Association Rules
Association_Rule

Support: 2/8
Confidence: 1/3
Milk Bread
Support: 2/8 Confidence: 2/3
Frequent Itemsets and Strong Rules
Support and Confidence are bounded by thresholds:
C3 {{1, 2,3}}
24
Lk Ck+1
{X Yk | X , Y Lk , X i Yi , i [1, k 1], X k Yk }
Ordered List
L2 {{1, 2},{2,3}} L2 {{1,3},{2,3}} L2 {{1, 2},{1,3},{2,3}} L2 {{1, 2},{1,3}}
Searching for rules in the form of: Bread Butter
6
7
Support of an Itemset
Itemset
Bread
Butter Chips Jelly
Support
6/8
3/8 2/8 3/8
Itemset
Bread, Butter
Support
Minimum support σ
Minimum confidence Φ A frequent (large) itemset is an itemset with support larger than σ. A strong rule is a rule that is frequent and its confidence is higher than Φ. Association Rule Problem Given I, D, σ and Φ, to find all strong rules in the form of XY. The number of all possible association rules is huge. Brute force strategy is infeasible. A smart way is to find frequent itemsets first.
关于规则的英语作文
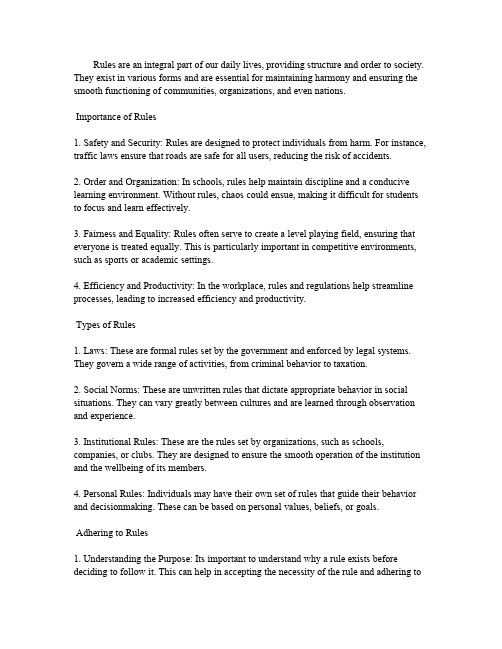
Rules are an integral part of our daily lives,providing structure and order to society. They exist in various forms and are essential for maintaining harmony and ensuring the smooth functioning of communities,organizations,and even nations.Importance of Rules1.Safety and Security:Rules are designed to protect individuals from harm.For instance, traffic laws ensure that roads are safe for all users,reducing the risk of accidents.2.Order and Organization:In schools,rules help maintain discipline and a conducive learning environment.Without rules,chaos could ensue,making it difficult for students to focus and learn effectively.3.Fairness and Equality:Rules often serve to create a level playing field,ensuring that everyone is treated equally.This is particularly important in competitive environments, such as sports or academic settings.4.Efficiency and Productivity:In the workplace,rules and regulations help streamline processes,leading to increased efficiency and productivity.Types of Rulesws:These are formal rules set by the government and enforced by legal systems. They govern a wide range of activities,from criminal behavior to taxation.2.Social Norms:These are unwritten rules that dictate appropriate behavior in social situations.They can vary greatly between cultures and are learned through observation and experience.3.Institutional Rules:These are the rules set by organizations,such as schools, companies,or clubs.They are designed to ensure the smooth operation of the institution and the wellbeing of its members.4.Personal Rules:Individuals may have their own set of rules that guide their behavior and decisionmaking.These can be based on personal values,beliefs,or goals. Adhering to Rules1.Understanding the Purpose:Its important to understand why a rule exists before deciding to follow it.This can help in accepting the necessity of the rule and adhering toit willingly.2.Respecting Authority:Rules are often set by those in authority,and respecting this authority is crucial for maintaining social order.3.Consequences of Noncompliance:Being aware of the potential consequences of breaking a rule can be a strong motivator for compliance.4.Personal Responsibility:Each individual has a responsibility to follow rules,not just for their own benefit but for the benefit of the community as a whole.Challenges with Rules1.Rigidity:Sometimes,rules can be too rigid,not allowing for flexibility in certain situations,which may lead to frustration or conflict.2.Inequality:Rules can sometimes be biased or unfair,favoring certain groups over others,leading to social injustice.plexity:In some cases,the complexity of rules can make it difficult for individuals to understand and comply with them,leading to unintentional rulebreaking.ConclusionWhile rules are necessary for the functioning of society,its also important to periodically review and update them to ensure they remain relevant and fair.As members of society, we have a role to play in both following and contributing to the development of rules that benefit everyone.By doing so,we can create a more harmonious and efficient world.。
