Computing the pipelined phaserotation FFT
基于强化学习的部分线性离散时间系统的最优输出调节

基于强化学习的部分线性离散时间系统的最优输出调节
庞文砚;范家璐;姜艺;LEWIS Frank Leroy
【期刊名称】《自动化学报》
【年(卷),期】2022(48)9
【摘要】针对同时具有线性外部干扰与非线性不确定性下的离散时间部分线性系统的最优输出调节问题,提出了仅利用在线数据的基于强化学习的数据驱动控制方法.首先,该问题可拆分为一个受约束的静态优化问题和一个动态规划问题,第一个问题可以解出调节器方程的解.第二个问题可以确定出控制器的最优反馈增益.然后,运用小增益定理证明了存在非线性不确定性离散时间部分线性系统的最优输出调节问题的稳定性.针对传统的控制方法需要准确的系统模型参数用来解决这两个优化问题,提出了一种数据驱动离线策略更新算法,该算法仅使用在线数据找到动态规划问题的解.然后,基于动态规划问题的解,利用在线数据为静态优化问题提供了最优解.最后,仿真结果验证了该方法的有效性.
【总页数】12页(P2242-2253)
【作者】庞文砚;范家璐;姜艺;LEWIS Frank Leroy
【作者单位】东北大学流程工业综合自动化国家重点实验室;德克萨斯大学阿灵顿分校
【正文语种】中文
【中图分类】TP3
【相关文献】
1.基于T-S模糊模型离散时间非线性网络系统的输出跟踪控制
2.基于平均驻留时间切换离散线性系统的降阶输出反馈控制
3.基于Q学习算法的随机离散时间系统的随机线性二次最优追踪控制
4.一般非线性离散时间系统的输出调节
5.基于零和博弈的部分未知线性离散系统多智能体分布式最优跟踪控制
因版权原因,仅展示原文概要,查看原文内容请购买。
计算机科学与技术专业使用阈值技术的图像分割等毕业论文外文文献翻译及原文
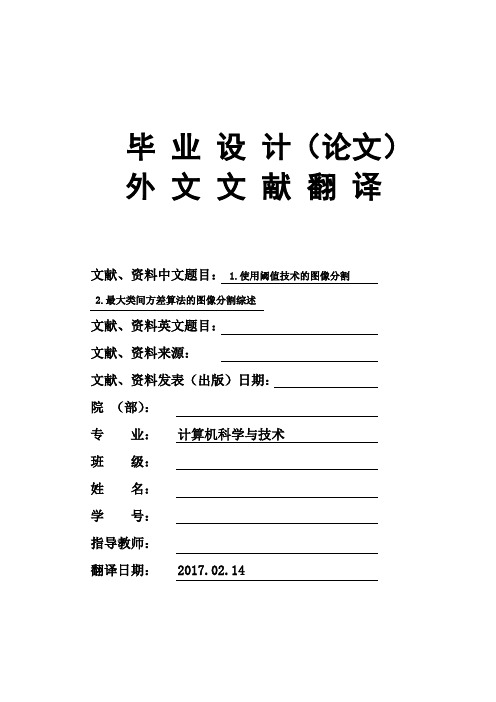
毕业设计(论文)外文文献翻译文献、资料中文题目: 1.使用阈值技术的图像分割2.最大类间方差算法的图像分割综述文献、资料英文题目:文献、资料来源:文献、资料发表(出版)日期:院(部):专业:计算机科学与技术班级:姓名:学号:指导教师:翻译日期: 2017.02.14毕业设计(论文)题目基于遗传算法的自动图像分割软件开发翻译(1)题目Image Segmentation by Using ThresholdTechniques翻译(2)题目A Review on Otsu Image Segmentation Algorithm使用阈值技术的图像分割 1摘要本文试图通过5阈值法作为平均法,P-tile算法,直方图相关技术(HDT),边缘最大化技术(EMT)和可视化技术进行了分割图像技术的研究,彼此比较从而选择合的阈值分割图像的最佳技术。
这些技术适用于三个卫星图像选择作为阈值分割图像的基本猜测。
关键词:图像分割,阈值,自动阈值1 引言分割算法是基于不连续性和相似性这两个基本属性之一的强度值。
第一类是基于在强度的突然变化,如在图像的边缘进行分区的图像。
第二类是根据预定义标准基于分割的图像转换成类似的区域。
直方图阈值的方法属于这一类。
本文研究第二类(阈值技术)在这种情况下,通过这项课题可以给予这些研究简要介绍。
阈分割技术可分为三个不同的类:首先局部技术基于像素和它们临近地区的局部性质。
其次采用全局技术分割图像可以获得图像的全局信息(通过使用图像直方图,例如;全局纹理属性)。
并且拆分,合并,生长技术,为了获得良好的分割效果同时使用的同质化和几何近似的概念。
最后的图像分割,在图像分析的领域中,常用于将像素划分成区域,以确定一个图像的组成[1][2]。
他们提出了一种二维(2-D)的直方图基于多分辨率分析(MRA)的自适应阈值的方法,降低了计算的二维直方图的复杂而提高了多分辨率阈值法的搜索精度。
这样的方法源于通过灰度级和灵活性的空间相关性的多分辨率阈值分割方法中的阈值的寻找以及效率由二维直方图阈值分割方法所取得的非凡分割效果。
基于自注意力机制和多尺度输入输出的医学图像分割算法
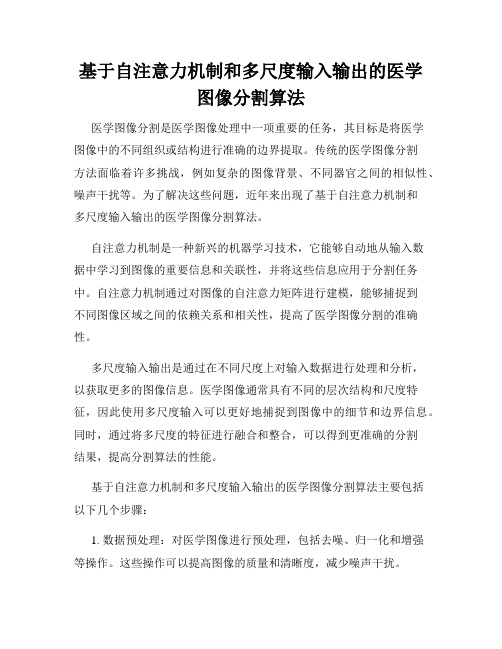
基于自注意力机制和多尺度输入输出的医学图像分割算法医学图像分割是医学图像处理中一项重要的任务,其目标是将医学图像中的不同组织或结构进行准确的边界提取。
传统的医学图像分割方法面临着许多挑战,例如复杂的图像背景、不同器官之间的相似性、噪声干扰等。
为了解决这些问题,近年来出现了基于自注意力机制和多尺度输入输出的医学图像分割算法。
自注意力机制是一种新兴的机器学习技术,它能够自动地从输入数据中学习到图像的重要信息和关联性,并将这些信息应用于分割任务中。
自注意力机制通过对图像的自注意力矩阵进行建模,能够捕捉到不同图像区域之间的依赖关系和相关性,提高了医学图像分割的准确性。
多尺度输入输出是通过在不同尺度上对输入数据进行处理和分析,以获取更多的图像信息。
