Interpreting Face Images using Active Appearance Models
人脸识别英文专业词汇教学提纲
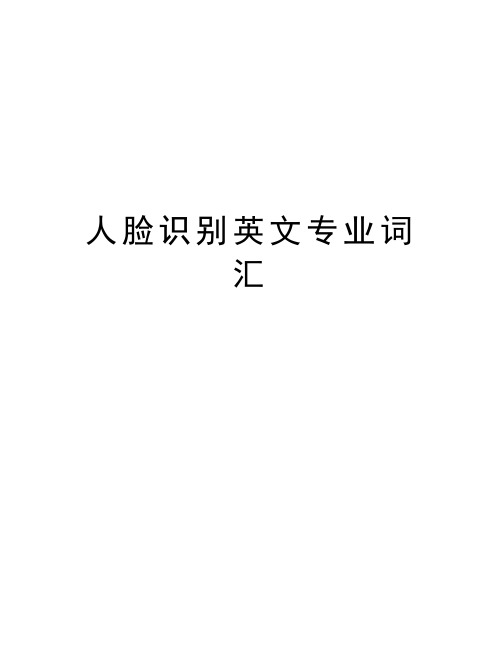
人脸识别英文专业词汇gallery set参考图像集Probe set=test set测试图像集face renderingFacial Landmark Detection人脸特征点检测3D Morphable Model 3D形变模型AAM (Active Appearance Model)主动外观模型Aging modeling老化建模Aging simulation老化模拟Analysis by synthesis 综合分析Aperture stop孔径光标栏Appearance Feature表观特征Baseline基准系统Benchmarking 确定基准Bidirectional relighting 双向重光照Camera calibration摄像机标定(校正)Cascade of classifiers 级联分类器face detection 人脸检测Facial expression面部表情Depth of field 景深Edgelet 小边特征Eigen light-fields本征光场Eigenface特征脸Exposure time曝光时间Expression editing表情编辑Expression mapping表情映射Partial Expression Ratio Image局部表情比率图(,PERI) extrapersonal variations类间变化Eye localization,眼睛定位face image acquisition 人脸图像获取Face aging人脸老化Face alignment人脸对齐Face categorization人脸分类Frontal faces 正面人脸Face Identification人脸识别Face recognition vendor test人脸识别供应商测试Face tracking人脸跟踪Facial action coding system面部动作编码系统Facial aging面部老化Facial animation parameters脸部动画参数Facial expression analysis人脸表情分析Facial landmark面部特征点Facial Definition Parameters人脸定义参数Field of view视场Focal length焦距Geometric warping几何扭曲Street view街景Head pose estimation头部姿态估计Harmonic reflectances谐波反射Horizontal scaling水平伸缩Identification rate识别率Illumination cone光照锥Inverse rendering逆向绘制技术Iterative closest point迭代最近点Lambertian model朗伯模型Light-field光场Local binary patterns局部二值模式Mechanical vibration机械振动Multi-view videos多视点视频Band selection波段选择Capture systems获取系统Frontal lighting正面光照Open-set identification开集识别Operating point操作点Person detection行人检测Person tracking行人跟踪Photometric stereo光度立体技术Pixellation像素化Pose correction姿态校正Privacy concern隐私关注Privacy policies隐私策略Profile extraction轮廓提取Rigid transformation刚体变换Sequential importance sampling序贯重要性抽样Skin reflectance model,皮肤反射模型Specular reflectance镜面反射Stereo baseline 立体基线Super-resolution超分辨率Facial side-view面部侧视图Texture mapping纹理映射Texture pattern纹理模式Rama Chellappa读博计划:1.完成先前关于指纹细节点统计建模的相关工作。
黑龙江省鹤岗市第一中学2022-2023学年高三上学期11月月考英语试题
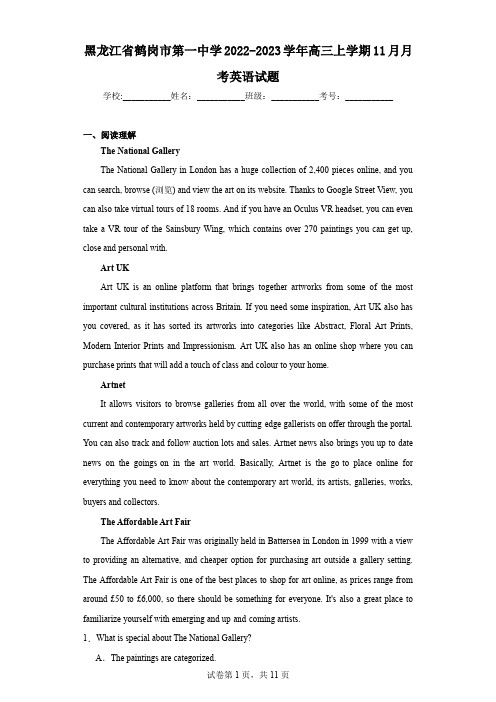
黑龙江省鹤岗市第一中学2022-2023学年高三上学期11月月考英语试题学校:___________姓名:___________班级:___________考号:___________一、阅读理解The National GalleryThe National Gallery in London has a huge collection of 2,400 pieces online, and you can search, browse (浏览) and view the art on its website. Thanks to Google Street View, you can also take virtual tours of 18 rooms. And if you have an Oculus VR headset, you can even take a VR tour of the Sainsbury Wing, which contains over 270 paintings you can get up, close and personal with.Art UKArt UK is an online platform that brings together artworks from some of the most important cultural institutions across Britain. If you need some inspiration, Art UK also has you covered, as it has sorted its artworks into categories like Abstract, Floral Art Prints, Modern Interior Prints and Impressionism. Art UK also has an online shop where you can purchase prints that will add a touch of class and colour to your home.ArtnetIt allows visitors to browse galleries from all over the world, with some of the most current and contemporary artworks held by cutting-edge gallerists on offer through the portal. You can also track and follow auction lots and sales. Artnet news also brings you up-to-date news on the goings-on in the art world. Basically, Artnet is the go-to place online for everything you need to know about the contemporary art world, its artists, galleries, works, buyers and collectors.The Affordable Art FairThe Affordable Art Fair was originally held in Battersea in London in 1999 with a view to providing an alternative, and cheaper option for purchasing art outside a gallery setting. The Affordable Art Fair is one of the best places to shop for art online, as prices range from around £50 to £6,000, so there should be something for everyone. It's also a great place to familiarize yourself with emerging and up-and-coming artists.1.What is special about The National Gallery?A.The paintings are categorized.B.It has a great collection from all over the world.C.It can make you as if you were really in the gallery.D.Its inspiration comes from important cultural institutions.2.Which website best suits people who want to know the latest information about art?A.Art UK.B.The National Gallery.C.The Affordable Art Fair.D.Artnet.3.What do Art UK and The Affordable Art Fair have in common?A.They have online shopping service.B.You can find Floral Art Prints in them.C.They provide cheap choices for the public.D.You can know some promising artists in them.Leia was fresh out of college when she began working as a member of a business-development team at a company. Though her skills had earned her the job, she was the youngest person in the team. “Everybody else was pretty much twice my age,” she says. “I exhibited” too much ambition’ in the eyes of my superiors. I heard about comments being made behind my back. There were even a couple of times when my superiors referred to my age right in front of me, saying I was too young: “What does a 23-year-old know about these things?”Leia tried to change her appearance at work. “I changed the way I dressed. I tried to dress older, more ‘ladylike’. I changed my mannerisms and tried to act older,” she says. “It worked, to an extent.” The comments about her age and inexperience lessened, but Leia says she still felt like her growth potential was limited. She left the company soon after.What Leia experienced was ageism, traditionally seen as something only older people face. For instance, older workers might be judged based on assumptions that they won’t fit into a progressive office or learn technology quickly. A US study showed that nearly two-thirds of workers aged 45 and older had seen or experienced age discrimination. But younger workers face age discrimination, too. In fact, new research shows it may actually be the youngest team members who are bearing the brunt of workplace ageism right now, potentially impacting their careers.Leia says removing ageism entirely will ultimately require a fundamental change to corporate culture, which has long tied seniority to skill. “We prize years of experience a littletoo much, and I don’t think years of experience and skill are necessarily correlated,” she says. “Steve Jobs was 21 when he founded Apple. We don’t know how much younger people actually have to contribute. Hopefully, more employers are realizing it.”4.What did Leia’s co-workers mainly talk about behind her back?A.Her appearance.B.Her younger age.C.Her way of behaving.D.Her family background.5.Why did Leia quit her job?A.She disliked dressing more ladylike.B.She received many negative comments.C.She was under much pressure from her work.D.She needed more room for her career growth.6.What can we know about older workers according to the text?A.They may be quick in learning technology.B.They have trouble adapting to a progressive office.C.They experience more ageism than younger workers.D.A small part of them were faced with age discrimination.7.Why does Leia mention Steve Jobs in the last paragraph?A.To share information about Apple Company.B.To tell us Steve Jobs’ major contributions.C.To show that age and experience are not connected.D.To prove that experience matters to young people.As artificial intelligence(AI) systems become more advanced, we can expect them to be used more often in the world of human medicine and healthcare. AI is designed to imitate(模仿)the human brain in decision making and learning, so with the computing power to learn tasks in days or even hours, it is possible to create medical AIs that rapidly outperform doctors in certain tasks.Data plays a hugely important role in helping AI systems learn about human medicine. AI systems are trained on large data sets gathered from real-life cases. Providing detailed patient information in quantities is a crucial factor for their success.One of the most important areas for influencing global health is in the field of epidemiology(流行病学)。
Unit 1 Interpreting 口译概况
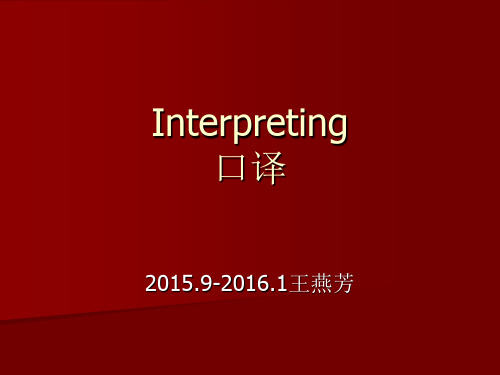
心动不如行动!
