From Artificial Intelligence. 67 (1994), 377-408. JUSTIFICATION AND DEFEAT
ArtificialIntelligence发展为智能进化
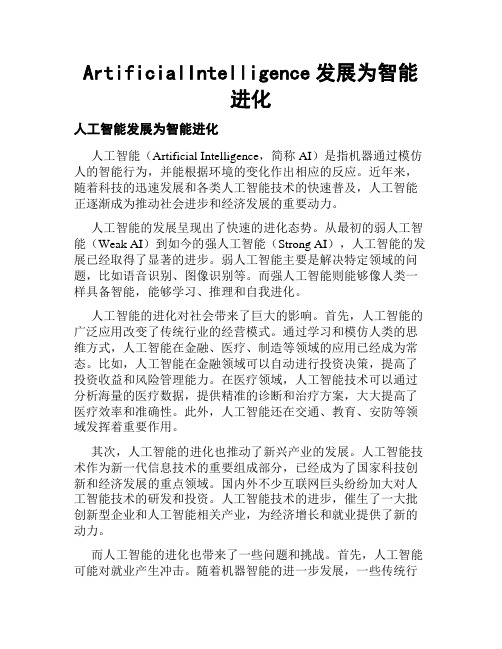
ArtificialIntelligence发展为智能进化人工智能发展为智能进化人工智能(Artificial Intelligence,简称AI)是指机器通过模仿人的智能行为,并能根据环境的变化作出相应的反应。
近年来,随着科技的迅速发展和各类人工智能技术的快速普及,人工智能正逐渐成为推动社会进步和经济发展的重要动力。
人工智能的发展呈现出了快速的进化态势。
从最初的弱人工智能(Weak AI)到如今的强人工智能(Strong AI),人工智能的发展已经取得了显著的进步。
弱人工智能主要是解决特定领域的问题,比如语音识别、图像识别等。
而强人工智能则能够像人类一样具备智能,能够学习、推理和自我进化。
人工智能的进化对社会带来了巨大的影响。
首先,人工智能的广泛应用改变了传统行业的经营模式。
通过学习和模仿人类的思维方式,人工智能在金融、医疗、制造等领域的应用已经成为常态。
比如,人工智能在金融领域可以自动进行投资决策,提高了投资收益和风险管理能力。
在医疗领域,人工智能技术可以通过分析海量的医疗数据,提供精准的诊断和治疗方案,大大提高了医疗效率和准确性。
此外,人工智能还在交通、教育、安防等领域发挥着重要作用。
其次,人工智能的进化也推动了新兴产业的发展。
人工智能技术作为新一代信息技术的重要组成部分,已经成为了国家科技创新和经济发展的重点领域。
国内外不少互联网巨头纷纷加大对人工智能技术的研发和投资。
人工智能技术的进步,催生了一大批创新型企业和人工智能相关产业,为经济增长和就业提供了新的动力。
而人工智能的进化也带来了一些问题和挑战。
首先,人工智能可能对就业产生冲击。
随着机器智能的进一步发展,一些传统行业的工作可能会被自动化取代。
例如,自动驾驶技术的成熟可能会导致大规模的失业。
其次,人工智能还面临着法律和伦理问题。
比如,人工智能技术的使用是否会损害个人隐私?人工智能是否会对社会秩序和道德产生负面影响?这些问题需要社会各界共同关注和解决。
Artificial_Intelligence人工智能(AI)英语演讲

Artificial_Intelligence人工智能(AI)英语演讲Artificial Intelligence: Revolutionizing the FutureIntroduction:Ladies and gentlemen,Today, I stand before you to shed light on one of the most transformative technologies of our time, Artificial Intelligence (AI). It is an honor to deliver this speech on the potential, challenges, and impact of AI in shaping our future. AI, often referred to as the pinnacle of human innovation, carries immense potential to revolutionize various aspects of our lives, from healthcare to transportation, from education to entertainment. With its ever-expanding capabilities, AI has the power to redefine the boundaries of human achievement. So let us delve into the realm of Artificial Intelligence and explore its significance.I. Understanding Artificial Intelligence:Artificial Intelligence is a branch of computer science that focuses on the development of intelligent machines capable of performing tasks that would typically require human intelligence. These tasks encompass problem-solving, learning, speech recognition, decision-making, and much more. AI systems are designed to analyze vast amounts of data, identify patterns, and generate insights, enabling them to emulate human cognitive abilities.II. Applications of Artificial Intelligence:1. Healthcare:AI has the potential to revolutionize healthcare by enabling accurate diagnostics, personalized treatment plans, and drug discovery. Medical professionals can rely on AI systems to analyze patient data, suggest treatment options, and predict disease