医学图像通常具有不同的层次结构和尺度特征,因此使用多尺度输入可以更好地捕捉到图像中的细节和边界信息。
同时,通过将多尺度的特征进行融合和整合,可以得到更准确的分割结果,提高分割算法的性能。
基于自注意力机制和多尺度输入输出的医学图像分割算法主要包括以下几个步骤:1. 数据预处理:对医学图像进行预处理,包括去噪、归一化和增强等操作。
这些操作可以提高图像的质量和清晰度,减少噪声干扰。
2. 特征提取:使用卷积神经网络(CNN)等方法对医学图像进行特征提取,得到图像在不同尺度上的特征表示。
这些特征包括颜色、纹理、形状等信息,能够帮助算法更好地理解和分析图像。
3. 自注意力机制:通过自注意力机制对提取的特征进行建模和整合。
自注意力机制能够自动学习到图像中的重要信息和关联性,并将这些信息应用于分割任务中。
通过自注意力机制,算法可以更准确地捕捉到图像中不同区域之间的依赖关系和相关性。
4. 多尺度输入输出:通过在不同尺度上对输入数据进行处理和分析,获取更多的图像信息。
可以使用图像金字塔、多尺度卷积等方法对输入图像进行多尺度处理,在不同尺度上提取特征。
同时,通过将多尺度的特征进行融合和整合,得到更准确的分割结果。
caimr计算方法
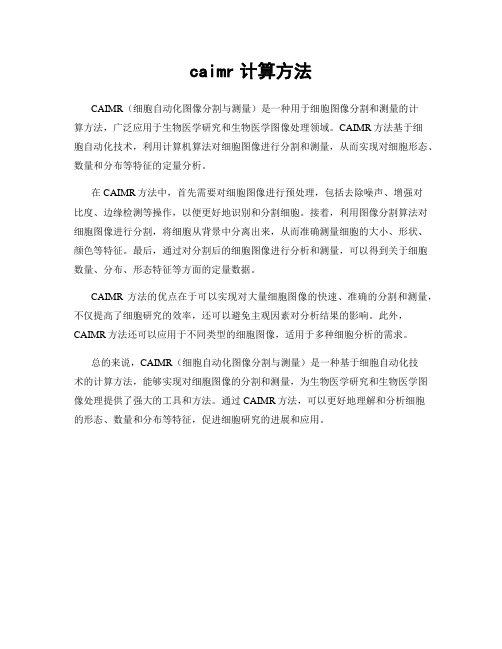
caimr计算方法
CAIMR(细胞自动化图像分割与测量)是一种用于细胞图像分割和测量的计
算方法,广泛应用于生物医学研究和生物医学图像处理领域。
CAIMR方法基于细
胞自动化技术,利用计算机算法对细胞图像进行分割和测量,从而实现对细胞形态、数量和分布等特征的定量分析。
在CAIMR方法中,首先需要对细胞图像进行预处理,包括去除噪声、增强对
比度、边缘检测等操作,以便更好地识别和分割细胞。
接着,利用图像分割算法对细胞图像进行分割,将细胞从背景中分离出来,从而准确测量细胞的大小、形状、颜色等特征。
最后,通过对分割后的细胞图像进行分析和测量,可以得到关于细胞数量、分布、形态特征等方面的定量数据。
CAIMR方法的优点在于可以实现对大量细胞图像的快速、准确的分割和测量,不仅提高了细胞研究的效率,还可以避免主观因素对分析结果的影响。
此外,CAIMR方法还可以应用于不同类型的细胞图像,适用于多种细胞分析的需求。
总的来说,CAIMR(细胞自动化图像分割与测量)是一种基于细胞自动化技
术的计算方法,能够实现对细胞图像的分割和测量,为生物医学研究和生物医学图像处理提供了强大的工具和方法。
通过CAIMR方法,可以更好地理解和分析细胞
的形态、数量和分布等特征,促进细胞研究的进展和应用。
NEURAL NETWORK COMPUTATION METHOD, DEVICE, READABL

专利名称:NEURAL NETWORK COMPUTATIONMETHOD, DEVICE, READABLE STORAGEMEDIA AND ELECTRONIC EQUIPMENT发明人:Zhuoran ZHAO,Zhenjiang WANG申请号:US17468136申请日:20210907公开号:US20220076097A1公开日:20220310专利内容由知识产权出版社提供专利附图:摘要:The present application discloses a neural network computation method includes determining the size of the first feature map obtained when the processorcomputes the present layer of the neural network before performing convolution computation on the next layer of the neural network; determining a convolution computation order of the next layer according to the size of the first feature map and the size of the second feature map for a convolution supported by the next layer; performing convolution computation instructions from the next layer based on the convolution computation order. Exemplary embodiments in the present disclosure decrease the interlayer feature map data access overhead and reduce the idle time of a computation unit by leaving out the storage of the first feature map and the loading process of the second feature map.申请人:HORIZON (SHANGHAI) ARTIFICIAL INTELLIGENCE TECHNOLOGY CO., LTD.地址:Shanghai CN国籍:CN更多信息请下载全文后查看。