口译的培训模式
同声传译模式: SI=L + M + P + C 同声传译(Simultaneous Interpreting)= 听力与分析(Listening and Analysis)+ 短期 记忆(Short-term memory effort) + 言语传 达(Speech Production)
Interpreting 口译
2015.9-2016.1王燕芳
Interpreting 口译
商务英语口译(黄敏) VOA慢速英语 VOA标准英语 CN理论、口译背景知识以及对学 生进行口译基本技巧的训练,使学生初步掌握口 译程序和基本技巧,初步学会口译记忆方法、口 译笔记、口头概述、公众演讲等基本技巧和口译 基本策略,培养学生关心时事的信息意识,积累 知识,掌握文献检索、资料查询的基本方法;培 养学生的话语分析能力,提高学生的逻辑思维能 力、语言组织能力和双语表达能力,提高学生跨 文化交际的能力和英、汉两种语言互译的能力。
难易程度 证书有效期
初级:三级 > 三级 > 中级 中级:二级 > 二级 > 高级 高级:一级 > 一级 > 无 每3年重新注册 登记一次,一次 注册有效期3年
暂无
暂无
口译的发展前景
在“非全日制就业人员工资指导价位”表 中列出的54种行业里,同声传译以每小时 2000元人民币的价格拔得头筹。 同声传译价目表中,英语类1天1.2万~2.1 万元人民币,非英语类是1.8万元人民币。 业内人士称,平均每星期做两次同声翻译, 一年平均50多万RMB。 交传一天的平均工资在3000-6000RMB.
口译与笔译的异同
人脸表情识别英文参考资料
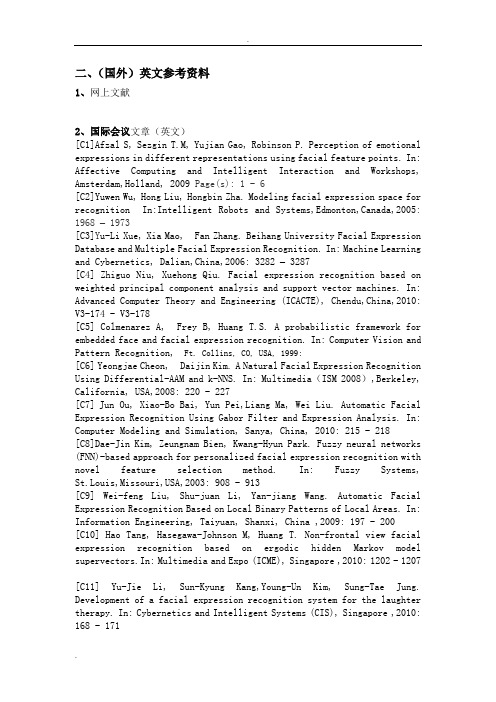
二、(国外)英文参考资料1、网上文献2、国际会议文章(英文)[C1]Afzal S, Sezgin T.M, Yujian Gao, Robinson P. Perception of emotional expressions in different representations using facial feature points. In: Affective Computing and Intelligent Interaction and Workshops, Amsterdam,Holland, 2009 Page(s): 1 - 6[C2]Yuwen Wu, Hong Liu, Hongbin Zha. Modeling facial expression space for recognition In:Intelligent Robots and Systems,Edmonton,Canada,2005: 1968 – 1973[C3]Y u-Li Xue, Xia Mao, Fan Zhang. Beihang University Facial Expression Database and Multiple Facial Expression Recognition. In: Machine Learning and Cybernetics, Dalian,China,2006: 3282 – 3287[C4] Zhiguo Niu, Xuehong Qiu. Facial expression recognition based on weighted principal component analysis and support vector machines. In: Advanced Computer Theory and Engineering (ICACTE), Chendu,China,2010: V3-174 - V3-178[C5] Colmenarez A, Frey B, Huang T.S. A probabilistic framework for embedded face and facial expression recognition. In: Computer Vision and Pattern Recognition, Ft. Collins, CO, USA, 1999:[C6] Yeongjae Cheon, Daijin Kim. A Natural Facial Expression Recognition Using Differential-AAM and k-NNS. In: Multimedia(ISM 2008),Berkeley, California, USA,2008: 220 - 227[C7]Jun Ou, Xiao-Bo Bai, Yun Pei,Liang Ma, Wei Liu. Automatic Facial Expression Recognition Using Gabor Filter and Expression Analysis. In: Computer Modeling and Simulation, Sanya, China, 2010: 215 - 218[C8]Dae-Jin Kim, Zeungnam Bien, Kwang-Hyun Park. Fuzzy neural networks (FNN)-based approach for personalized facial expression recognition with novel feature selection method. In: Fuzzy Systems, St.Louis,Missouri,USA,2003: 908 - 913[C9] Wei-feng Liu, Shu-juan Li, Yan-jiang Wang. Automatic Facial Expression Recognition Based on Local Binary Patterns of Local Areas. In: Information Engineering, Taiyuan, Shanxi, China ,2009: 197 - 200[C10] Hao Tang, Hasegawa-Johnson M, Huang T. Non-frontal view facial expression recognition based on ergodic hidden Markov model supervectors.In: Multimedia and Expo (ICME), Singapore ,2010: 1202 - 1207[C11] Yu-Jie Li, Sun-Kyung Kang,Young-Un Kim, Sung-Tae Jung. Development of a facial expression recognition system for the laughter therapy. In: Cybernetics and Intelligent Systems (CIS), Singapore ,2010: 168 - 171[C12] Wei Feng Liu, ZengFu Wang. Facial Expression Recognition Based on Fusion of Multiple Gabor Features. In: Pattern Recognition, HongKong, China, 2006: 536 - 539[C13] Chen Feng-jun, Wang Zhi-liang, Xu Zheng-guang, Xiao Jiang. Facial Expression Recognition Based on Wavelet Energy Distribution Feature and Neural Network Ensemble. In: Intelligent Systems, XiaMen, China, 2009: 122 - 126[C14] P. Kakumanu, N. Bourbakis. A Local-Global Graph Approach for Facial Expression Recognition. In: Tools with Artificial Intelligence, Arlington, Virginia, USA,2006: 685 - 692[C15] Mingwei Huang, Zhen Wang, Zilu Ying. Facial expression recognition using Stochastic Neighbor Embedding and SVMs. In: System Science and Engineering (ICSSE), Macao, China, 2011: 671 - 674[C16] Junhua Li, Li Peng. Feature difference matrix and QNNs for facial expression recognition. In: Control and Decision Conference, Yantai, China, 2008: 3445 - 3449[C17] Yuxiao Hu, Zhihong Zeng, Lijun Yin,Xiaozhou Wei, Jilin Tu, Huang, T.S. A study of non-frontal-view facial expressions recognition. In: Pattern Recognition, Tampa, FL, USA,2008: 1 - 4[C18] Balasubramani A, Kalaivanan K, Karpagalakshmi R.C, Monikandan R. Automatic facial expression recognition system. In: Computing, Communication and Networking, St. Thomas,USA, 2008: 1 - 5[C19] Hui Zhao, Zhiliang Wang, Jihui Men. Facial Complex Expression Recognition Based on Fuzzy Kernel Clustering and Support Vector Machines. In: Natural Computation, Haikou,Hainan,China,2007: 562 - 566[C20] Khanam A, Shafiq M.Z, Akram M.U. Fuzzy Based Facial Expression Recognition. In: Image and Signal Processing, Sanya, Hainan, China,2008: 598 - 602[C21] Sako H, Smith A.V.W. Real-time facial expression recognition based on features' positions and dimensions. In: Pattern Recognition, Vienna,Austria, 1996: 643 - 648 vol.3[C22] Huang M.W, Wang Z.W, Ying Z.L. A novel method of facial expression recognition based on GPLVM Plus SVM. In: Signal Processing (ICSP), Beijing, China, 2010: 916 - 919[C23] Xianxing Wu; Jieyu Zhao; Curvelet feature extraction for face recognition and facial expression recognition. In: Natural Computation (ICNC), Yantai, China, 2010: 1212 - 1216[C24]Xu Q Z, Zhang P Z, Yang L X, et al.A facial expression recognition approach based on novel support vector machine tree. In Proceedings of the 4th International Symposium on Neural Networks, Nanjing, China, 2007: 374-381.[C25] Wang Y B, Ai H Z, Wu B, et al. Real time facial expression recognition with adaboost.In: Proceedings of the 17th International Conference on Pattern Recognition , Cambridge,UK, 2004: 926-929.[C26] Guo G, Dyer C R. Simultaneous feature selection and classifier training via linear programming: a case study for face expression recognition. In: Proceedings of the 2003 IEEE Computer Society Conference on Computer Vision and Pattern Recognition, Madison, W isconsin, USA, 2003,1: 346-352.[C27] Bourel F, Chibelushi C C, Low A A. Robust facial expression recognition using a state-based model of spatially-localised facial dynamics. In: Proceedings of the 5th IEEE International Conference on Automatic Face and Gesture Recognition, Washington, DC, USA, 2002: 113-118·[C28] Buciu I, Kotsia I, Pitas I. Facial expression analysis under partial occlusion. In: Proceedings of the 2005 IEEE International Conference on Acoustics, Speech, and Signal Processing, Philadelphia, PA, USA, 2005,V: 453-456.[C29] ZHAN Yong-zhao,YE Jing-fu,NIU De-jiao,et al.Facial expression recognition based on Gabor wavelet transformation and elastic templates matching. Proc of the 3rd International Conference on Image and Graphics.Washington DC, USA,2004:254-257.[C30] PRASEEDA L V,KUMAR S,VIDYADHARAN D S,et al.Analysis of facial expressions using PCA on half and full faces. Proc of ICALIP2008.2008:1379-1383.[C31]LEE J J,UDDIN M Z,KIM T S.Spatiotemporal human facial expression recognition using Fisher independent component analysis and Hidden Markov model[C]//Proc of the 30th Annual International Conference of IEEE Engineering in Medicine and Biology Society.2008:2546-2549.[C32] LITTLEWORT G,BARTLETT M,FASELL. Dynamics of facial expression extracted automatically from video. In: Proceedings of IEEE Conference on Computer Vision and Pattern Recognition, Workshop on Face Processing inVideo, Washington DC,USA,2006:80-81.