outcomes. Furthermore, AI-powered robots can assist in surgeries, making procedures more precise and reducing human errors.2. Transportation:The transportation industry is already witnessing the integration of AI technology. Self-driving cars guided by AI algorithms are reducing accidents and improving efficiency. AI also plays a crucial role in optimizing traffic control systems, leading to smoother traffic flow and reduced congestion.3. Education:AI has the power to transform the education sector by personalizing the learning experience for students. Adaptive learning platforms powered by AI algorithms can tailor educational content to individual needs, enhancing engagement and knowledge retention. Additionally, AI-powered chatbots can provide instant support to students, answering their queries and facilitating a seamless learning process.4. Entertainment:AI is revolutionizing the entertainment industry by enabling personalized recommendations, content creation, and virtual reality experiences. Streaming platforms utilize AI algorithms to analyze user preferences andsuggest relevant content. Moreover, AI algorithms can generate music, movies, and art, showcasing the endless possibilities of AI-driven creativity.III. Challenges and Ethical Considerations:While the potential of AI is vast, its development does not come without challenges and ethical considerations. It is essential to address these concerns to ensure that the deployment of AI adheres to ethical standards.1. Job Displacement:AI has raised concerns about job displacement, as automation threatens to replace certain job roles. However, history has shown that technological advancements create new job opportunities as old ones become obsolete. It is crucial to foster a workforce that embraces the changes brought by AI technology through upskilling and reskilling initiatives.2. Data Privacy:AI systems rely heavily on vast amounts of data, raising concerns about data privacy and security. Striking a balance between utilizing data for innovation and safeguarding individuals' privacy rights is of utmost importance. Strict regulations and robust data protection measures are necessary to prevent misuse of personal information.3. Bias and Fairness:AI algorithms are only as unbiased as the data they are trained on. If fed biased or incomplete data, AI systems can perpetuate societal biases. It is crucial to ensure the development of AI systems that are fair, transparent,and accountable. Ethical guidelines and diverse development teams can help address this concern.IV. Conclusion:In