Forecasting models of wind power in Northeastern of Brazil
[美]R·格伦·哈伯德《宏观经济学》R.GlennHubbard,AnthonyP
![[美]R·格伦·哈伯德《宏观经济学》R.GlennHubbard,AnthonyP](https://img.taocdn.com/s3/m/c5cc42cdd05abe23482fb4daa58da0116c171f30.png)
Macroeconomics R. GLENN HUBBARD COLUMBIA UNIVERSITY ANTHONY PATRICK O’BRIEN LEHIGH UNIVERSITY MATTHEW RAFFERTY QUINNIPIAC UNIVERSITY Boston Columbus Indianapolis New York San Francisco Upper Saddle RiverAmsterdam Cape Town Dubai London Madrid Milan Munich Paris Montreal Toronto Delhi Mexico City So Paulo Sydney Hong Kong Seoul Singapore Taipei TokyoAbout the AuthorsGlenn Hubbard Professor Researcher and Policymaker R. Glenn Hubbard is the dean and Russell L. Carson Professor of Finance and Economics in the Graduate School of Business at Columbia University and professor of economics in Columbia’s Faculty of Arts and Sciences. He is also a research associate of the National Bureau of Economic Research and a director of Automatic Data Processing Black Rock Closed- End Funds KKR Financial Corporation and MetLife. Professor Hubbard received his Ph.D. in economics from Harvard University in 1983. From 2001 to 2003 he served as chairman of the White House Council of Economic Advisers and chairman of the OECD Economy Policy Commit- tee and from 1991 to 1993 he was deputy assistant secretary of the U.S. Treasury Department. He currently serves as co-chair of the nonpar-tisan Committee on Capital Markets Regulation and the Corporate Boards Study Group. ProfessorHubbard is the author of more than 100 articles in leading journals including American EconomicReview Brookings Papers on Economic Activity Journal of Finance Journal of Financial EconomicsJournal of Money Credit and Banking Journal of Political Economy Journal of Public EconomicsQuarterly Journal of Economics RAND Journal of Economics and Review of Economics and Statistics.Tony O’Brien Award-Winning Professor and Researcher Anthony Patrick O’Brien is a professor of economics at Lehigh University. He received a Ph.D. from the University of California Berkeley in 1987. He has taught principles of economics money and banking and interme- diate macroeconomics for more than 20 years in both large sections and small honors classes. He received the Lehigh University Award for Distin- guished Teaching. He was formerly the director of the Diamond Center for Economic Education and was named a Dana Foundation Faculty Fel- low and Lehigh Class of 1961 Professor of Economics. He has been a visit- ing professor at the University of California Santa Barbara and Carnegie Mellon University. Professor O’Brien’s research has dealt with such issues as the evolution of the U.S. automobile industry sources of U.S. economiccompetitiveness the development of U.S. trade policy the causes of the Great Depression and thecauses of black–white income differences. His research has been published in leading journals in-cluding American Economic Review Quarterly Journal of Economics Journal of Money Credit andBanking Industrial Relations Journal of Economic History Explorations in Economic History andJournal of PolicyHistory.Matthew Rafferty Professor and Researcher Matthew Christopher Rafferty is a professor of economics and department chairperson at Quinnipiac University. He has also been a visiting professor at Union College. He received a Ph.D. from the University of California Davis in 1997 and has taught intermediate macroeconomics for 15 years in both large and small sections. Professor Rafferty’s research has f ocused on university and firm-financed research and development activities. In particular he is interested in understanding how corporate governance and equity compensation influence firm research and development. His research has been published in leading journals including the Journal of Financial and Quantitative Analysis Journal of Corporate Finance Research Policy and the Southern Economic Journal. He has worked as a consultantfor theConnecticut Petroleum Council on issues before the Connecticut state legislature. He has alsowritten op-ed pieces that have appeared in several newspapers including the New York Times. iii Brief Contents Part 1: Introduction Chapter 1 The Long and Short of Macroeconomics 1 Chapter 2 Measuring the Macroeconomy 23 Chapter 3 The Financial System 59 Part 2: Macroeconomics in the Long Run: Economic Growth Chapter 4 Determining Aggregate Production 105 Chapter 5 Long-Run Economic Growth 143 Chapter 6 Money and Inflation 188 Chapter 7 The Labor Market 231 Part 3: Macroeconomics in the Short Run: Theory and Policy Chapter 8 Business Cycles 271 Chapter 9 IS–MP: A Short-Run Macroeconomic Model 302 Chapter 10 Monetary Policy in the Short Run 363 Chapter 11 Fiscal Policy in the Short Run 407 Chapter 12 Aggregate Demand Aggregate Supply and Monetary Policy 448 Part 4: Extensions Chapter 13 Fiscal Policy and the Government Budget in the Long Run 486 Chapter 14 Consumption and Investment 521 Chapter 15 The Balance of Payments Exchange Rates and Macroeconomic Policy 559 Glossary G-1 Index I-1ivContentsChapter 1 The Long and Short of Macroeconomics 1WHEN YOU ENTER THE JOB MARKET CAN MATTER A LOT ........................................................ 11.1 What Macroeconomics Is About........................................................................... 2 Macroeconomics in the Short Run and in the Long Run .................................................... 2 Long-Run Growth in the United States ............................................................................. 3 Some Countries Have Not Experienced Significant Long-Run Growth ............................... 4 Aging Populations Pose a Challenge to Governments Around the World .......................... 5 Unemployment in the United States ................................................................................. 6 How Unemployment Rates Differ Across Developed Countries ......................................... 7 Inflation Rates Fluctuate Over Time and Across Countries................................................. 7 Econo mic Policy Can Help Stabilize the Economy .. (8)International Factors Have Become Increasingly Important in Explaining Macroeconomic Events................................................................................. 91.2 How Economists Think About Macroeconomics ............................................. 11 What Is the Best Way to Analyze Macroeconomic Issues .............................................. 11 Macroeconomic Models.................................................................................................. 12Solved Problem 1.2: Do Rising Imports Lead to a Permanent Reductionin U.S. Employment. (12)Assumptions Endogenous Variables and Exogenous Variables in EconomicModels ........................................................................................................ 13 Forming and Testing Hypotheses in Economic Models .................................................... 14Making the Connection: What Do People Know About Macroeconomicsand How Do They KnowIt .............................................................................................. 151.3 Key Issues and Questions of Macroeconomics ............................................... 16An Inside Look: Will Consumer Spending Nudge Employers to Hire................................ 18Chapter Summary and Problems ............................................................................. 20 Key Terms and Concepts Review Questions Problems and Applications Data Exercise Theseend-of-chapter resource materials repeat in all chapters.Chapter 2 Measuring the Macroeconomy 23HOW DO WE KNOW WHEN WE ARE IN ARECESSION ........................................................... 23Key Issue andQuestion .................................................................................................... 232.1 GDP: Measuring Total Production and Total Income ..................................... 25 How theGovernment Calculates GDP (25)Production and Income (26)The Circular Flow of Income (27)An Example of Measuring GDP (29)National Income Identities and the Components of GDP (29)vvi CONTENTS Making the Connection: Will Public Employee Pensions Wreck State and Local Government Budgets.................................................................... 31 The Relationship Between GDP and GNP........................................................................ 33 2.2 Real GDP Nominal GDP and the GDP Deflator.............................................. 33 Solved Problem 2.2a: Calculating Real GDP . (34)Price Indexes and the GDP Deflator (35)Solved Problem 2.2b: Calculating the Inflation Rate ..........................................................36 The Chain-Weighted Measure of Real GDP ....................................................................37 Making the Connection: Trying to Hit a Moving Target: Forecasting with “Real-Time Data” .................................................................................. 37 Comparing GDP Across Countries................................................................................... 38 Making the Connection: The Incredible Shrinking Chinese Economy ................................ 39 GDP and National Income .............................................................................................. 40 2.3 Inflation Rates and Interest Rates ....................................................................... 41 The Consumer Price Index .............................................................................................. 42 Making the Connection: Does Indexing Preserve the Purchasing Power of Social Security Payments ................................................................ 43 How Accurate Is theCPI ............................................................................................... 44 The Way the Federal Reserve Measures Inflation ............................................................ 44 InterestRates .................................................................................................................. 45 2.4 Measuring Employment and Unemployment .. (47)Answering the Key Question ............................................................................................ 49 An Inside Look: Weak Construction Market Persists.......................................................... 50 Chapter 3 The Financial System 59 THE WONDERFUL WORLD OFCREDIT ................................................................................... 59 Key Issue and Question .................................................................................................... 59 3.1 Overview of the Financial System ...................................................................... 60 Financial Markets and Financial Intermediaries ................................................................ 61 Making the Connection: Is General Motors Making Cars or Making Loans .................... 62 Making the Connection: Investing in the Worldwide Stock Market . (64)Banking and Securitization (67)The Mortgage Market and the Subprime Lending Disaster (67)Asymmetric Information and Principal–Agent Problems in Financial Markets...................68 3.2 The Role of the Central Bank in the Financial System (69)Central Banks as Lenders of Last Resort ..........................................................................69 Bank Runs Contagion and Asset Deflation ....................................................................70 Making the Connection: Panics Then and Now: The Collapse of the Bank of United States in 1930 and the Collapse of Lehman Brothers in2008 (71)3.3 Determining Interest Rates: The Market for Loanable Funds and the Market forMoney .......................................................................................... 76 Saving and Supply in the Loanable Funds Market ........................................................... 76 Investment and the Demand for Loanable Funds ............................................................ 77 Explaining Movements in Saving Investment and the Real Interest Rate (78)CONTENTS .。
基于相空间重构的神经网络短期风速预测