[C33] Kotsia I, Nikolaidis N, Pitas I. Facial Expression Recognition in Videos using a Novel Multi-Class Support Vector Machines Variant. In: Acoustics, Speech and Signal Processing, Honolulu, Hawaii, USA, 2007: II-585 - II-588[C34] Ruo Du, Qiang Wu, Xiangjian He, Wenjing Jia, Daming Wei. Facial expression recognition using histogram variances faces. In: Applications of Computer Vision (WACV), Snowbird, Utah, USA, 2009: 1 - 7[C35] Kobayashi H, Tange K, Hara F. Real-time recognition of six basic facial expressions. In: Robot and Human Communication, Tokyo , Japan,1995: 179 - 186[C36] Hao Tang, Huang T.S. 3D facial expression recognition based on properties of line segments connecting facial feature points. In: Automatic Face & Gesture Recognition, Amsterdam, The Netherlands, 2008: 1 - 6[C37] Fengjun Chen, Zhiliang Wang, Zhengguang Xu, Donglin Wang. Research on a method of facial expression recognition.in: Electronic Measurement & Instruments, Beijing,China, 2009: 1-225 - 1-229[C38] Hui Zhao, Tingting Xue, Linfeng Han. Facial complex expression recognition based on Latent DirichletAllocation. In: Natural Computation (ICNC), Yantai, Shandong, China, 2010: 1958 - 1960[C39] Qinzhen Xu, Pinzheng Zhang, Wenjiang Pei, Luxi Yang, Zhenya He. An Automatic Facial Expression Recognition Approach Based on Confusion-Crossed Support Vector Machine Tree. In: Acoustics, Speech and Signal Processing, Honolulu, Hawaii, USA, 2007: I-625 - I-628[C40] Sung Uk Jung, Do Hyoung Kim, Kwang Ho An, Myung Jin Chung. Efficient rectangle feature extraction for real-time facial expression recognition based on AdaBoost.In: Intelligent Robots and Systems, Edmonton,Canada, 2005: 1941 - 1946[C41] Patil K.K, Giripunje S.D, Bajaj P.R. Facial Expression Recognition and Head Tracking in Video Using Gabor Filter .In: Emerging Trends in Engineering and Technology (ICETET), Goa, India, 2010: 152 - 157[C42] Jun Wang, Lijun Yin, Xiaozhou Wei, Yi Sun. 3D Facial Expression Recognition Based on Primitive Surface Feature Distribution.In: Computer Vision and Pattern Recognition, New York, USA,2006: 1399 - 1406[C43] Shi Dongcheng, Jiang Jieqing. The method of facial expression recognition based on DWT-PCA/LDA.IN: Image and Signal Processing (CISP), Yantai,China, 2010: 1970 - 1974[C44] Asthana A, Saragih J, Wagner M, Goecke R. Evaluating AAM fitting methods for facial expression recognition. In: Affective Computing and Intelligent Interaction and Workshops, Amsterdam,Holland, 2009:1-8[C45] Geng Xue, Zhang Youwei. Facial Expression Recognition Based on the Difference of Statistical Features.In: Signal Processing, Guilin, China, 2006[C46] Metaxas D. Facial Features Tracking for Gross Head Movement analysis and Expression Recognition.In: Multimedia Signal Processing, Chania,Crete,GREECE, 2007:2[C47] Xia Mao, YuLi Xue, Zheng Li, Kang Huang, ShanWei Lv. Robust facial expression recognition based on RPCA and AdaBoost.In: Image Analysis for Multimedia Interactive Services, London, UK, 2009: 113 - 116[C48] Kun Lu, Xin Zhang. Facial Expression Recognition from Image Sequences Based on Feature Points and Canonical Correlations.In: Artificial Intelligence and Computational Intelligence (AICI), Sanya,China, 2010: 219 - 223[C49] Peng Zhao-yi, Wen Zhi-qiang, Zhou Yu. Application of Mean Shift Algorithm in Real-Time Facial Expression Recognition.In: Computer Network and Multimedia Technology, Wuhan,China, 2009: 1 - 4[C50] Xu Chao, Feng Zhiyong, Facial Expression Recognition and Synthesis on Affective Emotions Composition.In: Future BioMedical InformationEngineering, Wuhan,China, 2008: 144 - 147[C51] Zi-lu Ying, Lin-bo Cai. Facial Expression Recognition with Marginal Fisher Analysis on Local Binary Patterns.In: Information Science and Engineering (ICISE), Nanjing,China, 2009: 1250 - 1253[C52] Chuang Yu, Yuning Hua, Kun Zhao. The Method of Human Facial Expression Recognition Based on Wavelet Transformation Reducing the Dimension and Improved Fisher Discrimination.In: Intelligent Networks and Intelligent Systems (ICINIS), Shenyang,China, 2010: 43 - 47[C53] Stratou G, Ghosh A, Debevec P, Morency L.-P. Effect of illumination on automatic expression recognition: A novel 3D relightable facial database .In: Automatic Face & Gesture Recognition and Workshops (FG 2011), Santa Barbara, California,USA, 2011: 611 - 618[C54] Jung-Wei Hong, Kai-Tai Song. Facial expression recognition under illumination variation.In: Advanced Robotics and Its Social Impacts, Hsinchu, Taiwan,2007: 1 - 6[C55] Ryan A, Cohn J.F, Lucey S, Saragih J, Lucey P, De la Torre F, Rossi A. Automated Facial Expression Recognition System.In: Security Technology, Zurich, Switzerland, 2009: 172 - 177[C56] Gokturk S.B, Bouguet J.-Y, Tomasi C, Girod B. Model-based face tracking for view-independent facial expression recognition.In: Automatic Face and Gesture Recognition, Washington, D.C., USA, 2002: 287 - 293[C57] Guo S.M, Pan Y.A, Liao Y.C, Hsu C.Y, Tsai J.S.H, Chang C.I. A Key Frame Selection-Based Facial Expression Recognition System.In: Innovative Computing, Information and Control, Beijing,China, 2006: 341 - 344[C58] Ying Zilu, Li Jingwen, Zhang Youwei. Facial expression recognition based on two dimensional feature extraction.In: Signal Processing, Leipzig, Germany, 2008: 1440 - 1444[C59] Fengjun Chen, Zhiliang Wang, Zhengguang Xu, Jiang Xiao, Guojiang Wang. Facial Expression Recognition Using Wavelet Transform and Neural Network Ensemble.In: Intelligent Information Technology Application, Shanghai,China,2008: 871 - 875[C60] Chuan-Yu Chang, Yan-Chiang Huang, Chi-Lu Yang. Personalized Facial Expression Recognition in Color Image.In: Innovative Computing, Information and Control (ICICIC), Kaohsiung,Taiwan, 2009: 1164 - 1167 [C61] Bourel F, Chibelushi C.C, Low A.A. Robust facial expression recognition using a state-based model of spatially-localised facial dynamics. In: Automatic Face and Gesture Recognition, Washington, D.C., USA, 2002: 106 - 111[C62] Chen Juanjuan, Zhao Zheng, Sun Han, Zhang Gang. Facial expression recognition based on PCA reconstruction.In: Computer Science and Education (ICCSE), Hefei,China, 2010: 195 - 198[C63] Guotai Jiang, Xuemin Song, Fuhui Zheng, Peipei Wang, Omer A.M.Facial Expression Recognition Using Thermal Image.In: Engineering in Medicine and Biology Society, Shanghai,China, 2005: 631 - 633[C64] Zhan Yong-zhao, Ye Jing-fu, Niu De-jiao, Cao Peng. Facial expression recognition based on Gabor wavelet transformation and elastic templates matching.In: Image and Graphics, Hongkong,China, 2004: 254 - 257[C65] Ying Zilu, Zhang Guoyi. Facial Expression Recognition Based on NMF and SVM. In: Information Technology and Applications, Chengdu,China, 2009: 612 - 615[C66] Xinghua Sun, Hongxia Xu, Chunxia Zhao, Jingyu Yang. Facial expression recognition based on histogram sequence of local Gabor binary patterns. In: Cybernetics and Intelligent Systems, Chengdu,China, 2008: 158 - 163[C67] Zisheng Li, Jun-ichi Imai, Kaneko M. Facial-component-based bag of words and PHOG descriptor for facial expression recognition.In: Systems, Man and Cybernetics, San Antonio,TX,USA,2009: 1353 - 1358[C68] Chuan-Yu Chang, Yan-Chiang Huang. Personalized facial expression recognition in indoor environments.In: Neural Networks (IJCNN), Barcelona, Spain, 2010: 1 - 8[C69] Ying Zilu, Fang Xieyan. Combining LBP and Adaboost for facial expression recognition.In: Signal Processing, Leipzig, Germany, 2008: 1461 - 1464[C70] Peng Yang, Qingshan Liu, Metaxas, D.N. RankBoost with l1 regularization for facial expression recognition and intensity estimation.In: Computer Vision, Kyoto,Japan, 2009: 1018 - 1025[C71] Patil R.A, Sahula V, Mandal A.S. Automatic recognition of facial expressions in image sequences: A review.In: Industrial and Information Systems (ICIIS), Mangalore, India, 2010: 408 - 413[C72] Iraj Hosseini, Nasim Shams, Pooyan Amini, Mohammad S. Sadri, Masih Rahmaty, Sara Rahmaty. Facial Expression Recognition using Wavelet-Based Salient Points and Subspace Analysis Methods.In: Electrical and Computer Engineering, Ottawa, Canada, 2006: 1992 - 1995[C73][C74][C75][C76][C77][C78][C79]3、英文期刊文章[J1] Aleksic P.S., Katsaggelos A.K. Automatic facial expression recognition using facial animation parameters and multistream HMMs.IEEE Transactions on Information Forensics