conclusion, Artificial Intelligence is an awe-inspiring technology that continues to shape our world. From healthcare to transportation, education to entertainment, the potential of AI knows no bounds. It is essential for us as a society to embrace the opportunities presented by AI while addressing the challenges it poses. By doing so, we can harness the power of AI to create a better and more prosperous future for all.Thank you all for your kind attention.。
人工智能原理MOOC期末考试答案(仅供学习交流)
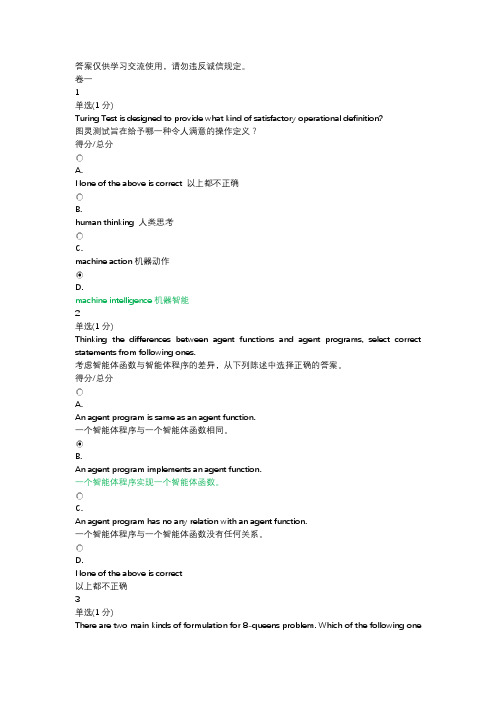
答案仅供学习交流使用,请勿违反诚信规定。
卷一1单选(1分)Turing Test is designed to provide what kind of satisfactory operational definition?图灵测试旨在给予哪一种令人满意的操作定义?得分/总分A.None of the above is correct 以上都不正确B.human thinking 人类思考C.machine action 机器动作D.machine intelligence 机器智能2单选(1分)Thinking the differences between agent functions and agent programs, select correct statements from following ones.考虑智能体函数与智能体程序的差异,从下列陈述中选择正确的答案。
得分/总分A.An agent program is same as an agent function.一个智能体程序与一个智能体函数相同。
B.An agent program implements an agent function.一个智能体程序实现一个智能体函数。
C.An agent program has no any relation with an agent function.一个智能体程序与一个智能体函数没有任何关系。
D.None of the above is correct以上都不正确3单选(1分)There are two main kinds of formulation for 8-queens problem. Which of the following oneis the formulation that starts with all 8 queens on the board and moves them around?有两种8皇后问题的形式化方式。
1-7 Artificial Intelligence(AI)
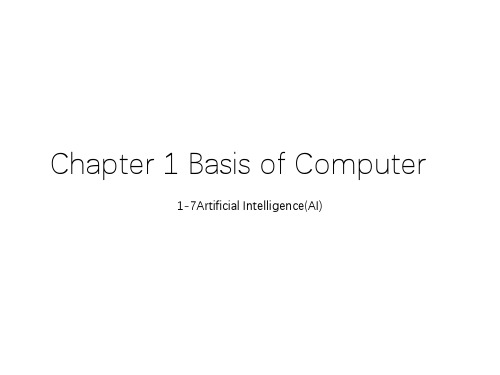
systems that learn new concepts and tasks ,systems that can reason and draw useful conclusions about the world around us, systems that can understand a natural language or perceive and comprehend a visual scene, and systems that perform
确实,在不同地执行某些任 务中它们会实际上超过人的 一些能力。重要的一点是这 些系统都能有效而高效率地 执行智能任务。
Key words: exceed [ɪkˈsi:d] vt. 超过; 超越; 胜过
它具体体现了有意识地和无 意识地通过学习和经验获得 的所有知识和技艺:高度精 确的视觉和听觉感知;思维; 想象;交谈、读、写、驾车、 记忆和回忆事实、表达和感 受情感的能力,以及更多。
Expanded vocabulary: embody [ɪmˈbɒdi] vt. 表现 conscious [ˈkɒnʃəs] adj. 有意识的 refine[rɪˈfaɪn] vt. 提炼; 改善
1-7Artificial Intelligence(AI)
In spite of these impressive achievements,
we still have not been able to produce co-
ordinated, autonomous systems which
1-7Artificial Intelligence(AI)
Can we ever expect to build systems which exhibit these characteristics? The answer to this question is yes! Systems have already been developed to perform many types of intelligent tasks, and expectations are high for near term development of even more impressive systems. We now have systems which can learn from examples, from being told, from past related experiences, and through reasoning.
