Questions_Answers_Webinar_SAMA5D4_internal_July_21
Answer英语答案

Answer英语答案习题参考答案第1章填空题:1. 计算理论、计算机、信息处理2. 算法设计和程序设计3. 有限性、可执⾏性、机械性、确定性、终⽌性4. 有确定的算法5. ⼆进制6. 在给定相同的输⼊时,A和B有相同的输出、A和B计算等价7. 存储器8. 电⼦管和继电器9. 光计算机、⽣物计算机、量⼦计算机10. 巨型化、微型化、⽹络化、智能化11. ASCII码,712. 213. bmp, jpg14. ⽂字15. 时间和幅值16. ⽂件,数据库17. ⽩盒,⿊盒18. 是⼀种⽆处不在的计算模式简答题:1.简述计算机采⽤⼆进制的原因。
(1)⼆进制只有0和1两个基本符号(2)⼆进制的算术运算规则简单,且适合逻辑运算。
2. 图灵机模型主要由哪4个部分组成?⼀条⽆限长的纸带,⼀个读写头,⼀套控制规则,⼀个状态寄存器。
3.图灵机在形式上可以⽤哪七个元素描述?它们分别表⽰什么含义?(参考教材p7回答) 4.图灵机模型中的四个要素是什么?输⼊信息,输出信息、程序(规则)、内部状态。
5.简述图灵机的⼯作过程。
图灵机的⼯作过程可以简单的描述为:读写头从纸带上读出⼀个⽅格中的信息,然后根据它内部的状态对程序进⾏查表(规则表Table),得出⼀个输出动作,确定是向纸带上写信息还是使读写头向前或向后移动到下⼀个⽅格。
同时,程序还会说明下⼀时刻内部状态转移到哪⾥。
6. 简述问题求解的⼀般过程。
需求分析,系统(模型)设计,编码与调试,系统测试。
7 .简述基于计算机的信息处理的⼀般过程。
信息采集,信息的表⽰和压缩,信息存储和组织,信息传输,信息发布,信息检索。
8. 简述⾼性能计算机涉及的主要关键技术。
答:软硬件技术、通信技术、纳⽶技术第2章1.计算机系统主要由(硬件系统)和(软件系统)组成。
2.说明以下计算机中的部件是属于主机系统、软件系统、还是属于外部设备。
(1)CPU (主机系统)(2)内存条(主机系统)(3)⽹卡(主机系统)(4)键盘和⿏标(外设)(5)显⽰器(外设)(6)Windows操作系统(软件系统)3.控制芯⽚组是主板的的核⼼部件,它由(北桥芯⽚)部分和(南桥芯⽚)部分组成。
学术英语理工类课后题答案
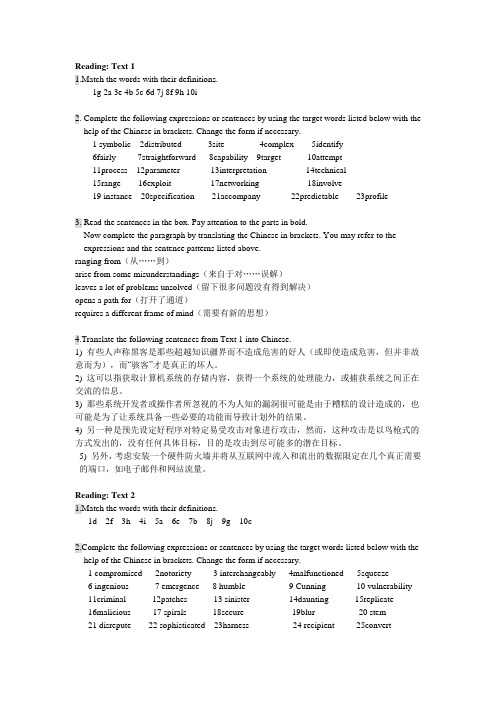
Reading: Text 11.Match the words with their definitions.1g 2a 3e 4b 5c 6d 7j 8f 9h 10i2. Complete the following expressions or sentences by using the target words listed below with the help of the Chinese in brackets. Change the form if necessary.1 symbolic 2distributed 3site 4complex 5identify6fairly 7straightforward 8capability 9target 10attempt11process 12parameter 13interpretation 14technical15range 16exploit 17networking 18involve19 instance 20specification 21accompany 22predictable 23profile3. Read the sentences in the box. Pay attention to the parts in bold.Now complete the paragraph by translating the Chinese in brackets. You may refer to the expressions and the sentence patterns listed above.ranging from(从……到)arise from some misunderstandings(来自于对……误解)leaves a lot of problems unsolved(留下很多问题没有得到解决)opens a path for(打开了通道)requires a different frame of mind(需要有新的思想)4.Translate the following sentences from Text 1 into Chinese.1) 有些人声称黑客是那些超越知识疆界而不造成危害的好人(或即使造成危害,但并非故意而为),而“骇客”才是真正的坏人。
关于网上搜索答案我的看法英语作文
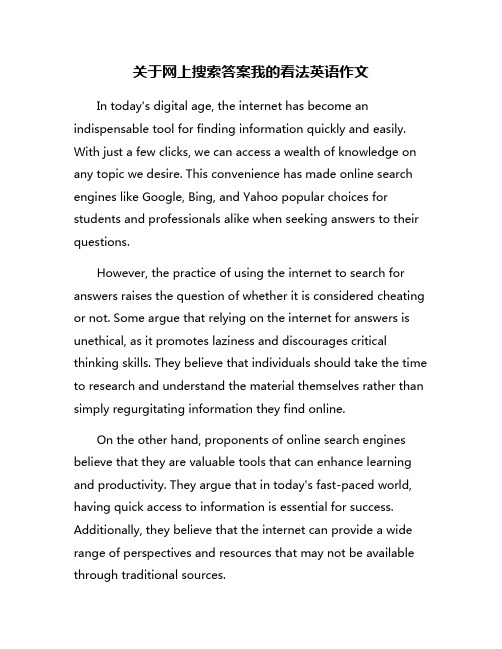
关于网上搜索答案我的看法英语作文In today's digital age, the internet has become an indispensable tool for finding information quickly and easily. With just a few clicks, we can access a wealth of knowledge on any topic we desire. This convenience has made online search engines like Google, Bing, and Yahoo popular choices for students and professionals alike when seeking answers to their questions.However, the practice of using the internet to search for answers raises the question of whether it is considered cheating or not. Some argue that relying on the internet for answers is unethical, as it promotes laziness and discourages critical thinking skills. They believe that individuals should take the time to research and understand the material themselves rather than simply regurgitating information they find online.On the other hand, proponents of online search engines believe that they are valuable tools that can enhance learning and productivity. They argue that in today's fast-paced world, having quick access to information is essential for success. Additionally, they believe that the internet can provide a wide range of perspectives and resources that may not be available through traditional sources.In my opinion, using the internet to search for answers is not inherently good or bad. It ultimately depends on how the information is used. If individuals are using the internet to supplement their learning and gain a deeper understanding of a topic, then it can be a valuable tool. However, if people are relying solely on the internet for answers without critically evaluating the information, then it can be detrimental to their learning and development.As a student, I have found online search engines to be incredibly helpful in my academic pursuits. They have allowed me to access a wide range of resources and perspectives that have enriched my learning experience. However, I also recognize the importance of critical thinking and analysis in evaluating the information I find online. It is essential to verify the credibility of sources and consider multiple viewpoints before drawing conclusions.In conclusion, while online search engines can be valuable tools for finding information quickly and easily, it is important to use them responsibly. It is crucial to supplement online research with critical thinking skills and independent analysis to ensure a well-rounded understanding of a topic. By striking a balance between using the internet as a resource and developing one'sown critical thinking skills, individuals can harness the full potential of online search engines while fostering intellectual growth and development.。
关于上网搜索答案这一现象英语作文
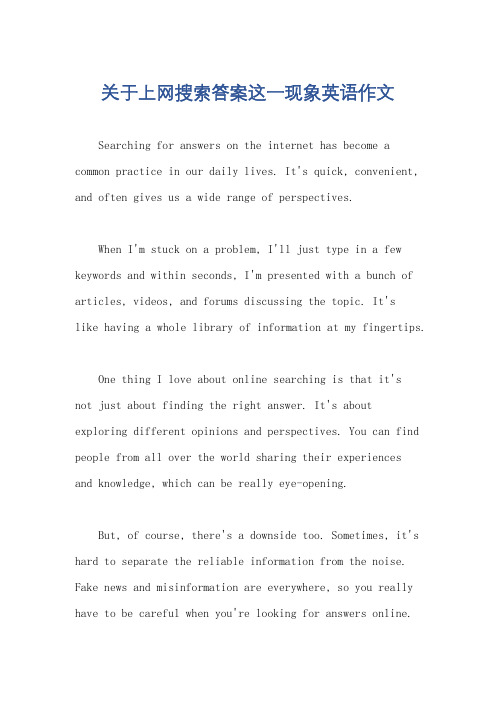
关于上网搜索答案这一现象英语作文Searching for answers on the internet has become a common practice in our daily lives. It's quick, convenient, and often gives us a wide range of perspectives.When I'm stuck on a problem, I'll just type in a few keywords and within seconds, I'm presented with a bunch of articles, videos, and forums discussing the topic. It'slike having a whole library of information at my fingertips.One thing I love about online searching is that it'snot just about finding the right answer. It's aboutexploring different opinions and perspectives. You can find people from all over the world sharing their experiencesand knowledge, which can be really eye-opening.But, of course, there's a downside too. Sometimes, it's hard to separate the reliable information from the noise. Fake news and misinformation are everywhere, so you really have to be careful when you're looking for answers online.Overall, though, I think the internet has made it easier for us to access knowledge and learn new things. We can find answers to almost any question, anytime, anywhere. It's a pretty amazing tool, if you use it wisely.。
网上搜答案之我见英语作文
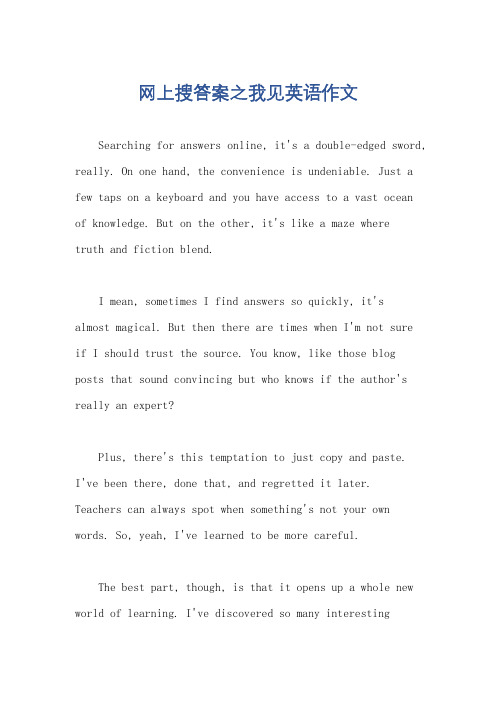