基于相空间重构的神经网络短期风速预测廖志强;李太福;余德均;程杨;姚立忠【摘要】针对风速具有较强的混沌特性,预测难度较大,提出了一种基于相空间重构的神经网络短期风速预测方法:对数据进行小波降噪,运用互信息法和虚假最近邻点法确定最佳的延迟时间和嵌入维数,对样本空间进行重构,使新的样本能够表征原始时间序列动态特性,更能反映风速变化特性.在此基础上运用BP神经网络进行短期风速预测.实验结果表明短期风速预测精度得到提高.%Considering the wind speed built-in a strong chaos characteristic and difficult to forecast, a short-term wind speed prediction method based on phase space reconstruction and neural network is proposed to predict wind speed data of time series. The data noises are reduced by wavelet de-noising. Optimal delay time is obtained by mutual information method and embedding dimension is also calculated by false nearest neighbor (FNN) method which reconstructed the sample space. The new space can not only be characterized by the dynamic characteristics of the original time series but also reflect the characteristics of wind. Finally, BP network can be used to forecast short-term wind speed. The experimental results show that the accuracy of short-term wind speed prediction is improved.【期刊名称】《江南大学学报(自然科学版)》【年(卷),期】2012(011)001【总页数】5页(P14-18)【关键词】相空间重构;互信息法;虚假最近邻点法;BP神经网络;风速预测【作者】廖志强;李太福;余德均;程杨;姚立忠【作者单位】西安石油大学电子工程学院,陕西西安710065;重庆科技学院电气与信息学院,重庆401331;重庆电力高等专科学校实践教学部,重庆400053;重庆市农业科学院,重庆401329;西安石油大学电子工程学院,陕西西安710065【正文语种】中文【中图分类】TP183鉴于石油、天然气、煤炭等燃料日益枯竭,并对环境造成污染,各国都在积极发展各种绿色能源技术。
上海市风华中学2024-2025学年高三上学期9月阶段测试英语试题

上海市风华中学2024学年度第一学期高三年级英语阶段测试(2024.9)(满分140分考试时间:120分钟)第Ⅰ卷I. Listening ComprehensionSection A 10%Directions: In Section A, you will hear ten short conversations between two speakers. At the end of each conversation, a question will be asked about what was said The conversations and the questions will be spoken only once. After you hear a conversation and the question about it, read the four possible answers on your paper, and decide which one is the best answer to the question you have heard.1. A. She has no appetite at all. B. She wants to dine out.C. She is too tired to go out.D. She prefers to cook at home.2. A. 6 pounds B. 7 pounds. C. 8 pounds. D. 9 pounds.3. A. At the professor's office. B. In the bookstore.C. In the library.D. In the laboratory.4. A. Because something went wrong with his car.B. Because his car was broken in an accident.C. Because he wanted to take a walk for a rest.D. Because he was stuck in a traffic jam.5. A. The morning flight. B. The afternoon flight.C. The evening flight.D. The midnight flight.6. A. She is not interested in going camping with him.B. She wants the man to stay at home with her.C. She thinks the man needs to have a good rest.D. She thinks the man should prepare for the exams.7. A. Some major revisions are needed. B. It should be revised by a tutor.C. Only a few changes should be made.D. The draft needs no revision at all.8 A. He is going away for a while. B. He worked hard to earn money.C. He did very well in the exam.D. He can't wait to have a rest.9. A. He forgot to bring his own camera. B. He is not good at taking pictures.C. He cannot take a photo with the camera.D. He doesn't know how to use the camera.10. A. She was interrupted by a visiting friend. B. She didn't come back until midnight.C. She stayed up late for the final exam.D. She visited her friend instead of studying.Section BDirections: In Section B, you will hear two short passages, and you will be asked several questions on each of the passages. The passages will be read twice, but the questions will be spoken only once. When you hear a question, read the four possible answers on your paper and decide which one would be the best answer to the question you have heard.Questions 11 through 13 are based on the following passage.11. A. They can maintain their body temperature stable.B. They conserve enough energy before the long sleep.C. They can keep their heart beat at a regular rate.D. They have their weight increased to the maximum.12. A. By staying in bidıng places and eating little.B. By seeking extra food and warm shelter.C. By growing thicker hair to stay warm.D. By storing enough food in advance.13. A To stay safe. B. To save energy. C. To get more food. D. To protect the young. Questions 14 through 16 are based on the following passage.14. A. Four to six hours. B. Six to nine hours.C. Around eight hours.D. More than eight hours.15. A. They may not be able to concentrate well.B. They may get the feeling of being drunk.C. They may suffer from high blood pressure.D. They may lose weight easily in a short period of time.16. A. Military people are used to being deprived of sleep.B. Training can make people sleep less and suffer less.C. People can bank sleep by sleeping more beforehand.D. Sleeping earlier than usual makes people sleep less.Questions 17 through 20 are based on the following passage.17. A. Double Eleven sales in 2021.B. Unreliable factors of online shopping.C. Key points of Taobao's success ı n sales.D. Advantages and disadvantages of online shopping.18. A. People who are good at doing business.B. People who work seven days a week.C. People who have very busy schedules.D. People who dislike telephone shopping.19. A. Consumers can save a lot of time.B. It provides round- the- clock service.C. People can buy things without leaving their homes or offices.D. The quality of the product is the same as what is described online.20. A. Inferior quality. B. Various retailers.C. Efficient sales return.D. Convenient delivery.II. Grammar and VocabularySection ADirections: After reading the passages below, fill in the blanks to make the passages coherent and grammatically correct. For the blanks with a given word, fill in each blank with the proper form of the given word; for the other blanks, use one word that best fits each blankAI Weather Forecasting Can't Replace Humans- YetAs Hurricane Lee was curving(呈曲线)northward to the west of Bermuda in mid- September of last year, forecasters were busily consulting weather models and data from hurricane- hunter aircraft to calculate (21)________ the dangerous storm was likely to make landfall (着陆): New England or farther east, in Canada. The sooner the meteorologists(气象学家)could do so, the earlier they could warn those in the path of damaging wind gusts and fierce storm surges By six days ahead of landfall, it was clear that Lee (22)________(follow)the eastward path, and warnings were issued, accordingly. But (mother tool- an experimental AI model called GraphCast-(23)________(mate)that outcome accurately three whole days before the forecasters' traditional models.GraphCast's prediction is a window into AI's potential (24)________(improve)weather forecasts. But whether it is a forecaster of a true sea change in the field or will simply become one of many tools (25)________ human forecasters consult to determine which way the winds will blow is still up in the air.GraphCast, developed by Google DeepMind, is the latest of several AI weather models (26)________(release)in recent years. Google's Metnet, first introduced in2020, is already being used in products such as the company's “now cast” in its weather app. All are advertised as having an accuracy that is comparable with or higher than(27)________ on the best non-AI forecasting computer models and have caused a sensation in meteorology, with GraphCast (28)________(cause)the most significant stir so far.The DeepMind research team had put GraphCast through its paces by feeding it historical weather data to see if it could accurately “predict” what happened. The study showed the AI performed equal to or even better than the gold standard.Yet (29)________ GraphCast becomes probabilistic-- and even if the model's resolution improves and the AI becomes more accurate in its forecasts of rain and storm intensity - modeling remains just a single component of the weather- prediction pipeline, says Hendrik Tolman, senior adviser for advanced modeling systems at the NWS. However, every expert described GraphCast and other Al models as additional devices in their tool kit. If AI (30)________produce accurate forecasts quickly and cheaply, there's no reason not to begin using it together with existing methods.But will there be a world where AI models replace physics- based models— and people -- in the future? Forecasts suggest there's little chance.Section BDirections: Complete the following passage by using the words or phrases in the box. Each word or phrase can only be used once. Note that there is one word or phrase more than you need.Alzheimer's Drug Approved Despite Fierce DebateThe -U. S. Food and Drug Administration (FDA)recently approved the drug Aduhelm, produced by Americanbiotechnology company Biogen with Japan's Eisai Co., to treat patients with Alzheimer's(老年痴呆症)disease. The approval was based on study results showing that the drug seemed“(31)________ likely” to benefit Alzheimer's patients, the FDA said.The decision, which could (32)________ millions of Alzheimer's patients and their families, has sparked disagreements among medical researchers. While the drug was shown to be effective in slowing the mental decline in patients' suffering from the disease, it was not proven to be effective in (33)________ its effects, the Associated Press reported. citing a study. The rate of mental decline in patients that had been administered Aduhelm was slowedby 22 percent when compared to patients who had received a placebo(安慰剂). But even given these results, on a test that is conducted to evaluate the cognitive and (34)________ abilities of a patient, patients who were administered Adubelm only showed an increase of 0.39 in their- scores. And it's unclear how such metrics (度量标准)translate into practical benefits, like greater(35)________ or the ability to recall important details.The FDA's review of the drug has become a flashpoint in (36)________ debates over standards used to evaluate therapies for hard- to- treat conditions. On one side, groups representing Alzheimer's patients and their families sayany new therapy - even one of small benefit -deserves approval. But many experts ward that(37)________ the drug could set a dangerous example by opening the door to treatments of questionable benefit.Alzheimer's is an irreversible, (38)________ brain disorder that slowly attacks areas of the brain that are essential to memory, reasoning, communication, and basic daily tasks. In the final stages of the disease, the patientswill lose the ability to(39)________Science doesn't fully understand what causes Alzheimer's, but there's broad agreement that the brain plaque(斑点)that is being (40)________ by Aduhelm is one of the contributing factors. Evidence suggests family history, education, and chronic conditions like heart disease may all play a role. “This is a sign of hope but not the final answer,” said Dr. Richard Hodes, director of the U. S. National Institute on Aging.III. Reading ComprehensionSection ADirections: For each blank in the following passage there are four words or phrases marked A, B, C and D. Fill in each blank with the word or phrase that best fits the context.Some people like to read the instructions from start to finish before they take action while others study the diagrams and then jump right in. This 41 for one approach over another when learning new information is not uncommon. Indeed, the notion that people learn in different ways is such a universal belief in American culture that there is a thriving industry dedicated to 42 learning styles and training teachers to meet the needs of different learners.Just because a notion is popular, 43 , doesn't make it true. A recent review of learning styles found evidence to clearly support the idea that outcomes are 44 when instructional techniques align with (匹配)individuals' learning styles. Most previous investigations on learning styles focused on classroom learning, and assessed whether instructional style 45 outcomes for different types of learners. But is the 46 really where most of the serious learning occurs? Some might argue that, in this era of flipped classrooms and online course materials, students 47 more of the information on their own. That might explain why instructional style in the classroom matters little. It also 48 the possibility that learning styles do matter. Perhaps a 49 betweenstudents' individual learning styles and their study strategies is the key to ideal outcomes.To explore this 50 , researchers asked students enrolled in an anatomy class (解剖课)to complete an online learning styles assessment, answer questions about their study strategies and report details about the 51 they used outside of class(e. g. flash cards, review of lecture notes, anatomy coloring books).Scores suggested that most students used multiple learning styles, but that no particular style 52 better outcomes than another. The focus in this study, however, was not on whether a particular learning style was more53 . Despite knowing their own, self- reported learning preferences, nearly 70% of students 54 to employ study techniques that supported those preferences. Given the popular belief that learning styles matter, and the fact that many students 55 poor academic performance on the lack of a match between their learning style and teachers' instructional methods, one might expect students to rely on techniques that support their personal learning preferences when working on their own.41. A. preference B. tendency C. phenomenon D. practice42. A. identifying B. exposing C. revealing D. establishing43. A. therefore B. moreover C. however D. instead44. A. best B. acceptable C. disappointing D. undesirable45. A. impacted B challenged C. confirmed D. supported46. A. network B. classroom C. school D. lecture47. A. require B. collect C. master D. demand48. A. limits B eliminates C examines D. raises49. A. comparison B. link C. balance D. match50. A. issue B. possibility C. field D. proposal51. A. equipment B. techniques C. notebooks D. assistance52. A. originated in B. resulted from C. resulted in D. took over53. A. important B. advantageous C meaningful D popular54. A. failed B. managed C. struggled D. attempted55. A. count B. concentrate C. blame D. conductSection BDirections: Read the following four passages. Each passage is followed by several questions or unfinished statements. For each of them there are four choices marked A, B, C and D. Choose the one that fits best according to the information given in the passage you have just read.