ARTIFICIAL INTELLIGENCE——人工智能(英文)

ARTIFICIAL INTELLIGENCE——人工智能1 Artificial intelligence (AI) is, in theory, the ability of an artificial mechanism to demonstrate some form of intelligent behavior equivalent to the behaviors observed in intelligent living organisms. Artificial intelligence is also the name of the field of science and technology in which artificial mechanisms that exhibit behavior resembling intelligence are developed and studied.2 The term AI itself, and the phenomena actually observed, invite --- indeed demand --- philosophical speculation about what in fact constitutes the mind or intelligence. These kinds of questions can be considered separately, however, from a description of the various endeavors to construct increasingly sophisticated mechanisms that exhibit “intelligence.”3 Research into all aspects of AI is vigorous. Some concern exists among workers in the field, however, that both the progress and expectations of AI have been overstated. AI programs are primitive when compared to the kinds of intuitive reasoning and induction of which the human brain or even the brains of much less advanced organisms are capable. AI has indeed shown great promise in the area of expert systems --- that is, knowledge-based expert programs --- but while these programs are powerful when answering questions within a specific domain, they are nevertheless incapable of any type of adaptable, or truly intelligent, reasoning.4 Examples of AI systems include computer programs that perform such tasks as medical diagnoses and mineral prospecting. Computers have also been programmed to display some degree of legal reasoning, speech understanding, vision interpretation, natural-language processing, problem solving, and learning. Although most of these systems have proved valuable either as research vehicles or in specific, practical applications, most of them are also still very far from being perfected.5 CHARACTERISTICS OF AI: No generally accepted theories have yet emerged within the field of AI, owing in part to the fact that AI is a very young science. It is assumed, however, that on the highest level an AI system must receive input from its environment, determine an action or response, and deliver an output to its environment. A mechanism for interpreting the input is needed. This need has led to research in speech understanding, vision, and natural language. The interpretation must be represented in some form that can be manipulated by the machine.6 In order to achieve this goal, techniques of knowledge representation are invoked. The AI interpretation of this, together with knowledge obtained previously, ismanipulated within the system under study by means of some mechanism or algorithm. The system thus arrives at an internal representation of the response or action. The development of such processes requires techniques of expert reasoning, common-sense reasoning, problem solving, planning, signal interpretation, and learning. Finally, the system must网construct an effective response. This requires techniques of natural-language generation.7 THE FIFTH-GENERATION ATTEMPT: In the 1980s, in an attempt to develop an expert system on a very large scale, the Japanese government began building powerful computers with hardware that made logical inferences in the computer language PROLOG. (Following the idea of representing knowledge declaratively, the logic programming PROLOG had been developed in England and France. PROLOG is actually an inference engine that searches declared facts and rules to confirm or deny a hypothesis. A drawback of PROLOG is that it cannot be altered by the programmer.) The Japanese referred to such machines as “fifth-generation” computers.8 By the early 1990s, however, Japan had forsaken this plan and even announced that they were ready to release its software. Although they did not detail reasons for their abandonment of the fifth-generation program, U.S scientists faulted their efforts at AI as being too much in the direction of computer-type logic and too little in the direction of human thinking processes. The choice of PROLOG was also criticized. Other nations were by then not developing software in that computer language and were showing little further enthusiasm for it. Furthermore, the Japanese were not making much progress in parallel processing, a kind of computer architecture involving many independent processors working together in parallel—a