网上搜答案之我见英语作文Searching for answers online, it's a double-edged sword, really. On one hand, the convenience is undeniable. Just a few taps on a keyboard and you have access to a vast oceanof knowledge. But on the other, it's like a maze wheretruth and fiction blend.I mean, sometimes I find answers so quickly, it'salmost magical. But then there are times when I'm not sureif I should trust the source. You know, like those blog posts that sound convincing but who knows if the author's really an expert?Plus, there's this temptation to just copy and paste.I've been there, done that, and regretted it later. Teachers can always spot when something's not your own words. So, yeah, I've learned to be more careful.The best part, though, is that it opens up a whole new world of learning. I've discovered so many interestingtopics and perspectives just by browsing through forums and discussions. It's like a never-ending adventure.But I also think it's important to remember that online isn't the only source of knowledge. There's still a placefor books, libraries, and real-life experts. After all, nothing beats having a conversation with someone who's passionate about a subject.So in conclusion, searching for answers online is great, but it should be done with caution. Don't be afraid to.。
大一英语ismart测试答案

大一英语ismart测试答案1、---Excuse me sir, where is Room 301?---Just a minute. I’ll have Bob ____you to your room. [单选题] *A. show(正确答案)B. showsC. to showD. showing2、We have made a _______ tour plan to Sydney. [单选题] *A. two dayB. two daysC. two-day(正确答案)D. two-days3、Where have you _______ these days? [单选题] *A. been(正确答案)B. beC. isD. are4、--Can I _______ your dictionary?--Sorry, I’m using it. [单选题] *A. borrow(正确答案)B. lendC. keepD. return5、Seldom _____ in such a rude way. [单选题] *A.we have been treatedB. we have treatedC. have we been treated(正确答案)D. have treated6、The street was named _____ George Washington who led the American war for independence. [单选题] *A. fromB. withC. asD. after(正确答案)7、5 He wants to answer the ________ because it is an interesting one. [单选题] *A.problemB.question(正确答案)C.doorD.plan8、—______ is it from your home to the bookstore?—About 15 kilometers.()[单选题] *A. How far(正确答案)B. How muchC. How longD. How many9、Boys and girls, _______ up your hands if you want to take part in the summer camp(夏令营).[单选题] *A. puttingB. to putC. put(正确答案)D. puts10、Jim is a(n) _______. He is very careful and likes to work with numbers. [单选题] *A. secretaryB. tour guideC. accountant(正确答案)D. English teacher11、Mary's watch is more expensive than _____. [单选题] *A. Susan's(正确答案)B. that of Susan'sC. that of SusanD. Susan12、I can’t hear you _______. Please speak a little louder. [单选题] *A. clearly(正确答案)B. lovelyC. widelyD. carelessly13、—When are you going to Hainan Island for a holiday? —______ the morning of 1st May.()[单选题] *A. InB. AtC. On(正确答案)D. For14、He didn't allow _____ in his room. Actually he didn't allow his family _____ at all. [单选题] *A. to smoke; to smokeB. smoking; to smoke(正确答案)C. to smoke; smokingD. smoking; smoking15、97.Go ______ the square and you will find the theatre. [单选题] *A.aboveB.atC.across(正确答案)D.on16、Jim, it’s dark now. Please _______ the light in the room. [单选题] *A. turn on(正确答案)B. turn upC. turn offD. turn down17、16.We asked ______ engineer we met before to help repair the radio yesterday. [单选题] *A.aB.anC.the(正确答案)D./18、He doesn’t smoke and hates women _______. [单选题] *A. smokesB. smokeC. smokedD. smoking(正确答案)19、Sam is going to have the party ______ Saturday evening. ()[单选题] *A. inB. on(正确答案)C. atD. to20、73.The moonlight goes ____ the window and makes the room bright. [单选题] * A.acrossB.through(正确答案)C.overD.in21、6.—How can we get to the school?—________ bus. [单选题] *A.ToB.OnC.By(正确答案)D.At22、27.My father is a professor and he works in__________ university. [单选题] * A.a (正确答案)B.anC./D.the23、—______?—He can do kung fu.()[单选题] *A. What does Eric likeB. Can Eric do kung fuC. What can Eric do(正确答案)D. Does Eric like kung fu24、He _______ maths. [单选题] *A. does well in(正确答案)B. good atC. is well inD. does well at25、We had a(an)_____with him about this problem last night. [单选题] *A.explanationB.impressionC.exhibitionD.discussion(正确答案)26、The hall in our school is _____ to hold 500 people. [单选题] *A. big enough(正确答案)B. enough bigC. very smallD. very big27、Kids will soon get tired of learning _____ more than they can. [单选题] *A. if they expect to learnB. if they are expected to learn(正确答案)C. if they learn to expectD. if they are learned to expect28、The man called his professor for help because he couldn’t solve the problem by _______. [单选题] *A. herselfB. himself(正确答案)C. yourselfD. themselves29、He _______ walks to school, because he lives near school. [单选题] *A. sometimes(正确答案)B. neverC. doesn’tD. don’t30、I will _______ at the school gate. [单选题] *A. pick you up(正确答案)B. pick up youC. pick you outD. pick out you。
Learning to Extract Answers in Question Answering Experimental Studies

ABSTRACT.Question Answering(QA)systems are complex programs able to answer a question in natural language.Their source of information is a given corpus or,as assumed here,the Web.To achieve their goal,these systems perform various subtasks among which the last one, called answer extraction,is very similar to an Information Extraction task.The main objective of this study it to adapt machine learning techniques defined for Information Extraction tasks to the slightly different task of answer extraction in QA systems.The specificities of QA sys-tems are identified and exploited in this adaptation.Three algorithms,assuming an increasing abstraction of natural language texts,are tested and compared.RÉSUMÉ.Les systèmes Question/Réponse sont des programmes complexes capables de répondre àune question en langage naturel,en utilisant comme source d’information soit un corpus donné,soit,comme c’est le cas ici,le Web.Pour cela,ces systèmes réalisent différentes sous-tâches parmi lesquelles la dernière,appelée extraction de la réponse,est très similaireàune tâche d’Extraction d’Information.L’objectif de cet article est d’adapter les techniques d’ap-prentissage automatique utilisées en Extraction d’Informationàl’extraction de la réponse.Les spécificités des systèmes Question/Réponse sont identifiées et utilisées dans cette adaptation. Trois algorithmes utilisant une abstraction croissante du texte sont testés et comparés. KEYWORDS:Question Answering,Machine Learning,Information ExtractionMOTS-CLÉS:Systèmes Question-Réponse,Extraction d’information,Apprentissage automatique1.This research was partially supported by:“CPER2000-2006,Contrat de Planétat-région Nord/Pas-de-Calais:axe TACT,projet TIC”;fonds européens FEDER“TIC-Fouille Intelli-gente de données-Traitement Intelligent des Connaissance”OBJ2-phasing out-2001/3-4.1 -n3.2.www.grappa.univ-lille3.fr2CORIA’05.1.IntroductionQuestion answering(QA)systems are complex systems that,given a question asked in natural language,canfind an answer to this question,in a corpus or in the Web,and justify it by quoting their source(s).From the user’s point of view,they can be considered as an