数据挖掘方法——关联规则(自己整理)
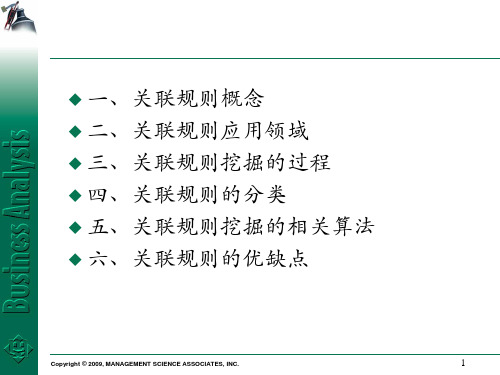
小结:Apriori算法可以分为频繁项集的生成和关联规则的生成两 大步骤;FP-Growth算法可以分成FP-Tree的生成,频繁项集的生成和 关联规则的生成3大步骤。
Copyright © 2009, MANAGEMENT SCIENCE ASSOCIATES, INC.
9
五、关联规则挖掘的相关算法
Copyright © 2009, MANAGEMENT SCIENCE ASSOCIATES, INC.
Copyright © 2009, MANAGEMENT SCIENCE ASSOCIATES, INC.
7
五、关联规则挖掘的相关算法
1.Apriori算法:使用候选项集找频繁项集 Apriori算法是一种最有影响的挖掘布尔关联规则频繁项集的算法。其核心是 基于两阶段频集思想的递推算法。该关联规则在分类上属于单维、单层、布尔关 联规则。在这里,所有支持度大于最小支持度的项集称为频繁项集,简称频集。 该算法的基本思想是:首先找出所有的频集,这些项集出现的频繁性至少和 预定义的最小支持度一样。然后由频集产生强关联规则,这些规则必须满足最小 支持度和最小可信度。然后使用第1步找到的频集产生期望的规则,产生只包含集 合的项的所有规则,其中每一条规则的右部只有一项,这里采用的是中规则的定 义。一旦这些规则被生成,那么只有那些大于用户给定的最小可信度的规则才被 留下来。为了生成所有频集,使用了递推的方法。 Apriori算法可以产生相对较小的候选项目集,扫描数据库的次数由最大频繁 项目集的项目数决定。因此,该算法适合于最大频繁项目集相对较小的数据集中 的关联规则挖掘问题。 Apriori算法的两大缺点:1.可能产生大量的候选集;2.可能需要重复扫描数据库。
关联分析的目的:找出数据库中隐藏的关联网。一般用Support(支 持度)和Confidence(可信度)两个阀值来度量关联规则的相关性,引入 lift(提高度或兴趣度)、相关性等参数,使得所挖掘的规则更符合需求。
公平恪守道德的英语作文

Adherence to morality is a cornerstone of a fair society.In an essay that emphasizes the importance of moral integrity,one might explore various aspects of how moral principles guide our actions and decisions,leading to a just and equitable environment.Title:Upholding Morality for a Fair SocietyIn the pursuit of fairness,morality plays an indispensable role.It is the compass that guides our actions and decisions,ensuring that we treat others with respect and dignity.A society that is grounded in moral principles is one where justice is not just a concept,but a reality that is lived and breathed by its citizens.The Foundation of MoralityMorality is the set of principles or rules that distinguish right from wrong.It is the ethical framework that we use to evaluate our actions and the actions of others.In a fair society, these principles are not just personal beliefs but are collectively agreed upon and upheld by all members of the community.Integrity and TrustIntegrity is a key component of morality.It is the quality of being honest and having strong moral principles.When individuals act with integrity,they build trust within their community.This trust is essential for a fair society,as it fosters cooperation and collaboration,allowing for the equitable distribution of resources and opportunities.Justice and FairnessJustice is the fair and impartial treatment of all members of a society.It is closely linked to morality,as moral principles guide our understanding of what is fair and just.A society that is committed to justice ensures that all individuals have equal access to opportunities and are protected under the law,regardless of their background or status. The Role of EducationEducation is a powerful tool in instilling moral values.Through education,we can teach the principles of fairness,respect,and empathy.By learning about different cultures and perspectives,we can develop a deeper understanding of the importance of treating others with dignity and respect.The Impact of Moral LeadershipLeaders play a crucial role in setting the moral tone of a society.When leaders act with integrity and fairness,they inspire others to do the same.This sets a standard for behavior that permeates throughout the community,creating a culture of moral responsibility and ethical conduct.Challenges and SolutionsDespite the importance of morality,there are challenges in maintaining a fair society. Corruption,discrimination,and inequality can undermine moral principles.To combat these issues,societies must implement strong legal frameworks,promote transparency, and encourage active citizen participation in governance.ConclusionIn conclusion,the adherence to moral principles is essential for creating a fair society.It is through our collective commitment to integrity,justice,and education that we can build a world where fairness is not just an ideal,but a lived experience for all.By embracing our moral responsibilities,we can work towards a future where every individual is treated with the respect and dignity they deserve.。
Top Down FP-Growth for Association Rule Mining