(A)I was sure that I was to be killed. I became terribly nervous. I fumbled (摸索)in my pockets to see if there were any cigarettes, which had escaped their search. I found one and because of my shaking hands, I could barely get it to my lips. But I had no matches; they had taken those. I looked through the bars at my jailer. He did not make eye contact with me. I called out to him, “Have you got a light?” He looked at me, shrugged and came over to light my cigarette. As he came close and lit the match, his eyes unconsciously locked with mine. At that moment, I smiled. I don't know why I did that. Perhaps it was nervousness, perhaps it was because, when you get very close, one to another, it is very hard not to smile. In any case, I smiled. In that instant, it was as though a spark jumped across thegap between our two hearts, our two human souls. I know he didn't want to, but my smile leaped through the bars and generated a smile on his lips, too. He lit my cigarette but stayed near, looking at me directly in the eyes and continuing to smile.I kept smiling at him, now aware of him as a person and not just a jailer. And his looking at me seemed to have a new dimension too.“Do you have kids?” he asked.“Yes, here, here.”I took out my wallet and nervously fumbled for the pictures of my family. He, too, took out the pictures of his family and began to talk about his plans and hopes for them. My eyes filled with tears. I said that I feared that I'd never see my family again, never have the chance to see them grow up. Tears came to his eyes, too. Suddenly, without another word, he unlocked my cell and silently led me out. Out of the jail, quietly and by back routes, out of the town. There, at the edge of town, he released me. And without another word, he turned back toward the town.My life was saved by a smile, yes, the smile- the unaffected, unplanned, natural connection between people. I really believe that if that part of you and that part of me could recognize each other, we wouldn't be enemies. We couldn't have hate or envy or fear.56. The underlined sentence indicates that the author and the jailer started to have a ________ conversation.A. less impersonalB. more intenseC. less formalD. more friendly57. Which is true based on the first paragraph?A. My hands were shaking because of fear.B. The jailer was going to shoot me.C. I smile8 because I had to beg for life.D. He smiled to me because he wanted to.58. Their eyes were filled with tears because they both ________.A. took out the pictures of their familiesB. missed their families far awayC. had plans and hopes for futureD. feared that they would die59. How does a smile succeed in saving the author's life?A. By asking for the jailer to light a cigarette.B. By planning for an exchange of family pictures.C. By establishing natural connection between people.D. By hiding the human feelings of hate, envy or fear.(B)CareersHome>> How To ApplyFAQs on preparing your ApplicationQ: Should I target my Application to a specific Job Opening(JO)?A: Yes. Naturally, a customized cover note will also help you focus on the key aspects of your Application that relateto the JO, but it is also in your interest to target the Application according to the responsibilities and competencies of the position.Q: What's the difference between duties and achievements?A: Duties describe the specific responsibilities of your job. They accurately reflect what you are doing or have done in each of your previous jobs. In other words, it is the “what you do” of your job. Achievements describe in specific terms “how well” you did in your job.Q: Many of my achievements are team- based, how do I draft them in my Application?A: You should include your team- based achievements in your Application. Indicate that you were part of a team, and describe your specific role in reaching the goal.FAQs on general Application guidelinesQ: Can I save my Application?A: Yes. You should save your Application when you make changes and/ or update it. It is recommended that you save different versions of your Application in Word format and then edit the Application online according to the post for which you are applying.Q: Can I update my Application to apply for a new JO?A: Yes. Each time you apply for a new JO, we recommend that you review your Application and update it , if appropriate, or target it to better reflect your suitability for the new JO. Your updates will not affect the content of Applications previously submitted against other JOs.Q: Must I use up all the available characters in each section of my Application?A: No. In fact, doing so may result in an unnecessary lengthy Application. Unless you have an enormous range of experiences, there is no reason to use up all the space given. Applicants are encouraged to list their duties and achievements in a clear and brief manner.60. Which of the following descriptions best shows your achievements?A. I've developed various interests, ranging from oil painting to designing model.B. I'm good at creating proposals for new product ideas aimed at a specific market.C. I'm in charge of the clearance, production and distribution of information material.D. I succeeded in directing a video presentation, assisting our group to win the first prize.61. If you want to apply for another JO, you'd better __________.A. target your focus on your interest in the JOB. save your latest application in Word formatC. Serape one application with all your competenciesD. update your application to match new requirements62. Applicants are expected to __________ in their applications.A. introduce what JOs they have previously applied toB. list the greatest achievements they have made in detailC. give key information about their experiences and achievementsD provide the results of their tests, assessments and examinations(C)Atlantis is the legendary island that sank beneath the waves in the distant past, taking down with it an advanced civilization. Is it possible that we will ever find it? Or, more importantly did it even exist?The short answer to both: No. All available evidence indicates that the philosopher Plato, sometime around 360 B.C., invented the island nation to illustrate a point about the dangers of aggressive imperialism(势力扩张). In Plato's telling, Atlantis was no utopia. Rather, it was a centrist to an idealized version of Athens from long before Plato's time. This ancient Athens was very similar to Plato's notion of the ideal state. Plato laid out the details for what such a state would look like in his famous work, I he Republic. It should be small and virtuous. The residents of Atlantis, on the other hand, were eventually “filled with an unjust lust for possessions and power," according to Plato's character who described the island.In Plato's texts, Atlantis was “larger than Libya and Asia combined,”(which, in Plato's time. would have referred to modern- day northern Africa and over half of Turkey). It was situated in the Atlantic Ocean, somewhere outward from the Strait of Gibraltar. It's a landmass large enough that, if it really existed somewhere underwater in the Atlantic, it would certainly appear on sonar maps of the ocean floor.So how did Atlantis come to represent a lost utopic civilization? For that, you can mostly blame (or thank)Ignatius Donnelly. In 1882, the former U. S. Congressman published Atlantis. The Antediluvian World. The book laid out 13 hypotheses, centered on the idea that Atlantis had truly existed, and indeed represented a place “where early mankind dwelt for ages in peace and happiness. According to Donnelly, Atlantis was the original source of many ancient civilizations around the world. If one followed the clues in Plato's writing, Donnelly believed, Atlantis could be found. He was inspired by a remarkable discovery in the early 1870s. An amateur archaeologist claimed to have unearthed the legendary city of Troy based on Homer's The Iliad. If Troy, long thought to be fictional, was real, why shouldn't Atlantis be, too?Donnelly was certain of his theory, predicting that hard evidence of the sunken city would soon be found, and that museums around the world would one day be filled with artifacts from Atlantis. Yet about 140 years have passed without a trace of evidence. The Atlantis legend has been kept alive, fueled by the public's imagination and fascination with the idea of a hidden. long- lost utopia. Yet the “lost city of Atlantis was never lost; it is where it always was: in Plato's books.63. What can we learn about Plato?A. He predicted that Atlantis would be' destroyed by aggressive imperialism.B. He was inspired by utopia to gradually form the notion of the ideal state.C. He created the setting in which residents of Atlantis were not virtuous.D. He witnessed Atlanteans' pursuit of an unjust lust for possessions and power.64. Homer's The Iliad is mentioned ___________.A. to demonstrate the actual existence of the legendary city of Troy.B. as indirect evidence of the credibility of Plato's account of Atlantis.C. because it is a great piece of fictional writing about an ancient legend.D. because it contains many clues about the legendary city of Troy.65. According to the passage, Atlantis was ___________.A. a long- lost small utopia with many virtuous residents.B. a large landmass situated in the Atlantic Ocean.C. the original source of many ancient civilizations.D. Plato's invention against which to highlight his ideal.66. Which of the following is the best title for this passage?A. Plato, Atlantis and How the City Collapsed and Finally Got LostB Plato Told a Lie, and Ignatius Donnelly was to Blame for ItC. The History, Legends, and Evidence of the Lost City of AtlantisD. Where Is the Lost City of Atlantis — and Does It Even Exist?Section CDirections: Read the passage carefully. Fill in each blank with a proper sentence given in the box. Each sentence can be used only once. Note that there are two more sentences than you need.Bringing Light to the Darkness with Crisco ArtMost paintings are best enjoyed in galleries with good lighting. But an Italian artist who goes by the name Crisco is changing the way we look at paintings with a new approach: glow (发光)- in- the- dark paint.Crisco's paintings are beautiful in normal lighting, but it is when the lights go down that they really come alive. (67)_________ His art mostly shows landscapes. Trees, horizons, and especially starry skies come alive with the glow of his paints. At the center of most of his work, there is often a human or animal figure. The figure may be just a shadow surrounded by the glowing colors, but it often appears to be the source of the light. (68)_________ Instead, they are all bright pictures of hope, life, wonder, and growth. They are Crisco's way o t adding a little light to the world.Crisco's full name is Cristoforo Scorpiniti.(69)_________ Instead of letting a negative experience get the best of him, he threw himself into a new pursuit: art. According to Crisco, he paints with glowing colors to inspire hope. Though his paintings often show night scenes that look good in the dark, Crisco does not focus on the darkness. Instead, he uses his paintings to express positivity by creating light in the darkness.A lot of his best work has come out of just painting what he felt at the time without any plan or structure.