method increasingly important in the field of computer science. The Japanese have now defined a “sixth-generation” goal instead, called the Real World Computing Project, that veers away from the expert-systems approach that works only by built-in logical rules.9 THE FUTURE OF AI RESEARCH: One impediment to building even more useful expert systems has been, from the start, the problem of input---in particular, the feeding of raw data into an AI system. To this end, much effort has been devoted to speech recognition, character recognition, machine vision, and natural-language processing. A second problem is in obtaining knowledge. It has proved arduous toextract knowledge from an expert and then code it for use by the machine, so a great deal of effort is also being devoted to learning and knowledge acquisition.10 One of the most useful ideas that has emerged from AI research, however, is that facts and rules (declarative knowledge) can be represented separately from decision-making algorithms (procedural knowledge). This realization has had a profound effect both on the way that scientists approach problems and on the engineering techniques used to produce AI systems. By adopting a particular procedural element, called an inference engine, development of an AI system is reduced to obtaining and codifying sufficient rules and facts from the problem domain. This codification process is called knowledge engineering. Reducing system development to knowledge engineering has opened the door to non-AI practitioners. In addition, business and industry have been recruiting AI scientists to build expert systems.11 In particular, a large number of these problems in the AI field have been associated with robotics. There are, first of all, the mechanical problems of getting a machine to make very precise or delicate movements. Beyond that are the much more difficult problems of programming sequences of movements that will enable a robot to interact effectively with a natural environment, rather than some carefully designed laboratory setting. Much work in this area involves problem solving and planning.12 A radical approach to such problems has been to abandon the aim of developing “reasoning” AI systems and to produce, instead, robots that function “reflexively”. A leading figure in this field has been Rodney Brooks of the Massachusetts Institute of Technology. These AI researchers felt that preceding efforts in robotics were doomed to failure because the systems produced could not function in the real world. Rather than trying to construct integrated networks that operate under a centralizing control and maintain a logically consistent model of the world, they are pursuing a behavior-based approach named subsumption architecture.13 Subsumption architecture employs a design technique called “layering,”---a form of parallel processing in which each layer is a separate behavior-producing network that functions on its own, with no central control. No true separation exists, in these layers, between data and computation. Both of them are distributed over the same networks. Connections between sensors and actuators in these systems are kept short as well. The resulting robots might be called “mindless,” but in fact they have demonstrated remarkable abilities to learn and to adapt to real-life circumstances.14 The apparent successes of this new approach have not convinced many supporters of integrated-systems development that the alternative is a valid one for drawing nearer to the goal of producing true AI. The arguments that have arisen between practitioners of the two different methodologies are in fact profound ones. They have implications about the nature of intelligence in general, whether natural or artificial。
The-Moral-Dilemmas-of-Artificial-Intelligence
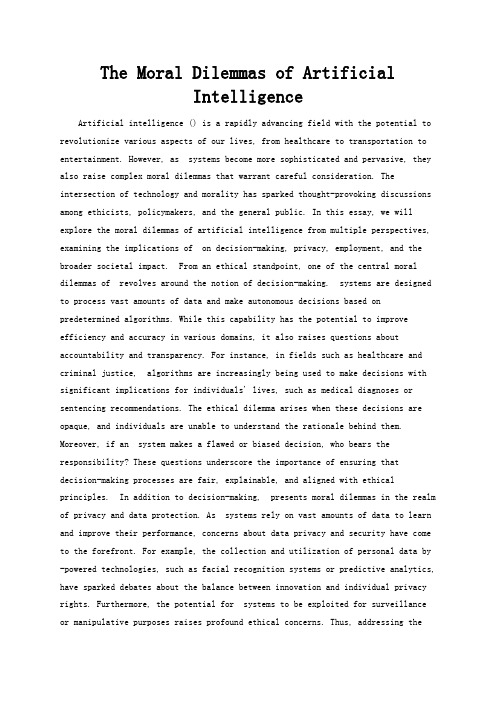