用于生命科学的人工智能定量相位成像方法
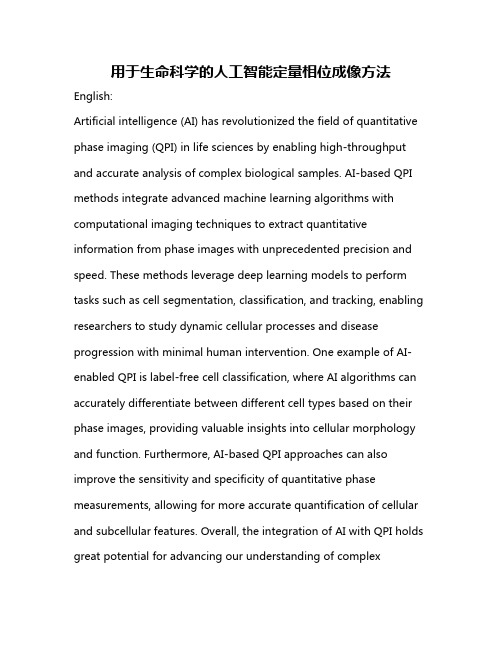
用于生命科学的人工智能定量相位成像方法English:Artificial intelligence (AI) has revolutionized the field of quantitative phase imaging (QPI) in life sciences by enabling high-throughput and accurate analysis of complex biological samples. AI-based QPI methods integrate advanced machine learning algorithms with computational imaging techniques to extract quantitative information from phase images with unprecedented precision and speed. These methods leverage deep learning models to perform tasks such as cell segmentation, classification, and tracking, enabling researchers to study dynamic cellular processes and disease progression with minimal human intervention. One example of AI-enabled QPI is label-free cell classification, where AI algorithms can accurately differentiate between different cell types based on their phase images, providing valuable insights into cellular morphology and function. Furthermore, AI-based QPI approaches can also improve the sensitivity and specificity of quantitative phase measurements, allowing for more accurate quantification of cellular and subcellular features. Overall, the integration of AI with QPI holds great potential for advancing our understanding of complexbiological systems and accelerating the development of novel diagnostic and therapeutic strategies in the life sciences.中文翻译:人工智能(AI)已经彻底改革了生命科学中的定量相位成像(QPI)领域,通过实现对复杂生物样品的高通量和准确分析。
基于动态规划提取信号小波脊和瞬时频率
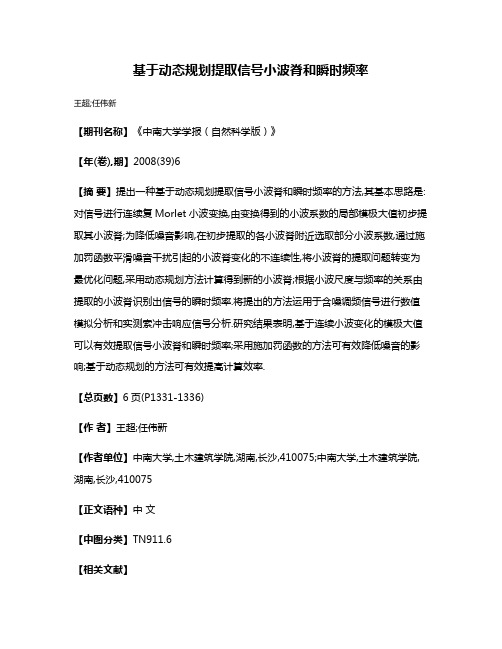
基于动态规划提取信号小波脊和瞬时频率
王超;任伟新
【期刊名称】《中南大学学报(自然科学版)》
【年(卷),期】2008(39)6
【摘要】提出一种基于动态规划提取信号小波脊和瞬时频率的方法,其基本思路是:对信号进行连续复Morlet小波变换,由变换得到的小波系数的局部模极大值初步提取其小波脊;为降低噪音影响,在初步提取的各小波脊附近选取部分小波系数,通过施加罚函数平滑噪音干扰引起的小波脊变化的不连续性,将小波脊的提取问题转变为最优化问题,采用动态规划方法计算得到新的小波脊;根据小波尺度与频率的关系由提取的小波脊识别出信号的瞬时频率.将提出的方法运用于含噪调频信号进行数值模拟分析和实测索冲击响应信号分析.研究结果表明,基于连续小波变化的模极大值可以有效提取信号小波脊和瞬时频率;采用施加罚函数的方法可有效降低噪音的影响;基于动态规划的方法可有效提高计算效率.