(70)_________ With over half a million followers on Instagram, Crisco is already popular on social media for his unique paintings. He'll surely only get more famous in the future for his inspiring paintings that beautifully mix darkness and light.IV. Summary Writing71. Directions: Read the following passage. Summarize the main idea and the main point(s)of the passage in no more than 60 words. Use your own words as far as possible.Are EV Really Environmentally Friendly?Many consumers are opting for an electric vehicle (EV)or plug- in hybrid electric vehicle (PHEV)to replace their polluting gas- powered cars. These electrified vehicles are rising to popularity on the premise of environmental conservation and eliminating the need for harmful emissions. There are a couple of things. however, to consider before concluding that EVs are the most environmentally friendly option for consumers.Where do electric cars get their energy? Although EVs create no emissions on board, they typically draw power from lithium- ion batteries. These batteries require charging, either at home or via a publicly accessible charging station. Since EV charging infrastructure is mainly reliant on the power grid - specifically, the grid draws power from plants like coal plants - although your EV does not produce any harmful emissions as you drive it, burning fossil fuels is involved in fueling it. Moreover, temperature extremes like excessive coldness or heat can dramatically reduce lithium- ion battery life. Carnegie Mellon University's Department of Engineering and Technology says that the most extreme cases of coldness will compromise efficiency by as much as 40%. The decreased efficiency is an issue if the power stored in the battery packs of EVs is sourced from fossil fuel- burning.Besides the power source, metal s such as lithium and cobalt are wrapped up in environmentally and socially questionable processes, too. One of the first environmental issues lithium batteries pose is how to dispose of them properly. In an average battery recycling plant, all parts of the battery are shredded into a powder using a mechanical shredder and then either melted or dissolved into acid —recycling lithium batteries isn't as simple. Lithium batteries are typically made up of a mix of different elements including cobalt, nickel, manganese and iron —cobalt especially known to be a hazardous substance. In addition, most studies associate lithium mining in South America from salt brine with salinization(盐化)of freshwater that the locals need to survive. Since the mineral contains dangerous substances, the mining process also contaminates the local water basins. So, lithium extraction exposes the local ecosystems to poisoning and other related health problems.V. TranslationDirections: Translate the following sentences into English, using the words given in the brackets.72.他那种急于求成的心态让他无缘冠军宝座。
风电功率预测论文:风电功率预测预测模型功率曲线最大信息熵原理

风电功率预测论文:大型风电场短期功率预测研究【中文摘要】中国的风电场大都是集中的大容量风电场,而且分布在负荷较少地区,风电的随机性和间歇性,给电力系统的安全稳定运行造成了严峻的挑战,不利于风电的进一步发展。
选取内蒙古一个实际风电场为例,对风电场短缺功率预测的多种模型进行研究和改进,主要的工作包括以下几个方面:根据建立的风机之间的相关系数矩阵,对风机的数据进行预处理;利用神经网络确定对功率输出相关度最大的变量,确定预测目标。
对每台风机分时段建立功率特性曲线是符合实际需要的。
时间序列模型建模简单,具有较好的短期预测精度,是最常用的线性预测模型。
神经网络模型能较好的应对序列的波动,具有理论丰富和高精度等特点,是常用的非线性模型。
SVM收敛速度快,学习能力强,泛化能力好,即使风速距离变化时,也能有效的预测序列的变化趋势。
小波分解组合模型能较好的应对非平稳时间序列,具有良好的预测精度。
卡尔曼滤波组合模型几乎没有增加建模的计算量,而且没有提高所建时间序列模型的阶次,但卡尔曼滤波算法的预测精度却极大地提高。
基于最大信息熵原理的组合模型,能有效的提高系统的鲁棒性和预测精度。
【英文摘要】Chinese wind plants are large-scale plants and most of them are located in where the load is few. It's a challenge to the power system safely and stably operation that the wind's intermittence and random which would be an obstacle to the wind power development in the future. Taking a wind power plant in Nei Monggu as an example, the paper studied some kinds of forecasting models. The paper's main works are as follows:According the the established correlation coefficient matrix of wind turbines, turbines...【关键词】风电功率预测预测模型功率曲线最大信息熵原理【英文关键词】Wind Power forecasting forecasting models power curve maximum entropy principle【目录】大型风电场短期功率预测研究致谢5-6摘要6-7ABSTRACT71 引言12-201.112-141.1.1 研究背景12-131.1.2 研究目的和意义13-141.2 风电场功率预测的分类141.2.1 按预测时间分类141.2.2 按预测对象分类141.3 风电场功率预测的方法与原理14-151.3.1 基于历史数据的风电场功率预测151.3.2 基于数值气象的风电场功率预测151.4 风电场功率预测的研究现状15-171.4.1 国外研究现状15-161.4.2 国内研究现状16-171.5 研究内容17-202 风电场参数统计规律20-302.1 引言202.2 风速和风向基本知识202.3 风电场运行数据的统计20-232.3.1 风速和风向统计21-222.3.2 发电量的统计22-232.4 风电场运行数据的预处理23-252.4.1 坏数据的剔除242.4.2 缺失数据的补充24-252.5 变量相关度研究25-272.5.1 平均影响值模型(MIV)252.5.2 算例分析25-272.6 风电场功率预测误差指标27-282.6.1 常用预测误差指标27-282.7 小结28-303 基于时间序列的风速和功率预测研究30-443.1 引言303.2 时间序列模型30-373.2.1 时间序列模型简介30-313.2.2 时间序列建模流程31-373.3 时间序列分析模型在风速和风电功率预测中的应用研究37-433.3.1 时间序列分析法在Matlab中的实现37-393.3.2 预测结果及分析39-433.4 小结43-444 基于人工神经网络的风速和功率预测研究44-644.1 引言444.2 神经网络模型44-524.2.1 人工神经网络模型44-454.2.2 代表性神经网络45-524.3 遗传算法优化52-554.3.1 遗传算法原理52-534.3.2 建模流程与实现53-554.4 神经网络在风速和风电功率预测中的应用研究55-614.4.1 神经网络在Matlab中的实现56-584.4.2 ARIMA与BP神经网络组合模型58-594.4.3 预测结果及分析59-614.5 小结61-645 基于支持向量机的风速和功率预测研究64-745.1 引言645.2 支持向量机基础64-665.2.1 理论基础64-665.2.2 核函数665.3 支持向量机的参数选择66-675.4 Libsvm工具箱简介67-685.5 支持向量机在风速和风电功率预测中的应用研究68-725.5.1 支持向量机在Matlab中的实现流程68-705.5.2 预测结果及分析70-725.6 小结72-746 基于小波分解的风速和功率预测研究74-866.1 引言746.2 小波分析基础74-796.2.1 小波函数74-756.2.2 小波变换分类75-766.2.3 多辩分分析76-776.2.4 Mallat算法77-796.3 小波分析在风速和风电功率预测中的应用研究79-846.3.1 小波母函数和小波分解层数的选取79-806.3.2 小波分解和ARIMA组合模型80-826.3.3 预测结果及分析82-846.4 小结84-867 基于卡尔曼滤波的风速/功率预测86-947.1 引言867.2 卡尔曼滤波基础86-887.2.1 卡尔曼原理简介86-887.2.2 卡尔曼滤波建模流程887.3 卡尔曼滤波在风速/风电功率预测中的应用研究88-937.3.1 卡尔曼滤波和ARIMA组合模型89-917.3.2 预测结果及分析91-937.4 小结93-948 风机功率曲线建模研究94-1008.1 引言948.2 风电场功率曲线建模94-978.2.1 风力发电机的功率曲线94-958.2.2 功率曲线拟合的方法95-978.3 风向预测97-988.4 小结98-1009 基于最大信息熵原理的综合预测方法研究100-1129.1 引言1009.2 基于最大信息熵原理的风电场组合模型100-1079.2.1 最大信息熵原理100-1039.2.2 基于最大信息熵原理的风电场组合模型风速预测103-1059.2.3 预测结果及分析105-1079.3 基于功率曲线建模的预测结果和直接预测结果比较107-1089.4 大型风电场短期功率预测系统的总框架108-1119.5 小结111-11210 结论112-11410.1 论文工作总结112-11310.2 论文创新点11310.3 课题研究展望113-114参考文献114-118附录A118-128附录B128附录C128-130索引130-132作者简历132-136学位论文数据集136。
基于灰色模型的风速_风电功率预测研究

第38卷第19期电力系统保护与控制Vol.38 No.19 2010年10月1日 Power System Protection and Control Oct. 1, 2010 基于灰色模型的风速-风电功率预测研究李俊芳,张步涵,谢光龙,李 妍,毛承雄(电力安全与高效湖北省重点实验室(华中科技大学),湖北 武汉 430074)摘要:风场中风速变化带来的风电功率波动是影响风电质量的重要因素。
基于灰色模型,对超短时平稳风速进行了一步至四步预测,并且检验了预测误差情况。
对不稳定风和阵风进行风速预测,以平稳风为例,根据实际风电功率和对应时序风速的关系建模,得到了风电功率随风速变化的各类模型下的拟合参数。
为了提高风电功率的预测精度,通过从分段函数和整体建模两个角度比较各种模型的准确程度,得到了适宜于作为风电功率特性曲线的函数模型。
通过预测的超短期风速在两种情况建模时风电功率模拟值与实际值的比较,得到了更适宜作为风电功率特性的模型。
用我国某风场的数据验证了方法的有效性。
关键词:风场;灰色模型;实时风速预测;风电功率特性曲线;信赖域法Grey predictor models for wind speed-wind power predictionLI Jun-fang,ZHANG Bu-han,XIE Guang-long,LI Yan,MAO Cheng-xiong(Electric Power Security and High Efficiency Lab,Huazhong University of Science and Technology,Wuhan 430074,China)Abstract:High variability in generated wind power that results from the wind speed is the key factor that impacts power quality B.ased on grey predictor models this paper presents one,-step to four-step average ten-minute wind speed forecasting and gives the residual error for steady wind Wind speed predictions for unsteady wind and gust are also made Then taking the steady..,wind speed for instance fitt,ing parameters in various models that wind power changes with wind speed are obtained by modelling the relationship between real wind power and sequential wind speed. In order to enhance the forecasting precision of wind power, the function model fitting for wind power characteristic is established through comparing the precision of different models from the aspect of piecewise function and overall model This paper compares the two modelling methods and finds the appropriate one Compared..the simulated value with actual value better model fitting for wind power characteristics is obtained Finally the actual data from,.,wind farm in China proves that this method is effective.This work is supported by Special Fund of the National Basic Research Program of China (No.2009CB219702).Key words:wind farm;grey predictor model;real time wind speed forecasting;wind power characteristic;trust region method 中图分类号: TM715 文献标识码:A 文章编号: 1674-3415(2010)19-0151-090 引言随着新能源发电在世界各国的开展,风能发电由于其具有比传统能源发电更加清洁环保、以及取之不尽用之不竭的特点,是目前世界上首选的可再生能源发电模式。
风力发电原理英语作文
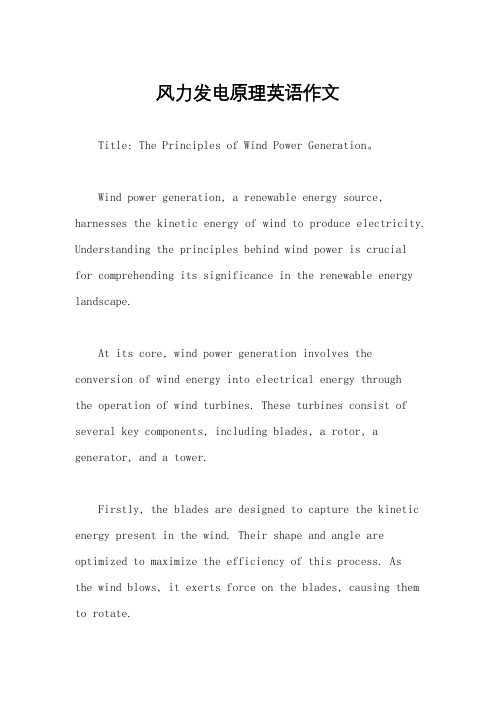
风力发电原理英语作文Title: The Principles of Wind Power Generation。
Wind power generation, a renewable energy source, harnesses the kinetic energy of wind to produce electricity. Understanding the principles behind wind power is crucialfor comprehending its significance in the renewable energy landscape.At its core, wind power generation involves the conversion of wind energy into electrical energy throughthe operation of wind turbines. These turbines consist of several key components, including blades, a rotor, a generator, and a tower.Firstly, the blades are designed to capture the kinetic energy present in the wind. Their shape and angle are optimized to maximize the efficiency of this process. Asthe wind blows, it exerts force on the blades, causing them to rotate.Next, the rotating blades turn a rotor connected to a generator. This rotor spins within a magnetic field, inducing an electric current in the generator's coils through electromagnetic induction. The generatedelectricity is then transmitted through cables connected to the turbine and subsequently integrated into the electrical grid for distribution.The height at which a wind turbine is positioned playsa crucial role in its efficiency. Wind speeds tend to increase with altitude due to reduced surface friction, making higher turbine placements more advantageous. Therefore, wind turbines are often installed on tall towers to capitalize on stronger and more consistent winds.Several factors influence the amount of electricity generated by a wind turbine. Wind speed is a primary determinant, as the power output of a turbine increaseswith higher wind speeds. Additionally, the size and designof the turbine, as well as the site's geographical location, terrain, and local weather patterns, all impact itsperformance.One of the key advantages of wind power generation isits sustainability. Unlike fossil fuels, wind energy is abundant and inexhaustible, making it a renewable resource with minimal environmental impact. By harnessing wind power, we can reduce our reliance on non-renewable energy sources and mitigate climate change.Furthermore, wind power is a form of clean energy, asit produces no greenhouse gas emissions or air pollutants during operation. This aspect is particularly crucial in combating global warming and air pollution, contributing to a healthier and more sustainable environment for future generations.However, it's essential to acknowledge the challenges associated with wind power generation. One such challengeis intermittency, as wind is not always predictable or consistent. To address this issue, advancements in energy storage technologies, grid integration, and forecasting methods are continuously being pursued to enhance thereliability and stability of wind power systems.In conclusion, wind power generation harnesses the natural force of wind to produce electricity, offering a sustainable and environmentally friendly alternative to conventional energy sources. By understanding the principles underlying wind power, we can appreciate its role in transitioning towards a cleaner and more sustainable energy future.。
Wind Power Forecast
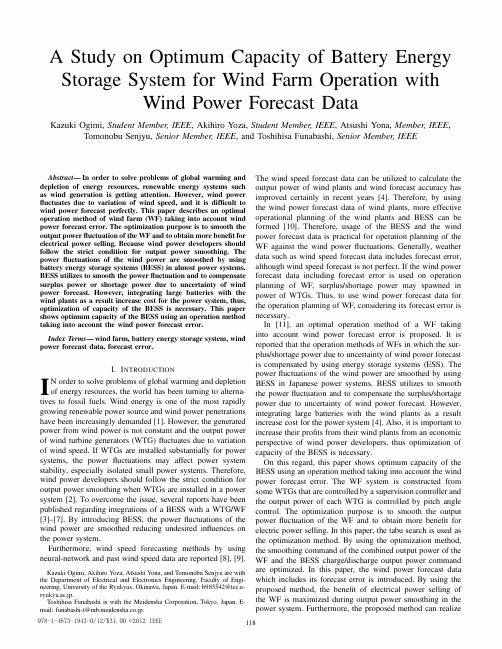
(WF)
WF and to obtain more benefit for
Index Terms- wind farm, battery energy storage system, wind
power forecast data, forecast error.
order to solve problems of global warming and depletion of energy resources, the world has been turning to alterna tives to fossil fuels. Wind energy is one of the most rapidly growing renewable power source and wind power penetrations have been increasingly demanded [1]. However, the generated power from wind power is not constant and the output power of wind turbine generators (WTG) fluctuates due to variation of wind speed. If WTGs are installed substantially for power systems, the power fluctuations may affect power system stability, especially isolated small power systems. Therefore, wind power developers should follow the strict condition for output power smoothing when WTGs are installed in a power system [2]. To overcome the issue, several reports have been published regarding integrations of a BESS with a WTGIWF [3]-[7]. By introducing BESS, the power fluctuations of the wind power are smoothed reducing undesired influences on the power system. Furthermore, wind speed forecasting methods by using neural-network and past wind speed data are reported [8], [9].
