDYIn this case study we consider the R (s ) profile shown in Fig 1a. These values were generated at grid-points separated by a distance of 0.5 m , using the exponential covariance model c R (s ,s ')=c R ,0exp(−|s '−s |/a R ) with c R ,0=1 (sec/mm )2 and a R =6 m , and a mean of m R =2 sec/mm . The histogram of R (s ) values (Fig. 1b) is approximately symmetric with akurtosis coefficient of m R ,4/c R ,02≅3, while the corresponding K (s )=1/R (s ) values have an asymmetric distribution (Fig. 1c). The hydraulic head H (s ) values calculated by solving Darcy law, Eq. (1), with H 0=100 m and q (s )=q =0.05 mm /sec are shown in Fig. 2a. The mean hydraulic head m H (s )=H o −q m R s is shown with a dashed line in Fig. 2a, and the histogram of hydraulic head fluctuation H (s )−m H (s ) is shown with plain lines (Fig. 2b) at points corresponding to s =5 m , s =25 m and s =75 m . These histograms were obtained by using 2,000 H (s ) realizations, and they show that the variance of H (s ) increases as s increases. In Fig. 2b (dotted line) the prior pdf's for the hydraulic head at points s =5 m , s =25 m and s =75 m . Note that these are in excellent agreement with the experimental histogram calculated from the data.In a first test case, we consider the estimation of H (s )−m H (s ) using sparse measurements for H (s ) and R (s ). The selected hard and soft (interval) data are shown with triangles and error bars, respectively, in Fig 1a for R (s ), and in Fig 2a for H (s ). In Fig 3a the estimated head fluctuation profile was derived from simple kriging (SK) using only hard head data; the actual head fluctuation profile is also shown for comparison. Note the poor SK estimates at unobserved locations. The head fluctuation profile in Fig. 3b was obtained from BME using hard and soft (interval) head data as well as the Darcy law. Note that the BME estimated profile5010015020000.511.522.533.544.5Distance s (in meter)R (s ) (i n s e c /m m )(a)K (mm/sec)F r e q u e n c y(c)R (sec/mm)F r e q u e n c y(b)Figure 1: (a) Simulated profile for the hydraulic resistivity R (s ). Histograms of (b) the hydraulicresistivity R (s ), and (c) the hydraulic conductivity K (s )=1/R (s ).respectively. (b) H (s ) histogram at different spatial locations s .is a substantial improvement over the classical SK method. Indeed, by being able to incorporate the Darcy law, the BME method allowed to account for hard and soft (interval) hydraulic conductivity data, as well.In a second case study we consider the problem of estimating the hydraulic resistivity R (s )using sparse measurements of R (s ) and H (s ). This is sometimes called the inverse problem.While it is possible to obtain accurate measurements of the hydraulic head H (s ), it is difficult to directly measure the hydraulic resistivity. Therefore, in case study 2, we consider that the measurements for H (s ) are the hard data shown with triangles in Fig. 4a, while the information for R (s ) consists of the soft interval data shown with error bars in Fig. 4b. For illustration purposes we calculate BME estimates for 3 different mapping situations. In the first situation we assume that the specificatory knowledge consists of only the soft data for R (s ), and we show the corresponding BME profile for R (s ) in Fig. 4b. Note that this type of soft information commonly arises when estimating hydraulic resistivities, and the BME method provides a rigorous framework to account for such soft information. In the second mapping situation we assume that the specificatory knowledge includes both the soft data for R (s ), as well as the hard data for H (s ) shown with triangles in Fig. 4a. Using this information we obtain the profile shown in Fig. 4c, which clearly provides a substantial improvement over that of Fig. 4b. Note that the profile of Fig. 4c respects the soft data but also reproduces the important features of theH y d r a u l i c H e a d F l u c t u a t i o n (i n m e t e r )(triangles); (b) BME using hard and soft (interval) head data and the Darcy law. Dotted linesrepresent the actual head fluctuation profile.resistivity R (s ) obtained from: (b) BME using R (s ) data; (c) BME using data for both R (s ) and H (s ); and (d) BME using only H (s ) data. The dotted line represents the true R (s ) profile.true resistivity profile shown in dotted line. This unique feature of the BME approach is due to the fact that BME accounts for the Darcy law which relates H (s ) and R (s ), leading to substantial improvements in the estimation of the hydraulic resistivity. Finally, in Fig. 4d we show the R (s ) profile using only hard H (s ) data (triangles in Fig. 4a). Note that in this case the important features of the hydraulic resistivity profiles are well preserved.ACKNOWLEDGMENTS: This work was supported by grants from the National Institute of Environmental Health Sciences (Grant no. P42 ES05948-02) the Department of Energy (Grant no. DE-FC09-93SR18262), and the Army Research Office (Grant no. DAAG55-98-1-0289), and by the Computational Science Graduate Fellowship Program of the Office of Scientific Computing.REFERENCES1.CHRISTAKOS, G., "A Bayesian/maximum-entropy view to the spatial estimationproblem". Mathematical Geology, 22(7), 763-776 (1990).2.CHRISTAKOS, G.- Random Field Models in Earth Sciences. Academic Press,San Diego, CA, 1992.3.CHRISTAKOS, G. and X. LI - "Bayesian maximum entropy analysis and mapping:farewell to kriging estimators?" Mathematical Geology,30(4), 435-462 (1998).4.CHRISTAKOS, G, D.T. HRISTOPULOS and SERRE, M.L. - BME studies of stochasticdifferential equation representing physical laws -part I". In Proc. of the 5th Annual Confer.of the Internat. Assoc.for Mathematical Geology, Trodheim, Norway (1999).5.SERRE, M. L., P. BOGAERT and G. CHRISTAKOS. "Latest Computational Results inSpatiotemporal Prediction Using the Bayesian Maximum Entropy Method". In Proc. of the 4th Annual Confer. of the Internat. Assoc. for Mathematical Geology, Eds. Buccianti A., Nardi G., Potenza R., De Frede Editore, Napoli, 117-122 (1998).6.SERRE, M. L. and CHRISTAKOS, G.- "Modern geostatistics: computational BMEanalysis in the light of uncertain physical knowledge - the Equus Beds study". Journal of Stochastic Environmental Research and Risk Analysis, 13(1), 1-26 (1999)。