improvement over traditional search engines such as Google or AltaVista because they provide a more direct and precise access to the desired information.The counterpart is thatfinding the correct answer to a question requires much more analysis and processing than a typical search engine.Traditionally,a Question Answering system is divided into three steps:question analysis,passage retrieval and answer extraction.Our focus will be on the last task (answer extraction)which can be compared with an Information Extraction(IE)task. The purpose of IE is to automaticallyfill a database from a corpus of texts in natural language or semi-structured data from the Web.IE is an active research area in which many improvements have been made recently.Among them,we are particularly in-terested in machine learning techniques and techniques that deal with internet data sources[SOD99,KUS02,CAR04].Our objective is to improve the answer extraction task in QA systems on the Web by using insights provided by recent machine learning techniques developed for IE. Some specificities of QA systems can be useful here.As a matter of fact,in QA systems,thefirst steps of the process give hints that can help the answer extraction. Unlike in IE,we can take advantage of the outcomes of these steps.We focus our study on using common outcomes of the question analysis step:the class of the ques-tion and the keyword(s).To summarize,in this paper,we experimentally study how to use machine learning techniques developed for IE in answer extraction,taking into account QA systems specificities.We propose several ways to adapt existing IE algo-rithms,mainly differing by the document representation they adopt.We have built a dataset to evaluate and analyze several alternatives.This dataset if freely available on.Our ex-periments deal with various encodings and three machine learning algorithms.The first algorithm,suggested by[RA V02],applies on raw text.It relies on a document representation that is a sequence of token values(a token is either a word or a punc-tuation symbol)and builds extraction patterns by searching for the largest common subsequences of tokens present around the answer.RAPIER,defined by[CAL98], is the second tested algorithm.It exploits deeper information about the documents, including part of speech tags,and elaborates complex extraction rules using both to-ken values and POS tags.The third one is PAF developed in the Mostrare group [MAR04].PAF relies on supervised classification and is veryflexible from the docu-ment representation point of view.In our experiments with PAF,token values are not used but only token types(POS tag,punctuation,case,...)and simple numerical fea-tures computable from the texts(lengths,etc.).These three algorithms are worth being compared because they make very different assumptions on how to represent a text inLearning to Extract Answers in QA3 natural language.The representations used correspond to three levels of abstraction. For each algorithm,we also study the influence of using the keyword information.The next section in an overview of QA systems.We present our experiments and our analysis in Section3.2.Overview of Question Answering SystemsAlthough thefirst works in this researchfield go back to the1960s and1970s with the works of[GRE61],[WOO73]and[LEH78],Question Answering systems became popular in the late1990s.This emergence was encouraged by the TREC-8 conference in1999,where a QA track[VOO99]was initiated and where thefirst large-scale evaluation of such systems took place.This evaluation led to a consen-sus on the general architecture of a QA system.Now,every QA system performs three successive tasks:question analysis,information retrieval and answer extraction. Although we will mainly focus on the third one,we introduce each of them and the assumptions made at each step,to justify our own choices.We also briefly review previous attempts to introduce machine learning techniques in QA systems.2.1.Question AnalysisFirst,an analysis of the question is performed,its goal being to extract the infor-mation needed to perform the next two steps from the question.In all systems,the result is at least a classification of the question into a class.The set of possible classes is predefined,and ranges from few basic sets only depending on thefirst word of the question(“where”,“when”,etc.)to veryfine sets of hierarchical classes.But this information alone is not precise enough to directly allow to answer the question.So,another goal of this task is usually to extract words giving crucial in-formation about the subject of the question.The notion of“focus”is often employed. We prefer to use the term keywords.A keyword is a word or a sequence of words. The number of keywords to be extracted from a question depends on its class.For example,in the question“When was W.A.Mozart born?”,the question class can be and the only keyword needed is“W.A.Mozart”.So,the classis of arity1,meaning that only one keyword must be given with the class to specify a unique answer.This very precise class could easily be replaced by a less precise one,for example,but with two keywords:“W.A.Mozart”and“born”.In the following,the assumption is made that the couple(question class,keywords) is a synthetic representation of the question and that this representation is necessary and sufficient to correctly answer the question.Some systems keep more precise a track of the initial question,such as AskMSR[BRI02],including syntactic features. We do not pretend that a syntactic analysis is useless,for example to distinguish“Who killed Lee Harvey Oswald?”from“Who did Lee Harvey Oswald kill?”