and Security, 2006, 1(1):3-11 [J2] Kotsia I,Pitas I. Facial Expression Recognition in Image Sequences Using Geometric Deformation Features and Support Vector Machines. IEEE Transactions on Image Processing, 2007, 16(1):172 – 187[J3] Mpiperis I, Malassiotis S, Strintzis M.G. Bilinear Models for 3-D Face and Facial Expression Recognition. IEEE Transactions on Information Forensics and Security, 2008,3(3) : 498 - 511[J4] Sung J, Kim D. Pose-Robust Facial Expression Recognition Using View-Based 2D+3D AAM. IEEE Transactions on Systems and Humans, 2008 , 38 (4): 852 - 866[J5]Yeasin M, Bullot B, Sharma R. Recognition of facial expressions and measurement of levels of interest from video. IEEE Transactions on Multimedia, 2006, 8(3): 500 - 508[J6] Wenming Zheng, Xiaoyan Zhou, Cairong Zou, Li Zhao. Facial expression recognition using kernel canonical correlation analysis (KCCA). IEEE Transactions on Neural Networks, 2006, 17(1): 233 - 238 [J7]Pantic M, Patras I. Dynamics of facial expression: recognition of facial actions and their temporal segments from face profile image sequences. IEEE Transactions on Systems, Man, and Cybernetics, Part B: Cybernetics, 2006, 36(2): 433 - 449[J8] Mingli Song, Dacheng Tao, Zicheng Liu, Xuelong Li, Mengchu Zhou. Image Ratio Features for Facial Expression Recognition Application. IEEE Transactions on Systems, Man, and Cybernetics, Part B: Cybernetics, 2010, 40(3): 779 - 788[J9] Dae Jin Kim, Zeungnam Bien. Design of “Personalized” Classifier Using Soft Computing Techniques for “Personalized” Facial Expression Recognition. IEEE Transactions on Fuzzy Systems, 2008, 16(4): 874 - 885 [J10] Uddin M.Z, Lee J.J, Kim T.-S. An enhanced independent component-based human facial expression recognition from video. IEEE Transactions on Consumer Electronics, 2009, 55(4): 2216 - 2224[J11] Ruicong Zhi, Flierl M, Ruan Q, Kleijn W.B. Graph-Preserving Sparse Nonnegative Matrix Factorization With Application to Facial Expression Recognition. IEEE Transactions on Systems, Man, and Cybernetics, Part B:Cybernetics, 2011, 41(1): 38 - 52[J12] Chibelushi C.C, Bourel F. Hierarchical multistream recognition of facial expressions. IEE Proceedings - Vision, Image and Signal Processing, 2004, 151(4): 307 - 313[J13] Yongsheng Gao, Leung M.K.H, Siu Cheung Hui, Tananda M.W. Facial expression recognition from line-based caricatures. IEEE Transactions on Systems, Man and Cybernetics, Part A: Systems and Humans, 2003, 33(3): 407 - 412[J14] Ma L, Khorasani K. Facial expression recognition using constructive feedforward neural networks. IEEE Transactions on Systems, Man, and Cybernetics, Part B: Cybernetics, 2004, 34(3): 1588 - 1595[J15] Essa I.A, Pentland A.P. Coding, analysis, interpretation, and recognition of facial expressions. IEEE Transactions on Pattern Analysis and Machine Intelligence, 1997, 19(7): 757 - 763[J16] Anderson K, McOwan P.W. A real-time automated system for the recognition of human facial expressions. IEEE Transactions on Systems, Man, and Cybernetics, Part B: Cybernetics, 2006, 36(1): 96 - 105[J17] Soyel H, Demirel H. Facial expression recognition based on discriminative scale invariant feature transform. Electronics Letters 2010, 46(5): 343 - 345[J18] Fei Cheng, Jiangsheng Yu, Huilin Xiong. Facial Expression Recognition in JAFFE Dataset Based on Gaussian Process Classification. IEEE Transactions on Neural Networks, 2010, 21(10): 1685 – 1690[J19] Shangfei Wang, Zhilei Liu, Siliang Lv, Yanpeng Lv, Guobing Wu, Peng Peng, Fei Chen, Xufa Wang. A Natural Visible and Infrared Facial Expression Database for Expression Recognition and Emotion Inference. IEEE Transactions on Multimedia, 2010, 12(7): 682 - 691[J20] Lajevardi S.M, Hussain Z.M. Novel higher-order local autocorrelation-like feature extraction methodology for facial expression recognition. IET Image Processing, 2010, 4(2): 114 - 119[J21] Yizhen Huang, Ying Li, Na Fan. Robust Symbolic Dual-View Facial Expression Recognition With Skin Wrinkles: Local Versus Global Approach. IEEE Transactions on Multimedia, 2010, 12(6): 536 - 543[J22] Lu H.-C, Huang Y.-J, Chen Y.-W. Real-time facial expression recognition based on pixel-pattern-based texture feature. Electronics Letters 2007, 43(17): 916 - 918[J23]Zhang L, Tjondronegoro D. Facial Expression Recognition Using Facial Movement Features. IEEE Transactions on Affective Computing, 2011, pp(99): 1[J24] Zafeiriou S, Pitas I. Discriminant Graph Structures for Facial Expression Recognition. Multimedia, IEEE Transactions on 2008,10(8): 1528 - 1540[J25]Oliveira L, Mansano M, Koerich A, de Souza Britto Jr. A. Selecting 2DPCA Coefficients for Face and Facial Expression Recognition. Computingin Science & Engineering, 2011, pp(99): 1[J26] Chang K.I, Bowyer W, Flynn P.J. Multiple Nose Region Matching for 3D Face Recognition under Varying Facial Expression. Pattern Analysis and Machine Intelligence, IEEE Transactions on2006, 28(10): 1695 - 1700 [J27] Kakadiaris I.A, Passalis G, Toderici G, Murtuza M.N, Yunliang Lu, Karampatziakis N, Theoharis T. Three-Dimensional Face Recognition in the Presence of Facial Expressions: An Annotated Deformable Model Approach.IEEE Transactions on Pattern Analysis and Machine Intelligence, 2007, 29(4): 640 - 649[J28] Guoying Zhao, Pietikainen M. Dynamic Texture Recognition Using Local Binary Patterns with an Application to Facial Expressions. IEEE Transactions on Pattern Analysis and Machine Intelligence, 2007, 29(6): 915 - 928[J29] Chakraborty A, Konar A, Chakraborty U.K, Chatterjee A. Emotion Recognition From Facial Expressions and Its Control Using Fuzzy Logic. IEEE Transactions on Systems, Man and Cybernetics, Part A: Systems and Humans, 2009, 39(4): 726 - 743[J30] Pantic M, RothkrantzL J.M. Facial action recognition for facial expression analysis from static face images. IEEE Transactions on Systems, Man, and Cybernetics, Part B: Cybernetics, 2004, 34(3): 1449 - 1461 [J31] Calix R.A, Mallepudi S.A, Bin Chen, Knapp G.M. Emotion Recognition in Text for 3-D Facial Expression Rendering. IEEE Transactions on Multimedia, 2010, 12(6): 544 - 551[J32]Kotsia I, Pitas I, Zafeiriou S, Zafeiriou S. Novel Multiclass Classifiers Based on the Minimization of the Within-Class Variance. IEEE Transactions on Neural Networks, 2009, 20(1): 14 - 34[J33]Cohen I, Cozman F.G, Sebe N, Cirelo M.C, Huang T.S. Semisupervised learning of classifiers: theory, algorithms, and their application to human-computer interaction. IEEE Transactions on Pattern Analysis and Machine Intelligence, 2004, 26(12): 1553 - 1566[J34] Zafeiriou S. Discriminant Nonnegative Tensor Factorization Algorithms. IEEE Transactions on Neural Networks, 2009, 20(2): 217 - 235 [J35] Zafeiriou S, Petrou M. Nonlinear Non-Negative Component Analysis Algorithms. IEEE Transactions on Image Processing, 2010, 19(4): 1050 - 1066[J36] Kotsia I, Zafeiriou S, Pitas I. A Novel Discriminant Non-Negative Matrix Factorization Algorithm With Applications to Facial Image Characterization Problems. IEEE Transactions on Information Forensics and Security, 2007, 2(3): 588 - 595[J37] Irene Kotsia, Stefanos Zafeiriou, Ioannis Pitas. Texture and shape information fusion for facial expression and facial action unit recognition. Pattern Recognition, 2008, 41(3): 833-851[J38]Wenfei Gu, Cheng Xiang, Y.V. Venkatesh, Dong Huang, Hai Lin. Facial expression recognition using radial encoding of local Gabor features andclassifier synthesis.Pattern Recognition, In Press, Corrected Proof, Available online 27 May 2011[J39] F Dornaika, E Lazkano, B Sierra. Improving dynamic facial expression recognition with feature subset selection.Pattern Recognition Letters, 2011, 32(5): 740-748[J40] Te-Hsun Wang, Jenn-Jier James Lien. Facial expression recognition system based on rigid and non-rigid motion separation and 3D pose estimation. Pattern