【总页数】6页(P1331-1336)
【作者】王超;任伟新
【作者单位】中南大学,土木建筑学院,湖南,长沙,410075;中南大学,土木建筑学院,湖南,长沙,410075
【正文语种】中文
【中图分类】TN911.6
【相关文献】
1.基于小波脊的UM71信号瞬时特征提取 [J], 林王仲;戴云陶
2.基于改进小波脊提取算法的数字信号瞬时频率估计方法 [J], 汪赵华;郭立;李辉
3.基于二进小波变换的实信号的多尺度Hilbert变换和瞬时频率提取 [J], 蔡毓;刘贵忠;侯兴松
4.基于SWT的自适应多脊提取的滚动轴承瞬时频率估计 [J], 李延峰;韩振南;王志坚;武学峰
5.基于最大坡度法提取非平稳信号小波脊线和瞬时频率 [J], 刘景良;任伟新;王超;黄文金
因版权原因,仅展示原文概要,查看原文内容请购买。
基于序贯设计和高斯过程模型的结构动力不确定性量化方法
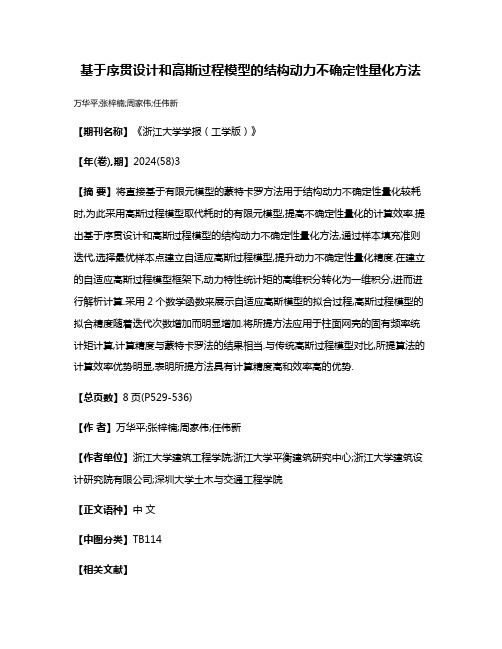
基于序贯设计和高斯过程模型的结构动力不确定性量化方法万华平;张梓楠;周家伟;任伟新
【期刊名称】《浙江大学学报(工学版)》
【年(卷),期】2024(58)3
【摘要】将直接基于有限元模型的蒙特卡罗方法用于结构动力不确定性量化较耗时,为此采用高斯过程模型取代耗时的有限元模型,提高不确定性量化的计算效率.提出基于序贯设计和高斯过程模型的结构动力不确定性量化方法,通过样本填充准则迭代,选择最优样本点建立自适应高斯过程模型,提升动力不确定性量化精度.在建立的自适应高斯过程模型框架下,动力特性统计矩的高维积分转化为一维积分,进而进行解析计算.采用2个数学函数来展示自适应高斯模型的拟合过程,高斯过程模型的拟合精度随着迭代次数增加而明显增加.将所提方法应用于柱面网壳的固有频率统计矩计算,计算精度与蒙特卡罗法的结果相当.与传统高斯过程模型对比,所提算法的计算效率优势明显,表明所提方法具有计算精度高和效率高的优势.
【总页数】8页(P529-536)
【作者】万华平;张梓楠;周家伟;任伟新
【作者单位】浙江大学建筑工程学院;浙江大学平衡建筑研究中心;浙江大学建筑设计研究院有限公司;深圳大学土木与交通工程学院
【正文语种】中文
【中图分类】TB114
【相关文献】
1.基于渗透系数序贯高斯模拟的水库渗漏量不确定性分析
2.基于Stochastic Kriging模型的不确定性序贯试验设计方法
3.基于序贯高斯条件模拟的土壤重金属含量预测与不确定性评价——以宜兴市土壤Hg为例
4.基于广义协同高斯过程模型的结构不确定性量化解析方法
5.基于高斯过程模型的定性定量因子混合补充试验设计方法
因版权原因,仅展示原文概要,查看原文内容请购买。
- 1、下载文档前请自行甄别文档内容的完整性,平台不提供额外的编辑、内容补充、找答案等附加服务。
- 2、"仅部分预览"的文档,不可在线预览部分如存在完整性等问题,可反馈申请退款(可完整预览的文档不适用该条件!)。
- 3、如文档侵犯您的权益,请联系客服反馈,我们会尽快为您处理(人工客服工作时间:9:00-18:30)。
Computing the Pipelined Phase-Rotation FFTLanghorne P.Withers,Jr.,John E.Whelchel,David R.O’Hallaron,Peter J.LieuJuly13,1993CMU-CS-93-174School of Computer ScienceCarnegie Mellon UniversityPittsburgh,PA15213David O’Hallaron and Peter Lieu are in the School of Computer Science at Carnegie ngWithers and John Whelchel are with E-Systems,Inc.AbstractThe phase-rotation FFT is a new form of the FFT that replaces data movement with multiplications by constant phasor multipliers.The result is an FFT that is simple to pipeline.This paper reports some fundamental new improvements to the original phase-rotation FFT design,provides a complete description of the algorithm directly in terms of the parallel pipeline,and describes a radix-2implementation on the iWarp computer system that balances computation and communication to run at the full-bandwidth of the communications links,regardless of the input data set size.Supported in part by the Advanced Research Projects Agency,Information Science and Technology Office,under the title "Research on Parallel Computing,"ARPA Order No.7330.Work furnished in connection with this research is provided under prime contract MDA972–90–C–0035issued by ARPA/CMO to Carnegie Mellon University,and in part by the Air Force Office of Scientific Research under Contract F49620–92–J–0131.Also supported in part by an E-Systems IR&D program.Keywords:multicomputers,signal processing,Fast Fourier Transform1.IntroductionThe Fast Fourier Transform(FFT)is an important algorithm with many applications in signal processing and scientific computing.The Whelchel phase-rotation FFT[9]derives from the Pease constant-geometry FFT[7],which itself derives from the original Cooley-Tukey FFT[4]expressed in terms of Kronecker products.The phase-rotation FFT of radix is designed for a pipeline of parallel data channels.At each time step,in each stage,the pipeline carries the next data points,one from each channel,into a Discrete Fourier Transform(DFT)kernel.Unlike earlier pipelined FFT’s[5,6],the phase-rotation FFT has the key property that no data is switched across channels,except within the DFT kernel and at the input and output.The phase-rotation approach extends easily to higher radices,reducing memory and latency while preserving the high throughput and parallel shuffling simplicity of lower radix versions.The phase-rotation FFT has also been extended to a vector-radix,multidimensional parallel-pipeline FFT with the same qualities of the one-dimensional algorithm,and without transposes[10].This paper describes the results of a project to implement the phase-rotation FFT on a parallel computer system.There are three main results:First,the digit-reversing shuffle step in the original version of the phase-rotation FFT[9]is a potential pipeline bottleneck because it requires communication between the data channels.We describe a new version that corrects this problem by using a parallel-pipeline digit-reversing step.Second,although the structure of the phase-rotation FFT is extremely simple,we have learned from experience that generating the appropriate twiddles and shuffle indices from the original matrix formula-tion[9]is quite difficult,even for the designers of the algorithm!To try to help the implementer,we have reformulated the phase-rotation FFT.We present a new set of recipes for generating the twiddles and shuffle indices directly in terms of the parallel pipeline.Finally,we describe mapping strategies for the phase-rotation FFT on the iWarp,a parallel computer system developed by Intel and Carnegie Mellon[1,2].We describe afine-grained approach for an-point radix-2phase-rotation FFT that balances computation and communication to run at the full40Mbytes/sec rate of the iWarp physical links,regardless of the size of the input data sets.Section2introduces the phase-rotation concept.Section3formally defines the improved FFT algorithm. Section4gives the recipes for generating the twiddles and shuffle indices in terms of the parallel pipeline. Finally,Section5describes the full-bandwidth implementation on iWarp.2.The basic ideaThis section introduces the concept of the phase-rotation FFT.Starting with the Pease constant-geometry FFT,we informally derive the pipelined phase-rotation FFT,identifying the key insights along the way.2.1.Constant-geometry FFTFigure1(a)shows theflowgraph for a radix--point decimation-in-frequency(DIF)constant-geometry FFT,with2and8.There are stages.Each stage contains kernels.Each kernel is an operator that performs an-point DFT.For radix2,each kernel inputs two complex numbers and outputs two complex numbers.