.But the difference between both sentences can be reflected in different class assignments.The4CORIA’05.first question’s representation could be(,Lee Harvey Oswald)while the second one’s could be(,Lee Harvey Oswald).These couples(questionclass,keywords)are the essential QA-specific data that will be used to perform the next steps.rmation RetrievalThen,QA systems perform an IR task whose objective is to build a limited corpus of relevant documents,i.e.portions of texts in which the answer is very likely to befound.The purpose of this step is to reduce the search space of the next step.Selected passages must be long enough to contain the answer and some useful context.Butwhen using the Web as a corpus,which is the case here,it is impossible to apply NLP techniques on the corpus beforehand.Therefore,the selected passages should not be too long,to be able to perform various NLP techniques such as POS tagging without dramatically increasing processing time.2.3.Answer ExtractionFinally,the last task to be performed is the extraction of all candidate answers from the set of extracted passages.Due to the difficulty of the whole QA task,the candidate answers are then ranked to get the top5answers.This task is very similar to the one of Information Extraction,which consists infilling a database(whose structure is known)from natural language texts.In answer extraction,the answer can be considered as the(unique)piece of data to be extracted,and the question class represents its type.Document representation plays a critical role in Information Extraction,hence alsoin answer extraction.Texts are usually considered as sequences of tokens,a token being either a word or a punctuation symbol.POS tags are often added to each token, and sometimes even syntactic structures are taken into account[LIG04].Externalresources like named entity recognizers or semantic information(such as Wordnet) can also be exploited[POI03].In our study,only POS tags and simple numerical descriptions computable from the texts(length,number of occurrences,etc.)will beused,in order to have a reasonable computation time.An important question to consider is the representation of the keyword itself insidethe passage.It is intuitively efficient to abstract it,that is to forget its value and replace it by either a type or a description that indicates“a part of text that containsthe keyword”.For example,in the question“When was Mozart born?”,the question keyword is“Mozart”.Abstracting it by a tag“ ”in documents allows to generate extraction rules like“ was born in ”rather than“Mozart was born in ”.This seems much better because it is more generic,as it is correct for the other questions of the same class.One of our purposes is to test theefficiency of this abstraction.Learning to Extract Answers in QA5 The extraction itself is usually performed by hand-made patterns which describe the possible contexts in which the answer can occur.A set of patterns is associated with every question class.And,of course,the patterns themselves often include the abstracted keyword.But the extraction cannot always be described in terms of patterns.One of the techniques we use is based on decision trees.Similarly,some question answering sys-tems,such as Javelin[NYB03],choose among different approaches(SVM,statistical extraction,patterns,etc.)depending on the question class in order to maximize their system’s performances.Finally,to rank the candidate answers,a confidence score is calculated.The score of a candidate answer can rely on its frequency,on its type(using a named entity tagger),as in[ABN00].This score can also rely on the proximity of the keyword(s), but this won’t be the case in the following.2.4.Machine Learning and QA systemsIt is only recently that several attempts to introduce machine learning techniques to help process some of the tasks of a QA system have appeared.The advantages of introducing such techniques are obvious.The building of hand-made patterns used in question analysis and answer extraction tasks is long and fastidious.Learning them automatically would certainly be easier.Furthermore,employing learning techniques is the best way to automatically adapt a QA system to some specificities,such as:the language used(hand-made patterns are language-specific)and the domain of special-ity(some patterns may also be specific to a domain)for specialized corpora.Most of previous works trying to combine machine learning and QA focused on thefirst steps,for example on learning to automatically classify a question,or learn-ing to locate and expand the keywords in the question in order to generate queries [USU04].To our knowledge,thefirst attempt to learn answer extraction patterns was the one of[RA V02],using alignment learning with suffix trees.But,we have seen that the answer extraction task shares many features with the IE task.In this domain,several machine learning techniques have been proposed.Our purpose is to adapt some of them to answer extraction,and to analyze their efficiency in this context.3.ExperimentsThe main objective of this section is to use IE learning algorithms for answer ex-traction,and to evaluate the interest of using the keyword in those algorithms to make them more QA oriented.We will use three radically different IE learning algorithms. To evaluate the performances of the extraction rules learnt for each question class by these algorithms,we build a data set from documents provided by Google.We adapt6CORIA’05.the protocol proposed by[RA V02]by making clearer the evaluation protocol,intro-ducing QA cross-checking,in the spirit of standard machine learning cross validation.3.1.Building the corpusAs we did not develop a complete QA system,the question analysis step is not implemented but only simulated.The experiments we make are based on only two predefined classes:and,both of arity1.The inputs of our programs are thus couples(question class,keyword),which are supposed to correctlyrepresent the initial questions.is supposedly an easy question class be-cause of its very specific aspect,whereas is considered more difficult,since it includes localisation of towns,sites,lakes,etc.For each class,we choose100distinct keywords.Each keyword is associated witha list of all its valid answers,called Answers.For example,the question(,“Danube”)has several valid answers,such as"Hungary","Austria",etc.Input:the set S of keyword/Answers couples.1:Corpus=∅2:for each couple(keyword,Answers)in S do3:Documents=top100documents retrieved by Google containing both keyword and at least one answer∈Answers4:for each document in Documents do5:for each answer in Answers do6:Let s be the smallest passage containing both keyword and answer7:Let s2be s with surrounding context(50characters)from document 8:tokenize(s2)9:Add s2to Corpus10:end for11:end for12:end forOutput:CorpusLearning to Extract Answers in QA7 a valid answer are selected,leaving some context(50characters)before and after this passage.For example,if the text is“the great composer Ludwig V on Beethoven was born in1770in Bonn,Germany.”,the selected passage is something like“great composer Ludwig V on Beethoven was born in1770in Bonn”(the context is in italics).The next step consists in tokenizing these passages to match our document rep-resentation.A document is considered as a sequence of tokens.As already stated,a token is either a word or a punctuation