M.-S. Chen, P.S. Yu, and B. Liu (Eds.): PAKDD 2002, LNAI 2336, pp. 334-340, 2002.© Springer-Verlag Berlin Heidelberg 2002Top Down FP-Growth for Association Rule MiningKe Wang, Liu Tang, Jiawei Han, and Junqiang LiuSchool of Computing Science, Simon Fraser University {wangk, llt, han, jliui}@cs.sfu.caAbstract. In this paper, we propose an efficient algorithm, called TD-FP-Growth (the shorthand for Top-Down FP-Growth), to mine frequent patterns.TD-FP-Growth searches the FP-tree in the top-down order, as opposed to the bottom-up order of previously proposed FP-Growth. The advantage of the top-down search is not generating conditional pattern bases and sub-FP-trees, thus,saving substantial amount of time and space. We extend TD-FP-Growth to mine association rules by applying two new pruning strategies: one is to push multiple minimum supports and the other is to push the minimum confidence.Experiments show that these algorithms and strategies are highly effective in reducing the search space.1 IntroductionAssociation rule mining has many important applications in real life. An association rule represents an interesting relationship written as B A ⇒, read as “if A occurs,then B likely occurs”. The probability that both A and B occur is called the support ,and written as count (AB). The probability that B occurs given that A has occurred is called the confidence . The association rule mining problem is to find all association rules above the user-specified minimum support and minimum confidence. This is done in two steps: step 1, find all frequent patterns ; step 2, generate association rules from frequent patterns .This two-step mining approach suffers from several drawbacks. First, only a single uniform minimum support is used, though the distribution of data in reality is not uniform. Second, the two-step process does not consider the confidence constraint at all during the first step. Pushing the confidence constraint into the first step can further reduce search space and hence improve efficiency.In this paper, we develop a family of algorithms, called TD-FP-Growth , for mining frequent patterns and association rules. Instead of exploring the FP-tree in the bottom-up order as in [5], TD-FP-Growth explores the FP-tree in the top-down order. The advantage of the top-down search is not constructing conditional pattern bases and sub-trees as in [5]. We then extend TD-FP-Growth to mine association rules by applying two new pruning strategies: TD-FP-Growth(M) pushes multiple minimum supports and TD-FP-Growth(C) pushes the minimum confidence.2 Related WorkSince its introduction [1], the problem of mining association rules has been the subject of many studies [8][9][10][11]. The most well known method is the Apriori’s anti-monotone strategy for finding frequent patterns [3]. However, this method suffersTop Down FP-Growth for Association Rule Mining 335 from generating too many candidates. To avoid generating many candidates, [4] proposes to represent the database by a frequent pattern tree (called the FP-tree). The FP-tree is searched recursively in a bottom-up order to grow longer patterns from shorter ones. This algorithm needs to build conditional pattern bases and sub-FP-trees for each shorter pattern in order to search for longer patterns, thus, becomes very time and space consuming as the recursion goes deep and the number of patterns goes large.As far as we know, [7][8] are the only works to explicitly deal with non-uniform minimum support. In [7], a minimum item support (MIS) is associated with each item. [8] bins items according to support and specifies the minimum support for combinations of bins. Unlike those works, our specification of minimum support is associated with the consequent of a rule, not with an arbitrary item or pattern.3 TD-FP-Growth for Frequent Pattern MiningAs in [5], TD-FP-Growth first constructs the FP-tree in two scans of the database. In the first scan, we accumulate the count for each item. In the second scan, only the frequent items in each transaction are inserted as a node into the FP-tree. Two transactions share the same