(For simplicity,twiddles are not explicitly shown in theflowgraph.)Each stage in the constant-geometry FFT performs an identical perfect stride-by-shuffle of its data vector,where.If the data vector is regarded as an array,stored in column-major order, then the perfect shuffle simply transposes it into an array.For example,the following transpose is a stride-by-4perfect shuffle,for8points and radix2:0415 26 370123 4567The data items in example above,labeled by their indices in the original column vector,are regarded as equivalent to a42array composed by a stride-by-4unstacking of the8-point column vector.After the transpose,the24array is equivalent to a new8-point column vector composed by a stride-by-2stacking. As we shall see,this transpose creates difficulties when we try to pipeline the constant-geometry FFT.And it is precisely these difficulties that the phase-rotation FFT addresses.2.2.Pipelining the FFTEach stage of the constant-geometry FFT can be computed on a single processor by pipelining the data. For example,Figure1(b)shows the pipeline for a single stage with radix 2.The pipeline consists of a sequence of operators connected by pipeline segments.Each pipeline segment consists of parallel channels.Each channel carries a stream of data points,which are labeled in this example by their indices from the original column vectors in Figure1(a).For each pipeline segment,the data points in the same position in each stream are known as an-frame,or simply,a frame.For example,in Figure1(b),the first frame in the pipeline segment between and is(0,4),the second frame is(1,5),and so on.At each time step,the twiddle operators()collectively read a frame(one complex number per operator),perform an element-wise complex multiplication,and write the resulting frame.Notice that each stream is operated on independently.Similarly,the kernel operator()reads a frame,computes the radix-kernel,and writes the resulting frame.In this case,the streams are not independent;each data item in the output frame is a function of every data point in the input frame.The twiddle and kernel operators pipeline nicely because during each time step they independently read and write a single number.However,the pipelined shuffle operator()is less well behaved.To produce one output frame,the shuffle operator must read and store the data points from each stream.Thus, requires memory cycles to produce each frame.(Notice that transposes the data directly into an pipeline segment;even starting with data already in an pipeline,still performs“row-to-column”motions.)This is an example of the memory-bank conflict discussed in[8,pp.31-32].The conflict is clear in Figure1(b).To assemble itsfirst output frame,must read both0and4from the upper stream to its left. Then it must read1and5from the lower stream,and so on.twiddles parallel pipeline shufflekerneltwiddlesframe-wise cyclic rotations parallel pipeline shufflekernel pipeline stage 0kernelsstage 1kernels stage 2kernels stride-by-4shuffle stride-by-4shuffle(a)(b)(c)(d)v arying frame-wise cyclic rotations v arying 01234567Figure 1:Derivation of the phase-rotation FFT.(a)Initial con-stant-geometry FFT.(b)Pipelined constant geometry FFT.(c)Pipelined FFTbased on cyclic rotations.(d)Pipelined phase-rotation FFT.0246 13570123 45670257 13460527 4163Figure2:Replacing the perfect shuffle with three simpler shuffles.We would like to replace the troublesome perfect shuffle operation with a parallel-pipeline shuffle,where each stream is read and written independently and in parallel.The next section describes the insights that make this possible.2.3.The phase-rotation conceptThis section describes how to replace the perfect shuffle by a parallel-pipeline shuffle,so that we can access the data streams in parallel.The basic idea is to rotate the data within frames,and then compensate for these motions by phase rotations of the twiddle factors.We begin with a“detour”around the perfect shuffle.That is,wefind a sequence of three simpler shuffles that is equivalent to the perfect shuffle.This idea is shown graphically in Figure2for an-point radix-2 example.Each radix-2pipeline segment is represented as matrix.Each row in the matrix corresponds to a stream,and each column corresponds to a frame.Frames(columns)are arranged left-to-right in reverse-time order in the matrix.Thefirst step in Figure2is a set of cyclic rotations,called,which rotates each frame.These rotations are frame-wise in the sense that only data points contained in the same frame are rotated across the streams.Notice that in the radix-2case,half of the rotations leave the corresponding frame unchanged. The next step is a parallel-pipeline shuffle,which permutes the data in each stream.Notice that no data points need to be transferred between streams in this step.The last step is another set of frame-wise cyclic rotations,called,which leave the data in the same order that the perfect shuffle would.Note that and change the number of