Current methods and advances in forecasting of wind power generation

ReviewCurrent methods and advances in forecasting of wind power generationAoife M.Foley a ,b ,d ,*,Paul G.Leahy a ,b ,Antonino Marvuglia c ,Eamon J.McKeogh a ,baDept.of Civil &Environmental Engineering,School of Engineering,University College Cork,College Rd.,Cork,Ireland bEnvironmental Research Institute,University College Cork,Lee Rd,Cork,Ireland cCork Constraint Computation Center (4C),University College Cork,Western Gateway Building,Cork,Ireland dSchool of Mechanical and Aerospace Engineering,Ashby Building,Queen ’s University Belfast,Stranmillis Road,BT95AH,Northern Ireland,UKa r t i c l e i n f oArticle history:Received 27February 2011Accepted 21May 2011Available online 1July 2011Keywords:MeteorologyNumerical weather prediction Probabilistic forecastingWind integration wind power forecastinga b s t r a c tWind power generation differs from conventional thermal generation due to the stochastic nature of wind.Thus wind power forecasting plays a key role in dealing with the challenges of balancing supply and demand in any electricity system,given the uncertainty associated with the wind farm power output.Accurate wind power forecasting reduces the need for additional balancing energy and reserve power to integrate wind power.Wind power forecasting tools enable better dispatch,scheduling and unit commitment of thermal generators,hydro plant and energy storage plant and more competitive market trading as wind power ramps up and down on the grid.This paper presents an in-depth review of the current methods and advances in wind power forecasting and prediction.Firstly,numerical wind prediction methods from global to local scales,ensemble forecasting,upscaling and downscaling processes are discussed.Next the statistical and machine learning approach methods are detailed.Then the techniques used for benchmarking and uncertainty analysis of forecasts are overviewed,and the performance of various approaches over different forecast time horizons is examined.Finally,current research activities,challenges and potential future developments are appraised.Ó2011Elsevier Ltd.All rights reserved.1.IntroductionOver the last decade there has been rapid growth in wind generation of electricity,with the installed wind power capacity worldwide has increased almost fourfold from circa 24.3GW to an expected 203.5GW this year [1].In power systems,balance is maintained by continuously adjusting generation capacity and by controlling demand.As wind is inherently variable,wind power is a fluctuating source of electrical energy.Short-term forecasts (ranging from 1h up to 72h)are useful in power system planning for unit commitment and dispatch,and for electricity trading in certain electricity markets where wind power and storage can be traded or hedged.Medium-term forecasts and predictions (ranging from 3days to 7days)are needed to plan maintenance of the wind farms,unit commitment and maintenance outages of thermal generators and to schedule grid maintenance and energy storage operations.Forecast errors typically increase as the time horizonincreases.However,this is always not the case,as shown in Fig.1[2].When specifying a wind power prediction model,the desired time horizon will dictate the final choice,as the different models are differently suited to certain power system planning and market activities which occur over different timescales.Wind forecasting for energy generation and power systems operations mainly focuses on the immediate short-term of seconds to minutes,the short-term of hours up to two days,and the medium term of 2e 7days.This is because power systems opera-tions such as regulation,load following,balancing,unit commit-ment and scheduling,are carried out within these timeframes.The science of wind power prediction is described as the application of the theories and practices of both meteorology and climatology speci fically to wind power generation [3].The prediction of short-term wind power patterns is discussed in Landberg [4].Traditional thermal generators are also intermittent but with more predictability than wind power.Nevertheless,thermal plant can experience sudden unplanned outages.In power systems a traditional generator is usually described as ‘dispatchable ’,whereas wind generation is often referred to as ‘non-dispatchable ’.Accurate wind power forecasting reduces the risk of uncertainty and allows for better grid planning and integration of wind into power systems.However,a common conclusion is that as the levels*Corresponding author.School of Mechanical and Aerospace Engineering,Ashby Building,Queen ’s University Belfast,Stranmillis Road,BT95AH,Northern Ireland,UK.Tel.:þ353872874092;fax:þ353214276648.E-mail addresses:aoife.foley@ucc.ie ,foley.aoife@ (A.M.Foley).Contents lists available at ScienceDirectRenewable Energyjournal h omepage:w/locate/renene0960-1481/$e see front matter Ó2011Elsevier Ltd.All rights reserved.doi:10.1016/j.renene.2011.05.033Renewable Energy 37(2012)1e 8of wind power penetration increase additional system balancing is required.The cost of the balancing is linked to the flexibility of the existing power system.Wind power forecasting tools are therefore invaluable because they enable better dispatch,scheduling and unit commitment of thermal generators,hydro plant and energy storage plant and more competitive market trading as wind power ramps up and down.Overall they reduce the financial and technical risk of uncertainty of wind power production for all electricity market participants.This paper provides a detailed review of current methods and recent advances in wind power forecasting.The paper contains three sections.Section 2overviews benchmarking and uncertainty anal-ysis,examines current forecasting methods,starting with a discus-sion of time horizons,followed by descriptions of numerical wind prediction,ensemble forecasting,upscaling and downscaling methods,and physical,statistical and learning approach methods.Section 3presents current research activities and potential future advances.Finally,Section 4gives a brief summary and conclusion.2.Current forecasting &prediction methodsForecasting models for wind power can be divided into two overall groups.The first group is based upon analysis of historical time series of wind,and a second group uses forecasted values from a numerical weather prediction (NWP)model as an input.However,wind power forecasting is generally described in terms of physical methods,traditional statistical or ‘black box ’methods and more recently the so-called learning approaches,arti ficial intelli-gence or ‘gray box ’methods.Hybrid methods can involve some aspect of all of these.The models in the first group use the statistical approach to forecast mean hourly wind speed or to directly forecast electric power production.The models in the second group use explanatory variables (mainly hourly mean wind speed and direction)derived from a meteorological model of the wind dynamics to predict wind power N-steps ahead.The models of the first group provide goodresults,in the majority of cases,in the estimation of mean monthly or even higher temporal scale (quarterly,annual)wind speed.However,in the short-term horizon,(mean daily or hourly wind speed forecasts),the in fluence of atmospheric dynamics becomes more important,so that the use of the models of the second group becomes essential [5].There are three steps in wind power forecasting:firstly deter-mining wind speed from a model;then calculating the wind power output forecast or prediction;and finally regional forecasting or upscaling or downscaling,which may be applied over different time horizons.Very short-term forecasting models are usually statistically-based.For statistical and the learningapproachFig.1.Actual and short term forecast total system wind power generation on the 10th January 2011on the Republic of Ireland System (data provided by Eirgrid).Table 1Some wind power forecasting &prediction models.Model nameDeveloper(s)MethodSome geographical locations of applicationsPrediktorndberg at Risø,DenmarkPhysicalSpain,Denmark,Republic of Ireland,Northern Ireland,France,Germany,USA,Scotland &JapanWPPTEltra/Elsamcollaboration with Informatics andMathematical Modeling at Danmarks Tekniske Universitet (DTU),DenmarkStatistical Denmark,Australia,Canada,Republic of Ireland,Holland,Sweden,Greece &Northern IrelandZephyr Risø&IMM ay DTU,DenmarkHybridDenmark&AustraliaPrevientoOldenburg UniversityHybrid Germany,Northern Irelande Wind TM True Wind Inc.,USA Hybrid USA SipreólicoUniversity Carlos III,Madrid,Spain &RedEléctrica de Espa na Statistical SpainWPMSInstitut für Solare Energieversorgungstechnik (ISET),GermanyStatistical Germany WEPROGJ.Jørgensen &C.Möhrlen at University College CorkHybrid Ireland,Denmark and Germany GHForecaster Garrad Hassan Statistical Greece,GreatBritain &USAAWPPS École des Mines,Paris Statistical Crete,Madeira,Azores &IrelandLocalPred &RegioPredM.Perez at Centro Nacional de Energias Renovables (CENER)and Centro de Investigaciones Energéticas,Medioambientales y Tecnalógicas,Spain (CIEMET)Hybrid Spain and Ireland Alea WindAleasoft at the Universitat Polytécnica de Catalunya,Spain (UPC)Statistical Spain SOWIE Eurowind GmbH,Germany Physical Germany,Austria&SwitzerlandEPREVInstituto de Engenharia de Sistemas e Computadores do Porto (INESC),Instituto de Engenharia Mecânica e Gestão Industrial (INEGI)and Centro de Estudos de Energia Eólica eEscoamentos Atmosféricos (CEsA)in Portugal Statistical Portugal SciroccoAeolis Forecasting Services,Netherlands Hybrid Netherlands,Germany &SpainA.M.Foley et al./Renewable Energy 37(2012)1e 82methods a large amount of historical time series data is essential. The persistence method,also known as the naïve predictor,can be used to benchmark other methods.Persistence usually performs better than NWP methods for short-term prediction horizons of up to about3e6h at a local level,whereas the climatologic mean is better for prediction horizons longer than15h[6].Table1presents a non-exhaustive list of wind power software models developed internationally.2.1.Numerical weather Prediction&wind forecastingIn developing a NWP-based wind power prediction model the selection of the particular NWP model is a critical step.Important selection criteria include the geographical area,the resolution (both spatial and temporal)and the forecast horizon,as well as the accuracy required and the computational time and number of runs. NWP models usually have three main components,the dynamic center,which represents the adiabatic non-viscousflow,the physical equations describing variability of the meteorological processes(e.g.turbulence and radiation)and the information gathering software code.Therefore the output of an NWP model is a detailed forecast of the state of the atmosphere at a given time, not just the wind.NWP forecasts are not specifically produced for the electricity industry and are used by a variety of industries, sectors and government agencies.NWP is sensitive to initial conditions and to overcome this ensemble forecasting is used[7]. Nielsen et al.[8]demonstrated that if several NWP forecasts are used the forecast error decreases.Louka et al.[9]showed that the Kalmanfilter can remove systematic forecast errors in NWP wind speed forecasts.Ocean models are not included in most NWP as sea surface water temperatures are described by climatology.Specific NWP models have been developed to identify storms in the Pacific and Atlantic, which tend to be ensemble NWP models(e.g.Typhoon Ensemble Model by the Japan Meteorological Agency).Most meteorological services provide only on-shore and near-shore weather predictions to meet their client needs.Hence,the focus to date of global NWP models has been to provide more accurate weather forecasts on land.As global NWP models need boundary conditions to solve their equations,mostly land surface properties including temperature are used.NWP holds best for time horizons greater than4h.Most models are multi-step and provide look-ahead times for numerous horizons but the bulk of these tools only produce a single expected value for each forecast timescale and are referred to as deterministic, spot or point forecasts.Hence their use for stochastic optimization and risk assessment is limited[10].At a regional and mesoscale level another family of NWP models was developed to focus on particularly local weather phenomena. Examples include the hydrostatic ETA model,the HIRLAM model and the ALADIN model[11e13].Further examples include the freely downloadable MM5regional model developed at the Pennsylvania State University and used by the National Center of Atmospheric Research in the United States of America(USA)and the more recent Weather Research and Forecast(WRF)regional model[14,15].Some NWP models are used at a regional level to predict wind power in a country or in a region of a country.Predicting the wind power output from each individual wind farm can be time consuming so instead an approach called‘upscaling’is used.In upscaling the wind power output from a sample number of wind farms forms the basis of reference data.Upscaling can have the apparent effect of reducing forecast error because it becomes averaged over the whole region[16].The process of downscaling involves the production of more detailed spatial information from coarse NWP outputs using physical and/or statistical models[17].Physical downscaling models are similar to NWP but run at