upper path if their first few frequent items are same. Each node in the tree is labeled by an item. An I_node refers to a node labeled by item I. For each item I, all I_nodes are linked by a side-link. Associated with each node v is a count, denoted by count(v), representing the number of transactions that pass through the node. At the same time, a header table H(Item, count, side-link) is built. An entry (I, H(I),ptr) in the header table records the total count and the head of the side-link for item I, denoted by H(I) and ptr respectively. Importantly, the items in each transaction are lexicographically ordered, and so are the labels on each path in FP-tree and the entries in a header table. We use Example 3.1 to illustrate the idea of TD-FP-Growth.Example 3.1 A transaction database is given as in the following Figure 3.1. Suppose that the minimum support is 2. After two scans of transaction database, the FP-tree and the header table H is built as Figure 3.1.The top-down mining of FP-tree is described below. First, entry a at the top of H is frequent. Since a_node only appears on the first level of the FP-tree, we just need to output {aFigure 3.1 Transaction table, FP-tree and HThen, for entry b in H, following the side-link of b, we walk up the paths starting from b_node in the FP-tree once to build a sub-header-table for b, denoted H_b.336 Ke Wang et al.These paths are in bold face in Figure 3.2. During the walk up, we link up encountered nodes of the same label by a side-link, and accumulate the count for such nodes. In Figure 3.2, there are two paths starting with b_node: root-b and root-a-b. By walking up these paths, we accumulate the count of the a_node to 1 because path root-a-b only occurs once in the database. We also create an entry a in the sub-header table H_b. The count of entry a is 1 since the count of a is actually the count of pattern {a, b}.a_node is now linked by the side-link for entry a in H_b and the count is modified to 1. Since the minimum support is 2, pattern {a, b} is infrequent. This finishes mining patterns with b as the last item. H_b now can be deleted from the memory. If pattern {a, b} is frequent, we will continue to build sub-header-table H_ab and mine FP-tree recursively. In general, when we consider an entry I in H_x, we will mine all frequent patterns that end up with Ix. In this way, TD-FP-GrowthSimilarly, we find frequent patterns: {c}, {b, c} and {a, c} for entry c, and {e}, {b, e}, {c, e} and {b, c, e} for entry e.Algorithm 1: TD-FP-GrowthInput: a transaction database, with items in each transaction sorted in the lexicographic order, a minimum support: minsup. Output: frequent patterns above the minimum support. Method: build the FP-tree; then call mine-tree (∅, H); Procedure mine-tree(X, H)(1) for each entry I (top down order) in H do(2)if H(I) >= minsup, then(3)output IX;(4)create a new header table H I by call buildsubtable(I);(5)mine-tree(IX, H I);Procedure buildsubtable(I)(1) for each node u on the side-link of I do(2)walk up the path from u once do if encounter a J_node v then(3)link v into the side-link of J in H I;(4)count(v) = count(v) + count(u);(5)H I (J) = H I (J) + count(u);Unlike FP-Growth, TD-FP-Growth processes nodes at upper levels before processing those at lower levels. This is important to ensure that any modificationTop Down FP-Growth for Association Rule Mining 337 made at upper levels would not affect lower levels. Indeed, as we process a lower level node, all its ancestor nodes have already been processed. Thus, to obtain the “conditional pattern base” of a pattern (as named in [5]), we simply walk up the paths above the nodes on the current side-link and update the counts on the paths. In this way, we update the count information on these paths “in place” without creating a copy of such paths. As a result, during the whole mining process, no additional conditional pattern bases and sub-trees are built. This turns out to be a big advantage over the bottom-up FP-Growth that has to build a conditional pattern base and sub-tree for each pattern found. Our