symbol.Spaces,tabulations,line feed,etc.are not considered as tokens but as token delimiters and hence are not taken into account.The opportunity to realize abstraction of the keyword as presented in Section2.3 leads us to build two versions of each corpus.Finally,document preparation as well as the way to use the keyword differ with the choice of the learning algorithm.3.2.Evaluation protocol:QA cross-checkingIn order to evaluate the performance of the rules learnt by each tested algorithm, with or without using the keyword,we define a new evaluation protocol,inspired by machine learning cross-validation,and independent of the learning algorithm.Because of the way we built the learning corpus,it is mandatory to build a new separate testing corpus.Indeed,if we separated the corpus built as described in the previous section into two distinct parts,a learning corpus and a testing corpus,a typ-ical cross validation technique could be applied.But this corpus is exclusively made of passages containing both the keyword and a valid answer.So,the results obtained would necessarily differ from the ones obtained in a real QA process,where the pas-sages extracted in the information retrieval step are selected without knowing the an-swer,and hence contain the keyword but not necessarily a valid answer.To avoid this cheat,a new testing corpus must be built.Building the testing corpus is quite similar to building the learning corpus.We only change two aspects of algorithm1.First,for each question,we send Google a query consisting of the keyword alone.Second,the keyword is used to retrieve small passages.In each document,the selected passages arefixed-sized windows around all the occurrences of the keyword.The window size is clearly a parameter to adjust. Roughly,larger is the window,better is the recall but worst is the precision.We have made experiments with several window sizes that confirm this intuition.The results presented here are for a window size of200characters before and after the keyword.Finally,the complete evaluation protocol is given in algorithm2.We have two corpora,the learning corpus L and the testing corpus T,built from a set S of100 (keyword,Answers)couples c0to c99.In both corpora,each passage is associated to a couple.Hence,each corpus is divided into10parts:l0to l9for the learning corpus,and t0to t9for the testing corpus,each part i containing passages associated to10couples c10i to c10i+9.This way we build a partition of our corpora.Then10 iterations are performed.In each iteration k,extraction rules are learnt on L\l k,and8CORIA’05.Input:S={c j|j∈[0,99]},the set of keyword/Answers couples.L,the learning corpus.T,the testing corpus.1:Build the partition of L={l i|i∈[0,9]},where l i is the set of passages of L corresponding to the keyword/Answers couples c10i to c10i+92:Build the partition of T={t i|i∈[0,9]},where t i is the set of passages of T corresponding to the keyword/Answers couples c10i to c10i+93:for i from0to9do4:Rules=Learn(L\l i).5:for each c j represented in t i do6:Apply Rules on the passages of t i corresponding to c j.7:Rank the extracted candidate answers.8:RR j=Reciprocal Rank of the correct answer.9:end for10:end forOutput:MRR=Mean of the RR j2.The MRR is themean of these scores.To evaluate whether an extracted answer is correct,we perform an“exact match”evaluation,i.e.an extracted answer is considered correct if it is iden-tical to the correct answer.For example,if the expected answer is"Paris","paris"will be considered as correct,but"in Paris"will not.Moreover,for each question,only the best ranked correct answer counts.For example,if the expected correct answers are "Paris"or"France",and the system has extracted"Paris","Lille"and"France",the correct extracted answer"France"won’t count since"Paris"has also been extracted and ranked higher.3.2.1.StatisticsTable1shows statistics on our learning and testing corpora.Every passage of the learning corpus contains both the keyword and at least one correct answer,whereas every passage of the testing corpus contains the keyword and hopefully the answer,but it is not compulsory.In each iteration of our evaluation with questions, we learn on approximately1900annotated passages and test on appromimately2900 passages,while with questions,we learn on3000annotated passages and test on2000passages.Learning to Extract Answers in QA9 We can also notice that only93questions have at least one correspond-ing passage in the testing corpus containing the correct answer.Therefore,7questions will not be answered in the answer extraction step.3374207275546one passage with a correct answer100/100Algorithm3Alignment Learning Algorithm10CORIA’05.To take advantage of the keyword in this algorithm,we use the method introduced by[RA V02],which differs from the algorithm in Fig3on two points.First,the key-word is abstracted.It is replaced by the tag in the corpus and only frequent subsequences containing both the tags and are kept.The second difference concerns the pattern ranking.Instead of measuring the precision on the learning corpus,we measure it on the corresponding part of the testing corpus.This means the precision is measured on passages associated with the same questions as the learning corpus,but containing the keyword and not necessarily the answer(c.f.3.1).These two changes make the algorithm more adapted to a QA system.3.3.2.Extraction algorithmBoth with and without using the keyword,once the rules have been applied on the documents,we have a list of all the occurrences of the candidate answers along with their confidence score,which is the precision of the pattern which extracted it.To rank these candidate answers,we compute a score for each candidate answer which is the sum of the confidence scores of all the occurrences of this candidate answer,as shown in Fig4.For example,if the candidate answer“1756”has been extracted twice with the score0.5and0.3,the score of“1756”is0.8.Input:List of answers along with their confidence score.1:for each(answer,confidence)do2:add confidence to score answer3:end for4:Sort the answers according to score answerOutput:Ordered list of the candidate answers.MRR#Answers0.45234/93used63/1000.513Table2.Results using Alignment Learning with Suffix Trees.Column#Answers givesthe number of times at least one of the correct answers belongs to the top5answers are considered incorrect because they contain the exact birth date and not just thebirth year,for example"January20,1946"instead of"1946",against5with the rules learnt