Recognition, 2009, 42(5): 962-977[J41] Hyung-Soo Lee, Daijin Kim.Expression-invariant face recognition by facial expression transformations.Pattern Recognition Letters, 2008, 29(13): 1797-1805[J42] Guoying Zhao, Matti Pietikäinen. Boosted multi-resolution spatiotemporal descriptors for facial expression recognition. Pattern Recognition Letters, 2009, 30(12): 1117-1127[J43] Xudong Xie, Kin-Man Lam. Facial expression recognition based on shape and texture. Pattern Recognition, 2009, 42(5):1003-1011[J44] Peng Yang, Qingshan Liu, Dimitris N. Metaxas Boosting encoded dynamic features for facial expression recognition. Pattern Recognition Letters, 2009,30(2): 132-139[J45] Sungsoo Park, Daijin Kim. Subtle facial expression recognition using motion magnification. Pattern Recognition Letters, 2009, 30(7): 708-716[J46] Chathura R. De Silva, Surendra Ranganath, Liyanage C. De Silva. Cloud basis function neural network: A modified RBF network architecture for holistic facial expression recognition.Pattern Recognition, 2008, 41(4): 1241-1253[J47] Do Hyoung Kim, Sung Uk Jung, Myung Jin Chung. Extension of cascaded simple feature based face detection to facial expression recognition.Pattern Recognition Letters, 2008, 29(11): 1621-1631[J48] Y. Zhu, L.C. De Silva, C.C. Ko. Using moment invariants and HMM in facial expression recognition. Pattern Recognition Letters, 2002, 23(1-3): 83-91[J49] Jun Wang, Lijun Yin. Static topographic modeling for facial expression recognition and puter Vision and Image Understanding, 2007, 108(1-2): 19-34[J50] Caifeng Shan, Shaogang Gong, Peter W. McOwan. Facial expression recognition based on Local Binary Patterns: A comprehensive study. Image and Vision Computing, 2009, 27(6): 803-816[J51] Xue-wen Chen, Thomas Huang. Facial expression recognition: A clustering-based approach. Pattern Recognition Letters, 2003, 24(9-10): 1295-1302[J52] Irene Kotsia, Ioan Buciu, Ioannis Pitas.An analysis of facial expression recognition under partial facial image occlusion. Image and Vision Computing, 2008, 26(7): 1052-1067[J53] Shuai Liu, Qiuqi Ruan. Orthogonal Tensor Neighborhood Preserving Embedding for facial expression recognition.Pattern Recognition, 2011, 44(7):1497-1513[J54] Eszter Székely, Henning Tiemeier, Lidia R. Arends, Vincent W.V. Jaddoe, Albert Hofman, Frank C. Verhulst, Catherine M. Herba. Recognition of Facial Expressions of Emotions by 3-Year-Olds. Emotion, 2011, 11(2): 425-435[J55] Kathleen M. Corcoran, Sheila R. Woody, David F. Tolin.Recognition of facial expressions in obsessive–compulsive disorder. Journal of Anxiety Disorders, 2008, 22(1): 56-66[J56] Bouchra Abboud, Franck Davoine, MôDang. Facial expression recognition and synthesis based on an appearance model. Signal Processing: Image Communication, 2004, 19(8): 723-740[J57] Teng Sha, Mingli Song, Jiajun Bu, Chun Chen, Dacheng Tao. Feature level analysis for 3D facial expression recognition. Neurocomputing, 2011, 74(12-13) :2135-2141[J58] S. Moore, R. Bowden. Local binary patterns for multi-view facial expression recognition. Computer Vision and Image Understanding, 2011, 15(4):541-558[J59] Rui Xiao, Qijun Zhao, David Zhang, Pengfei Shi. Facial expression recognition on multiple manifolds.Pattern Recognition, 2011, 44(1):107-116[J60] Shyi-Chyi Cheng, Ming-Yao Chen, Hong-Yi Chang, Tzu-Chuan Chou. Semantic-based facial expression recognition using analytical hierarchy process.Expert Systems with Applications, 2007, 33(1): 86-95[J71] Carlos E. Thomaz, Duncan F. Gillies, Raul Q. Feitosa. Using mixture covariance matrices to improve face and facial expression recognitions. Pattern Recognition Letters, 2003, 24(13): 2159-2165[J72]Wen G,Bo C,Shan Shi-guang,et al. The CAS-PEAL large-scale Chinese face database and baseline evaluations.IEEE Transactions on Systems,Man and Cybernetics,part A:Systems and Hu-mans,2008,38(1):149-161. [J73] Yongsheng Gao,Leung ,M.K.H. Face recognition using line edge map.IEEE Transactions on Pattern Analysis and Machine Intelligence,2002,24:764-779.[J74] Hanouz M,Kittler J,Kamarainen J K,et al. Feature-based affine-invariant localization of faces.IEEE Transactions on Pat-tern Analysis and Machine Intelligence,2005,27:1490-1495.[J75] WISKOTT L,FELLOUS J M,KRUGER N,et al.Face recognition by elastic bunch graph matching.IEEE Trans on Pattern Analysis and Machine Intelligence,1997,19(7):775-779.[J76] Belhumeur P.N, Hespanha J.P, Kriegman D.J. Eigenfaces vs. fischerfaces: recognition using class specific linear projection.IEEE Trans on Pattern Analysis and Machine Intelligence,1997,15(7):711-720 [J77] MA L,KHORASANI K.Facial Expression Recognition Using ConstructiveFeedforward Neural Networks. IEEE Transactions on Systems, Man and Cybernetics, Part B,2007,34(3):1588-1595.[J78][J79][J80][J81][J82][J83][J84][J85][J86][J87][J88][J89][J90]。
学术英语课后答案 unit1

学术英语理工教师手册Unit 1 Choosing a TopicI Teaching ObjectivesIn this unit , you will learn how to:1.choose a particular topic for your research2.formulate a research question3.write a working title for your research essay4.enhance your language skills related with reading and listening materials presented in this unit II. Teaching Procedures1.Deciding on a topicTask 1Answers may vary.Task 21 No, because they all seem like a subject rather than a topic, a subject which cannot be addressed even by a whole book, let alone by a1500-wordessay.2Each of them can be broken down into various and more specific aspects. For example, cancer can be classified into breast cancer, lung cancer, liver cancer and so on. Breast cancer can have such specific topics for research as causes for breast cancer, effects of breast cancer and prevention or diagnosis of breast cancer.3 Actually the topics of each field are endless. Take breast cancer for example, we can have the topics like:Why Women Suffer from Breast Cancer More Than Men?A New Way to Find Breast TumorsSome Risks of Getting Breast Cancer in Daily LifeBreast Cancer and Its Direct Biological ImpactBreast Cancer—the Symptoms & DiagnosisBreastfeeding and Breast CancerTask 31 Text 1 illustrates how hackers or unauthorized users use one way or another to get inside a computer, while Text2 describes the various electronic threats a computer may face.2 Both focus on the vulnerability of a computer.3 Text 1 analyzes the ways of computer hackers, while Text 2 describes security problems of a computer.4 Text 1: The way hackers “get inside” a computerText 2: Electronic threats a computer facesYes, I think they are interesting, important, manageable and adequate.Task 41Lecture1:Ten Commandments of Computer EthicsLecture 2:How to Deal with Computer HackersLecture 3:How I Begin to Develop Computer Applications2Answersmay vary.Task 5Answers may vary.2 Formulating a research questionTask 1Text 3Research question 1: How many types of cloud services are there and what are they? Research question 2: What is green computing?Research question 3: What are advantages of the cloud computing?Text 4Research question 1: What is the Web 3.0?Research question 2: What are advantages and disadvantages of the cloud computing? Research question 3: What security benefits can the cloud computing provide?Task 22 Topic2: Threats of Artificial IntelligenceResearch questions:1) What are the threats of artificial intelligence?2) How can human beings control those threats?3) What are the difficulties to control those threats?3 Topic3: The Potentials of NanotechnologyResearch questions:1) What are its potentials in medicine?2) What are its potentials in space exploration?3) What are its potentials in communications?4 Topic4: Global Warming and Its EffectsResearch questions:1) How does it affect the pattern of climates?2) How does it affect economic activities?3) How does it affect human behavior?Task 3Answers may vary.3 Writing a