rotations per frame at different paces,one slow and one fast.These varying rates are difficult to see in the radix-2case,but are much more apparent in the higher-radix cases.If we apply the idea in Figure2)to each stage of the pipelined FFT in Figure1(b),replacing each perfect shuffle with three simpler shuffles,we get a pipelined FFT based on cyclic rotations,which is shown in Figure1(c).The kind of basic frame-wise rotations in Figure1(c)that is applied at slow-varying,and then fast-varying rates,is represented in general by the cyclic(circular)shift permutation matrix,made by permuting the rows of the identity matrix down by one row,and moving the bottom row up to the top.For≡Figure3:Interpretation of F C D Fexample,40001100001000010The key insight of the phase-rotation FFT is that the cyclic shift theorem for the DFT can be applied to the cyclic shift operators in Figure1(c).In matrix form,the cyclic shift theorem for a DFT is the relation1 where121is a set of twiddles,and the DFT matrix of size is11where2FFT with a parallel-pipeline shuffle,followed by a frame-wise cyclic rotation.The advantage of this new approach is that during the digit-reversing step at the end,all communication between streams is limited to data points within a single frame.For radix and points(1),the1-dimensional phase-rotation FFT is a matrix factorization of the-point DFT matrix.Starting with the Pease constant-geometry factorization,we replace its perfect shuffles by.Similarly,at the left end we replace the radix-index-digit-reversing permutation of data points by,where is another parallel-pipeline shuffle that will be defined formally in Section4.The phase-rotation FFT is then defined by:1vigorousalgebraicshuffling1(2)Let as before,and2.is a direct(tensor,Kronecker)productdiag.We interpret this as a kernel DFT operating on successive frames of points placed in the pipeline.For1:,the other parts of(2)are defined by112111111112:1(3) The direct sums are of the form1diag011and denotes the transpose of.See[10]for more on the basic definitions and relations used to derive (2),as well as the generalization to higher dimension FFT’s.Note that the stages in(2)are counted in reverse time order by the index.This is in keeping with the fact that(2)is a decimation-in-frequency(DIF)version of the FFT.The transpose of(2),with the product 1,is the decimation-in-time(DIT)version of the phase-rotation FFT.A shuffle and its inverse remain at the input and output ends of the pipeline,respectively.As we have seen,is a completely frame-wise rotation.It rotates(commutates)the data within each successive frame(column-vector)of the pipeline segment for a stage.There is also an implicit frame-wise broadcast within each FFT kernel engine,when an-point DFT is somehow computed.So in the phase-rotation FFT,data motion is all parallel,except for frame-wise motions at I/O and at every FFT kernel.The simplicity of the phase-rotation FFT is that no data point ever moves both down and across the pipeline in one time-step.4.Pipeline recipesWhile the structure of the pipelined phase-rotation FFT is extremely simple,experience has taught us that generating the appropriate twiddles and shuffle indices from the matrix formulations of(2)and(3)is difficult and confusing.To address this problem,we have developed a collection of recipes for generating the phase-rotation twiddles and shuffle indices off-line.The recipes are defined for any1D phase-rotation FFT of points.Following[8],they are written in a M ATLAB-like format.As we saw in(2),the pipelined phase-rotation FFT performs a typical“twiddle,shuffle,kernel”cycle at each stage.Only the twiddles vary from stage to stage,and there is a digit-reversing shuffle equivalent at the end.To implement this FFT using parallel pipeline segments(one per stage),we insert the -vector of input data into the pipeline as an array:thefirst points of go into thefirst frame (column),the second points go into the second frame,and so on.We must also have a shuffle address and a twiddle factor ready for each point in the pipeline.In other words,we would like tofill one copy of the pipeline segment with addresses,and another copy with twiddles.Then the processors in each stage of the pipeline will know what to do at each time-step0: 1. Using the current frame of addresses,they will fetch the current-frame of data0:10:1 and the current-frame of twiddles0:10:1(pointwise in parallel),multiply these two frames pointwise,then do an-point DFT of the twiddled data frame.That is how each stage is implemented in the parallel pipeline.The twiddle and shuffle recipes in this section are“in place”in the sense that they work inside the pipeline segments that will contain the desired addresses and twiddles.They are not“in place”in the usual sense,as we will freely use an input and an output copy of a pipeline segment.This approach avoids constructing and operating with large matrices(each containing only non-zero elements).Eachparallel-pipeline function recipe is given a name similar to that of the matrix factor in the FFT(2) that it effectively implements.4.1.Shuffle recipesAs a convention,pipeline addresses(pipeline array row and column indices)run0:1and0:1, respectively.To do parallel-pipeline shuffles,we only need the horizontal(column)addresses,since the data inside each pipe will only jump within that stream(row).The cross-stream shuffles,Cslow and Cfast,are implemented using,a cyclic rotation of a