higher resolu-tion over a smaller area.Statistical downscaling models use power and/or wind speed at an actual wind farm and NWP to generate a transfer function,which can be used to predict wind power from other wind farms in a region.Table3provides a list of a number of NWP global and regional models in use.2.2.Ensemble forecastingEnsemble forecasting employs a number of different model runs to predict a large sample of possible future weather outcomes.The results are then evaluated by examining the distribution across all ensemble‘members’of the forecast variables.Another ensemble approach is the multi-model approach,which uses a number of NWP models to produce an ensemble[18].It is referred to as a multi-NWP method.The members of the ensemble arise from different variants of the same NWP model(like different physical parameterization of the sub-grid physical processes,or different initial conditions,or different data assimilation techniques).They can also arise from completely different NWP models.An inter-esting feature of ensemble forecasting lies into the fact that it also provides an estimation of the reliability of the forecast.The idea is that when the different ensemble members differ widely the forecast is affected by a large uncertainty;when there is a closer agreement between the ensemble member forecasts,the uncer-tainty in the prediction is lower.Table2Commonly-used error measures.Measure Formula PurposeBiasBias k¼e k¼1TX Nt¼1etþk.twhere N T¼number of prediction errors for each look-ahead time k for the considered time horizon Bias signifies if the method over-estimates or under-estimates the forecast variable.It gives low results for statistical methods.If MOS are used in physical methods it also gives low results.It does not indicate the level of skill of the forecast methodMSE MSE k¼e k¼1N TX Nt¼1etþk.t!2MSE expose thecontribution of positiveand negative errors tothe lack of accuracy.Random and systematicerrors influence MSERMSE RMSE k¼ffiffiffiffiffiffiffiffiffiffiffiffiMSE kp¼ffiffiffiffiffiffiffiffiffiffiffiffiffiffiffiffiffiffiffiffiffiffiffiffiffiffiffiffiffiffiffiffiffiffiffiffiffiffiffiffiffi1N TX Nt¼1etþk.t!2v uu tRMSE is easier to interpretit is expressed in the sameunits as the forecastedvariableSDE SDE k¼ffiffiffiffiffiffiffiffiffiffiffiffiffiffiffiffiffiffiffiffiffiffiffiffiffiffiffiffiffiffiffiffiffiffiffiffiffiffiffiffiffiffiffiffiffiffiffiffiP Nt¼1"etþk.tÀe k#2v uu uu utSDE is a guesstimate ofthe error distribution.Therefore only randomerrors are a factor in SDE.SkillScore Imp ref gðkÞ¼g refðkÞÀgðkÞg refwhere Imp¼the improvement withrespect to,g ref(k)¼value for thereference approach and g(k)¼value for the advancedapproach,for time horizon k.Skill score can use MAE,RMSE or SDE includingthe normalized versionsof all three.The result isoften changed toa percentage bymultiplying by100andpresenting it asa percentageimprovement on theresult of the referenceapproach.If the resultsare always less than100,the forecast is veryaccurate.A.M.Foley et al./Renewable Energy37(2012)1e83The MSEP approach is another ensemble method,based on predictions from one NWP with different schemes[19].A study of MSEP in Ireland compared against validated results from Denmark and Germany established that forecast errors increased with increasing capacity factor due to an increase in abnormal weather events and higher than normal wind speeds[20].In Ireland,for instance,a study showed that using a power curve derived from measured wind and power can improve the forecast root mean square error(RMSE)by nearly20%in comparison to using the power curve only[21].The nonlinearity of the wind power curves leads to a further amplification of the error,such that small varia-tions in the wind speed may result in much larger deviations in the power.2.3.Physical methodsSeveral physical models based on the use of weather data have been developed for wind speed forecasting and wind power predictions[22].The physical models generally make use of global databases of meteorological measurements or atmospheric meso-scale models,but they require large computational systems in order to achieve accurate results[23].In the physical approach a detailed description of the lower atmosphere is used to estimate the wind power output.An overview of some of the neural,geostatistical and hybrid models used for space-temporal wind forecasting is con-tained in Cellura et al.[24].The numerical codes for windfield modeling over rough terrain are generally divided into two types: dynamic models(also called prognostic)and kinematic models (also called diagnostic)[25].In these models the momentum and energy equations are not solved explicitly but considered indirectly using parametric relations and/or wind data[26].Computational fluid dynamics(CFD)is also used as an alternative method to the power law to adjust for the local conditions of the physical terrain [27].Model output statistics(MOS)are often used to avoid systematic forecasting errors and to correct the predicted power output for unknowns[28].2.4.Statistical and learning approach methodsIn the statistical approach a vast amount of data is analyzed and meteorological processes are not explicitly represented.The link between historical power production and weather is determined and then used to forecast the future power output.Unlike physical methods,statistical methods involve only one-step to convert the input variables into power output.Hence,the methods used are described as‘black box’.Generally a statistical relationship is developed between the weather forecast or prediction and the potential power output from the wind farm.Other statistical techniques used include autoregressive(AR), moving average(MA),autoregressive moving average model, (ARMA)and autoregressive integrated moving average model (ARIMA),the Box-Jenkins methodology and the use of the Kalman filter.Torres et al.[29]found it was possible to get20%error reduction compared to persistence to forecast average hourly wind speed for a10h forecast horizon at a number of locations using nine years of historical data using an ARMA model.Classical time series analysis is not the only way to model the statistical relationship among the data.The main soft computing(or machine learning) approaches used are artificial neural networks(ANN)and fuzzy systems,but also other models,like gray predictors or support vector machines(SVM)have been applied.Learning approach methods are also often referred to as artificial intelligence(AI)methods.They are called learning approaches because they learn from the relationship between the predicted wind and forecasted power output using historical time series.More recently,they have been referred to as ‘gray box’methods.Wind speed and output power were forecasted using a grey predictor with a look-ahead time of1h with an accuracy respectively11.2%and12.2%better than persistence in terms of mean absolute error[30].In some studies an improvement, depending on the forecast horizon,between9.5%and28.4%over persistence was the result of using a genetic algorithm(GA)to optimize a fuzzy inference system(FIS)model[31].ANN’s‘learn from experience’using data.For this reason,the approach they are based upon is called data-driven approach.Table3Global®ional NWP models.Name Developer(s)TypeGlobal Forecast System(GFS)National Oceanic and AtmosphericAdministration(NOAA),USGlobal Action de Recherche pour laPetite Echelle et la GrandeEchelle(ARPEGE)Météo-France(METEO FRANCE)GlobalGlobal Meteorological Model (GME)Deutscher Wetterdienst(DWD),GermanyGlobalGlobal Environmental Multi-scale Model(GEM)Recherche en Prévision Numérique (RPN),Meteorological ResearchBranch(MRB),and the Canadian Meteorological Center(CMC)GlobalNavy Operational GlobalAtmospheric PredictionSystem(NOGAPS)United States Navy(USN)GlobalIntermediate GeneralCirculation Model(IGCM)NCAS Center for Global Atmospheric Modeling,University of Reading,United Kingdom(UK)GlobalUnified Model(UM)Met Office,UK GlobalIntegrated Forecast System(IFS) Note uses the same code as ARPEGE European Centre for Medium-Range Weather Forecasts(ECMWF),EnglandGlobalGSM Japan Meteorological Agency(JMA)Global Global Analysis and Prediction(GASP)Bureau of Meteorology,Australia GlobalHigh Resolution Limited Area Model(HiRLAM)Current members include:Danmarks Meteorologiske Institut(DMI),EESTI Meteoroloogia JaHüdroloogia Insitut(EMHI),Ilmatieteen Laitos(FMI),VeðurstofaÍslands(VI),MetÉireann,Koniklijk Nederlands MeteorologischInstituut(KNMI),Meteorologiskinstutt(met.no),Agencia Estatal de Meteorología(AEMET)and Swedish Meteorological and HydrologicalInstitute(SMHI)RegionalLokal-modell(LM)DWD,Germany Regional ALADIN Météo-France with a consortiumof16European partnersRegional Mesoscale Model5(MM5)Mesoscale Prediction Group in theMesoscale and MicroscaleMeteorology Division,NationalCenter for Atmospheric Research(NCAR)RegionalMSM and a number of EnsemblemodelsJapan Meteorological Service RegionalWeather Research andForecasting(WRF)Model A collaboration in the US,whichincludes NCAR,the National Oceanicand Atmospheric Administration(National Center for Environmental Prediction(NCEP)and the ForecastSystems Laboratory(FSL)),the AirForce Weather Agency(AFWA),theNaval Research Laboratory(NRL),the University of Oklahoma and theFederal Aviation Administration(FAA)RegionalConsortium for Small-Scale Modeling(COSMO)A collaboration of6European metservices led by the Federal Office ofMeteorology and ClimatologyMeteoSwissRegionalA.M.Foley et al./Renewable Energy37(2012)1e84A number of studies apply the most commonly used neural models, which is the standard multi-layer perceptron(MLP)network method[32]or the recurrent version of NN[33].Welch at al[34] compares three types of neural networks(namely MLP,simulta-neous recurrent neural network(SRN)and Elman recurrent neural network)trained using particle swarm optimization(PSO)for short-term prediction of wind speed.Ramirez-Rosado et al. compared forecasting schemes in which NWP predictions were enhanced by various neural network and other machine learning approaches and combined with turbine power curve models and demonstrated significant improvements over persistence[35]. Recently,researchers have started to use decision tree techniques in data mining with interesting results[36].The results indicate that the predictive power of individual variables is dependent on the seasons,with wind power most strongly related to atmospheric pressure in summer and to humidity in winter.Wind power fore-casts were determined at10wind farms and compared to the NWP data at each wind farm using classical MLP ANNs,mixture of experts,SVM and nearest neighbor with PSO[37].The main conclusion is that combining several models for day-ahead fore-casts produces better results.Jursa and Rohrig[38]presented an approach which combined the ANN and the nearest-neighbor approaches in an optimization model and the result was an improvement of10.75%in the normalized RMSE of the prediction compared to persistence (where the improvement equals RMSE persistence minus RMSE model divided by RMSE persistence).In summary,five data-mining models used in wind speed and wind power prediction include SVM,MLP ANN,regression trees and random forests.The review of published literature and data indicates that the MLP ANN outperforms the other four models in both very-short and short and long-term forecasts.The direct approach of feeding the wind ensembles NWP directly into the model also outperformed the integrated approach for both very-short and short and long-term models[39].Mohandes et al.[40]compared SVM to a multi-layer perceptron ANN model to predict wind speed.The SVM model gave lower RMSE than the MLP ANN model and it was established that SVM outperforms MLP for system orders from1to11.In data-mining repeating patterns are identified.In Kusiak et al.[41]four time series models with different prediction horizons were developed with data-mining algorithms and it was established that the least accurate and stable was the integrated k nearest neighbor(kNN)for power rson and Westrick[42]used a support vector classifier to estimate the forecasting error,obtaining lower mean square error and mean absolute percentage error than traditional SVM.A novel approach for the analysis and modeling of wind vectorfields was introduced by Goh et al.[43]and developed by Mandic et al.[44]where the wind vector is represented as a complex-valued quantity and,unlike the other commonly used approaches,wind speed and direction are modeled simultaneously.Negnevitsky et al.[45]combine two AI methods,ANN and fuzzy logic in a hybrid approach to develop an adaptive neural fuzzy system model(ANFIS).Fuzzy models are employed in cases where a system is difficult to model exactly or vagueness is the problem formulation is characterized by some indefinite and vague elements.In Damousis et al.[46]a fuzzy model was implemented for the prediction of wind speed and the produced electrical power at a wind park.The model was trained using a genetic algorithm-based learning.The efficiency of short-term forecasting was improved for ranges from a few minutes to several hours ahead. However,the main drawback of the proposed method is the large number of fuzzy rule base and the consequent large computational time.Pinson and G.Kariniotakis[47]developed a prediction system that integrates models based on adaptive fuzzy-neural networks configured for short and long-term forecasting.Recently,Bayesian methods have started to be employed for wind speed prediction.Miranda and Dunn[48]used an autore-gressive model based on a Bayesian approach to obtain1-h-aheadFig.2.Some prediction errors(as percentage RMSE)as a function of forecast horizon from different studies(Black markers indicate wind power generation prediction models, whereas grey markers indicate wind speed prediction models).A.M.Foley et al./Renewable Energy37(2012)1e85。