without using the keyword.Considering these answers correct brings the MRRto0.672with the keyword information and0.475without.Moreover,with questions and the rules learnt with the keyword infor-mation,we notice that,in most cases,when a correct answer has been extracted but isnot in the top5answers,which happens13times,all the answers ranked higher are just noise and none of them are dates,not even numbers.This particular case showsthe main weakness of these patterns.Indeed,they are based on raw text only and donot provide any information concerning the answer to be extracted.Adding informa-tion about the answer would help solve this problem.For example,withquestions,the POS tag could tell that the answer must be a number.Overall,looking at the patterns makes it easy to explain why those learnt using thekeyword perform better on both question classes.Indeed,both on and on questions,when the keyword is not used,the algorithm learns patterns that are very precise but overly specific.Most of the patterns contain the value of the key-word,for example“Adams( ”or“Hitchcock( ”with questions,and“Parthenon, ”or“Balaton, ”with ques-tions.Since the keyword is not abstracted,these patterns are too specific and cannot be used to answer different questions.When the keyword is abstracted,the patterns are more general and the previous patterns are replaced by“ ( ”for questions and“ , ”for questions.3.4.Experiments with RAPIER3.4.1.AlgorithmThe second approach consists in using the IE system RAPIER[CAL98]to learn answer extraction patterns and extract the answers.RAPIER’s patterns have a more abstract view of texts.Indeed,not only do they use the value of the token,but they also use its POS tag.These patterns are sequences of tokens on which there are two types of constraint,each constraint being a disjunction of possible values.Thefirst constraints are word constraints and concern the value of the token.For example,if the constraint is a list of the words a,b and c,then the token must be one of these threewords.The second constraints are called Part-Of-Speech constraints and indicate which POS tag the token must have.Having these two constraints allows the system to generalize his patterns and,for example,learn a pattern saying that a token must have the POS tag“NN”(noun)without explicitly giving its value.The reader is reported to[CAL98]for further explanation on how RAPIER works.Since RAPIER does not give a confidence score for each candidate answers,we cannot rank the candidate answers as we did with alignment learning.But RAPIER’s patterns set is ordered,so we rank our candidate answers accordingly to the rank of the pattern that extracted them.For example,if“1756”is extracted by rule1and“1845”by rule3,the best candidate answer is“1756”.3.4.2.DataSet Pre-processingTo meet RAPIER requirements,we had to add POS tags to our passages.We used Brill’s POS tagger[BRI92]to do so.To take advantage of the keyword information, we also abstracted it by replacing all its occurrences by the tag .3.4.3.ResultsMRR#Answers0.49110/93used74/1000.085Table3.Results using RAPIER.Column#Answers gives the number of times at least one of the correct answers belongs to the top5answersTable3shows the results.Thefirst thing to notice is that RAPIER does not per-form well on questions.Indeed,whether the keyword information is used or not,the results are equally poor,meaning it is totally independent of the keyword. The problem in this case is that RAPIER never generalizes on the answer to extract, i.e.in all the patterns it learns,the word constraints on the tokens to be extracted are lists of the answers encountered during the learning process.Therefore,the patterns are far too specific to the questions in the learning corpus,and hence cannot be used to answer other questions.Here,RAPIER may need more examples to learn on in order to perform a proper generalization and learn more efficient patterns.Oppositely,on questions,the system performs well and using the key-word improves the performances.For example,whether the keyword is used or not, RAPIER is able to learn a pattern saying that the answer is a number,preceded by a “(”and followed by a“-”,as in“Mozart(1756-1791”.But when using the keyword, RAPIER is also able to learn that the token preceding the“(”is precisely the keyword, whereas when the keyword is not abstracted,RAPIER is at best able to learn that this token is a proper noun.Unfortunately,this is the only generalized pattern produced by RAPIER in our experiments.All the other patterns are over specific for the samereason as with questions.Once again,a larger set of examples may have helped RAPIER to learn more general patterns.But providing larger sets of examples is difficult for some question classes and may be expensive.3.5.Experiments with PAF3.5.1.AlgorithmThe third learning algorithm we used is PAF[MAR04].This algorithm’s ap-proach is very different from the previous two algorithms.Indeed,it does not learn patterns,but classifiers.The passages of our corpora being tokenized,a separator is defined as the position between two successive tokens.Thus,to identify the correct answer,we need to identify its start separator and its end separator.Separators are represented as an attribute-valued vector.PAF learns to identify separators using su-pervised classification.The classifiers learnt are readable decision trees produced by Quinlan’s C5.To rank the candidate answers given by PAF,a score for each candidate answer is computed the same way as was done with alignment learning.3.5.2.DataSet Pre-processingFor the classifier to be able to effectively learn how to classify the separators,we had to choose a document representation,i.e.a list of attributes.In our experiments, we considered the following attributes for each token:–the Part-Of-Speech tag(Brill’s POS tagger[BRI92]was used);–an attribute saying whether the token is a word,a number or a punctuation sym-bol.This is a generalization over the Part-Of-Speech tag;–the case of the token:allCaps,lowercase,UpperInitial or LowerInitial;–the length of the token(i.e.its number of characters).Note that this document representation is an abstraction of the initial text,as it does not use the string value of the tokens.Hence,unlike RAPIER,PAF cannot learn lists of the answers encountered in the learning corpus.To use the keyword,we could not abstract it since the string values of the tokens are not considered.Therefore,we added an attribute that represents the distance between the keyword and the answer.For example in the passage“Mozart(1756-”the distance is1token.The order in which the keyword and the answer appear is also represented. In our example,the keyword is before the answer.3.5.3.ResultsTable4shows the results,which outperform the other ones.On the one hand,with questions,the system performs very well and the keyword attribute helps improve the results.At the root of the decision tree learnt without using the keyword attribute,one can read that the answer must be a number of length4,which is not。