frame(a vertical slice of the parallel pipeline)that has the effect of.takes a column-vector01211012.function=Cslowfor1:for1:::1endendfunction=Cfastfor1:for1:::1endendThe inverses of Cslow and Cfast are formed by simply reversing.Next,we define some perfect shuffles.function=S!stride byfor0:1for10::2111:2:1endendfunction=S1!stride byfor0:1for10::211:1:21endendTo implement the parallel-pipeline shuffles,S,S 1,and Q,we will use the parallel-pipeline addresses,which are computed by the following function: function=Saddressesfor0:1::endfunction S1=SThe pipeline addresses for Q are obtained by block-perfect shuffles(along the length of the pipeline)of the addresses for S:function Q=Sfunction=Dslowtwiddles2for0:1for0:1:1211endendThe inverses of and are just their complex conjugates,and are generated simply by replacing by1.For stages1:(counted down from),we generate pipelined twiddles byfunction=T:0:!copy columns1endendendThe rest of the twiddle arrays can now be defined in terms of the shuffles:=S11=Cslow=Cslow1=S1=Cslow=S1111if1=1end=5.Implementation issuesIn this section we describe issues that arise when the phase-rotation FFT is implemented on a real parallel system.In particular,we describe implementation approaches for the radix-2FFT on the iWarp system. The main result is a scalable implementation of the pipelined phase-rotation FFT that runs at the full40 Mbytes/second rate of the iWarp physical links.5.1.iWarpThe iWarp is a private-memory multicomputer developed jointly by Intel and Carnegie Mellon[1,2].iWarp systems are2-dimensional tori of iWarp nodes,ranging in size from4to1024nodes.Each node consists of an iWarp component,up to16Mbytes of off-chip local memory,and a set of8unidirectional communication links that physically connect the node to four neighboring nodes.The iWarp component is a VLSI chip that contains a processing agent and a communication agent.The processing agent is a general-purpose load-store microprocessor,centered around a12832-bit register file,that runs at a maximum rate of20MFLOPs.The local memory is accessed at a rate of160Mbytes/sec. Each link runs at40Mbytes/sec,for a maximum aggregate bandwidth of320Mbytes/sec per node.The key feature of the iWarp is its communication system,which is summarized in Figure4.Each communication agent contains a set of20hardware FIFO queues.Each queue can hold up to832-bit words.iWarp nodes communicate with other nodes using unidirectional point-to-point structures calledFigure4:iWarp communication concepts.pathways.Each pathway is a sequence of queues.Pathways can be created and destroyed dynamically at runtime.Figure4shows a pair of such pathways.Data traveling along a pathway passes from queue to queue automatically,without disturbing the computations on intermediate nodes.For example,in Figure4,data items traveling over the pair of pathways do not disturb the computation on node1.The latency from queue to queue is small,ranging from100-300nanoseconds.Multiple pathways can share the same link.For example,in Figure4,two pathways share the link from node1to node2.In this case,the pathways share the link bandwidth in a round-robin fashion,one word at a time.If only one pathway is sending data over a link,then it gets the entire link bandwidth.If multiple pathways are sending data over a link,then the link can be utilized at the full40Mbytes/sec,and each pathway is guaranteed a proportional fraction of the bandwidth.User programs can directly access the queues,one word at a time,by reading and writing special registers in the registerfile called gates.To an iWarp instruction,a gate is just another register in the registerfile.The important point is that a program can read or write a word in a queue with the latency of a register access.A single instruction can read and write up to4words from queues,with a maximum aggregate bandwidth of160Mbytes/sec.Gates can be accessed directly from user-level C programs.5.2.Mapping strategies on iWarpThe problem is to develop a mapping of theflowgraph in Figure1(d)to an iWarp array.The simplest mapping strategy is to assign eachflowgraph node to a unique processor node of a linear array,route theflowgraph arcs through this array,and then embed the resulting linear array in the iWarp torus.This approach,called the PHASE5mapping because it uses5iWarp nodes for each FFT stage,is shown in Figure5(a).Each iWarp node in PHASE5executes a small node program that implements itsflowgraph operator. Each twiddle node()repeatedly reads a complex number from its input pathway(via the gates),multiplies it by the appropriate twiddle(precomputed off-line using the recipes in Section4.2),and sends the result to its output pathway(again,via the gates).Each shuffle operator()repeatedly reads a complex data item from its input pathway,stores it in memory,and uses the appropriate shuffle index(again precomputed off-line using the recipes in Section4.1)to send an appropriate double-buffered data point to the output(a)(b)Figure5:Strategies for mapping one stage of the FFT onto a linear array.(a)PHASE5mapping.