- 1、下载文档前请自行甄别文档内容的完整性,平台不提供额外的编辑、内容补充、找答案等附加服务。
- 2、"仅部分预览"的文档,不可在线预览部分如存在完整性等问题,可反馈申请退款(可完整预览的文档不适用该条件!)。
- 3、如文档侵犯您的权益,请联系客服反馈,我们会尽快为您处理(人工客服工作时间:9:00-18:30)。
Abstract—Wind Power forecasting is extremely important to assist in planning and programming studies for the operation of wind power generation. Several studies have shown that the Brazilian wind potential can contribute significantly to the electricity supply, especially in the Northeast Brazil, where winds present an important feature of being complementary in relation to the flows of the San Francisco River. However, using wind power to generate electricity has some drawbacks, such as uncertainties in generation and some difficulty in planning and operation of the power system. This paper presents actual results of wind power forecasting for two parks in the region of northeastern Brazil with four different models. Models that perform power generation forecasting using the forecasted wind speeds and the wind power curve of the park are called Wind to Power (W2P) and models that perform power generation forecasting using the historical power generation of the park are called Power to Power (P2P). The models perform forecasting of wind power generation with 6 hours ahead, discretized by 10 minutes and with 5 days ahead, discretized by 30 minutes. Models that directly predict the wind power (P2P) got the best results. These models were more suitable for use in the power systems operation planning considering the wind parks analyzed in northeastern Brazil.I.I NTRODUCTIONmong all alternative energy sources currently explored, wind energy is one of the most successful. One reason for this is the incentive policy by various countries, which ensures the purchase of electricity produced from wind power, even if it does not offer competitive prices. Germany and Denmark were the first countries to adopt policies to encourage the development of wind generation, followed by several countries, including Brazil. With the creation of the Incentive Program for Alternative Sources of Electric Energy (PROINFA) [1] in Brazil, around 1000 MW have been installed in wind farms by 2011. Note that the Manuscript received February 28, 2013.Ronaldo R. B. de Aquino, is with the Federal University of Pernambuco- UFPE, Academico Helio Ramos s/n, Cidade Universitária, Cep: 50.740-530 – Recife – PE – Brazil (corresponding author to provide phone: 55-81-21268986;e-mail: rrba@ufpe.br).Teresa B. Ludermir, is with the Federal University of Pernambuco-UFPE (e-mail:tbl@cin.ufpe.br).Otoni Nobrega Neto, is with the Federal University of Pernambuco- UFPE (e-mail:otoni.nobrega@ufpe.br).Aida A. Ferreira, is with the Federal Institute of Education, Science and Technology of Pernambuco-IFPE, Av Professor Luis Freire, 500, Cidade Universitaria, Cep:50.740-530 – Recife – PE – Brazil (e-mail: aidaferreira@.br).Milde M. S. Lira, is with the Federal University of Pernambuco -UFPE (e-mail: milde@ufpe.br).Manoel A. Carvalho Jr, is with the Federal University of Pernambuco- UFPE (e-mail:macj@ufpe.br). wind energy is the one with the highest growth in northeastern Brazil from 796 MW in 2011 to 6,405 MW in 2016, which corresponds to a growth of 4.9% to 24.4% of the total installed power [2]. This demonstrates the importance of wind generation for the electric and energetic operation planning of the Brazilian interconnected electric system.Because of the importance of wind generation in Europe, the ANEMOS project was created. The reports [3] and [4] have presented the state of the art and a protocol for models evaluation for wind power forecasting (WPF), respectively. The use of wind energy for electric power generation presents some inconveniences related to the uncertainties in the generation. Such inconveniences are basically caused by the variability of the wind speed. Therefore, the application of tools or techniques that are able to forecast the energy to be generated by those sources is essential to an appropriate integration of the wind source with the electric power system [5] and [6].As shown in [7], the time frontiers that separate the different time horizons are not consensus defined for the WPF problem, but usually can be classified in three categories: the very short term limited to four hours; the short term limited to fourth-eight hours; and the medium term from very short term up to seven days ahead.Many researchers, motivated by the importance of wind power forecasting for the integration of this energy in the electrical system, developed models for the three forecasting horizons using many techniques of artificial intelligence.A very-short term WPF was presented in [8], using as solution technique, a data mining approach with a forecasting of four hours ahead. In [9], a very-short term WPF was presented for forecasting three hours ahead, using multilayer perceptron (MLP), wavelet transform (WT), adaptive-network-based fuzzy inference systems (ANFIS) and particle swarm optimization.A short term WPF was shown in [10], [11] and [12]. In the first article, the authors used MLP, wavelet transform and neuro-fuzzy system to perform a forecasting of 24 hours ahead. In the second, the authors used a numerical weather prediction (NWP) combined with a radial basis function (RBF) network and fuzzy logic to perform a forecasting of 48 hours ahead. In the third, the authors used a reservoir compute approach to perform a forecasting of 24 hours ahead.A medium term WPF, in accordance to [7], was developed in [13] and [14] using locally recurrent multilayer networks combined with NWP as forecasting model.This paper shows a very-short term WPF for forecasting 6 hours ahead and a medium term WPF for forecasting 5 daysForecasting Models of Wind Power in Northeastern of Brazil Ronaldo R. B. de Aquino, Teresa B. Ludermir, Otoni Nobrega Neto, Aida A. Ferreira,Milde M. S. Lira, Manoel A. Carvalho Jr.Aahead, both based on neural network approach.The structure of this paper is as follows: in section II, the composition and analysis of the wind speed time series are shown. Then, in section III, the data preprocessing is presented. The study and development of the models are accomplished and analyzed in section IV. Comparisons between forecasts obtained with the proposed and reference models are done in section V. Finally, in section VI, conclusions are drawn.II. A RRANGEMENT FOR THE TIME SERIESReal data from two wind farms (Park A and B) located in two states of northeastern coast line Brazil are used in this work. The time series available for model development were: number of active machinery, wind speed, temperature (in Celsius), pressure, direction, and average wind power. All variables were provided in intervals of 4 seconds and transformed in mean values at intervals of 10, 30 and 60 minutes. Besides these data, we used time series of forecasting hourly average wind speed for the 5-day horizon, provided daily by the numerical model ETA, resulting in 120 values.Due to the low quality of the series was not possible using some of the variables, although some are of utmost importance for accuracy of the models. Thus, the models developed were based only on series of power and speed (actual and forecasting). The historical data set corresponds to the period from April 2010 to July 2012.The period used for training models that perform forecasting of wind power generation with 6 hours ahead were from 04/01/2010 to 12/31/2011. The period used for simulations were from 01/01/2012 to 07/31/2012.The period used for training models that perform forecasting of wind power generation with 5 days ahead were from 04/01/2010 to 05/31/2011. The period used for simulations was from 01/01/2012 to 07/31/2012.Fig. 1 and Fig. 2 represent the dispersion of data pairs of average wind speed (x axis) and wind power (y axis) provided by the park. The red curve is the power curve, PC(v), obtained regarded as the feature of grouping wind. The green curves are PC(v) ± 3.σ(v), where σ(v) is the standard deviation for small region centered at v. The curves were normalized by the total number of machines in eachpark.Fig. 1. Historical data of the wind speed and power generation time seriesfor the Park A.Fig. 2. Historical data of the wind speed and power generation time series for the Park B.III. D ATA P REPROCESSINGIn this section, the basic procedures for processing the wind speed data are defined before creating the proposed forecasting models.A. NormalizationThe forecasting models based on Artificial Neural Networks (ANN) demand consistent treatment of the data in order to guarantee reasonably good performance and their effective application. In general, normalization is a straightforward and effective treatment for the data [15].Normalization is carried out to assure that all variables used in the model inputs have equal importance during training; therefore the activation functions of artificial neurons are limited. In this paper, the data were normalized according to (1), also shown in [15], whose values ranged from 0 to 1.minmax min)()(X X X t X t X −−=(1)where:X and X are the wind speed time series non-normalized and normalized, respectively; max X andmin X are the maximum and the minimum absolute value ofthe wind speed time series, respectively.B. Definition of Forecast ErrorsTwo types of errors are used in this paper to illustrate the performance of the wind power forecasting model: the mean squared error– MSE, according to (2), and the normalized mean absolute error – NMAE, according to (3).,)(1)(12∑=+=N t s s k t e N k MSE(2) Where N is the number of data used for the model evaluation; k is the forecasting horizon (number of time-steps); e s (t+k ) is the error corresponding to time t+k for the wind power forecasting made at origin time t ; and s (t+k ) is the measured wind power at time t+k .,)(100)(1∑=+⋅=Nt P instPk t e P N k NMAE(3)Where N is the number of data used for the model evaluation; k is the forecast horizon (number of time-steps); e P (t+k ) is the error corresponding to time t+k for the power generation forecast made at origin time t ; and P inst is the installed wind power capacity.C. Reference Models It is worthwhile developing and implementing a wind power forecasting tool if it is able to beat reference models, which are the result of simple considerations and not ofmodeling efforts. Probably the most common reference model used in the frame of wind power prediction or in themeteorological field is the persistence model [3]. This naive predictor tool assumes that the conditions at the time of theforecast will not change. For example, if it is sunny and 40o Celsius today, the persistence method predicts that it will be sunny and 40oCelsius tomorrow. The persistence method works well when weather patterns change very little and features on the weather maps move very slowly. It also works well in places like Northeast Brazil, where summer time weather conditions vary little from day to day. The persistence forecast method tends to be used as benchmark against which all other models are compared [5]. The persistence was measured as shown in (4):),()(t y k t y PERS =+(4) Where y PERS (t+k ) is the power generation forecasting for time t+k ; and y (t ) is the measured power generation at timet . In this work, we also used the daily persistence. This model performs the prediction considering that the variable’s future value will be the same as the last one measured 24 hours before.),24()(_−+=+k t y k t y D PERS(5) IV. D EVELOPMENT OF THE F ORECASTING M ODELS In this paper models that perform power generationforecasting using the forecasted wind speeds and the windpower curve of the park are called Wind to Power (W2P)and models that perform power generation forecasting usingthe historical power generation of the park are called Powerto Power (P2P).This paper presents two different models (W2P and P2P)for the forecast of wind power generation with 6 hoursahead, discretized by 10 minutes and two different models(W2P and P2P) for the forecast of wind power generationwith 5 days ahead, discretized by 30 minutes.All of them were developed using Multi-Layer Perceptron(MLP), in accordance with ours previous experience [15]. It is important to note that this form of prediction was used because it is robust and adequate to the problem, as well as due to data quality. A more sophisticated approach could have been used [10], but the database prevented their use at this time.To perform the data handling and the development of the proposed models, MATLAB® software and the Neural Network Toolbox™ Version 6.0.2 (R2009a) were used.A. Models for 6 hours aheadThe Wind to Power forecast model for 6 hours ahead (W2P 6h ) is composed of six ANN, one for each hour. The ANN for the first hour is illustratedFig. 3. The outputs are the six 10min intervals that compose the first hour.Fig. 3. Wind to Power model with forecasting of 6 hours ahead (W2P 6h ).Where: • h – Hour of forecasting, discretized by 10 minutes. • V h-i – Actual average wind speed in the h-i time. Thisbox represents a vector with six values of 10 minutes, where i is the before quantity hours.