The Moral Dilemmas of ArtificialIntelligenceArtificial intelligence () is a rapidly advancing field with the potential to revolutionize various aspects of our lives, from healthcare to transportation to entertainment. However, as systems become more sophisticated and pervasive, they also raise complex moral dilemmas that warrant careful consideration. The intersection of technology and morality has sparked thought-provoking discussions among ethicists, policymakers, and the general public. In this essay, we will explore the moral dilemmas of artificial intelligence from multiple perspectives, examining the implications of on decision-making, privacy, employment, and the broader societal impact. From an ethical standpoint, one of the central moral dilemmas of revolves around the notion of decision-making. systems are designed to process vast amounts of data and make autonomous decisions based on predetermined algorithms. While this capability has the potential to improve efficiency and accuracy in various domains, it also raises questions about accountability and transparency. For instance, in fields such as healthcare and criminal justice, algorithms are increasingly being used to make decisions with significant implications for individuals' lives, such as medical diagnoses or sentencing recommendations. The ethical dilemma arises when these decisions are opaque, and individuals are unable to understand the rationale behind them. Moreover, if an system makes a flawed or biased decision, who bears the responsibility? These questions underscore the importance of ensuring that decision-making processes are fair, explainable, and aligned with ethical principles. In addition to decision-making, presents moral dilemmas in the realm of privacy and data protection. As systems rely on vast amounts of data to learn and improve their performance, concerns about data privacy and security have come to the forefront. For example, the collection and utilization of personal data by -powered technologies, such as facial recognition systems or predictive analytics, have sparked debates about the balance between innovation and individual privacy rights. Furthermore, the potential for systems to be exploited for surveillance or manipulative purposes raises profound ethical concerns. Thus, addressing themoral dilemmas of in the context of privacy entails establishing robust regulations and ethical guidelines to safeguard individuals' privacy rights while fostering technological advancement. Moreover, the rise of has prompted discussions about its impact on employment and the future of work. While proponents of argue that it can automate repetitive tasks and free up human workers to focus on more creative and complex endeavors, skeptics raise concerns about job displacement and the potential erosion of meaningful work opportunities. The moral dilemma lies in reconciling the potential societal benefits of -driven automation with the potential consequences for individuals whose livelihoods maybe disrupted. Ensuring a fair transition to an -augmented workforce and addressing the ethical implications of job displacement require a careful examination oflabor policies, education and training programs, and social safety nets. Furthermore, the broader societal impact of raises profound moral dilemmas concerning equity, justice, and the distribution of benefits and risks. As technologies proliferate, there is a risk that they may exacerbate existing disparities and privilege certain groups over others. For instance, biased algorithms can perpetuate discriminatory outcomes in areas such as lending, hiring, and law enforcement, amplifying social inequities. Additionally, the geopolitical implications of , including concerns about autonomous weapons and geopolitical competition, add another layer of moral complexity. Addressing these dilemmas necessitates a holistic approach that prioritizes equity, diversity, and inclusion in development and deployment, as well as international cooperation and ethical frameworks to mitigate potential harms. In conclusion, the moral dilemmas of artificial intelligence encompass a wide range of complex ethical considerations, from decision-making and privacy to employment and societal impact. As continues to advance and integrate into various facets of our lives, it is essential to confront these dilemmas through an ethical lens, placing human values and well-being at the center of development and deployment. By engaging inmultidisciplinary dialogue, involving stakeholders from diverse backgrounds, and proactively shaping policies and norms, we can navigate the moral complexities of and steer its trajectory toward beneficial outcomes for humanity. Ultimately, the ethical stewardship of requires a collective commitment to upholding ethicalprinciples and ensuring that technology serves the common good. In crafting this essay, I aimed to present a comprehensive exploration of the moral dilemmas of artificial intelligence, drawing on diverse perspectives and ethical considerations. I endeavored to emulate a human response by incorporating emotional elements and conveying the urgency of addressing these dilemmas in a thoughtful and empathetic manner. As continues to evolve, it is imperative to engage in ongoing ethical discourse and decision-making to navigate the complex terrain of ethics and steer technological innovations toward positive societal impact.。