自然语言处理_Question-Answer Dataset(试题答案数据集)
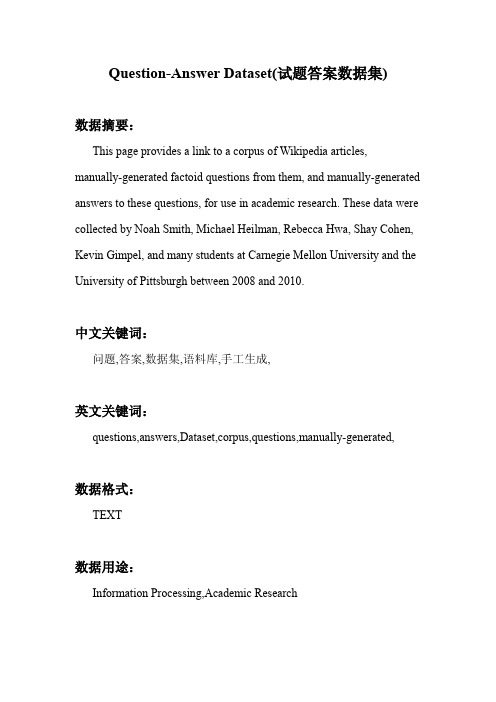
Question-Answer Dataset(试题答案数据集)数据摘要:This page provides a link to a corpus of Wikipedia articles, manually-generated factoid questions from them, and manually-generated answers to these questions, for use in academic research. These data were collected by Noah Smith, Michael Heilman, Rebecca Hwa, Shay Cohen, Kevin Gimpel, and many students at Carnegie Mellon University and the University of Pittsburgh between 2008 and 2010.中文关键词:问题,答案,数据集,语料库,手工生成,英文关键词:questions,answers,Dataset,corpus,questions,manually-generated,数据格式:TEXT数据用途:Information Processing,Academic Research数据详细介绍:Question-Answer DatasetThis page provides a link to a corpus of Wikipedia articles, manually-generated factoid questions from them, and manually-generated answers to these questions, for use in academic research. These data were collected by Noah Smith, Michael Heilman, Rebecca Hwa, Shay Cohen, Kevin Gimpel, and many students at Carnegie Mellon University and the University of Pittsburgh between 2008 and 2010.DownloadManually-generated factoid question/answer pairs with difficulty ratings from Wikipedia articles. Dataset includes articles, questions, and answers.Version 1.1 released August 6, 2010README.v1.1; Question_Answer_Dataset_v1.1.tar.gzArchived ReleasesVersion 1.0 released February 18, 2010README.v1.0; Question_Answer_Dataset_v1.0.tar.gzFurther ReadingPlease cite this paper if you write any papers involving the use of the data above: Question Generation as a Competitive Undergraduate Course ProjectNoah A. Smith, Michael Heilman, and Rebecca HwaIn Proceedings of the NSF Workshop on the Question Generation Shared Task and Evaluation Challenge, Arlington, VA, September 2008.AcknowledgmentsThis research project was supported by NSF IIS-0713265 (to Smith), an NSF Graduate Research Fellowship (to Heilman), NSF IIS-0712810 and IIS-0745914 (to Hwa), and Institute of Education Sciences, U.S. Department of Education R305B040063 (to Carnegie Mellon).数据预览:点此下载完整数据集。
- 1、下载文档前请自行甄别文档内容的完整性,平台不提供额外的编辑、内容补充、找答案等附加服务。
- 2、"仅部分预览"的文档,不可在线预览部分如存在完整性等问题,可反馈申请退款(可完整预览的文档不适用该条件!)。
- 3、如文档侵犯您的权益,请联系客服反馈,我们会尽快为您处理(人工客服工作时间:9:00-18:30)。
Q&A Session for Intro to SAMA5D4Date: Monday, July 21, 2014________________________________________________________________‐roland Joeres (roland.joeres@) ‐ 12:30 AMQ: Do we support Hardware OpenGL2.0? In my understanding this is needed for Qt5.A: We do not support a OpenGL2.0 stack as we don’t have a GPU to accelerate. Qt5 has new graphic features that uses the OpenGL2.0. We are checking what limitation we will have on our platform without this 3D acceleration.________________________________________________________________‐Willi Buehler (willi.buehler@) ‐ 12:35 AMQ: SAMA5D31 price 5,40$ to SAMA5D4x 7,50$ is 8% increase??? please check your calculator!!!A: This is an error in the currency. The SAMA5D31 is 5.40€ (= 6.95$) not 5.40$. The increase is 8% based on DBC pricing compare to D31.________________________________________________________________‐Matt Wood (matt.wood@) ‐ 8:07 AMQ: Can you elaborate on the ECC co‐processor?A: This question has been answered verbally.‐ 2 slides presentation of the algorithms supported is available. This has been sent to Matt by e‐mail.________________________________________________________________‐Matt Wood (matt.wood@) ‐ 8:08 AMQ: What is the status of ARM TrustZone support?A: This question has been answered verbally.‐ We have investigated the porting a Software hypervisor on top of the ARM TrustZone. The cost is extremely high so there is no firm offer from Atmel until we have a serious business opportunity.________________________________________________________________‐Kevin Vap (kevin.vap@) ‐ 8:10 AMQ: Is the ITTIAM codec & NEON comparisons done with or without the video decoder?A: This question has been answered verbally.‐ They are doing tests on ITTIAM codes for encoding a H264 video stream using Neon. The Vdec is not used during this operation.________________________________________________________________‐Ray Barth (ray.barth@) ‐ 8:10 AMQ: Will there be future consideration for a smaller package. A 14x14 or 16x16 package is too large for many of my customer requirements. To be chosen on a large customer platform, a smaller package as a minimum requirement.A: What are your customer’s application ? In our roadmap we will adress application requiering small form factor (and lox profile) with Cortex A5 device (+ neon, L2 cache but NO video). Release next year. This would probably be a better fit. Otherwise please let me know opportunity size and technical requierements________________________________________________________________‐Carlos Marciales (carlos.marciales@) ‐ 8:10 AMQ: Will you support the HW decoder with OS support (Android and Linux)? You have not done a very good job of supporting the HW decoder in the 9M10.A: We will support the HW video decoder with our free Linux distribution. We are focusing on H264 stacks and we’ll support Gstreamer.Gstreamer is an open source project, royalty free, which goes on top of OpenMax for video API. At this moment we do not have plan to support Video for RTOS/Bare metal________________________________________________________________‐Matt Wood (matt.wood@) ‐ 8:11 AMQ: Can the video decoder be used to operate on streams and recirect the processed data to memory or peripherals and not the TFT LCDC?A: Yes you can decode a stream of data with the Vdec and store the files decompressed files in external memory or even a SD card. Then you can push the data stored in memory via the to the screen display using the LCD controller.________________________________________________________________‐Robert Laventure (venture@) ‐ 8:18 AMQ: For which applications is 720P decryption sufficient? For the current designs that I'm hearing about, they require 1080P. I'm also hearing about 4K.A: We do have application were 720p is sufficient. This is good enough for Home control panel for example. We have opportunities with D4 for advertissement panel in lift as well as video surveillance cameras needing 720p. 4K is for TV screen. Let me know what application designs request 1080p that we may be able to support with our future MPU devices.