• P h-i – Actual average active power in the h-i time.This box represents a vector with six values of 10 minutes, where i is the before quantity hours. • V p (l) – Forecasting average wind speed for the l-th output (l = 1, 2,..., 36). • P p (l) -Forecasting average active power for the l-th output (l = 1, 2,..., 36). • CM 1 –Code of the month in which the forecast is being made, first value.• CM 2 –Code of the month in which the forecast is being made, second value.• CH -Code of the forecasting first hour.The Power to Power forecast model for 6 hours ahead(P2P 6h) is composed of six ANN one for each hour. TheANN for the first hour is illustrated in Fig. 4. The outputs are the six 10min intervals that compose the first hour. Observe that the models use two fields to represent the months (CM 1 and CM 2) because they were encoded by sine V h-2V h-3 V h-24V h-25 V h-23CM 1CH CM 1and cosines. The models use the time in decimal value in the range 0 to 1.Fig. 4. Power to Power forecasting model for 6 hours ahead (P2P 6h ).B. Models for 5 days aheadThe Wind to Power forecast model for 5 days ahead (W2P 5d ) and the Power to Power forecast model for 5 days ahead (P2P 5d ) are composed of five ANN, one for each day. Fig. 5Fig. 5 and Fig. 6 show these models. The outputs are the fourth-eight 30min intervals that compose the day. It is important to note that the first 6 hours (or 12 outputs) of these models come from the six hour ahead models (W2P 6h and P2P 6h ). Where:• V pm (h ) –Forecasting average wind speed by numerical model ETA for the hour h (h = 1, 2,..., 120).• V p (l ) -Forecasting average wind speed for the l-th output (l = 1, 2,..., 240).• V (h-i ) - actual average wind speed for the hour h (h = 1, 2,..., 120 ; i = 1, 2, ..., 24).• P p (l ) -Forecasting average active power for the l-th output (l = 1, 2,..., 240).• P (h-i ) -actual average active power for the hour h (h = 1, 2,..., 120 ; i = 1, 2, ..., 24).• CM 1: Code of the month in which the forecast is being made, first value.• CM 2: Code of the month in which the forecast is being made, second value.C. Training of the ANN Forecasting ModelsAll training databases were normalized to lie within the interval [0, 1] and divided in three sets: training set (60%), validation set (20%), and test set (20%).The method for improving generalization and avoid overfitting is called early stopping. This technique is automatically provided for all of the supervised network creation functions in Neural Network Toolbox™ of MATLAB®.Fig. 5. Wind to Power forecasting model for 5 days ahead (W2P 5d ). P h-2 P h-3 P h-24 P h-25 P h-23 CM 1 CHCM 1 V pm 7V pm 24 CM 1 CM 2VV pm 97V pm 120 CM 1CM 2Fig. 6. Power to Power forecasting model for 5 days ahead (P2P 5d ).The ANN models are formed by MLP fully connected neural networks whose training algorithms is Resilient Propagation (RPROP). The ANN architectures consist of an input layer, a hidden layer and an output layer. The number of neurons in the hidden layer was determined by varying the number of neurons in this layer. The amount that provided the best performance during training, in the validation dataset, was thus selected. The hidden layer neurons use the hyperbolic tangent activation function. The number of neurons in the output layer is the same of the number of exits in the model. The neuron activation function used was sigmoid logistics.D. Choosing the Best ANN for Each ModelThe best ANN for each neural model was chosen by 10-fold cross-validation method, where 10 partitions were randomly generated to train, validate and test the ANNs. In this method, the samples are divided into 10 mutually exclusive partitions. For each of 10 iterations, d different partitions are used to test the ANNs and all the others (10 – d) are used to train and validate [15].It is expected that by applying this technique, the mean absolute error value in each experiment on the test set represents the expected output of the ANN created by the 10 experiments, as shown in [16] and [17]. In this paper, the selection of the best ANN was done based in the fold with lower MAE, averaged in ten initializations.V. R ESULTS AND C OMPARISONSThis section presents results and comparisons between W2P 6h , P2P 6h and Pers (persistence) for the 6 hours ahead forecasting and the comparisons between W2P 5d , W2P 5d and Pers_D (diary persistence) for the 5 days ahead forecasting. It’s well known that forecasting accuracy depends on the specific challenges of wind park location, the surrounding terrain, local climatology and data collection. But even so, we will make some comparisons in order to contextualize our work within the state of the art.A. 6 Hours Ahead Wind Power ForecastingThe results (Fig. 7) presented in the simulation period for the park A shows that since the first 10 min interval the P2P 6h model is similar to reference model. The hourly average Fig.8 shows for the first hour identical results between Pers model and P2P 6h model.The W2P 6h model overcomes the Pers model after the third hour. However, never overcomes P2P 6h that is the bestmodel from the second hour, Fig. 8.Fig. 7. NMAE (% of wind farm capacity) of power generation forecasts for Park A . Average of simulation period in intervals of 10min.CM CM 1 CM CM 2As an example a simulation is presented in Fig.9 for the Park A, in which the wind power generation (actual) and models predictions (P2P 6h , W2P h6 and Pers) can becompared.Fig. 8. NMAE (% of wind farm capacity) of power generation forecasts forPark A . Average of simulation period for each hour.Fig. 9. Wind power generation forecasts for Park A. Simulation for February, 01, 2012 at 8h.The Fig. 10 shows the results of the NMAE to the Park B in the simulation period, in which the P2P 6h model has smaller errors from the first to twelfth interval of 10 min. From the second hour W2P 6h model outperforms the otherssee Fig. 11.Fig. 10. NMAE (% of wind farm capacity) of power generation forecasts forPark B . Average of simulation period in intervals of 10min.Fig. 11. NMAE (% of wind farm capacity) of power generation forecasts forPark B . Average of simulation period for each hour.Fig. 12. Wind of power generation forecasts for Park B . Simulation for May, 15, 2012 at 8h.As an example a simulation is presented in Fig. 12 for the Park B, in which the wind power generation (actual) andmodels predictions (P2P 6h , W2P 6h and PERS) can be compared. In Fig. 8 and Fig. 11 we can observed that the average errors for 06 hours ahead is about 15% and 12% for the Park A and Park B, respectively. Noting that the error in the firsthour of 7.83% and 6.39% for Parks A and B, respectively. These results are similar to others as we can be observed in reference [3], [7], [8] and [11].B. 5 Days Ahead Wind Power ForecastingForecasting from 1 to 72 hours ahead, on an hourly basis, were characterized as medium term WPF in [3] and [7]. However, it is not easy to find references to studies with more than 72 hours ahead and discretized in 10 or 30 minutes.As shown in [7], typical model results for the WPF problem up to 48 hours ahead is around 13-16%.WPF models with forecasting of 72 hours ahead, in a 1 hour step, [13] and [14]. Although they use the same database of a 10.2 MW wind farm, they are dealing with many different recurrent ANNs. For reference [13] the best predictor presents a NMAE of 13.0%, while [14] shows a NMAE of 11.0%.The results presented in the simulation period for the Park A (Fig. 13) shows clearly that W2P 5d and P2P 5d models are superior to the model PERS_D in the first 48 intervals of 30 min. After this interval there are intervals in which the PERS_D model presents small error. However, in Fig.14 can be observed that the daily errors for P2P 5d and W2P 5d models are small than the PERS_D model. And the smallest average error, 20.74%, was obtained by the model W2P 5d . In case it is considered only the first three days (72 hours ahead prediction) daily error average falls to 19.00%.Fig. 13. NMAE (% of wind farm capacity) of power generation forecastsfor Park A . Average of simulation period in intervals of 30 min.Fig. 14. NMAE (% of wind farm capacity) of power generation forecastsfor Park A . Average of simulation period for each day.Fig. 15. Wind power generation forecasts for Park A. Simulation on March, 29, 2012 at 9h.As an example a simulation is presented in Fig.15 and Fig.18 for the Park A and B, respectively, in which the wind power generation(actual) and models predictions(P2P 5d , W2P 5d and PERS_D) can be compared and verified the difficulty of carrying out this prediction.Results presented for the simulation period for the park B, Fig. 16, and show that the model W2P 5d has high errors between the intervals 24-50 and 190-205. Fig. 17 shows that the P2P 5d model has small average error. And the smallest average error, 14.48%, was obtained by the model P2P 5d . In case it is considered only the first three days (72 hours ahead prediction) daily error average falls to 13.68%.Fig. 16. NMAE (% of wind farm capacity) of power generation forecasts forPark B . Average of simulation period in intervals of 30 min.Fig. 17. NMAE (% of wind farm capacity) of power generation forecastsfor Park B . Average of simulation period for each day.Fig. 18. Wind power generation forecasts for Park B. Simulation on February, 01, 2012 at 9h.VI. C ONCLUSIONSThe models developed in this paper are based on time series analysis of wind speeds and wind power through computational intelligence. The forecast horizons presented fall within very short-term forecasts for up to six hours ahead and medium-term forecasts up to five days ahead, which is an appropriate horizon to subsidize the operation planning of power systems.All developed models provided good forecasts for all the look-ahead times considered, for the shorter ones (from 1 to 6 hours ahead) and for the medium ones (from 1 to 120 hours ahead)These good forecasts were comparable with the ones of the references presented here.In the case of Park A the model W2P 5d was the best in the average of the day and also for each day. In the case of the Park B the P2P 5d model was the best.R EFERENCES[1] Incentive Program for Alternative Sources of Electric Energy –PROINFA. Available: /elb/data/Pages/LUMISABB61D26PTBRI E.htm[2] The Energy Operation Plan- PEN, National Electric System Operator-ONS. Available:.br/download/avaliacao_condicao/operacao_energe tica/PEN%202012_VOL1_RELATORIO%20EXECUTIVO.pdf[3] H. Giebel, G.; Kariniotakis, G.; Brownsword, R. The State of the artin Short-Term Prediction of Wind Power – A Literature Overview,2003. Available: http://anemos.cma.fr/download/ANEMOS_D1.1_StateOfTheArt_v1.1.pdf[4] Madsen, H. et al. A Protocol for Standardizing the PerformanceEvaluation of Short-term Wind Power Prediction Models, 2003. Available :http://anemos.cma.fr/download/ANEMOS_D2.3_EvaluationProtocol.pdf[5] S. Waston, “Fresh Forecast”, IEE Power Engineer, pp 36-38, 2005. [6] M. Ahlstrom, L. Jones, R. Zavadil, W. Grant, “The future of windforecasting and utility operations,” IEEE Power & Energy Magazine, vol. 3, no. 6, Dec. 2005, pp. 57-64.[7] C. Monteiro, R. Bessa, V. Miranda, A. Botterud, J. Wang, and G.Conzelmann, "Wind Power Forecasting: State-of-the-Art 2009,"ANL/DIS-10-1, Argonne National Laboratory, Nov. 2009. Available:/projects/windpowerforecasting.html[8] Kusiak, A., Haiyang Zheng, Zhe Song, "Short-Term Prediction ofWind Farm Power: A Data Mining Approach",IEEE Transactions on Energy Conversion, vol. 24, no. 1, pp. 125 - 136, Mar. 2009.[9] Catalão, J.P.S., Pousinho, H.M.I., Mendes, V.M.F.,"Hybrid Wavelet-PSO-ANFIS Approach for Short-Term Wind Power Forecasting in Portugal", IEEE Transactions on Sustainable Energy, vol. 2, no. 1, pp. 50 - 59, Jan. 2011.[10] R. R. B. Aquino, H T. V. Gouveia, M. M. S. Lira, A. A. Ferreira, O.Nobrega Neto and M. A. Carvalho Jr., “Wind Forecasting and Wind Power Generation: Looking for the Best Model Based on Artificial Intelligence”, in Proceedings of WCCI 2012 IEEE World Congress on Computational , 2012, Brisbane, Australia, pp. 1– 8.[11] G. Sideratos, and N. D. Hatziargyriou, "An Advanced StatisticalMethod for Wind Power Forecasting," IEEE Transactions on Power Systems, vol. 22, no. 1, pp. 258-265, Feb. 2007.[12] A. A. Ferreira, T. B. Ludermir, R. R. B. Aquino, M. M. S. Lira, O. N.Neto , “Investigating the use of reservoir computing for forecasting the hourly wind speed in short–term”, in Proceedings of InternationalJoint Conference on Neural Networks, 2008, Hong Kong, pp. 1950–1957.[13]T.G. Barbounis and J.B. Theocharis, “Long-term wind speed and power forecasting using local recurrent neural network models,” IEEE Transactions on Energy Conversion,vol. 21, no. 1, pp. 273–284, 2006. [14]T.G. Barbounis and J.B. Theocharis, “Locally recurrent neural networks for long-term wind speed and power prediction,” Neurocomputing, vol. 69, pp. 466–496, 2006.[15]R. R. B. .Aquino, M.M. S. Lira, J. B. Oliveira, M. A. Carvalho Jr., O.Nobrega. Neto, G. J. .Almeida, “Application of wavelet and neural network models for wind speed and power generation forecasting in a brazilian experimental wind park”, in Proceedings of International Joint Conference on Neural Networks, 2009, Atlanta, pp. 172–178. [16] S. Haykin, “Neural networks: A comprehensive foundation”, Prentice Hall: NJ, 2nd ed., 1998.[17]L Prechelt, “Proben1 – A set of neural network benchmark problems and benchmarking rules”, Technical Report, 1994, pp. 21-94.。