experiments confirm this performance gain.4 TD-FP-Growth for Association Rule MiningIn this section, we extend TD-FP-Growth for frequent pattern mining to association rule mining. We consider two new strategies for association rule mining problem: push multiple minimum supports and push the confidence constraint.In the discussion below, we assume that items are divided into class items and non-class items. Each transaction contains exactly one class item and several non-class items. We consider only rules with one class item on the right-hand side. Class items are denoted by C1,…,C m.4.1 TD-FP-Growth(M) for Multiple Minimum SupportsIn a ToyotaSale database, suppose that Avalon appears in fewer transactions, say 10%, whereas Corolla appears in more transactions, say 50%. If a large uniform minimum support, such as 40%, is used, the information about Avalon will be lost. On the contrary, if a small uniform minimum support, such as 8%, is used, far too many uninteresting rules about Corolla will be found. A solution is to adopt different minimum supports for rules of different classes.We adopt TD-FP-Growth to mine association rules using non-uniform minimum supports. The input to the algorithm is one minsup i for each class C i, and the minimum confidence minconf. The output is all association rules X ⇒C i satisfying minsup i and minconf. The algorithm is essentially TD-FP-Growth with the following differences. (1) We assume that the class item C i in each transaction is the last item in the transaction. (2) Every frequent pattern XC i must contain exactly one class item C I, where X contains no class item. The support of X is represented by H(I) and the support of XC i is represented by H(i, I). (3) The minimum support for XC i is minsup i.(4) We prune XC i immediately if its confidence computed by by H(i, I)/H(I) is below the minimum confidence.4.2 TD-FP-Growth(C) for Confidence PruningA nice property of the minimum support constraint is the anti-monotonicity: if a pattern is not frequent, its supersets are not frequent either. All existing frequent pattern mining algorithms have used this property to prune infrequent patterns. However, the minimum confidence constraint does not have a similar property. This is the main reason that most association rule mining algorithms ignore the confidence requirement in the step of finding frequent patterns. However, if we choose a proper338 Ke Wang et al.definition of support, we can still push the confidence requirement inside the search of patterns. Let us consider the following modified notion of support.Definition 4.1: The (modified) support of a rule A ⇒ B is count(A).We rewrite the minimum confidence constraint C: count(AB)/count(A)>=minconf to count(AB)>=count(A)*minconf. From the support requirement count(A)>=minsup, we have a new constraint C’: count(AB) >= minsup * minconf. Notice that C’ is anti-monotone with respect to the left-hand side A. Also, C’ is not satisfied, neither is C. This gives rise to the following pruning strategy.Theorem 4.1: (1) If A ⇒B does not satisfy C’, A ⇒ B does not satisfy the minimum confidence either. (2) If A ⇒ B does not satisfy C’, no rule AX ⇒B satisfies the minimum confidence, where X is any set of items.Now, we can use both the minimum support constraint and the new constraint C’to prune the search space: if a rule A ⇒ B fails to satisfy both the minimum support and C’, we prune all rules AX ⇒ B for any set of items X. In this way, the search space is tightened up by intersecting the search spaces of the two constraints.5 Experiment ResultsAll experiments are performed on a 550MHz AMD PC with 512MB main memory, running on Microsoft Windows NT4.0. All programs are written in Microsoft Visual C++ 6.0. We choose several data sets from UC_Irvine Machine Learning Database Repository: /~mlearn/MLRepository.html.dataset# of trans # of itemsper transclass distribution# of distinctitemsDna-train200061C1=23.2%, C2=24.25%, C3= 52.55%240 Connect-46755743C1=65.83%, C2= 24.62%, C3= 9.55%126Forest58101213C1= 36.36%, C2= 48.76%, C3= 