________________________________________________________________‐Brian Hammill (brian.hammill@) ‐ 8:24 AMQ: Is the video decoder the same or similar to the one on the SAM9M10?A: Yes it’s from the same IP provider, but a newer version, supporting newest codecs and all H264 profiles.________________________________________________________________‐Brian Hammill (brian.hammill@) ‐ 8:25 AMQ: Why 528 MHz for the SAMA5D4 vs slightly faster speed for the SAMA4D3 at 536 MHz? Not a big difference but curious why the limitation?A: Because it’s not the same design architecture, not the same process options, not the same voltage trails, etc…________________________________________________________________‐Mike Miceli (michael.miceli@) ‐ 8:31 AMQ: One of the stated applications is entry level industrial HMI. Why is it considered entry level?A: This question has been answered verbally.‐ Industrial HMI also used dual core or Intel class of processor depending on the application. I refer to entry range as a comparison to these higher-end performance hungry application where we could not fit.________________________________________________________________‐Brian Hammill (brian.hammill@) ‐ 8:33 AMQ: What clock speed is the iMX6 solo lite running for the< 300 mW consumption that is comparable to our part running at 528 MHz?A: The IMX6 solo-lite power consumption number are available on FSL web site (Apps note called‘I.Mx6SL power numbers.pdf’ ). They show ~300mW for a MP3 audio playback application. There is no other number available or coremark/dhrystone bench. This is the value shown in the slide deck.________________________________________________________________‐Brian Hammill (brian.hammill@) ‐ 8:35 AMQ: What is the system bus and DDR clock speed of the SAMA5D4?A: CPU speed is 528MHz - System speed = DDR clock = 176MHz________________________________________________________________‐Martin Squibbs (martin.squibbs@) ‐ 8:35 AMQ: The static power was not compared in the competitive table – do you have this information ?A: There are apps note on FSL web pages for power consumption numbers where I extracted the values.SAMA5D4 (typical 25°c) : Back-up mode = 7µA - Ultra Low power mode = 8.5mW – Idle = 111mWFSL solo : Deep sleep mode = 23mW - Idle mode= 179mW (DDR3)FSL solo-lite: Deep sleep mode = 3.7mW - Idle mode= 15mW (LPDDR2)________________________________________________________________‐Martin Squibbs (martin.squibbs@) ‐ 8:35 AMQ: You mentioned H264 Codec for Youtube, and V8 for Google – what are the endmarkets/applications/products for the V8 Google Codec ?A: The differences between the 2 are the Royalties that must be paid by the end equipment manufacturer. Google (On2) developped this VP8 format to avoid the H264. Eventually H264 royalties were well negotiated and not a high as expected. So VP8 did not took-off as promised. VP8 is commontly supported by WebM mediafile particularly suited for web application such as Chrome, firefox….Android (from 2.3) Adobe flash payer and video conferencing via Skype support this format. But it’s not spread into the marlet. The lead opportunity of D4 only requested H264 so far and we are focusing to release Linux distribution for H264 support. If a VP8 customer comes we will be able to do a porting.________________________________________________________________‐Martin Squibbs (martin.squibbs@) ‐ 8:36 AMQ: Is a tamper detect in any way able to prevent and protect access to 512B of fused data ?A: The 512bit of fuses are in the dedicated customer matrix. The access to this matrix is protected by TrustZone: only a ‘trusted’ application has access to read or write .________________________________________________________________‐Robert Laventure (venture@) ‐ 8:36 AMQ: Has the BU identified target opportunities? i.e. specific customers you would like us to investigate as lead opportunities?A: see target application slide of the webinar. We do have won a large opportunity in secure gateway for smartgrid and the D4 is a good fit versus security requierement in this segment. Also customers are evaluating the D4 for the following applications: video surveillance camera, gambling machines, lift control panel, bar code scanner, digital walkie‐talkie w/ video…________________________________________________________________‐Matt Wood (matt.wood@) ‐ 8:37 AMQ: can you please provide the Android and Linux demo and source code links?A: This question has been answered verbally.‐ Linux and android sources can be requested to the Linux team.________________________________________________________________‐Alex Ferris (alex.ferris@) ‐ 8:38 AMQ: It looked like the competitive cost comparison compared the atmel "cost" (DBC) to our competitors 1k "resale". Is that correct?A: Yes this is correct._______________________________________________________________‐Kevin Vap (kevin.vap@) ‐ 8:45 AMQ: Is the ITTIAM codec & NEON comparisons done with or without the video decoder?A: (redundant questions– answer above)________________________________________________________________‐Alex Ferris (alex.ferris@) ‐ 8:47 AMQ: It looked like the competitive cost comparison compared the atmel "cost" (DBC) to our competitors 1k "resale". Is that correct?A: (redundant question– answer above)‐Alex Ferris (alex.ferris@) ‐ 8:48 AMQ: Who/What PCI Security processor would Atmel recommend to use with the D4 when competing against Freescale?A: Atmel has only one compliant MCU for system level PCI certification: the AT91SO MCU which is under transfer to UMC (after closure of Lfoundry). Volume production January 2015. Freescale competition is Cortex M4 Kinetis (K21 or K61). Maxim competition is MAXQ1850._______________________________________________________________________________________________________________________________‐Tom Hope Harper and Two (thope@) ‐ 8:05 AMQ: Does the difference in power scheme mean that the ActiveSemi will not power this device?A: The Activesemi chip do power the D4. The chip is present on the SAMA5D4 EK.The region are organizing the training. We have included the A5D4 training presentation in the training agenda section. Presentation are under preparation. But I don’t know schedule for NA training. Glen Nilsen is responsible for trainings.。