working titleTask 1Answers may vary.Task 21 Lecture 4 is about the security problems of cloud computing, while Lecture 5 is about the definition and nature of cloud computing, hence it is more elementary than Lecture 4.2 The four all focus on cloud computing. Although Lecture 4 and Text 4 address the same topic, the former is less optimistic while the latter has more confidence in the security of cloud computing. Text3 illustrates the various advantages of cloud computing.3 Lecture 4: Cloud Computing SecurityLecture 5: What Is Cloud Computing?Task 3Answers may vary.4 Enhancing your academic languageReading: Text 11.Match the words with their definitions.1g 2a 3e 4b 5c 6d 7j 8f 9h 10i2. Complete the following expressions or sentences by using the target words listed below with the help of the Chinese in brackets. Change the form if necessary.1 symbolic 2distributed 3site 4complex 5identify6fairly 7straightforward 8capability 9target 10attempt11process 12parameter 13interpretation 14technical15range 16exploit 17networking 18involve19 instance 20specification 21accompany 22predictable 23profile3. Read the sentences in the box. Pay attention to the parts in bold.Now complete the paragraph by translating the Chinese in brackets. You may refer to the expressions and the sentence patterns listed above.ranging from(从……到)arise from some misunderstandings(来自于对……误解)leaves a lot of problems unsolved(留下很多问题没有得到解决)opens a path for(打开了通道)requires a different frame of mind(需要有新的思想)4.Translate the following sentences from Text 1 into Chinese.1) 有些人声称黑客是那些超越知识疆界而不造成危害的好人(或即使造成危害,但并非故意而为),而“骇客”才是真正的坏人。
八年级上册英语笔记
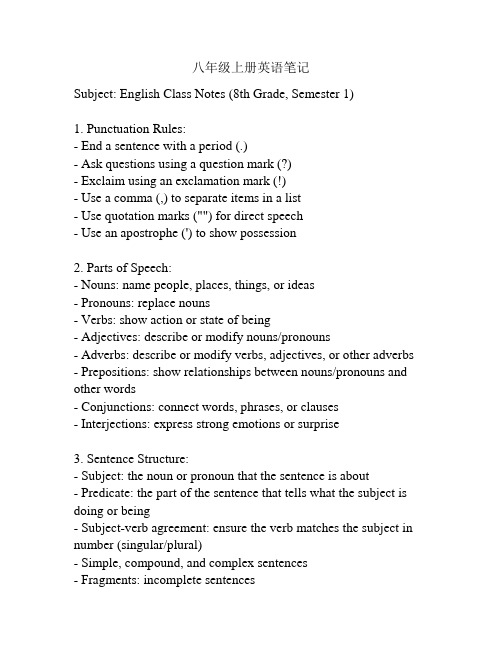
八年级上册英语笔记Subject: English Class Notes (8th Grade, Semester 1)1. Punctuation Rules:- End a sentence with a period (.)- Ask questions using a question mark (?)- Exclaim using an exclamation mark (!)- Use a comma (,) to separate items in a list- Use quotation marks ("") for direct speech- Use an apostrophe (') to show possession2. Parts of Speech:- Nouns: name people, places, things, or ideas- Pronouns: replace nouns- Verbs: show action or state of being- Adjectives: describe or modify nouns/pronouns- Adverbs: describe or modify verbs, adjectives, or other adverbs - Prepositions: show relationships between nouns/pronouns and other words- Conjunctions: connect words, phrases, or clauses- Interjections: express strong emotions or surprise3. Sentence Structure:- Subject: the noun or pronoun that the sentence is about- Predicate: the part of the sentence that tells what the subject is doing or being- Subject-verb agreement: ensure the verb matches the subject in number (singular/plural)- Simple, compound, and complex sentences- Fragments: incomplete sentences- Run-Ons: two or more sentences combined without proper punctuation4. Reading Comprehension Strategies:- Preview: skim the text to get a general idea- Predict: make predictions based on titles, headings, and illustrations- Question: generate questions before, during, and after reading- Visualize: create mental images while reading- Connect: relate the text to personal experiences or prior knowledge- Infer: make logical deductions based on clues within the text- Summarize: briefly retell the main ideas in your own words5. Vocabulary Building Techniques:- Context clues: use surrounding words to determine meaning- Word families: identify related words (root words, prefixes, suffixes)- Dictionary: consult a dictionary for precise definitions- Synonyms and antonyms: find words with similar or opposite meanings- Note-taking: write down new words and their definitions- Reading: expose yourself to a variety of texts to encounter new vocabulary- Flashcards: create flashcards to review and memorize new words Note: These are just some of the key points covered in the 8th-grade English class. It is essential to review and practice these concepts regularly to strengthen language skills.Certainly! Here are some additional topics that may be covered in an 8th-grade Englishclass:6. Literary Devices:- Simile: a comparison using "like" or "as"- Metaphor: a direct comparison without using "like" or "as"- Personification: giving human qualities to non-human objects- Hyperbole: exaggerated statements or claims- Alliteration: the repetition of initial consonant sounds- Onomatopoeia: words that imitate sounds- Imagery: the use of vivid language to create mental images- Symbolism: using an object or action to represent something else 7. Reading Strategies:- Active reading: engaging with the text by highlighting, underlining, and taking notes- Annotation: marking important information or making comments in the margins- Making predictions: using context clues to anticipate what will happen next- Identifying main ideas: understanding the central themes or key points in a text- Analyzing characters: examining their traits, motivations, and development- Interpreting figurative language: understanding the deeper meaning behind metaphors or similes- Analyzing author's purpose: determining why the author wrote the text and their intended message8. Writing Skills:- Thesis statement: a clear and concise statement that expresses themain argument or point of an essay- Topic sentences: the main idea of each paragraph, supporting the thesis statement- Paragraph structure: a topic sentence, supporting details, and a concluding sentence- Transitions: words or phrases that connect ideas and create flow between sentences and paragraphs- Descriptive writing: using sensory details to paint a vivid picture for the reader- Persuasive writing: presenting arguments and evidence to convince the reader of a particular viewpoint- Narrative writing: telling a story with characters, setting, conflict, and resolution9. Grammar and Usage:- Subject-verb agreement: ensuring that the subject and verb match in number and tense- Pronoun-antecedent agreement: making sure pronouns agree in number and gender with the nouns they replace- Verb tenses: understanding the different forms of verbs and when to use them- Active and passive voice: identifying who is performing the action in a sentence- Parallel structure: using consistent grammatical structures within a sentence or paragraph- Sentence variety: using a combination of sentence types (simple, compound, complex) to create impact and flow- Sentence combining: joining two or more sentences into one to avoid repetitive or choppy writing10. Research Skills:- Conducting research: using reliable sources (books, websites, databases) to gather information- Note-taking: summarizing and organizing information while conducting research- Paraphrasing and citing sources: using your own words to express information and giving credit to the original source- Outlining: creating an organized structure for an essay or report before writing- Plagiarism awareness: understanding the consequences of using someone else's work without proper citationRemember, this is just a broad overview of topics that may be covered in an 8th-grade English class. Each curriculum may vary, and teachers may have additional areas of focus. It's important to actively participate, ask questions, and practice the skills and concepts learned in order to improve in English language and literature.。
Adobe Acrobat SDK 开发者指南说明书
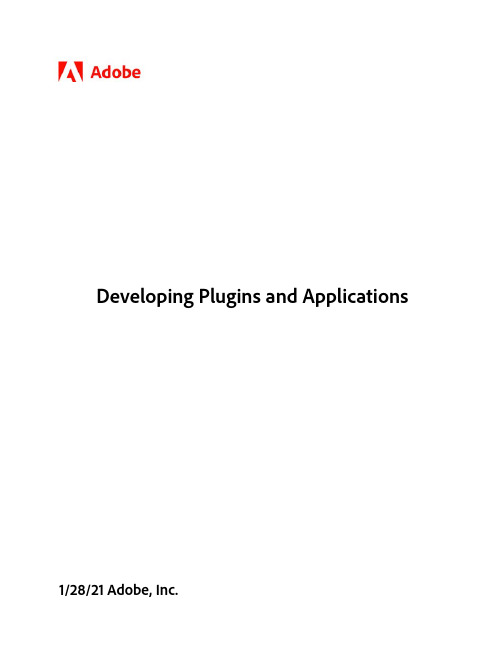
This guide is governed by the Adobe Acrobat SDK License Agreement and may be used or copied only in accordance with the terms of this agreement. Except as permitted by any such agreement, no part of this guide may be reproduced, stored in a retrieval system, or transmitted, in any form or by any means, electronic, mechanical, recording, or otherwise, without the prior written permission of Adobe. Please note that the content in this guide is protected under copyright law.