6.15%, C4:0.47%, C5= 1.63%, C6= 2.99%, C7= 3.53%15916 Table 5.1 data sets tableTable 5.1 shows the properties for the three data sets. C onnect-4 is the densest, meaning that there are a lot of long frequent patterns. Dna-train comes the next in density. Forest is relatively sparse comparing with the other two data sets, however, it is a large data set with 581012 transactions.5.1 Frequent Pattern MiningIn this experiment, we evaluate the performance gain of TD-FP-Growth on mining frequent patterns. We compare it with Apriori and FP-Growth. Figure 5.1 shows a set of curves on the scalability with respect to different minimum supports (minsup). These experiments show that TD-FP-Growth is the most efficient algorithm for all data sets and minimum supports tested.Top Down FP-Growth for Association Rule Mining 3395.2 TD-FP-Growth for Multiple Minimum SupportsIn this experiment, we evaluate the performance gain of using multiple minimum supports. We compare three algorithms: TD-FP-Growth(M), TD-FP-Growth(U), and Apriori. TD-FP-Growth(U) is TD-FP-Growth(M) in the special case that there is only a single minimum support equal to the smallest minimum supports specified. To specify the minimum support for each class, we multiply a reduction factor to the percentage of each class. For example, if there are two classes in a data set and the percentage of them is 40% and 60%, with the reduction factor of 0.1, the minimum supports for the two classes are 4% and 6% respectively. And the uniform minimum support is set to 4%.As shown in Figure 5.2, TD-FP-Growth(M) scales best for all data sets. Take C onnect-4 as the example. As we try to mine rules for the rare class draw, which occurs in 9.55% of the transactions, the uniform minimum support at reduction factor of 0.95 is 9.55% * 95%, TD-FP-Growth(U) ran for more than 6 hours and generated more 115 million rules. However, with the same reduction factor, TD-FP-Growth(M) generated only 2051 rules and took only 5 seconds. That’s why in Figure 5.2 for C onnect-4, the run time for the two algorithms with uniform minimum support is not included.5.3 TD-FP-Growth for Confidence PruningIn this experiment, we evaluate the effectiveness of confidence pruning. The results are reported in Figure 5.3. TD-FP-Growth(NC) is TD-FP-Growth(C) with confidence pruning turned off. We fix the minimum support and vary the minimum confidence. As shown in Figure 5.3, TD-FP-Growth(C) is more efficient than Apriori and TD-FP-Growth(NC), for all data sets, minimum supports, and minimum confidences tested.340 Ke Wang et al.Figure 5.3 confidence-pruning results—time comparison6 ConclusionIn this paper, we proposed an efficient algorithm for frequent pattern mining, calledTD-FP-Growth . The main advantage of TD-FP-Growth is not building conditional pattern bases and conditional FP-tree as the previously proposed FP-Growth does.We extended this algorithm to mine association rules by applying two new pruning strategies. We studied the effectiveness of TD-FP-Growth and the new pruning strategies on several representative data sets. The experiments show that the proposed algorithms and strategies are highly effective and outperform the previously proposed FP-Growth .Reference[1] R. Agrawal, T. Imielinski, and A. Swami. Mining association rules between sets of items in large databases. ACM-SIGMOD 1993, 207-216.[2] H. Toivonen. Sampling large databases for association rules. VLDB 1996, 134-145.[3] R. Agrawal and S. Srikant. Mining sequential patterns. ICDE 1995, 3-14.[4] J. Han, J. Pei and Y. Yin. Mining Frequent patterns without candidate generation. SIGMOD 2000, 1-12.[5] R. Srikant and R. Agrawal. Mining generalized association rules. VLDB 1995, 407-419.[6] R. Srikant, Q. Vu, and R. Agrawal. Mining association rules with item constraints. VLDB 1996, 134-145.[7] B. Liu, W. Hsu, and Y. Ma. Mining association rules with multiple minimum supports.KDD 1999, 337-341[8] K. Wang, Y. He and J. Han. Mining frequent patterns using support constraints. VLDB 2000, 43-52.。
R-Agrawalg关于关联规则的开创性论文

X =) Y , where X I, Y I, and X \ Y = .