人工智能伦理 英译本
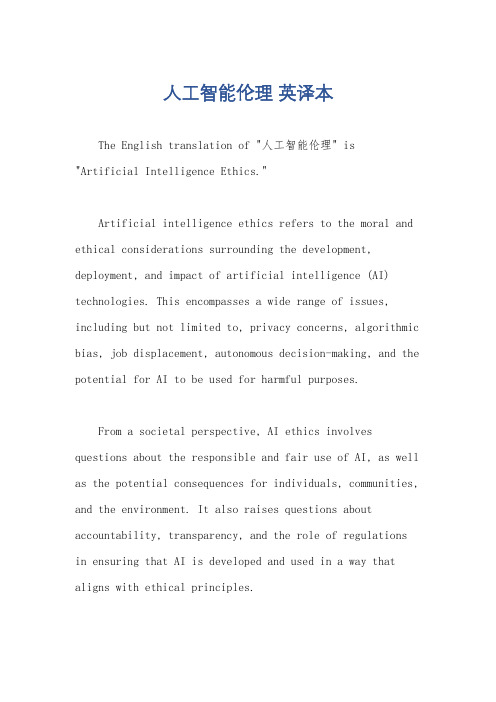
人工智能伦理英译本The English translation of "人工智能伦理" is"Artificial Intelligence Ethics."Artificial intelligence ethics refers to the moral and ethical considerations surrounding the development, deployment, and impact of artificial intelligence (AI) technologies. This encompasses a wide range of issues, including but not limited to, privacy concerns, algorithmic bias, job displacement, autonomous decision-making, and the potential for AI to be used for harmful purposes.From a societal perspective, AI ethics involves questions about the responsible and fair use of AI, as well as the potential consequences for individuals, communities, and the environment. It also raises questions about accountability, transparency, and the role of regulationsin ensuring that AI is developed and used in a way that aligns with ethical principles.From a technical perspective, AI ethics involves considerations about the design and implementation of AI systems, including how to ensure that they are aligned with human values, how to mitigate biases, and how to make AI systems transparent and understandable to users.Overall, the field of artificial intelligence ethics is complex and multifaceted, requiring careful consideration of a wide range of ethical, legal, and societal implications. It is an important area of study and discussion as AI technologies continue to advance and become more integrated into various aspects of our lives.。
Introduction+to+Artificial+Intelligence+in+English
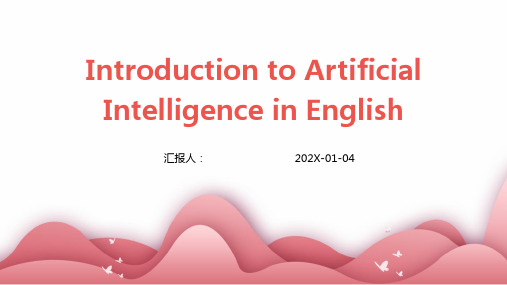
Environmental perception: AI technology can recognize and process environmental information around vehicles, such as road signs, vehicles, pedestrians, etc.
Game recommendation system
AI can recommend suitable games and game content to players based on their gaming behavior and preferences.
The Challenges and Future Development of Artistic Intelligence
Intelligent control: AI technology can achieve intelligent control of household equipment, such as lighting, air conditioning, doors and windows, etc.
Security Threats
AI systems can create moral challenges, such as ethical decisions in autonomous vehicles or medical applications where human lives are at stake
Lake of Clear Legal Framework
Computational complexity
AI systems often face computational challenges due to the complexity of the algorithms and the large amounts of data required for training
- 1、下载文档前请自行甄别文档内容的完整性,平台不提供额外的编辑、内容补充、找答案等附加服务。
- 2、"仅部分预览"的文档,不可在线预览部分如存在完整性等问题,可反馈申请退款(可完整预览的文档不适用该条件!)。
- 3、如文档侵犯您的权益,请联系客服反馈,我们会尽快为您处理(人工客服工作时间:9:00-18:30)。
1
1. Introduction
The purpose of this paper is to exhibit some problematic cases of defeasible or nonmonotonic reasoning that tend to be handled incorrectly by all of the theories of defeasible and nonmonotonic reasoning in the current literature, and then to propose a way of dealing with these cases. The paper will focus on my own argument-based approach to defeasible reasoning,1 together with default logic2 and circumscription.3 The problems, however, are general ones that seem to recur within all extant theories. I will assume a familiarity with default logic and circumscription. By contrast with either, my own argument-based approach to defeasible reasoning emphasizes that the subject is reasoning. For present purposes, the most convenient representation of arguments is as graphs, where the nodes represent steps of inference. I will call these inference graphs. A simple example is given in figure 1. The exact structure of the arguments and the nodes will not be important for the purposes of this paper. For instance, we can accommodate suppositional reasoning (conditionalization, reasoning by cases, reductio ad absurdum, etc.) by incorporating suppositions into the nodes, but whether we do that is not germane to this paper.4 (P&Q ) P (Q&P ) Figure 1. An inference graph. When a reasoner reasons, it is natural to regard it as producing a number of separate arguments aimed at supporting different conclusions. But we can also combine all of the reasoning into