译林版高中英语选择性必修第一册精品课件 UNIT 4 Section A
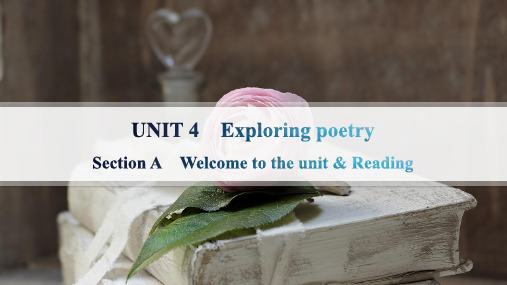
第三步 深读课文 融会提能 Activity 6 课文语篇填空
Poetry is a combination of “sound” and “sense”.More than any other type of literature,it usually implies a deeper meaning 1. beyond the words on the page.So,how to reveal this hidden dimension?
√D.A burning desire to explore the poetry is what you need.
4.Which of the following will the lecture agree with? A.Readers should keep thinking logically.
Haiku is a Japanese form of poetry that consists of 17 syllables. It has a format6 of three lines, containing 5, 7, and 5 syllables respectively. It is not a traditional form of English poetry, but is very popular with English writers. It is easy to write and,like the cinquain, can give a clear picture and create a special feeling using very few words. The haiku poem (E) on the right is a translation from Japanese, which shows a moment in the life of a delicate7 butterfly.
- 1、下载文档前请自行甄别文档内容的完整性,平台不提供额外的编辑、内容补充、找答案等附加服务。
- 2、"仅部分预览"的文档,不可在线预览部分如存在完整性等问题,可反馈申请退款(可完整预览的文档不适用该条件!)。
- 3、如文档侵犯您的权益,请联系客服反馈,我们会尽快为您处理(人工客服工作时间:9:00-18:30)。
Interpreting Face Images using Active Appearance ModelsG.J.Edwards,C.J.Taylor and T.F.CootesWolfson Image Analysis Unit,Department of Medical Biophysics,University of Manchester,Manchester M139PT,U.K.gje@AbstractWe demonstrate a fast,robust method of interpreting face images using an Active Appearance Model(AAM).An AAM contains a statistical model of shape and grey-level appear-ance which can generalise to almost any face.Matching to an image involvesfinding model parameters which min-imise the difference between the image and a synthesised face.We observe that displacing each model parameter from the correct value induces a particular pattern in the residuals.In a training phase,the AAM learns a linear model of the correlation between parameter displacements and the induced residuals.During search it measures the residuals and uses this model to correct the current param-eters,leading to a betterfit.A good overall match is ob-tained in a few iterations,even from poor starting estimates. We describe the technique in detail and show it matching to new face images.1IntroductionThere is currently a great deal of interest in model-based approaches to the interpretation of face images[9][4][7] [6][3].The attractions are two-fold:robust interpretation is achieved by constraining solutions to be face-like;and the ability to‘explain’an image in terms of a set of model pa-rameters provides a natural interface to applications of face recognition.In order to achieve these objectives,the face model should be as complete as possible-able to synthe-sise a very close approximation to any face image which will need to be interpreted.Although model-based methods have proved quite success-ful,none of the existing methods uses a full,photo-realistic model and attempts to match it directly by minimising the difference between the model-synthesised face and the im-age under interpretation.Although suitable photo-realistic models exist,(e.g.Edwards et al[3]),they typically involve a very large number of parameters(50-100)in order to deal with the variability due to differences between individuals, and changes in pose,expression,and lighting.Direct opti-misation over such a high dimensional space seems daunt-ing.In this paper,we show that a direct optimisation approach is feasible and leads to an algorithm which is rapid,accu-rate,and robust.In our proposed method,we do not attempt to solve a general optimisation each time we wish tofit the model to a new face image.Instead,we exploit the fact the optimisation problem is similar each time-we can learn these similarities off-line.This allows us tofind rapid direc-tions of convergence even though the search space has very high dimensionality.In this paper we discuss the idea of im-age interpretation by synthesis and describe previous related work.In section2we explain how we build compact mod-els of face appearance which are capable of generating syn-thetic examples of any individual,showing any expression, under a range of poses,and under any lighting conditions. We then describe how we rapidly generate face hypotheses giving possible locations and approximate scales.In sec-tion4we describe our Active Appearance Model algorithm in detail and in5demonstrate itsperformance.In recent years many model-based approaches to the in-terpretation of face images have been described.One moti-vation is to achieve robust performance by using the model to constrain solutions to be face-like.A model also provides the basis for a broad range of applications by’explaining’the appearance of a given face image in terms of a compact set of model parameters.These parameters are often used to characterize the identity,pose or expression of a face.In or-der to interpret a new image,an efficient method offinding the best match between image and model is required.Models which can synthesise full faces have been de-scribed by several authors.Turk and Pentland[9]devel-oped the‘eigenface’approach.However,this is not robust to shape changes in faces,and does not deal well with vari-ability in pose and expression.Ezzat and Poggio[4]synthe-sise new views of a face from a set of example views,but cannot generalize to unseen faces.Nastar et al[7]use a3D model of the grey-level surface,allowing full synthesis of shape and appearance.However the proposed search algo-rithm is likely to get stuck in local minima so is not robust. Lanitis et al[6]used separate models of shape and the lo-cal grey-level appearance of a‘shape-normalised’face.Ed-wards et al[3]extended this by also modelling the correla-tions between shape and grey-level appearance.Fitting such models to new images is achieved in most cases by minimis-ing an error measure between the predicted appearance and the image,and is typically time consuming when the full model is used.Edwards et al[3]follow Lanitis et al[6]in using an Active Shape Model tofind the face shape quickly. They then warp the image into a normalised frame andfit a model of the grey-level appearance to the whole face in this frame.This is effective,but as the ASM search does not use all the information available,it is not always robust. Our new approach can be seen as an extension of this idea, using all the information in a full appearance model tofit to the image.Our aim is take appearance models similar those described by Edwards et al[3]andfit them directly to face images.These models are both specific and detailed, allowing a complete description of a new face.By using all the information available,we expect to obtain robust performance.This approach involves a very high dimen-sional search problem,but we show below that an efficient method of solution exists.Efficient stochastic methods of fitting rigid models to images have been described by Vi-ola and Wells[10]and Matas et al[5].We adopt a similar strategy for generating face hypotheses when we have no initial knowledge of where the face may lie in an image. Given a hypothesis,we must refine it to obtain a betterfit to the image.This involves estimating both the shape and the grey-level appearance of the face.Covell[2]demonstrated that the parameters of an eigen-feature model can be used to drive shape model points to the correct place.Similarly, Black and Yacoob[1]used local,hand-crafted models of imageflow to track facial features.We use a generalisation of these ideas,using a model which relates the match resid-ual to the error in the appearance parameters.In a parallel development Sclaroff and Isidoro[8],have demonstrated‘Active Blobs’for tracking.The approach is broadly similar in that they use image differences to drive tracking,learning the relationship between image error and parameter offset in an off-line processing stage.The main difference is that Active Blobs are derived from a single ex-ample,whereas Active Appearance Models use a training set of examples.Sclaroff and Isidoro are primarily inter-ested in tracking and use an initial frame as a template.Theyassume that the object being tracked may be non-rigid,or that projective effects may render it so in the image plane, and allow deformations consistent with low energy mesh distortion(derived using a Finite Element method).A sim-ple polynomial model is used to allow changes in intensity across the object.Active Appearance Models learn what are valid shape and intensity variations from their training set.Sclaroff and Isidoro suggest applying a robust kernel to the image differences,an idea we will use in later work.Also,since annotating the training set is the most time con-suming part of building an AAM,the Active Blob approach may be useful for’bootstrapping’from thefirst example.2Modelling Facial AppearanceIn this section we outline how our facial appearance models were generated.The approach follows that de-scribed in Edwards et al[3]to which the reader is directed for details.Some familiarity with the basic approach is re-quired to understand our new Active Appearance Model al-gorithm.The models were generated by combining a model of face shape variation with a model of the appearance varia-tions of a shape-normalised face.The models were trained on400face images,each labelled with122landmark points representing the positions of key features.The shape model was generated by representing each set of landmarks as avector,and applying a principal component analysis (PCA)to the data.Any example can then be approximatedusing:(1)where is the meanshape,is a set of orthogonal modes of variationand is a set of shape parameters.If each example image is warped so that its control points match the mean shape(using a triangulation algorithm) we can sample the grey levelinformation from this shape-normalised face patch.By applying PCA to this data we obtain a similarmodel:(2)The shape and appearance of any example can thus be summarised by thevectorsand.Since there are correlations between the shape and grey-level variations, we apply a further PCA to the concatenated vectors,to obtain a combined model of theform:(3)(4) 2where is a vector of appearance parameters control-ling both the shape and grey-levels of the model,andand map the valueof to changes in the shape and shape-normalised grey-level data.A face can be synthe-sized for agiven by generating the shape-free grey-level image from thevector and warping it using the control points describedby (see [3]for details).The 400examples lead to 23shapeparameters,,114grey-levelparameters,,but only 80combined appearance modelparameters,being required to explain 98%of the observed variation.Figure 