The rule X =) Y holds in the transaction set D with
con dence c if c% of transactions in D that contain
X also contain Y . The rule X =) Y has support s
An algorithm for nding all association rules, henceforth referred to as the AIS algorithm, was presented in 4]. Another algorithm for this task, called the SETM algorithm, has been proposed in 13]. In this paper, we present two new algorithms, Apriori and AprioriTid, that di er fundamentally from these algorithms. We present experimental results showing
PSarnotcieaegdoi,nCgshoilfe,th1e99240th VLDB Conference
tires and auto accessories also get automotive services done. Finding all such rules is valuable for crossmarketing and attached mailing applications. Other applications include catalog design, add-on sales, store layout, and customer segmentation based on buying patterns. The databases involved in these applications are very large. It is imperative, therefore, to have fast algorithms for this task.
关联规则挖掘AssociationRuleMining背景简介

Brute-force approach:
– List all possible association rules – Compute the support and confidence for each rule – Prune rules that fail the minsup and minconf thresholds Computationally prohibitive!
Apriori principle:
– If an itemset is frequent, then all of its subsets must also be frequent
Apriori principle holds due to the following property of the support measure:
TID Items
– k-itemset
An itemset that contains k items
1 2 3 4 5
Bread, Milk Bread, Diaper, Beer, Eggs Milk, Diaper, Beer, Coke Bread, Milk, Diaper, Beer Bread, Milk, Diaper, Coke
Frequent Itemset Generation
null
A
B
C
D
E
AB
AC
AD
AE
BC
BD
BE
CD
CE
DE
关联规则(associationrule)

关联规则(Association Rules)是数据挖掘领域中的一种重要技术,用于发现数据集中的不显而易见的模式和关系。
它通过分析数据中的项目集合之间的频繁项集,来找出这些项集之间的关联规则,从而揭示数据之间的潜在联系和趋势。
关联规则反映了一个事物与其他事物之间的相互依存性和关联性。
关联规则的应用非常广泛,常见的应用包括购物篮分析。
通过发现顾客放入其购物篮中的不同商品之间的联系,可以分析顾客的购买习惯,从而帮助零售商了解哪些商品频繁地被顾客同时购买。
这种关联的发现有助于零售商制定更有效的营销策略和促销方案。
此外,关联规则还可以应用于价目表设计、商品促销、商品的排放和基于购买模式的顾客划分等领域。
在关联规则分析中,常用的评估标准包括支持度、置信度和提升度。
支持度表示几个关联的数据在数据集中出现的次数占总数据集的比重。
置信度则是指一个数据出现后,另一个数据出现的概率,或者说是数据的条件概率。
提升度则用于衡量关联规则的效果,即使用规则后的效果相对于不使用规则的效果的提升程度。
挖掘关联规则的方法之一是使用Apriori算法。
该算法基于频繁项集的子集也必须是频繁项集的概念,通过迭代的方式生成候选频繁项集,并计算其支持度和置信度,从而找出满足预设阈值的关联规则。
总的来说,关联规则是一种强大的数据挖掘技术,可以帮助企业和研究者从大量数据中发现隐藏的模式和关联,从而制定更有效的决策和策略。