a single inference graph that records the overall state of the reasoner’s inferences, showing precisely what inferences have been made and how inferences are based upon one another. This comprehensive inference graph can provide the central data structure used in evaluating a reasoner’s beliefs. Accordingly, we can think of the function of reasoning to be that of building the inference graph. The inference relations between nodes of the inference graph are recorded in inference links. Where ν and η are nodes, 〈ν,η〉 is an inference link iff ν was inferred (in one step) The most recent formulation of this theory is in [16,17,18]. It has been developed over a period of twenty five years. See also [7,8,9,10,12,13,15]. 2 See particularly [19] and particularly [5] and [6]. For more on suppositional reasoning, see [16]. 2
from a set of nodes one of which was η. If 〈ν,η〉 is an inference link then η is an immediate inference ancestor of η. A reasoner might construct more than one argument supporting a single conclusion. It will simplify the discussion if we keep the inference links embodied in the different arguments separate. That can be done by having a different node for each argument. Nodes and their inference links then become unambiguous. This will allow us to regard the different nodes as defeated by different defeaters. An inference branch is a finite sequence of nodes each of which is an immediate inference ancestor for the next. Let us say that η is an inference ancestor of ν iff there is an inference branch connecting η to ν. Justified beliefs are those mandated by the rules for belief updating. What an agent is justified in believing is a function of both what input premises have been supplied and how far the agent has gotten in its reasoning. A necessary condition for a belief to be jusitified is that the agent has engaged in reasoning that produced an argument supporting the belief, but that is not a sufficient condition because the agent may also have produced an argument that defeats the first argument. Justification is defeasible in the sense that a belief may be justified at one time and become unjustified later as a result of further reasoning producing a new argument that defeats the first. This leads to a distinction between justification and warrant, where the latter is “justification in the limit”, i.e., justification when all possible relevant reasoning has been completed.5 This paper will not be concerned with warrant, but rather with the question of what beliefs are justified at any given time given the current state of the agent’s inference graph. The agent’s reasoning is encoded in the inference graph, the nodes of which correspond to inferences. Defeat relations can also be encoded in the inference graph by introducing a new set of links. Where µ and ν are nodes of the inference graph, 〈µ,ν〉 is a defeat link iff ν defeats µ. These defeat relations will result in some of the nodes being defeated and others undefeated. A justified belief is one corresponding to an undefeated node of the inference graph. To complete this account, we need a characterization of the circumstances under which a node is defeated or undefeated. The defeat status of a node is a function of its defeat relations to other nodes. The theory that will be proposed here will be independent of the exact nature of these defeat relations, but for illustrative purposes I will sketch my preferred account. According to that account, reasoning proceeds by constructing arguments, where reasons provide the atomic links in arguments. Conclusive reasons are deductive reasons, and they logically entail their conclusions. Defeasibility arises from the fact that not all reasons are conclusive. Those that are not are prima facie reasons. Prima facie reasons create a presumption in favor of their conclusion, but it is defeasible. I will encode a reason as an ordered pair 〈Γ,p〉, where Γ is the set of premises of the reason and p is the conclusion. I will occasionally refer to the premises as a “reason for” the conclusion. Considerations that defeat prima facie reasons are defeaters. There are two importantly different kinds of defeaters. Where P is a prima facie reason for Q, R is a rebutting defeater iff R is a reason for denying Q . All work on nonmonotonic logic and defeasible reasoning has recognized the existence of rebutting defeaters, but it has sometimes been overlooked that there are other defeaters