1shows an unseen example image alongside the model reconstruction of the face patch (overlaid on the orig-inalimage).3Generating Face HypothesesWe adopt a two-stage strategy for matching the ap-pearance model to face images.The first step is to find an approximate match using a simple and rapid approach.We assume no initial knowledge of where the face may lie in the image,or of it’s scale and orientation.A simple eigen-face model[9]is used for this stage of the location.A correlationscore,,between the eigen-face representation of the imagedata,and the imageitself,can be calculated at various scales,positions andorientations:(5)Although in principle the image could be searched ex-haustively,it is much more efficient to use a stochastic scheme similar to that of Matas et al [5].We sub-sample both the model and image to calculate the correlation scoreusing only a small fraction of the model sample points.Fig-ure 2shows typical face hypotheses generated using this method.The average time for location was around 0.2sec using 10%of the model samplepoints.4Active Appearance Model SearchWe now address the central algorithm:given a full appearance model as described above and a reasonable starting approximation we propose a scheme for adjusting the model parameters efficiently,such that a synthetic face is generated,which matches the image as closely as possible.We first outline the basic idea,before giving details of thealgorithm.We wish to treat interpretation as an optimisation prob-lem in which we minimise the difference between a real face image and one synthesised by the appearance model.A differencevector can bedefined:(6)where is the vector of grey-level values in the image,and ,is the vector of grey-level values for the current model parameters:3To locate a best match between model and image,we wish to minimize the magnitude of the differencevector,,by varying the modelparameters,.Since the model has around80parameters,this appearsatfirst to be a very difficult optimisation problem involvingsearch in a very high-dimensional space.We note,however,that each attempt to match the model to a new face image,is actually a similar optimisation problem.We propose tolearn something about how to solve this class of problemsin advance.By providing a-priori knowledge of how toadjust the model parameters during during image search,we arrive at an efficient run-time algorithm.In particular,we might expect the spatial patternin,to encode infor-mation about how the model parameters should be changedin order to achieve a betterfit.For example,if the largestdifferences between the model and the image occurred atthe sides of the face,that would imply that a parameter thatadjusted the width of the model face should be adjusted.This expected effect is seen infigure3.In adopting this approach there are two parts to the prob-lem:learning the relationshipbetween and the error inthe modelparameters,and using this knowledge in aniterative algorithm forminimising.The simplest model we could choose for the relationshipbetween and the error in the model parameters(and thusthe correction which needs to be made)islinear:(7)This turns out to be a good enough approximation toprovide good results.Tofind,we perform multiplemultivariate linear regression on a large sample of knownmodeldisplacements,,and the corresponding differenceimages,.We can generate these large sets of randomdisplacements,by perturbing the‘true’model parametersfor the images in the training set by a known amount.As well as pertubations in the model parameters,we alsomodel small displacements in2D position,scale,andorientation.These extra4parameters are included in theregression;for simplicity of notation,they can,however,be regarded simply as extra elements of thevector.Inorder to obtain a well-behaved relationship it is importantto choose carefully the frame of reference in which theimage difference is calculated.The most suitable frame ofreference is the shape-normalised face patch described insection2.We calculate a difference thus:for the currentlocation of the model,calculate the image grey-levelsamplevector,,by warping the image data at the currentlocation into the shape-normalised face patch.This iscompared with the model grey-level samplevector,,calculated using equation4:(8)Thus,we can modify equation7:(9)The best range of valuesof to use during trainingis determined experimentally.Ideally we seek to modela relationship that holds over as large a rangeerrors,as possible.However,the real relationship is foundto be linear only over a limited range of values In ourexperiments,the model used80parameters.The optimumpertubation level was found to be around0.5standarddeviations(over the training set)for each model parameter.Each parameter was perturbed from the mean by a valuebetween0and1standard deviation.The scale,angleand position were perturbed by values ranging from0to+/-10%(positional displacements are relative to theface width.)After performing linear regression,we cancalculate an R statistic for each parameterperturbation,to measure how well the displacement is‘predicted’by the errorvector.The average R value for the80parameters was0.82,with a maximum of0.98(the1stparameter)and a minimum of0.48.Figure3illustrates theshape-free error image reconstructedfor,for a deviationof2standard deviations in the1st model parameter,and ahorizontal displacement of10pixels.4Given a method for predicting the correction whichneeds to made in the model parameters we can construct aniterative method for solving our optimisation problem.Fora given model projection into theimage,,we calcuate thegrey-level sample errorvector,,and update the modelestimatethus:(10)If the initial approximation is far from the correct solu-tion the predicted model parameters at thefirst iteration willgenerally not be very accurate but should reduce the energyin the difference image.This can be ensured byscalingso that the prediction reduces the magnitude of thedifferencevector,,for all the examples in the trainingset.Given the improved value of the model parameters,the prediction made in the next iteration should be better.The procedure is iterated to convergence.Typically thealgorithm converges in around5-10iterations from fairlypoor starting approximations-more quantitative data aregiven in the results section.5Experimental ResultsThe method was tested on a set of80previously unseenface images.Figure4shows three example images used fortesting and the‘true’model reconstruction,based on hand-annotation of the face location and shape.Figure5illustrates the result of applying AAM search tothese images.The left hand image shows the original over-laid with the initial hypothesis for face location.In practise,we usually have better starting hypotheses than shown here,however,in order to illustrate the convergence properties ofAAM search,we have deliberately displaced the hypothe-ses generated by the stochastic generator,so as to make theproblem‘harder’.Alongside the initial approximation areshown the search result afters iterations1,5and12,respec-tively.We tested the reconstruction error of AAM searchover a test set of80unseen images.The reconstructionerror for each image is calculated as the magnitude of theshape-normalised grey-level samplevector,.Figure6show a graph of reconstruction error versus iteration:Two plots are shown:The solid curve is a plot of averageerror versus iteration for the test set.The dashed curveshows the worst case encountered in the test.The twohorizontal lines indicate the error measured when themodel isfitted using accurate,hand-labelled points,for theaverage and worst case respectively.The error is measuredin average grey-level difference per sample pixel,wherepixels take a value from0to63.5Iteration6Discussion and ConclusionsWe have demonstrated an iterative scheme for fit-ting an Active Appearance Model to face images.The method makes use of learned correlation between model-displacement and the resulting difference image.Given a reasonable initial starting position,the search converges quickly,and is comparable in speed to an Active Shape ing AAMs real-time tracking should be possible on a standard PC.However,since all the image evidence is used,the procedure is more robust than ASM search alone.We are currently investigating further efficiency improvements,for example,subsampling both model and image,as was used in the method for hypotheses generation.It is intended to use AAM search to track faces in sequences,using the tracking scheme of Edwards et al [3].This scheme requires both off-line and on-line ’de-coupling’of sources of variation due to ID,Pose,Lighting and Expression.The decoupling makes use of the full appearance model and thus provides more information when used with full AAM search than with ASM search alone.The dynamic constraints and evidence integration of the tracking scheme provide further robustness and thus we expect excellent performance from a full AAM tracking scheme.References[1]M.J.Black and Y .Yacoob.Recognizing Facial Expres-sions under Rigid and Non-Rigid Facial Motions.In Inter-national Workshop on Automatic Face and Gesture Recog-nition 1995,pages 12–17,Zurich,1995.[2]M.Covell.Eigen-points:Control-point Location using Prin-cipal Component Analysis.In International Workshop on Automatic Face and Gesture Recognition 1996,pages 122–127,Killington,USA,1996.[3]G.J.Edwards,C.J.Taylor,and T.Cootes.Learning toIden-tify and Track Faces in Image Sequences.In British Machine Vision Conference 1997,Colchester,UK,1997.[4]T.Ezzat and T.Poggio.FacialAnalysis and Synthesis UsingImage-Based Models.In International Workshop on Auto-matic Face and Gesture Recognition 1996,pages 116–121,Killington,Vermont,1996.[5]K.J.J.Matas and J.Kittler.Fast Face Localisation andVerification.In British Machine Vision Conference 1997,Colchester,UK,1997.[6]nitis,C.Taylor,and T.Cootes.Automatic Interpre-tation and Coding of Face Images Using Flexible Models.IEEE Transactions on Pattern Analysis and Machine Intelli-gence ,19(7):743–756,1997.[7] C.Nastar,B.Moghaddam,and A.Pentland.GeneralizedImage Matching:Statistical Learning of Physically-Based Deformations.In European Conference on Computer Vision ,volume 1,pages 589–598,Cambridge,UK,1996.[8]S.Sclaroff and J.Isidoro.Active Blobs.In Interna-tional Conference on Computer Vision ,pages 1146–1153,Mumbai,India,1998.[9]M.Turk and A.Pentland.Eigenfaces for Recognition.Jour-nal of Cognitive Neuroscience ,3(1):71–86,1991.[10]P.Viola and W.W.III.Alignment by Maximization of Mu-tual Information.In International Conference on Com-puter Vision ,pages 16–23,Cambridge,USA,1995.6。