(b)PHASE3mapping.pathway.The kernel node()repeatedly reads two complex numbers from its input pathways,performs the radix-2DFT kernel operation,and outputs two complex numbers to its output pathways.Another approach,the PHASE3mapping,combines the twiddle and shuffle operators on a single node, as shown in Figure5(b),so that each stage requires3nodes instead of5nodes.As we shall see,the communication and computation throughputs of the two mappings are identical.The advantage of the PHASE3mapping is that it is more node-efficient,requiring fewer nodes per stage than the PHASE5 mapping.The advantage of the PHASE5mapping is its simplicity.Each node is assigned exactly one operator from theflowgraph.Figure6shows a working implementation of a16K-point radix-2phase-rotation FFT on a64-node iWarp array at Carnegie Mellon.The large squares are iWarp nodes,labeled with the corresponding operator and stage number.The small squares are queues.The arrows are iWarp pathways.The implementation is based on the PHASE3mapping from Figure5(b).Each of the14FFT stages uses3nodes,with an additional3 nodes for the parallel-pipeline digit-reversing step at the end.5.3.PerformanceWhile the details are beyond the scope of this paper,each iteration of each node program in the PHASE3 and PHASE5mappings runs in at most8clocks.At the peak rate of40Mbytes/sec,each link can produce and consume a32-bitfloating-point number every2clocks.Further,each data point in the pipeline is a complex number consisting of a pair of32-bitfloating-point words.As a result,each pathway requires exactly half of the available link bandwidth.Since each link is shared by two pathways,and since the iWarp communication agent gives each pathway an equal share of the link bandwidth,without disturbing the computations on intermediate nodes,each link is fully utilized.The result is a radix-2FFT that runs at the full40Mbytes/sec rate of an iWarp link,regardless of the number of points in the FFT!Since each sample consists of8bytes,the FFT runs at a constant rate of5Msamples/sec Given a sufficient number of nodes,the iWarp phase-rotation FFT’s will produce arbitrarily large FFT’s at this rate.Perhaps even more important,the performance is the same on smaller FFT’s.Another way to characterize the performance of the PHASE3and PHASE5mappings is by its com-putational throughput,expressed as millions offloating-point operations per second(MFLOPS).However, there is a subtlety involved in using MFLOPS as a performance measure.The iWarp phase-rotation FFTD0D0F0D1D1F1D2D2D5F4D4D4F3D3D3F2D5F5D6D6F6D7D7F7D10D10F9D9D9F8D8D8F10D11D11F11D12D12F12D13sink D14D14F13D13Figure6:16K-point pipelined phase-rotation FFT running at40Mbytes/sec(350MFLOPS)on iWarpperforms16floating-point operations per iteration per stage(2adds and4multiplies by each twiddle opera-tor,and4adds by the kernel operator).But the standard formula for computing FFT MFLOPS is5log floating-point operations per N-point FFT[3],which implies10floating-point operations per iteration per stage.Therefore,in order to do fair comparisons with other FFT algorithms,we must compute the phase rotation performance using the standard of10floating-point operations per iteration per stage,even though the phase-rotation FFT is actually performing16floating-point operations per iteration per stage.Since each node program executes its computation in at most8clocks,and since each clock is50 nanoseconds,each stage of the iWarp phase-rotation FFT runs at a rate of1109nanoseconds10fp operations50nanosecondsvalidates a simple and realistic approach for building scalable pipelined FFT’s on a programmable parallel system.Further,the implementation demonstrates that,given a balanced parallel computer architecture with word-level access to the communication links,it is possible to build FFT’s that run at the full link bandwidth of the links,even when the FFT’s are relatively small.AcknowledgementsWe would like to thank Tom Warfel and LeeAnn Tzeng for their help with the iWarp implementation,and Doug Noll and Doug Smith for discussions that led to the more node-efficient mapping.References[1]B ORKAR,S.,C OHN,R.,C OX,G.,G LEASON,S.,G ROSS,T.,K UNG,H.T.,L AM,M.,M OORE,B.,P ETERSON,C.,P IEPER,J.,R ANKIN,L.,T SENG,P.S.,S UTTON,J.,U RBANSKI,J.,AND W EBB,J.iWarp:An integrated solution to high-speed parallel computing.In Supercomputing’88(Nov.1988),pp.330–339.[2]B ORKAR,S.,C OHN,R.,C OX,G.,G ROSS,T.,K UNG,H.T.,L AM,M.,L EVINE,M.,M OORE,B.,M OORE,W.,P ETERSON,C.,S USMAN,J.,S UTTON,J.,U RBANSKI,J.,AND W EBB,J.Supporting systolic and memory communication in iWarp.In Proceedings of the17th Annual International Symposium on Computer Architecture (Seattle,WA,May1990),pp.70–81.[3]C ARLSON,D.Ultra-performance FFTs for the CRAY-2and CRAY Y-MP supercomputers.Journal of Super-computing6(1992),107–115.[4]C OOLEY,J.,AND T UKEY,J.An algorithm for the machine computation of complex Fourier series.Mathematicsof Computation19(Apr.1965),297–301.[5]C ORINTHIOS,M.The design of a class of Fast Fourier Transform computers.IEEE Transactions on ComputersC-20(June1971),617–623.[6]M C C LELLAN,J.,AND P URDY,R.Radar signal processing.In Applications of Digital Signal Processing,A.Oppenheim,Ed.Prentice-Hall,Englewood Cliffs,NJ,1978.[7]P EASE,M.An adaptation of the Fast Fourier Transform for parallel processing.Journal of the Association forComputing Machinery15(1968),252–264.[8]V AN L OAN,putational Frameworks for the Fast Fourier Transform.SIAM,Philadelphia,PA,1992.[9]W HELCHEL,J.,O’M ALLEY,J.,R INARD,W.,AND M C A RTHUR,J.The systolic phase rotation FFT-a newalgorithm and parallel processor architecture.In Proceedings of ICASSP‘90(Apr.1990),pp.1021–1024. [10]W ITHERS,J R.,L.,AND W HELCHEL,J.The multidimensional phase-rotation FFT-a new parallel architecture.InProceedings of ICASSP‘91(May1991),pp.2889–2892.。